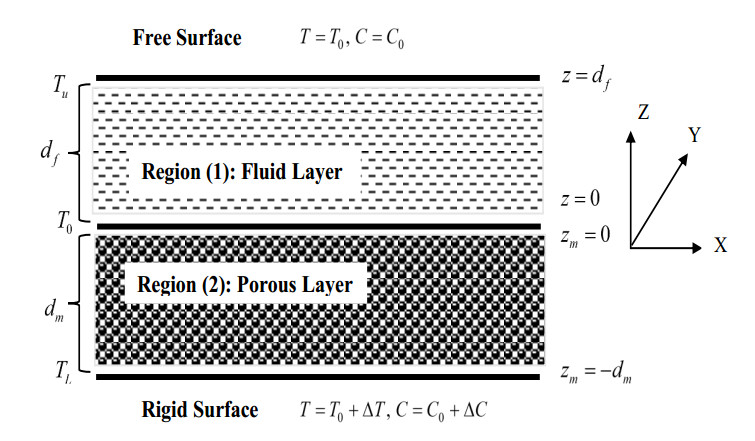
A delay differential equation model of an infectious disease is considered and analyzed. In this model, the impact of information due to the presence of infection is considered explicitly. As information propagation is dependent on the prevalence of the disease, the delay in reporting the prevalence is an important factor. Further, the time lag in waning immunity related to protective measures (such as vaccination, self-protection, responsive behaviour etc.) is also accounted. Qualitative analysis of the equilibrium points of the model is executed and it is observed that when the basic reproduction number is less unity, the local stability of the disease free equilibrium (DFE) depends on the rate of immunity loss as well as on the time delay for the waning of immunity. If the delay in immunity loss is less than a threshold quantity, the DFE is stable, whereas, it loses its stability when the delay parameter crosses the threshold value. When, the basic reproduction number is greater than unity, the unique endemic equilibrium point is found locally stable irrespective of the delay effect under certain parametric conditions. Further, we have analyzed the model system for different scenarios of both delays (i.e., no delay, only one delay, and both delay present). Due to these delays, oscillatory nature of the population is obtained with the help of Hopf bifurcation analysis in each scenario. Moreover, at two different time delays (delay in information's propagation), the emergence of multiple stability switches is investigated for the model system which is termed as Hopf-Hopf (double) bifurcation. Also, the global stability of the endemic equilibrium point is established under some parametric conditions by constructing a suitable Lyapunov function irrespective of time lags. In order to support and explore qualitative results, exhaustive numerical experimentations are carried out which lead to important biological insights and also, these results are compared with existing results.
Citation: Anuj Kumar, Yasuhiro Takeuchi, Prashant K Srivastava. Stability switches, periodic oscillations and global stability in an infectious disease model with multiple time delays[J]. Mathematical Biosciences and Engineering, 2023, 20(6): 11000-11032. doi: 10.3934/mbe.2023487
[1] | Mohammed A. Almalahi, Satish K. Panchal, Fahd Jarad, Mohammed S. Abdo, Kamal Shah, Thabet Abdeljawad . Qualitative analysis of a fuzzy Volterra-Fredholm integrodifferential equation with an Atangana-Baleanu fractional derivative. AIMS Mathematics, 2022, 7(9): 15994-16016. doi: 10.3934/math.2022876 |
[2] | Kishor D. Kucche, Sagar T. Sutar, Kottakkaran Sooppy Nisar . Analysis of nonlinear implicit fractional differential equations with the Atangana-Baleanu derivative via measure of non-compactness. AIMS Mathematics, 2024, 9(10): 27058-27079. doi: 10.3934/math.20241316 |
[3] | Najat Almutairi, Sayed Saber . Chaos control and numerical solution of time-varying fractional Newton-Leipnik system using fractional Atangana-Baleanu derivatives. AIMS Mathematics, 2023, 8(11): 25863-25887. doi: 10.3934/math.20231319 |
[4] | Saeed M. Ali, Mohammed S. Abdo, Bhausaheb Sontakke, Kamal Shah, Thabet Abdeljawad . New results on a coupled system for second-order pantograph equations with ABC fractional derivatives. AIMS Mathematics, 2022, 7(10): 19520-19538. doi: 10.3934/math.20221071 |
[5] | Muhammad Altaf Khan, Muhammad Ismail, Saif Ullah, Muhammad Farhan . Fractional order SIR model with generalized incidence rate. AIMS Mathematics, 2020, 5(3): 1856-1880. doi: 10.3934/math.2020124 |
[6] | Mohamed I. Abbas, Maria Alessandra Ragusa . Nonlinear fractional differential inclusions with non-singular Mittag-Leffler kernel. AIMS Mathematics, 2022, 7(11): 20328-20340. doi: 10.3934/math.20221113 |
[7] | Hasib Khan, Jehad Alzabut, J.F. Gómez-Aguilar, Praveen Agarwal . Piecewise mABC fractional derivative with an application. AIMS Mathematics, 2023, 8(10): 24345-24366. doi: 10.3934/math.20231241 |
[8] | Eman A. A. Ziada, Salwa El-Morsy, Osama Moaaz, Sameh S. Askar, Ahmad M. Alshamrani, Monica Botros . Solution of the SIR epidemic model of arbitrary orders containing Caputo-Fabrizio, Atangana-Baleanu and Caputo derivatives. AIMS Mathematics, 2024, 9(7): 18324-18355. doi: 10.3934/math.2024894 |
[9] | Abdelatif Boutiara, Mohammed M. Matar, Jehad Alzabut, Mohammad Esmael Samei, Hasib Khan . On ABC coupled Langevin fractional differential equations constrained by Perov's fixed point in generalized Banach spaces. AIMS Mathematics, 2023, 8(5): 12109-12132. doi: 10.3934/math.2023610 |
[10] | Rahat Zarin, Abdur Raouf, Amir Khan, Aeshah A. Raezah, Usa Wannasingha Humphries . Computational modeling of financial crime population dynamics under different fractional operators. AIMS Mathematics, 2023, 8(9): 20755-20789. doi: 10.3934/math.20231058 |
A delay differential equation model of an infectious disease is considered and analyzed. In this model, the impact of information due to the presence of infection is considered explicitly. As information propagation is dependent on the prevalence of the disease, the delay in reporting the prevalence is an important factor. Further, the time lag in waning immunity related to protective measures (such as vaccination, self-protection, responsive behaviour etc.) is also accounted. Qualitative analysis of the equilibrium points of the model is executed and it is observed that when the basic reproduction number is less unity, the local stability of the disease free equilibrium (DFE) depends on the rate of immunity loss as well as on the time delay for the waning of immunity. If the delay in immunity loss is less than a threshold quantity, the DFE is stable, whereas, it loses its stability when the delay parameter crosses the threshold value. When, the basic reproduction number is greater than unity, the unique endemic equilibrium point is found locally stable irrespective of the delay effect under certain parametric conditions. Further, we have analyzed the model system for different scenarios of both delays (i.e., no delay, only one delay, and both delay present). Due to these delays, oscillatory nature of the population is obtained with the help of Hopf bifurcation analysis in each scenario. Moreover, at two different time delays (delay in information's propagation), the emergence of multiple stability switches is investigated for the model system which is termed as Hopf-Hopf (double) bifurcation. Also, the global stability of the endemic equilibrium point is established under some parametric conditions by constructing a suitable Lyapunov function irrespective of time lags. In order to support and explore qualitative results, exhaustive numerical experimentations are carried out which lead to important biological insights and also, these results are compared with existing results.
The study of non-Newtonian fluids such as the Walters fluid, Rivlin-Ericksen fluid, and pair stress fluid is critically necessary due to the expanding importance of these fluids in various domains such as industries and modern technologies. In convective problems, it is preferable to study fluid flow with free boundaries in the presence of a solute gradient due to the wide range of applications in the ionosphere, astrophysics, and atmospheric physics. Several liquid systems contain more than two components. As a result, the stability of multi-object systems must be considered. Few authors have looked into triple diffusive convection for fluid/porous media [1,2,3,4]. Heat generated by chemical reactions in the fluid brought on by radiation from an external medium might result in the development of an internal heat source (sink), which can help speed up or slow down convection. A perturbation approach was used by Raghunatha et al. [5] to study the three-component convection in a porous layer. Patil et al. [6,7] investigate triple diffusive boundary layer flow along an external flow velocity that is exponentially and vertically decreasing. The effects of the magnetic field and heat source on three-component convection in an Oldroyd-B liquid were investigated using the Galerkin method by Gayathri et al. [8]. Archana et al. [9] studied the triple diffusive flow in the presence of nanofluid. Heat transfer has been one of the top contenders to get involved in many applications, which has drawn the attention of many researchers to the study of porous media [10]. Eyring-Powell nanomaterials were examined from the triple diffusion perspective by Khan et al. [11]. The effects triple-diffusive mixed convection was studied numerically by Sushma et al. [12] for Casson fluid. Li et al. [13] studied the double diffusion for nanofluid with mixed convection in the presence of a heat source. They discovered that the viscoelastic parameter and Hartmann number cause the wall shear stress to rise. The triple-mass diffusion for nanoparticle mixes in Carreau-Yasuda material was explored by Sohail et al. [14]. In presence of chemical reaction and heat source, Sharma and Gandhi [15] examined the MHD on heat and mass transmission in a Darcy-Forchheimer porous medium. Using Oldroyd-B type model with heat source, Arshika et al. [16] explored the impact of sinusoidal and nonsinusoidal waveforms on triple diffusive-convection in viscoelastic liquids. Using lie-group transformation, Nagendramma et al. [17] look into the dynamics of triple diffusive convection. They found that the influence of heat and mass transfer rates decreased for both fluid flow scenarios as the Lewis number increased. In the presence of heat source/sink [18,19,20,21] and nanofluid [22,23,24,25,26] few authors have attempted to study the convective stability. The inverse problems in porous media have been studied extensively see [27,28].
Recently, for the composite layers, Manjunatha and Sumithra [29,30] examined the problem of triple component convection in a combined layer for three different temperature profiles with and without a magnetic field and obtained the corresponding thermal Marangoni numbers. The double-diffusive convection in the existence of a heat source and temperature profiles were studied by Manjunatha and Sumithra [31] and Manjunatha et al. [32]. They found that a linear model is unstable and an inverted parabolic model is more stable.
The current work examines the stability of the onset of triple-diffusive convective stability in a fluid and fluid-saturated porous layer in the presence of a constant heat source and uses an exact technique to investigate the effect of temperature gradients on the corresponding thermal Marangoni (surface-tension-driven) numbers. Furthermore, the investigation of non-uniform fundamental temperature gradients at the start is intriguing since it opens up new perspectives on how convective instability is managed. The discussion takes into account the following non-dimensional basic temperature gradients: linear, parabolic, and inverted parabolic temperature profiles. Over a wide range of controlling physical parameters, the eigenvalue problem is analytically resolved using the exact technique. Numerous applications in astronomy, engineering, geophysics, climatology, and crystal formation (see Rudolph et al. [33]) will surely benefit from this work.
Consider horizontally infinite fluid and fluid-saturated porous layers of depth df and dm respectively. The lower rigid surface zm=−dm and upper free surface boundary z=df are maintained at constant temperature and concentration T=T0 and C=C0. At the upper free region, surface tension (σt) force acts which varies linearly with temperature and concentration respectively in the form σt=σ0−σTΔT and σt=σ0−σCΔC. Where σT=−∂σt∂T, σC=−∂σt∂C and σ0 is the unperturbed value. The fluid-saturated porous medium interface is located at position at z=0=zm, and the temperature and concentration difference between the lower and higher bounds is denoted by ΔT and ΔC. A coordinate system is used as shown in Figure 1. The relevant equations with Oberbeck-Boussinesq approximation for two regions as following Roberts [34], Char & Chiang [35], Del Rio & Whitaker [36], Othman [37] and Shivakumara et al. [38,39].
Region-1, Fluid layer:
∇.→qf=0, | (1) |
ρ0[∂→qf∂t+(→qf.∇)→qf]=−∇Pf+μf∇2→qf, | (2) |
∂Tf∂t+(→qf.∇)Tf=κf∇2Tf+Qf, | (3) |
∂Cf1∂t+(→qf.∇)Cf1=κf1∇2Cf1, | (4) |
∂Cf2∂t+(→qf.∇)Cf2=κf2∇2Cf2. | (5) |
Region-2, Porous layer:
∇m.→qm=0, | (6) |
ρ0[1ϕ∂→qm∂t+1ϕ2(→qm.∇m)→qm]=−∇mPm−μmK→qm, | (7) |
M∂Tm∂t+(→qm.∇m)Tm=κm∇2mTm+Qm, | (8) |
ϕ∂Cm1∂t+(→qm.∇m)Cml=κm1∇2mCm1, | (9) |
ϕ∂Cm2∂t+(→qm.∇m)Cm2=κm2∇2mCm2, | (10) |
where the subscript 'f' refers to the fluid layer (region-1) and the subscript 'm' refers to the porous medium (region-2), →qf=(u,v,w),→qm=(um,vm,wm) are the velocity vectors, ρ0 is the reference fluid density, Pf,Pm are the pressures, Qf,Qm are the constant heat sources, κf,κm are the thermal diffusivities, Cf1,Cf2,Cm1,Cm2 are the salinity field1 and salinity field2, K is the permeability, M is the ratio of heat capacities, ϕ is the porosity, μf,μm are the fluid viscosities, and κf1,κf2,κm1,κm2 are the solute1diffusivities and solute2 diffusivities.
The basic state is quiescent in regions 1 and 2, respectively.
[→qf,Pf,Tf,χf(z),Cfi]=[0,Pfb(z),Tfb(z),−dTbdz=CfBb(z)],fori=1,2, | (11) |
[→qm,Pm,Tm,χm(zm),Cmi]=[0,Pmb(zm),Tmb(zm),−dTmbdzm,Cmib(zm)],fori=1,2, | (12) |
The basic state of Tfb(z),Tmb(zm), Cfib(z),Cmib(zm), for i=1,2, are
−∂Tfb∂z=Qfz(z−df)2κf+(T0−Tu)dfχf(z),0≤z≤df, | (13) |
−∂Tmb∂zm=Qmzm(zm+dm)2κm+(Tl−T0)dmχm(zm),−dm≤zm≤0, | (14) |
Cfib(z)=Ci0−(Ci0−Ciu)zdf,0≤z≤df, | (15) |
Cmib(zm)=Ci0−(Cil−Ci0)zmdm,−dm≤zm≤0, | (16) |
where the basic state is denoted by the subscript 'b', T0=κfdmTu+κmdfTlκdm+κmdf+dfdm(Qmdm+Qfdf)2(κfdm+κmdf) is the interface temperature, Ci0=κfidmCiu+κmidfCilκfidm+κmidf is the interface concentrations for i=1,2. df∫0χf(z)dz=ΔTfdf,dm∫0χm(zm))dzm=ΔTmdm are the temperature gradient in region-1 and region-2.
To examine the stability of the system, we perturb the system as
[→qf,Pf,Tf,Cfi]=[→q′f,Pb(z)+P′f,Tfb(z)+θ′f,Cfib(z)+C′ft],fori=1,2, | (17) |
[→qm,Pm,Tm,Cmi]=[→q′m,PB(z)+P′m,Tmb(zm)+θ′m,CmB(zm)+C′mi],fori=1,2, | (18) |
where the perturbed primed quantities are those that are very small in relation to the primed quantities of the fundamental state. After substituting Eqs (17) and (18) into Eqs (1–10), linearized in usual manner, eliminate the pressure term from the Eqs (2) and (7) by executing curl twice, and retain the vertical element, the governing stability equations were eventually determined.
Region-1:
1Prf∂∂t(∇2→qf)=∇4→qf, | (19) |
∂θf∂t−(χf(z)+R∗a(2z−1))→qf=∇2θf, | (20) |
∂Cf1∂t=→qf+τf1∇2Cf1, | (21) |
∂Cf2∂t=→qf+τf2∇2Cf2, | (22) |
Region-2:
β2Prm∂∂t(∇2→qm)=−∇2→qm, | (23) |
M∂θm∂t−χm(zm)→qm−R∗am(1+2zm)→qm=∇2θm, | (24) |
ϕ∂Cm1∂t=→qm+τm1∇2Cm1, | (25) |
ϕ∂Cm2∂t=→qm+τm2∇2Cm2, | (26) |
here ∇2=∂2∂x2+∂2∂y2+∂2∂z2 is the Laplacian operator.
Establish dimensionless values using standard linear stability analysis methods.
(x,y,z)=df(x∗,y∗,z∗),θ′=ΔTfθ∗,Pf=μfκfd2fP∗,(u,v,w)=κdf(u∗,v∗,w∗),∇=1df∇∗,t=d2fκft∗,χf(z)=ΔTfdfχf(z)∗}, | (27) |
(xm,ym,zm)=dm(x∗m,y∗m,z∗m−1),θ′m=ΔTmθ∗m,Pm=μmκmd2mP∗m,(um,vm,wm)=κmdm(u∗,v∗,w∗),∇=1dm∇∗,tm=ϕd2mκ2mt∗m,χm(zm)=ΔTmdmχ(zm)∗}, | (28) |
The normal mode approach for the fluid and fluid-saturated porous media respectively,
[w′T′fC′f1C′f2]=[Wf(z)Θf(z)Σf1(z)Σf2(z)]exp[i(αxx+αyy)+ϖt], | (29) |
[w′mT′mC′m1C′m2]=[Wm(zm)Θm(zmm)Σm1(zm)Σm2(zm)]exp[i(αxmx+αymy)+ϖmt], | (30) |
where αx,αy and αxm,αym are wavenumbers in x and y direction respectively, and ϖ,ϖm are growth rate (real or complex) in region-1 and region-2 respectively.
Nondimensionalized using (27) and (28), and introducing Eqs (29) and (30) into (19)–(26), the equations are becomes
Region-1: 0≤zf≤1
(D2f−a2f+ϖPr)(D2f−a2f)Wf(z)=0, | (31) |
(D2f−a2f+ϖ)Θf(z)+[χf(z)+R∗a(2zf−1)]Wf(z)=0, | (32) |
(τf1(D2f−a2f)+ϖ)Σf1(z)+Wf(z)=0, | (33) |
(τf2(D2f−a2f)+ϖ)Σf2(z)+Wf(z)=0, | (34) |
Region-1: −1≤zm≤0
(1−β2ϖmPrm)(D2m−a2m)Wm(zm)=0, | (35) |
(D2m−a2m+Mϖm)Θm(zm)=−[χm(zm)+R∗am(2zm+1)]Wm(zm), | (36) |
(τm1(D2m−a2m)+ϖmϕ)Σm1(zm)=−Wm(zm), | (37) |
(τm2(D2m−a2m)+ϖmϕ)Σm2(zm)=−Wm(zm), | (38) |
where Pr=vfκf, Prm=νmκm are the Prandtl numbers and β=√Kd2m is the square root of Darcy number.
For the current problem, the approach of linear stability analysis and the idea of stability exchange are appropriate and effective so take σ=0 and wm=0. (Refer Manjunatha and Sumithra [30] and Shivakumara et al. [38]).The eigenvalue problem (31)–(38) takes the form.
Region-1: 0≤zf≤1
(D2f−a2f)(D2f−a2f)Wf(z)=0, | (39) |
(D2f−a2f)Θf(z)+[χf(z)+R∗a(2zf−1)]Wf(z)=0, | (40) |
(τf1(D2f−a2f))Σf1(z)+Wf(z)=0, | (41) |
(τf2(D2f−a2f))Σf2(z)+Wf(z)=0, | (42) |
Region-1: −1≤zm≤0
(D2m−a2m)Wm(zm)=0, | (43) |
(D2m−a2m)Θm(zm)=−[χm(zm)+R∗am(2zm+1)]Wm(zm), | (44) |
(τm1(D2m−a2m))Σm1(zm)=−Wm(zm), | (45) |
(τm2(D2m−a2m))Σm2(zm)=−Wm(zm), | (46) |
where, Df=ddz,Dm=ddzm are the Differential operator, af=√αx2+αy2,am=√αxm2+αym2 are the overall horizontal wave numbers, R∗a=RIf2(T0−Tu),R∗am=RIm2(Tl−T0) are the corrected internal Rayleigh numbers, RIf=Qfd2fκf,RIm=Qmd2mκm are the internal Rayleigh numbers, Wf(z),Wm(zm) are the vertical component of velocities, τf1=κf1κf,τf2=κf2κf are the diffusivity ratios, τm1=κm1κm,τm2=κm2κm are the diffusivity ratios in porous region, Σf1(z),Σf2(z) are the salinity distributions, Σm1(zm),Σm2(zm) are the salinity distributions on porous region, and Θf(z),Θm(zm) are the amplitude temperatures. For the composite system, the overall horizontal wave numbers must be am=ˆdaf, here ˆd=dmdf is the depth ratio.
The bottom layer is a rigid surface, while the top border is a free surface, with temperature and concentration/salinity affecting surface tension. Prior to applying the normal mode technique, each boundary condition is nondimensionalized (see Sumithra [2]).
D2fWf(z)+Mta2Θf(z)+MS1a2Σf1(z)+MS2a2Σf2(z)=0,atz=1, | (47) |
Wf(z)=0,Wm(zm)=0,DmWm(zm)=0,atz=1,zm=−1. |
ˆTWf(z)=Wm(zm),D2fWf(z)+a2fWf(z)=ˆμˆTˆd2[D2mWm(zm)+a2mWm(zm)],ˆTˆd3β2[D3fWf(z)−3a2fDfWf(z)]=[ˆμβ2(D3m−3a2mDm)−Dm]Wm(zm),},atz=0,zm=0, |
DfΘf(z)=0,DmΘm(zm)=0,},atz=1,zm=−1, |
Θf(z)=ˆTΘm(zm),DfΘf(z)=DmΘm(zm),}, at z=0,zm=0, ,atz=0,zm=0, |
DfΣf1(z)=0,DfΣf2(z)=0,DmΣm1(zm)=0,DmΣm2(zm)=0},atz=1,zm=−1, |
Σf1(z)=ˆS1Σm1(zm),DfΣf1(z)=DmΣm1(zm)Σf2(z)=ˆS2Σm2(zm),DfΣf2(z)=DmΣm2(zm)},atz=0,zm=0, | (48) |
where Mt=σTΔTdfμfκf denotes the thermal Marangoni (surface-tension-driven) number (TMN), MSi=σC(Ciu−Ci0)dfμfκf for i=1,2 denotes the solute Marangoni (surface-tension-driven) numbers (SMN's), β is the porous parameter, ˆSi=Cil−Ci0Ci0−Ciu for i=1,2 is the solute diffusivity ratio, ˆT is the thermal ratio, and ˆμ=μmμf is the viscosity ratio.
The eigenvalue problem with an eigenvalue of Mt is formed by the boundary conditions of (48) and the Eqs (39)−(46). We address this problem using the exact technique procedure, which produces acceptable results when dealing with difficulties of this nature (see Manjunatha and Sumithra [29,30]).
The velocity profiles are obtained from Eqs (39) and (43) as follows:
Wf(z)=B1[coshafz+b1zcoshafz+b2sinhafz+b3zsinhafz], | (49) |
Wm(zm)=B1[b4coshamzm+b5sinhamzm], | (50) |
where b′is(i=1,2,3,4,5) need to be calculated utilizing the suitable velocity conditions of (48) yields to b1=amcotham2a3fβ2ˆd3,b2=−1−(b1+b3)tanhaf,b3=a2mˆμ−a2fˆd2afˆd2,b4=ˆT,b5=ˆTcotham.
Using the solutal boundary conditions (48), the salinity profiles are obtained from Eqs (41), (42), (45) and (46) as follows
Σf1(z)=B1[b18coshafz+b19sinhafz+g2(z)], | (51) |
Σf2(z)=B1[b22coshafz+b23sinhafz+g3(z)], | (52) |
Σm1(zm)=A1[b20coshamzm+b21sinhamzm+g2m(zm)], | (53) |
Σm2(zm)=B1[b24coshamzm+b25sinhamzm+g3m(zm)], | (54) |
Where, g2(z)=−z4a2fτf1[(2afb1−b2+afb3z)coshafz+(2af−b3+afb2z)sinhafz], g2m(zm)=−zmcoshamzm2amτm1[(b5+b4tanhamzm)],b18=ˆS1b20,b19=1af(b21am+N1−N2), b20=N6amcosham−N3N5amsinham(N5+N4cotham), b21=N3N4+N6amsinhamamsinham(N5+N4cotham), g3(z)=−z4a2fτf2[(2afb1−b2+afb3z)coshafz+(2af−b3+afb2z)sinhafz],g3m(zm)=−zmsinhamzm2amτm2[(b5cothamzm+b4)], b22=ˆS2b24,b23=1af(b25am+N8−N9), b24=N13amcosham−N10N12amsinham(N12+N11cotham),b25=N10N11+N13amsinhamamsinham(N12+N11cotham).
We considered linear, parabolic and inverted parabolic profiles, these profiles have been discussed numerically and analytically by Shivakumara et al. [38,39,40] using the Galerkin procedure for porous layers in absence of heat source and in a linear case by Kaloni and Lou [41] by applying a compound matrix method for single layers. We have revisited these instances in order to determine the analytical reliability of the results for the two layers on the onset of triple-diffusive convection in a fluid and saturated porous layer.
Introducing model into (40) and (44), the linear profile takes the form
Θf(z)=B1[b6coshafz+b7sinhafz+g1(z)], | (55) |
Θm(zm)=B1[b8coshamzm+b9sinhamzm+g1m(zm)]. | (56) |
From (47), the thermal Marangoni (surface-tension-driven) number (TMN) for the linear model is
Mt1=−Λ1−a2fMS1Λ2−a2fMS2Λ3a2fΘf(1), | (57) |
where Λ1=a2f(coshaf+b1sinhaf)+b2(a2fcoshaf+2afsinhaf)+b3(a2fsinhaf+2afcoshaf), Λ2=[b18coshaf+b18sinhaf+g2(1)], Λ3=[b25coshaf+b2ssinhaf+g3(1)], Θf(1)=B1[b6coshaf+b3sinhaf+g1(1)], g1(z)=B1[Δ1−Δ2+Δ3−Δ4],g1m(zm)=B1[Δ5−Δ6].
Introducing model (Sparrow et al. [42]) into (40) and (44), the profile takes the form
Θf(z)=B1[b10coshafz+b11sinhafz+g4(z)], | (58) |
Θm(zm)=B1[b12coshamzm+b13sinhamzm+g4m(zm)]. | (59) |
From (47), the TMN for the model is
Mt2=−Λ1−a2fMS1Λ2−a2fMS2Λ3a2fΘf(1), | (60) |
where Θf(1)=B1[b10coshaf+b11 sinh af+g4(1)],
g4(z)=B1[Δ17−Δ18+Δ10−Δ20],g4m(zm)=B1[Δ21−Δ22].
When model is introduced into (40) and (44), the profile becomes
Θf(z)=B1[b14coshafz+b15sinhafz+g5(z)], | (61) |
Θm(zm)=B1[b18coshamzm+b13sinhamzm+g5m(zm)]. | (62) |
From (47), the TMN for the profile is
Mt3=−Λ1−a2fMS1Λ2−a2fMS2Λ3a2fΘf(1), | (63) |
where Θf(1)=B1[b4coshaf+b15sinhaf+g5(1)], g5(z)=B1[Δ31−Δ32+Δ33−Δ34],g5m(zm)=B1[Δ35−Δ36] (See Appendix).
The present study aims at solving exactly the problem of Marangoni convection in presence of a heat source and temperature gradients. The exact method provides useful results and also general basic temperature profiles can be readily treated with minimum mathematical computations. The graphs are plotted using MATHEMATICA version 11. The TMNs Mt1 for model 1 as linear, Mt2 model 2 as parabolic, and Mt3 model 3 as inverted parabolic temperature profiles have been investigated with the object of understanding the control of convection. The depth ratio ˆd is used to draw the limits. The effects of the parameters β,R∗aR∗am,Ms1,Ms2 and τf2 for linear, parabolic and inverted parabolic profiles on all three TMNs are depicted in Figures 2−7. The major finding is that for a given set of fixed parameter values, the inverted parabolic profile is the most stable, while the linear profile is the most unstable, as the corresponding TMNs are highest and lowest, respectively, for porous layer dominant systems, i.e., Mt1≺Mt2≺Mt3.
Figure 2 depicts the fluctuations of the porous parameter β on the three TMNs for linear, parabolic, and inverted temperature profiles for β=0.1,0.5,1,5,10. The TMNs for all three profiles rise when the value of β, i.e., the permeability of the porous layer, increases. As a result, raising the porosity parameter can influence the onset of triple-diffusive convection, which is physically sensible because the fluid has more ways to travel. As a result, the system has reached a state of equilibrium. The effect of corrected internal Rayleigh numbers R∗a and R∗am on the onset of triple-diffusive convection is explained in Figures 3 and 4 respectively, for all three profiles. Negative values of these parameters indicate heat sinks, while the positive values indicate heat sources. It is evident from the figures that these parameters are effective for larger values of depth ratio, i.e., for porous layer dominant composite layer systems and the effect of R∗am is drastic when compared to that of R∗a. For a given depth ratio, the thermal Marangoni number rises as the value of these parameters rises, indicating a delay in the commencement of the onset of triple-diffusive convection, which is particularly pronounced for R∗am. The effects of MS1 and MS2, the solute1, solute2 Marangoni numbers for MS1=MS2=1,5,10,25,50 on the onset of triple-diffusive convection is displayed in Figures 5 and 6. The TMNs drop for each of the three temperature profiles as the solute1 Marangoni number values are raised. So, the onset of triple-diffusive convection can be postponed; hence, the system can be destabilized. The system easily stabilizes if more high-solute diffusivity salts are added. However, it is clear that the solute2 Marangoni number turns the process around. Therefore, by increasing the solute2 Marangoni number, the onset of triple-diffusive convection can be postponed, stabilizing the system and helping to determine its stability features. This demonstrates that there is a third diffusing component, at which point the onset of triple-diffusive convection influences the stability of the system.
Figure 7 displays the effects of τf2, the ratio of solute2 diffusivity to thermal diffusivity fluid in the fluid layer, which is the significance of the presence of a third diffusing component, for Mt1,Mt2 and Mt3 respectively, for the values τf2=0.1,0.3,0.5,0.7,0.9. Smaller depth ratio values, or fluid layer dominating composite layer systems, have no effect on the TMNs for any of the three profiles. In a composite layer system with a porous layer as the dominating layer, the system can become unstable as τf2 is increased because onset of triple-diffusive convection begins to develop more quickly. This is perfectly understandable because, in contrast to the energy stability theory, the linear stability theory specifies sufficient requirements for stability.
The conclusions presented above provide a general framework for investigating the role of the porous parameter, corrected internal Rayleigh numbers, solutal Marangoni (surface-tension-driven) numbers, and fluid thermal diffusivity ratio on the onset of triple-diffusive convection in a fluid and fluid-saturated layer of the porous medium. For a variety of fundamental uniform and non-uniform temperature gradients in the presence of a constant heat source, the principle of exchange of stability is found to be valid, and the problem of eigenvalue is solved using the exact technique.
The investigation's findings are as follows:
The parameters in the study have a larger influence on the porous layer dominant composite layer systems than that on the fluid layer dominant composite systems.
The larger values of the porous parameter, corrected internal Rayleigh numbers and solute Marangoni (surface-tension-driven) number Ms2 and the lower values of τf2 and Ms1 are preferable for controlling the onset of triple-diffusive convection.
The system is stabilized by the porous parameter, corrected internal Rayleigh numbers, and the solute2 Marangoni (surface-tension-driven) number, while the system is destabilized by the solute1 Marangoni (surface-tension-driven) number and the ratio of the solute2 diffusivity to the thermal diffusivity of the fluid.
The inverted parabolic temperature (model 3) profile is the most stable and hence suitable for controlling the onset of triple-diffusive convection whereas the linear temperature (model 1) profile is the most unstable for augmenting the onset of triple-diffusive convection for the set of parameters chosen for this investigation.
The system is more stable for model 3 and less stable for model 1. i.e., Mt1≺Mt2≺Mt3.
This work can be extended to temperature-dependent heat sources and Soret and Dupour effects to analyses the onset of triple-diffusive convection.
The authors are thankful for the support of Princess Nourah bint Abdulrahman University Researchers Supporting Project number (PNURSP2023R163), Princess Nourah bint Abdulrahman University, Riyadh, Saudi Arabia.
Authors are declaring no conflict of interest.
Δ1=zcoshafz(2Φ1+Φ2z)(b1+tanhafz)4af,Δ2=Φ2zcoshafz4a2f(1+b1tanhafz), |
Δ3=z(3a2fzΦ1+2a2fz2Φ2+3Φ2)coshafz12a3f(b3+b2tanhafz),Δ4=zcoshafz(Φ1+Φ2z)4a2f(b2+b3tanhafz),Δ5=zm(2Φ1m+Φ2mzm)sinhamzm4am(b5cothamzm+b4), |
Δ6=Φ2mzmsinhamzm4a2m(b4cothamzm+b5), |
Φ1=R∗a−1,Φ2=−2R∗a,Φ1m=−(R∗am+1),Φ2m=−2R∗am,b6=b8ˆT,b7=1a(b9am+δ3−δ2),b8=δ8δ9,b9=δ6δ7,δ1=−B1[Δ7+Δ8+Δ9+Δ10],Δ7=(2a2fΦ1+Φ2(a2f−1))coshaf4a2f(1+b1tanhaf),Δ8=(Φ2+2Φ1)coshaf4af(b1+tanhaf),Δ9=coshaf[(3a2f−3)Φ1+(2a2f−3)Φ2]12a2f(b2+b3tanhaf),Δ10=[a2fΦ1+Φ2(a2f+1)]coshaf4a3f(b3+b2tanhaf),δ2=B1[(2a2fb1−afb2)Φ1+(b3−af)Φ24a3f],δ3=B1[2Φ1mb54am−b4Φ2m4a2m],δ4=−B1[Δ11+Δ12],Δ11=[(Φ2m−2Φ1m)a2m−Φ2m4a2m](b4cosham−b5sinham),Δ12=(2Φ1m−Φ2m4am)(b5cosham−b4sinham),δ5=δ1−(δ3−δ2)coshaf,δ6=δ4afˆTsinhaf+δ5amsinham,δ7=amcoshamafˆTsinhaf+a2mcoshafsinham,δ8=δ4coshaf−δ5cosham,δ9=−afcoshamˆTsinhaf−amcoshafsinham, |
N0=1τf1[12(coshaf+b1sinhaf)+12af(b1coshaf+sinhaf)+N100], |
N100=(a2f−1)4a2f(b2coshaf+b3sinhaf)+14af(b3coshaf+b2sinhaf), |
N1=−b52amτm1,N2=1τf14a2f(b2−2afb1), |
N3=−12τm1(b4cosham−b5sinham)+12amτm1(b5cosham−b4sinham), |
N4=ˆS1afsinhaf, |
N5=amcoshaf,N6=N0−(N1−N2)coshaf, |
N7=1τf2[12(coshaf+b1sinhaf)+12af(b1coshaf+sinhaf)+N70], |
N70=(a2f−1)4a2f(b2coshaf+b3sinhaf)+14af(b3coshaf+b2sinhaf), |
N8=−b52amτm2,N9=1τf24a2f(b2−2afb1), |
N10=−12τm2(b4cosham−b5sinham)+12amτm2(b5cosham−b4sinham), |
N11=ˆS2afsinhaf,N12=amcoshaf,N13=N7−(N8−N9)coshaf, |
Δ17=z(2Φ3+Φ4z)coshafz4af(b1+tanhafz),Δ18=Φ4zcoshafz4a2f(1+b1tanhafz)Δ19=z(3a2fzΦ3+2a2fz2Φ4+3Φ4)coshafz12a3f(b3+b2tanhafz),Δ20=z(Φ3+Φ4z)coshafz4a2f(b2+b3tanhafz)Δ21=zm(2Φ3m+Φ4mzm)sinhamzm4am(b5cothamzm+b4), |
Δ22=E4mzmsinhamzm4a2m(b4cothamzm+b5),Φ3=R∗a,Φ4=−2(R∗a+1),Φ3m=−R∗am,Φ4m=−2(R∗am+1),b10=b12ˆT,b11=1af(b13am+δ12−δ11),b12=δ17δ18,b13=δ15δ16,δ10=−B1[Δ23+Δ24+Δ25+Δ26],Δ23=[2a2fΦ3+Φ4(a2f−1)]coshaf4a2f(1+b1tanhaf),Δ24=(Φ4+2Φ3)coshaf4af(b1+tanhaf),Δ25=[(3a2f−3)Φ3+(2a2f−3)Φ4]coshaf12a2f(b2+b3tanhaf),Δ26=[a2fΦ3+Φ4(a2f+1)]coshaf4a3f(b3+b2tanhaf),δ11=B1[(2a2fb1−afb2)Φ3+(b3−af)Φ44a3f],δ12=B1[2Φ3mb54am−b4Φ4m4a2m],δ13=−B1[Δ27+Δ28],Δ27=[Φ4m−2Φ3m4−Φ4m4a2m](b4cosham−b5sinham),Δ28=(2Φ3m−Φ4m)sinham4am(b5cotham−b4),δ14=δ10−(δ12−δ11)coshaf,δ15=δ13afˆTsinhaf+δ14amsinham,δ16=δ7,δ17=δ13coshaf−δ14cosham,δ18=δ9, |
Δ31=z(2Φ5+Φ6z)coshafz4af(b1+tanhafz),Δ32=Φ6zcoshafz4a2f(1+b1tanhafz),Δ33=z(3a2fzΦ5+2a2fz2Φ6+3Φ6)coshafz12a3f(b3+b2tanhafz),Δ34=z(Φ5+Φ6z)coshafz4a2f(b2+b3tanhafz),Δ35=zm(2Φ5m+Φ6mzm)sinhamzm4am(b5cothamzm+b4),Δ36=Φ6mzmsinhamzm4a2m(b4cothamzm+b5),Φ5=R∗a−2,Φ6=2(1−R∗a),Φ5m=−2−R∗am,Φ6m=2(1−R∗am),b14=b16ˆT,b15=1af(b17am+δ22−δ21),b16=δ26δ27,b17=δ24δ25,δ19=−B1[Δ37+Δ38+Δ39+Δ40],Δ37=[2a2fΦ5+Φ6(a2f−1)]coshaf4a2f(1+b1tanhaf),Δ38=(Φ6+2Φ5)coshaf4af(b1+tanhaf),Δ39=[3(a2f−1)Φ5+(2a2f−3)Φ6]coshaf12a2f(b2+b3tanhaf),Δ40=[a2fΦ5+Φ6(a2f+1)]coshaf4a3f(b3+b2tanhaf),δ20=−B1[Δ41+Δ42],Δ41=[(Φ6m−2Φ5m)a2m−Φ6m]sinham4a2m(b4cotham−b5),Δ42=[2Φ5m−Φ6m]sinham4am(b5cotham−b4),δ21=B1[(2a2fb1−afb2)Φ5+(b3−af)Φ64a3f],δ22=B1[2Φ5mb5am−b4Φ6m4a2m],δ23=δ19−(δ22−δ21)coshaf,δ24=δ20afˆTsinhaf+δ23amsinham,δ25=δ7,δ26=δ20coshaf−δ23cosham,δ27=δ9. |
[1] | F. Brauer, C. Castillo-Chavez, Mathematical Models in Population Biology and Epidemiology, Springer, 2001. |
[2] | O. Diekmann, J. A. P. Heesterbeek, Mathematical Epidemiology of Infectious Diseases: Model Building, Analysis and Interpretation, John Wiley & Sons, 2000. |
[3] |
H. W. Hethcote, The mathematics of infectious diseases, SIAM Rev., 42 (2000), 599–653. https://doi.org/10.1137/S0036144500371907 doi: 10.1137/S0036144500371907
![]() |
[4] |
W. O. Kermack, A. G. McKendrick, A contribution to the mathematical theory of epidemics, Proc. R. Soc. Lond. A, 115 (1927), 700–721. https://doi.org/10.1098/rspa.1927.0118 doi: 10.1098/rspa.1927.0118
![]() |
[5] |
M. E. Alexander, C. Bowman, S. M. Moghadas, R. Summers, A. B. Gumel, B. M. Sahai, A vaccination model for transmission dynamics of influenza, SIAM J. Appl. Dyn. Syst., 3 (2004), 503–524. https://doi.org/10.1137/03060037 doi: 10.1137/03060037
![]() |
[6] |
A. B. Gumel, S. Ruan, T. Day, J. Watmough, F. Brauer, P. van den Driessche, et al., Modelling strategies for controlling SARS outbreaks, Proc. R. Soc. B: Biol. Sci., 271 (2004), 2223–2232. https://doi.org/10.1098/rspb.2004.2800 doi: 10.1098/rspb.2004.2800
![]() |
[7] |
S. Lee, G. Chowell, C. Castillo-Chávez, Optimal control for pandemic influenza: the role of limited antiviral treatment and isolation, J. Theor. Biol., 265 (2010), 136–150. https://doi.org/10.1016/j.jtbi.2010.04.003 doi: 10.1016/j.jtbi.2010.04.003
![]() |
[8] |
X. Liu, Y. Takeuchi, S. Iwami, SVIR epidemic models with vaccination strategies, J. Theor. Biol., 253 (2008), 1–11. https://doi.org/10.1016/j.jtbi.2007.10.014 doi: 10.1016/j.jtbi.2007.10.014
![]() |
[9] |
Z. Qiu, Z. Feng, Transmission dynamics of an influenza model with vaccination and antiviral treatment, Bull. Math. Biol., 72 (2010), 1–33. https://doi.org/10.1007/s11538-009-9435-5 doi: 10.1007/s11538-009-9435-5
![]() |
[10] |
A. Kumar, P. K. Srivastava, Vaccination and treatment as control interventions in an infectious disease model with their cost optimization, Commun. Nonlinear Sci. Numer. Simul., 44 (2017), 334–343. https://doi.org/10.1016/j.cnsns.2016.08.005 doi: 10.1016/j.cnsns.2016.08.005
![]() |
[11] |
A. Kumar, P. K. Srivastava, Y. Takeuchi, Modeling the role of information and limited optimal treatment on disease prevalence, J. Theor. Biol., 414 (2017), 103–119. https://doi.org/10.1016/j.jtbi.2016.11.016 doi: 10.1016/j.jtbi.2016.11.016
![]() |
[12] |
Y. Yuan, N. Li, Optimal control and cost-effectiveness analysis for a COVID-19 model with individual protection awareness, Phys. A: Stat. Mech. Appl., 603 (2022), 127804. https://doi.org/10.1016/j.physa.2022.127804 doi: 10.1016/j.physa.2022.127804
![]() |
[13] |
P. A. Gonzˊalez-Parra, S. Lee, L. Velazquez, C. Castillo-Chavez, A note on the use of optimal control on a discrete time model of influenza dynamics, Math. Biosci. Eng., 8 (2011), 183–197. doi: 10.3934/mbe.2011.8.183 doi: 10.3934/mbe.2011.8.183
![]() |
[14] |
S. M. Kassa, A. Ouhinou, The impact of self-protective measures in the optimal interventions for controlling infectious diseases of human population, J. Math. Biol., 70 (2015), 213–236. https://doi.org/10.1007/s00285-014-0761-3 doi: 10.1007/s00285-014-0761-3
![]() |
[15] |
A. Kumar, P. K. Srivastava, Y. Dong, Y. Takeuchi, Optimal control of infectious disease: Information-induced vaccination and limited treatment, Physica A: Stat. Mech. Appl., 542 (2020), 123196. https://doi.org/10.1016/j.physa.2019.123196 doi: 10.1016/j.physa.2019.123196
![]() |
[16] |
A. Yadav, P. K. Srivastava, A. Kumar, Mathematical model for smoking: Effect of determination and education, Int. J. Biomath., 8 (2015), 1550001. https://doi.org/10.1142/S1793524515500011 doi: 10.1142/S1793524515500011
![]() |
[17] | World Health Organization (WHO), Government of Senegal boosts Ebola awareness through SMS campaign, 2014. Avaliable from: http://http://www.who.int/features/2014/senegal-ebola-sms/en/. |
[18] |
A. Ahituv, V. J. Hotz, T. Philipson, The responsiveness of the demand for condoms to the local prevalence of AIDS, J. Hum. Resour., 31 (1996), 869–897. https://doi.org/10.2307/146150 doi: 10.2307/146150
![]() |
[19] |
D. Greenhalgh, S. Rana, S. Samanta, T. Sardar, S. Bhattacharya, J. Chattopadhyay, Awareness programs control infectious disease-multiple delay induced mathematical model, Appl. Math. Comput., 251 (2015), 539–563. https://doi.org/10.1016/j.amc.2014.11.091 doi: 10.1016/j.amc.2014.11.091
![]() |
[20] |
Y. Liu, J. Cui, The impact of media coverage on the dynamics of infectious disease, Int. J. Biomath., 1 (2008), 65–74. https://doi.org/10.1142/S1793524508000023 doi: 10.1142/S1793524508000023
![]() |
[21] |
A. K. Misra, A. Sharma, V. Singh, Effect of awareness programs in controlling the prevalence of an epidemic with time delay. J. Biol. Syst., 19 (2011), 389–402. https://doi.org/10.1142/S0218339011004020 doi: 10.1142/S0218339011004020
![]() |
[22] |
T. Philipson, Private vaccination and public health: an empirical examination for US measles, J. Hum. Resour., 31 (1996), 611–630. https://doi.org/10.2307/146268 doi: 10.2307/146268
![]() |
[23] |
J. Cui, Y. Sun, H. Zhu, The impact of media on the control of infectious diseases, J. Dyn. Differ. Equations, 20 (2008), 31–53. https://doi.org/10.1007/s10884-007-9075-0 doi: 10.1007/s10884-007-9075-0
![]() |
[24] |
A. d'Onofrio, P. Manfredi, Information-related changes in contact patterns may trigger oscillations in the endemic prevalence of infectious diseases, J. Theor. Biol., 256 (2009), 473–478. https://doi.org/10.1016/j.jtbi.2008.10.005 doi: 10.1016/j.jtbi.2008.10.005
![]() |
[25] |
S. Funk, E. Gilad, C. Watkins, V. A. A. Jansen, The spread of awareness and its impact on epidemic outbreaks, Proc. Natl. Acad. Sci. U.S.A., 106 (2009), 6872–6877. https://doi.org/10.1073/pnas.08107621 doi: 10.1073/pnas.08107621
![]() |
[26] |
I. Z. Kiss, J. Cassell, M. Recker, P. L. Simon, The impact of information transmission on epidemic outbreaks, Math. Biosci., 225 (2010), 1–10. https://doi.org/10.1016/j.mbs.2009.11.009 doi: 10.1016/j.mbs.2009.11.009
![]() |
[27] |
Y. Li, C. Ma, J. Cui, The effect of constant and mixed impulsive vaccination on SIS epidemic models incorporating media coverage, Rocky Mt. J. Math., 38 (2008), 1437–1455. DOI: 10.1216/RMJ-2008-38-5-1437 doi: 10.1216/RMJ-2008-38-5-1437
![]() |
[28] |
R. Liu, J. Wu, H. Zhu, Media/psychological impact on multiple outbreaks of emerging infectious diseases, Comput. Math. Methods Med., 8 (2007), 153–164. https://doi.org/10.1080/17486700701425870 doi: 10.1080/17486700701425870
![]() |
[29] | P. Manfredi, A. d'Onofrio, Modeling the Interplay Between Human Behavior and the Spread of Infectious Diseases, Springer Science & Business Media, 2013. |
[30] |
K. Cooke, P. Van. den Driessche, X. Zou, Interaction of maturation delay and nonlinear birth in population and epidemic models, J. Math. Biol., 39 (1999), 332–352. https://doi.org/10.1007/s002850050194 doi: 10.1007/s002850050194
![]() |
[31] |
D. Greenhalgh, Q. J. A. Khan, F. I. Lewis, Recurrent epidemic cycles in an infectious disease model with a time delay in loss of vaccine immunity, Nonlinear Anal. Theory Methods Appl., 63 (2005), e779–e788. https://doi.org/10.1016/j.na.2004.12.018 doi: 10.1016/j.na.2004.12.018
![]() |
[32] |
G. Huang, Y. Takeuchi, W. Ma, D. Wei, Global stability for delay SIR and SEIR epidemic models with nonlinear incidence rate, Bull. Math. Biol., 72 (2010), 1192–1207. https://doi.org/10.1007/s11538-009-9487-6 doi: 10.1007/s11538-009-9487-6
![]() |
[33] | Y. Kuang, Delay Differential Equations: with Applications in Population Dynamics, Academic Press, 1993. |
[34] |
N. Kyrychko, K. B. Blyuss, Global properties of a delayed SIR model with temporary immunity and nonlinear incidence rate, Nonlinear Anal. Real World Appl., 6 (2005), 495–507. https://doi.org/10.1016/j.nonrwa.2004.10.001 doi: 10.1016/j.nonrwa.2004.10.001
![]() |
[35] |
M. Liu, E. Liz, G. Röst, Endemic bubbles generated by delayed behavioral response: global stability and bifurcation switches in an SIS model, SIAM J. Appl. Math., 75 (2015), 75–91. https://doi.org/10.1137/140972652 doi: 10.1137/140972652
![]() |
[36] |
Y. Song, J. Wei, Bifurcation analysis for chen's system with delayed feedback and its application to control of chaos, Chaos, Solitons Fractals, 22 (2004), 75–91. https://doi.org/10.1016/j.chaos.2003.12.075 doi: 10.1016/j.chaos.2003.12.075
![]() |
[37] |
L. Wen, X. Yang, Global stability of a delayed SIRS model with temporary immunity, Chaos, Solitons Fractals, 38 (2008), 221–226. https://doi.org/10.1016/j.chaos.2006.11.010 doi: 10.1016/j.chaos.2006.11.010
![]() |
[38] |
T. Cheng, X. Zou, A new perspective on infection forces with demonstration by a DDE infectious disease model, Math. Biosci. Eng., 19 (2022), 4856–4880. doi: 10.3934/mbe.2022227 doi: 10.3934/mbe.2022227
![]() |
[39] |
A. d'Onofrio, P. Manfredi, E. Salinelli, Vaccinating behaviour, information, and the dynamics of SIR vaccine preventable diseases, Theor. Popul. Biol., 71 (2007), 301–317. https://doi.org/10.1016/j.tpb.2007.01.001 doi: 10.1016/j.tpb.2007.01.001
![]() |
[40] |
P. K. Srivastava, M. Banerjee, P. Chandra, A primary infection model for HIV and immune response with two discrete time delays, Differ. Equations Dyn. Syst., 18 (2010), 385–399. https://doi.org/10.1007/s12591-010-0074-y doi: 10.1007/s12591-010-0074-y
![]() |
[41] |
P. K. Srivastava, P. Chandra, Hopf bifurcation and periodic solutions in a dynamical model for HIV and immune response, Differ. Equations Dyn. Syst., 16 (2008), 77–100. https://doi.org/10.1007/s12591-008-0006-2 doi: 10.1007/s12591-008-0006-2
![]() |
[42] |
H. Zhao, Y. Lin, Y. Dai, An SIRS epidemic model incorporating media coverage with time delay, Comput. Math. Methods Med., 2014 (2014). https://doi.org/10.1155/2014/680743 doi: 10.1155/2014/680743
![]() |
[43] |
Z. Lv, X. Liu, Y. Ding, Dynamic behavior analysis of an SVIR epidemic model with two time delays associated with the COVID-19 booster vaccination time, Math. Biosci. Eng., 20 (2023), 6030–6061. https://doi.org/10.3934/mbe.2023261 doi: 10.3934/mbe.2023261
![]() |
[44] |
Y. Ma, Y. Cui, M. Wang, Global stability and control strategies of a SIQRS epidemic model with time delay, Math. Methods Appl. Sci., 45 (2022), 8269–8293. https://doi.org/10.1002/mma.8309 doi: 10.1002/mma.8309
![]() |
[45] |
A. Mezouaghi, S. Djillali, A. Zeb, K.S. Nisar, Global proprieties of a delayed epidemic model with partial susceptible protection, Math. Biosci. Eng., 19 (2022), 209–224. https://doi.org/10.3934/mbe.2022011 doi: 10.3934/mbe.2022011
![]() |
[46] |
H. Yang, Y. Wang, S. Kundu, Z. Song, Z. Zhang, Dynamics of an SIR epidemic model incorporating time delay and convex incidence rate, Results Phys., 32 (2022), 105025. https://doi.org/10.1016/j.rinp.2021.105025 doi: 10.1016/j.rinp.2021.105025
![]() |
[47] |
A. Kumar, P. K. Srivastava, A. Yadav, Delayed information induces oscillations in a dynamical model for infectious disease, Int. J. Biomath., 12 (2019), 1950020. https://doi.org/10.1142/S1793524519500207 doi: 10.1142/S1793524519500207
![]() |
[48] |
M. V. Barbarossa, M. Polner, G. Röst, Stability switches induced by immune system boosting in an sirs model with discrete and distributed delays, SIAM J. Appl. Math., 77 (2017), 905–923. https://doi.org/10.1137/16M1077234 doi: 10.1137/16M1077234
![]() |
[49] |
M. V. Barbarossa, G. Röst, Immuno-epidemiology of a population structured by immune status: a mathematical study of waning immunity and immune system boosting, J. Math. Biol., 71 (2015), 1737–1770. https://doi.org/10.1007/s00285-015-0880-5 doi: 10.1007/s00285-015-0880-5
![]() |
[50] | D. Wodarz, Killer Cell Dynamics: Mathematical and Computational Approaches to Immunology, Springer, 2007. |
[51] |
Q. An, E. Beretta, Y. Kuang, C. Wang, H. Wang, Geometric stability switch criteria in delay differential equations with two delays and delay dependent parameters, J. Differ. Equations, 266 (2019), 7073–7100. https://doi.org/10.1016/j.jde.2018.11.025 doi: 10.1016/j.jde.2018.11.025
![]() |
[52] | X. Lin, H. Wang, Stability analysis of delay differential equations with two discrete delays, Can. Appl. Math. Q., 20 (2012), 519–533. |
[53] |
D. Li, B. Chai, W. Liu, P. Wen, R. Zhang, Qualitative analysis of a class of SISM epidemic model influenced by media publicity, Math. Biosci. Eng., 17 (2020), 5727–5751. https://doi.org/10.3934/mbe.2020308 doi: 10.3934/mbe.2020308
![]() |
[54] |
A. Yadav, P. K. Srivastava, The impact of information and saturated treatment with time delay in an infectious disease model, J. Appl. Math. Comput., 66 (2021), 277–305. https://doi.org/10.1007/s12190-020-01436-2 doi: 10.1007/s12190-020-01436-2
![]() |
[55] |
Z. Zhang, G. ur Rahman, J. F. Gómez-Aguilar, J. Torres-Jiménez, Dynamical aspects of a delayed epidemic model with subdivision of susceptible population and control strategies, Chaos, Solitons Fractals, 160 (2022), 112194. https://doi.org/10.1016/j.chaos.2022.112194 doi: 10.1016/j.chaos.2022.112194
![]() |
[56] |
H. Zhao, M. Zhao, Global hopf bifurcation analysis of an susceptible-infective-removed epidemic model incorporating media coverage with time delay, J. Biol. Dyn., 11 (2017), 8–24. https://doi.org/10.1080/17513758.2016.1229050 doi: 10.1080/17513758.2016.1229050
![]() |
[57] |
Y. Liu, J. Wei, Bifurcation analysis in delayed nicholson blowflies equation with delayed harvest, Nonlinear Dyn., 105 (2021), 1805–1819. https://doi.org/10.1007/s11071-021-06651-5 doi: 10.1007/s11071-021-06651-5
![]() |
[58] | S. Ruan, J. Wei, On the zeros of transcendental functions with applications to stability of delay differential equations with two delays, Dyn. Contin. Discrete Impuls. Syst. A: Math. Anal., 10 (2003), 863–874. |
[59] |
X. Wang, A simple proof of descartes's rule of signs, Am. Math. Mon., 111 (2004), 525. https://doi.org/10.1080/00029890.2004.11920108 doi: 10.1080/00029890.2004.11920108
![]() |
[60] |
H. I. Freedman, V. S. H. Rao, The trade-off between mutual interference and time lags in predator-prey systems, Bull. Math. Biol., 45 (1983), 991–1004. https://doi.org/10.1016/S0092-8240(83)80073-1 doi: 10.1016/S0092-8240(83)80073-1
![]() |
[61] | J. K. Hale, Functional Differential Equations, Springer, New York, 1977. |
[62] | J. P. La Salle, The Stability of Dynamical Systems, Society for Industrial and Applied Mathematics, SIAM, Philadelphia, 1976. |
[63] |
H. Jiang, T. Zhang, Y. Song, Delay-induced double hopf bifurcations in a system of two delay-coupled van der pol-duffing oscillators, Int. J. Bifurcation Chaos, 25 (2015), 1550058. https://doi.org/10.1142/S0218127415500583 doi: 10.1142/S0218127415500583
![]() |
[64] |
H. Zang, T. Zhang, Y. Zhang, Stability and bifurcation analysis of delay coupled van der pol-duffing oscillators, Nonlinear Dyn., 75 (2014), 35–47. https://doi.org/10.1007/s11071-013-1047-9 doi: 10.1007/s11071-013-1047-9
![]() |
[65] |
M. Adimy, F. Crauste, S. Ruan, Periodic oscillations in leukopoiesis models with two delays, J. Theor. Biol., 242 (2006), 288–299. https://doi.org/10.1016/j.jtbi.2006.02.020 doi: 10.1016/j.jtbi.2006.02.020
![]() |