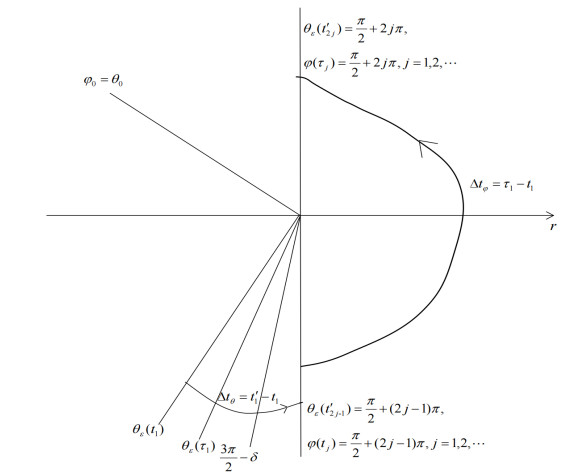
In this study, the stability and stabilization analyses are discussed for Takagi-Sugeno (T-S) fuzzy systems with input saturation. A fuzzy-based sampled-data control is designed to stabilize the T-S fuzzy systems. Based on the Lyapunov method and some integral inequality techniques, a set of sufficient conditions is obtained as linear matrix inequality (LMI) constraints to guarantee the asymptotic stability of the considered system. In this process, the linear switching method is utilized to design a controller that is dependent on the membership function, and an integral inequality is utilized. Additionally, determination of the controller parameters is achieved by resolving a series of LMI constraints. The effectiveness of these criteria is demonstrated through a real system that is modeled by the T-S system.
Citation: YeongJae Kim, YongGwon Lee, SeungHoon Lee, Palanisamy Selvaraj, Ramalingam Sakthivel, OhMin Kwon. Design and experimentation of sampled-data controller in T-S fuzzy systems with input saturation through the use of linear switching methods[J]. AIMS Mathematics, 2024, 9(1): 2389-2410. doi: 10.3934/math.2024118
[1] | Keyu Zhang, Yaohong Li, Jiafa Xu, Donal O'Regan . Nontrivial solutions for a fourth-order Riemann-Stieltjes integral boundary value problem. AIMS Mathematics, 2023, 8(4): 9146-9165. doi: 10.3934/math.2023458 |
[2] | Yinyin Wu, Fanfan Chen, Qingchi Ma, Dingbian Qian . Subharmonic solutions for degenerate periodic systems of Lotka-Volterra type with impulsive effects. AIMS Mathematics, 2023, 8(9): 20080-20096. doi: 10.3934/math.20231023 |
[3] | Rui Wu, Yi Cheng, Ravi P. Agarwal . Rotational periodic solutions for fractional iterative systems. AIMS Mathematics, 2021, 6(10): 11233-11245. doi: 10.3934/math.2021651 |
[4] | Chunlian Liu, Shuang Wang . Multiple periodic solutions of second order parameter-dependent equations via rotation numbers. AIMS Mathematics, 2023, 8(10): 25195-25219. doi: 10.3934/math.20231285 |
[5] | Xiao Wang, D. D. Hai . On a class of one-dimensional superlinear semipositone (p,q) -Laplacian problem. AIMS Mathematics, 2023, 8(11): 25740-25753. doi: 10.3934/math.20231313 |
[6] | Famei Zheng . Periodic wave solutions of a non-Newtonian filtration equation with an indefinite singularity. AIMS Mathematics, 2021, 6(2): 1209-1222. doi: 10.3934/math.2021074 |
[7] | Chunlian Liu, Shuang Wang, Fanfan Chen . Resonance with Landesman-Lazer conditions for parameter-dependent equations: a multiplicity result via the Poincaré-Birkhoff theorem. AIMS Mathematics, 2024, 9(10): 28320-28340. doi: 10.3934/math.20241374 |
[8] | Jian Liu . Further generalization of Walker’s inequality in acute triangles and its applications. AIMS Mathematics, 2020, 5(6): 6657-6672. doi: 10.3934/math.2020428 |
[9] | Limin Li . Normwise condition numbers of the indefinite least squares problem with multiple right-hand sides. AIMS Mathematics, 2022, 7(3): 3692-3700. doi: 10.3934/math.2022204 |
[10] | Shaoyuan Xu, Yan Han, Qiongyue Zheng . Fixed point equations for superlinear operators with strong upper or strong lower solutions and applications. AIMS Mathematics, 2023, 8(4): 9820-9831. doi: 10.3934/math.2023495 |
In this study, the stability and stabilization analyses are discussed for Takagi-Sugeno (T-S) fuzzy systems with input saturation. A fuzzy-based sampled-data control is designed to stabilize the T-S fuzzy systems. Based on the Lyapunov method and some integral inequality techniques, a set of sufficient conditions is obtained as linear matrix inequality (LMI) constraints to guarantee the asymptotic stability of the considered system. In this process, the linear switching method is utilized to design a controller that is dependent on the membership function, and an integral inequality is utilized. Additionally, determination of the controller parameters is achieved by resolving a series of LMI constraints. The effectiveness of these criteria is demonstrated through a real system that is modeled by the T-S system.
In this paper, we consider the existence of periodic solutions of the indefinite planar system
z′=f(t,z),z=(x,y)∈R2, | (1.1) |
where f∈C(R×R2,R2) is 2π-periodic with respect to the first variable and locally Lipschitz -continuous with respect to the second variable, guaranteeing uniqueness for the associated Cauchy problems.
Resonance and non-resonance problems are typical models in ordinary differential equations. There are many interesting results on these topics.
Especially, many authors are interested in the study of these problems under asymmetric conditions, even one-sided superlinear growth conditions, which can be traced back to the work of Dancer [1] and Fučík [2]. Dancer and Fučík studied the existence of 2π-periodic solutions of the equation
x″+f(t,x)=0 | (1.2) |
when f(t,x)=ax+−bx–p(t), where p is a 2π-periodic and continuous function, n is a positive integer, x+=max{x,0} and x−=max{−x,0}, satisfying
1√a+1√b=2n. | (1.3) |
If the positive number pair (a,b) does not satisfy (1.3), then for any 2π-periodic continuous function p, Eq (1.2) has a 2π-periodic solution provided that a≠b. For n=1,2,…, all of the number pairs (a,b) satisfying (1.3) are called the Fučík spectrum. When a=b, Eq (1.2) with f as above becomes
x″+n2x=p(t). | (1.4) |
The linear Eq (1.4) has the classical linear resonance phenomenon, that is, periodic solutions and unbounded solutions cannot coexist; thus either the solutions of Eq (1.4) are all periodic solutions (cf. [3]), or the solutions of Eq (1.4) are all unbounded solutions.
Based on the study of the asymmetric nonlinearity f satisfying
A+≤lim infx→+∞f(t,x)x≤lim supx→+∞f(t,x)x≤B+, | (1.5) |
A−≤lim infx→−∞f(t,x)x≤lim supx→−∞f(t,x)x≤B−, | (1.6) |
for some suitable constants A±,B±∈[0,+∞], the existence of solutions to Eq (1.2) is strictly related to the position of the rectangle W=[A+,B+]×[A−,B−] with respect to the set called the Fučík spectrum. According to the "position", there are three kinds of problems, i.e., non-resonance (cf. [1,2,4,5,6,7,8,9,10,11,12,13]), simple resonance (cf. [14,15,16]) and double resonance (cf. [14,17,18,19,20]). Especially, the book [21] is devoted to both resonance and non-resonance situations.
In particular, Drábek and Invernizzi [4] generalized Fučík's work [2] to Eq (1.2), and assumed that (1.5) and (1.6) hold uniformly for t, and for some integer n, the relations
1√A++1√A−<2n,1√B++1√B−>2n+1 | (1.7) |
hold. And a complementary result was obtained by Mawhin and Ward [5]; they considered a Liénard equation together with an assumption of the type A+>0,A−>0,B−<1/4; a similar case refers to A+>0,A−>0 and B+<1/4.
Thereafter, Fabry and Habets [7] generalized the above work and considered a non-resonance situation with respect to the Dancer-Fučík spectrum, specifically, where f is allowed to have superlinear growth on one side as follows.
a+(t)≤lim infx→+∞f(t,x)x≤lim supx→+∞f(t,x)x≤b+(t), |
a−(t)≤lim infx→−∞f(t,x)x, |
where a±(t), b+(t) satisfy the non-resonance conditions similar to that between two neighboring Fučík spectrum curves, and the following sign condition
lim infx→+∞sgn(x)f(t,x)>0. |
It is noted that the particularity of the non-resonance conditions given in [7] is that f is allowed to grow superlinearly on the side of x<0.
In these situations mentioned above the function f is possibly superlinear on one side. Concerning this problem, one need also notice the early works of Schmitt [22], Reissig [23], Fonda and Zanolin [6], Fernandes and Zanolin [24] and Fabry and Fonda [25].
In recent years, Qian [8] studied the existence of solutions of the Liénard equation by applying topological degree theory under asymmetric conditions. Such a work in [8] gave a contribution in the direction of considering the one-sided growth restrictions for the Liénard equation, as in [26,27]. And then, Fonda and Sfecci [9] generalized the work of Fabry and Habets [7] and proposed a general method to prove the existence of periodic solutions for planar systems similar to system (1.1) that can be applied to many different circumstances involving the case of systems with superlinear growth in some direction; their work contributed in the direction of considering the one-sided growth restrictions for planar systems. Fonda and Sfecci [10] generalized the work in [9] to the case of higher dimensions and applied the corresponding results to the periodic problems associated with a system of coupled oscillators with one-sided superlinear nonlinearities and other types of retraction forces.
About the non-resonance case, one needs to also notice the works that yielded multiplicity results of periodic solutions done by Rebelo and Zanolin [28,29], Liu [30], Zanini and Zanolin [31] and so on.
The cases of simple and double resonance seem to start from the work of Fabry [14], which considered the periodic boundary value problems under Landesman-Lazer conditions for Eq (1.2), where at least one of the inequalities in (1.7) is replaced by an equality; such cases mean that the rectangle [A+,B+]×[A−,B−] touches one or two of the Fučík curves. Sfecci [20] dealt with the problem of the existence of T−periodic solutions for Eq (1.2) with the asymmetric nonlinearity satisfying the following one-sided superlinear growth at infinity,
limx→−∞f(t,x)x=+∞; |
a double resonance condition, namely, there exists a constant c>0 and an integer N>0 such that
μNx−c≤f(t,x)≤μN+1x+c |
for every x>0 and every t∈[0,T], where μj=(jπ/T)2, and the Landesman-Lazer conditions (see the details in Theorem 1.1 of [20]).
Especially, there are a series of works on the existence of unbounded solutions (cf. [32,33,34] and the references therein) and coexistence of periodic solutions and unbounded solutions (cf. [32,35,36] and the references therein) under asymmetric conditions.
In this paper, we would like to generalize the results in [8,9] to the case of non-resonance with one-sided superlinear growth for the indefinite planar system described by system (1.1) from a rotation number viewpoint; we do not assume the sign conditions similar to that in [6,7,8,9]. Sign conditions were frequently needed in the previous works; as we know, without sign conditions, some classical theories or methods may not be used, and one has to overcome the difficulties stemming from the variable signs of nonlinearities and seek (or even develop) suitable methods. So the non-resonance in the sense of rotation number is different from the non-resonance in [6,7,8,9] (see Remark 1.2 in this paper, Example 1.1 in [37] or Remark 1.3 in [38]). The rotation number is an important index for dynamical systems on the torus or second order linear periodic equations with variable coefficients; it plays a very important role in the study, and some authors have used it to solve problems, see for instance [39,40,41,42,43,44].
Now back to our generalization, the main difficulty of this problem is how to use the rotation numbers of positively homogeneous systems to estimate the rotation angle difference of solutions of system (1.1) under the one-sided superlinear growth conditions and the sign-varying condition. To this end, we develop a system methodology of "tracking" the angle difference of the solutions of system (1.1) on each small interval on the given side under sign-varying conditions, which is different from that in [13], because the the assumptions on the right half-plane are different, and so are the methods used to estimate the lower bound of the rotation angle difference. One can complete the estimation of the lower bound directly by exploiting the relationship between the rotation numbers and the 2π-rotation numbers (see the definition in Section 2 in [43]) of the solutions in [13].
Here, and throughout the paper, J=(0−110) is the standard symplectic matrix. ρ(Li),i=1,2 represents the rotation numbers of the systems z′=Li(t,z),i=1,2; see the definition in Section 2.
In this paper, we suppose that
(H0)f∈C(R×R2,R2) is 2π-periodic with respect to t, the solution of the initial value problem of system (1.1) is unique and exists globally;
(H1) ρ(L1)>n2,L1(t,z) is continuous, 2π-periodic with respect to t, positively homogeneous of degree 1 and odd with respect to z, and when x≤0 (x is the first component of z),
lim infλ→+∞⟨Jf(t,λz)λ,z⟩≥⟨JL1(t,z),z⟩ |
holds uniformly for a.e. t∈[t0,t0+2π] and z∈S1;
(H2) ρ(L2)<n+12,L2(t,z) is continuous, 2π-periodic with respect to t, positively homogeneous of degree 1 and odd with respect to z, and when x≤0 (x is the first component of z),
lim supλ→+∞⟨Jf(t,λz)λ,z⟩≤⟨JL2(t,z),z⟩ |
holds uniformly for a.e. t∈[t0,t0+2π] and z∈S1;
(H3) When x>0 (x is the first component of z),
limλ→+∞⟨Jf(t,λz)λ,z⟩=+∞ |
holds uniformly for a.e. t∈[t0,t0+2π] and z∈S1;
(H4) ysgn(f1(t,0,y))<0 (f1 is the first component of f) and ysgn(Li1(t,0,y))<0 (Li1 is the first component of Li), i=1,2 when |y|≫1.
Then our main result is as follows.
Theorem 1.1. Suppose that conditions (H0)−(H4) hold, then system (1.1) has a 2π-periodic solution.
Remark 1.1. Regarding condition (H0), we directly assume the existence, uniqueness and global existence of solutions, because the application of the Poincaré-Bohl theorem is based on the assumption that the Poincaré map of the system is well defined, which requires the global existence of solutions of the corresponding initial value problem. In fact, there are many conditions guaranteeing the existence, uniqueness and global existence of solutions wherein the uniqueness of solutions of the initial value problem can be guaranteed by the local Lipschitz continuity of f with respect to z. And for global existence, for example, it can be guaranteed that, if f(t,z) satisfies the quasi-linear growth condition, namely, there exists a,b∈L1(t0,t0+2π) such that
|f(t,z)|≤a(t)|z|+b(t),fora.e. t∈[0,2π] and z∈R. |
In [9], Fonda and Sfecci introduced a rotating regular spiral assumption and an angular speed controlling assumption to guarantee the global existence of the solutions to system (1.1); see the assumptions (H1) and (H3) in Theorem 2.3 in [9].
Remark 1.2. In Theorem 1.1, we do not assume the sign conditions. As we know, sign conditions were frequently needed in the previous works on periodic solutions problems, including the classic works of Jacobowitz and Hartman [45,46], Ding, Iannacci and Zanolin [47], Fonda and Toader [48] and the references therein. Once there is a lack of sign conditions, the continuation method (see its applications in [49,50]) may not be used. The basic idea of the continuation method is to reduce nonlinear systems similar to system (1.1) to simple systems by applying homotopy invariance of the topological degree, and the simple systems are usually autonomous ones. Then one can compute the topological degrees of some of the operators associated with the autonomous systems, and the degrees are not zero; when the indefinite weight appears, the reduced autonomous systems are not easy to find, but the indefinite systems still meet some non-resonant conditions, such as the non-resonant conditions in the assumptions (H1) and (H2), (H2) in [37] and (HPB) in [38]. Consider the one-sided superlinear conditions for the system
−y′=g1(t,x),x′=g2(t,y) |
in Corollary 3.11 of [9], which requires all constants in the formulas (36)–(39) to be positive. Compared with the asymmetric problems in Theorem 1.1 in [8], the sign conditions are needed (Because the oddness assumption for the positively homogeneous systems may not be satisfied in [8], the comparison lies only in the sign conditions). So the notion of non-resonance with one-sided superlinearity in the sense of rotation numbers is different from that in [8,9] in the sense without sign conditions.
Remark 1.3. Fabry and Fonda [12] studied periodically forced planar Hamiltonian systems, have obtained sharp non-resonance results, and have provided new insights into the non-resonance problems by using the energy and a phase as variables. Under the framework, some classical situations can be covered, like asymmetric oscillators, positively homogeneous Hamiltonians of degree 2 or Hamiltonians with separated variables. In the final section of [12], the authors considered the more general system Jz′=F(t,z), which is similar to system (1.1) in the present paper, and obtained existence conditions for the periodic solutions via a comparison with Hamiltonian systems. However, such Hamiltonian systems may not be found in indefinite problems, so we obtain the existence of the periodic solutions via a comparison with the positively homogeneous planar systems z′=Li(t,z),i=1,2, which may not be isochronous.
Remark 1.4. In Theorem 1.1, conditions (H1)–(H3) show the control of the angular velocity by the homogeneous systems on the left half-plane and the superlinearity on the right half-plane, and (H4) shows that the solution curve rotates counterclockwise. These conditions have equivalent forms, so Theorem 1.1 could be stated in a more general way. We will discuss this in Section 4.
Remark 1.5. In Theorem 1.1, n is an integer, and it can be equal to 0. In this case, we can obtain that the solution curve of system (1.1) rotates less than one turn in 2π time, so it also fits the framework of the Poincaré-Bohl theorem adding other conditions.
For the controls with half-integers, from the superlinear growth condition for the right half-plane, one can see that the solution curve for the system z′=f(t,z) can rotate half a circle in a sufficiently short time when it is on the right half-plane, this means that the solution curve for the system z′=L(t,z) has not rotated out of the left half-plane if their initial state is on the left half-plane (see the proof of Lemma 3.8). Thus, in this sense, half-integers for the rotation numbers of the systems z′=Li(t,z),i=1,2 are enough to estimate the rotation angle difference. And half-integers are also used for the control of the rotations in [41].
For the reader's convenience, we present the Poincaré-Bohl fixed point theorem as follows.
Theorem 1.2. (Poincaré-Bohl theorem) Let Ω⊂Rm be an open bounded set containing the origin, and φ:¯Ω→Rm be a continuous function such that
φ(u)≠λu,foreveryu∈∂Ωandλ>1. |
Then, φ has a fixed point in ¯Ω.
This paper is organized as follows. We introduce the rotation numbers and rotation angle differences of the positive homogeneous systems in Section 2. We show the estimations of the rotation angle differences of the solutions of system (1.1) in Section 3. In Section 4, we give the proof, more general states and applications of Theorem 1.1. Finally, in Section 5, we give the proofs of some technical lemmas.
In this section, similar to that in Section 2 in [13], we consider the relationship between the rotation number of the positively homogeneous planar system
z′=L(t,z),z=(x,y)∈R2, | (2.1) |
which is oddly symmetric, and the rotation angle difference of the planar system (1.1). For the sake of presentation, we retell them as follows.
Let L:R×R2→R2 be a L1-Carathédory function, 2π-periodic with respect to the first variable, positively homogeneous of degree 1 with respect to the second variable, namely
L(t,λz)=λL(t,z),∀λ≥0,∀z∈R2, |
and it is also odd with respect to the second variable. In the following, denote by Q the set of all of the functions with the above properties.
Next, we define the rotation number of system (2.1) and the rotation angle difference of system (2.1) and system (1.1).
Assume that the solution of the initial value problem of system (2.1) exists and is unique. For a solution z(t;z0) with the initial value z0≠(0,0), by the polar coordinates transformation, it can be written as
x(t)=r(t)cosθ(t),y(t)=r(t)sinθ(t); |
thus we have
θ′(t)=−⟨Jz′,z⟩|z|2=−⟨JL(t,z),z⟩|z|2=−⟨JL(t,(cosθ,sinθ)),(cosθ,sinθ)⟩=:ψ(t,θ;L). | (2.2) |
Denote by θ(t;t0,θ0) the unique solution of Eq (2.2) with θ(t0;t0,θ0)=θ0. Since ψ(t,θ;L) is 2π-periodic for t and π-periodic for θ when L(t,z) is 2π-periodic for t and odd for z, Eq (2.2) is a differential equation on a torus. Thus
θ(t+2π;t0+2π,θ0)=θ(t;t0,θ0) |
and
θ(t;t0,θ0+π)=θ(t;t0,θ0)+π | (2.3) |
hold for arbitrary θ0,t0∈R. Therefore, we can define the rotation number of system (2.1) as follows;
ρ(L):=limt→∞θ(t0+t;t0,θ0)−θ0t=limk→∞θ(t0+2kπ;t0,θ0)−θ02kπ, |
and it is independent of t0 and θ0; see Theorem 2.1 in Chapter 2 of Hale [51]. By extension, we refer to ρ(L) as the rotation number of the positively homogeneous planar system (2.1).
Then, we give the definitions of the rotation angle differences of system (2.1) and system (1.1). Denote the rotation angle difference of system (2.1) in the time segment [t0,t′0] by △θ(t0,t′0), △θ(t0,t′0):=θ(t′0;t0,θ0)−θ0 and denote the rotation angle difference of system (1.1) in the time segment [t0,t′0] by △φ(t0,t′0), △φ(t0,t′0):=φ(t′0;t0,r0,φ0)−φ0, where φ(t;t0,r0,φ0) denotes the angle component of the solutions of system (1.1) satisfying the initial condition z(t0;t0,z0)=z0, t′0≤t0+2π.
Based on the above definitions, we consider the comparison lemma about the relationship between the rotation angle differences of system (1.1) and system (2.1).
First, similar to that in [37,41,43,52], we consider the relationship between the rotation number ρ(L) of system (2.1) and the rotation angle difference △θ(t0,t′0) of its solutions in the time segment [t0,t′0]. The proofs of Lemma 2.2 and Lemma 2.3 are also similar to that in [37]. For convenience, we will give the proofs of Lemmas 2.1–2.3 in Section 5.
Lemma 2.1. Let n∈Z; then
(i) ρ(L)<n⇒△θ(t0,t′0)<2nπ, ∀(t0,θ0)∈R2, t′0≤t0+2π;
(ii) ρ(L)>n⇒△θ(t0,t0+2π)>2nπ, ∀(t0,θ0)∈R2;
(iii) ρ(L)=n if and only if there is at least one nontrivial 2π-periodic solution θ(t;t0,θ0) of Eq (2.2) with θ(t0+2π;t0,θ0)−θ0=2nπ.
Next, we state the depending relationship lemma about the rotation angle difference △θ(t0,t′0) of system (2.1) and the function L(t,z).
Lemma 2.2. Assume Li(t,z)∈Q,i=1,2 and that for all z∈R2,
⟨JL2(t,z)−JL1(t,z),z⟩≥0 | (2.4) |
holds for a.e. t∈[t0,t′0], t′0≤t0+2π. Then
△θ2(t0,t)≥△θ1(t0,t),∀t∈[t0,t′0]. |
Second, we state the comparison result for the rotation angle difference of system (2.1) for different functions Li(t,z)(i=1,2).
Lemma 2.3. Suppose Li(t,z)∈Q, i=1,2. Assume also that for all ε>0, there exists δ>0 such that
⟨JL2(t,z),z⟩≥⟨JL1(t,z),z⟩−δ|z|2 | (2.5) |
holds for all z∈R2 and a.e. t∈[t0,t′0], t′0≤t0+2π. Then
△θ2(t0,t)≥△θ1(t0,t)−ε,∀t∈[t0,t′0]. | (2.6) |
Third, similar to the discussion in Section 2 in [13], we give the relationship of the rotation angle difference between system (2.1) and system (1.1). From (H1) and (H2), we deduce the following two properties.
(H′1) When x≤0, there exists L1(t,z)∈Q satisfying ∀δ>0,∃l(t)∈L1([t0,t′0],R+) such that
⟨Jf(t,λz)λ,z⟩≥⟨JL1(t,z),z⟩−δ|z|2−l(t) | (2.7) |
holds uniformly for a.e. t∈[t0,t′0], z∈S1 and λ≥1;
(H′2) When x≤0, there exists L2(t,z)∈Q satisfying ∀δ>0,∃l(t)∈L1([t0,t′0],R+) such that
⟨Jf(t,λz)λ,z⟩≤⟨JL2(t,z),z⟩+δ|z|2+l(t) |
holds uniformly for a.e. t∈[t0,t′0], z∈S1 and λ≥1.
Then, applying the above two lemmas we have the following comparison lemma.
Lemma 2.4. (Comparison Lemma A) Suppose Li∈Q,i=1,2 and f:R×R2→R2 is 2π-periodic with respect to the first variable t and continuous with respect to the second variable z.
(i) If (H′1) holds, then for each ε>0, there exists Rε>0 such that
△φ(t0,t)≥△θ1(t0,t)−ε,∀t∈[t0,t′0] | (2.8) |
holds for every solution of system (1.1) satisfying |z(t)|≥Rε,∀t∈[t0,t′0].
(ii) If (H′2) holds, then for each ε>0, there exists Rε>0 such that
△φ(t0,t)≤△θ2(t0,t)+ε,∀t∈[t0,t′0] | (2.9) |
holds for every solution of system (1.1) satisfying |z(t)|≥Rε,∀t∈[t0,t′0].
Remark 2.1. By the assumption of oddness, the solutions of z′=L(t,z) spend the same time in the left and right half-plane. In fact, using Eq (2.2) we can calculate the times spent by the solutions in the left half-plane and right half-plane using
△t=∫θ2θ11θ′dθ=−∫θ2θ11⟨JL(t,(cosθ,sinθ)),(cosθ,sinθ)⟩dθ. |
Then we can obtain that △tL=△tR by a variable substitution. △tL and △tR denote the times spent by the solutions in the left and right half-planes, respectively.
In this section, we denote the solution of the equation θ′=ψ(t,θ)−ε by θε(t;t0,θ0), where ε>0, and the angle component of the solution of system (1.1) by φ(t;t0,r0,φ0). Because we only discuss the solutions for sufficiently large r0 in the following parts and the estimation formulas hold for sufficiently large r(t), we sometimes note φ(t;t0,r0,φ0) as φ(t;t0,φ0) for short.
First, similar to Lemma 4.3 in [37] and Lemmas 3.4 and Lemma 3.5 in [13], we give an orientation- preserving lemma and a sign lemma.
Lemma 3.1. (Orientation preserving lemma) Suppose that all of the solutions of the equation θ′=ψ(t,θ) satisfying the initial value conditions exist uniquely; let θ(t;t0,θ0) and θ(t;t0,θ1) be two solutions of Eq (2.2) with the respective initial values θ(t0;t0,θ0)=θ0 and θ(t0;t0,θ1)=θ1, t∈[t0,t′0). If θ0<θ1(θ0>θ1), then
θ(t;t0,θ0)<θ(t;t0,θ1)(θ(t;t0,θ0)>θ(t;t0,θ1)),t∈[t0,t′0). |
Lemma 3.2. (Sign lemma) Assume (H4); if θ(t∗;t0,θ0)≥π2+kπ, then ∀t>t∗, and we have θ(t;t0,θ0)>π2+kπ.
Second, we give the comparison lemma after the following two preliminary lemmas.
Lemma 3.3. Suppose that ε>0, and ψ(t,θ) is a continuous function, 2π-periodic with respect to t, differentiable and π-periodic with respect to θ. Let θ(t;t0,θ0) and θε(t;t0,θ0) be solutions of θ′=ψ(t,θ) and θ′=ψ(t,θ)−ε, respectively. Then there exists Kε<0 such that θε(t)≤θ(t)+Kε(t−t0),t≥t0.
Proof. Let ¯θ(t)=θε(t)−θ(t); then ¯θ(t) satisfies
(¯θ(t))′=a(t)¯θ(t)−ε, | (3.1) |
where a(t)=∂ψ∂θ(t,ξ) and ξ(t) is between θ(t) and θε(t).
Integrating on both sides of Eq (3.1) from t0 to t, we have
¯θ(t)=∫tt0−εexp(∫tsa(τ)dτ)ds. |
Take Kε=maxt0≤t≤t0+2π−εexp(∫tt0a(s)ds); then
¯θ(t)≤Kε(t−t0). |
And by a direct application of the definition of the rotation number of the positively homogeneous system (2.1), we have the following lemma.
Lemma 3.4. Suppose ρ(L1)>n2; then there exists ε>0 such that ρ(Lε)>n2, where Lε(t,z)=L1(t,z)−εJz.
We know that there exists Lε such that ρ(Lε)>n2 under the conditions of Lemma 3.1. For this ε>0, by the definition of rotation numbers there exists δ>0 such that
θε(t0+2π;t0,θ0)−θ0>nπ+2δ | (3.2) |
holds for an arbitrary t0 and θ0.
Lemma 3.5. (Comparison Lemma B) Suppose that ε>0, ψ(t,θ) is a continuous function, 2π-periodic with respect to t, differentiable and π-periodic with respect to θ. Then for the above ε>0, there exists rε with r0≥rε such that
φ(t;t′0,θ′0)>θε(t;t′0,θ′0)+δ |
holds for the angle variable of solutions in the left plane when t≥t′0+tε, ∀t′0∈[t0,t0+2π]. It is given that tε=min{t−t′0|t>t′0,θε(t)−θε(t′0)≥δ}>0 and δ is sufficiently small such that Kεtε+δ<0.
Proof. θε(t;t0,θ0) satisfies
θ′ε(t)=ψε(t,θε(t)), |
where ψε=−⟨JL(t,cosθ,sinθ),(cosθ,sinθ)⟩−ε=ψ(t,θ)−ε. Take ψM=max(t,θ)∈[0,2π]×[0,2π]|ψε(t,θ)|; then tε≥δψM>0. By (H1) and Comparison Lemma A we can obtain that, for Kε and tε, there exists rε>0 such that
φ(t;t′0,r′0,θ′0)>θ(t;t′0,θ′0)−ε>θ(t;t′0,θ′0)+Kεtε+δ |
holds for the angle variable of solutions in the left half-plane when r′0≥rε, where ε<−Kεtε−δ.
Then by Lemma 3.3 and Kε<0,
θε(t;t′0,θ′0)≤θ(t;t′0,θ′0)+Kε(t−t′0)≤θ(t;t′0,θ′0)+Kεtε |
holds when t≥t′0+tε. Thus
φ(t;t′0,r′0,θ′0)>θε(t;t′0,θ′0)+δ, |
that is
φ(t;t′0,θ′0)>θε(t;t′0,θ′0)+δ. |
Lemma 3.6. Assume (H3), then Δφt→0 when r0→+∞, where φ denotes the angle component of the solutions of system (1.1) and Δφt denotes the time spent by the solutions of system (1.1) to rotate through the right half-plane.
Proof. Using the polar coordinate transformation x=rcosφ,y=rsinφ we can obtain
φ′=−1r2⟨Jf(t,z),z⟩, |
then
Δφt=∫π2−π2r2|⟨Jf(t,z),z⟩|dφ=∫π2−π21|⟨Jf(t,(rcosφ,rsinφ))r,(cosφ,sinφ)⟩|dφ. | (3.3) |
Passing to the limit as r0→+∞, by the global existence of solutions, we can obtain r(t)→+∞. Then using (H3) and the dominated convergence theorem, and by (3.3) we have
limr0→+∞Δφt=0. |
Similar to the proof of Lemma 3.7 in [13], we have the following lemma.
Lemma 3.7. Assume that conditions (H0),(H2) and (H4) hold; and then we have that ∃R1>0 for every solution of system (1.1) satisfying |z(t)|≥R1, ∀t∈[t0,t0+2π],
φ(t0+2π;t0,φ0)−φ0<2(n+1)π |
holds for an arbitrary t0 and φ0.
Lemma 3.8. Assume that conditions (H0), (H1),(H3) and (H4) hold; then we have that ∃R2>0 for every solution of system (1.1) satisfying |z(t)|≥R2, ∀t∈[t0,t0+2π],
φ(t0+2π;t0,φ0)−φ0>2nπ |
holds for an arbitrary t0 and φ0.
Before the proof, we want to emphasize that estimates in this lemma are uniform in the radius, and that these estimates are completed under the state that the radius is sufficiently large.
Proof. Case 1. Without loss of generality, we suppose θε(t0+2π;t0,θ0)−θ0<(n+1)π. First, suppose θ0∈[π2,3π2−δ] and let θε(t′j)=π2+jπ,j=1,⋯,n with t0<t′1<⋯<t′n<t0+2π. Then, by (3.2) we have
3π2−θ0+θε(t0+2π;t0,θ0)−θε(t′n)>π. | (3.4) |
It suffices to show that
φ(t0+2π;t0,φ0,r0)−φ0>2nπ |
holds when r0 is sufficiently large. Without loss of generality, we suppose φ(t0+2π;t0,φ0,r0)−φ0<2(n+1)π and denote φ0=θ0 and φ(t)=φ(t;t0,φ0,r0) for short. Suppose that tj and τj,j=1,⋯,n satisfy
φ(tj)=π2+(2j−1)π,φ(τj)=π2+2jπ,j=1,⋯,n, |
respectively.
Next we compare τ1 with t′1. Right now θε(t) and φ(t) have the same initial state (t0,θ0), and both are in the left half-plane (see Figure 1, where △tθ=t′1−t1 denotes the time spent by the solution of system (2.1) rotating from t1 to t′1). By assumptions and Lemma 3.5 we obtain
φ(t;t0,θ0)>θε(t;t0,θ0)+δ,t∈(t0,t′1]∩(t0,τ1] |
when θ0<3π2−δ.
Then
θε(t1;t0,θ0)<φ(t1;t0,θ0)=π2+π, |
so, we have
θε(t1;t0,θ0)<θε(t′1;t0,θ0); |
then by the orientation-preserving lemma (Lemma 3.1) we can obtain t1<t′1. By the definition of φ(tj) and φ(τj), j=1,2,⋯,n, when the solution curve moves from t1 to τ1, it is in the right half-plane (see Figure 1, where △tφ=τ1−t1 denotes the time used by the solution of system (1.1) rotating from t1 to τ1). Then by (H3) and Lemma 3.6 we know that the time τ1−t1 used by φ from t1 to τ1 is shorter than the time used by θε from t1 to t′1 denoted by tε, that is τ1−t1<tε; then we can obtain τ1<t′1.
So
θε(τ1;t0,θ0)<θε(t′1;t0,θ0)=θε(t′1;t′1,π2+π). |
Take θ1=θε(τ1;t0,θ0), then θ1<π2+π.
Therefore, by the orientation-reserving lemma (Lemma 3.1),
θε(t;τ1,θ1)<θε(t;τ1,π2+π) |
holds for t>τ1.
Next we compare τ2 with t′2. φ starts from (τ1,5π2) to (t2,φ(t2))=(t2,π2+3π) right now; meanwhile θε also starts from τ1 to t2.
First, we compare t2 and t′2. Suppose t2≥t′2, and apply Lemma 3.5 on the interval [τ1,t2]. Because L1(t,z) is odd for z, (H1) holds; then we can obtain
φ(t2;τ1,π2+2π)>θε(t2;τ1,π2+2π)>θε(t2;t′1,π2+2π), | (3.5) |
θε(t′2;t′1,π2+2π)=θε(t′2;t′1,π2+π)+π≤θε(t2;t′1,π2+2π); | (3.6) |
thus by (3.5) and (3.6) we have
π2+3π>π2+2π+π=π2+3π, |
which is a contradiction. Therefore, t2<t′2. And by (H3) and Lemma 3.6 we know that the time used by φ from t2 to τ2 is shorter than the time used by θε from t2 to t′2 denoted by t′ε, that is τ2−t2<t′ε; so we know τ2<t′2.
So
θε(τ2;t0,θ0)<θε(t′2;t0,θ0)=θε(t′2;t′2,π2+2π). |
Take θ2=θε(τ2;t0,θ0); then θ2<π2+2π.
Therefore, by the orientation-reserving lemma (Lemma 3.1),
θε(t;τ2,θ2)<θε(t;τ2,π2+2π) |
holds for t>τ2.
Then we can obtain τn<t′n by a similar discussion as above. Notice that θε(t′n)=π2+nπ, so
θε(τn;t0,θ0)<θε(t′n;t0,θ0)=θε(t′n;t′n,π2+nπ)=π2+nπ, |
that is
θn=θε(τn;t0,θ0)<π2+nπ. |
By the orientation-reserving lemma (Lemma 3.1) we have
θε(t;τn,θn)<θε(t;τn,π2+nπ), |
when t>τn. By Lemma 3.5, the translation property and the above inequality, and as compared on [τn,t0+2π], we have
φ(t0+2π;τn,π2+2nπ)>θε(t0+2π;τn,π2+2nπ)=θε(t0+2π;τn,π2+nπ)+nπ>θε(t0+2π;τn,θn)+nπ=θε(t0+2π;t0,θ0)+nπ; |
combined with (3.4), we have
φ(t0+2π;τn,π2+2nπ)>π2+nπ+θ0−3π2+π+nπ=θ0+2nπ, |
that is
φ(t0+2π;t0,θ0)−θ0>2nπ. |
Case 2. Suppose θ0∈[3π2−δ,3π2], consider the solution θε(t;t0,3π2−δ) of Eq (2.2) starting from (t0,3π2−δ) and denote θε(t′j)=π2+jπ,φ(tj)=π2+(2j−1)π,φ(τj)=π2+2jπ,j=1,⋯,n.
Next we compare τ1 and t′1. Right now, the initial state of θε(t) is (t0,3π2−δ), which means that θ0=3π2−δ, the initial state of φ(t) is (t0,φ0), with φ0≥θ0, and that both are in the left half-plane. Then by Lemma 3.5,
φ(t;t0,φ0)>θε(t;t0,θ0),t>t0 |
holds when φ0∈[3π2−δ,3π2]; then θε(t1;t0,θ0)<φ(t1;t0,φ0)=π2+π; similar to that in Case 1 we have t1<t′1. And by the superlinearity on the right half-plane we know that the time used by φ from t1 to τ1 is shorter than the time used by θε from t1 to t′1 denoted by t′ε, that is τ1−t1<t′ε; then we can obtain τ1<t′1.
Similarly, we can obtain
τn<t′n, |
and by (3.2) we have
3π2−θ0+θε(t0+2π;t0,3π2−δ)−θε(t′n)>π+δ, |
namely
θε(t0+2π;t0,3π2−δ)−θε(t′n)>π. |
Then
φ(t0+2π;τn,π2+2nπ)>θε(t0+2π;τn,π2+2nπ)>θε(t0+2π;t′n,π2+2nπ)=θε(t0+2π;t′n,π2+nπ)+nπ>θε(t′n)+π+nπ=2nπ+3π2. |
Since φ0≤3π2, we have
φ(t0+2π;t0,φ0)−φ0>2nπ. |
Case 3. Suppose θ0∈(−π2,π2]; we discuss it in two cases.
First, when θ0=π2, take φ0=θ0. Right now θε(t) and φ(t) have the same initial state; denote θε(t′j)=π2+jπ and φ(tj)=π2+(2j−1)π,φ(τj)=π2+2jπ,j=1,⋯,n. According to the discussion in Case 1 we can prove
τ2<t′2,⋯,τn<t′n. |
And by Lemma 3.5, the translation property and (3.2), we have
φ(t0+2π;t0,θ0)=φ(t0+2π;τn,π2+2nπ)>θε(t0+2π;τn,π2+2nπ)>θε(t0+2π;t′n,π2+2nπ)=θε(t0+2π;t′n,π2+nπ)+nπ>θ0+nπ+nπ, |
that is
φ(t0+2π;t0,θ0)−θ0>2nπ. |
Second, when θ0∈(−π2,π2), we consider the solution θε(t;t0,−π2) of Eq (2.2) starting from (t0,−π2), and denote θε(t′j)=π2+jπ and φ(tj)=π2+(2j−1)π,φ(τj)=π2+2jπ,j=0,⋯,n; suppose that φ(t) and θε(t) have the same initial state (t0,−π2). By the superlinearity on the right half-plane we know that the time used by φ from t0 to τ0 is shorter than the time used by θε from t0 to t′0 denoted by t∗ε; thus we have τ0<t′0; then we can prove
τ1<t′1,⋯,τn<t′n, |
similar to the discussion in Case 1.
Thus
φ(t0+2π;t0,θ0)=φ(t0+2π;τn,π2+2nπ)>θε(t0+2π;τn,π2+2nπ)>θε(t0+2π;t′n,π2+2nπ)=θε(t0+2π;t′n,π2+nπ)+nπ, |
and by (3.2) we have
π2−θ0+θε(t0+2π;t′n,π2+nπ)−θε(t′n)>2δ, |
namely
θε(t0+2π;t′n,π2+nπ)>θ0+nπ+2δ; |
thus
φ(t0+2π;t0,θ0)−θ0>2nπ. |
Remark 3.1. In the proof of Lemma 3.8, an explanatory figure is used to to show where the counting of the rotations starts and the estimates of the rotation angle differences in each region. Figure 1 corresponding to Case 1, shows the tracking at the beginning. The statement on the other small time periods can be seen similarly.
Remark 3.2. The proof of Lemma 3.8 is based on the system methodology of "tracking" the angle difference of the solutions of system (1.1) on each small interval on the given side; it gives an estimation of the lower bound of the rotation angle difference of the solutions of system (1.1). This is different from the proof of Lemma 3.6 in [13] and the proof of Lemma 4.2 in [37]. In fact, the proof idea of the lower bound of the rotation angle difference is similar to thta in [13,37], namely, one can describe the 2π-rotation numbers of the solutions (from which one can calculate the rotation angle differences) of the positive homogeneous systems by the rotation numbers of themselves first; then through the discussion of the relationship of the 2π-rotation numbers between the planar systems and the positive homogeneous systems, one can finally realize the estimations of the 2π-rotation number of the planar system by using the rotation numbers of the positive homogeneous systems. Moreover, the "tracking" is different from that in the proof of Lemma 3.7 in [13] because of the superlinearity on the right half-plane.
In this section, we consider the proof, more general states and applications of our main result.
First, the proof idea of Theorem 1.1 is similar to that in [37], but there are some differences in details, so we state the proof as follows.
Proof of Theorem 1.1. Suppose that z(t;z0)=(x(t;z0),y(t;z0) is a solution of system (1.1) satisfying the initial value condition z(t0;z0)=z0=(x0,y0). Take ΩM={z∈R2:|z|<M}, and define the Poincaré map
P:¯ΩM→R2,(x0,y0)↦(x(t0+2π;z0),y(t0+2π;z0)). |
By (H0) we have the global existence of solutions; thus P is well defined. Moreover, the uniqueness of the solution implies that the solution depends continuously on the initial value. Therefore P is continuous. In addition, P has a fixed point z0∈R2 if and only if system (1.1) has a 2π periodic solution. Then our aim is to prove that, for M>0 sufficiently large, P has a fixed point by the Poincaré-Bohl theorem.
Applying the global existence of the solutions of system (1.1) again, as well as the continuous dependence of the solutions with respect to the initial values, we can prove the elastic properties of the solutions of system (1.1). That is, for R1 in Lemma 3.7 and R2 in Lemma 3.8, there exists MR>0 such that |z(t;z0)|>Ri, i=1,2 for t∈[t0,t0+2π] when |z0|≥MR.
Let M=MR. For any z0∈∂ΩM, we have |z(t;z0)|>Ri, i=1,2 for t∈[t0,t0+2π]. Then by conditions (H1)–(H4) and Lemma 3.7 and Lemma 3.8 we have
2nπ<φ(t0+2π;t0,φ0)−φ0<2(n+1)π, |
where n∈N, which implies that z(t;z0) is not able to rotate any integer number of turns in 2π time for t∈[t0,t0+2π]. Hence P(z0)≠λz0 for z0∈∂ΩM and λ>1. Using the Poincaré-Bohl theorem, we have that P has a fixed point. The proof is thus completed.
Second, we consider a more general state of Theorem 1.1. We assume the following conditions.
(H′4) When |y|≫1, ysgn(f1(t,0,y))>0 (f1 is the first component of f) and ysgn(Li1(t,0,y))>0 (Li1 is the first component of Li), i=1,2.
(H5) ρ(L1)>n2,L1(t,z) is continuous, 2π-periodic with respect to t, positively homogeneous of degree 1 and odd with respect to z; and when x≥0(x is the first component of z),
lim infλ→+∞⟨Jf(t,λz)λ,z⟩≥⟨JL1(t,z),z⟩ |
holds uniformly for a.e. t∈[t0,t0+2π] and z∈S1.
(H6) ρ(L2)<n+12,L2(t,z) is continuous, 2π-periodic with respect to t, positively homogeneous of degree 1 and odd with respect to z; and when x≥0 (x is the first component of z),
lim supλ→+∞⟨Jf(t,λz)λ,z⟩≤⟨JL2(t,z),z⟩ |
holds uniformly for a.e. t∈[t0,t0+2π] and z∈S1.
(H7) When x<0 (x is the first component of z),
limλ→+∞⟨Jf(t,λz)λ,z⟩=+∞ |
holds uniformly for a.e. t∈[t0,t0+2π] and z∈S1.
Then by the similar proof idea of Theorem 1.1, we have the following more general state of Theorem 1.1.
Theorem 4.1. Assume (H0), and that (H1)−(H2)−(H3)−(H4), (H1)−(H2)−(H3)−(H′4), (H5)−(H6)−(H7)−(H4) or (H5)−(H6)−(H7)−(H′4) hold; then system (1.1) has a 2π-periodic solution.
More generally, {x≤0} and {x>0} in conditions (H1)−(H3) can be replaced by {y≤0} and {y>0}, and {x≥0} and {x<0} in conditions (H5)−(H7) can be replaced by {y≥0} and {y<0}; Assumption (H4) and (H′4) need to be replaced by the following conditions.
(H8) When |x|≫1, xsgn(f2(t,x,0))<0 (f2 is the second component of f) and xsgn(Li2(t,x,0))<0 (Li2 is the second component of Li), i=1,2.
(H′8) When |x|≫1, xsgn(f2(t,x,0))>0 (f2 is the second component of f) and xsgn(Li2(t,x,0))>0 (Li2 is the second component of Li), i=1,2.
Then we can obtain similar results to that in Theorem 4.1. Since the expression is similar, the result and some conditions are not stated here.
Finally, we give an application of Theorem 1.1 (or Theorem 4.1).
Example 4.1. Consider the following equations
x″+ai(t)x=0,x″+bi(t)x=0,i=1,2, |
where ai(t),bi(t)∈L1([0,2π]) and ai(t)<bi(t), i=1,2, are 2π-periodic functions with definitions are as follows.
a1(t)={(n+1/2)2,t∈[0,π],c(t),t∈[π,2π],a2(t)={(n+δ)2,t∈[0,π],−λ2,t∈[π,2π], |
b1(t)={(n+3/4)2,t∈[0,π],−μ2,t∈[π,2π],b2(t)={(n+1/2)2,t∈[0,π],d(t),t∈[π,2π], |
where n∈N, c(t),d(t)∈L1([π,2π]), d(t)≥0, δ≥2(n+δ)arctan|λ|/π>0 and arctanμ≥π/2−π/(4(n+3/4)). For i=1 or i=2, we suppose that
ai(t)≤lim infx→−∞g(t,x)x≤lim supx→−∞g(t,x)x≤bi(t) | (4.1) |
holds uniformly for a.e. t∈[0,2π], and that
limx→+∞g(t,x)x=+∞, |
where g(t,x) is continuous, 2π-periodic with respect to t and locally Lipschitz continuous with respect to x. Then we can prove (the proof is similar to that in [38])
n/2<ρ(a1),ρ(a2),ρ(b1),ρ(b2)<(n+1)/2. |
Through verification, we can see that the conditions in Theorem 1.1 all hold; then x″+g(t,x)=0 has a 2π-periodic solution.
Remark 4.1. In this paper, we mainly consider the indefinite systems, where "indefinite" means that the nonlinearity in the research model is sign-varying. Specifically, for the typical second-order equation x″+a(t)h(x)=0, "indefinite" means that a(t) is sign-varying. And for x″+g(t,x)=0, it means that g(t,x) is sign-varying. In Example 4.1, x″+g(t,x)=0 is an indefinite equation, because by (4.1), we have that g(t,x) is sign-varying when −x≫1. And the equivalent system of x″+g(t,x)=0 is x′=−y,y′=g(t,x), which is an indefinite planar system. Besides, there are many indefinite systems (equations), such as x″+ai(t)x=0,i=1,2 and x″+b1(t)x=0, where ai(t),i=1,2 and b1(t) are defined as in Example 4.1.
First, we recall the definition of the rotation number of an orientation-preserving homeomorphism. Let h:R→R be an orientation-preserving homeomorphism such that
h(θ+2nπ)=h(θ)+2nπ,for allθ∈R,n∈Z. | (5.1) |
Then, we can define the rotation number of h as follows
ρ(h)=limk→∞hk(θ)−θ2kπ, |
and it is independent of the choice of θ; where hk is a map meaning k times iterations of h.
Before the proof of Lemma 2.1, similar to Proposition 2.1 in [41] or Lemma 2.1 in [52], we give the following lemma.
Lemma 5.1. Let h:R→R be an orientation-preserving homeomorphism satisfying (5.1) and n be an integer number. Then
(i) ρ(h)≤n if and only if minθ∈R(h(θ)−θ)≤2nπ,
(ii) ρ(h)≥n if and only if maxθ∈R(h(θ)−θ)≥2nπ.
Proof of Lemma 2.1. First, let h:R→R be the Poincaré map of Eq (2.2), that is
h:R→R,θ0↦θ(t0+2π;t0,θ0). |
By the uniqueness of the solutions of Eq (2.2) with respect to the initial values, the orientation-reserving lemma (Lemma 3.1) holds. Thus, h is an orientation-preserving homeomorphism. Then by (2.3), we have
h(θ0+2nπ)=h(θ0+nπ)+nπ=h(θ0)+2nπ,for allθ0∈R,n∈Z. |
Then, by (ⅱ) of Lemma 3.3, we have
ρ(L)<n⇒maxθ0∈R(h(θ0)−θ0)<2nπ; |
thus, △θ(t0,t′0)≤maxθ0∈R(θ(t0+2π;t0,θ0)−θ0)<2nπ. Therefore (ⅰ) holds.
In a similar way, by (ⅰ) of Lemma 3.3, we have
ρ(L)>n⇒minθ0∈R(h(θ0)−θ0)>2nπ; |
thus, △θ(t0,t0+2π)≥minθ0∈R(θ(t0+2π;t0,θ0)−θ0)>2nπ. Therefore (ⅱ) holds.
Consequently, (ⅲ) can be proved.
Next, similar to the proofs of Lemma 3.2 and Lemma 3.3 in [37], we prove Lemma 2.2 and Lemma 2.3.
Proof of Lemma 2.2. For each θ0∈R, let θ(t;θ0,L) be the unique solution of Eq (2.2) satisfying θ(t0;θ0,L)=θ0. Denote ϑ(t)=:θ2(t)−θ1(t), where θi(t)=θ(t;θ0,Li) for i=1,2. Note that ϑ(t0)=0. We have
dϑ(t)dt=ψ(t,θ2;L2)−ψ(t,θ1;L1)=(ψ(t,θ2;L2)−ψ(t,θ1;L2))+ψ(t,θ1;L2))−(ψ(t,θ1;L1)=:a(t)ϑ(t)+b(t), |
where a(t)=∂ψ∂θ(t,ξ;L2), ξ=ξ(t) is in a closed interval with θ1(t) and θ2(t) being its boundary points; also b(t)=⟨J(L1(t,(cosθ1,sinθ1))−L2(t,(cosθ1,sinθ1))),(cosθ1,sinθ1)⟩. Then,
ϑ(t)=∫tt0b(s)exp(∫tsa(τ)dτ)ds. |
It follows from (2.4) that b(t)≤0 for a.e. t∈[t0,t′0]. Thus ϑ(t)≤0 for all t∈[t0,t′0]; then we have
θ0−θ2(t)≥θ0−θ1(t),∀ t∈[t0,t′0], |
in the clockwise sense. Or in the counterclockwise sense,
−θ2(t)−(−θ0)≥−θ1(t)−(−θ0),∀ t∈[t0,t′0]. |
Thus we have
△θ2(t0,t)≥△θ1(t0,t),∀t∈[t0,t′0], |
which completes the proof of Lemma 2.2.
Proof of Lemma 2.3. Let ε>0 be fixed. For δ>0 small enough, suppose that (2.5) holds for all z∈R2 and a.e. t∈[t0,t′0]. According to Lemma 2.2, we have
△θ2(t0,t)≥△θδ(t0,t),∀t∈[t0,t′0]. |
Here, △θδ(t0,t) denotes the rotation angle difference of the solution z(t) of the planar system z′=L1(t,z)+δJz. Hence, to prove the result, it suffices to prove that there exists a δ=δ(ε)>0 such that
△θδ(t0,t)≥△θ1(t0,t)−ε,∀t∈[t0,t′0]. | (5.2) |
If not, for each n∈N, there would be tn∈[t0,t′0] such that
△θ1n(t0,tn)<△θ1(t0,tn)−ε. | (5.3) |
Without loss of generality, we suppose tn→τ∈[t0,t′0]. Since △θ1(⋅,⋅) is continuous on [t0,t′0]×R, passing to the upper limit on both sides of (5.3) gives
lim supn→∞△θ1n(t0,tn)≤△θ1(t0,τ)−ε. | (5.4) |
Let zn(t) and z(t) be, respectively, solutions of
z′=L1(t,z)+1nJzandz′=L1(t,z), |
with zn(t0)=vn and z(t0)=v. According to the theorem of continuous dependence of the solutions, it follows that zn(t)→z(t) uniformly on t∈[t0,t′0]. Hence,
△θ1n(t0,tn)=∫tnt0−⟨Jz′n,zn⟩|zn|2dt=∫tnt0−⟨JL1(t,zn),zn⟩−1n⟨zn,zn⟩|zn|2dt→∫τt0−⟨JL1(t,z),z⟩|z|2dt=△θ1(t0,τ), |
which is a contradiction with (5.4). Therefore, (2.6) is proved, and this completes the proof of Lemma 2.3.
In this paper, we have obtained the existence of 2π-periodic solutions for system (1.1) under non-resonance conditions with one-sided superlinear growth, it is formulated in an original way, with sufficiently general assumptions and overcoming the difficulties from the sign-varying conditions.
On the other hand, we have generalized the rotation number approach and developed a system methodology of "tracking" the angle difference of solutions of the system (1.1) on each small interval on the given side under sign-varying conditions.
The author thanks professor D. Qian for his key guidance, and also thanks the referee, who made professional comments and suggestions on this article. This work was supported by the National Natural Science Foundation of China (Grant No. 12101337).
The author declares that there is no conflict of interest.
[1] |
T. Takagi, M. Sugeno, Fuzzy identification of systems and its applications to modeling and control, IEEE T. Syst. Man Cy., 15 (1985), 116–132. http://dx.doi.org/10.1109/Tsmc.1985.6313399 doi: 10.1109/Tsmc.1985.6313399
![]() |
[2] |
Y. Liu, S. M. Lee, Stability and stabilization of Takagi-Sugeno fuzzy systems via sampled-data and state quantized controller, IEEE T. Fuzzy Syst., 24 (2016), 635–644. http://dx.doi.org/10.1109/Tfuzz.2015.2469099 doi: 10.1109/Tfuzz.2015.2469099
![]() |
[3] |
O. M. Kwon, M. J. Park, J. H. Park, S. M. Lee, Stability and stabilization of T-S fuzzy systems with time-varying delays via augmented Lyapunov-Krasovskii functionals, Inform. Sci., 372 (2016), 1–15. http://dx.doi.org/10.1016/j.ins.2016.08.026 doi: 10.1016/j.ins.2016.08.026
![]() |
[4] |
Y. J. Liu, J. H. Park, B. Z. Guo, Y. J. Shu, Further results on stabilization of chaotic systems based on fuzzy memory sampled-data control, IEEE T. Fuzzy Syst., 26 (2018), 1040–1045. http://dx.doi.org/10.1109/Tfuzz.2017.2686364 doi: 10.1109/Tfuzz.2017.2686364
![]() |
[5] |
L. K. Wang, H. K. Lam, A new approach to stability and stabilization analysis for continuous-time Takagi-Sugeno fuzzy systems with time delay, IEEE T. Fuzzy Syst., 26 (2018), 2460–2465. http://dx.doi.org/10.1109/Tfuzz.2017.2752723 doi: 10.1109/Tfuzz.2017.2752723
![]() |
[6] |
S. H. Lee, M. J. Park, O. M. Kwon, R. Sakthivel, A sampled-data control problem of neural-network-based systems using an improved free-matrix-based inequality, J. Franklin I., 356 (2019), 8344–8365. http://dx.doi.org/10.1016/j.jfranklin.2019.08.001 doi: 10.1016/j.jfranklin.2019.08.001
![]() |
[7] |
C. Briat, A. Seuret, A looped-functional approach for robust stability analysis of linear impulsive systems, Syst. Control Lett., 61 (2012), 980–988. http://dx.doi.org/10.1016/j.sysconle.2012.07.008 doi: 10.1016/j.sysconle.2012.07.008
![]() |
[8] |
L. Hetel, J. Daafouz, S. Tarbouriech, C. Prieur, Stabilization of linear impulsive systems through a nearly-periodic reset, Nonlinear Anal.-Hybri., 7 (2013), 4–15. http://dx.doi.org/10.1016/j.nahs.2012.06.001 doi: 10.1016/j.nahs.2012.06.001
![]() |
[9] |
E. Fridman, A. Seuret, J. P. Richard, Robust sampled-data stabilization of linear systems: An input delay approach, Automatica, 40 (2004), 1441–1446. http://dx.doi.org/10.1016/j.automatica.2004.03.003 doi: 10.1016/j.automatica.2004.03.003
![]() |
[10] |
P. Naghshtabrizi, J. P. Hespanha, A. R. Teel, Exponential stability of impulsive systems with application to uncertain sampled-data systems, Syst. Control Lett., 57 (2008), 378–385. http://dx.doi.org/10.1016/j.sysconle.2007.10.009 doi: 10.1016/j.sysconle.2007.10.009
![]() |
[11] |
E. Fridman, A refined input delay approach to sampled-data control, Automatica, 46 (2010), 421–427. http://dx.doi.org/10.1016/j.automatica.2009.11.017 doi: 10.1016/j.automatica.2009.11.017
![]() |
[12] |
K. Liu, E. Fridman, Wirtinger's inequality and Lyapunov-based sampled-data stabilization, Automatica, 48 (2012), 102–108. http://dx.doi.org/10.1016/j.automatica.2011.09.029 doi: 10.1016/j.automatica.2011.09.029
![]() |
[13] |
A. Seuret, A novel stability analysis of linear systems under asynchronous samplings, Automatica, 48 (2012), 177–182. http://dx.doi.org/10.1016/j.automatica.2011.09.033 doi: 10.1016/j.automatica.2011.09.033
![]() |
[14] |
A. Seuret, F. Gouaisbaut, Wirtinger-based integral inequality: Application to time-delay systems, Automatica, 49 (2013), 2860–2866. http://dx.doi.org/10.1016/j.automatica.2013.05.030 doi: 10.1016/j.automatica.2013.05.030
![]() |
[15] |
A. Seuret, C. Briat, Stability analysis of uncertain sampled-data systems with incremental delay using looped-functionals, Automatica, 55 (2015), 274–278. http://dx.doi.org/10.1016/j.automatica.2015.03.015 doi: 10.1016/j.automatica.2015.03.015
![]() |
[16] |
H. B. Zeng, J. H. Park, S. P. Xiao, Y. J. Liu, Further results on sampled-data control for master-slave synchronization of chaotic Lur'e systems with time delay, Nonlinear Dynam., 82 (2015), 851–863. http://dx.doi.org/10.1007/s11071-015-2199-6 doi: 10.1007/s11071-015-2199-6
![]() |
[17] |
T. H. Lee, J. H. Park, Stability analysis of sampled-data systems via free-matrix-based time-dependent discontinuous Lyapunov approach, IEEE T. Automat. Contr., 62 (2017), 3653–3657. http://dx.doi.org/10.1109/Tac.2017.2670786 doi: 10.1109/Tac.2017.2670786
![]() |
[18] |
H. B. Zeng, K. L. Teo, Y. He, A new looped-functional for stability analysis of sampled-data systems, Automatica, 82 (2017), 328–331. http://dx.doi.org/10.1016/j.automatica.2017.04.051 doi: 10.1016/j.automatica.2017.04.051
![]() |
[19] |
T. H. Lee, J. H. Park, Improved criteria for sampled-data synchronization of chaotic Lur'e systems using two new approaches, Nonlinear Anal.-Hybri., 24 (2017), 132–145. http://dx.doi.org/10.1016/j.nahs.2016.11.006 doi: 10.1016/j.nahs.2016.11.006
![]() |
[20] |
T. Li, R. T. Yuan, S. M. Fei, Z. T. Ding, Sampled-data synchronization of chaotic lur'e systems via an adaptive event-triggered approach, Inform. Sci., 462 (2018), 40–54. http://dx.doi.org/10.1016/j.ins.2018.06.012 doi: 10.1016/j.ins.2018.06.012
![]() |
[21] |
N. Gunasekaran, G. S. Zhai, Q. Yu, Sampled-data synchronization of delayed multi-agent networks and its application to coupled circuit, Neurocomputing, 413 (2020), 499–511. http://dx.doi.org/10.1016/j.neucom.2020.05.060 doi: 10.1016/j.neucom.2020.05.060
![]() |
[22] |
K. Tanaka, T. Hori, H. O. Wang, A multiple Lyapunov function approach to stabilization of fuzzy control systems, IEEE T. Fuzzy Syst., 11 (2003), 582–589. http://dx.doi.org/10.1109/Tfuzz.2003.814861 doi: 10.1109/Tfuzz.2003.814861
![]() |
[23] |
B. J. Rhee, S. Won, A new fuzzy Lyapunov function approach for a Takagi-Sugeno fuzzy control system design, Fuzzy Set. Syst., 157 (2006), 1211–1228. http://dx.doi.org/10.1016/j.fss.2005.12.020 doi: 10.1016/j.fss.2005.12.020
![]() |
[24] |
L. K. Wang, H. K. Lam, New stability criterion for continuous-time Takagi-Sugeno fuzzy systems with time-varying delay, IEEE T. Cybernetics, 49 (2019), 1551–1556. http://dx.doi.org/10.1109/Tcyb.2018.2801795 doi: 10.1109/Tcyb.2018.2801795
![]() |
[25] |
R. M. Zhang, D. Q. Zeng, J. H. Park, Y. J. Liu, S. M. Zhong, A new approach to stabilization of chaotic systems with nonfragile fuzzy proportional retarded sampled-data control, IEEE T. Cybernetics, 49 (2019), 3218–3229. http://dx.doi.org/10.1109/Tcyb.2018.2831782 doi: 10.1109/Tcyb.2018.2831782
![]() |
[26] |
J. R. Zhao, S. Y. Xu, J. H. Park, Improved criteria for the stabilization of T-S fuzzy systems with actuator failures via a sampled-data fuzzy controller, Fuzzy Set. Syst., 392 (2020), 154–169. http://dx.doi.org/10.1016/j.fss.2019.09.004 doi: 10.1016/j.fss.2019.09.004
![]() |
[27] |
L. Yang, J. Y. Zhang, C. Ge, W. Li, Z. W. Zhao, Stability and stabilization for uncertain fuzzy system with sampled-data control and state quantization, Appl. Intell., 51 (2021), 7469–7483. http://dx.doi.org/10.1007/s10489-021-02206-8 doi: 10.1007/s10489-021-02206-8
![]() |
[28] |
Y. H. Zhang, H. Y. Li, J. Sun, W. He, Cooperative adaptive event-triggered control for multiagent systems with actuator failures, IEEE T. Syst. Man Cy., 49 (2019), 1759–1768. http://dx.doi.org/10.1109/Tsmc.2018.2883907 doi: 10.1109/Tsmc.2018.2883907
![]() |
[29] |
Y. M. Li, J. X. Zhang, W. Liu, S. C. Tong, Observer-based adaptive optimized control for stochastic nonlinear systems with input and state constraints, IEEE T. Neur. Net. Lear., 33 (2022), 7791–7805. http://dx.doi.org/10.1109/Tnnls.2021.3087796 doi: 10.1109/Tnnls.2021.3087796
![]() |
[30] |
L. K. Wang, H. K. Lam, J. H. Gu, Stability and stabilization for fuzzy systems with time delay by applying polynomial membership function and iteration algorithm, IEEE T. Cybernetics, 52 (2022), 11604–11613. http://dx.doi.org/10.1109/Tcyb.2021.3072797 doi: 10.1109/Tcyb.2021.3072797
![]() |
[31] |
H. B. Zeng, K. L. Teo, Y. He, W. Wang, Sampled-data stabilization of chaotic systems based on a T-S fuzzy model, Inform. Sci., 483 (2019), 262–272. http://dx.doi.org/10.1016/j.ins.2019.01.046 doi: 10.1016/j.ins.2019.01.046
![]() |
[32] |
P. Y. Tang, Y. C. Ma, Non-fragile sampled-date dissipative analysis for uncertain T-S fuzzy time delay system with actuator saturation, ISA T., 106 (2020), 109–123. http://dx.doi.org/10.1016/j.isatra.2020.07.006 doi: 10.1016/j.isatra.2020.07.006
![]() |
[33] |
R. Sakthivel, R. Sakthivel, O. M. Kwon, P. Selvaraj, Disturbance rejection for singular semi-markov jump neural networks with input saturation, Appl. Math. Comput., 407 (2021), 126301. http://dx.doi.org/10.1016/j.amc.2021.126301 { doi: 10.1016/j.amc.2021.126301
![]() |
[34] |
H. K. Lam, F. H. F. Leung, Stabilization of chaotic systems using linear sampled-data controller, Int. J. Bifurcat. Chaos, 17 (2007), 2021–2031. https://dx.doi.org/10.1142/S0218127407018191 doi: 10.1142/S0218127407018191
![]() |
[35] |
X. L. Zhu, B. Chen, D. Yue, Y. Wang, An improved input delay approach to stabilization of fuzzy systems under variable sampling, IEEE T. Fuzzy Syst., 20 (2012), 330–341. https://dx.doi.org/10.1109/TFUZZ.2011.2174242 doi: 10.1109/TFUZZ.2011.2174242
![]() |
[36] |
Z. G. Wu, P. Shi, H. Su, J. Chu, Sampled-data fuzzy control of chaotic systems based on T-S fuzzy model, IEEE T. Fuzzy Syst., 22 (2014), 153–163. https://dx.doi.org/10.1109/TFUZZ.2013.2249520 doi: 10.1109/TFUZZ.2013.2249520
![]() |
[37] |
Z. P. Wang, H. N. Wu, On fuzzy sampled-data control of chaotic systems via a time-dependent Lyapunov functional approach, IEEE T. Cybernetics, 45 (2015), 819–829. https://dx.doi.org/10.1109/TCYB.2014.2336976 doi: 10.1109/TCYB.2014.2336976
![]() |
[38] |
T. H. Lee, J. H. Park, New methods of fuzzy sampled-data control for stabilization of chaotic systems, IEEE T. Syst. Man Cy. Syst., 48 (2018), 2026–2034. https://dx.doi.org/10.1109/TSMC.2017.2690803 doi: 10.1109/TSMC.2017.2690803
![]() |
[39] | Q. Inc, Inverted pendulum experiment-SRV02 RTOPEN user manual, Ontario, Canada, 2012. Available from: https://www.quanser.com/products/rotary-inverted-pendulum/. |
[40] |
K. J. Åström, K. Furuta, Swinging up a pendulum by energy control, Automatica, 36 (2000), 287–295. http://dx.doi.org/10.1016/S0005-1098(99)00140-5 doi: 10.1016/S0005-1098(99)00140-5
![]() |