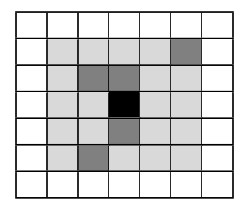
Small-world networks and scale-free networks are well-known theoretical models within the realm of complex graphs. These models exhibit "low" average shortest-path length; however, key distinctions are observed in their degree distributions and average clustering coefficients: in small-world networks, the degree distribution is bell-shaped and the clustering is "high"; in scale-free networks, the degree distribution follows a power law and the clustering is "low". Here, a model for generating scale-free graphs with "high" clustering is numerically explored, since these features are concurrently identified in networks representing social interactions. In this model, the values of average degree and exponent of the power-law degree distribution are both adjustable, and spatial limitations in the creation of links are taken into account. Several topological metrics are calculated and compared for computer-generated graphs. Unexpectedly, the numerical experiments show that, by varying the model parameters, a transition from a power-law to a bell-shaped degree distribution can occur. Also, in these graphs, the degree distribution is most accurately characterized by a pure power-law for values of the exponent typically found in real-world networks.
Citation: A. Newton Licciardi Jr., L.H.A. Monteiro. A network model of social contacts with small-world and scale-free features, tunable connectivity, and geographic restrictions[J]. Mathematical Biosciences and Engineering, 2024, 21(4): 4801-4813. doi: 10.3934/mbe.2024211
[1] | A. N. Licciardi Jr., L. H. A. Monteiro . A complex network model for a society with socioeconomic classes. Mathematical Biosciences and Engineering, 2022, 19(7): 6731-6742. doi: 10.3934/mbe.2022317 |
[2] | F. S. Vannucchi, S. Boccaletti . Chaotic spreading of epidemics in complex networks of excitable units. Mathematical Biosciences and Engineering, 2004, 1(1): 49-55. doi: 10.3934/mbe.2004.1.49 |
[3] | Abdelkarim Lamghari, Dramane Sam Idris Kanté, Aissam Jebrane, Abdelilah Hakim . Modeling the impact of distancing measures on infectious disease spread: a case study of COVID-19 in the Moroccan population. Mathematical Biosciences and Engineering, 2024, 21(3): 4370-4396. doi: 10.3934/mbe.2024193 |
[4] | Yang Pan, Jinhua Yang, Lei Zhu, Lina Yao, Bo Zhang . Aerial images object detection method based on cross-scale multi-feature fusion. Mathematical Biosciences and Engineering, 2023, 20(9): 16148-16168. doi: 10.3934/mbe.2023721 |
[5] | Dawei Li, Suzhen Lin, Xiaofei Lu, Xingwang Zhang, Chenhui Cui, Boran Yang . IMD-Net: Interpretable multi-scale detection network for infrared dim and small objects. Mathematical Biosciences and Engineering, 2024, 21(1): 1712-1737. doi: 10.3934/mbe.2024074 |
[6] | Yongheng Zhang, Yuliang Lu, GuoZheng Yang . Link importance assessment strategy based on improved $ k $-core decomposition in complex networks. Mathematical Biosciences and Engineering, 2022, 19(7): 7019-7031. doi: 10.3934/mbe.2022331 |
[7] | Nadia Loy, Andrea Tosin . A viral load-based model for epidemic spread on spatial networks. Mathematical Biosciences and Engineering, 2021, 18(5): 5635-5663. doi: 10.3934/mbe.2021285 |
[8] | Meili Tang, Qian Pan, Yurong Qian, Yuan Tian, Najla Al-Nabhan, Xin Wang . Parallel label propagation algorithm based on weight and random walk. Mathematical Biosciences and Engineering, 2021, 18(2): 1609-1628. doi: 10.3934/mbe.2021083 |
[9] | Linard Hoessly, Carsten Wiuf . Fast reactions with non-interacting species in stochastic reaction networks. Mathematical Biosciences and Engineering, 2022, 19(3): 2720-2749. doi: 10.3934/mbe.2022124 |
[10] | Siyuan Shen, Xing Zhang, Wenjing Yan, Shuqian Xie, Bingjia Yu, Shizhi Wang . An improved UAV target detection algorithm based on ASFF-YOLOv5s. Mathematical Biosciences and Engineering, 2023, 20(6): 10773-10789. doi: 10.3934/mbe.2023478 |
Small-world networks and scale-free networks are well-known theoretical models within the realm of complex graphs. These models exhibit "low" average shortest-path length; however, key distinctions are observed in their degree distributions and average clustering coefficients: in small-world networks, the degree distribution is bell-shaped and the clustering is "high"; in scale-free networks, the degree distribution follows a power law and the clustering is "low". Here, a model for generating scale-free graphs with "high" clustering is numerically explored, since these features are concurrently identified in networks representing social interactions. In this model, the values of average degree and exponent of the power-law degree distribution are both adjustable, and spatial limitations in the creation of links are taken into account. Several topological metrics are calculated and compared for computer-generated graphs. Unexpectedly, the numerical experiments show that, by varying the model parameters, a transition from a power-law to a bell-shaped degree distribution can occur. Also, in these graphs, the degree distribution is most accurately characterized by a pure power-law for values of the exponent typically found in real-world networks.
The study of complex networks experienced significant advances, over two decades ago, due to the expansion of the Internet [1,2] and, more recently, due to the outbreak of the COVID-19 pandemic [3,4]. The characterization of the connection patterns among computers and among humans has been crucial to understand, for instance, the dissemination of factual and false information [5], the propagation of computer viruses [6], and the spreading of contagious diseases [7].
In addition to the network model based on purely random connections, first theoretically analyzed by Gilbert [8] and Erdös and Rényi [9,10], two other network models have garnered widespread attention and utilization in the academic research. These models are commonly referred to as "small world" and "scale free". Such models present crucial differences in their degree distributions P(k) and average clustering coefficients ⟨c⟩. Recall that, in undirected graphs, the degree k of a node is the number of links connected to this node, P(k) denotes the percentage of nodes with degree k, the clustering coefficient c of a node is defined as the ratio of the number of links among its neighbors to the maximum number of possible links among these neighbors, and the average degree ⟨k⟩ and the average clustering ⟨c⟩ are the average values of k and c, respectively, by taking into account all N nodes composing the network [11,12,13,14]. Small-world graphs, originally proposed by Watts and Strogatz [15,16], emerge from a rewiring process applied to a regular lattice with a constant N. In this transformative process, a portion of the regular links are probabilistically replaced by random links. Such graphs exhibit "high" average clustering coefficient and bell-shaped degree distribution (here, "high" ⟨c⟩ means ⟨c⟩≫⟨c⟩random=⟨k⟩/N, in which ⟨c⟩random is the average clustering obtained for a purely random network with the same values of ⟨k⟩ and N). Scale-free networks, employed by Barabási and colleagues [11,17] to investigate the topology of various complex systems, are built from a preferential attachment rule (previously introduced by other authors [18,19,20]), wherein the probability of a new node forming a connection with a pre-existing node is directly proportional to the degree of this pre-existing node. Such graphs exhibit "low" ⟨c⟩ and power-law degree distribution. Despite being widely employed, both these approaches have their shortcomings [21,22,23]: for instance, the rewiring process may be perceived as somewhat contrived and the preferential attachment demands that the new node possesses awareness of the degrees of all nodes composing the growing network.
Social interactions among individuals, groups, and even corporations have been represented by graphs [24,25,26,27]. In real-world scenarios, these interactions are simultaneously characterized by "high" ⟨c⟩ and P(k)∼k−γ; that is, a degree distribution following a power law. Therefore, actual social networks concurrently exhibit topological properties found in small-world and scale-free graphs [11,12,13,14]. For instance, for a network in which the nodes represent actors and actresses and a link between two nodes implies that they have acted in the same movie together, ⟨c⟩=0.79 and γ=2.3 [11,15,17]. The model investigated in this article embodies these characteristics in a network with fixed number of nodes. Furthermore, it presents "low" average shortest-path length ⟨ℓ⟩, as observed in real-world networks and also in purely random, small-world, and scale-free graphs [11,12,13,14] (here, "low" ⟨ℓ⟩ means ⟨ℓ⟩≈⟨ℓ⟩random≃logN/log⟨k⟩, in which ⟨ℓ⟩random is the average shortest-path length obtained for a purely random network with the same values of ⟨k⟩ and N).
The network model numerically investigated here was originally proposed by us [28] for analyzing the impact of socioeconomic stratification on word-of-mouth dissemination of information about COVID-19. In that study [28], however, the influence of the model parameters on the network structure was not explored. This model is based on the assumption that each individual typically interacts with a local community, in close physical proximity, composed of nearby family members, friends, neighbors, coworkers. In the model, the individuals can establish a maximum number of links within a limited area representing the geographic region where they live. Thus, the individuals are primarily locally connected, leading to "high" clustering. The number of links per individual is randomly taken from a power-law distribution. Therefore, the network topology becomes scale free, since P(k) obeys a power law. Simpler versions of this model were already employed in studies on neurophysiology [29], game theory [30], and epidemiology [31,32,33].
There have been proposed computational methods for growing scale-free networks with tunable clustering coefficient and/or degree distribution [34,35,36,37,38,39]. Also, there have been proposed computational methods for creating networks that take into account the distances between nodes [29,30,40,41,42]. The algorithm numerically explored here enables adjusting the power-law exponent γ of P(k)∼k−γ and the average degree ⟨k⟩ in a fixed-size network with geographic constraints. Realistic values of γ and ⟨k⟩ have been determined for several social groups [11,12,28] and they can be used in this algorithm.
In short, this article is about a network model with ⟨ℓ⟩≈⟨ℓ⟩random, ⟨c⟩≫⟨c⟩random and P(k)∼k−γ, with tunable values of ⟨k⟩ and γ, in which geographic constraints are considered in the probabilistic creation of links. A network model with all these features was not found in the literature. The remainder of this article is organized as follows. In Section 2, the developed algorithm is explained. In Section 3, network metrics usually employed to characterize the topological structure of graphs are listed. In Section 4, the results obtained from numerical simulations are presented. In Section 5, these results are discussed and the possible relevance of the network model is stressed.
Consider a square grid composed of η×η cells, in which each cell represents an individual. Therefore, there are N=η2 individuals in this social group. To mitigate edge effects, the left and right edges are connected, and similarly, the top and bottom edges are also connected. Thus, all individuals residing in this grid are geographically equivalent. In this network model, social contacts among individuals can occur within a neighborhood of radius r. These social contacts are represented by undirected links between individuals. These links are established through a random wiring process, in which an individual is connected to k others positioned within the square matrix of size (2r+1)×(2r+1) centered around such an individual (self-connections and multiple links are not allowed) [28,29,30]. In cellular automata literature, r is called Moore's radius [43]. Figure 1 illustrates an individual (black cell) with k=5 neighbors (dark gray cells) in a Moore neighborhood with r=2 (light gray cells).
In the model, the probability qi of connecting an individual to another individual belonging to the neighborhood layer i (i=1,2,...,r) is calculated from [28,29,30]:
qi=2(r+1−i)r(r+1) | (2.1) |
Notice that ∑ri=1qi=1 and qi decreases with i. Therefore, the greater the distance between two individuals is, the smaller the probability of them being connected. For instance, for r=2, then q1=2/3 and q2=1/3. Since the links are primarily local, this model can be suitable for representing face-to-face encounters in a social group. Such encounters can impact, for instance, information diffusion, infection spreading, and goods exchange.
The degree k of each individual is obtained as follows. First, a number x is randomly picked from the standard uniform distribution U(0,1). Then, the value of k is determined from P(k)=x; thus, k=P−1(x) (as in an inverse transform sampling) with P(k)=Ak−γ [28], in which A is the normalization constant and γ is a positive exponent. As the degree distribution P(k) follows a power law, the resulting graph will exhibit scale-free characteristics [11,12,13,14,17]. The normalization condition ∑kmaxk=kminP(k)=1 imposes that the constant A must be equal to:
A=1∑kmaxk=kmink−γ | (2.2) |
By definition [11,12,13,14], P(k)=nk/N, in which nk is the number of individuals with degree k; hence, ∑kmaxk=kminnk=N. As a consequence:
nk=Nk−γ∑kmaxk=kmink−γ | (2.3) |
The average degree ⟨k⟩ is a relevant metric of realistic networks. In order to adjust the value of ⟨k⟩ of the resulting graph to the target value ktar found in a real-world society, the minimum degree kmin and the maximum degree kmax of P(k) must be conveniently chosen. Recall that ⟨k⟩ can be computed from [11,12,13,14]:
⟨k⟩=kmax∑k=kminkP(k) | (2.4) |
By taking into consideration Eqs (2.2) and (2.3), then:
⟨k⟩=∑kmaxk=kmink−γ+1∑kmaxk=kmink−γ | (2.5) |
Notice that the condition nkmax≥1 (that is, there is at least one individual with k=kmax) implies:
kmax≤(N∑kmaxk=kmink−γ)1/γ | (2.6) |
Equations (2.5) and (2.6) can be used to computationally construct a graph with ⟨k⟩=ktar. Notice that (2r+1)2−1≥kmax; that is, the maximum number of potential neighbors must be greater than or equal to kmax (for instance, if r=1, it is impossible to build a graph with kmax=9, because each individual will have at most 8 neighbors).
To better explain how the values of kmin and kmax are chosen, Figure 2 shows ⟨k⟩ (the blue line) and kmax (the green line) as functions of kmin for N=4900, γ=2.5 and ⟨k⟩tar=10 (the red line). The vertical axis is logarithmically scaled. The plot of ⟨k⟩ was obtained from Eq (2.5) and the plot of kmax from Eq (2.6) (by considering the equals sign). Notice that for kmin=3, then ⟨k⟩≃⟨k⟩tar and, consequently, kmax=113. Therefore, for γ=2.5 and ⟨k⟩tar=10, the algorithm takes kmin=3 and kmax=113.
A pseudocode of the algorithm is given below. The input parameter values are: η (which determines the total number of individuals N), r (the neighborhood radius), γ (the exponent of the power law), and ktar (the average degree of the actual social group). The aim is to build a primarily locally connected graph with ⟨k⟩=ktar and P(k) obeying a power law. In the algorithm, nk is taken as the integer part of the real number calculated from Eq (2.3); hence, ⟨k⟩ in the graph is usually smaller than ktar in the early stages of a simulation. If ⟨k⟩>ktar after building an initial graph (which is very rare), the algorithm stops because a graph with the specified values of ktar and γ cannot be built with the proposed computational method.
Algorithm 1: Pseudocode of the algorithm developed for implementing the network model. |
1. set the input parameter values: η, r, γ, ktar |
2. compute kmin and kmax satisfying Eqs (2.5) and (2.6), by assuming that ⟨k⟩=ktar |
3. determine the values of nk for kmin≤k≤kmax from Eq (2.3) |
4. assign a degree k to each individual from an inverse transform sampling, by considering the values of nk calculated in step 3 |
5. create random links between the individuals by taking into account Eq (2.1) |
6. when all individuals have achieved their specified degrees, the wiring process is interrupted |
7. if there are isolated subgraphs, they are randomly connected to the largest subgraph |
8. compute ⟨k⟩ from Eq (2.4) |
9. stop if ⟨k⟩≥ktar |
10. if ⟨k⟩<ktar, then delete all links and return to step 5 |
11. if ⟨k⟩<ktar after 10 trials, then add 1 to kmin and return to step 3 |
The developed algorithm sequentially creates M≃Nktar/2 links in order to obtain ⟨k⟩=⟨k⟩tar and P(k)=Ak−γ (recall that ⟨k⟩=2M/N [11,12,13,14], in which M is the number of links). Some observations regarding the pseudocode are as follows:
● About step 4, the algorithm takes into account the integer part of nk determined from Eq (2.3) (for instance, if n5=67.12, then the algorithm will create a graph with 67 nodes with k=5).
● About step 5, if there is already a link with the randomly selected node, a new random selection must be made (recall that self-connections and multiple links are prohibited).
● About step 7, in dozens of simulations for networks with 1000≤N≤10000, isolated subgraphs were never found. The idea of step 7 is to connect a randomly chosen node from an isolated subgraph to another randomly chosen node from the largest subgraph.
● Algorithm convergence problems can occur only for γ≤1, which is a value of γ not found in realistic social networks.
The topological structure of graphs is usually characterized by computing the metrics presented in the next section.
The connection pattern of graphs can be quantified by calculating P(k), ⟨k⟩, ⟨l⟩, ⟨c⟩, ⟨Cc⟩, and ⟨Cb⟩. These statistical measures are defined below.
As mentioned in the previous section, P(k)=nk/N and ⟨k⟩=∑kmaxk=kminkP(k), in which nk is the number of nodes with degree k.
For undirected graphs, the average shortest-path length ⟨ℓ⟩ is obtained from [12,13,28]:
⟨ℓ⟩=2∑N−1i=1∑Nj=i+1ℓijN(N−1) | (3.1) |
in which ℓij is the shortest distance (the minimum number of links) between the nodes i and j. This metric influences the velocity that information can travel in the network. The smaller ⟨ℓ⟩, the quicker the communication among the nodes.
For the node i, the clustering coefficient ci is defined as [12,13,28]:
ci=2biki(ki−1) | (3.2) |
in which bi is the number of connections among its ki neighbors. Since this metric reflects the local connectivity, it is commonly used to identify communities.
Centrality measures are calculated to evaluate the relevance of the nodes composing the network. The closeness centrality Cc(i) of the node i is defined as [13,28,44]:
Cc(i)=N−1∑Nj=1lij | (3.3) |
This metric reveals how quickly the node i can communicate with others in the network.
The betweenness centrality Cb(i) of the node i is defined as [13,28,44]:
Cb(i)=2(N−1)(N−2)N−1∑j=1N∑s=j+1gjs(i)gjs | (3.4) |
in which gjs is the number of the shortest paths between the nodes j and s, and gjs(i) is the number of the shortest paths between the nodes j and s passing through the node i. This metric quantifies the extent to which the node i facilitates communication among other nodes.
For the whole network, average values of the metrics defined by Eqs (3.2)–(3.4) are computed from ⟨c⟩=∑Ni=1ci/N, ⟨Cc⟩=∑Ni=1Cc(i)/N, and ⟨Cb⟩=∑Ni=1Cb(i)/N.
In the next section, the numerical results obtained in simulations are presented. Dozens of graphs were computationally created and analyzed. Then, nine graphs were selected, because they serve as representative examples.
Numerical simulations were performed to investigate the influence of r, γ, and ktar on the metrics described in the previous section. In these simulations, η=70 (thus, the graphs are composed of N=4900 nodes). Three sets of simulations were run with the following parameter values:
● set 1: r=10, γ=3, and ktar∈{20,45,100};
● set 2: ktar=20, γ=3, and r∈{5,20,60};
● set 3: r=10, ktar=15, and γ∈{0.3,2.5,8}.
Figure 3 shows how P(k) varies with k for these three sets of parameter values. Set 1 corresponds to the first column of Figure 3, set 2 to the second column, and set 3 to the third column. Tables 1, 2, and 3 present the metrics computed for sets 1, 2, and 3, respectively. The averages and the standard deviations of these metrics were obtained in 5 simulations with the same parameter values. Notice that the standard deviations are less than 0.1%.
ktar | 100×⟨Cc⟩ | 100×⟨Cb⟩ | ⟨ℓ⟩ | 100×⟨c⟩ | ⟨k⟩ | Δk |
20 | 23.90±0.03 | 0.06511±0.00003 | 4.189±0.008 | 6.513±0.003 | 20.1±0.3 | 29.20±0.03 |
45 | 28.59±0.02 | 0.05103±0.00002 | 3.499±0.005 | 11.687±0.004 | 45.2±0.2 | 56.4±0.1 |
100 | 31.72±0.03 | 0.04397±0.00002 | 3.153±0.006 | 22.352±0.008 | 100.3±0.1 | 83.80±0.05 |
r | 100×⟨Cc⟩ | 100×⟨Cb⟩ | ⟨ℓ⟩ | 100×⟨c⟩ | ⟨k⟩ | Δk |
5 | 16.50±0.02 | 0.10339±0.00004 | 6.064±0.004 | 16.937±0.004 | 20.0±0.1 | 28.20±0.03 |
20 | 29.24±0.03 | 0.04956±0.00001 | 3.427±0.003 | 2.826±0.002 | 20.2±0.2 | 34.10±0.06 |
60 | 31.74±0.02 | 0.04406±0.00002 | 3.158±0.004 | 1.278±0.001 | 20.0±0.1 | 89.40±0.08 |
γ | 100×⟨Cc⟩ | 100×⟨Cb⟩ | ⟨ℓ⟩ | 100×⟨c⟩ | ⟨k⟩ | Δk |
0.3 | 24.63±0.02 | 0.06286±0.00003 | 4.079±0.006 | 7.084±0.003 | 21.5±0.8 | 14.20±0.05 |
2.5 | 22.51±0.02 | 0.07046±0.00004 | 4.451±0.008 | 5.911±0.002 | 15.8±0.1 | 28.40±0.01 |
8.0 | 21.48±0.03 | 0.07469±0.00004 | 4.658±0.009 | 4.413±0.003 | 15.3±0.2 | 5.080±0.003 |
These tables also present Δk=kcut−kmin, in which kcut is the degree above which the data deviate more significantly from the straight lines shown in Figure 3. These lines were obtained from the least squares fitting method [45] with the value of γ given in each plot. In fact, various real-world degree distributions are usually better described by [11,12,23,28,46]:
P(k)=Ak−γ10−k/kcut | (4.1) |
that is, by a power law with exponential cutoff. Equation (4.1) implies:
logP(k)=logA−γlogk−k/kcut | (4.2) |
Therefore, the linear regression yields a satisfactory fit in the log-log plot for k≪kcut.
For r=10 and ktar=15 (as adopted in set 3), Figure 4 exhibits how Δk depends on the exponent γ for 0.3≤γ≤10.
Here, the algorithm developed to represent face-to-face social interactions through graphs was numerically explored, by investigating the impact of r, γ, and ⟨k⟩tar on the graph structure. As shown in Figure 3, the algorithm can generate graphs with P(k)∼k−γ, despite the exponential cutoff. Tables 1–3 indicate that ⟨k⟩≃ktar for most graphs. An evident exception is the plot with r=10, γ=0.3, and ktar=15. In this case, P(k) exhibits a bell-shaped curve, which is typical of purely random and small-world networks [11]. This result was unexpected, but it is a consequence of taking γ<1 (which is not found in actual networks [11,12,23,46]). For γ→0, then P(k)→A, kmax→∞ and Δk→0. In this limit case, due to the geographical restrictions imposed by Eq (2.1), P(k) becomes a Poisson-like distribution in the log-log plot. The simulations also show that, as γ increases, the bell-shaped curve in P(k) is stretched along the k-axis and its maximum shifts to the right. Thus, the typical tail usually found in P(k) for scale-free networks [11,17] appears here as a vestige of the stretched Poisson-like distribution.
For purely random networks, ⟨ℓ⟩random≃logN/log⟨k⟩ [11], which is a convenient formula to estimate ⟨ℓ⟩ for the graphs analyzed here. For instance, for r=60, γ=3, and ktar=20 (see Table 2), then ⟨ℓ⟩=3.16≈⟨ℓ⟩random≃log4899/log20.04=2.83. Also, for purely random networks, ⟨c⟩random=⟨k⟩/N [11]. For the graphs analyzed here, ⟨c⟩≫⟨c⟩random. For instance, for r=10, γ=2.5, and ktar=15 (see Table 3), then ⟨c⟩=5.9%≫⟨c⟩random=15.776/4899=0.3%. Therefore, for the graphs exhibited in Figure 2, ⟨ℓ⟩≈⟨ℓ⟩random and ⟨c⟩≫⟨c⟩random, which are typical topological properties of small-world networks [15].
Table 1 shows that, by increasing ktar, ⟨ℓ⟩ decreases and ⟨c⟩ increases. Thus, by raising the average number of links per individual, the distances become shorter and the neighbors become more connected, as expected. Also, ⟨Cc⟩ increases and ⟨Cb⟩ decreases, because the addition of links improves communication and reduces the relative importance of single nodes. The interval Δk also increases with ktar. Hence, the higher ktar is, the larger the interval within a pure power law remains accurate to describe P(k).
Table 2 shows that, by increasing r, ⟨ℓ⟩ and ⟨c⟩ decrease. Therefore, by enlarging the area where the connections are made, the neighbors can become geographically further apart, which can reduce the path (the minimum number of links) between individuals. However, the neighborhood connectivity is also reduced. Consistently, ⟨Cc⟩ increases and ⟨Cb⟩ decreases. In addition, Δk increases with r.
Table 3 shows that ⟨ℓ⟩ and ⟨Cb⟩ increase with γ and ⟨c⟩ and ⟨Cc⟩ decrease with γ. Also, Figure 4 reveals that Δk presents a maximum for 2≲γ≲3, which contains the values of γ usually identified in real-world networks [11,12,13,14]. This is a striking result, since the graphs analyzed here were not created from a preferential attachment rule, which naturally leads to a power law with γ≈3 [11,12,17,18,19,20]. In fact, it is surprising to find out that, in the primarily locally connected graphs created from Eqs (2.1) and (2.3), the interval Δk in which P(k) is a pure power law is greater for 2≲γ≲3. In Figure 4, r=10 and ktar=15; however, the simulations show that this result holds for other values of r and ktar.
In short, the developed algorithm can generate graphs with scale-free and small-world features with the desired values of γ and ⟨k⟩. Such graphs can be used to represent the social contacts in real-world societies. The relation between Δk and γ was an unexpected outcome. This relation suggests the following conjecture: social networks present 2≲γ≲3 because the connectivity in these networks is influenced by the spatial location of their nodes. Usually, the spatial coordinates of the nodes are neglected in network models. For instance, consider the network of actors and actresses mentioned in Section 1. In general, most of the artists involved in a movie are from the same country, but this fact is ignored in the process of graph construction. Evidently, this geographical issue can affect the formation of links, as shown in this study. Another well-known example in which location clearly matters is the network of human sexual contacts [47]. Therefore, the study presented here suggests that the spatial location of the constituent nodes should really be taken into account in social network models based on face-to-face encounters.
The authors declare they have not used artificial intelligence (AI) tools in the creation of this article.
The data used to support the findings of this study are available from the first author (newton. licciardijr@gmail.com) upon request.
The authors declare that there are no conflicts of interest regarding the publication of this paper.
LHAM is partially supported by Conselho Nacional de Desenvolvimento Científico e Tecnológico (CNPq) under the grant #302946/2022-5. This study was financed in part by the Coordenação de Aperfeiçoamento de Pessoal de Nível Superior (CAPES) - finance code 001.
[1] |
A. L. Barabási, R. Albert, H. Jeong, Scale-free characteristics of random networks: the topology of the World-Wide Web, Physica A, 281 (2000), 69–77. https://doi.org/10.1016/S0378-4371(00)00018-2 doi: 10.1016/S0378-4371(00)00018-2
![]() |
[2] |
R. Cohen, K. Erez, D. ben-Avraham, S. Havlin, Resilience of the Internet to random breakdowns, Phys. Rev. Lett., 85 (2000), 4626–4628. https://doi.org/10.1103/PhysRevLett.85.4626 doi: 10.1103/PhysRevLett.85.4626
![]() |
[3] |
H. A. Herrmann, J. M. Schwartz, Why COVID-19 models should incorporate the network of social interactions, Phys. Biol., 17 (2020), 065008. https://doi.org/10.1088/1478-3975/aba8ec doi: 10.1088/1478-3975/aba8ec
![]() |
[4] |
G. S. Hartnett, E. Parker, T. R. Gulden, R. Vardavas, D. Kravitz, Modelling the impact of social distancing and targeted vaccination on the spread of COVID-19 through a real city-scale contact network, J. Complex Netw., 9 (2021), cnab042. https://doi.org/10.1093/comnet/cnab042 doi: 10.1093/comnet/cnab042
![]() |
[5] |
D. Camacho, A. Panizo-LLedot, G. Bello-Orgaz, A. Gonzalez-Pardo, E. Cambria, The four dimensions of social network analysis: An overview of research methods, applications, and software tools, Inf. Fusion, 63 (2020), 88–120. https://doi.org/10.1016/j.inffus.2020.05.009 doi: 10.1016/j.inffus.2020.05.009
![]() |
[6] |
R. Pastor-Satorras, A. Vespignani, Epidemic spreading in scale-free networks, Phys. Rev. Lett., 86 (2001), 3200–3203. https://doi.org/10.1103/PhysRevLett.86.3200 doi: 10.1103/PhysRevLett.86.3200
![]() |
[7] |
D. Brockmann, D. Helbing, The hidden geometry of complex, network-driven contagion phenomena, Science, 342 (2013), 1337–1342. https://doi.org/10.1103/10.1126/science.1245200 doi: 10.1103/10.1126/science.1245200
![]() |
[8] |
E. N. Gilbert, Random graphs, Ann. Math. Statist., 30 (1959), 1141–1144. https://doi.org/10.1214/aoms/1177706098 doi: 10.1214/aoms/1177706098
![]() |
[9] | P. Erdös, A. Rényi, On random graphs Ⅰ, Publ. Math. Debrecen, 6 (1959), 290–297. |
[10] | P. Erdös, A. Rényi, On the evolution of random graphs, Publ. Math. Inst. Hungar. Acad. Sci., 5 (1960), 17–61. |
[11] |
R. Albert, A. L. Barabási, Statistical mechanics of complex networks, Rev. Mod. Phys., 74 (2002), 47–97. https://doi.org/10.1103/RevModPhys.74.47 doi: 10.1103/RevModPhys.74.47
![]() |
[12] |
M. E. J. Newman, The structure and function of complex networks, SIAM Rev., 45 (2003), 167–256. https://doi.org/10.1137/S003614450342480 doi: 10.1137/S003614450342480
![]() |
[13] |
S. Boccaletti, V. Latora, Y. Moreno, M. Chavez, D. U. Hwanga, Complex networks: Structure and dynamics, Phys. Rep., 424 (2006), 175–308. https://doi.org/10.1016/j.physrep.2005.10.009 doi: 10.1016/j.physrep.2005.10.009
![]() |
[14] |
F. Battiston, G. Cencetti, I. Iacopini, V. Latora, M. Lucas, A. Patania, et al., Networks beyond pairwise interactions: Structure and dynamics, Phys. Rep., 874 (2020), 1–92. https://doi.org/10.1016/j.physrep.2020.05.004 doi: 10.1016/j.physrep.2020.05.004
![]() |
[15] |
D. J. Watts, S. H. Strogatz, Collective dynamics of 'small-world' networks, Nature, 393 (1998), 440–442. https://doi.org/10.1038/30918 doi: 10.1038/30918
![]() |
[16] |
S. H. Strogatz, Exploring complex networks, Nature, 410 (2001), 268–276. https://doi.org/10.1038/35065725 doi: 10.1038/35065725
![]() |
[17] |
A. L. Barabási, R. Albert, Emergence of scaling in random networks, Science, 286 (1999), 509–512. https://doi.org/10.1126/science.286.5439.509 doi: 10.1126/science.286.5439.509
![]() |
[18] |
G. U. Yule, A mathematical theory of evolution, based on the conclusions of Dr. J. C. Willis, Philos. Trans. R. Soc. London Ser. B, 213 (1925), 21–87. https://doi.org/10.1098/rstb.1925.0002 doi: 10.1098/rstb.1925.0002
![]() |
[19] |
H. A. Simon, On a class of skew distribution functions, Biometrika, 42 (1955), 425–440. https://doi.org/10.1093/biomet/42.3-4.425 doi: 10.1093/biomet/42.3-4.425
![]() |
[20] |
D. J. S. Price, A general theory of bibliometric and other cumulative advantage processes, J. Amer. Soc. Inform. Sci., 27 (1976), 292–306. https://doi.org/10.1002/asi.4630270505 doi: 10.1002/asi.4630270505
![]() |
[21] |
G. Lima-Mendez, J. van Helden, The powerful law of the power law and other myths in network biology, Mol. Biosyst., 5 (2009), 1482–1493. https://doi.org/10.1039/b908681a doi: 10.1039/b908681a
![]() |
[22] | W. Willinger, D. Alderson, J. C. Doyle, Mathematics and the Internet: A source of enormous confusion and great potential, Not. Am. Math. Soc., 56 (2009), 586–599. |
[23] |
A. D. Broido, A. Clauset, Scale-free networks are rare, Nat. Commun., 10 (2019), 1017. https://doi.org/10.1038/s41467-019-08746-5 doi: 10.1038/s41467-019-08746-5
![]() |
[24] |
C. Kasper, B. Voelkl, A social network analysis of primate groups, Primates, 50 (2009), 343–356. https://doi.org/10.1007/s10329-009-0153-2 doi: 10.1007/s10329-009-0153-2
![]() |
[25] |
S. Hennemann, B. Derudder, An alternative approach to the calculation and analysis of connectivity in the world city network, Environ. Plan. B-Plan. Des., 41 (2014), 392–412. https://doi.org/10.1068/b39108 doi: 10.1068/b39108
![]() |
[26] |
Y. L. Chuang, T. Chou, M. R. D'Orsogna, A network model of immigration: Enclave formation vs. cultural integration, Netw. Heterog. Media, 14 (2019), 53–77. https://doi.org/10.3934/nhm.2019004 doi: 10.3934/nhm.2019004
![]() |
[27] |
R. Munoz-Cancino, C. Bravo, S. A. Rios, M. Grana, On the combination of graph data for assessing thin-file borrowers' creditworthiness, Expert Syst. Appl., 213 (2023), 118809. https://doi.org/10.1016/j.eswa.2022.118809 doi: 10.1016/j.eswa.2022.118809
![]() |
[28] |
A. N. Licciardi Jr., L. H. A. Monteiro, A complex network model for a society with socioeconomic classes, Math. Biosci. Eng., 19 (2022), 6731–6742. https://doi.org/10.3934/mbe.2022317 doi: 10.3934/mbe.2022317
![]() |
[29] |
L. H. A. Monteiro, D. C. Paiva, J. R. C. Piqueira, Spreading depression in mainly locally connected cellular automaton, J. Biol. Syst., 14 (2006), 617–629. https://doi.org/10.1142/S0218339006001957 doi: 10.1142/S0218339006001957
![]() |
[30] |
P. H. T. Schimit, B. O. Santos, C. A. Soares, Evolution of cooperation in Axelrod tournament using cellular automata, Physica A, 437 (2015), 204–217. https://doi.org/10.1016/j.physa.2015.05.111 doi: 10.1016/j.physa.2015.05.111
![]() |
[31] |
P. H. T. Schimit, L. H. A. Monteiro, On the basic reproduction number and the topological properties of the contact network: An epidemiological study in mainly locally connected cellular automata, Ecol. Model., 220 (2009), 1034–1042. https://doi.org/10.1016/j.ecolmodel.2009.01.014 doi: 10.1016/j.ecolmodel.2009.01.014
![]() |
[32] |
H. A. L. R. Silva, L. H. A. Monteiro, Self-sustained oscillations in epidemic models with infective immigrants, Ecol. Complex., 17 (2014), 40–45. https://doi.org/10.1016/j.ecocom.2013.08.002 doi: 10.1016/j.ecocom.2013.08.002
![]() |
[33] |
L. H. A. Monteiro, D. M. Gandini, P. H. T. Schimit, The influence of immune individuals in disease spread evaluated by cellular automaton and genetic algorithm, Comput. Meth. Programs Biomed., 196 (2020), 105707. https://doi.org/10.1016/j.cmpb.2020.105707 doi: 10.1016/j.cmpb.2020.105707
![]() |
[34] |
K. Klemm, V. M. Eguiluz, Growing scale-free networks with small-world behavior, Phys. Rev. E, 65 (2002), 057102. https://doi.org/10.1103/PhysRevE.65.057102 doi: 10.1103/PhysRevE.65.057102
![]() |
[35] |
P. Holme, B. J. Kim, Growing scale-free networks with tunable clustering, Phys. Rev. E, 65 (2002), 026107. https://doi.org/10.1103/PhysRevE.65.026107 doi: 10.1103/PhysRevE.65.026107
![]() |
[36] |
Z. Z. Zhang, L. L. Rong, B. Wang, S. G. Zhou, J. H. Guan, Local-world evolving networks with tunable clustering, Physica A, 380 (2007), 639–650. https://doi.org/10.1016/j.physa.2007.02.045 doi: 10.1016/j.physa.2007.02.045
![]() |
[37] |
H. X. Yang, Z. X. Wu, W. B. Du, Evolutionary games on scale-free networks with tunable degree distribution, EPL, 99 (2012), 10006. https://doi.org/10.1209/0295-5075/99/10006 doi: 10.1209/0295-5075/99/10006
![]() |
[38] |
E. R. Colman, G. J. Rodgers, Complex scale-free networks with tunable power-law exponent and clustering, Physica A, 392 (2013), 5501–5510. https://doi.org/10.1016/j.physa.2013.06.063 doi: 10.1016/j.physa.2013.06.063
![]() |
[39] |
L. Wang, G. F. Li, Y. H. Ma, L. Yang, Structure properties of collaboration network with tunable clustering, Inf. Sci., 506 (2020), 37–50. https://doi.org/10.1016/j.ins.2019.08.002 doi: 10.1016/j.ins.2019.08.002
![]() |
[40] |
C. P. Warren, L. M. Sander, I. M. Sokolov, Geography in a scale-free network model, Phys. Rev. E, 66 (2002), 056105. https://doi.org/10.1103/PhysRevE.66.056105 doi: 10.1103/PhysRevE.66.056105
![]() |
[41] |
J. M. Kumpula, J. P. Onnela, J. Saramäki, K. Kaski, J. Kertész, Emergence of communities in weighted networks, Phys. Rev. Lett., 99 (2007), 228701. https://doi.org/10.1103/PhysRevLett.99.228701 doi: 10.1103/PhysRevLett.99.228701
![]() |
[42] |
Y. Murase, J. Török, H. H. Jo, K. Kaski, J. Kertész, Multilayer weighted social network model, Phys. Rev. E, 90 (2014), 052810. https://doi.org/10.1103/PhysRevE.90.052810 doi: 10.1103/PhysRevE.90.052810
![]() |
[43] | S. Wolfram, Cellular automata and complexity: Collected papers, Westview Press, Boulder, 1994. |
[44] |
A. Landherr, B. Friedl, J. Heidemann, A critical review of centrality measures in social networks, Bus. Inf. Syst. Eng., 2 (2010), 371–385. https://doi.org/10.1007/s12599-010-0127-3 doi: 10.1007/s12599-010-0127-3
![]() |
[45] | L. Ljung, System identification: Theory for the user, Prentice-Hall, Upper Saddle River, 1998. |
[46] |
A. Clauset, C. R. Shalizi, M. E. J. Newman, Power-law distributions in empirical data, SIAM Rev., 51 (2009), 661–703. https://doi.org/10.1137/070710111 doi: 10.1137/070710111
![]() |
[47] |
F. Liljeros, C. R. Edling, L. A. N. Amaral, H. E. Stanley, Y. Aberg, The web of human sexual contacts, Nature, 411 (2001), 907–908. https://doi.org/10.1038/35082140 doi: 10.1038/35082140
![]() |
1. | Xinyuan Luo, Jian Yin, Danqi Wei, Complex-Systems Analysis of the CSI 300 Index: Evolution, Resilience, and Prediction in Stock Correlation Network, 2024, 12, 2079-8954, 285, 10.3390/systems12080285 |
ktar | 100×⟨Cc⟩ | 100×⟨Cb⟩ | ⟨ℓ⟩ | 100×⟨c⟩ | ⟨k⟩ | Δk |
20 | 23.90±0.03 | 0.06511±0.00003 | 4.189±0.008 | 6.513±0.003 | 20.1±0.3 | 29.20±0.03 |
45 | 28.59±0.02 | 0.05103±0.00002 | 3.499±0.005 | 11.687±0.004 | 45.2±0.2 | 56.4±0.1 |
100 | 31.72±0.03 | 0.04397±0.00002 | 3.153±0.006 | 22.352±0.008 | 100.3±0.1 | 83.80±0.05 |
r | 100×⟨Cc⟩ | 100×⟨Cb⟩ | ⟨ℓ⟩ | 100×⟨c⟩ | ⟨k⟩ | Δk |
5 | 16.50±0.02 | 0.10339±0.00004 | 6.064±0.004 | 16.937±0.004 | 20.0±0.1 | 28.20±0.03 |
20 | 29.24±0.03 | 0.04956±0.00001 | 3.427±0.003 | 2.826±0.002 | 20.2±0.2 | 34.10±0.06 |
60 | 31.74±0.02 | 0.04406±0.00002 | 3.158±0.004 | 1.278±0.001 | 20.0±0.1 | 89.40±0.08 |
γ | 100×⟨Cc⟩ | 100×⟨Cb⟩ | ⟨ℓ⟩ | 100×⟨c⟩ | ⟨k⟩ | Δk |
0.3 | 24.63±0.02 | 0.06286±0.00003 | 4.079±0.006 | 7.084±0.003 | 21.5±0.8 | 14.20±0.05 |
2.5 | 22.51±0.02 | 0.07046±0.00004 | 4.451±0.008 | 5.911±0.002 | 15.8±0.1 | 28.40±0.01 |
8.0 | 21.48±0.03 | 0.07469±0.00004 | 4.658±0.009 | 4.413±0.003 | 15.3±0.2 | 5.080±0.003 |
ktar | 100×⟨Cc⟩ | 100×⟨Cb⟩ | ⟨ℓ⟩ | 100×⟨c⟩ | ⟨k⟩ | Δk |
20 | 23.90±0.03 | 0.06511±0.00003 | 4.189±0.008 | 6.513±0.003 | 20.1±0.3 | 29.20±0.03 |
45 | 28.59±0.02 | 0.05103±0.00002 | 3.499±0.005 | 11.687±0.004 | 45.2±0.2 | 56.4±0.1 |
100 | 31.72±0.03 | 0.04397±0.00002 | 3.153±0.006 | 22.352±0.008 | 100.3±0.1 | 83.80±0.05 |
r | 100×⟨Cc⟩ | 100×⟨Cb⟩ | ⟨ℓ⟩ | 100×⟨c⟩ | ⟨k⟩ | Δk |
5 | 16.50±0.02 | 0.10339±0.00004 | 6.064±0.004 | 16.937±0.004 | 20.0±0.1 | 28.20±0.03 |
20 | 29.24±0.03 | 0.04956±0.00001 | 3.427±0.003 | 2.826±0.002 | 20.2±0.2 | 34.10±0.06 |
60 | 31.74±0.02 | 0.04406±0.00002 | 3.158±0.004 | 1.278±0.001 | 20.0±0.1 | 89.40±0.08 |
γ | 100×⟨Cc⟩ | 100×⟨Cb⟩ | ⟨ℓ⟩ | 100×⟨c⟩ | ⟨k⟩ | Δk |
0.3 | 24.63±0.02 | 0.06286±0.00003 | 4.079±0.006 | 7.084±0.003 | 21.5±0.8 | 14.20±0.05 |
2.5 | 22.51±0.02 | 0.07046±0.00004 | 4.451±0.008 | 5.911±0.002 | 15.8±0.1 | 28.40±0.01 |
8.0 | 21.48±0.03 | 0.07469±0.00004 | 4.658±0.009 | 4.413±0.003 | 15.3±0.2 | 5.080±0.003 |