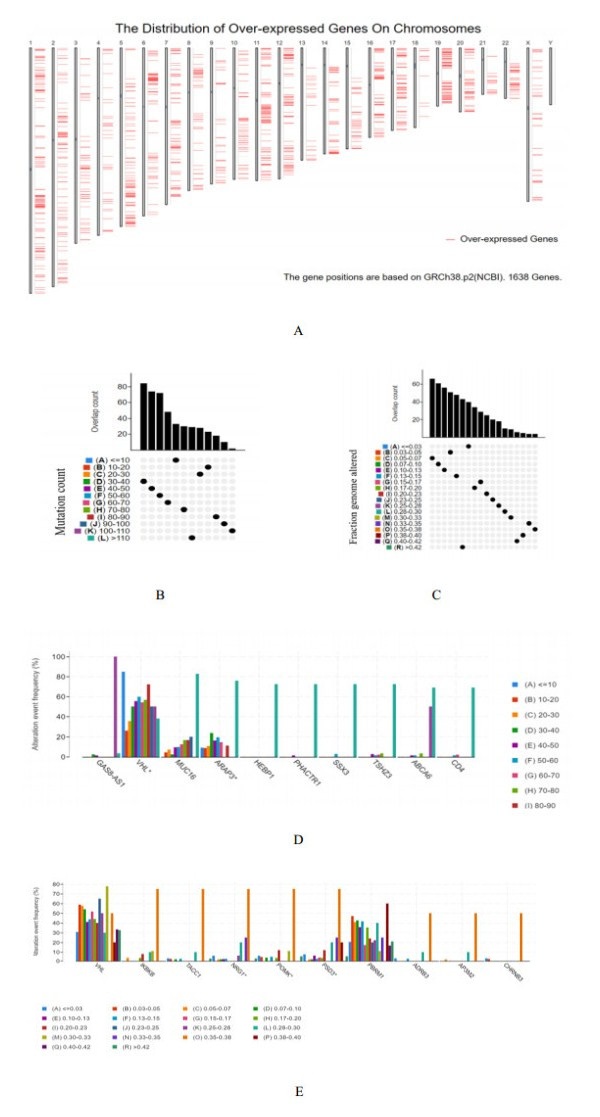
Induction motors have been widely used in industry, agriculture, transportation, national defense engineering, etc. Defects of the motors will not only cause the abnormal operation of production equipment but also cause the motor to run in a state of low energy efficiency before evolving into a fault shutdown. The former may lead to the suspension of the production process, while the latter may lead to additional energy loss. This paper studies a fuzzy rule-based expert system for this purpose and focuses on the analysis of many knowledge representation methods and reasoning techniques. The rotator fault of induction motors is analyzed and diagnosed by using this knowledge, and the diagnosis result is displayed. The simulation model can effectively simulate the broken rotator fault by changing the resistance value of the equivalent rotor winding. And the influence of the broken rotor bar fault on the motors is described, which provides a basis for the fault characteristics analysis. The simulation results show that the proposed method can realize fast fault diagnosis for rotators of induction motors.
Citation: Tangsheng Zhang, Hongying Zhi. A fuzzy set theory-based fast fault diagnosis approach for rotators of induction motors[J]. Mathematical Biosciences and Engineering, 2023, 20(5): 9268-9287. doi: 10.3934/mbe.2023406
[1] | Tongmeng Jiang, Pan Jin, Guoxiu Huang, Shi-Cheng Li . The function of guanylate binding protein 3 (GBP3) in human cancers by pan-cancer bioinformatics. Mathematical Biosciences and Engineering, 2023, 20(5): 9511-9529. doi: 10.3934/mbe.2023418 |
[2] | Prathibha Ambegoda, Hsiu-Chuan Wei, Sophia R-J Jang . The role of immune cells in resistance to oncolytic viral therapy. Mathematical Biosciences and Engineering, 2024, 21(5): 5900-5946. doi: 10.3934/mbe.2024261 |
[3] | Yujia Xi, Liying Song, Shuang Wang, Haonan Zhou, Jieying Ren, Ran Zhang, Feifan Fu, Qian Yang, Guosheng Duan, Jingqi Wang . Identification of basement membrane-related prognostic signature for predicting prognosis, immune response and potential drug prediction in papillary renal cell carcinoma. Mathematical Biosciences and Engineering, 2023, 20(6): 10694-10724. doi: 10.3934/mbe.2023474 |
[4] | Sufang Wu, Hua He, Jingjing Huang, Shiyao Jiang, Xiyun Deng, Jun Huang, Yuanbing Chen, Yiqun Jiang . FMR1 is identified as an immune-related novel prognostic biomarker for renal clear cell carcinoma: A bioinformatics analysis of TAZ/YAP. Mathematical Biosciences and Engineering, 2022, 19(9): 9295-9320. doi: 10.3934/mbe.2022432 |
[5] | Samantha Erwin, Stanca M. Ciupe . Germinal center dynamics during acute and chronic infection. Mathematical Biosciences and Engineering, 2017, 14(3): 655-671. doi: 10.3934/mbe.2017037 |
[6] | Jun Wang, Mingzhi Gong, Zhenggang Xiong, Yangyang Zhao, Deguo Xing . Immune-related prognostic genes signatures in the tumor microenvironment of sarcoma. Mathematical Biosciences and Engineering, 2021, 18(3): 2243-2257. doi: 10.3934/mbe.2021113 |
[7] | Jie Chen, Jinggui Chen, Bo Sun, Jianghong Wu, Chunyan Du . Integrative analysis of immune microenvironment-related CeRNA regulatory axis in gastric cancer. Mathematical Biosciences and Engineering, 2020, 17(4): 3953-3971. doi: 10.3934/mbe.2020219 |
[8] | Caiyun Wu, Cong Ma, Jing Yuan, Pei Zhou . Exploration of potential therapeutic and prognostic value of CXC chemokines in cervical squamous cell carcinoma and endocervical adenocarcinoma based on bioinformatics analysis. Mathematical Biosciences and Engineering, 2021, 18(6): 8201-8222. doi: 10.3934/mbe.2021407 |
[9] | Xin Lin, Xingyuan Li, Binqiang Ma, Lihua Hang . Identification of novel immunomodulators in lung squamous cell carcinoma based on transcriptomic data. Mathematical Biosciences and Engineering, 2022, 19(2): 1843-1860. doi: 10.3934/mbe.2022086 |
[10] | Yong Luo, Qingbiao Chen, Jingbo Lin . Identification and validation of a tumor mutation burden-related signature combined with immune microenvironment infiltration in adrenocortical carcinoma. Mathematical Biosciences and Engineering, 2022, 19(7): 7055-7075. doi: 10.3934/mbe.2022333 |
Induction motors have been widely used in industry, agriculture, transportation, national defense engineering, etc. Defects of the motors will not only cause the abnormal operation of production equipment but also cause the motor to run in a state of low energy efficiency before evolving into a fault shutdown. The former may lead to the suspension of the production process, while the latter may lead to additional energy loss. This paper studies a fuzzy rule-based expert system for this purpose and focuses on the analysis of many knowledge representation methods and reasoning techniques. The rotator fault of induction motors is analyzed and diagnosed by using this knowledge, and the diagnosis result is displayed. The simulation model can effectively simulate the broken rotator fault by changing the resistance value of the equivalent rotor winding. And the influence of the broken rotor bar fault on the motors is described, which provides a basis for the fault characteristics analysis. The simulation results show that the proposed method can realize fast fault diagnosis for rotators of induction motors.
Globally, renal cell carcinoma (RCC) is among the most common malignant tumors. It is the 6th and 10th most frequently new-diagnosed tumor in men and women, respectively [1,2]. According to the classification recommendations of the World Health Organization, renal cell carcinoma, with highly significant histological heterogeneities, is composed of up to 16 subgroups. Among them, clear cell renal cell carcinoma accounts for about 80% of all RCC types [3,4,5]. For patients presenting with early-stage ccRCC, as revealed by computed tomography, surgical excision is always the optimal treatment option with the maximal probability of being cured [6]. However, due to its asymptomatic features during the early stages and the lack of annual health examination, nearly 30% of these cases are diagnosed as metastatic ccRCC during the first consultation, implying that these patients have lost the chance of receiving radical resection [7,8]. In general, due to insensitivities, radiotherapy and chemotherapy are not optimal for patients with terminal ccRCC [9]. In recent years, various advances, particularly the emergence and upgrade of tyrosine kinase inhibitors together with immune checkpoint inhibitors, have improved the treatment of advanced unresectable ccRCC. These drugs have been shown to significantly prolong the overall survival (OS) or disease-free survival (DFS) outcomes in a subset of these patients [10,11,12]. However, due to intertumor and intratumor heterogeneity, some patients are not fully responsive or exhibit resistance to these regimens, resulting in dismal prognostic outcomes [13,14]. Hence, it is important to develop new remedies for treatment of patients with late ccRCC.
Cancer vaccine, which is aimed at activating patients' immunity against malignant tumor cells and improving the suppression status of the tumor immune micro-environment (TIME), has drawn plenty of attention from researchers worldwide [15,16]. Different types of vaccines, mainly comprising nucleic acids (DNA or mRNA), peptides, tumor cells, dendritic cells, among others, constitute the landscape of this promising remedy [17]. Messenger RNA (mRNA), encoding at least one tumor antigen, can be transported into the cytoplasm of APCs, translated into proteins, and be presented on the surface of these cells, thereby improving anti-tumor immunity [18]. The rationality of the mRNA cancer vaccine is malignant cells expressing tumor specific and tumor associated antigens. Both antigens can provide pertinent information on development of mRNA vaccines, which can generate targeted proteins with immunogenicity to induce immunoreactions, particularly in the local tumor microenvironment [19]. Tumor associated antigens are expressed at a low level in non-malignant cells but are predominantly over-expressed in malignant cells due to some certain somatic mutations, resulting in abundant presentations on the surface of cancer cells and recognition by T cells to initiate immune responses against cancer. Therefore, they are promising options for cancer vaccine development [20].
Due to technological advances, the mRNA vaccine has been more feasible as the lower innate immunogenicity, enhancements in stability and delivery system [21]. In the era of the COVID-19 pandemic, mRNA-based vaccines were confirmed to be safe, tolerable, and effective [22]. Compared to other vaccine types, mRNA-based vaccines have some unique advantages, including simultaneous activation of humoral and cell immunity, encoding of more epitopes to be presented by APCs and without the possibility of integration into the host cell genome [23]. Some completed clinical trials involving several malignant solid tumors, such as prostate cancer, breast cancer, and melanoma, have revealed the favorable application prospects of mRNA cancer vaccine monotherapy or in combination with other immunotherapies [24,25,26]. Rittig et al. reported that mRNA-based vaccines are feasible and beneficial for patients with metastatic RCC [27]. In addition, as with other immune-based treatments, the efficacies of mRNA vaccines are also, to an extent, dependent on TIME status, in particular, the infiltration levels and functions of CD8+ T cells [28].
In the present study, we investigated the potential of using ccRCC-related tumor antigens to develop mRNA-based vaccines. Elevated expressions of a tumor antigen that was positively associated with infiltration scores of APCs, were correlated with better survival outcomes. Additionally, patients were assigned into different groups in accordance with tumor immune subtypes, which can be used to guide the appropriate patient selection for receiving the mRNA vaccine. In brief, we elucidate on the development of mRNA vaccines and personalized medicines for ccRCC patients.
Raw sequencing data in counts form derived from ccRCC patients involved in the TCGA project along with their corresponding phenotypes and survival data were downloaded from the comprehensive Xena Platform [29] (https://xena.ucsc.edu/). In addition, open data in maf format, which is only available for partial ccRCC patients in the TCGA cohort (n = 336) and containing mutation-relevant information, were accessed at the Genomic Data Commons Data Portal (https://portal.gdc.cancer.gov/). Samples that lacked survival data or had survival time less than 30 days were excluded. Genes that were expressed in more than half of the samples were retained for subsequent analyses. The R package "maftools" was used to determine the mutated genes, mutation frequency and tumor mutation burden (TMB) among the ccRCC subjects. Additionally, the "somaticinteractions" function in this package was used to evaluate expression correlations among the mutated genes of interest.
The cBioPortal (v4.1.4, http://www.cbioportal.org/) for cancer genomics is an online tool incorporating multiple data from, but not limited to, the TCGA, Cancer Cell Line Encyclopedia (CCLE) and International Cancer Genome Consortium (ICGC) [30]. We used cBioPortal to explore and visualize the ccRCC genome alteration status based on 451 samples from TCGA for identification of potential tumor antigens.
The Gene Expression Profiling Interactive Analysis (GEPIA), as a form of online web server (http://gepia2.cancer-pku.cn/), integrates the raw sequencing data from samples in the TCGA database as well as Genotype-Tissue Expression project (GTEx) and has been widely used for gene expression and survival analyses of 33 cancer types [31]. Over-expressed genes in ccRCC tumor tissues, compared to normal kidney tissues, were obtained by ANOVA with two cutoff values (Log2 fold change > 1 together with q < 0.05) and were then mapped on chromosomes for visualization. All patients with survival information were divided into high-and low-expression groups based on median expression values of the genes. The Kaplan-Meier method in combination with log rank test were performed to determine whether there were significant differences in OS or DFS outcomes between groups.
In 2017, Li et al. developed the Tumor IMmune Estimation Resource (TIMER) for comprehensive analysis of tumor-infiltrating immune cells in various cancer types [32]. TIMER, a versatile web server (https://cistrome.shinyapps.io/timer/), was used to estimate the abundances of several immune cell types in cancer tissues and has multiple functional modules, including the gene module where users can determine if the expression levels of a given gene are correlated with the infiltration degrees of some immune cells. We used this module to assess the association between potential tumor antigens and abundances of three kinds of immune infiltrates, including B cells, macrophages and dendritic cells. After eliminating the impact of tumor purity, the partial Spearman's rho value was calculated, with p ≤ 0.05 denoting statistical significance.
The single cell RNA sequencing data of one ccRCC sample was downloaded from the gene expression omnibus (GEO), accession number GSM4630028 [33], and used to determine whether the aforementioned antigens were highly expressed in tumor cells. A panel of technical functions in the "Seurat" package were successively used for confirmation at single-cell resolution. Briefly, cells with 200–5000 genes and mitochondrial gene percentage < 10% were eligible for subsequent analyses. After the count data was normalized, highly varied genes were identified and subjected to principal-component analysis for dimensionality reduction. The appropriate number of principal components was acquired by the "JackStraw" function, and these components were further used to cluster cells into different types at a resolution of 0.6. Then, the "FindAllMarkers" function was used to search for marker genes in each cell type, while the ccRCC-specific marker genes (CA9 and NDUFA4L2) [34] were selected for annotation of cancer cells.
The immune-related gene list was acquired from the ImmPort database (https://www.immport.org/shared/genelists). After the removal of duplicated genes, a total of 1,793 genes remained. Expression levels of immune relevant genes in TCGA ccRCC samples were extracted to constitute a new matrix, after which the "ConsensusClusterPlus" R package [35] was used to identify the underlying immune subtypes among these patients. The partition around medoids cluster algorithm accompanied with the "1-Pearson correlation" distance was used for clustering. The total number of subsampling was set as 500, and four in five samples were simultaneously selected for each resampling. The upper limit of the resulting clusters was set as 6, and the optimal partition was visually evaluated by virtue of the consensus matrix combined with the consensus cumulative distribution function. Then, principal component analysis was performed to assess if samples from different subtypes could be well distinguished. Inter-group differences in overall survival time of patients were determined using the "survival" R package. Correlations between immune subtypes and clinical features, molecular subtypes, tumor mutation burden were explored to describe these intrinsic features among the defined immune subtypes.
Stromal and immune scores for each sample were calculated using the "Estimate" algorithm [36]. Then, on the basis of a gene list containing twenty-eight immune cell types, the single sample gene set enrichment analysis (ssGSEA) method in the "GSVA" package was used to determine the abundance of these cell types [37]. Lastly, based on gene expression characteristics of twenty-two kinds of immune cells, the "CIBERSORT" algorithm was used to calculate the proportion of these cells in samples [38].
Two individual gene sets, encompassing immunogenic cell death (ICD) modulators and immune checkpoints (ICP), were retrieved from previously published articles [39,40]. Then, expressions of these genes in diverse immune subgroups were extracted and compared by performing the Wilcoxon rank sum test.
The co-expression modules were identified and correlated with the immune subtypes using the WGCNA package in R software [41]. Non- or low-varying genes, evaluated using the median absolute deviation (MAD), were filtered out. Thereafter, a sample tree was plotted to detect whether the samples had outliers. Then, the optimal soft threshold was calculated per the inputted gene expression profile and was served as an actual argument of the "blockwiseModules" function which was a one-step method to construct the co-expression matrix. Subsequently, the genes were clustered into different color modules based on the topological overlap matrix (TOM). After that, the correlations between modules and the immune subtypes were explored. Lastly, gene ontology (GO) analysis was carried out using the "clusterProfiler" package [42] to annotate the cellular location and biological functions of the module genes that were strongly correlated with the immune subtypes.
Genomic alterations are known to influence one's response to anticancer drugs. In this study, the Genomics of Drug Sensitivity in Cancer (GDSC) database was used to provide the gene expression profile of numerous cancer cell lines and half maximal inhibitory concentration (IC50) of hundreds of common compounds against these cell strains [43]. Using datasets from GDSC as a training set, the R package oncoPredict [44] can predict sensitivity to anticancer agents via constructing a ridge regression model based on the gene expression matrix obtained from clinical samples of tumor tissues. Drug-sensitivity analysis was investigated in patients with different immune subtypes.
To identify potential tumor antigens for ccRCC, 1,638 over-expressed genes with the potential to encode tumor associated antigens (TAA) were determined via differential gene-expression profiling between normal and malignant renal parenchyma tissues (Figure 1A). Variations in tumor mutation counts and altered genome fractions in ccRCC cases were analyzed to screen the TAA-encoding mutated genes, resulting in 11,686 mutation genes (Figure 1B, C). Mutational analysis revealed that GAS8 antisense RNA 1 (GAS8-AS1), von Hippel-Lindau tumor suppressor (VHL), mucin 16 (MUC16), ankyrin repeat, and PH domain 3 (ARAP3), heme binding protein 1 (HEBP1), phosphatase and actin regulator 1 (PHACTR1), SSX family member 3 (SSX3), teashirt zinc finger homeobox 3 (TSHZ3), ATP binding cassette subfamily A member 6 (ABCA6), as well as CD4 molecule (CD4), were the top 10 highly mutated genes in the mutation count group (Figure 1D). The top 10 highly altered genes in the fraction genome altered group included von Hippel-Lindau tumor suppressor (VHL), inhibitor of nuclear factor kappa B kinase subunit beta (IKBKB), transforming acidic coiled-coil containing protein 1 (TACC1), neuregulin 1 (NRG1), protein O-mannose kinase (POMK), pleckstrin and Sec7 domain containing 3 (PSD3), polybromo 1 (PBRM1), adrenoceptor beta 3 (ADRB3), adaptor related protein complex 3 subunit mu 2 (AP3M2) and cholinergic receptor nicotinic beta 3 subunit (CHRNB3) (Figure 1E). Taken together, 629 overexpressed and mutated genes were identified for further analysis.
Based on the afore-mentioned intersecting result, genes with prognostic values were screened for development of an mRNA vaccine. Six and seven genes were found to be respectively correlated with OS and DFS of ccRCC patients, and finally, two common genes, LDL receptor related protein 2 (LRP2) and dedicator of cytokinesis 8 (DOCK8) were identified (Figure 2A). Compared to the low expression group, patients in the high LRP2 expression group exhibited a superior survival time (Figure 2B, C), while patients with high expressions of DOCK8 were also associated with better survival outcomes (Figure 2D, E). These findings suggest that the two genes may have immune-stimulatory functions. Expressions of LRP2 and DOCK8 were positively correlated with the abundance of macrophages, dendritic cells and B cells (Figure 3A, B). Then, expressions of LRP2 and DOCK8 in different stages were determined (Figure 3C, D) using the UALCAN database [45]. It was established that with progressing stages, expressions of the two genes gradually decreased, implying that mRNA vaccines, based on the two potential tumor antigens, may yield better immunogenicity in early-stage ccRCC patients. The two genes (LRP2 and DOCK8) with potential immune-stimulating effects are potential candidates for ccRCC-mRNA vaccine development.
After quality control, 21,549 genes and 5666 cells remained, and 20 principal components were used to cluster these cells, leading to various cell types (Figure 4A). Cell clusters (0, 1, 12) with simultaneously higher expressions of CA9 and NDUFA4L2 were annotated as ccRCC cells (Figure 4B). In Figure 4C, D, LRP2 was mainly expressed in tumor cells, but DOCK8 was not, hence only LRP2 could be regarded as an authentic tumor antigen for development of an mRNA vaccine.
Immune-typing can be used to reflect, to a great extent, the immune status in heterogeneous tumor micro-environments, which informs the selection of patients to be administered with the mRNA vaccine. Therefore, consensus clustering, according to expression levels of immune-related genes, was performed in TCGA ccRCC samples. Considering the results from delta area, consensus CDF and consensus matrix, the optimal cluster number (n = 2) was determined, and accordingly, two robust immune subtypes (IS1 and IS2) were identified (Figure 5A–C). Survival analysis revealed significant differences between the two groups, with patients in the IS2 subtype having better OS outcomes than those in the IS1 subtype (Figure 5D). In addition, principal components analysis (PCA) was performed to assess subtype assignments, which revealed that most of the samples in the IS1 group were distinct from samples in the IS2 group (Figure 5E). The distribution ratio of immune subtypes identified herein in six well-known pan-cancer immune subtypes [46], encompassing C1 (Would Healing), C2 (IFN-γ Dominant), C3 (Inflammatory), C4 (Lymphocyte Depleted), C5 (Immunologically Quiet) as well as C6 (TGF-β), were explored, showing a high abundance of IS2 in C4 and C5 subtypes (Figure 5F). The distribution ratios of IS1 and IS2 in different grades and stages of ccRCC patients were investigated (Figure 5G, H). Lower grades and stages were associated with IS2 while higher grades and stages were associated with IS1. Expressions of LRP2 were significantly high in the IS2 group (Figure 5I). Taken together, immunophenotyping is a potential robust indicator for assessment of the clinical outcomes of ccRCC patients.
Tumor mutation burden and somatic mutation rates play a vital role in anti-cancer immunity, and generally, higher TMB levels are associated with stronger immunogenicity, leading to increased immunotherapeutic efficacies [47]. Thus, TMB and mutations of the two subtypes were determined and compared. The IS1 exhibited higher TMB and number of mutated genes but without statistical significance (Figure 6A, B). Further, highly mutated genes, such as VHL (47%) and PBRM1 (40%), across the two immune subtypes in ccRCC patients were revealed by somatic mutation analysis (Figure 6C). Then, expression correlations of the frequently mutated genes were explored (Figure 6D). There were no marked differences in immune subtypes with regard to the magnitude of mutation burden.
During ccRCC progression, a dysregulated TIME plays a pivotal role and largely influences the outcomes of mRNA vaccine therapy. First, based on the ESTIMATE method, immune and stromal scores were directly obtained from each included sample, and tumor purity was calculated as: Tumor purity = cos (0.6049872018 + 0.0001467884 × ESTIMATE score) [36]. In Figure 7A–C, compared with the IS2 group, the IS1 group had significantly high immune and stromal scores, which implied a lower tumor purity. Then, ssGSEA was used to assess the abundance of 28 immune cell types in the two immune groups. Differences in abundance of immune cells between the two groups were significant (Figure 7D). Compared to IS2, IS1 exhibited a significantly high abundance of activated B cells, type 1 T helper cells, myeloid-derived suppressor cells (MDSC), activated CD4 T cells, macrophages, activated CD8 T cells and regulatory T cells among others. CIBERSORT analysis (Figure 7E) revealed that compared with IS2, IS1 samples had significant high proportions of CD8 T cells, regulatory T cells, plasma cells and M0 macrophages with low proportions of M2 macrophages, CD4 memory resting T cells, resting mast cells and monocytes.
Both ICP and ICD modulators have important effects on modulation of host anti-tumor immunity, thereby affecting the immunotherapeutic efficacy, including that of the mRNA vaccine. Therefore, differential expressions of the above immune-related molecules were determined in these immune subtypes. A total of 47 ICP were detected in the TCGA ccRCC cohort and a large proportion of these genes (more than 30), including PDCD1, CD274 and CTLA4, were significantly highly expressed in the IS1 subtype with only two genes (TNFRSF14, TNFRSF4) being over-expressed in the IS2 subtype (Figure 8A). Moreover, 13 ICD modulator-related genes, including CXCL10, FRP1, IFNAR2, IFNE, CALR, LRP1, PANX1, P2RY2, ANXA1, P2RX7, HGF, MET and TLR4, were significantly elevated in IS1 tumors, while EIF2A, IFNAR1 and HMGB1 were up-regulated in IS2 tumors (Figure 8B). Therefore, immunotyping is a potential indicator of the efficacy the mRNA vaccine as the subtypes enable comprehensive assessment of the expressions of ICP and ICD modulators. Moreover, effectiveness of the mRNA vaccine can be damaged in the context of over-expressed ICP and under-expressed ICD modulators.
The classification of high-varying genes, using gene co-expression modules, was achieved and correlated with different immune subtypes. Based on the results of mean connectivity coupled with scale-free fit index, five was set as the soft-thresholding power for subsequent analyses (Figure 9A). Then, 10 modules (distinguished by distinct colors) were identified via average linkage hierarchical clustering (Figure 9B) and the number of genes in each module established (Figure 9C). Next, correlation analysis of module to trait (that is, in this situation, immune subtypes) was performed. According to the correlation coefficient and p-value (Figure 9D), the three mostly positively correlated modules for IS1 were the black (MEblack: rho: 0.74, p < 0.01), yellow (MEyellow: rho: 0.53, p < 0.01) and grey (MEgrey: rho: 0.39, p < 0.01) modules, while the blue (MEblue: rho: -0.18, p < 0.01) and green (MEgreen: rho: -0.28, p < 0.01) modules were negatively correlated with IS1. Module membership vs. gene significance scores of genes in the MEblack were visualized in the scatter plot (Figure 9E), which confirmed the highly positive relationship between the black module and IS1 (r = 0.89, p < 0.01). GO enrichment analysis of genes extracted from the black module suggested that these genes were mainly involved in the B cell receptor signaling pathway, phagocytosis and complement activation, among others (Figure 9F).
To determine whether patients with the immune subtype that was less suitable for mRNA vaccination could be more sensitive to other clinically available drugs, anti-cancer drug sensitivity for each sample in the TCGA-ccRCC cohort was predicted with the magnitude of the IC50 value (Figure 10A–F). Surprisingly, most of the drugs, including sunitinib, cabozantinib, temsirolimus, and tivozanib, were fitter for patients in the IS1 cluster, where they exhibited a relatively low IC50. There was a slight diversity with regard to the sensitivity of sorafenib and pazopanib between the two subtypes, with IS2 being more sensitive to sorafenib than IS1. Taken together, although IS1 patients may be less suitable for receiving the mRNA vaccine, they are more suitable for targeted therapy involving drugs that target the vascular endothelial growth factor receptor (VEGFR) and mammalian target of rapamycin (mTOR). The diversity in treatment sensitivity between the two subtypes also confirmed the heterogeneity among patients, which reflects the urgency of individualized treatments.
Among the different renal cancer types, ccRCC is the most common and is highly aggressive. For patients with early stage diagnosis, the 5-year survival rate after reasonable therapies is more than 90% and may reduce to approximately 12% due to concomitant metastasis [48]. Elucidation of the genesis and progression of ccRCC have enabled the development of molecularly targeted agents for applications in advanced patients. For instance, sunitinib, everolimus and nivolumab have been proven to improve the survival rates in partial patients [49]. However, the efficacy of these drugs is always limited or temporary and many patients ultimately die of metastases. Therefore, there is a need to develop new alternative or combinational therapeutics.
Tumor antigen supplemented with or without an adjuvant is the core of a representative cancer vaccine, being responsible for supporting immune cells to recognize and eliminate cancer cells [50]. Traditional chemotherapy, molecular targeted therapy and immunotherapy are associated with more development time and cost, recurrent drug resistance or side-effects and limited therapeutic potency; in contrast, the cancer vaccine is more promising for its' maximal specific effects, lower toxicity and long-lasting immunological memory [51]. Given these merits, three types of cancer vaccines against ccRCC embodying autologous tumor cells, dendritic cells and peptides have been developed and evaluated in clinical trials. Jocham et al. reported that patients in the autologous renal tumor cell vaccine group (n = 177) had a significantly high 5-year progression-free survival rate than the control group (no vaccine, n = 202), and only 12 treatment-associated adverse events were observed [52]. IMA901, which contains multiple tumor-related peptides, is a therapeutic vaccine that can induce T cell responses in patients with metastatic RCC, resulting in better disease control and longer overall survival outcomes [53]. AGS-003 is prepared using tumor RNA and dendritic cells derived from autologous monocytes of metastatic ccRCC patients. Compared with sunitinib monotherapy, the combination of AGS-003 and sunitinib was associated with prolonged survival outcomes [54]. Despite these results, none of them are currently available for routine use in clinics, suggesting the need for development of other kinds of vaccines. The mRNA-based vaccine platform has more novelty with the potential to be a major breakthrough for several reasons. First, it can be designed to synchronously deliver multiple tumor-associated antigens and induce antibody-mediated humoral as well as cell-mediated immune responses, decreasing the possibility of vaccine resistance [55]. Second, being different from the peptide vaccine, it can encode whole-length tumor antigens, which enables APCs to simultaneously present more epitopes, increasing the likelihood of a wide T cell response [56]. Last, it is non-infectious and free of proteins or contaminations in the production process, implying better tolerability and safety [57]. Generally, the open reading frame encoding vaccine antigens and structural components, including the 5'-cap, 3'-tail and two untranslated regions form the core of a typical mRNA vaccine. By lowering the immunogenicity and enhancing the stability as well as delivery efficiency of mRNA vaccines, their feasibility can be further increased. For instance, the immunogenicity of mRNA can be reduced by chemical modifications of nucleotides, while its stability can be improved via the addition of AU- or GC-rich sequences [58,59]. Studies have reported tumor regression after vaccination alone or in combination with other therapies, such as immune checkpoint blockers or chemotherapeutics [60]. However, regarding ccRCC, mRNA vaccination is still in its infancy and further investigations should aim at mining tumor antigens with superior immunogenicity.
In this study, various preliminary targeting antigens were revealed by analyses of somatic mutations and over-accumulated genes of ccRCC. Given that these antigens were predicted by altered gene profiles, and may not have significant functions in ccRCC, their prognostic value and correlations with APCs infiltrations were further investigated. Then, expressions of these potential antigens were further investigated at the single-cell level. Finally, one tumor antigen (LRP2), which was positively correlated with both survival time and richness of APCs, was identified to be a promising candidate for vaccine development. Based on the above analyses, the mRNA sequence of LRP2 can be chemically modified and synthesized using in vitro transcription (IVT) systems. After encapsulation with lipid nanoparticles, the optimized LRP2-encoding mRNA vaccine can be injected into ccRCC patients to induce immune responses against cancer cells. The potentiality of this tumor antigen for development of an anti-ccRCC mRNA vaccine has been reported. For example, LRP2 was highly expressed in malignant melanoma tissues, compared to benign nevi, and siRNA-mediated suppression of LRP2 expression significantly inhibited melanoma cell proliferation and survival [61]. Metformin decreases the proliferative abilities of human papillary thyroid cancer cells by inducing the apoptosis of TPC-1 cells via the downregulation of LRP2 [62]. In addition, LRP2 expressions gradually increased from premalignant oral leukoplakia to oral squamous cell carcinoma and were associated with metallothioneins, indicating the onco-driving potential of this interaction [63].
Only a small number of patients that have adopted cancer vaccine-based regimens have exhibited increased objective response rates and prolonged survival time; therefore, ccRCC patients were stratified according to the expression status of immune related genes to achieve appropriate uses of cancer vaccine therapy. Two immune subtypes were finally identified, with distinct clinical and molecular characteristics. Compared to patients in the IS2 cluster, patients within IS1 exhibited significantly low overall survival outcomes, indicating that immunotype is a robust prognostic indicator for ccRCC. Apart from prognosis, the abundance of the two immune subtypes was compared by grade and stage, revealing that patients with advanced ccRCC accounted for a greater percentage of IS1. A higher IS2 proportion was found in the immunological "cold" subtypes (C4 and C5), and administration of an mRNA vaccine in IS2 patients may induce host immune responses towards a "hot" state. Furthermore, compared with IS1, IS2 exhibited higher expressions of LRP2, suggesting that mRNA vaccines may confer more benefits to patients with IS2. Intriguingly, the two subtypes manifested significantly different sensitivities to several drugs that were routinely used in clinical settings, providing a reference for patients who were not suitable for vaccination and emphasizing the necessity of personalized treatment.
Various immune cells and multiple immune-associated molecules, such as ICP and ICD modulators, are vital components of the TIME that can greatly influence the outcomes of the mRNA vaccine. IS1 had higher immune scores than IS2, indicating the immune "hot" status of IS1, and was further validated by widely elevated infiltrations of different immune cells. Notably, expressions of cells with immune-suppressive abilities, especially regulatory T cells and myeloid-derived suppressor cells, were enhanced in IS1, thereby inhibiting the functions of immune effector cells by various mechanisms [64]. The majority of ICP, including PD-1, PD-L1 and CTLA4, were highly expressed in IS1, suggesting that IS1 was dominated by an immune-suppressive microenvironment, which can inhibit the performance of ICD modulators [65]. Taken together, IS1 was characterized by elevated expressions of multiple immune-suppressive cells and molecules that can compromise the effectiveness of mRNA vaccines, therefore, combination or sequential therapies with immune checkpoint blockers should be considered.
For cancer, the magnitude of the immunogenicity (in other words, the capacity for eliciting adaptive immune responses) is dependent on tumor antigens, infiltration degree of immune cells and the presence of immunomodulatory molecules in the tumor microenvironment [66]. Although it has a relatively lower tumor mutational burden, ccRCC has been described as an immunogenic cancer since survival benefits were observed, more than two decades ago, in patients treated with interleukin-2 and interferon-α. The subsequent success of immune checkpoint inhibitors further consolidated this concept [67]. Hence, mRNA-type cancer vaccine, as an alternative or assistant immunotherapy, may have broad application prospects in ccRCC.
In conclusion, LRP2 is a potential target for the development of a ccRCC mRNA vaccine, and patients in the IS2 cluster may highly benefit from this therapy. Additionally, this study provides some insights for the development of mRNA vaccines against ccRCC and the selection of suitable vaccine-receiving patients.
This study was supported by the Key Research and Development Program of Guangxi (2021AB13014), Major Project of Guangxi Innovation Driven (AA18118016), and Guangxi Key Laboratory for Genomic and Personalized Medicine [grant number 16-380-54, 17-259-45, 19-050-22, 19-185-33, 20-065-33].
The authors declare that they have no conflicts of interest.
[1] |
Z. Guo, K. Yu, N. Kumar, W. Wei, S. Mumtaz, M. Guizani, Deep distributed learning-based POI recommendation under mobile edge networks, IEEE Internet Things J., 10 (2022), 303–317. https://doi.org/10.1109/JIOT.2022.3202628 doi: 10.1109/JIOT.2022.3202628
![]() |
[2] |
Y. Li, H. Ma, L. Wang, S. Mao, G. Wang, Optimized content caching and user association for edge computing in densely deployed heterogeneous networks, IEEE Trans. Mob. Comput., 21 (2020), 2130–2142. https://doi.org/10.1109/TMC.2020.3033563 doi: 10.1109/TMC.2020.3033563
![]() |
[3] |
Z. Guo, K. Yu, A. K. Bashir, D. Zhang, Y. D. Al-Otaibi, M. Guizani, Deep information fusion-driven POI scheduling for mobile social networks, IEEE Network, 36 (2022), 210–216. https://doi.org/10.1109/MNET.102.2100394 doi: 10.1109/MNET.102.2100394
![]() |
[4] |
L. Yang, Y. Li, S. X. Yang, Y. Lu, T. Guo, K. Yu, Generative adversarial learning for intelligent trust management in 6G wireless networks, IEEE Network, 36 (2022), 134–140. https://doi.org/10.1109/MNET.003.2100672 doi: 10.1109/MNET.003.2100672
![]() |
[5] |
Q. Zhang, K. Yu, Z. Guo, S. Garg, J. J. P. C. Rodrigues, M. M. Hassan, et al., Graph neural networks-driven traffic forecasting for connected internet of vehicles, IEEE Trans. Network Sci. Eng., 9 (2022), 3015–3027. https://doi.org/10.1109/TNSE.2021.3126830 doi: 10.1109/TNSE.2021.3126830
![]() |
[6] |
L. Zhao, Z. Bi, A. Hawbani, K. Yu, Y. Zhang, M. Guizani, ELITE: An intelligent digital twin-based hierarchical routing scheme for softwarized vehicular networks, IEEE Trans. Mob. Comput., 2022 (2022). https://doi.org/10.1109/TMC.2022.3179254 doi: 10.1109/TMC.2022.3179254
![]() |
[7] |
Y. Zhu, W. Zheng, Observer-based control for cyber-physical systems with DoS attacks via a cyclic switching strategy, IEEE Trans. Autom. Control, 65 (2019), 3714–3721. https://doi.org/10.1109/TAC.2019.2953210 doi: 10.1109/TAC.2019.2953210
![]() |
[8] |
L. Zhao, Z. Yin, K. Yu, X. Tang, L. Xu, Z. Guo, et al., A fuzzy logic based intelligent multi-attribute routing scheme for two-layered SDVNs, IEEE Trans. Netw. Serv. Manage., 2022 (2022). https://doi.org/10.1109/TNSM.2022.3202741 doi: 10.1109/TNSM.2022.3202741
![]() |
[9] |
L. Chen, Y. Zhu, C. K. Ahn, Adaptive neural network-based observer design for switched systems with quantized measurements, IEEE Trans. Neural Networks Learn. Syst., 2021 (2021), 1–14. https://doi.org/10.1109/TNNLS.2021.3131412 doi: 10.1109/TNNLS.2021.3131412
![]() |
[10] |
J. Zhang, Q. Yan, X. Zhu, K. Yu, Smart industrial IoT empowered crowd sensing for safety monitoring in coal mine, Digital Commun. Networks, 2022 (2022). https://doi.org/10.1016/j.dcan.2022.08.002 doi: 10.1016/j.dcan.2022.08.002
![]() |
[11] |
Z. Cai, X. Zheng, A private and efficient mechanism for data uploading in smart cyber-physical systems, IEEE Trans. Network Sci. Eng., 7 (2020), 766–775. https://doi.org/10.1109/TNSE.2018.2830307 doi: 10.1109/TNSE.2018.2830307
![]() |
[12] |
Z. Guo, K. Yu, A. Jolfaei, F. Ding, N. Zhang, Fuz-Spam: Label smoothing-based fuzzy detection of spammers in internet of things, IEEE Trans. Fuzzy Syst., 30 (2022), 4543–4554. https://doi.org/10.1109/TFUZZ.2021.3130311 doi: 10.1109/TFUZZ.2021.3130311
![]() |
[13] |
Z. Cai, X. Zheng, J. Yu, A differential-private framework for urban traffic flows estimation via taxi companies, IEEE Trans. Ind. Inf., 15 (2019), 6492–6499. https://doi.org/10.1109/TⅡ.2019.2911697 doi: 10.1109/TⅡ.2019.2911697
![]() |
[14] |
Z. Zhou, Y. Li, J. Li, K. Yu, G. Kou, M. Wang, et al., Gan-siamese network for cross-domain vehicle re-identification in intelligent transport systems, IEEE Trans. Network Sci. Eng., 2022 (2022), 1–12. https://doi.org/10.1109/TNSE.2022.3199919 doi: 10.1109/TNSE.2022.3199919
![]() |
[15] |
Z. Zhou, Y. Su, J. Li, K. Yu, Q. J. Wu, Z. Fu, et al., Secret-to-image reversible transformation for generative steganography, IEEE Trans. Dependable Secure Comput., 2022 (2022), 1–17. https://doi.org/10.1109/TDSC.2022.3217661 doi: 10.1109/TDSC.2022.3217661
![]() |
[16] |
S. Xia, Z. Yao, Y. Li, S. Mao, Online distributed offloading and computing resource management with energy harvesting for heterogeneous MEC-enabled IoT, IEEE Trans. Wireless Commun., 20 (2021), 6743–6757. https://doi.org/10.1109/TWC.2021.3076201 doi: 10.1109/TWC.2021.3076201
![]() |
[17] |
Z. Guo, Y. Shen, S. Wan, W. Shang, K. Yu, Hybrid intelligence-driven medical image recognition for remote patient diagnosis in internet of medical things, IEEE J. Biomed. Health Inf., 26 (2022), 5817–5828. https://doi.org/10.1109/JBHI.2021.3139541 doi: 10.1109/JBHI.2021.3139541
![]() |
[18] |
Z. Cai, Q. Chen, Latency-and-coverage aware data aggregation scheduling for multihop battery-free wireless networks, IEEE Trans. Wireless Commun., 20 (2021), 1770–1784. https://doi.org/10.1109/TWC.2020.3036408 doi: 10.1109/TWC.2020.3036408
![]() |
[19] |
C. Chen, Z. Liao, Y. Ju, C. He, K. Yu, S. Wan, Hierarchical domain-based multi-controller deployment strategy in SDN-enabled space-air-ground integrated network, IEEE Trans. Aerosp. Electron. Syst., 58 (2022), 4864–4879. https://doi.org/10.1109/TAES.2022.3199191 doi: 10.1109/TAES.2022.3199191
![]() |
[20] |
H. Jafari, J. Poshtan, Fault detection and isolation based on fuzzy‐integral fusion approach, IET Sci. Meas. Technol., 13 (2019), 296–302. https://doi.org/10.1049/iet-smt.2018.5005 doi: 10.1049/iet-smt.2018.5005
![]() |
[21] |
Y. Lu, L. Yang, S. X. Yang, Q. Hua, A. K. Sangaiah, T. Guo, et al., An intelligent deterministic scheduling method for ultralow latency communication in edge enabled industrial internet of things, IEEE Trans. Ind. Inf., 19 (2022), 1756–1767. https://doi.org/10.1109/TⅡ.2022.3186891 doi: 10.1109/TⅡ.2022.3186891
![]() |
[22] |
S. K. Gundewar, P. V. Kane, Condition monitoring and fault diagnosis of induction motor, J. Vib. Eng. Technol., 9 (2021), 643–674. https://doi.org/10.1007/s42417-020-00253-y doi: 10.1007/s42417-020-00253-y
![]() |
[23] |
A. Choudhary, D. Goyal, S. L. Shimi, A. Akula, Condition monitoring and fault diagnosis of induction motors: A review, Arch. Comput. Methods Eng., 26 (2019), 1221–1238. https://doi.org/10.1007/s11831-018-9286-z doi: 10.1007/s11831-018-9286-z
![]() |
[24] |
D. Fan, G. P. Jiang, Y. R. Song, Y. W. Li, G. Chen, Novel epidemic models on PSO-based networks, J. Theor. Biol., 477 (2019), 36–43. https://doi.org/10.1016/j.jtbi.2019.06.006 doi: 10.1016/j.jtbi.2019.06.006
![]() |
[25] |
I. Dilmi, A. Bouguerra, A. Djrioui, L. Chrifi-Alaoui, Interval type-2 fuzzy logic-second order sliding mode based fault detection and active fault-tolerant control of brushless DC motor, J. Eur. Syst. Automatisés, 54 (2021), 475–485. https://doi.org/10.18280/jesa.540311 doi: 10.18280/jesa.540311
![]() |
[26] |
O. E. Hassan, M. Amer, A. K. Abdelsalam, B. W. Williams, Induction motor broken rotor bar fault detection techniques based on fault signature analysis–a review, IET Electr. Power Appl., 12 (2018), 895–907. https://doi.org/10.1049/iet-epa.2018.0054 doi: 10.1049/iet-epa.2018.0054
![]() |
[27] | H. MERABET, T. Bahi, K. BEDOUD, D. DRICI, A fuzzy logic based approach for the monitoringof open switch fault in a SVM voltage sourceinverter fed induction motor drive, J. Autom. Syst. Eng., 12 (2018), 48–66. |
[28] |
P. Kumar, A. S. Hati, Deep convolutional neural network based on adaptive gradient optimizer for fault detection in SCIM, ISA Trans., 111 (2021), 350–359. https://doi.org/10.1016/j.isatra.2020.10.052 doi: 10.1016/j.isatra.2020.10.052
![]() |
[29] |
D. K. Soother, J. Daudpoto, A brief review of condition monitoring techniques for the induction motor, Trans. Can. Soc. Mech. Eng., 43 (2019), 499–508. https://doi.org/10.1139/tcsme-2018-0234 doi: 10.1139/tcsme-2018-0234
![]() |
[30] |
A. Mehta, A. Choudhary, D. Goyal, B. S. Pabla, Infrared thermography based fault diagnosis and prognosis for rotating machines, J. Univ. Shanghai Sci. Technol., 23 (2021), 22–29. https://doi.org/10.1155/2021/9947300 doi: 10.1155/2021/9947300
![]() |
[31] |
S. Kavitha, N. S. Bhuvaneswari, R. Senthilkumar, N. R. Shanker, Magnetoresistance sensor-based rotor fault detection in induction motor using non-decimated wavelet and streaming data, Automatika, 63 (2022), 525–541. https://doi.org/10.1080/00051144.2022.2052533 doi: 10.1080/00051144.2022.2052533
![]() |
[32] | A. Ebrahimi, H. Ahmad, R. Roshanfekr, Stator winding short circuit fault detection in three-phase Induction Motors using combination type-2 Fuzzy logic and Support Vector Machine classifier optimized by Fractional-order Chaotic Particle Swarm optimization algorithm, Comput. Intell. Electr. Eng., 12 (2021), 37–48. |
[33] |
A. Chouhan, P. Gangsar, R. Porwal, C. K. Mechefske, Artificial neural network–based fault diagnosis for induction motors under similar, interpolated and extrapolated operating conditions, Noise Vibr. Worldwide, 52 (2021), 323–333. https://doi.org/10.1177/09574565211030709 doi: 10.1177/09574565211030709
![]() |
[34] |
C. G. Dias, C. M. de Sousa, A neuro-fuzzy approach for locating broken rotor bars in induction motors at very low slip, J. Control Autom. Electr. Syst., 29 (2018), 489–499. https://doi.org/10.1007/s40313-018-0388-5 doi: 10.1007/s40313-018-0388-5
![]() |
[35] | D. Bouneb, T. Bahi, H. MERABET, Vibration for detection and diagnosis bearing faults using adaptive neurofuzzy inference system, J. Electr. Syst., 14 (2018), 95–104. |
[36] |
Z. Zhu, Y. Lei, G. Qi, Y. Chai, N. Mazur, Y. An, et al., A review of the application of deep learning in intelligent fault diagnosis of rotating machinery, Measurement, 206 (2022), 112346. https://doi.org/10.1016/j.measurement.2022.112346 doi: 10.1016/j.measurement.2022.112346
![]() |
[37] |
X. Huang, G. Qi, N. Mazur, Y. Chai, Deep residual networks-based intelligent fault diagnosis method of planetary gearboxes in cloud environments, Simul. Modell. Pract. Theory, 116 (2022), 102469. https://doi.org/10.1016/j.simpat.2021.102469 doi: 10.1016/j.simpat.2021.102469
![]() |
[38] |
G. Qi, Z. Zhu, K. Erqinhu, Y. Chen, Y. Chai, J. Sun, Fault-diagnosis for reciprocating compressors using big data and machine learning, Simul. Modell. Pract. Theory, 80 (2018), 104–127. https://doi.org/10.1016/j.simpat.2017.10.005 doi: 10.1016/j.simpat.2017.10.005
![]() |
[39] |
X. Shen, G. Shi, H. Ren, W. Zhang, Biomimetic vision for zoom object detection based on improved vertical grid number YOLO algorithm, Front. Bioeng. Biotechnol., 10 (2022), 905583. https://doi.org/10.3389/fbioe.2022.905583 doi: 10.3389/fbioe.2022.905583
![]() |
[40] |
X. Zhang, T. Feng, Q. Niu, X. Deng, A novel swarm optimization algorithm based on a mixed-distribution model, Appl. Sci., 8 (2018), 632. https://doi.org/10.3390/app8040632 doi: 10.3390/app8040632
![]() |
[41] |
A. Glowacz, Thermographic fault diagnosis of shaft of BLDC motor, Sensors, 22 (2022), 8537. https://doi.org/10.3390/s22218537 doi: 10.3390/s22218537
![]() |
[42] |
A. Głowacz, W. Głowacz, Z. Głowacz, Recognition of armature current of DC generator depending on rotor speed using FFT, MSAF-1 and LDA, Ekspl. Niezawodność, 17 (2015), 64–69. https://doi.org/10.17531/ein.2015.1.9 doi: 10.17531/ein.2015.1.9
![]() |
[43] |
A. Glowacz, Fault diagnostics of acoustic signals of loaded synchronous motor using SMOFS-25-EXPANDED and selected classifiers, Tehnički vjesnik, 23 (2016), 1365–1372. https://doi.org/10.17559/TV-20150328135652 doi: 10.17559/TV-20150328135652
![]() |
[44] |
O. AlShorman, F. Alkahatni, M. Masadeh, M. Irfan, A. Glowacz, F. Althobiani, et al., Sounds and acoustic emission-based early fault diagnosis of induction motor: A review study, Adv. Mech. Eng., 13 (2021). https://doi.org/10.1177/1687814021996915 doi: 10.1177/1687814021996915
![]() |
[45] |
A. Glowacz, R. Tadeusiewicz, S. Legutko, W. Caesarendra, M. Irfan, H. Liu, et al., Fault diagnosis of angle grinders and electric impact drills using acoustic signals, Appl. Acoust., 179 (2021), 108070. https://doi.org/10.1016/j.apacoust.2021.108070 doi: 10.1016/j.apacoust.2021.108070
![]() |