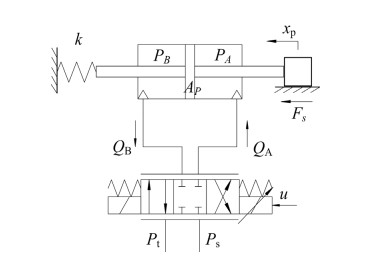
Electro-hydraulic servo system (EHSS) plays an important role in many industrial and military applications. However, its high-performance tracking control is still a challenging mission due to its nonlinear system dynamics and model uncertainties. In this paper, a novel adaptive robust integral method of the sign of the error (ARISE) with extended state observer (ESO) is proposed. Firstly, the nonlinear mathematical model of typical EHSS with modeling uncurtains and uncertain nonlinear is established. Then, ESO is used to estimate the state and lumped disturbance, of which the unknown parameter estimations can be updated by the novel adaptive law. Results shows that the novel controller achieves better tracking performance in maximum tracking error, average tracking error and standard deviation of the tracking error.
Citation: Xiaohan Yang, Yinghao Cui, Zhanhang Yuan, Jie Hang. RISE-based adaptive control of electro-hydraulic servo system with uncertain compensation[J]. Mathematical Biosciences and Engineering, 2023, 20(5): 9288-9304. doi: 10.3934/mbe.2023407
[1] | Xinyu Shao, Zhen Liu, Baoping Jiang . Sliding-mode controller synthesis of robotic manipulator based on a new modified reaching law. Mathematical Biosciences and Engineering, 2022, 19(6): 6362-6378. doi: 10.3934/mbe.2022298 |
[2] | Xiangfei Meng, Guichen Zhang, Qiang Zhang . Robust adaptive neural network integrated fault-tolerant control for underactuated surface vessels with finite-time convergence and event-triggered inputs. Mathematical Biosciences and Engineering, 2023, 20(2): 2131-2156. doi: 10.3934/mbe.2023099 |
[3] | Xiaoqiang Dai, Hewei Xu, Hongchao Ma, Jianjun Ding, Qiang Lai . Dual closed loop AUV trajectory tracking control based on finite time and state observer. Mathematical Biosciences and Engineering, 2022, 19(11): 11086-11113. doi: 10.3934/mbe.2022517 |
[4] | Yi Zhang, Yue Song, Song Yang . T-S fuzzy observer-based adaptive tracking control for biological system with stage structure. Mathematical Biosciences and Engineering, 2022, 19(10): 9709-9729. doi: 10.3934/mbe.2022451 |
[5] | Chengxi Wu, Yuewei Dai, Liang Shan, Zhiyu Zhu, Zhengtian Wu . Data-driven trajectory tracking control for autonomous underwater vehicle based on iterative extended state observer. Mathematical Biosciences and Engineering, 2022, 19(3): 3036-3055. doi: 10.3934/mbe.2022140 |
[6] | Chao Wang, Cheng Zhang, Dan He, Jianliang Xiao, Liyan Liu . Observer-based finite-time adaptive fuzzy back-stepping control for MIMO coupled nonlinear systems. Mathematical Biosciences and Engineering, 2022, 19(10): 10637-10655. doi: 10.3934/mbe.2022497 |
[7] | Xingjia Li, Jinan Gu, Zedong Huang, Chen Ji, Shixi Tang . Hierarchical multiloop MPC scheme for robot manipulators with nonlinear disturbance observer. Mathematical Biosciences and Engineering, 2022, 19(12): 12601-12616. doi: 10.3934/mbe.2022588 |
[8] | Vladimir Djordjevic, Hongfeng Tao, Xiaona Song, Shuping He, Weinan Gao, Vladimir Stojanovic . Data-driven control of hydraulic servo actuator: An event-triggered adaptive dynamic programming approach. Mathematical Biosciences and Engineering, 2023, 20(5): 8561-8582. doi: 10.3934/mbe.2023376 |
[9] | Na Zhang, Jianwei Xia, Tianjiao Liu, Chengyuan Yan, Xiao Wang . Dynamic event-triggered adaptive finite-time consensus control for multi-agent systems with time-varying actuator faults. Mathematical Biosciences and Engineering, 2023, 20(5): 7761-7783. doi: 10.3934/mbe.2023335 |
[10] | Chaoyue Wang, Zhiyao Ma, Shaocheng Tong . Adaptive fuzzy output-feedback event-triggered control for fractional-order nonlinear system. Mathematical Biosciences and Engineering, 2022, 19(12): 12334-12352. doi: 10.3934/mbe.2022575 |
Electro-hydraulic servo system (EHSS) plays an important role in many industrial and military applications. However, its high-performance tracking control is still a challenging mission due to its nonlinear system dynamics and model uncertainties. In this paper, a novel adaptive robust integral method of the sign of the error (ARISE) with extended state observer (ESO) is proposed. Firstly, the nonlinear mathematical model of typical EHSS with modeling uncurtains and uncertain nonlinear is established. Then, ESO is used to estimate the state and lumped disturbance, of which the unknown parameter estimations can be updated by the novel adaptive law. Results shows that the novel controller achieves better tracking performance in maximum tracking error, average tracking error and standard deviation of the tracking error.
Ap | Ram area |
Ct | Internal leakage coefficient |
P1, P2, | Oil pressures inside the chambers of the actuator, respectively |
PL | Load pressure |
Pr | Return pressure |
Ps | Supply pressure |
Q1, Q2 | Oil flow in both chambers of the actuator |
V01, V02 | Initial volume of oil inlet and outlet chamber, respectively |
V1, V2 | Control volume of oil inlet and outlet chamber, respectively |
fv | Damping coefficient |
g1, g2 | Pressure difference of inlet and outlet |
k | Spring stiffness |
k1, k2, k3, kr | Positive gains |
kt | Total gain of the servo valve |
mp | Mass of load |
q1, q2 | Model errors because of internal leakage |
u | Control voltage |
xp | Position of load |
β | Gain |
βe1, βe2 | Oil effective bulk modulus of the actuator, respectively |
ω | Bandwidth of the extended state observer |
ARISE | Adaptive robust integral of the sign of the error |
ESO | Extended state observer |
RISE | Robust integral of the sign of the error |
Electric hydraulic servo system (EHSS) is widely used in industry applications due to its high power ratio and fast response [1,2,3,4,5,6]. With the rapid development of technology, more severe control requirements are put forward for EHSS, and high-performance control becomes eagerly needed to address its behavioral nonlinearity and uncertainties, such as flow nonlinearity, pressure dynamic nonlinearity, uncertain parameters, unmolded nonlinearity, and external disturbances [7,8,9,10].
Nowadays, many researchers focus on advanced control strategies such as robust adaptive control [11,12], sliding mode control [13,14], back-stepping control [15,16], robust integral of the sign of the error (RISE) [17,18] for EHSS. Yue and Yao [17] proposed an adaptive robust integral of the sign of the error control (ARISE), which can adjust the robust gain online through adaptive method to solve the potential high gain feedback of symbolic function. Yao et al. [19] designed a novel ARSE to address noise pollution in the acquisition of acceleration signal, which can compensate the error of friction model and other bounded disturbances [20]. The above literature shows that RISE/ARISE control greatly improves the trajectory tracking accuracy of EHSS. However, those controllers regard the parameter adaptive error, unmolded error, and external disturbance as lumped disturbance, which severely limits the control accuracy. Control strategy based on disturbance observer can compensate the influence of disturbance and uncurtains effectively, which has been used in the field of control theory and engineering [21,22,23,24,25,26]. Especially, the extended state observer (ESO), which is the core of ADRC and has been widely used in disturbance estimation and suppression [27,28,29,30,31,32].
In this paper, the sign function is replaced with a modified arctangent function to smooth the nonlinearity of sign function. Then, the state and external disturbance can be estimated by ESO, respectively. Finally, the residual observation error is compensated to further enhance the tracking accuracy by ARISE. The Lyapunov theory proves the EHSS can achieve asymptotic s. Simulation results show the proposed controller has a better performance in maxi-mum tracking error, average tracking error and standard deviation of the tracking error.
The typical working principle of EHSS is shown in Figure 1. The double rod symmetrical hydraulic cylinder is controlled by servo valve to drive the load.
In this paper, the force balance equation of EHSS can be given as
mp¨xp=PLAp−kxp−fv˙xp+f(xp,˙xp,t) | (1) |
where mp and xp represent mass and displacement of load respectively; PL is the pressure difference; Ap is the effective area of the piston; k is the spring stiffness; fv is the combined coefficient; f(xp,˙xp,t) indicates the lumped uncertain; Pressure dynamics of the two chambers are given by [15].
{˙P1=βe1/βe1V1V1(Q1−Ap˙xp−CtPL+q1(t))˙P2=βe2/βe2V2V2(Ap˙xp+CtPL−Q2−q2(t)) | (2) |
where
{Q1=ktg1(P1,xv)uQ2=ktg2(P2,xv)u | (3) |
{g1=√(Ps−Pt+(Ps−2P1+Pt)⋅s(u))/2g2=√(Ps−Pt−(Ps−2P2+Pt)⋅s(u))/2 | (4) |
The pressure difference dynamics between the two chambers is expressed as follows
˙PL=βekt(g1/g1V1+g2/g2V2V2V1+g2/g2V2V2)u−βeAp(1/1V1+1/1V2V2V1+1/1V2V2)˙xp−βeCt(1/1V1+1/1V2V2V1+1/1V2V2)PL+βe(q1/q1V1+q2/q2V2V2V1+q2/q2V2V2) | (5) |
where βe1, βe2 are the effective elastic modulus in two chamber and βe1 = βe2 = βe; V1 = V01 + Ap; V2 = V02 - Ap·xp represents the control volume of return chamber; V01 and V02 are the initial volumes of the two chambers respectively; Ct is the internal leakage coefficient of the cylinder; Q1 (t) and Q2 (t) are the oil flowrate of the two chamber of the cylinder respectively; q1(t) and q2(t) are model errors because of internal leakage in the two chambers; g1 is the pressure difference at the oil inlet and g2 is the pressure difference at the oil outlet; Ps is the supplied pressure; Pr is the return pressure, kt is the total gain of the servo valve; u is the control voltage and s(u) is expressed as
s(u)={1u>00u=0−1u<0 | (6) |
To make it smooth and differentiable, the sign function s(u) is replaced by Eq (7).
farctan(u)=2arctan(Ku)/π | (7) |
Thus, g1, g2 in Eq (4) can be rewritten as Eq (8).
{g′1=√(Ps−Pt+(Ps−2P1+Pt)⋅farctan(u))/2g′2=√(Ps−Pt−(Ps−2P2+Pt)⋅farctan(u))/2 | (8) |
Substituting Eqs (2)-(5) into Eq (1), and thus
mp⃛xp=Apβukl(g1/V1+g2/V2)u−A2pβu(1/V1+1/V2)ˉxp−ApβeCt(1/V1+1/V2)PL+Apβu(q1/V1+q2/V2)−k˙xp−fv¨xp+˙f | (9) |
Rewritten Eq (9), and thus,
mp⃛xp=Apβukt(g′1/V1+g2/V2)u−A2pβe(1/V1+1/V2)ˉxp−ApβeCt(1/V1+1/V2)FL+Apβe(q1/V1+q2/V2)−k˙xp−fv¨xp+˙f+f′ | (10) |
where f′ is the approximation error caused by using the continuously differentiable function farctan(u).
According to Eq (1)
PL=mpAp¨xp+kApxp+fvAp˙xp−fAp | (11) |
Substituting Eq (11) into Eq (10), thus
mp⃛xp=Apβekt(g′1/V1+g′2/V2)u−βeCt(1/V1+1/V2)kxp−(A2pβe(1/V1+1/V2)+fvβeCt(1/V1+1/V2)+k)˙xp−(mpβeCt(1/V1+1/V2)+fv)¨xp+βeCt(1/V1+1/V2)f+˙f+f′+Apβe(q1/V1+q2/V2) | (12) |
Define state variables as x=[x1,x2,x3]T=[xp,˙xp,¨xp]T and output variables as y=x1=xp, so the state space model of EHSS can be expressed as
{˙x1=x2˙x2=x3˙x3=θ1u−θ2x1−θ3x2−θ4x3+Δ | (13) |
where θ1=Apβekt(g′1V1+g′1V2)/mp,θ2=βeCt(1V1+1V2)k/mp, θ3=(A2pβe(1V1+1V2)+fvβeCt(1V1+1V2)+k)/mp, θ4=(mpβeCt(1V1+1V2)+fv)/mp,Δ=(βeCt(1V1+1V2)f+˙f+f′+Apβe(q1V1+q1V2))/mp.
In practice, the parameters m, k, βe and Ct may not be known accurately, so it is necessary to consider the uncertainties of these parameters. Define vector as θ=[θ1,θ2,θ3,θ4] and improve the tracking performance of the system through the adaptive method.
The purpose of the system controller is to design a bounded control input u so that y = x1 can track the desired trajectory yd(t) = x1d(t). Therefore, the following assumptions should be given.
Assumption 1: The desired trajectory x1d is five times differentiable and each is bounded. In practice, the load pressure of hydraulic cylinder meets 0 < PL < Ps.
Assumption 2: The range of parametric uncertainties is
θ∈Ωθ | (14) |
ΩθΔ{θ:θmin≤θ≤θmax} | (15) |
where θmin=[θ1min,⋯,θ4min]T, θmax=[θ1max,⋯,θ4max]T are known.
Assumption 3: The time-varying perturbation Δ (t) of Eq (10) is smooth enough so that
∣˙Δ(t)∣≤δ1&∣¨Δ(t)∣≤δ2 | (16) |
where δ1, δ2 are known positive constants.
ESO can estimate the uncertainty disturbance comprehensively, so we use ESO to estimate the lumped disturbance and compensate it feed forward to achieve better tracking accuracy.
Define x4 = Δ(t), ˙x4=δ(t) and the expanded state space can be written as
{˙x1=x2˙x2=x3˙x3=θ1u−θ2x1−θ3x2−θ4x3+x4˙x4=δ | (17) |
Defining ˆx=x−ˆx as the estimation error of x, where ˆx represents the estimated value of x.
According to the expanded state space model, the ESO is designed as
{˙ˆx1=ˆx2+4ω0(x1−ˆx1)˙ˆx2=ˆx3+6ω20(x1−ˆx1)˙ˆx3=ˆθ1u−ˆθ2x1−ˆθ3x2−ˆθ3x3+ˆx4+4ω30(x1−ˆx1)˙ˆx4=ω40(x1−ˆx1) | (18) |
where ω0 is the bandwidth of the extended state observer, ˆθi is the estimated value of the unknown parameters θi, I = 1, 2, 3, 4.
The dynamic equation of observation error can be obtained by subtracting Eq (17) an Eq (18):
{˙˜x1=˜x2−4ϖ0˜x1˙˜x2=˜x3−6ω20˜x1˙˜x3=˜x4+˜θTΦ1−4ω30˜x1˙˜x4=δ−ω40˜x1 | (19) |
where ˜θ=[˜θ1,˜θ2,˜θ3,˜θ4]T, Φ1=[u,x1,x2,xξ,x4]T.
Let
ξi=˜xiωi−10,i=1,2,3,4 | (20) |
Then Eq (17) can be written as
[˙ξ1˙ξ2˙ξ3˙ξ4]=ϖ0[−4100−6010−4001−1000]⏟A[ξ1ξ2ξ3ξ4]+[0010]⏟B1˜θTΦϖ20+[0001]⏟B2δϖ30 | (21) |
˙ξ=ω0Aξ+B1˜θTΦω20+B2δϖ30 | (22) |
Since matrix A is a Hurwitz matrix, and there is a positive definite symmetric matrix P which satisfies the following equation
ATP+PA=−I | (23) |
The symmetric positive definite matrix P is:
P=[17/1788−1/122−11/11881/122−1/12211/1188−1/122−17/−1788−11/1188−1/12217/1788−1/1221/122−17/−1788−1/12291/9188] |
Considering Eq (14) and Eq (15), the discontinuous projection can be defined as [11].
Projˆαi(⋅i)={0,⋅i>0andˆαi=αimax0,⋅i<0andˆαi=αimin⋅i,otherwise | (24) |
where ˆα denote the estimate of α and ˜α denote the estimate error, ˜α=ˆα−α,i=1,2,3,4.
Using the adaptation law as follow:
˙ˆα=Projˆα(Γτ(t)),ˆα(0)=Ωˆα | (25) |
where Γ is the diagonal positive definite adaptation rate matrix, τ is an adaptation function. For any adaption function τ, the adaptation Eq (25) satisfies follow [3]:
ˆα∈Ω˙α≜{ˆα:αmax≤ˆα≤αmin} | (26) |
˜αT[Γ−1Projˆα(Γτ)−τ]≤0,∀τ | (27) |
Defining the following error variables
{z1=x1−x1d,z2=˙z1+k1z1z3=˙z2+k2z2,r=˙z3+k3z3 | (28) |
where x1d is the given trajectory; k1 k2 k3 are the positive feedback gain and r is the auxiliary error signal. Because r contains the differentiation of acceleration, it is considered to be unmeasurable in practice and only used for auxiliary design. According to Eq (28), r has the following expansion:
r=θ1u−θ2x1 d−θ3˙x1 d−θ4¨xld+Δ−⃛xld−(θ2−k1θ3−k31+θ4k21)z1−(k21+k1k2−k22θ3−θ4k1−θ4k2)z2+(k1+k2+k3−θ4)z3 | (29) |
Dividing Eq (29) by θ1, and thus
α1r=u−α1⃛x1 d−α2x1 d−α3˙xld−α4¨x1 d+α1Δ−(α2−k1α3−α1k31+α4k21)z1−((k21+k1k2)α1−k22α3−α4k1−α4k2)z2+((k1+k2+k3)α1−α4)z3 | (30) |
where α1=1/1θ1θ1, α2=θ2/θ2θ1θ1, α3=θ3/θ3θ1θ1, α4=θ4/θ4θ1θ1.
The model-based controller is designed as follows:
{u=ua+us,us=(us1+us2),us1=−k3z3us2=−krz3+krz3(0)−∫to[krk3z3(τ)+βS(z3(τ))]dτua=ˆα1⃛x1d+ˆα2x1d+ˆα3˙x1d+ˆα4¨x1d−ˆα1ˆΔ=ˆαTΦ2−ˆα1ˆΔ | (31) |
where ˆα=[ˆα1,ˆα2,ˆα3,ˆα4]T represents the estimated value of α=[α1,α2,α3,α4]T; kr>0 is the gain of controller; β>0 is the robust gain; ua is the feedforward model compensation, us1 is the linear robust feedback term, us2 is the RISE control term, Φ2=[⃛x1d,x1d,˙x1d,¨x1d]T.
Substituting Eq (31) into Eq (30) and note that d=−ˆα1ˆΔ+α1Δ
α1r=˜αTΦ2+d−(k3+kr)z3+krz3(0)−∫to[krk3z3(τ)+βS(z3(τ))]dτ−Az1−Bz2+Cz3 | (32) |
˜α=ˆα−α, Substitute Eq (25) into Eq (32), thus
α1˙r=Projˆα(Γτ)TΦ2+˜αT˙Φ2+˙d−(k3+kr−C)r−βS(z3)+Ak1z1−(A−Bk2)z2−(B+Ck3)z3 | (33) |
The overall structure of the designed control strategy is shown in Figure 2.
Lemma 1: Define variable L(t) as
L(t)=r[˙d−βsign(z3)] | (34) |
Define auxiliary function as
P(t)=β|z3(0)|−z3(0)˙d−∫t0L(v)dv | (35) |
According to [8], if the gain β satisfies the following inequality, then the auxiliary function P(t) is always positive definite.
β⩾ | (36) |
Theorem 1: Using the adaptive law Eq (25), and adaptive function \tau = - r{{\boldsymbol{\dot \Phi }}_2} , and the robust gain β satisfies inequality Eq (36) as well as the feedback gains k1, k2, k3, kr are sufficient to ensure that the matrix {\Lambda } defined below is positive definite, the adaptive robust integral of the sign of the error controller Eq (31) can make all signals bounded in the closed-loop system, and the system obtains asymptotic output tracking, i.e., {z_1} \to 0 as t \to \infty .
{\boldsymbol{\varLambda}} = \left[ {\begin{array}{*{20}{c}} {{k_1}}&{ - \frac{1}{2}}&0&{ - \frac{1}{2}{k_5}} \\ { - \frac{1}{2}}&{{k_2}}&{ - \frac{1}{2}}&{ - \frac{1}{2}{k_6}} \\ 0&{ - \frac{1}{2}}&{{k_3}}&{ - \frac{1}{2}{k_{67}}} \\ { - \frac{1}{2}{k_5}}&{ - \frac{1}{2}{k_6}}&{ - \frac{1}{2}{k_7}}&{{k_4}} \end{array}} \right] | (37) |
where {k_4} = \max \left({{{{\dot {\boldsymbol{\varPhi}} }}_2}^{\rm T}\boldsymbol{\varGamma }{{\boldsymbol{\varPhi} }_2}} \right) + {k_3} + {k_r} - C , {k_5} = A{k_1} , {k_6} = - \left({A - B{k_2}} \right) , {k_7} = - \left({B + C{k_3}} \right) , \max \left(\cdot \right) represents the maximum value of the matrix.
Proof: see Appendix A.
The nominal value of the physical parameters of the valve controlled symmetrical hydraulic cylinder are shows in Table 1. The following controllers are compared by simulation to validate the effectiveness of the designed controller.
Parameter | Value | Unit | Parameter | Value | Unit |
mp | 0.76167 | kg | fv | 100 | N/(m/s) |
Ap | 2.5 × 10-4 | m2 | V10 | 1 × 10-3 | m3 |
k | 10900 | N/m | V20 | 1 × 10-3 | m3 |
βe | 2 × 108 | Pa | kt | 5.656 × 10-8 | m3/(s·V·N1/2) |
Ct | 1 × 10-13 | m3/(Pa·s) | q1 | 1 × 10-12 | m3/s |
q2 | 1 × 10-12 | m3/s | Cd | 0.7 | |
Cv | 1 | / | Wp | 5 | e-3 |
Δp | 821, 993 | / | α | 69 | ° |
K | 1000 |
(1) Controller I: ESO based ARISE This is the controller designed in this paper. The controller parameters are selected as: k1 = 200, k2 = 180, k3 = 0.08, kr = 0.012, β = 0.05, ω = 100. According to the nominal value of the parameters, the estimated boundary of unknown parameter α are given as: αmin = [5 × 10-5 0.03 2.5 8 × 10-3] and αmax = [7 × 10-5 0.07 3.5 20.2 × 10-3]. The initial estimates of α is set as \hat \alpha (0) = [6.5×10-5 0.04 3 8.2×10-3] and Γ is set as diag[1 × 10-15 2 × 10-2 0.001 5 × 10-10].
(2) Controller II: ARISE without ESO. Compared to the controller II, there is no ESO compensation term and the other parameters are same to controller I. That is only uα in Eq (18) is replaced as: {u_a} = {\hat \alpha _1}{\dddot x_{1{\text{d}}}} + {\hat \alpha _2}{x_{1{\text{d}}}} + {\hat \alpha _3}{\dot x_{1{\text{d}}}} + {\hat \alpha _4}{\ddot x_{1{\text{d}}}} = {\hat \alpha ^{\rm T}}{{\boldsymbol{\varPhi }}_2}
(3) Controller III: PI controller. The parameters are set as kP = 410 and kI = 10, which are the optimal solutions after repeated debugging.
(4) Controller IV: BP neutral network PID controller. The structure of the neutral network is 3-5-3, and the learning rate \eta = 0.28 , inertia coefficient α = 0.3.
The desired trajectories are designed as three cases: normal motion with the motion trajectory
{x_{\text{d}}}\left(t \right) = {{10\arctan \left[{\sin \left({\pi t} \right)} \right]\left({1 - {e^{ - t}}} \right)} \mathord{\left/ {\vphantom {{10\arctan \left[{\sin \left({\pi t} \right)} \right]\left({1 - {e^{ - t}}} \right)} {0.7854}}} \right. } {0.7854}} mm, fast level motion with the motion trajectory {x_{\text{d}}}\left(t \right) = {{10\arctan \left[{\sin \left({4\pi t} \right)} \right]\left({1 - {e^{ - t}}} \right)} \mathord{\left/ {\vphantom {{10\arctan \left[{\sin \left({4\pi t} \right)} \right]\left({1 - {e^{ - t}}} \right)} {0.7854}}} \right. } {0.7854}} mm and low-level motion with the motion trajectory {x_{\text{d}}}\left(t \right) = {{10\arctan \left[{\sin \left({0.2\pi t} \right)} \right]\left({1 - {e^{ - t}}} \right)} \mathord{\left/ {\vphantom {{10\arctan \left[{\sin \left({0.2\pi t} \right)} \right]\left({1 - {e^{ - t}}} \right)} {0.7854}}} \right. } {0.7854}} mm. The external disturbance is designed as f\left(t \right) = {{20\arctan \left[{\sin \left({0.8\pi t} \right)} \right]\left({1 - {e^{ - t}}} \right)} \mathord{\left/ {\vphantom {{20\arctan \left[{\sin \left({0.8\pi t} \right)} \right]\left({1 - {e^{ - t}}} \right)} {0.7854}}} \right. } {0.7854}} N.
To compare the tracking responses of each controller quantitatively, three performance indices including maximum absolute value of the tracking error Me, average tracking error {\mu _{\text{e}}} , standard deviation of the tracking error σe, which were defined in are adopted to evaluate [20].
(1) Case I-normal level motion
The four controllers are tested for a normal motion trajectory xd(t) = 10arctan[sin(πt)] [1-exp(-t)]/0.7854 mm. The tracking performance are shown in Figures 4-6, the performance indices of the four controllers is shown in Table 2. From the Figures 4-6 and Table 1, it is obviously that the valve controlled symmetrical cylinder has the best tracking performance under the controller designed in this paper than other controllers. From Table 2, the amplitudes of steady-state tracking error of the controller III and controller IV are both about 0.6 mm, while controller I is about 0.003 mm and controller II is about 0.01 mm, it shows that the ARISE can deal with nonlinear and uncertainties and disturbance well but PI controller just has some robustness. By comparing the performance indices in Table 2 and tracking error in Figure 6 of controller I and controller II, it can be seen that controller I is better than controller II in all indices obviously, which indicates that the parameter adaptation in Figure 6 and ESO compensates for both parametric and uncertain lumped disturbance are effective. The control input u of controller I showed in Figure 3 is continuous and smooth, which makes it easy to implement in practice.
Indices | Me (mm) | μe (mm) | σe (mm) |
controller I | 0.00304985 | 0.000778897 | 0.00084379 |
controller II | 0.010971 | 0.00364635 | 0.00293964 |
controller III | 0.63097 | 0.299917 | 0.197092 |
controller IV | 0.630986 | 0.299916 | 0.197092 |
(2) Case II-low level motion
In this case, a low level reference trajectory xd(t) = 10arctan[sin(0.2πt)][1-exp(-t)]/0.7854 mm is tested. The results are shown in Figures 7-9 and the performance indices are listed in Table 3. From Figure 7 and Table 3, the amplitudes of steady-state tracking error of the controller III and controller IV are both about 0.076mm, while controller I and controller II both are about 9.5 × 10-5 mm, which shows that the ARISE can also deal with nonlinear and uncertainties and disturbance well in low level reference trajectory. By comparing the performance indices of controller I and controller II in Table 3, it can be seen that the maximum absolute value of the tracking error Me of the two controllers almost the same, but the average tracking error μe, and standard deviation of the tracking error σe of controller I are 6.536 × 10-6 mm and 8.833 × 10-6 mm respectively which are better than that of 2.87371 × 10-5 and 1.02822 × 10-5 of controller II obviously, which further validates the effectiveness of the desired parameter adaptation and ESO compensation. The parameter adaptation of controller I are omitted. The control input u of controller I showed in Figure 8 is continuous and there is slight high-frequency vibration. So the controller designed in this paper has the best tracking performance on low level reference trajectory than other controllers too.
Indices | Me(mm) | μe(mm) | σe(mm) |
controller I | 9.508 × 10-5 | 6.536 × 10-6 | 8.833 × 10-6 |
controller II | 9.58784 × 10-5 | 2.87371 × 10-5 | 1.02822 × 10-5 |
controller III | 7.5716 × 10-2 | 3.14012 × 10-2 | 2.00589 × 10-2 |
controller IV | 7.57345 × 10-2 | 3.13926 × 10-2 | 2.00719 × 10-2 |
(3) Case III-fast level motion
A faster level reference trajectory xd(t) = 10arctan[sin(4πt)][1-exp(-t)]/0.7854 mm is tested in this case. The results are shown in Figured 10-12 and the performance indices are listed in Table 4. From Figure 12 and Table 4, the amplitudes of steady-state tracking error of the controller III and controller IV are both about 1.24 mm, while controller I 1.34 × 10-2 mm and controller II 4.20256 × 10-2 mm, which shows that the ARISE can better deal with nonlinear and uncertainties and disturbance in fast level reference trajectory than PI controller too. In addition, comparing the performance indices of controller I and controller II in Table 4, it can be seen that the maximum absolute value of the average tracking error μe of the controller I is 4.14 × 10-3 mm and the controller II is 1.63032 × 10-2 mm, the standard deviation of the tracking error σe of controller I are 5.53 ×10-3 mm and the controller II is 1.30355 × 10-2 mm. These further validate the effectiveness of the desired parameter adaptation and ESO compensation. The control input u of controller I showed in Figure 11 is continuous and smooth. The result verifies that the controller designed in this paper still has high tracking accuracy in tracking performance on fast level reference trajectory.
Indices | Me(mm) | μe(mm) | σe(mm) |
controller I | 1.34 × 10-2 | 4.14 × 10-3 | 5.53 × 10-3 |
controller II | 4.20256 × 10-2 | 1.63032 × 10-2 | 1.30355 × 10-2 |
controller III | 1.238558 | 0.598359 | 0.391225 |
controller IV | 1.238657 | 0.598359 | 0.391224 |
In this paper, an ARISE with ESO controller is proposed for EHSS to address parametric uncertainties, uncertainty nonlinearities and unmolded disturbances. The proposed ARISE can compensate the dynamics uncertainties, thus guaranteeing asymptotic tracking and improving the adaptability and safety of EHSS. ESO can effectively estimate the state and lumped uncurtains. Simulation results shows that ARISE with ESO can obtain high tracking accuracy and better performance in tracking desired trajectory under all working conditions.
The authors declare there is no conflict of interest.
Define a Lyapunov function
V = \frac{1}{2} z_1^2+\frac{1}{2} z_2^2+\frac{1}{2} z_3^2+\frac{1}{2} \alpha_1 r^2+\frac{1}{2} \tilde{\boldsymbol{\alpha}}^{\mathrm{T}} \boldsymbol{\varGamma}^{-1} \tilde{\boldsymbol{\alpha}}+P | (A.1) |
It is Obvious that V is positive definite. The derivative of V is:
\dot{V} = z_1 \dot{z}_1+z_2 \dot{z}_2+z_3 \dot{z}_3+\alpha_1 r \dot{\eta}+\tilde{\boldsymbol{\alpha}}^{\mathrm{T}} \boldsymbol{\varGamma}^{-1} \dot{\hat{\boldsymbol{\alpha}}}+\dot{P} | (A.2) |
Substituting Eqs (25) and (28), L(t)and P(t), into Eq (A.2).
\begin{aligned} \dot{V} & = z_1\left(z_2-k_1 z_1\right)+z_2\left(z_3-k_2 z_2\right)+z_3\left(r-k_3 z_3\right)+ \\ & r\left\{-\dot{\boldsymbol{\varPhi}}_2^{\mathrm{T}} \boldsymbol{\varGamma} \boldsymbol{\Phi}_2 r+\tilde{\boldsymbol{\alpha}}^{\mathrm{T}} \dot{\boldsymbol{\varPhi}}_2+\dot{d}-\left(k_3+k_r-C\right) r-\beta \operatorname{sign}\left(z_3\right)+A k_1 z_1-\left(A-B k_2\right) z_2-\left(B+C k_3\right) z_3\right\} \\ & +\tilde{\boldsymbol{\alpha}}^T \boldsymbol{\varGamma}^{-1} \operatorname{Proj}_{\hat{\theta}}(\Gamma \tau)-r\left[\dot{d}-\beta \operatorname{sign}\left(z_3\right)\right] \\ & \leq z_1\left(z_2-k_1 z_1\right)+z_2\left(z_3-k_2 z_2\right)+z_3\left(r-k_3 z_3\right)+ \\ & r\left\{-\dot{\boldsymbol{\varPhi}}_2^{\mathrm{T}} \boldsymbol{\varGamma} \boldsymbol{\Phi}_2 r+\tilde{\boldsymbol{\alpha}}^{\mathrm{T}} \dot{\boldsymbol{\varPhi}}_2+\dot{d}-\left(k_3+k_r-C\right) r-\beta \operatorname{sign}\left(z_3\right)+A k_1 z_1-\left(A-B k_2\right) z_2-\left(B+C k_3\right) z_3\right\} \\ & +\tilde{\boldsymbol{\alpha}}^T \tau-r\left[\dot{d}-\beta \operatorname{sign}\left(z_3\right)\right] \\ & = -k_1 z_1^2-k_2 z_2^2-k_3 z_3^2-\left(\dot{\boldsymbol{\varPhi}}_2^{\mathrm{T}} \boldsymbol{\varGamma} \boldsymbol{\Phi}_2+k_3+k_r-C\right) r^2+z_1 z_2+z_2 z_3+z_3 r+\left(A k_1 z_1-A z_2\right) r+B k_2 z_2 r-\left(B+C k_3\right) z_3 r \\ & \triangleq-\boldsymbol{\eta}^{\mathrm{T}} \boldsymbol{\varLambda} \boldsymbol{\eta} \leq-\lambda_{\min }(\boldsymbol{\varLambda})\left(z_1^2+z_2^2+z_3^2+r^2\right) \triangleq-W \end{aligned} | (A.3) |
where \boldsymbol{\eta} = \left[z_1, z_2, z_3, r\right]^{\mathrm{T}}, \lambda \min (\boldsymbol{\varLambda}) is the minimum eigenvalue of matrix Λ, therefore V \in L_{\infty} and W \in L_2, so z1, z2, z3 and r are bounded. According to the assumptions 1 and 2, all states of the system are bounded so the actual control input u is bounded. According to Eqs (28) and (33), the derivative of W is bounded, so W is uniformly continuous. According to Barbarat's lemma, W→0 as t→∞, so the conclusion of the theorem 1 can be deduced, theorem 1 is proofed.
[1] |
Z. X. Jiao, J. X. Gao, Q. Hua, S. P. Wang, The velocity synchronizing control on the electro-hydraulic load simulator, Chin. J. Aeronaut., 17 (2004), 39–46. https://doi.org/10.1016/s1000-9361(11)60201-x doi: 10.1016/s1000-9361(11)60201-x
![]() |
[2] |
J. Koivumäki, J. Mattila, Stability-guaranteed impedance control of hydraulic robotic manipulators, IEEE-ASME Trans. Mech., 22 (2017), 601–612. https://doi.org/10.1109/tmech.2016.2618912 doi: 10.1109/tmech.2016.2618912
![]() |
[3] |
Y. H. Li, Z. L. Wang, Exact linearzization control for the displacement type of actuator with electrical and compound adjustment, Chin. J. Mech., 40 (2004), 21–25. https://doi.org/10.3321/j.issn:0577-6686.2004.11.005 doi: 10.3321/j.issn:0577-6686.2004.11.005
![]() |
[4] | J. M. Ma, Y. L. Fu, J. Li, B. Gao, Design simulation and analysis of integrated electrical hydrostatic actuator, Acta Aeronaut. Astronaut. Sin., 26 (2005), 79–83. |
[5] |
W. C. Sun, H. H. Pan, H. J. Gao, Filter-based adaptive vibration control for active vehicle suspensions with electrohydraulic actuators, IEEE Trans. Veh. Technol., 65 (2016), 4619–4626. https://doi.org/10.1109/TVT.2015.2437455 doi: 10.1109/TVT.2015.2437455
![]() |
[6] |
M. Law, M. Wabner, A. Colditz, M. Kolouch, S. Noack, S. Ihlenfeldt, Active vibration isolation of machine tools using an electro-hydraulic actuator, CIRP J. Manuf. Sci. Technol., 10 (2015), 36–48. https://doi.org/10.1016/j.cirpj.2015.05.005 doi: 10.1016/j.cirpj.2015.05.005
![]() |
[7] | H. E. Merritt, Hydraulic Control Systems, New York: Wiley, 1967 |
[8] |
B. Xian, D. M. Damson, M. S. de Queiroz, J. Chen, A continuous asymptotic tracking control strategy for uncertain nonlinear systems, IEEE Trans. Autom. Control., 49 (2004), 1206–1211. https://doi.org/10.1109/TAC.2004.831148 doi: 10.1109/TAC.2004.831148
![]() |
[9] |
J. Y. Yao, Z. X. Jiao, D. W. Ma, L. Yan, High-accuracy tracking control of hydraulic rotary actuators with modeling uncertainties, IEEE-ASME Trans. Mech., 19 (2014), 633–641. https://doi.org/10.1109/tmech.2013.2252360 doi: 10.1109/tmech.2013.2252360
![]() |
[10] | P. M. Patre, W. MacKunis, C. Makkar, W. E. Dixon, Asymptotic tracking for systems with structured and unstructured uncertainties, in Proceedings of the 45th IEEE Conference on Decision and Control, (2006), 441–446. https://doi.org/10.1109/cdc.2006.377377 |
[11] |
B. Yao, F. Bu, J. Reedy, G. T. C. Chiu, Adaptive robust motion control of single-rod hydraulic actuators: theory and experiments, IEEE-ASME Trans. Mech., 5 (2000), 79–91. https://doi.org/10.1109/3516.828592 doi: 10.1109/3516.828592
![]() |
[12] |
A. Mohanty, B. Yao, Indirect adaptive robust control of hydraulic manipulators with accurate parameter estimates, IEEE Trans. Control Syst. Technol., 19 (2011), 567–575. https://doi.org/10.1109/tcst.2010.2048569 doi: 10.1109/tcst.2010.2048569
![]() |
[13] |
J. Yang, J. Y. Su, S. H. Li, X. H. Yu, High-order mismatched disturbance compensation for motion control systems via a continuous dynamic sliding-mode approach, IEEE Trans. Ind. Inf., 10 (2014), 604–614. https://doi.org/10.1109/tii.2013.2279232 doi: 10.1109/tii.2013.2279232
![]() |
[14] |
D. Won, W. Kim, M. Tomizuka, High gain observer based integral sliding mode control for position tracking of electro-hydraulic servo systems, IEEE-ASME Trans. Mech., 22 (2017), 2695–2704. https://doi.org/10.1109/tmech.2017.2764110 doi: 10.1109/tmech.2017.2764110
![]() |
[15] |
J. Y. Yao, Z. X. Jiao, D. W. Ma, Extended-state-observer-based output feedback nonlinear robust control of hydraulic systems with backstepping, IEEE Trans. Ind. Electron., 61 (2014), 6285–6293. https://doi.org/10.1109/TIE.2014.2304912 doi: 10.1109/TIE.2014.2304912
![]() |
[16] |
W. Sun, H. J. Gao, O. Kaynak, Adaptive backstepping control for active suspension systems with hard constraints, IEEE-ASME Trans. Mech., 18 (2013), 1072–1079. https://doi.org/10.1109/tmech.2012.2204765 doi: 10.1109/tmech.2012.2204765
![]() |
[17] |
X. Yue, J. Y. Yao, Adaptive integral robust control of electro-hydraulic load simulator, Chin. Hydraul. Pneumatics, 12 (2016), 25–30. https://doi.org/10.11832 /j.issn.1000-4858.2016.12.004 doi: 10.11832/j.issn.1000-4858.2016.12.004
![]() |
[18] |
X. Yue, J. Y. Yao, Integral robust based asymptotic tracking control of electro-hydraulic load simulator, Acta Aeronaut. Astronaut. Sin., 38 (2017), 294–303. https://doi.org/10.7527/S1000-6893.2016.0152 doi: 10.7527/S1000-6893.2016.0152
![]() |
[19] |
J. Y. Yao, W. X. Deng, Z. X. Jiao, RISE-based adaptive control of hydraulic systems with asymptotic tracking, IEEE Trans. Autom. Sci. Eng., 14 (2017), 1524–1531. https://doi.org/10.1109/TASE.2015.2434393 doi: 10.1109/TASE.2015.2434393
![]() |
[20] |
S. B. Wang, J. Na, X. M. Ren, RISE-based adaptive asymptotic prescribed performance tracking control of nonlinear servo mechanisms, IEEE Trans. Syst. Man Cybern. Syst., 48 (2018), 2359–2370. https://doi.org/10.1109/TSMC.2017.2769683 doi: 10.1109/TSMC.2017.2769683
![]() |
[21] |
W. Bu, T. Li, J. Yang, Y. Yi, Disturbance observer-based event-triggered tracking control of networked robot manipulator, Meas. Control., 3 (2020), 1–7. https://doi.org/10.1177/0020294020911084 doi: 10.1177/0020294020911084
![]() |
[22] |
H. Rojas-Cubides, J. Cortés-Romero, J. Arcos-Legarda, Data-driven disturbance observer-based control: an active disturbance rejection approach, Control. Theory Technol., 19 (2021), 80–93. https://doi.org/10.1007/s11768-021-00039-x doi: 10.1007/s11768-021-00039-x
![]() |
[23] |
H. F. Li, Y. C. Wang, H. G. Zhang, Data-driven-based event-triggered tracking control for non-linear systems with unknown disturbance, IET Control. Theory Appl., 14 (2019), 2197–2206. https://doi.org/10.1049/iet-cta.2019.0051 doi: 10.1049/iet-cta.2019.0051
![]() |
[24] |
S. Li, H. Ren, C. Lu, Event-triggered adaptive fault-tolerant control for multi-agent systems with unknown disturbances, Discrete Contin. Dyn. A, 8 (2021): 1941–1956. https://doi.org/10.3934/dcdss.2020379 doi: 10.3934/dcdss.2020379
![]() |
[25] |
X. M. Yao, J. H. Park, L. G. Wu, L. Guo, Disturbance-observer-based composite hierarchical antidisturbance control for singular Markovian jump systems, IEEE Trans. Autom. Control, 64 (2019), 2875–2882. https://doi.org/10.1109/TAC.2018.2867607 doi: 10.1109/TAC.2018.2867607
![]() |
[26] |
X. M. Yao, L. Guo, Composite anti-disturbance control for Markovian jump nonlinear systems via disturbance observer, Automatica, 49 (2013), 2538–2545. https://doi.org/10.1016/j.automatica.2013.05.002 doi: 10.1016/j.automatica.2013.05.002
![]() |
[27] |
J. Q. Han, From PID technique to active disturbances rejection control technique, Control Eng. China, 9 (2002), 13–18. https://doi.org/10.14107/j.cnki.kzgc.2002.03.003 doi: 10.14107/j.cnki.kzgc.2002.03.003
![]() |
[28] |
X. F. Zeng, X. H. Wang, J. Zhang, G. Z. Shen, Disturbance compensated terminal sliding mode control for hypersonic vehicles, J. Beijing Univ. Aeronaut. Astronaut., 38 (2012), 1454–1458. https://doi.org/10.13700/j.bh.1001-5965.2012.11.007 doi: 10.13700/j.bh.1001-5965.2012.11.007
![]() |
[29] |
Z. J. Kang, X. Chen, Z. L. Cui, H. G. Yu, Bus voltage control method of DC distribution network based on ESO and terminal sliding mode control, Proc. CSEE, 38 (2012), 3235–3243. https://doi.org/10.13334/j.0258-8013.pcsee.171197 doi: 10.13334/j.0258-8013.pcsee.171197
![]() |
[30] |
L. Jin, S. J. Xu, Extended state observer based fault detection and recovery for flywheels, J. Beijing Univ. Aeronaut. Astronaut., 34 (2008), 1272–1275. https://doi.org/10.13700/j.bh.1001-5965.2008.11.013 doi: 10.13700/j.bh.1001-5965.2008.11.013
![]() |
[31] | C. L. Xia, J. H. Liu, W. Yu, Z. Q. Li, Variable structure control of BLDCM based on extended state observer, in IEEE International Conference Mechatronics and Automation, 2 (2005), 568–571. https://doi.org/10.1109/icma.2005.1626612 |
[32] |
H. Hang, Y. H. Li, L. M, Yang, A novel low pressure-difference fluctuation electro-hydraulic large flowrate control valve for fuel flowrate control of aeroengine afterburner system, Chin. J. Aeronaut., 11 (2021), 363–376. https://doi.org/10.1016/j.cja.2021.07.002 doi: 10.1016/j.cja.2021.07.002
![]() |
1. | Yiming Li, Zhongchao Zhang, Mingliang Bai, Guiqiu Song, Composite RISE control for vehicle-mounted servo system with unknown modeling uncertainties and unknown time-varying disturbances, 2024, 147, 00190578, 590, 10.1016/j.isatra.2024.02.017 | |
2. | Xiaohan Yang, Guozhen Cheng, Yinghao Cui, Jie Hang, ESO-based robust adaptive control for dual closed-loop fuel control system in aeroengine, 2024, 144, 09670661, 105835, 10.1016/j.conengprac.2023.105835 | |
3. | Haifang Zhong, Kailei Liu, Hongbin Qiang, Jing Yang, Shaopeng Kang, Model reference adaptive control of electro-hydraulic servo system based on RBF neural network and nonlinear disturbance observer, 2024, 0959-6518, 10.1177/09596518241277714 |
Parameter | Value | Unit | Parameter | Value | Unit |
mp | 0.76167 | kg | fv | 100 | N/(m/s) |
Ap | 2.5 × 10-4 | m2 | V10 | 1 × 10-3 | m3 |
k | 10900 | N/m | V20 | 1 × 10-3 | m3 |
βe | 2 × 108 | Pa | kt | 5.656 × 10-8 | m3/(s·V·N1/2) |
Ct | 1 × 10-13 | m3/(Pa·s) | q1 | 1 × 10-12 | m3/s |
q2 | 1 × 10-12 | m3/s | Cd | 0.7 | |
Cv | 1 | / | Wp | 5 | e-3 |
Δp | 821, 993 | / | α | 69 | ° |
K | 1000 |
Indices | Me (mm) | μe (mm) | σe (mm) |
controller I | 0.00304985 | 0.000778897 | 0.00084379 |
controller II | 0.010971 | 0.00364635 | 0.00293964 |
controller III | 0.63097 | 0.299917 | 0.197092 |
controller IV | 0.630986 | 0.299916 | 0.197092 |
Indices | Me(mm) | μe(mm) | σe(mm) |
controller I | 9.508 × 10-5 | 6.536 × 10-6 | 8.833 × 10-6 |
controller II | 9.58784 × 10-5 | 2.87371 × 10-5 | 1.02822 × 10-5 |
controller III | 7.5716 × 10-2 | 3.14012 × 10-2 | 2.00589 × 10-2 |
controller IV | 7.57345 × 10-2 | 3.13926 × 10-2 | 2.00719 × 10-2 |
Indices | Me(mm) | μe(mm) | σe(mm) |
controller I | 1.34 × 10-2 | 4.14 × 10-3 | 5.53 × 10-3 |
controller II | 4.20256 × 10-2 | 1.63032 × 10-2 | 1.30355 × 10-2 |
controller III | 1.238558 | 0.598359 | 0.391225 |
controller IV | 1.238657 | 0.598359 | 0.391224 |
Parameter | Value | Unit | Parameter | Value | Unit |
mp | 0.76167 | kg | fv | 100 | N/(m/s) |
Ap | 2.5 × 10-4 | m2 | V10 | 1 × 10-3 | m3 |
k | 10900 | N/m | V20 | 1 × 10-3 | m3 |
βe | 2 × 108 | Pa | kt | 5.656 × 10-8 | m3/(s·V·N1/2) |
Ct | 1 × 10-13 | m3/(Pa·s) | q1 | 1 × 10-12 | m3/s |
q2 | 1 × 10-12 | m3/s | Cd | 0.7 | |
Cv | 1 | / | Wp | 5 | e-3 |
Δp | 821, 993 | / | α | 69 | ° |
K | 1000 |
Indices | Me (mm) | μe (mm) | σe (mm) |
controller I | 0.00304985 | 0.000778897 | 0.00084379 |
controller II | 0.010971 | 0.00364635 | 0.00293964 |
controller III | 0.63097 | 0.299917 | 0.197092 |
controller IV | 0.630986 | 0.299916 | 0.197092 |
Indices | Me(mm) | μe(mm) | σe(mm) |
controller I | 9.508 × 10-5 | 6.536 × 10-6 | 8.833 × 10-6 |
controller II | 9.58784 × 10-5 | 2.87371 × 10-5 | 1.02822 × 10-5 |
controller III | 7.5716 × 10-2 | 3.14012 × 10-2 | 2.00589 × 10-2 |
controller IV | 7.57345 × 10-2 | 3.13926 × 10-2 | 2.00719 × 10-2 |
Indices | Me(mm) | μe(mm) | σe(mm) |
controller I | 1.34 × 10-2 | 4.14 × 10-3 | 5.53 × 10-3 |
controller II | 4.20256 × 10-2 | 1.63032 × 10-2 | 1.30355 × 10-2 |
controller III | 1.238558 | 0.598359 | 0.391225 |
controller IV | 1.238657 | 0.598359 | 0.391224 |