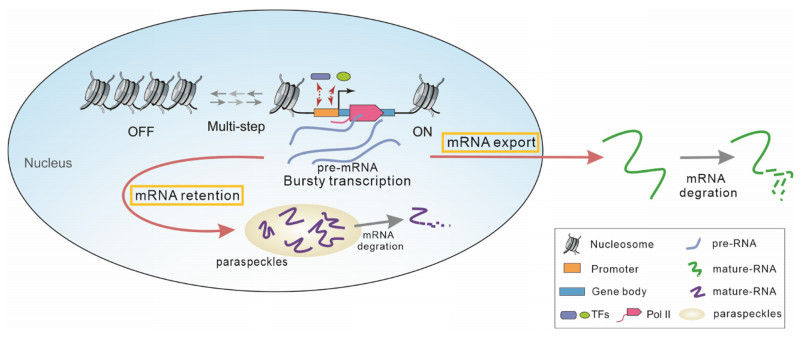
Long non-coding RNA (lncRNA) is considered to be a crucial regulator involved in various human biological processes, including the regulation of tumor immune checkpoint proteins. It has great potential as both a cancer biomolecular biomarker and therapeutic target. Nevertheless, conventional biological experimental techniques are both resource-intensive and laborious, making it essential to develop an accurate and efficient computational method to facilitate the discovery of potential links between lncRNAs and diseases. In this study, we proposed HRGCNLDA, a computational approach utilizing hierarchical refinement of graph convolutional neural networks for forecasting lncRNA-disease potential associations. This approach effectively addresses the over-smoothing problem that arises from stacking multiple layers of graph convolutional neural networks. Specifically, HRGCNLDA enhances the layer representation during message propagation and node updates, thereby amplifying the contribution of hidden layers that resemble the ego layer while reducing discrepancies. The results of the experiments showed that HRGCNLDA achieved the highest AUC-ROC (area under the receiver operating characteristic curve, AUC for short) and AUC-PR (area under the precision versus recall curve, AUPR for short) values compared to other methods. Finally, to further demonstrate the reliability and efficacy of our approach, we performed case studies on the case of three prevalent human diseases, namely, breast cancer, lung cancer and gastric cancer.
Citation: Li Peng, Yujie Yang, Cheng Yang, Zejun Li, Ngai Cheong. HRGCNLDA: Forecasting of lncRNA-disease association based on hierarchical refinement graph convolutional neural network[J]. Mathematical Biosciences and Engineering, 2024, 21(4): 4814-4834. doi: 10.3934/mbe.2024212
[1] | Gauhar Rahman, Iyad Suwan, Kottakkaran Sooppy Nisar, Thabet Abdeljawad, Muhammad Samraiz, Asad Ali . A basic study of a fractional integral operator with extended Mittag-Leffler kernel. AIMS Mathematics, 2021, 6(11): 12757-12770. doi: 10.3934/math.2021736 |
[2] | Ye Yue, Ghulam Farid, Ayșe Kübra Demirel, Waqas Nazeer, Yinghui Zhao . Hadamard and Fejér-Hadamard inequalities for generalized k-fractional integrals involving further extension of Mittag-Leffler function. AIMS Mathematics, 2022, 7(1): 681-703. doi: 10.3934/math.2022043 |
[3] | Bushra Kanwal, Saqib Hussain, Thabet Abdeljawad . On certain inclusion relations of functions with bounded rotations associated with Mittag-Leffler functions. AIMS Mathematics, 2022, 7(5): 7866-7887. doi: 10.3934/math.2022440 |
[4] | Rana Safdar Ali, Saba Batool, Shahid Mubeen, Asad Ali, Gauhar Rahman, Muhammad Samraiz, Kottakkaran Sooppy Nisar, Roshan Noor Mohamed . On generalized fractional integral operator associated with generalized Bessel-Maitland function. AIMS Mathematics, 2022, 7(2): 3027-3046. doi: 10.3934/math.2022167 |
[5] | Sabir Hussain, Rida Khaliq, Sobia Rafeeq, Azhar Ali, Jongsuk Ro . Some fractional integral inequalities involving extended Mittag-Leffler function with applications. AIMS Mathematics, 2024, 9(12): 35599-35625. doi: 10.3934/math.20241689 |
[6] | Anumanthappa Ganesh, Swaminathan Deepa, Dumitru Baleanu, Shyam Sundar Santra, Osama Moaaz, Vediyappan Govindan, Rifaqat Ali . Hyers-Ulam-Mittag-Leffler stability of fractional differential equations with two caputo derivative using fractional fourier transform. AIMS Mathematics, 2022, 7(2): 1791-1810. doi: 10.3934/math.2022103 |
[7] | Erhan Set, M. Emin Özdemir, Sevdenur Demirbaş . Chebyshev type inequalities involving extended generalized fractional integral operators. AIMS Mathematics, 2020, 5(4): 3573-3583. doi: 10.3934/math.2020232 |
[8] | Maryam Saddiqa, Ghulam Farid, Saleem Ullah, Chahn Yong Jung, Soo Hak Shim . On Bounds of fractional integral operators containing Mittag-Leffler functions for generalized exponentially convex functions. AIMS Mathematics, 2021, 6(6): 6454-6468. doi: 10.3934/math.2021379 |
[9] | Ghulam Farid, Maja Andrić, Maryam Saddiqa, Josip Pečarić, Chahn Yong Jung . Refinement and corrigendum of bounds of fractional integral operators containing Mittag-Leffler functions. AIMS Mathematics, 2020, 5(6): 7332-7349. doi: 10.3934/math.2020469 |
[10] | Hari M. Srivastava, Artion Kashuri, Pshtiwan Othman Mohammed, Abdullah M. Alsharif, Juan L. G. Guirao . New Chebyshev type inequalities via a general family of fractional integral operators with a modified Mittag-Leffler kernel. AIMS Mathematics, 2021, 6(10): 11167-11186. doi: 10.3934/math.2021648 |
Long non-coding RNA (lncRNA) is considered to be a crucial regulator involved in various human biological processes, including the regulation of tumor immune checkpoint proteins. It has great potential as both a cancer biomolecular biomarker and therapeutic target. Nevertheless, conventional biological experimental techniques are both resource-intensive and laborious, making it essential to develop an accurate and efficient computational method to facilitate the discovery of potential links between lncRNAs and diseases. In this study, we proposed HRGCNLDA, a computational approach utilizing hierarchical refinement of graph convolutional neural networks for forecasting lncRNA-disease potential associations. This approach effectively addresses the over-smoothing problem that arises from stacking multiple layers of graph convolutional neural networks. Specifically, HRGCNLDA enhances the layer representation during message propagation and node updates, thereby amplifying the contribution of hidden layers that resemble the ego layer while reducing discrepancies. The results of the experiments showed that HRGCNLDA achieved the highest AUC-ROC (area under the receiver operating characteristic curve, AUC for short) and AUC-PR (area under the precision versus recall curve, AUPR for short) values compared to other methods. Finally, to further demonstrate the reliability and efficacy of our approach, we performed case studies on the case of three prevalent human diseases, namely, breast cancer, lung cancer and gastric cancer.
Transcription is not only the most important but also the most complex step in gene expression. This double characteristic makes gene transcription receive lasting and extensive attention. With the development of measurement technologies (e.g., single-cell and single-molecule technologies), more molecular details of transcription have been experimentally uncovered. Nevertheless, some intermediate processes would not have been specified due to the complexity of gene transcription. Thus, traditional Markov models of gene transcription such as extensively studied ON-OFF models [1,2,3,4] would nor interpret experimental phenomena nor reveal the stochastic mechanisms of transcription. More biologically reasonable mathematical models need to be developed.
It is well known that gene transcription involves RNA nuclear retention (RNR) and nuclear RNA export (NRE). However, these two important processes were often ignored in previous studies [1,2,3,4,5,6,7,8]. Main reasons include that 1) NRE was before considered to be a transient process, compared to other processes occurring in transcription. It was reported that the NRE phase lasted about 20min on average and were gene-specific [9,10]; 2) For RNR, less than 30% poly (A+) RNA is nuclear-retained and undetectable in the cytoplasm [11]. Currently, more and more experimental evidences have indicated that RNR play a key role in biological processes, e.g., in S. cerevisiae cells, RNR may play a precautionary role during stress situations [12]; in plants, the RNR process of NLP7 can orchestrate the early response to nitrate [13]; and in the signaling pathway of antiviral innate immunity, the RNA helicase DDX46 acts like a negative regulator to induce nuclear retention of antiviral innate transcripts [14]. These experimental facts suggest that RNR or NRE cannot be neglected when one makes theoretical predictions of gene expression (including expression levels and noise).
Several works have revealed the respective roles of RNE and RNR in modulating stochastic gene expression [15,16,17,18,19]. One report exhibited that transcriptional burst attributed to promoter state switching could be substantially attenuated by the transport of mRNA from nucleus to cytoplasm [17]. Another report showed that slow pre-mRNA export from the nucleus could be an effective mechanism to attenuate protein variability arising from transcriptional burst [15]. In addition, RNR was also identified to buffer transcriptional burst in tissues and mammalian cells [16,18]. However, it has been experimentally confirmed that NRE and RNR can occur simultaneously in eukaryotes [20]. How these two dynamic processes cooperatively affect gene expression remains elusive and even unexplored.
As a matter of fact, gene activation, NRE and RNR are multistep processes. In general, transcription begins only when the chromatin template accumulates over time until the promoter becomes active [21,22], where the accumulating process is a multistep biochemical process in which some intermediate steps would not have been specified due to experimental technologies. A representative example is that inactive phases of the promoter involving the prolactin gene in a mammalian cell are differently distributed, showing strong memory [23]. Similarly, both the export of mRNAs generated in the nucleus to the cytoplasm through nuclear pores and the retention of mRNAs among nuclear speckles or paraspeckles are in general also multistep reaction processes [24]. All these multistep processes can create memories between reaction events, leading to non-Markov dynamics. Traditional Markov models are no longer suitable to the modeling of gene transcription with molecular memory, and non-Markov models can well model multistep processes involved in gene transcription [7].
In this paper, we introduce a non-Markov model of stochastic gene transcription. It considers not only RNR and NRE but also molecular memories created due to the multistep NRE process, due to RNR process, or due to the multistep activation process, thus including previous transcription models [1,2,3,4] as its special case. In order to solve this non-Markov model, we introduce effective transition rates, which explicitly decode the effect of molecular memory and by which we can transform the original non-Markov issue into an equivalent yet mathematical tractable Markov one. Based on this useful technique, we derive analytical results, which extend previous results [3,8,24,25] and provide insights into the role of molecular memory in affecting the nuclear and cytoplasmic mRNA mean and noise. The overall modeling and analysis provide a paradigm for studying more complex stochastic transcription processes.
Most previous studies [15,20,26] of gene transcription focused on the dynamics of NRE processes, where mature mRNAs were released to the cytoplasm with the help of nuclear pore complex (NPC) (Figure 1) [16,27]. The number of NPCs or the count of those assistant proteins that controlled NPC determined the speed of exporting mRNA. Measuring the exporting rate was often replaced by measuring the retention time in the nucleus, which however may vary with environmental changes [16,17,18,19]. Other previous studies of gene transcription [9,10,11,28,29] focused on the dynamics of transcription initiation and elongation, where elongation time (T) was measured by the length of a gene using the length of bases (L) and the average rate of elongation (v), i.e., T=L/Lvv. These studies assumed that all mature mRNAs were first exported to the cytoplasm and then translated into proteins. However, biological experiments indicated that there were a considerable part of mature mRNAs that stayed in the nucleus in a probabilistic manner and lasted a long period (Figure 1) [24].
Here, we consider two cases: one case where NRE dominates over RNR and the other case where RNR dominates over NRE. For both cases, the gene is assumed to have one "off" state (corresponding to the inactive form of the promoter) and one "on" state (corresponding to the active form), and the promoter is assumed to switch randomly between these two states. Only in "on" state, can the gene generate pre-mRNA. After an alternative splicing (AS) process or an alternative polyadenylation (APA) process, which occurs frequently at the 3' UTR, a portion of mature mRNAs (one type of transcripts) may be transported to the cytoplasm through NPC wherein they execute translation tasks. The rest mature mRNAs (another type of transcripts) may be remained in the nucleus for a long time, possibly assembling on the sub-cellular region (wherein they form nuclear speckles or paraspeckles [30,31,32]) with the assistance of proteins, some of which would have been unspecified. When the intracellular environment is changed, most of mature mRNAs will release to the cytoplasm in response to this change. In addition, most genes (especially in eukaryotic cells) are expressed in a bursty manner [1,2,3,4].
As pointed out in the introduction, gene transcription, NRE and RNR are all multistep reaction processes. In order to model these processes, we introduce a non-exponential waiting-time distribution for each intermediate reaction as done in ref. [7,33]. Since non-exponential waiting times lead to non-Markovian dynamics, the existing Markov theory cannot be used directly.
Assume that burst size in gene transcription follows a distribution described by prob{B=i}=αi, where each αi is a nonnegative constant and i=0,1,2,⋯. Let M1, M2 and M3 represent pre-mRNA, mature mRNA (one type of transcripts) transported to the cytoplasm and mature mRNA (another type of transcripts) retained in the nucleus respectively, and denote by m1, m2 and m3 their molecular numbers respectively. Thus, m=(m1,m2,m3)T represents the micro-state of the underlying system. Let W1(t;m), W2(t;m) and W3(t;m) be waiting-time distributions for the syntheses of pre-mRNAs, mature mRNA transported to the cytoplasm, and mature mRNA retained in the nucleus, respectively. Let W4(t;m) and W5(t;m) be waiting-time distributions for degradations of M2 and M3, respectively. To that end, the gene-expression model to be studied are described by the following five biochemical reactions labelled by Ri (1⩽i⩽5)
R1:DNAW1(t;m)→DNA+B×M1, R2:M1W2(t;m)→M2,R3: M1W3(t;m)→M3, R4:M2W4(t;m)→∅, R5:M3W5(t;m)→∅. | (1) |
Let ⟨B⟩ represent the mean burst size. Note that if α1=1 and αk=0 for all k≠1, this implies⟨B⟩≡1. In this case, the promoter is in the ON state all the time, and Eq (1) describes the constitutive gene expression. The other cases correspond to bursty gene expression. This is because B=0 implies that the promoter is in the OFF state (meaning that pre-mRNAs are not generated), whereas B>0 implies that the promoter is at the ON state (meaning that pre-mRNAs are generated).
For each reaction, there is a memory function [7,33]. Denote by Mi(t;m) memory function for reaction Ri (1⩽i⩽5). These memory functions can be expressed by waiting-time distributions in Eq (1). In fact, if we let ˜Mi(s;m) be the Laplace transform of memory function Mi(t;m), then ˜Mi(s;m) can be expressed as ˜Mi(s;m)=s˜φi(s;m)/s˜φi(s;m)[1−∑5i=1˜φi(s;m)][1−∑5i=1˜φi(s;m)], where ˜φi(s;m) is the Laplace transform of function φi(t;m) that can be expressed as φi(t;m)=Wi(t;m)∏k≠i[1−∫t0Wk(t′;m)dt′] (1⩽i⩽5) [7]. Let P(m;t) be the probability that the system is in state m at time t and ˜P(m;s) be the Laplace transform of P(m;t). With ˜Mi(s;m), we can show that the chemical master equation in the sense of Laplace transform takes the form
s˜P(m;s)−P(0;s)=(m1∑i=0αiE−i1−I)[˜M1(s;m)˜P(m;s)]+(E1E−12−I)[˜M2(s;m)˜P(m;s)] +(E1E−13−I)[˜M3(s;m)˜P(m;s)]+5∑j=4(Ej−I)[˜Mj(s;m)˜P(m;s)], | (2) |
where E is the step operator and E−1 is the inverse of E, and I is the unit operator.
Interestingly, we find that limit lims→0˜Mi(s;m) always exists, and if the limit function is denoted by Ki(m), then Ki(m) can be explicitly expressed by given waiting-time distributions Wk(t;m) (1⩽k⩽5), that is,
Ki(m)=∫ + ∞0Wi(t;m)[∏j≠i∫∞tWj(t′;m)dt′]dt∫ + ∞0[∏5j=1∫∞tWj(t′;m)dt′]dt,1⩽i⩽5. | (3) |
Note that s→0 corresponds to t→+∞ according to the definition of Laplace transform. However, t→+∞ corresponds to the steady-state case, which is our interest. We point out that function Ki(m), which will be called effective transition rate for reaction Ri, explicitly decodes the effect of molecular memory, where 1⩽i⩽5. More importantly, using these effective transition rates, we can construct a Markov reaction network with the same topology as the original non-Markov reaction network:
DNAK1(m)→DNA+B×M1, M1K2(m)→M2, M1K3(m)→M3, M2K4(m)→∅, M3K5(m)→∅. | (4) |
Moreover, two reaction networks have exactly the same chemical master equation at steady state:
(m1∑i=0αiE−i1−I)[K1(s;m)P(m)]+(E1E−12−I)[K2(m)P(m)] +(E1E−13−I)[K3(m)P(m)]+∑j∈{4,5}(Ej−I)[Kj(m)P(m)]=0, | (5) |
implying that both stationary behaviors are exactly the same (referring to Figure 2), where P(m) is the stationary probability density function corresponding to dynamic density probability function P(m;t).
In summary, by introducing an effective transition rate (Ki(m)) for each reaction Ri, given by Eq (3), a mathematically difficult non-Markov issue is transformed into a mathematical tractable Markov issue. This brings us convenience for theoretical analysis. In the following, we will focus on analysis of Eq (5).
Note that Gamma functions can well model multistep processes [34,35]. This is because the convolution of several exponential distributions is an Erlang distribution (a special case of Gamma distribution). Therefore, in order to model the effect of molecular memory on the mRNA expression, we assume that waiting-time distributions for gene activation, NRE and RNR processes are Gamma distributions: W1(t;m)=[Γ(L0)]−1tL0−1(k0)L0e−k0t, W2(t;m)=[Γ(Lc)]−1tLc−1(m1kc)Lce−m1kct andW3(t;m)=[Γ(Lr)]−1tLr−1(m1kr)Lre−m1krt, and that waiting-time distributions for the other processes are exponential ones, W4(t;m)=m2dce−m2dct, and W5(t;m)=m3dre−m3drt. Here Γ(⋅) is the common Gamma function, k0 is the mean transcription rate, kc and kr denote the mean synthesis rates for mRNAs in the nucleus and mRNAs in the cytoplasm respectively, dc and dr are the mean degradation rates of mRNA in the nucleus and mRNA in the cytoplasm respectively. Throughout this paper, L0, Lc and Lr are called memory indices since, e.g., L0=1 corresponds to the memoryless case whereas L0≠1 to the memory case.
Let k1 be the total synthesis rate of mRNAs in the cytoplasm, which is composed of two parts: one part is the rate at which pre-mRNA generates a transcript through the AS process or the APA process, and the other is the rate at which the mature mRNA is finally exported to the cytoplasm with the help of NPC. The rate of generating mature mRNAs, determined by the gene length, is generally fixed. In contrast, the rate of exporting mRNAs to the cytoplasm may change in a large range, depending on cellular environments. This is because some types of mRNAs are exported in a fast manner due to RNA binding proteins or linked splicing factors, and other types of mRNAs are exported in a slow manner and the corresponding genes are most intron-containing ones [19]. Thus, we can use k1 to characterize the export rate indirectly. Similarly, if we let k2 be the synthesis rate of mRNAs in the nucleus, then it also includes two parts: one part is the rate of pre-mRNA produced through an AS or APA process, and the other is the rate at which transcripts are transported to some sub-cellular regions (e.g., nuclear speckles or paraspeckles) under the assistance of some proteins. Here, we assume that k2 changes a little so that the involved processes are simplified. Owing to AS or APA processes, the lengths of mature mRNAs of the two kinds can be significantly different. Usually, the rate k1 is faster than the rate k2. The retention and export of transcripts are random, we introduce another parameter pr, called remaining probability throughout this paper, to characterize this randomness. Then, the practical export rate and the practical retention rate should be kc=k1(1−pr) and kr=k2pr respectively, where pr∈(0,1).
Based on the experimental data from Halpern's group [26] that measured the whole genome-wide catalogue of nuclear and cytoplasmic mRNA from MIN6 cells, we know that most genes (~70%) has more cytoplasmic transcripts than nuclear transcripts. Thus, we can get an approximate formula for remaining probability pr: pr=Nn/Nn(Nn+Nc)(Nn+Nc) where Nn is the number of transcripts in the nucleus and Nc the number of transcripts in the cytoplasm. By considering gene INS1 for which the value of ratio Nc/NcNnNn is the maximal (13.2±4.6), we can know that the value of pr is about 5%. In that paper, the authors also mentioned that about 30% of the genes in MIN6 cells have more transcripts in the nucleus than in the cytoplasm. By considering gene ChREBP for which the value of ratio cytoplasm/nucleus is about 0.05, we can know that the value of pr is about 95%. Therefore, the range of remaining probability (pr) in the whole genome is about 5~95%. It is reasonable that the 50% value of pr is set as a threshold. For convenience, we categorize models of eukaryotic gene expression into two classes: one class where the RNE process is dominant and the other class where the RNR process is dominant. For the former, pr<0.5 holds, implying that most mRNAs are exported to the cytoplasm through nuclear pores, whereas for the latter, pr>0.5 holds, implying that most mRNAs retain in the nucleus.
In the following analysis, memory indices Li (i=0,c,r), and remaining probability pr will be taken as key parameters while the other parameter values will be kept fixed. Without loss of generality, we assume that two degradation rates for mRNAs in the nucleus and cytoplasm are equal and denote by d the common degradation rate, i.e., dc=dr=d.
First, if we let xi represent the concentration of reactive species Mi, i.e., xi=limmi→∞Ω→∞mi/miΩΩ (i=1,2,3) where Ω represents the volume of the system, then the rate equations corresponding to the constructed-above Markov reaction network can be expressed as
dxdt=SK(x), | (6) |
where x=(x1,x2,x3)T is a column vector, S=(Sij)3×5=(⟨B⟩−1−100010−100010−1) is a matrix, and K(x)=(K1(x),K2(x),K3(x),K4(x),K5(x))T is a column vector of effective transition rates. The stead states or equilibriums of the system described by Eq (6), denote by xS, are determined by solving the algebraic equation group, i.e., SK(xS)=0.
Second, if denoting by ⟨X⟩ the mean of random variable X and taking approximation ⟨Mi⟩≈xSi (i=1,2,3), then we can derive the following matrix equation (see Appendix A):
ASΣS+ΣSATS+ΩDS=0, | (7) |
where two square matrices AS=(Aij)3×3 and DS=(Dij)3×3 evaluated at steady state are known, and covariance matrix ΣS=(σij) with σij=⟨(Mi−⟨Mi⟩)(Mj−⟨Mj⟩)⟩ is unknown. Note that diagonal elements σ22 and σ33 represent variances for random variables M2 (corresponding to mRNA in the cytoplasm) and M3 (corresponding to mRNA in the nucleus), which are our interest. In addition, we can also derive formulae similar to Eq (3) in the case of continuous variables.
In order to show the explicit effect of molecular memory on the mRNA expression in different biological processes, we consider two special cases: 1) The process of gene activation with memory and other processes are memoryless. 2) The process of nuclear RNA export with memory and other processes are memoryless. In other cases, there are in general no analytical results.
Case 1 L0≠1, Lc=1 and Lr=1. In this case, the five effect transition rates become: K1(x)=(kcx1+krx1+dx2+dx3)(k0)L0(kcx1+krx1+dx2+dx3+k0)L0−(k0)L0, K2(x)=kcx1, K3(x)=krx1, K4(x)=dx2, and K5(x)=dx3. Note that in the case of continuous variables, the corresponding effect transition rates Ki(x) (1⩽i⩽5) have the same expressions except for variable notations.
We can show that the means of mRNAs in the nucleus and in the cytoplasm are given respectively by (see Appendix B)
⟨M2⟩=˜k0˜kc,⟨M3⟩=˜k0˜kr, | (8) |
where ˜k0=12[(1+2⟨B⟩)1/1L0L0−1]k0kc+kr>0 with ⟨B⟩ being the expectation of burst size B (random variable), ˜kc=kcd and ˜kr=krd. Apparently, ⟨Mi⟩ (i=2,3) is a monotonically decreasing function of memory index L0, implying that molecular memory always reduces the mRNA expression level in the nucleus and cytoplasm. In addition, by noting kc=k1(1−pr) and kr=k2pr, we can know that if k2/k2k1k1 is fixed, then ⟨M3⟩ (i.e., the mean of mRNAs in the nucleus) is a monotonically increasing function of remaining probability pr whereas ⟨M2⟩ (i.e., the mean of mRNAs in the cytoplasm) is a monotonically decreasing function of pr. In addition, ⟨M3⟩ is a monotonically decreasing function of ρ=kc/kckrkr whereas ⟨M2⟩ is a monotonically increasing function of ρ. These results are in agreement with intuition.
Interestingly, we find that σ22 and σ33, the variances for mRNAs in the nucleus and in the cytoplasm resepctively, have the following relationship (see Appendix B)
σ22=(˜kr˜kc)2σ33+˜k0(˜kc+˜kr)˜kc˜kr, | (9) |
indicating that the mRNA variance in the cytoplasm, σ22, is larger than that in the nucleus, σ33.
From the viewpoint of experiments, the cytoplasmic mRNAs are easy to measure whereas the cytoplasmic mRNAs are difficult to measure. Therefore, we are interested in the cytoplasmic mRNA expression (including the level and noise). By complex calculations, we can further show that the cytoplasmic mRNA variance is given by
σ22=˜k0˜kc˜k3r˜k3c+˜k3r{2+˜kc˜kr+122˜b+[γ−(1+˜b)2](˜kc+˜kr)1+(1+˜b)(1+2˜b)(˜kc+˜kr)}. | (10) |
where ˜b=14⟨B⟩[L0+2(L0−1)⟨B⟩−L0(1+2⟨B⟩)(L0−1)/(L0−1)L0L0]>0 and γ=⟨B2⟩+⟨B⟩⟨B⟩ with ⟨B2⟩ being the second-order raw moment of burst size B. Furthermore, if we define the noise intensity as the ratio of the variance over the squared mean, then the noise intensity for the cytoplasmic mRNA, denoted by ηc, can be analytically expressed as
ηc=1˜k0˜kc˜k3r˜k3c+˜k3r{2+˜kc˜kr+122˜b+[γ−(1+˜b)2](˜kc+˜kr)1+(1+˜b)(1+2˜b)(˜kc+˜kr)}. | (11) |
Note that if L0=1, which correspond to the Markov case, then ˜k0=k0⟨B⟩kc+kr and ˜b=0. Thus, the cytoplasmic mRNA noise in the Markov case, denoted by ηc|L0=1, is given by
ηc|L0=1=1˜k0˜kc˜k3r˜k3c+˜k3r(˜kc+2˜kr˜kr+γ2˜kc+˜kr1+˜kc+˜kr). | (12) |
Therefore, the ratio of the noise in non-Markov (L0≠1) case over that in the Markov (L0=1) case
ηcηc|L0=1={˜kc+2˜kr˜kr+122˜b+[γ−(1+˜b)2](˜kc+˜kr)1+(1+˜b)(1+2˜b)(˜kc+˜kr)}(˜kc+2˜kr˜kr+γ2˜kc+˜kr1+˜kc+˜kr)−1, | (13) |
which may be more than the unit but may also be less than the unit, depending on the size of remaining probability. However, if L0 is large enough (e.g., L0>2), then the ratio in Eq (13) is always larger than the unit, implying that molecular memory amplifies the cytoplasmic mRNA noise.
Case 2 L0=1, Lc≠1 and Lr=1. In this case, five effect transition rates reduce to K1(x)=k0, K2(x)=(k0+krx1+dx2+dx3)(kcx1)Lc(k0+kcx1+krx1+dx2+dx3)Lc−(kcx1)Lc, K3(x)=kcx1, K4(x)=dx2, and K5(x)=dx3. It seems to us there are no analytical results as in Case 1. However, if pr=0 (i.e., if we do not consider nuclear retention), then we can show that the steady state is given by x1=k0k1ω,x2=k0⟨B⟩d,x3=0, where ω=(1+⟨B⟩)⟨B⟩1/1LcLc(1+2⟨B⟩)1/1LcLc−⟨B⟩1/1LcLc is a factor depending on both transcriptional burst and molecular memory. Moreover, the mRNA noise in the cytoplasm is given by (see Appendix C for derivation)
ηc=d(1+⟨B⟩)2k0⟨B⟩2dω(1+ω+⟨B⟩)+γLck1⟨B⟩k1(1+2⟨B⟩)dω(1+ω+⟨B⟩)+Lc⟨B⟩(1+2⟨B⟩)[dω+k1(1+⟨B⟩)]. | (15) |
In order to see the contribution of molecular memory to the cytoplasmic mRNA noise, we calculate the ratio of the noise in non-Markov (Lc≠1) case over that in the Markov (Lc=1) case
ηcηc|Lc=1=d+k12d+γk1(1+⟨B⟩)[2dω(1+ω+⟨B⟩)+γLck1⟨B⟩k1(1+2⟨B⟩)]dω(1+ω+⟨B⟩)+Lc⟨B⟩(1+2⟨B⟩)[dω+k1(1+⟨B⟩)], | (16) |
which is in general larger than the unit for large Lc>1 (corresponding to strong memory), indicating that molecular memory in general enlarges the cytoplasmic mRNA noise.
Here we numerically investigate the effect of molecular memory (Lc) from nuclear RNA export on the cytoplasm mRNA (M2) in the cases that the other model parameter values are fixed. Numerical results are demonstrated in Figure 3. From Figure 3(a), we observe that the mean level of the cytoplasm mRNA is a monotonically decreasing function of Lc, independent of the value choice of remaining probability (pr) (even though we only show the two cases of pr values). This is in agreement with our intuition since a more reaction step for mRNA synthesis inevitably leads to less mRNA molecules. On the other hand, we observe from Figure 3(b) that molecular memory reduces the cytoplasm mRNA noise (ηc) for smaller values of Lc but enlarges ηc for larger values of Lc, implying that there is an optimal Lc such that ηc arrives at the minimum. We emphasize that the dependences shown in Figure 3(a), (b) are qualitative since they are independent of the value choice of remaining probability.
Importantly, Figure 3 indicates that the results obtained by the linear noise approximation (solid lines) are well in agreement with the results obtained by the Gillespie algorithm [36]. Therefore, the linear noise approximation can be used in fast evaluation of the expression noise, and in the following, we will focus on results obtained by the linear noise approximation. In addition, we point out that most results obtained here and thereafter are qualitative since they are independent of the choice of parameter values. However, to demonstrate interesting phenomena clearly, we will choose special values of some model parameters.
Here we focus on numerically investigating joint effects of molecular memory (Lc) and remaining probability (pr) on the cytoplasm mRNA (M2). Figure 4(a) demonstrates effects of pr on the M2 noise for three values of Lc. We observe that with the increase of remaining probability, the cytoplasmic mRNA noise (ηc) first decreases and then increases, implying that there is a critical pr such that ηc arrives at the minimum (referring to empty circles in Figure 4(a)) or that remaining probability can minimize the cytoplasmic mRNA noise. Moreover, this minimum is independent of the values of memory index Lc. In addition, we find that the minimal ηc first increases and then decreases with increasing Lc (the inset of Figure 4(a)). In other words, noise of cRNA can derive a optimal value with the decrease of remaining probability and the increase of memory index. Figure 4(b) shows the dependences of ηc on Lc for three different values of remaining probability. We find that molecular memory can also make cytoplasmic mRNA noise reach the minimum (referring to empty circles in Figure 4(b)), and this minimal noise is monotonically increasing function of pr.
Here we focus on numerically analyzing joint effects of memory index L0 and remaining probability pr on the cytoplasm mRNA (M2). Figure 5(a) demonstrates effects of pr on the M2 noise for two representative values of L0 (note: L0=1 corresponds to the memoryless case whereas L0=2 corresponds to the memory case). We observe that with the increase of remaining probability, the cytoplasmic mRNA noise (ηc) first decreases and then increases, implying that there is a critical pr such that ηc reaches the minimum (referring to empty circles in Figure 5(a)) or that remaining probability can minimize the cytoplasmic mRNA noise. Moreover, this minimum (referring to empty circles) is a monotonically increasing function of memory index L0.
Figure 5(b) demonstrates that the cytoplasmic mRNA noise (ηc) is always a monotonically increasing function of memory index L0, independent of remaining probability. In addition, we observe that ηc is a monotonically increasing function of remaining probability (this can be seen by comparing three lines).
Here we consider the case that RNR is a multistep process, i.e., Lr≠1. Numerical results are demonstrated in Figure 6. We observe from Figure 6(a) that except for the case of Lr=1, which corresponds to the Markov process and for which the cytoplasmic mRNA noise (ηc) is a monotonically increasing function of remaining probability (pr), the dependences of ηc on pr are not monotonic in the cases of Lr≠1 (corresponding to non-Markov processes) but there is a threshold of pr such that ηc reaches the minimum (referring to empty circles), similarly to the case of Figure 5(a). Moreover, this minimal noise is a monotonically decreasing function of memory index Lr (referring to the inset of Figure 6(a)) but the monotonicity is opposite to that in the case of Figure 5(a).
Figure 6(b) shows how the cytoplasmic mRNA noise (ηc) depends on memory index Lr for two different values of remaining probability. Interestingly, we observe that there is an optimal value of Lr such that the cytoplasmic mRNA noise reaches the minimum. Moreover, the minimal ηc is a monotonically decreasing function of remaining probability (pr), referring to the inset in the bottom right-hand corner.
Gene transcription in eukaryotes involve many molecular processes, some of which are well known and others are little known and even unknown [37,38]. In this paper, we have introduced a non-Markov model of stochastic transcription, which simultaneously considers RNA nuclear retention and nuclear RNA export processes and in which we have used non-exponential waiting-time distributions (e.g., Gamma distributions) to model some unknown or unspecified molecular processes involved in, e.g., the synthesis of pre-mRNA and the export of mRNAs generated in the nucleus to the cytoplasm and the retention of mRNA in the nucleus. Since non-exponential waiting times can lead to non-Markov kinetics, we have introduced effective transition rates for the reactions underlying transcription to transform a mathematically difficult issue to a mathematically tractable one. As a result, we have derived the analytical expressions of mRNA means and noise in the nucleus and cytoplasm, which revealed the importance of molecular memory in controlling or fine-tuning the expressions of two kinds of mRNA. Our modeling and analysis provided a heuristic framework for studying more complex gene transcription processes.
Our model considered main events occurring in gene transcription such as bursty expression (burst size follows a general distribution), alternative splicing (by which two kinds of transcripts are generated), RNR (a part of RNA molecules that are kept in the nucleus) and RNE (another part of RNA molecules that are exported to the cytoplasm). Some popular experimental technologies such as single-cell sequence data [39], single-molecule fluorescence in-situ hybridization (FISH) [40] and electron micrographs (EM) of fixed cells [41] have indicated that RNR and NRE are two complex biochemical processes, each involving regulation by a large number of proteins or complexes [42]. In particular, the mRNAs exported to the cytoplasm involve the structure of nuclear pore complex (NPC) [43]. A number of challenging questions still remain unsolved, e.g., how do RNR and NRE cooperatively regulate the expressions of nuclear and cytoplasmic mRNAs? Why are these two dynamical processes necessary for the whole gene-expression processes when the cells survive in complex environments? And what advantages do they have in contrast to a single NRE process?
Despite simple, our model can not only reproduce results for pre-mRNA (nascent mRNA) means at steady state in previous studies but also give results in agreement with experimental data on the mRNA Fano factors (define as the ratio of variance over mean) of some genes. However, we point out that some results of Fano factors obtained using our model is not always in agreement with the experimental data, e.g., for five genes, RBP3, TAF5, TAF6, TAF12 and KAP104, results obtained by our model seem not in agreement with experimental data but results obtained by a previous theoretical model [44] seems better (data are not shown). In addition, for the PRB8 gene, results on Fano factor, obtained by our model and the previous model, are poorly in agreement with experimental data (data are not shown). This indicates that constructing a theoretical model for the whole transcription process still needs more work.
In spite of differences, our results are wholly in agreement with some experimental data or observations. First, the qualitative result that RNR always reduces the nuclear pre-mRNA noise and always amplifies the cytoplasmic mRNA noise is in agreement with some experimental observations [28,42,45] and also with intuition since the retention naturally increases the mean number of the nuclear pre-mRNAs but decreases the mean number of the cytoplasmic mRNAs. Second, we compare our theoretical predictions with experimental results [28,45]. Specifically, we use previously published experimental data for two yeast genes, RBP2 and MDN1 [28,45] to calculate the cytoplasmic mRNA Fano factors. Parameter k1 is set as k1≈0.29±0.013/min, which is based on experimental data [28] and the degradation rates of the cytoplasmic mRNAs for RBP2 and MDN1 are set according to dc=ln2/ln2t1/2t1/2, where t1/2 is an experimental mRNA half-life. Then, we can find that the results on the Fano factors of genes RBP2 and MDN1 are well in agreement with the experimental data [45].
At the whole genome scale, about 70% mRNAs in the nucleus are transported to the cytoplasm whereas about 30% mRNAs are retained in the nucleus [26]. This fact implies that the changing range of remaining probability is moderate or small. In addition, the nuclear export rate of a different gene is in general different. If this rate is not too large, then following the increase of remaining probability, the increase in the cytoplasmic mRNA noise is inevitable. This result indirectly interprets the reason why the noise at the protein level is quite large as shown in previous studies of gene expression [46].
Finally, for some genes, the relative changing ranges of remaining probability and nuclear export rate may be large at the transcription level. In this case, it is in theory sufficient that adjusting one of nuclear export rate and remaining probability can fine-tune the cytoplasmic mRNA noise if the mean burst size is fixed, but differences would exist between theoretical and experimental results since NRE and RNR occur simultaneously in gene expression and are functionally cooperative. In addition, since biological regulation may be different from the theoretical assumption made here, the nuclear or cytoplasmic mRNA noise predicted in theory may be overestimated.
This work was partially supported by National Nature Science Foundation of China (11931019), and Key-Area Research and Development Program of Guangzhou (202007030004).
All authors declare no conflicts of interest in this paper.
First, the chemical master equation for the constructed Markov reaction network reads
∂P(m;t)∂t=(m1∑i=0αiE−i1−I)[K1(m)P(m;t)]+(E1E−12−I)[K2(m)P(m;t)] +(E1E−13−I)[K3(m)P(m;t)]+∑j∈{4,5}{(Ej−I)[Kj(m)P(m;t)]}, | (A1) |
Second, the stead state or equilibrium of the system described by Eq (6) in the main text, denoted by xS=(xS1,xS2,xS3)T, can be obtained by solving the algebraic equation group
⟨B⟩K1(xS)−K2(xS)−K3(xS)=0K2(xS)−K4(xS)=0K3(xS)−K5(xS)=0 | (A2) |
Then, we perform the Ω-expansions [47] to derive a Lyapunov matrix equation for covariance matrix between Mi and Mj with i,j=1,2,3, i.e., for matrix Σ=(⟨(Mi−⟨Mi⟩)T(Mj−⟨Mj⟩)⟩)≡(σij). Note that
Ki(n)=Ki(x+Ω−1/−122z)=Ki(x)+Ω−1/−122∑j=p,c,rzj∂Ki(x)∂xj+o(Ω−1),i=1,2,3 | (A3a) |
m1∑i=0αiE−i1−I=−Ω−1/−122m1∑i=0iαi∂∂z1+12Ω−1m1∑i=0i2αi∂2∂z21+o(Ω−3/−322), | (A3b) |
E1E−12−I=−Ω−1/−122(∂∂z2−∂∂z1)+12Ω−1(∂2∂z21+∂2∂z22−2∂2∂z1∂z2)+o(Ω−3/−322), | (A3c) |
E1E−13−I=−Ω−1/−122(∂∂z3−∂∂z1)+12Ω−1(∂2∂z21+∂2∂z23−2∂2∂z1∂z3)+o(Ω−3/−322), | (A3d) |
Ej−I=−Ω−1/−122∂∂zj+12Ω−1∂2∂z2j+o(Ω−3/−322),j=2,3. | (A3e) |
Hereafter o(y) represents the infinitestmal quantity of the same order as y→0. We denote by Π(z;t) the probability density function for new random variable z. Then, the relationship between variables P(m;t) and Π(z;t) is
∂P(m;t)∂t=∂Π(z;t)∂t−Ω1/122∑i=1,2,3dxidt∂Π(z;t)∂zi. | (A4) |
By substituting Eqs (A3) and (A4) into Eq (A1) and comparing the coefficients of Ω1/122, we have
∑i=1,2,3dxidt∂Π(z;t)∂zi=⟨B⟩K1(x)∂Π(z;t)∂z1+K2(x)(∂Π(z;t)∂z2−∂Π(z;t)∂z1) +K3(x)(∂Π(z;t)∂z3−∂Π(z;t)∂z1)+∑j∈{2,3}Kj∂Π(z;t)∂zj, | (A5) |
which naturally holds due to Eq (6) in the main text, where ⟨B⟩=m1∑i=0iαi is the mean burst size. Comparing the coefficients of Ω0, we have
∂Π(z;t)∂t=−⟨B⟩∑j=1,2,3∂K1(x)∂xj∂[zjΠ(z;t)]∂z1−∑j=1,2,3∂K2(x)∂xj(∂[zjΠ(z;t)]∂z2−∂[zjΠ(z;t)]∂z1)−∑j=1,2,3∂K3(x)∂xj(∂[zjΠ(z;t)]∂z3−∂[zjΠ(z;t)]∂z1)−∑j∈{2,3}∑k=1,2,3∂Kj(x)∂xk∂[zkΠ(z;t)]∂zj+12⟨B2⟩K1(x)∂2Π(z;t)∂z21+12K2(x)(∂2Π(z;t)∂z21+∂2Π(z;t)∂z22−2∂2Π(z;t)∂z1∂z2)+12K3(x)(∂2Π(z;t)∂z21+∂2Π(z;t)∂z23−2∂2Π(z;t)∂z1∂z3)+12∑j∈{2,3}Kj∂2Π(z;t)∂z2j | (A6) |
where ⟨B2⟩=m1∑i=0i2αi is the second moment of burst size. Since Ki(x) is independent of z, Eq (A6) can be rewritten as
∂Π(z;t)∂t=−∑i,j∈{1,2,3}Aij∂[zjΠ(z;t)]∂zi+12∑i,j∈{1,2,3}Dij∂2Π(z;t)∂zi∂zj, | (A7) |
where the elements of matrix A=(Aij) take the form
A=(Aij)=(⟨B⟩∂K1∂x1−∂K2∂x1−∂K3∂x1⟨B⟩∂K1∂x2−∂K2∂x2−∂K3∂x2⟨B⟩∂K1∂x3−∂K2∂x3−∂K3∂x3∂K2∂x1−∂K4∂x1∂K2∂x2−∂K4∂x2∂K2∂x3−∂K4∂x3∂K3∂x1−∂K5∂x1∂K3∂x2−∂K5∂x2∂K3∂x3−∂K5∂x3) | (A8a) |
and matrix D=(Dij) takes the form
D=(⟨B2⟩K1+K2+K3−K2−K3−K2K2+K40−K30K3+K5). | (A8b) |
If we consider the stationary equation of Eq (A7), denote by AS and DS the corresponding matrices.
Third, the steady-state Fokker Planck equation allows a solution of the following form
Π(z)=1√(2π)3det(ΣS)exp(−12zTΣ−1Sz). | (A9) |
Here, matrix ΣS=(⟨(M−xS)T(M−xS)⟩)≡(σij) (covariance matrix) is determined by solving the following Lyapunov matrix equation
ASΣS+ΣSATS+DS=0. | (A10) |
Note that the diagonal elements of matrix ΣS are just the variances of the state variables, and the vector of the mean concentrations of the reactive species is given approximately by ⟨M⟩≈xS. Eq (A10) is an extension of the linear noise approximation in the Markov case [48].
In this case, we can show that effective transition rates are given by K1(x)=(x1kc+x1kr+x2d+x3d)(k0)L0(x1kc+x1kr+x2d+x3d+k0)L0−(k0)L0, K2(x)=x1kc, K3(x)=x1kr, K4(x)=x2d, and K5(x)=x3d, where x=(x1,x2,x3)T. Thus, accoridng to Eq (A2), we know that the steady state is given by
xs1=ak0kc+kr,xs2=kcdxs1,xs3=krdxs1, | (B1) |
where a = \frac{{{{\left({1 + 2\left\langle B \right\rangle } \right)}^{{1 \mathord{\left/ {\vphantom {1 {{L_0}}}} \right. } {{L_0}}}}} - 1}}{2} . Note that
\frac{{\partial {K_1}\left( \boldsymbol{x} \right)}}{{\partial {x_1}}} = \frac{{\left( {{k_c} + {k_r}} \right){{\left( {{k_0}} \right)}^{{L_0}}}}}{{{{\left[ {{k_0}\left( {1 + 2a} \right)} \right]}^{{L_0}}} - {{\left( {{k_0}} \right)}^{{L_0}}}}} - \frac{{2a{k_0}{L_0}\left( {{k_c} + {k_r}} \right){{\left( {{k_0}} \right)}^{{L_0}}}{{\left[ {{k_0}\left( {1 + 2a} \right)} \right]}^{{L_0} - 1}}}}{{{{\left\{ {{{\left[ {{k_0}\left( {1 + 2a} \right)} \right]}^{{L_0}}} - {{\left( {{k_0}} \right)}^{{L_0}}}} \right\}}^2}}} |
Therefore,
{\left. {\frac{{\partial {K_1}\left( \boldsymbol{x} \right)}}{{\partial {x_1}}}} \right|_{\boldsymbol{x} = {\boldsymbol{x}^S}}} = \frac{{\partial {K_1}\left( {{\boldsymbol{x}^S}} \right)}}{{\partial {x_1}}} = \left( {{k_c} + {k_r}} \right)\frac{{2\left\langle B \right\rangle - {L_0}\left[ {\left( {1 + 2\left\langle B \right\rangle } \right) - {{\left( {1 + 2\left\langle B \right\rangle } \right)}^{{{\left( {{L_0} - 1} \right)} \mathord{\left/ {\vphantom {{\left( {{L_0} - 1} \right)} {{L_0}}}} \right. } {{L_0}}}}}} \right]}}{{4{{\left\langle B \right\rangle }^2}}} . | (B2a) |
Completely similarly, we have
\frac{{\partial {K_1}\left( \boldsymbol{x} \right)}}{{\partial {x_2}}} = \frac{{\partial {K_1}\left( \boldsymbol{x} \right)}}{{\partial {x_3}}} = d\frac{{2\left\langle B \right\rangle - {L_0}\left[ {\left( {1 + 2\left\langle B \right\rangle } \right) - {{\left( {1 + 2\left\langle B \right\rangle } \right)}^{{{\left( {{L_0} - 1} \right)} \mathord{\left/ {\vphantom {{\left( {{L_0} - 1} \right)} {{L_0}}}} \right. } {{L_0}}}}}} \; \right]}}{{4{{\left\langle B \right\rangle }^2}}} . | (B2b) |
Thus, matrix {{\bf{{ A}}}_{\text{S}}} reduces to
{\bf{{ A}}} = \left( {{A_{ij}}} \right) = \left( {\begin{array}{*{20}{c}} {\left( {b\left\langle B \right\rangle - 1} \right)\left( {{k_c} + {k_r}} \right)}&{bd\left\langle B \right\rangle }&{bd\left\langle B \right\rangle } \\ {{k_c}}&{ - d}&0 \\ {{k_r}}&0&{ - d} \end{array}} \right) , | (B3) |
where b = \frac{{ - 1}}{{4{{\left\langle B \right\rangle }^2}}}\left[{{L_0} + 2\left({{L_0} - 1} \right)\left\langle B \right\rangle - {L_0}{{\left({1 + 2\left\langle B \right\rangle } \right)}^{{{\left({{L_0} - 1} \right)} \mathord{\left/ {\vphantom {{\left({{L_0} - 1} \right)} {{L_0}}}} \right. } {{L_0}}}}}} \right] . Meanwhile, the matrix {{\bf{{ D}}}_{\text{S}}} in Eq (A10) becomes
{{\bf{{ D}}}_{\text{S}}} = {\tilde k_0}\left( {\begin{array}{*{20}{c}} {\gamma \left( {{k_c} + {k_r}} \right)}&{ - {k_c}}&{ - {k_r}} \\ { - {k_c}}&{2{k_c}}&0 \\ { - {k_r}}&0&{2{k_r}} \end{array}} \right) , | (B4) |
where {\tilde k_0} = \frac{{a{k_0}}}{{{k_c} + {k_r}}} and \gamma = \frac{{\left\langle {{B^2}} \right\rangle + \left\langle B \right\rangle }}{{\left\langle B \right\rangle }} . We can directly derive the following relationships from Eq (A10):
\left( {b\left\langle B \right\rangle - 1} \right)\left( {{k_c} + {k_r}} \right){\sigma _{11}} + bd\left\langle B \right\rangle \left( {{\sigma _{12}} + {\sigma _{13}}} \right) = - \frac{{{{\tilde k}_0}}}{2}\gamma \left( {{k_c} + {k_r}} \right) | (B5a) |
\left( {b\left\langle B \right\rangle - 1} \right)\left( {{k_c} + {k_r}} \right){\sigma _{12}} + bd\left\langle B \right\rangle {\sigma _{22}} + bd\left\langle B \right\rangle {\sigma _{23}} + {k_c}{\sigma _{11}} - d{\sigma _{12}} = {\tilde k_0}{k_c} | (B5b) |
\left( {b\left\langle B \right\rangle - 1} \right)\left( {{k_c} + {k_r}} \right){\sigma _{13}} + bd\left\langle B \right\rangle {\sigma _{23}} + bd\left\langle B \right\rangle {\sigma _{33}} + {k_r}{\sigma _{11}} - d{\sigma _{13}} = {\tilde k_0}{k_r} | (B5c) |
and obtain the following relationships
{\sigma _{12}} = \frac{d}{{{k_c}}}{\sigma _{22}} - {\tilde k_0} , {\sigma _{13}} = \frac{d}{{{k_r}}}{\sigma _{33}} - {\tilde k_0} and {\sigma _{23}} = \frac{1}{2}\left({\frac{{{k_r}}}{{{k_c}}}{\sigma _{22}} + \frac{{{k_c}}}{{{k_r}}}{\sigma _{33}}} \right) - \frac{{{k_c} + {k_r}}}{{2d}}{\tilde k_0}. |
Substituting these relationships into Eq (B4a)–Eq (B4c) yields
\left( {b\left\langle B \right\rangle - 1} \right)\left( {{k_c} + {k_r}} \right){\sigma _{11}} + bd\left\langle B \right\rangle \left( {\frac{d}{{{k_c}}}{\sigma _{22}} + \frac{d}{{{k_r}}}{\sigma _{33}}} \right) = 2bd\left\langle B \right\rangle {\tilde k_0} - \frac{{{{\tilde k}_0}}}{2}\gamma \left( {{k_c} + {k_r}} \right) , | (B6a) |
\frac{{{k_c}}}{d}{\sigma _{11}} + \left( {2b\left\langle B \right\rangle - 1 + \frac{{3b\left\langle B \right\rangle - 2}}{2}\frac{{{k_r}}}{{{k_c}}} - \frac{d}{{{k_c}}}} \right){\sigma _{22}} + \frac{{b\left\langle B \right\rangle }}{2}\frac{{{k_c}}}{{{k_r}}}{\sigma _{33}} = \frac{{{k_c}}}{d}{\tilde k_0} + \left[ {\frac{{3b\left\langle B \right\rangle - 2}}{2}\frac{{{k_c} + {k_r}}}{d} - 1} \right]{\tilde k_0} , | (B6b) |
\frac{{{k_r}}}{d}{\sigma _{11}} + \frac{{b\left\langle B \right\rangle }}{2}\frac{{{k_r}}}{{{k_c}}}{\sigma _{22}} + \left( {2b\left\langle B \right\rangle - 1 + \frac{{3b\left\langle B \right\rangle - 2}}{2}\frac{{{k_c}}}{{{k_r}}} - \frac{d}{{{k_r}}}} \right){\sigma _{33}} = \frac{{{k_r}}}{d}{\tilde k_0} + \left[ {\frac{{3b\left\langle B \right\rangle - 2}}{2}\frac{{{k_c} + {k_r}}}{d} - 1} \right]{\tilde k_0} . | (B6c) |
The combination of Eq (B6a), (B6b) gives
{\sigma _{22}} = {\left( {\frac{{{k_r}}}{{{k_c}}}} \right)^2}{\sigma _{33}} + \frac{{{k_c}}}{{{k_r}}}\frac{{{k_c} + {k_r}}}{d}{\tilde k_0} , or {\sigma _{33}} = {\left( {\frac{{{k_c}}}{{{k_r}}}} \right)^2}\left( {{\sigma _{22}} - \frac{{{k_c} + {k_r}}}{d}\frac{{{k_c}}}{{{k_r}}}{{\tilde k}_0}} \right). | (B7a) |
The sum of Eq (B6b), (B6c) gives
\frac{{{k_c} + {k_r}}}{d}{\sigma _{11}} + \left[ {\left( {2b\left\langle B \right\rangle - 1} \right)\frac{{{k_c} + {k_r}}}{d} - 1} \right]\left( {\frac{d}{{{k_c}}}{\sigma _{22}} + \frac{d}{{{k_r}}}{\sigma _{33}}} \right) = \left[ {\frac{{3b\left\langle B \right\rangle - 1}}{2}\frac{{{k_c} + {k_r}}}{d} - 1} \right]{\tilde k_0} . | (B7b) |
The combination of Eq (B7b) and (B6a) yields
\frac{d}{{{k_c}}}{\sigma _{22}} + \frac{d}{{{k_r}}}{\sigma _{33}} = 1 - \frac{{b\left\langle B \right\rangle + \frac{1}{2}\left[ {{{\left( {b\left\langle B \right\rangle - 1} \right)}^2} - \gamma } \right]\frac{{{k_c} + {k_r}}}{d}}}{{1 + \left( {b\left\langle B \right\rangle - 1} \right)\left( {2b\left\langle B \right\rangle - 1} \right)\frac{{{k_c} + {k_r}}}{d}}} | (B7c) |
By substituting this equation into Eq (B7a), we finally obtain
{\sigma _{22}} = \frac{{{{\tilde k}_0}{{\tilde k}_c}\tilde k_r^3}}{{\tilde k_c^3 + \tilde k_r^3}}\left\{ {2 + \frac{{{{\tilde k}_c}}}{{{{\tilde k}_r}}} + \frac{1}{2}\frac{{2\tilde b + \left[ {\gamma - {{\left( {1 + \tilde b} \right)}^2}} \right]\left( {{{\tilde k}_c} + {{\tilde k}_r}} \right)}}{{1 + \left( {1 + \tilde b} \right)\left( {1 + 2\tilde b} \right)\left( {{{\tilde k}_c} + {{\tilde k}_r}} \right)}}} \right\} , | (B8a) |
and further
{\sigma _{33}} = \frac{{{{\tilde k}_0}{{\tilde k}_r}\tilde k_c^3}}{{\tilde k_c^3 + \tilde k_r^3}}\left\{ {1 - \frac{{\tilde k_c^3}}{{\tilde k_r^3}} - \frac{{\tilde k_c^4}}{{\tilde k_r^4}} + \frac{1}{2}\frac{{2\tilde b + \left[ {\gamma - {{\left( {1 + \tilde b} \right)}^2}} \right]\left( {{{\tilde k}_c} + {{\tilde k}_r}} \right)}}{{1 + \left( {1 + \tilde b} \right)\left( {1 + 2\tilde b} \right)\left( {{{\tilde k}_c} + {{\tilde k}_r}} \right)}}} \right\} . | (B8b) |
where \tilde b = - b\left\langle B \right\rangle = \frac{1}{{4\left\langle B \right\rangle }}\left[{{L_0} + 2\left({{L_0} - 1} \right)\left\langle B \right\rangle - {L_0}{{\left({1 + 2\left\langle B \right\rangle } \right)}^{{{\left({{L_0} - 1} \right)} \mathord{\left/ {\vphantom {{\left({{L_0} - 1} \right)} {{L_0}}}} \right. } {{L_0}}}}}} \right] > 0 with b < 0 , {\tilde k_c} = \frac{{{k_c}}}{d} , {\tilde k_r} = \frac{{{k_r}}}{d} , \gamma = \frac{{\left\langle {{B^2}} \right\rangle + \left\langle B \right\rangle }}{{\left\langle B \right\rangle }} .
Thus, the cytoplasmic mRNA noise is given by
{\eta _c} = \frac{{{\sigma _{22}}}}{{{{\left\langle {{M_2}} \right\rangle }^2}}} = \frac{1}{{{{\tilde k}_0}{{\tilde k}_c}}}\frac{{\tilde k_r^3}}{{\tilde k_c^3 + \tilde k_r^3}}\left\{ {1 + \frac{{{{\tilde k}_c} + {{\tilde k}_r}}}{{{{\tilde k}_r}}} + \frac{1}{2}\frac{{2\tilde b + \left[ {\gamma - {{\left( {1 + \tilde b} \right)}^2}} \right]\left( {{{\tilde k}_c} + {{\tilde k}_r}} \right)}}{{1 + \left( {1 + \tilde b} \right)\left( {1 + 2\tilde b} \right)\left( {{{\tilde k}_c} + {{\tilde k}_r}} \right)}}} \right\} , | (B9a) |
and the nuclear mRNA noise in the nucleus by
{\eta _r} = \frac{{{\sigma _{33}}}}{{{{\left\langle {{M_3}} \right\rangle }^2}}} = \frac{1}{{{{\tilde k}_0}{{\tilde k}_r}}}\frac{{\tilde k_c^3}}{{\tilde k_c^3 + \tilde k_r^3}}\left\{ {1 - \frac{{\tilde k_c^3}}{{\tilde k_r^3}} - \frac{{\tilde k_c^4}}{{\tilde k_r^4}} + \frac{1}{2}\frac{{2\tilde b + \left[ {\gamma - {{\left( {1 + \tilde b} \right)}^2}} \right]\left( {{{\tilde k}_c} + {{\tilde k}_r}} \right)}}{{1 + \left( {1 + \tilde b} \right)\left( {1 + 2\tilde b} \right)\left( {{{\tilde k}_c} + {{\tilde k}_r}} \right)}}} \right\} . | (B9b) |
In this case, we can show that five effect transition rates take the forms: {K_1}\left(\boldsymbol{x} \right) = {k_0} , {K_2}\left(\boldsymbol{x} \right) = \frac{{\left({{k_0} + {x_1}{k_r} + d{x_2} + d{x_3}} \right){{\left({{x_1}{k_c}} \right)}^{{L_c}}}}}{{{{\left({{k_0} + {x_1}{k_c} + {x_1}{k_r} + d{x_2} + d{x_3}} \right)}^{{L_c}}} - {{\left({{x_1}{k_c}} \right)}^{{L_c}}}}} , {K_3}\left(\boldsymbol{x} \right) = {x_1}{k_r} , {K_4}\left(\boldsymbol{x} \right) = d{x_2} , and {K_5}\left(\boldsymbol{x} \right) = d{x_3} . In order to derive analytical results, we assume that remaining probability is so small that {p_r} \approx 0 , implying {k_r} = 0 , {k_c} = {k_1} and {K_3}\left(\boldsymbol{x} \right) = 0 . By solving the steady-state deterministic equation
\left\{ \begin{array}{l} \left\langle B \right\rangle {K_1} - {K_2} - {K_3} = 0 \hfill \\ {K_2} - {K_4} = 0 \hfill \\ {K_3} - {K_5} = 0, \hfill \\ \end{array} \right. | (C1) |
we obtain the analytical expression of steady state ( {\boldsymbol{x}^S} ) given by
x_1^S = \frac{{{k_0}\left( {1 + \left\langle B \right\rangle } \right){{\left\langle B \right\rangle }^{{1 \mathord{\left/ {\vphantom {1 {{L_c}}}} \right. } {{L_c}}}}}}}{{{k_1}\left[ {{{\left( {1 + 2\left\langle B \right\rangle } \right)}^{{1 \mathord{\left/ {\vphantom {1 {{L_c}}}} \right. } {{L_c}}}}} - {{\left\langle B \right\rangle }^{{1 \mathord{\left/ {\vphantom {1 {{L_c}}}} \right. } {{L_c}}}}}} \right]}} , x_2^S = \frac{{{k_0}\left\langle B \right\rangle }}{d} and x_3^S = 0. | (C2) |
Note that the elements of Jacob matrix in the linear noise approximation reduce to
{a_{11}} = - \frac{{\partial {K_2}\left({{\boldsymbol{x}^S}} \right)}}{{\partial {x_1}}} , {a_{12}} = - \frac{{\partial {K_2}\left({{\boldsymbol{x}^S}} \right)}}{{\partial {x_2}}} , {a_{13}} = - \frac{{\partial {K_2}\left({{\boldsymbol{x}^S}} \right)}}{{\partial {x_3}}} , {a_{21}} = \frac{{\partial {K_2}\left({{\boldsymbol{x}^S}} \right)}}{{\partial {x_1}}} , {a_{22}} = \frac{{\partial {K_2}\left({{\boldsymbol{x}^S}} \right)}}{{\partial {x_2}}} - d , {a_{23}} = \frac{{\partial {K_2}\left({{\boldsymbol{x}^S}} \right)}}{{\partial {x_3}}} , {a_{31}} = 0 , {a_{32}} = 0 , and {a_{33}} = - d . Defferentiating function {K_2}\left(\boldsymbol{x} \right) with regard to {x_1} yields
\frac{{\partial {K_2}\left( \boldsymbol{x} \right)}}{{\partial {x_1}}} = \frac{{{L_c}\left( {{k_0} + {x_2}d} \right){{\left( {{k_1}} \right)}^{{L_c}}}{{\left( {{x_1}} \right)}^{{L_c} - 1}}}}{{{{\left( {{k_0} + {x_1}{k_1} + {x_2}d} \right)}^{{L_c}}} - {{\left( {{x_1}{k_1}} \right)}^{{L_c}}}}} - \frac{{{L_c}\left( {{k_0} + {x_2}d} \right){{\left( {{x_1}{k_1}} \right)}^{{L_c}}}\left[ {{k_1}{{\left( {{k_0} + {x_1}{k_1} + {x_2}d} \right)}^{{L_c} - 1}} - {{\left( {{k_1}} \right)}^{{L_c}}}{{\left( {{x_1}} \right)}^{{L_c} - 1}}} \right]}}{{{{\left[ {{{\left( {{k_0} + {x_1}{k_1} + {x_2}d} \right)}^{{L_c}}} - {{\left( {{x_1}{k_1}} \right)}^{{L_c}}}} \right]}^2}}} . |
Therefore,
{\left. {\frac{{\partial {K_2}\left( \boldsymbol{x} \right)}}{{\partial {x_1}}}} \right|_{\boldsymbol{x} = {\boldsymbol{x}^S}}} = - \frac{{\partial {K_2}\left( {{\boldsymbol{x}^S}} \right)}}{{\partial {x_1}}} = \frac{{{L_c}{k_0}\left\langle B \right\rangle }}{{{x_1}}}\left[ {\frac{{1 + 2\left\langle B \right\rangle }}{{1 + \left\langle B \right\rangle }} - \frac{{\left\langle B \right\rangle }}{{1 + \left\langle B \right\rangle }}{{\left( {\frac{{1 + 2\left\langle B \right\rangle }}{{\left\langle B \right\rangle }}} \right)}^{{{\left( {{L_c} - 1} \right)} \mathord{\left/ {\vphantom {{\left( {{L_c} - 1} \right)} {{L_c}}}} \right. } {{L_c}}}}}} \right] . | (C3) |
Completely similarly, we have
\frac{{\partial {K_2}\left( \boldsymbol{x} \right)}}{{\partial {x_2}}} = \frac{{\partial {K_2}\left( \boldsymbol{x} \right)}}{{\partial {x_3}}} = \frac{{d\left\langle B \right\rangle }}{{1 + \left\langle B \right\rangle }} - \frac{{d{L_c}{{\left\langle B \right\rangle }^2}}}{{1 + \left\langle B \right\rangle }}\frac{{{k_0}}}{{{k_1}{x_1}}}{\left( {\frac{{1 + 2\left\langle B \right\rangle }}{{\left\langle B \right\rangle }}} \right)^{{{\left( {{L_c} - 1} \right)} \mathord{\left/ {\vphantom {{\left( {{L_c} - 1} \right)} {{L_c}}}} \right. } {{L_c}}}}} . | (C4) |
Furthermore, the Jacob matrix becomes
{{\bf{A}}_s} = \left( {\begin{array}{*{20}{c}} {{a_{11}}}&{{a_{12}}}&{{a_{12}}} \\ { - {a_{11}}}&{ - {a_{12}} - d}&{ - {a_{12}}} \\ 0&0&{ - d} \end{array}} \right) , | (C5) |
where {a_{11}}{\text{ = }} - \frac{{\partial {K_2}\left({{\boldsymbol{x}^S}} \right)}}{{\partial {x_1}}} and {a_{12}}{\text{ = }} - \frac{{\partial {K_2}\left({{\boldsymbol{x}^S}} \right)}}{{\partial {x_2}}} are given by Eqs (C3) and (C4).
Meanwhile, the matrix {{\bf{D}}_s} in the linear noise approximation is given by
{{\bf{D}}_s} = \left( {\begin{array}{*{20}{c}} {{k_0}\left\langle {{B^2}} \right\rangle + {k_0}\left\langle B \right\rangle }&{ - {K_2}}&0 \\ { - {K_2}}&{2{K_2}}&0 \\ 0&0&0 \end{array}} \right) . | (C6) |
It follows from matrix equation {{\bf{{ A}}}_{\text{S}}}{{\bf{\Sigma}} _{\text{S}}} + {{\bf{\Sigma}} _{\text{S}}}{\bf{{ A}}}_{\text{S}}^{\text{T}} + {{\bf{D}}_{\text{S}}} = {\bf{0}} that
{\sigma _{11}} = - \frac{{{k_0}\left\langle {{B^2}} \right\rangle + {k_0}\left\langle B \right\rangle }}{{2{a_{11}}}} - \frac{{{a_{12}}}}{{a_{11}^2}}{K_2} + \frac{{{a_{12}}\left( {{a_{12}} + d} \right)}}{{a_{11}^2}}{\sigma _{22}} , | (C7a) |
{\sigma _{22}} = {k_0}\frac{{ - {a_{11}}\left( {\left\langle {{B^2}} \right\rangle + \left\langle B \right\rangle } \right) + 2d\left\langle B \right\rangle }}{{2d\left( {{a_{12}} + d - {a_{11}}} \right)}} . | (C7b) |
Substituting the expressions of {a_{11}} and {a_{12}} into Eq (C7b) yields
{\sigma _{22}} = \frac{{{k_0}\left\langle B \right\rangle }}{{2d}}\frac{{\frac{{{L_c}{k_1}}}{\omega }\frac{{1 + 2\left\langle B \right\rangle }}{{1 + \omega + \left\langle B \right\rangle }}\left( {\left\langle {{B^2}} \right\rangle + \left\langle B \right\rangle } \right) + 2d}}{{\frac{d}{{1 + \left\langle B \right\rangle }} + \frac{{{L_c}\left\langle B \right\rangle \left( {1 + 2\left\langle B \right\rangle } \right)}}{{1 + \omega + \left\langle B \right\rangle }}\left( {\frac{d}{{1 + \left\langle B \right\rangle }} + \frac{{{k_1}}}{\omega }} \right)}} , | (C8) |
where \omega = \frac{{\left({1 + \left\langle B \right\rangle } \right){{\left\langle B \right\rangle }^{{1 \mathord{\left/ {\vphantom {1 {{L_c}}}} \right. } {{L_c}}}}}}}{{{{\left({1 + 2\left\langle B \right\rangle } \right)}^{{1 \mathord{\left/ {\vphantom {1 {{L_c}}}} \right. } {{L_c}}}}} - {{\left\langle B \right\rangle }^{{1 \mathord{\left/ {\vphantom {1 {{L_c}}}} \right. } {{L_c}}}}}}} .
[1] |
Y. J. Chi, D. Wang, J. P. Wang, W. D. Yu, J. C. Yang, Long non-coding rna in the pathogenesis of cancers, Cells, 8 (2019), 1015. https://doi.org/10.3390/cells8091015 doi: 10.3390/cells8091015
![]() |
[2] |
S. Djebali, C. A. Davis, A. Merkel, A. Dobin, T. Lassmann, A. Mortazavi, et al., Landscape of transcription in human cells, Nature, 489 (2012), 101–108. https://doi.org/10.1038/nature11233 doi: 10.1038/nature11233
![]() |
[3] |
A. T. Willingham, A. P. Orth, S. Batalov, E. C. Peters, B. G. Wen, P. Aza-Blanc, et al., A strategy for probing the function of noncoding rnas finds a repressor of nfat, Science, 309 (2005), 1570–1573. https://doi.org/10.1126/science.1115901 doi: 10.1126/science.1115901
![]() |
[4] |
C. Xing, S. G. Sun, Z. Q. Yue, F. Bai, Role of lncrna lucat1 in cancer, Biomed. Pharmacother., 134 (2021), 111158. https://doi.org/10.1016/j.biopha.2020.111158 doi: 10.1016/j.biopha.2020.111158
![]() |
[5] |
L. Peng, M. Peng, B. Liao, G. H. Huang, W. B. Li, D. F. Xie, The advances and challenges of deep learning application in biological big data processing, Curr. Bioinf., 13 (2018), 352–359. https://doi.org/10.1163/9789004392533_041 doi: 10.1163/9789004392533_041
![]() |
[6] |
R. H. Wang, Y. Jiang, J. R. Jin, C. L. Yin, H. Q. Yu, F. S. Wang, et al., Deepbio: an automated and interpretable deep-learning platform for high-throughput biological sequence prediction, functional annotation and visualization analysis, Nucleic Acids Res., 51 (2023), 3017–3029. https://doi.org/10.1093/nar/gkad055 doi: 10.1093/nar/gkad055
![]() |
[7] |
L. H. Peng, J. W. Tan, W. Xiong, L. Zhang, Z. Wang, R. Y. Yuan, et al., Deciphering ligand-receptor-mediated intercellular communication based on ensemble deep learning and the joint scoring strategy from single-cell transcriptomic data, Comput. Biol. Med., 163 (2023), 107137. https://doi.org/10.1016/j.compbiomed.2023.107137 doi: 10.1016/j.compbiomed.2023.107137
![]() |
[8] |
W. Liu, Y. Yang, X. Lu, X. Z. Fu, R. Q. Sun, L. Yang, et al., Nsrgrn: a network structure refinement method for gene regulatory network inference, Briefings Bioinf., 24 (2023), bbad129. https://doi.org/10.1093/bib/bbad129 doi: 10.1093/bib/bbad129
![]() |
[9] |
J. C. Wang, Y. J. Chen, Q. Zou, Inferring gene regulatory network from single-cell transcriptomes with graph autoencoder model, PLos Genet., 19 (2023), e1010942. https://doi.org/10.1371/journal.pgen.1010942 doi: 10.1371/journal.pgen.1010942
![]() |
[10] |
L. Peng, C. Yang, L. Huang, X. Chen, X. Z. Fu, W. Liu, Rnmflp: predicting circrna-disease associations based on robust nonnegative matrix factorization and label propagation, Briefings Bioinf., 23 (2022), bbac155. https://doi.org/10.1093/bib/bbac155 doi: 10.1093/bib/bbac155
![]() |
[11] |
W. Liu, T. T. Tang, X. Lu, X. Z. Fu, Y. Yang, L. Peng, Mpclcda: predicting circrna-disease associations by using automatically selected meta-path and contrastive learning, Briefings Bioinf., 24 (2023), bbad227. https://doi.org/10.1093/bib/bbad227 doi: 10.1093/bib/bbad227
![]() |
[12] |
L. L. Zhuo, S. Y. Pan, J. Li, X. Z. Fu, Predicting mirna-lncrna interactions on plant datasets based on bipartite network embedding method, Methods, 207 (2022), 97–102. https://doi.org/10.1016/j.ymeth.2022.09.002 doi: 10.1016/j.ymeth.2022.09.002
![]() |
[13] |
Z. C. Zhou, Z. Y. Du, J. H. Wei, L. L. Zhuo, S. Y. Pan, X. Z. Fu, et al., Mham-npi: Predicting ncrna-protein interactions based on multi-head attention mechanism, Comput. Biol. Med., 163 (2023), 107143. https://doi.org/10.1016/j.compbiomed.2023.107143 doi: 10.1016/j.compbiomed.2023.107143
![]() |
[14] |
X. Chen, L. Wang, J. Qu, N. N. Guan, J. Q. Li, Predicting mirna-disease association based on inductive matrix completion, Bioinformatics, 34 (2018), 4256–4265. https://doi.org/10.1093/bioinformatics/bty503 doi: 10.1093/bioinformatics/bty503
![]() |
[15] |
X. Chen, D. Xie, L. Wang, Q. Zhao, Z. H. You, H. Liu, Bnpmda: Bipartite network projection for mirna-disease association prediction, Bioinformatics, 34 (2018), 3178–3186. https://doi.org/10.1093/bioinformatics/bty333 doi: 10.1093/bioinformatics/bty333
![]() |
[16] |
L. Huang, L. Zhang, X. Chen, Updated review of advances in micrornas and complex diseases: towards systematic evaluation of computational models, Briefings Bioinf., 23 (2022), bbac407. https://doi.org/10.1093/bib/bbac407 doi: 10.1093/bib/bbac407
![]() |
[17] |
C. C. Wang, C. C. Zhu, X. Chen, Ensemble of kernel ridge regression-based small molecule-mirna association prediction in human disease, Briefings Bioinf., 23 (2022), bbab431. https://doi.org/10.1093/bib/bbab431 doi: 10.1093/bib/bbab431
![]() |
[18] |
Z. J. Li, Y. X. Zhang, Y. Bai, X. H. Xie, L. J. Zeng, Imc-mda: Prediction of mirna-disease association based on induction matrix completion, Math. Biosci. Eng., 20 (2023), 10659–10674. https://doi.org/10.3934/mbe.2023471 doi: 10.3934/mbe.2023471
![]() |
[19] |
Q. Qu, X. Chen, B. Ning, X. Zhang, H. Nie, L. Zeng, et al., Prediction of mirna-disease associations by neural network-based deep matrix factorization, Methods, 212 (2023), 1–9. https://doi.org/10.1016/j.ymeth.2023.02.003 doi: 10.1016/j.ymeth.2023.02.003
![]() |
[20] |
L. Zhang, C. C. Wang, X. Chen, Predicting drug-target binding affinity through molecule representation block based on multi-head attention and skip connection, Briefings Bioinf., 23 (2022), bbac468. https://doi.org/10.1093/bib/bbac468 doi: 10.1093/bib/bbac468
![]() |
[21] |
L. Katusiime, Covid-19 and the effect of central bank intervention on exchange rate volatility in developing countries: The case of uganda, National Accounting Rev., 5 (2023), 23–37. https://doi.org/10.3934/NAR.2023002 doi: 10.3934/NAR.2023002
![]() |
[22] |
L. Grassini, Statistical features and economic impact of Covid-19, National Accounting Rev., 5 (2023), 38–40. https://doi.org/10.3934/NAR.2023003 doi: 10.3934/NAR.2023003
![]() |
[23] |
Z. Y. Bao, Z. Yang, Z. Huang, Y. R. Zhou, Q. H. Cui, D. Dong, Lncrnadisease 2.0: an updated database of long non-coding rna-associated diseases, Nucleic Acids Res., 47 (2019), D1034–D1037. https://doi.org/10.1093/nar/gky905 doi: 10.1093/nar/gky905
![]() |
[24] |
S. W. Ning, J. Z. Zhang, P. Wang, H. Zhi, J. J. Wang, Y. Liu, et al., Lnc2cancer: a manually curated database of experimentally supported lncrnas associated with various human cancers, Nucleic Acids Res., 44 (2016), D980–D985. https://doi.org/10.1093/nar/gkv1094 doi: 10.1093/nar/gkv1094
![]() |
[25] |
X. Chen, L. Huang, Computational model for disease research, Briefings Bioinf., 24 (2023), bbac615. https://doi.org/10.1093/bib/bbac615 doi: 10.1093/bib/bbac615
![]() |
[26] |
K. Albitar, K. Hussainey, Sustainability, environmental responsibility and innovation, Green Finance, 5 (2023), 85–88. https://doi.org/10.3934/GF.2023004 doi: 10.3934/GF.2023004
![]() |
[27] |
G. Desalegn, Insuring a greener future: How green insurance drives investment in sustainable projects in developing countries, Green Finance, 5 (2023), 195–210. https://doi.org/10.3934/GF.2023008 doi: 10.3934/GF.2023008
![]() |
[28] |
Y. Liang, Z. H. Zhang, N. N. Liu, Y. N. Wu, C. L. Gu, Y. L. Wang, Magcnse: predicting lncrna-disease associations using multi-view attention graph convolutional network and stacking ensemble model, BMC Bioinf., 23 (2022). https://doi.org/10.1186/s12859-022-04715-w doi: 10.1186/s12859-022-04715-w
![]() |
[29] |
Y. Kim, M. Lee, Deep learning approaches for lncrna-mediated mechanisms: A comprehensive review of recent developments, Int. J. Mol. Sci., 24 (2023), 10299. https://doi.org/10.3390/ijms241210299 doi: 10.3390/ijms241210299
![]() |
[30] |
Z. Q. Zhang, J. L. Xu, Y. N. Wu, N. N. Liu, Y. L. Wang, Y. Liang, Capsnet-lda: predicting lncrna-disease associations using attention mechanism and capsule network based on multi-view data, Briefings Bioinf., 24 (2022), bbac531. https://doi.org/10.1093/bib/bbac531 doi: 10.1093/bib/bbac531
![]() |
[31] |
N. Dwarika, The risk-return relationship and volatility feedback in south africa: a comparative analysis of the parametric and nonparametric bayesian approach, Quant. Finance Econ., 7 (2023), 119–146. https://doi.org/10.3934/QFE.2023007 doi: 10.3934/QFE.2023007
![]() |
[32] |
N. Dwarika, Asset pricing models in south africa: A comparative of regression analysis and the bayesian approach, Data Sci. Finance Econ., 3 (2023), 55–75. https://doi.org/10.3934/DSFE.2023004 doi: 10.3934/DSFE.2023004
![]() |
[33] |
Y. Q. Lin, X. J. Chen, H. Y. Lan, Analysis and prediction of american economy under different government policy based on stepwise regression and support vector machine modelling, Data Sci. Finance Econ., 3 (2023), 1–13. https://doi.org/10.3934/DSFE.2023001 doi: 10.3934/DSFE.2023001
![]() |
[34] |
N. Sheng, L. Huang, Y. T. Lu, H. Wang, L. L. Yang, L. Gao, et al., Data resources and computational methods for lncrna-disease association prediction, Comput. Biol. Med., 153 (2023), 106527. https://doi.org/10.1016/j.compbiomed.2022.106527 doi: 10.1016/j.compbiomed.2022.106527
![]() |
[35] |
J. H. Wei, L. L. Zhuo, S. Y. Pan, X. Z. Lian, X. J. Yao, X. Z. Fu, Headtailtransfer: An efficient sampling method to improve the performance of graph neural network method in predicting sparse ncrna-protein interactions, Comput. Biol. Med., 157 (2023), 106783. https://doi.org/10.1016/j.compbiomed.2023.106783 doi: 10.1016/j.compbiomed.2023.106783
![]() |
[36] |
P. Xuan, S. X. Pan, T. G. Zhang, Y. Liu, H. Sun, Graph convolutional network and convolutional neural network based method for predicting lncrna-disease associations, Cells, 8 (2019), 1012. https://doi.org/10.3390/cells8091012 doi: 10.3390/cells8091012
![]() |
[37] |
M. F. Leung, A. Jawaid, S. W. Ip, C. H. Kwok, S. Yan, A portfolio recommendation system based on machine learning and big data analytics, Data Sci. Finance Econ., 3 (2023), 152–165. https://doi.org/10.3934/DSFE.2023009 doi: 10.3934/DSFE.2023009
![]() |
[38] |
Q. W. Wu, J. F. Xia, J. C. Ni, C. H. Zheng, Gaerf: predicting lncrna-disease associations by graph auto-encoder and random forest, Briefings Bioinf., 22 (2021), bbaa391. https://doi.org/10.1093/bib/bbaa391 doi: 10.1093/bib/bbaa391
![]() |
[39] |
N. Sheng, L. Huang, Y. Wang, J. Zhao, P. Xuan, L. Gao, et al., Multi-channel graph attention autoencoders for disease-related lncrnas prediction, Briefings Bioinf., 23 (2022), bbab604. https://doi.org/10.1093/bib/bbab604 doi: 10.1093/bib/bbab604
![]() |
[40] |
L. Peng, C. Yang, Y. F. Chen, W. Liu, Predicting circrna-disease associations via feature convolution learning with heterogeneous graph attention network, IEEE J. Biomed. Health. Inf., 27 (2023), 3072–3082. https://doi.org/10.1109/JBHI.2023.3260863. doi: 10.1109/JBHI.2023.3260863
![]() |
[41] |
X. Liu, C. Z. Song, F. Huang, H. T. Fu, W. J. Xiao, W. Zhang, Graphcdr: a graph neural network method with contrastive learning for cancer drug response prediction, Briefings Bioinf., 23 (2022), bbab457. https://doi.org/10.1093/bib/bbab457 doi: 10.1093/bib/bbab457
![]() |
[42] |
G. Y. Fu, J. Wang, C. Domeniconi, G. X. Yu, Matrix factorization-based data fusion for the prediction of lncrna–disease associations, Bioinformatics, 34 (2018), 1529–1537. https://doi.org/10.1093/bioinformatics/btx794 doi: 10.1093/bioinformatics/btx794
![]() |
[43] | Z. Y. Lu, K. B. Cohen, L. Hunter, Generif quality assurance as summary revision, in Biocomputing 2007, World Scientific, (2007), 269–280. https://doi.org/10.1142/9789812772435_0026 |
[44] |
J. H. Li, S. Liu, H. Zhou, L. H. Qu, J. H. Yang, starBase v2. 0: decoding miRNA-ceRNA, miRNA-ncRNA and protein–RNA interaction networks from large-scale CLIP-Seq data, Nucleic Acids Res., 42 (2014), D92–D97. https://doi.org/10.1093/nar/gkt1248 doi: 10.1093/nar/gkt1248
![]() |
[45] |
W. Lan, Y. Dong, Q. F. Chen, R. Q. Zheng, J. Liu, Y. Pan, et al., Kgancda: predicting circrna-disease associations based on knowledge graph attention network, Briefings Bioinf., 23 (2022), bbab494. https://doi.org/10.1093/bib/bbab494 doi: 10.1093/bib/bbab494
![]() |
[46] |
Z. H. Guo, Z. H. You, D. S. Huang, H. C. Yi, Z. H. Chen, Y. B. Wang, A learning based framework for diverse biomolecule relationship prediction in molecular association network, Commun. Biol., 3 (2020). https://doi.org/10.1038/s42003-020-0858-8 doi: 10.1038/s42003-020-0858-8
![]() |
[47] |
D. Wang, J. Wang, M. Lu, F. Song, Q. H. Cui, Inferring the human microRNA functional similarity and functional network based on microRNA-associated diseases, Bioinformatics, 26 (2010), 1644–1650. https://doi.org/10.1093/bioinformatics/btq241 doi: 10.1093/bioinformatics/btq241
![]() |
[48] |
X. Chen, Predicting lncRNA-disease associations and constructing lncRNA functional similarity network based on the information of miRNA, Sci. Rep., 5 (2015), 13186. https://doi.org/10.1038/srep13186 doi: 10.1038/srep13186
![]() |
[49] |
X. Chen, G. Y. Yan, Novel human lncrna-disease association inference based on lncrna expression profiles, Bioinformatics, 29 (2013), 2617–2624. https://doi.org/10.1093/bioinformatics/btt426 doi: 10.1093/bioinformatics/btt426
![]() |
[50] |
D. Anderson, U. Ulrych, Accelerated american option pricing with deep neural networks, Quant. Finance Econ., 7 (2023), 207–228. https://doi.org/10.3934/QFE.2023011 doi: 10.3934/QFE.2023011
![]() |
[51] | T. N. Kipf, M. Welling, Semi-supervised classification with graph convolutional networks, preprint, arXiv: 1609.02907. https://doi.org/10.48550/arXiv.1609.02907 |
[52] |
L. Peng, Y. Tu, L. Huang, Y. Li, X. Z. Fu, X. Chen, Daestb: inferring associations of small molecule–mirna via a scalable tree boosting model based on deep autoencoder, Briefings Bioinf., 23 (2022), bbac478. https://doi.org/10.1093/bib/bbac478 doi: 10.1093/bib/bbac478
![]() |
[53] |
Z. Y. Chu, S. C. Liu, W. Zhang, Hierarchical graph representation learning for the prediction of drug-target binding affinity, Inf. Sci., 613 (2022), 507–523. https://doi.org/10.1016/j.ins.2022.09.043 doi: 10.1016/j.ins.2022.09.043
![]() |
[54] | M. Chen, Z. W. Wei, Z. F. Huang, B. L. Ding, Y. L. Li, Simple and deep graph convolutional networks, in Proceedings of the 37th International Conference on Machine Learning, PMLR, (2020), 1725–1735. |
[55] |
X. Chen, Katzlda: Katz measure for the lncrna-disease association prediction, Sci. Rep., 5 (2015), 16840. https://doi.org/10.1038/srep16840 doi: 10.1038/srep16840
![]() |
[56] |
C. Q. Lu, M. Y. Yang, F. Luo, F. X. Wu, M. Li, Y. Pan, et al., Prediction of lncrna–disease associations based on inductive matrix completion, Bioinformatics, 34 (2018), 3357–3364. https://doi.org/10.1093/bioinformatics/bty327 doi: 10.1093/bioinformatics/bty327
![]() |
[57] |
X. M. Wu, W. Lan, Q. F. Chen, Y. Dong, J. Liu, W. Peng, Inferring LncRNA-disease associations based on graph autoencoder matrix completion, Comput. Biol. Chem., 87 (2020), 107282. https://doi.org/10.1016/j.compbiolchem.2020.107282 doi: 10.1016/j.compbiolchem.2020.107282
![]() |
[58] |
M. Zeng, C. Q. Lu, Z. H. Fei, F. X. Wu, Y. H. Li, J. X. Wang, et al., Dmflda: a deep learning framework for predicting lncrna–disease associations, IEEE/ACM Trans. Comput. Biol. Bioinf., 18 (2020), 2353–2363. https://doi.org/10.1109/TCBB.2020.2983958. doi: 10.1109/TCBB.2020.2983958
![]() |
[59] |
R. Zhu, Y. Wang, J. X. Liu, L. Y. Dai, Ipcarf: improving lncrna-disease association prediction using incremental principal component analysis feature selection and a random forest classifier, BMC Bioinf., 22 (2021). https://doi.org/10.1186/s12859-021-04104-9 doi: 10.1186/s12859-021-04104-9
![]() |
[60] |
Y. S. Sun, Z. Zhao, Z. N. Yang, F. Xu, H. J. Lu, Z. Y. Zhu, et al., Risk factors and preventions of breast cancer, Int. J. Biol. Sci., 13 (2017), 1387–1397. https://doi.org/10.7150/ijbs.21635 doi: 10.7150/ijbs.21635
![]() |
[61] |
H. Jin, W. Du, W. T. Huang, J. J. Yan, Q. Tang, Y. B. Chen, et al., lncRNA and breast cancer: Progress from identifying mechanisms to challenges and opportunities of clinical treatment, Mol. Ther.–Nucleic Acids, 25 (2021), 613–637. https://doi.org/10.1016/j.omtn.2021.08.005 doi: 10.1016/j.omtn.2021.08.005
![]() |
[62] |
J. J. Xu, M. S. Hu, Y. Gao, Y. S. Wang, X. N. Yuan, Y. Yang, et al., Lncrna mir17hg suppresses breast cancer proliferation and migration as cerna to target fam135a by sponging mir-454-3p, Mol. Biotechnol., 65 (2023), 2071–2085. https://doi.org/10.1007/s12033-023-00706-1 doi: 10.1007/s12033-023-00706-1
![]() |
[63] | K. X. Lou, Z. H. Li, P. Wang, Z. Liu, Y. Chen, X. L. Wang, et al., Long non-coding rna bancr indicates poor prognosis for breast cancer and promotes cell proliferation and invasion, Eur. Rev. Med. Pharmacol. Sci., 22 (2018), 1358–1365. |
[64] |
F. Bray, J. Ferlay, I. Soerjomataram, R. L. Siegel, L. A. Torre, A. Jemal, Global cancer statistics 2018: Globocan estimates of incidence and mortality worldwide for 36 cancers in 185 countries, CA: Cancer J. Clinicians, 68 (2018), 394–424. https://doi.org/10.3322/caac.21492 doi: 10.3322/caac.21492
![]() |
[65] | Z. W. Wang, Y. Y. Jin, H. T. Ren, X. L. Ma, B. F. Wang, Y. L. Wang, Downregulation of the long non-coding RNA TUSC7 promotes NSCLC cell proliferation and correlates with poor prognosis, Am. J. Transl. Res., 8 (2016), 680–687. |
[66] | H. P. Deng, L. Chen, T. Fan, B. Zhang, Y. Xu, Q. Geng, Long non-coding rna hottip promotes tumor growth and inhibits cell apoptosis in lung cancer, Cell. Mol. Biol., 61 (2015), 34–40. |
[67] |
H. Sung, J. Ferlay, R. L. Siegel, M. Laversanne, I. Soerjomataram, A. Jemal, et al., Global cancer statistics 2020: Globocan estimates of incidence and mortality worldwide for 36 cancers in 185 countries. CA: Cancer J. Clinicians, 71 (2021), 209–249. https://doi.org/10.3322/caac.21660 doi: 10.3322/caac.21660
![]() |
[68] |
J. Q. Wang, L. P. Su, X. H. Chen, P. Li, Q. Cai, B. Q. Yu, et al., MALAT1 promotes cell proliferation in gastric cancer by recruiting SF2/ASF, Biomed. Pharmacother., 68 (2014), 557–564. https://doi.org/10.1016/j.biopha.2014.04.007 doi: 10.1016/j.biopha.2014.04.007
![]() |
[69] |
L. Ma, Y. J. Zhou, X. J. Luo, H. Gao, X. B. Deng, Y. J. Jiang, Long non-coding RNA XIST promotes cell growth and invasion through regulating miR-497/MACC1 axis in gastric cancer, Oncotarget, 8 (2017), 4125–4135. https://doi.org/10.18632/oncotarget.13670 doi: 10.18632/oncotarget.13670
![]() |
[70] |
H. T. Fu, F. Huang, X. Liu, Y. Qiu, W. Zhang, Mvgcn: data integration through multi-view graph convolutional network for predicting links in biomedical bipartite networks, Bioinformatics, 38 (2022), 426–434. https://doi.org/10.1093/bioinformatics/btab651 doi: 10.1093/bioinformatics/btab651
![]() |
1. | Noreen Saba, Sana Maqsood, Muhammad Asghar, Ghulam Mustafa, Faheem Khan, An extended Mittag Leffler function in terms of extended Wright complex hypergeometric function, 2025, 117, 11100168, 364, 10.1016/j.aej.2024.12.065 |