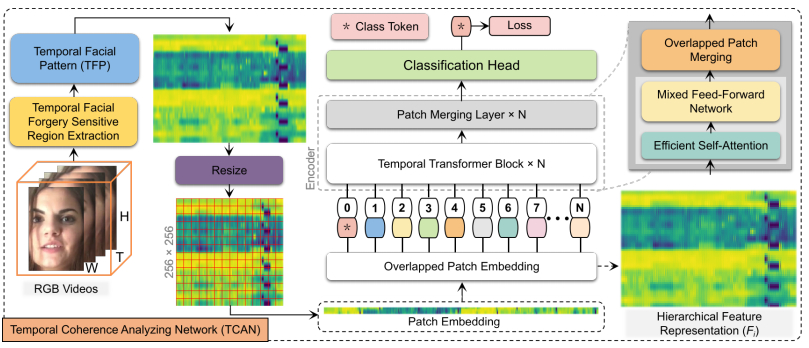
Neural signatures of working memory have been frequently identified in the spiking activity of different brain areas. However, some studies reported no memory-related change in the spiking activity of the middle temporal (MT) area in the visual cortex. However, recently it was shown that the content of working memory is reflected as an increase in the dimensionality of the average spiking activity of the MT neurons. This study aimed to find the features that can reveal memory-related changes with the help of machine-learning algorithms. In this regard, different linear and nonlinear features were obtained from the neuronal spiking activity during the presence and absence of working memory. To select the optimum features, the Genetic algorithm, Particle Swarm Optimization, and Ant Colony Optimization methods were employed. The classification was performed using the Support Vector Machine (SVM) and the K-Nearest Neighbor (KNN) classifiers. Our results suggest that the deployment of spatial working memory can be perfectly detected from spiking patterns of MT neurons with an accuracy of 99.65±0.12 using the KNN and 99.50±0.26 using the SVM classifiers.
Citation: Gayathri Vivekanandhan, Mahtab Mehrabbeik, Karthikeyan Rajagopal, Sajad Jafari, Stephen G. Lomber, Yaser Merrikhi. Applying machine learning techniques to detect the deployment of spatial working memory from the spiking activity of MT neurons[J]. Mathematical Biosciences and Engineering, 2023, 20(2): 3216-3236. doi: 10.3934/mbe.2023151
[1] | Yongsheng Lei, Meng Ding, Tianliang Lu, Juhao Li, Dongyue Zhao, Fushi Chen . A novel approach for enhanced abnormal action recognition via coarse and precise detection stage. Electronic Research Archive, 2024, 32(2): 874-896. doi: 10.3934/era.2024042 |
[2] | Min Li, Ke Chen, Yunqing Bai, Jihong Pei . Skeleton action recognition via graph convolutional network with self-attention module. Electronic Research Archive, 2024, 32(4): 2848-2864. doi: 10.3934/era.2024129 |
[3] | Hui Xu, Jun Kong, Mengyao Liang, Hui Sun, Miao Qi . Video behavior recognition based on actional-structural graph convolution and temporal extension module. Electronic Research Archive, 2022, 30(11): 4157-4177. doi: 10.3934/era.2022210 |
[4] | Jicheng Li, Beibei Liu, Hao-Tian Wu, Yongjian Hu, Chang-Tsun Li . Jointly learning and training: using style diversification to improve domain generalization for deepfake detection. Electronic Research Archive, 2024, 32(3): 1973-1997. doi: 10.3934/era.2024090 |
[5] | Lei Pan, Chongyao Yan, Yuan Zheng, Qiang Fu, Yangjie Zhang, Zhiwei Lu, Zhiqing Zhao, Jun Tian . Fatigue detection method for UAV remote pilot based on multi feature fusion. Electronic Research Archive, 2023, 31(1): 442-466. doi: 10.3934/era.2023022 |
[6] | Xiaoli Yang, Yan Liu . Abnormal dynamics of functional brain network in Apolipoprotein E ε4 carriers with mild cognitive impairment. Electronic Research Archive, 2024, 32(1): 1-16. doi: 10.3934/era.2024001 |
[7] | Rui Han, Shuaiwei Liang, Fan Yang, Yong Yang, Chen Li . Fully convolutional video prediction network for complex scenarios. Electronic Research Archive, 2024, 32(7): 4321-4339. doi: 10.3934/era.2024194 |
[8] | Lei Ma, Weiyu Wang, Yaozong Zhang, Yu Shi, Zhenghua Huang, Hanyu Hong . Multi-features combinatorial optimization for keyframe extraction. Electronic Research Archive, 2023, 31(10): 5976-5995. doi: 10.3934/era.2023304 |
[9] | Bin Zhang, Zhenyu Song, Xingping Huang, Jin Qian, Chengfei Cai . A practical object detection-based multiscale attention strategy for person reidentification. Electronic Research Archive, 2024, 32(12): 6772-6791. doi: 10.3934/era.2024317 |
[10] | Xing Wu, Shuai Mao, Luolin Xiong, Yang Tang . A survey on temporal network dynamics with incomplete data. Electronic Research Archive, 2022, 30(10): 3786-3810. doi: 10.3934/era.2022193 |
Neural signatures of working memory have been frequently identified in the spiking activity of different brain areas. However, some studies reported no memory-related change in the spiking activity of the middle temporal (MT) area in the visual cortex. However, recently it was shown that the content of working memory is reflected as an increase in the dimensionality of the average spiking activity of the MT neurons. This study aimed to find the features that can reveal memory-related changes with the help of machine-learning algorithms. In this regard, different linear and nonlinear features were obtained from the neuronal spiking activity during the presence and absence of working memory. To select the optimum features, the Genetic algorithm, Particle Swarm Optimization, and Ant Colony Optimization methods were employed. The classification was performed using the Support Vector Machine (SVM) and the K-Nearest Neighbor (KNN) classifiers. Our results suggest that the deployment of spatial working memory can be perfectly detected from spiking patterns of MT neurons with an accuracy of 99.65±0.12 using the KNN and 99.50±0.26 using the SVM classifiers.
The emergence of deepfake videos created by facial image manipulation methods poses a potential threat to our privacy and social safety. These deepfake videos can be effortlessly fabricated using publicly available software like Deepfakes [1] and DeepFaceLab [2]. Thus, developing effective and robust deepfake detection methods has become crucial. Fortunately, many deepfake detectors have been developed, which have shown promising results on various deepfake databases.
Current methods for detecting deepfake videos can be split into frame-level and video-level categories. Frame-level approaches [3,4,5,6,7,8] use a single video frame as intake and concentrate on identifying spatial forgery patterns such as RGB information, frequency statistics, auxiliary mask or blending boundary information, etc. Although these methods have achieved a great success, they have two critical drawbacks. First, the facial video created through frame-by-frame manipulation has two main types of artifacts. The first type is spatially associated, including checkboard, blur and blending boundary cues. The second type is temporal incoherence. Even cutting-edge forgery methods may create remarkably unpretentious facial images, they cannot eradicate unnatural and minute image transitions [4] among frames or temporal incoherence like facial parts jittering [5]. Unfortunately, the frame-level approaches fail to detect these temporal discriminatory traits and have capped performance. Second, the spatially associated cues are more dominant than temporal incoherence, and frame-level approaches tend to overfit the spatial cues. As a result, without a straightforward temporal design, current methods count more on spatial features than temporal incoherence to identify real or deepfake videos.
On the other hand, to address the limitations of frame-based methods, video-based deepfake detection techniques analyze a sequence of frames to identify inconsistencies in texture and structure within the video, making the detection process more resilient [9,10,11]. The early research employed general video analysis techniques such as 3-dimensional convolutional neural network (3D CNN) [12], recurrent neural network (RNN) [13], and long short-term memory (LSTM) [14]. However, these approaches are computationally expensive and have limitations in mining meaningful temporal features. This is because they are not designed explicitly for facial deepfake video detection, making it challenging to identify temporal inconsistencies. Furthermore, these models are less effective than frame-based methods, which can compute the video-level decision by averaging frame-level scores. Recently, researchers have proposed methods [15,16,17,18,19] that uniformly sample single frames at gaps to assemble the conclusive input sequence, resulting in better temporal modeling of deepfake videos. However, these approaches are more computationally intensive. Concurrently, while vision transformer [20] (ViT) methods have shown encouraging achievement in other computer vision studies, such as activity identification [21,22] and video object detection [23,24], their application to deepfake video detection has shown promising results as well. Not surprisingly, some researchers have tried to employ video transformers for detecting deepfake videos, but they suffer from high computational complexity, limited temporal modeling, and a lack of interpretability.
Our research is motivated by two observed facts. First, current facial image manipulation methods are explicitly designed at the image level, which requires applying the methods to each frame individually to generate a fake video. Regardless unpretentious shifts in appearance, like lighting, noise, and motion, often lead to temporal incoherent consequences, as shown in Figure 3. Second, detecting temporal incoherence is challenging since the annotated areas of the incoherence in the video are unavailable. Adopting complex temporal networks [25] to discern real from deepfake by temporal incoherence leads to high computational complexity, limited temporal modeling, and a lack of interpretability. Thus, detecting temporal incoherence requires more careful study and an interpretable approach.
To address these challenges for generalized deepfake video detection, in this paper, we devise a novel paradigm to mine and analyze comprehensive and unpretentious temporal coherence in two steps. In the first step, to leverage temporal coherence, we extract the spatial pixel variation of each red-green-blue (RGB) color channel from multiple facial areas that are forgery-sensitive, such as the forehead, eyes, nose, mouth, and chin areas. These areas form a set of multivariate sequences called the temporal facial pattern (TFP), which contains both local-successive temporal and global temporal perspectives. As the dynamic inconsistency occurs in local-successive temporal regions, these clues can characterize and interpret the distribution of forgery-sensitive areas in space and time, and they can be robustly used to expose deepfake videos. The second step involves interpreting the TFP significantly to understand the coherence of real and deepfake videos. Inspired by the affluent global attention attributes and self-attention mechanism of transformers in text and sequence processing, we propose a refined transformer framework, anointed temporal coherence analyzing network (TCAN), and employ its powerful mining ability to implicitly find the global intrinsic representation to expose the difference in coherence between real and synthetic videos. Specifically, in contrast to ViT, the TCAN framework redesigns the encoder and the classification head, eliminating some of its limitations of low-resolution features with fixed resolutions and high computation costs. In the first modification, the TCAN adapts position-free encoding, which helps adapt to any arbitrary resolution without impacting performance. Second, we introduce a hierarchical function that facilitates the encoder to generate high-resolution fine and low-resolution coarse features. Third, the classification head benefits from the transformer-induced features by aggregating information from different layers, combining global and local attention. Thus, we get explicit yet decisive representations for real and deepfake video classification. The proposed framework is depicted in Figure 1.
The key contribution of our approach includes:
● We propose a TCAN that explicitly analyzes the temporal clues to detect incoherence and exposes synthetic videos.
● We introduce a novel TFP based on the pixel variation of each RGB color channel from multiple regions in the possible forgery-sensitive facial areas to represent the local-successive temporal and global temporal views of real and deepfake videos.
● Thorough experiments on diverse datasets exhibit the dominance of our proposed approach concerning the generalization ability against unseen forgeries.
This paper is arranged as follows. We will begin with a summary of prior works in Section 2, followed by an explanation of our presented methodology in Section 3. Section 4 will present a detailed account of the experimental setup, and Section 5 will present our evaluation, analysis, and ablation study. Finally, in Section 6, we will conclude the paper by our conclusions and future work.
In deepfake synthesis, facial video manipulations are performed frame-by-frame, leading to inconsistencies between frames. Therefore, spatio-temporal incoherence can be used to detect deepfake videos more broadly. Some studies have focused on using temporal clues to detect fake face videos. In [12], 3D CNN is employed to illustrate spatio-temporal features. Some studies initially perform frame-level CNN feature extraction and then employ RNN [13,26] or LSTM [14,27] to learn the temporal artifacts. Nevertheless, the temporal clues extracted by these approaches are relatively crude. Gu et al. [10] present the spatial-temporal inconsistency learning (STIL) block to grasp temporal incoherence along two orthogonal paths. LipForensics was presented by Haliassos et al. [15], founded on high-level semantic abnormalities in mouth movements, to accomplish robust and generalizable deepfake detection. Recent studies [16,17,18,19] suggest constructing a local-global temporal standpoint to catch spatio-temporal cues.
There are specific methods based on transformers that are designed to detect deepfake videos. To capture long-scope dependencies along the temporal dimension and achieve generalizable deepfake detection, Zheng et al. [16] propose fully temporal convolutional network (FTCN), which uses transformers to mine spatio-temporal clues. Shao et al. [19] proposed SeqFakeFormer, which aims to accurately predict a sequential vector of face manipulation procedures instead of merely giving a binary label output. Additionally, Yu et al. [28] developed multiple spatiotemporal views transformer (MSVT), a new transformer model that incorporates local spatio-temporal views to capture dynamic inconsistency and global spatio-temporal views to extract video-wide features. Then, a global-local transformer integrates multi-level cues, providing a thorough spatio-temporal feature representation to enhance detection performance.
Similarly, many multi-modal detection approaches are proposed [29,30,31,32,33], which include methods based on the consistency among visual and audio modalities. The complementarity between the two modalities allows one modality to enhance the feature representation of the other. Cheng et al. [29] introduced a method based on voice-face conformity to detect manipulated deepfake video (VFD), which examines the uniformity between facial identity and audio in raw videos. Regardless, VFD cannot catch fiddled content without identity change, such as while glasses are removed. In another effort, Yang et al. proposed audio-visual-based learning for detecting deepfake (AVoiD-DF) [30], which includes a two-stream spatial-temporal encoder and a multi-modal coordinated decoder for collective intrinsic relationship learning. Despite only utilizing visual temporal clues, our proposed method aims to capture nuanced and thorough temporal features from local and global standpoints in an interpretable way with the least computation complexity, which is an improvement.
In this section, we foremost provide details regarding the multi-region TFP modeling process. Secondly, we present the transformer-based temporal coherence analyzing network for deepfake video detection, as illustrated in Figure 1. The TFP is modeled based on six facial areas, which are more susceptible to forgery artifacts, as shown in Figure 2. The areas from the forehead, eyes, nose, mouth, and chin are typically in constant motion. Hence, these regions will most likely contain temporal forgery cues while generating fake facial videos. A TFP is generated by placing these six regions over the length of a video, from top to bottom, in order from forehead to chin. This placement is designed with the patch embedding mechanism in mind, as used in a conventional transformer model. Every TFP row represents the varying color information extracted from each region in the pristine or deepfake video.
Further, the TCAN model relies on the hierarchical feature representation of the temporal hierarchical transformer encoder to analyze the temporal coherence within TFP for real and deepfake video identification. If we adopt a typical ViT and input the TFP directly for further learning, the original patch segmentation method cuts the images into nonoverlapping patches. This process will destroy the time information hidden in each row as it follows the specific patch size information and will discard discriminative information of some local neighboring informative regions. Therefore, to sort out this issue, we introduce the hierarchical representation through overlapping patches merging method to generate multi-level features similar to CNNs to focus on local information and modified ViT global attention mechanism based on sparse global connection, as illustrated in Figure 4, because generally ViT only generates fixed resolution feature map. Finally, all the features from the four encoder blocks within the TCAN are aggregated to obtain a final high-resolution fine and low-resolution coarse feature representation. Further, the self-attention and data-driven positional encoding mechanisms are employed to incorporate location information within TFP for temporal coherence analysis, and the classification head predicts the outcome based on this analysis.
The proposed multi-region TFP is a novel representation of the facial forgery-sensitive areas in terms of color variations that incorporate local and global cues. The approach is detailed in Figure 2 and further elaborated below.
In our approach, we employ an 81-point Dlib [34] face detector to catch the face landmarks. On the basis of detected landmarks, we determine the most instructive regions in the face that are susceptible to forgery, including the forehead (landmark points from 68 to 77), left eye (from 36 to 39), right eye (from 42 to 45), nose (from 27 to 35), mouth (from 48 to 67), and chin (from 4 to 12). As the detector can operate at a frame rate of 30 plus frames per second, we do face and landmark detection on each video sequence frame to obtain precise and uniform region facial areas.
We also must regard instances where some facial landmarks are not detected for rare frames, which can occur when the head of a person rotates or moves too quickly. This results in missing information on signals that vary in color over time. To address this problem, we artificially simulate the missing data cases by randomly masking a miniature portion of the temporal pattern along the temporal dimension. We then use this somewhat masked temporal pattern as augmented information to improve the performance of the TCAN model.
In real or deepfake video processing, each video sequence with c color space dimensions and T frames undergoes a face and landmark detection process. If a face and its landmarks are detected, the facial regions per frame are defined and split into n blocks, and the mean color intensity of each block is calculated over time. This helps analyze and understand the changes in color intensity of different facial regions over time.
Further, to enhance the signal-to-noise ratio (SNR) and reduce noise within TFP, our method relies on the variation in pixel values of the RGB channels of the entire facial area. This type of average pooling presentation offers greater robustness than analyzing individual pixels. For each region block, three temporal sequences are generated based on the various channels of the RGB color space. Moreover, a min-max normalization per sequence is applied. The n temporal sequences for each color dimension are arranged in rows to create a TFP of the areas that are susceptible to forgery in the video clip.
In the context of a video with c color space channels and T frames, we extract facial regions in each frame from the forehead, mouth, nose, and eye areas. Further, we divide the face region of each frame into n block regions Rt={R1t,R2t,…,Rnt}. Let C(x,y,t) represent the value at point (x,y) of the tth frame using the various color space dimensions. We determine the average pooling of every channel for the tth block region of the tth frame, where |Ri| represents the area of a block region (in terms of the number of pixels).
¯Ci(t)=∑x,y∈Ri⋅C(x,y,t)|Ri| | (3.1) |
So, for every video sequence, we can derive a set of temporal sequences 3×n for different channels of the RGB color space with the length of T, denoted as Ci={Ci(1),Ci(2),…,Ci(T)}, where C refers to one of the color channel within c and i refers to the region block index. After applying a min-max normalization to every temporal sequences and scaling the data into the [0,255] range, we arrange the n temporal signals into rows, which yields a TFP of the natural facial video sequence with T×n×c size. The TFP samples of real and deepfake videos from the FF++ [35] dataset with various compression levels are shown in Figure 3.
This section provides a brief overview of our proposed TCAN design. TCAN comprises two primary components: (1) a temporal hierarchical transformer encoder (THTE) that generates low-resolution fine and high-resolution coarse characteristics, and (2) a classification head, as illustrated in Figure 4. To begin, we divide a TFP of size H×W×3 into patches of size 7×7. In contrast to ViT [20], which employs size 16×16 patches, smaller patches are more favorable for fine-grain temporal coherence analysis. We then input these patches to the THTE, which generates multi-level features at {14,18,116,132} that preserve the actual TFP resolution. Finally, the classification head intakes these multi-level features to foresee the output class.
The THTE design is partly inspired by ViT [20,36] but tailored for deepfake video detection. It benefits from four key contributions, such as hierarchical feature representation, overlapped patch merging, efficient self-attention, and mixed feed-forward network. These modules are detailed below.
The representation module is designed to generate multi-level features similar to CNNs, unlike ViT, which produces a uni-resolution cue map. The high-resolution fine-grained and low-resolution coarse features extraction from an input TFP image can enhance the performance of temporal classification tasks. To achieve this, we use patch merging on an input TFP image of resolution H×W×3 to get a hierarchical feature map Fi with H2i+1×W2i+1×Ci resolution, where i∈{1,2,3,4}. The resolution of each level Ci+1 is greater than the previous level Ci, as demonstrated in Figure 4.
The process of merging patches in a TFP patch involves unifying an N×N×3 patch into a 1×1×C vector, which is used in baseline ViT. We can extend this process to confine a 2×2×Ci feature course into a 2×2×Ci+1 vector, thus obtaining hierarchical feature maps. Shrinking hierarchical features from F1(H4×W4×C1) to F2(H8×W8×C2) and iterating for additional feature maps in the hierarchy can be achieved using this method. However, this technique was originally devised to merge feature patches or non-overlapping images, resulting in the failure to keep the local continuity information about those patches, which is not suggestible for temporal information analysis. Thus, an overlapping patch merging method is employed instead, requiring us to define P, K, and S, where P is the padding size, K denotes patch size, and the stride between two adjacent patches is S. During experiments, we fixed P=3, K=7, and S=4 and P=1, K=3, and S=2 to achieve overlapping patch blending to produce features the exact size as the non-overlapping strategy.
Self-attention layer is the primary computational bottleneck for the encoder blocks. In the standard multi-head self-attention procedure, every head (Q,K,V) has the exact dimensions N×C as the length of the sequence (N=H×W). The self-attention is calculated as follows:
Attention(Q,K,V)=Softmax(QKT√dhead)⋅V. | (3.2) |
The computational sophistication of the current procedure is O(N2), which can be a challenge for processing large image resolutions. However, we have an alternative approach that utilizes the sequence-lessening technique described in [37]. This technique involves using a reduction ratio R to effectively shorten the sequence length without compromising its quality as follows:
ˆK=Reshape(NR,C⋅R)(K), | (3.3) |
K=Linear(C⋅R,C)⋅(ˆK). | (3.4) |
In the technical context, while K represents the sequence that needs to be reduced, (NR,C⋅R)(K) refers to the reshaping of K to match the shape of NR×(C⋅R). The Linear(Cin⋅Cout)(⋅), in this context, directs to a linear layer that takes a Cin-dimensional tensor as intake and generates a Cout-dimensional tensor as outcome. The resulting tensor K has dimensions NR×C, which reduces the self-attention mechanism complexity from O(N2) to O(N2R). We set R value to [64,16,4,1] from block 1 to 4.
The ViT [20] introduces positional encoding (PE) to incorporate location information. Nevertheless, the PE resolution is fixed, which causes accuracy drops when the inference resolution is dissimilar from the training resolution and the interpolation of positional code is required. To deal with this issue, the CPVT [38] model uses a 3×3 convolutional layer with the PE to create a data-controlled PE. We use a new mixed feed-forward network (mixed-FFN) approach that employs a 3×3 convolutional layer (Conv) straight in the FFN to consider the zero padding effect and minimize location information leakage, as in [39]. The mixed-FFN approach streamlines as in Eq (3.5).
xout=MLP(GELU(Conv3×3(MLP(xin))))+xin | (3.5) |
The self-attention module uses the feature xin. Each FFN in mixed-FFN combines a multilayer perceptron (MLP) and a 3×3 convolution. A 3×3 convolution is enough for our temporal transformer to get positional information required for temporal coherence analysis. In respective, we employ depth-wise convolutions to reduce parameters and enhance performance.
The TCAN model uses a classification head that consists of a single MLP layer. This layer allows us to fuse multi-level features from our THTE and achieve a more considerably effective receptive field (ERF) compared to conventional CNN encoders. Specifically, there are two main phases in the classification head. The first phase unifies the multi-level features Fi from THTE in the channel dimension. In the second phase, the concatenated feature embeddings are used to predict the output class categories. These steps can be formulated as:
y=Linear(4Ci,Ncls)(Concat(Fi)),∀i, | (3.6) |
where y denotes the predicted class, Linear(Ci,Ncls)(⋅) and represents a linear layer with Ci and Ncls as intake and outcome vector dimensions, respectively. Table 1 provides parameters of TCAN model.
Model modules | Information |
No. of encoder blocks | 4 |
No. of attention heads | [1, 2, 5, 8] |
Patch sizes | [7, 3, 3, 3] |
Strides | [4, 2, 2, 2] |
Classifier hidden size | 256 |
Parameters | 3.3 million |
FLOPs | 8.4 G |
This section outlines the datasets, the evaluation metric, hyperparameters, and the loss function.
The efficacy of the TCAN model was evaluated through experiments conducted on five renowned benchmark datasets, namely FaceForensics++ [35] (FF++), DeepFakeDetection (DFD) [40], Deepfake Detection Challenge [41] (DFDC), Celeb-DF-V2 [42] (CDF-V2), and DeeperForensics-1.0 [43] (Deeper). FF++ is comprised of subsets such as DeepFakes (DF), FaceSwap (FS), Face2Face (F2F), and NeuralTextures (NT), each having three compression ratios (raw (c0), high quality (c23), and low quality (c40)). For each dataset, 1000 videos were chosen and divided into separate subsets for training, validation, and testing as in [35]. A more detailed summary of the datasets is provided in Table 2.
Dataset | Manipulation methods | Real/Fake |
FF++ [35] | DF, F2F, FS, NT | 1000/4000 |
DFD [40] | Improved DF | 363/3068 |
DF-256, MM/NN, NTH, | ||
DFDC [41] | Audio swaps, DF-128, FSGAN, | 23,654/104,500 |
StyleGAN, refinement | ||
CDF-V2 [42] | Enhanced FaceSwap DF | 590/5639 |
Deeper [43] | DF-VAE with seven perturbations | 1000/9000 |
We employ the area under the receiver operating characteristic curve [44] (AUC) as an evaluation metric following the previous works [16,28], which is standard practice in most studies. The AUC values are calculated based on video-level evaluation.
In the following subsection, we provide information regarding key hyperparameters and preprocessing techniques used in the training and testing of the TCAN model. We employ the learning rate (lr) of 5e−5 and the AdamW optimizer with a cosine annealing learning rate scheduler. All models are trained with a maximum of 15 epochs. All the TCAN models are trained on a NVIDIA RTX 3090Ti with batch size 16, using mix-precision training [45] (bfloat-16). The input TFP is resized to 256×256 during the training process. We apply a normalization transformation to preprocess the data using dataset-specific mean and standard deviation values. These values (i.e., approximately [0.2292, 0.5373, 0.4988] for means and [0.1439, 0.1881, 0.0986] for standard deviations) ensure the dataset is standardized for all the experimentation purposes.
The cross-entropy loss function LCE [46] is employed in the training of the TCAN model.
LCE=−(y⋅log(p)+(1−y)⋅log(1−p)), | (4.1) |
where y represents the binary label, and p in [0,1] are the predicted probability for the positive class.
In this section, we evaluate our proposed deepfake video detection framework through experiments that included intra-, cross-dataset, and robustness scenarios. These experiments are designed to simulate the detection of previously unseen forgeries, cross-domain, and robustness settings against compression ratios. To train our framework, we used the FF++ [35] subsets at the c0 compression level, and for testing, we utilized the c0, c23, and c40 sets (see Tables 3 and 5) to perform intra- and robustness evaluations. We trained our framework on the complete FF++ [35] (c0) dataset for cross-dataset evaluations and tested it on the DFD [40], DFDC [41], CDF-V2 [42], and Deeper [43] datasets (refer to Table 4). We also compared our results in intra- and cross-dataset settings against state-of-the-art methods. Additionally, we performed an ablation study by replacing the TCAN with different state-of-the-art transformer methods. Using publicly available codes, we implemented the comparison methods in the same environment as ours.
Type | Methods | Trained on remaining three | Avg. | #params. | |||
DF | FS | F2F | NT | ||||
Frame-level | LRLNet [7] | 91.37 | 89.44 | 90.22 | 85.83 | 89.22 | 46.3 M |
PEL [8] | 89.78 | 87.67 | 88.91 | 87.78 | 88.54 | 33.7 M | |
Video-level | S-MIL [9] | 87.68 | 85.26 | 85.85 | 84.49 | 85.82 | 42.2 M |
STIL [10] | 90.71 | 88.73 | 91.73 | 88.78 | 89.99 | 32.5 M | |
LipForensics [15] | 97.83 | 90.51 | 98.02 | 96.90 | 95.82 | 36.0 M | |
FTCN [16] | 96.71 | 96.02 | 96.07 | 96.24 | 96.26 | 26.6 M | |
DIL [17] | 94.36 | 94.77 | 94.81 | 93.24 | 94.3 | 41.4 M | |
ECGL [18] | 92.49 | 90.96 | 89.59 | 89.19 | 90.56 | 37.3 M | |
SeqFakeFormer [19] | 96.82 | 95.08 | 95.75 | 95.27 | 95.73 | 104 M | |
Two-branch [27] | 89.81 | 87.28 | 90.06 | 86.92 | 88.52 | 67.1 M | |
MSVT [28] | 98.06 | 97.43 | 96.68 | 97.19 | 97.34 | 26.5 M | |
VDF [29] | 96.20 | 86.31 | 89.60 | 94.25 | 91.62 | 40 M | |
Audio-DF [30] | 97.31 | 94.74 | 94.50 | 95.10 | 95.41 | 89 M | |
TCAN (Ours) | 99.16 | 98.93 | 99.03 | 98.15 | 98.82 | 3.3 M |
Type | Methods | Trained on all four subsets | Avg. | #params. | |||
DFD | DFDC | CDF-V2 | Deeper | ||||
Frame-level | LRLNet [7] | 77.61 | 76.61 | 78.37 | 85.86 | 79.61 | 46.3 M |
PEL [8] | 89.29 | 65.87 | 70.29 | 70.41 | 73.97 | 33.7 M | |
Video-level | S-MIL [9] | 86.39 | 66.79 | 75.86 | 83.07 | 78.03 | 42.2 M |
STIL [10] | 87.21 | 67.22 | 76.17 | 83.94 | 78.64 | 32.5 M | |
LipForensics [15] | 84.25 | 73.62 | 83.60 | 97.00 | 84.62 | 36.0 M | |
FTCN [16] | 90.46 | 74.17 | 86.95 | 97.95 | 87.38 | 26.6 M | |
DIL [17] | 88.15 | 72.52 | 82.82 | 86.09 | 82.40 | 41.4 M | |
ECGL [18] | 90.18 | 73.09 | 85.86 | 96.62 | 86.94 | 37.3 M | |
SeqFakeFormer [19] | 90.22 | 74.32 | 84.26 | 95.96 | 86.19 | 104 M | |
Two-branch [27] | 86.19 | 70.18 | 75.79 | 81.34 | 78.38 | 67.1 M | |
MSVT [28] | 91.36 | 76.79 | 88.81 | 98.42 | 88.85 | 26.5 M | |
VDF [29] | 90.50 | 85.10 | 80.70 | 84.30 | 85.15 | 40 M | |
Audio-DF [30] | 91.12 | 82.31 | 86.20 | 96.50 | 89.03 | 89 M | |
TCAN (Ours) | 91.09 | 86.94 | 88.99 | 98.80 | 91.46 | 3.3 M |
Compression level | Trained on remaining three | Avg. | |||
DF | FS | F2F | NT | ||
c23 | 85.23 | 79.67 | 76.84 | 74.14 | 78.97 |
c40 | 70.21 | 73.16 | 70.59 | 69.28 | 70.81 |
The differences among facial forgery videos largely stem from variations in reference videos and facial alteration techniques. To assess the cross-manipulation generalization ability of our method against various deepfake detectors and eliminate potential biases oriented by distinct reference videos, we performed experimentations on the FF++ [35] dataset, as this dataset supplies fabricated videos generated by four facial forgery techniques for the same reference videos. We utilized the leave-one-out approach to evaluate the efficacy of our face forgery detectors, as presented in the study by [28]. The evaluation process entailed using each of the four categories of deepfake videos in the FF++ [35] dataset as a test dataset. Conversely, the remaining three kinds formed the training dataset. The training and evaluation phases are performed on the FF++ [35] dataset c0 variant. The outcomes are summarized in Table 3, while the learned feature spaces are visualized in Figure 5 using t-SNE [47] plots.
Table 3 demonstrates the impressive detection results of our approach, with a generalization AUC score of 98.82% for unique forgeries. Our approach surpasses most existent methods by a significant margin on all four types of manipulations in FF++ [35] (DF, FS, F2F, and NT) that use different methods and have distinct artifacts. Our method can learn generalized discriminative features on three manipulations and generalize to the remaining one. Compared to contemporary state-of-the-art approaches such as MSVT [28], FTCN [16], SeqFakeFormer [19], and LipForensics [15], our approach achieves a 1.48%, 2.56%, 3.09%, and 3.0% increase in video-level AUC, respectively. Moreover, our approach accomplishes the best performance with the tiniest number of parameters (M mean millions), without any external training data or pre-training, which demonstrates superiority of our framework.
Current deepfake videos are generally generated using a variety of synthesis sources. Therefore, it is crucial to test their cross-domain generalization capability. To test the generalization capacity of TCAN, we trained our model on the FF++ [35] dataset and tested it on DFD [40], DFDC [41], CDF-V2 [42], and Deeper [43] datasets. We achieved auspicious detection results corresponding to the aforementioned approaches in Table 4, indicating robustness improvement of our method. Our approach achieved promising results in comparison to both video-level and frame-level approaches. Specifically, our method achieved an outstanding AUC score of 98.80% on the Deeper [43] dataset, which was generated using advanced synthesis techniques on real videos from the FF++ [35] dataset. Likewise, our method yielded an AUC score of 91.09% on the DFD [40] dataset but slightly underperformed in comparison to the likes of MSVT [28] (91.36% AUC) and Audio-DF [30] (91.12% AUC), generated by Google using an improved deepfake generation pipeline. However, generalization was more challenging for unseen datasets, such as CDF-V2 [42] and DFDC [41]. We found that video-based methods, such as MSVT [28] (76.79% AUC), VDF [29] (85.10% AUC), Audio-DF [30] (82.31% AUC), three state-of-the-art transformer-based approaches, and our TCAN with 86.94% AUC, outperformed the frame-level state-of-the-art approaches on the DFDC [41] dataset, such as LRLNet [7] (76.61% AUC) and PEL [8] (65.87% AUC), which suggests that temporal information is crucial in enhancing the cross-domain generalization ability of deepfake video detection methods. Similar trends are also shown on the CDF-V2 [42] dataset in Table 4. Figure 6 shows the feature spaces learned by our method across different datasets.
Further, we have observed that LipForensics [15], FTCN [16], MSVT [28], VDF [29], and Audio-DF [30], all attempt to utilize the temporal incoherence in deepfake videos to enhance the generalization detection capability. In contrast, our approach differs because we rely on two separate paradigms: temporal modeling to extract facial color variation effectively and coherence analysis through self-attention to comprehend the short-term inter-frame incoherence within TFP. On the other hand, the approaches mentioned employ complex networks or a temporal transformer for temporal information extraction to enhance long-term relationship learning, which increases computational complexity. Nevertheless, MSVT [28] has more extended input video frames and may function better on datasets like DFD [40] and CDF-V2 [42], where the head poses and lighting circumstances are even among frames. Regardless, our approach offers several edges. Firstly, we achieve better cross-dataset performance on challenging datasets compared with MSVT [28], VDF [29], and Audio-DF [30], such as DFDC [41], where it is challenging to distinguish whether the incoherence is caused by deepfake cues, head pose shifts or lighting circumstances in elongate sequences. Secondly, our approach provides more reasonable interpretability as we individually oversee the temporal modeling and detection task in TCAN. Lastly, our model displays better intra-dataset performance against unseen manipulation sources and cross-dataset performance against unseen dataset source domains due to the fine-grain temporal incoherence learning ability of the transformer model based on the high-resolution fine and low-resolution coarse feature representation, resulting in better detection results on most datasets.
In the presented ablations, we scrutinize the robustness of our method against compression on FF++ [35] subsets and test the interoperability of the proposed TFP mechanism by adopting other state-of-the-art backbone transformer models.
The transmission of multimedia content through social media networks often goes through JPEG compression. Hence, robustness evaluation is a crucial scale to evaluate the performance of our proposed deepfake detector. By adjusting the quality factor of JPEG compression, we are able to control the level of perturbation in our robustness analysis. Our approach, TCAN, has demonstrated robustness in the results presented in Table 5. We assess the robustness of TCAN using the leave-one-out approach, following the methodology proposed by [28]. We employ the FF++ [35] dataset to train the TCAN model at compression level c0 and then test it at compression levels of c23 and c40.
At compression levels c23 and c40, our proposed TCAN framework performance drops by an approximate margin of 19% in comparison to the results stated in Table 3. The primary reason for the reduced detection performance is the lossy compression of test data, which discards some high-frequency image details and textures that contain forgery artifacts. Our TFP mechanism relies on the spatial information of facial videos, but compression makes it unable to extract the features as sensitively as those from the data at compression level c0. However, our proposed method, which includes temporal self-attention and feature fusion mechanisms, has been demonstrated to be positively influential in enhancing the robustness of the TCAN model.
We conduct an ablation study to assess the performance impact of integrating our proposed TFP mechanism with various state-of-the-art backbone models, including ViT [20], RegNet [48], SwinV2 [49], BEiT [50], and PoolFormer (PF) [51]. The evaluation results are compared against our TCAN method in Table 6, where the FF++ [35] dataset is used for training at compression level c0 with two combinations for intra- and cross-dataset evaluation.
Methods | Trained on remaining three | Trained on all four | Avg. | #params. | |||||
DF | FS | F2F | NT | DFDC | CDF-V2 | Deeper | |||
ViT [20] | 96.30 | 98.34 | 98.31 | 95.25 | 86.28 | 82.15 | 97.61 | 93.46 | 85.8 M |
RegNet [48] | 97.71 | 97.30 | 98.55 | 97.98 | 82.51 | 78.69 | 96.50 | 92.74 | 43.9 M |
SwinV2 [49] | 97.99 | 96.53 | 96.91 | 93.09 | 77.76 | 78.89 | 93.53 | 90.67 | 27.5 M |
BEiT [50] | 96.21 | 98.21 | 97.20 | 96.82 | 77.19 | 87.69 | 96.88 | 92.88 | 85.7 M |
PF [51] | 98.98 | 98.78 | 98.02 | 98.08 | 84.04 | 85.18 | 98.77 | 94.55 | 11.4 M |
TCAN (Our) | 99.16 | 98.93 | 99.03 | 98.15 | 86.94 | 88.99 | 98.80 | 95.71 | 3.3 M |
Integrating our TFP mechanism with ViT [20] demonstrates a competitive AUC of 93.46%, showcasing its adaptability and effectiveness. Our TFP mechanism performs well when unified with RegNet [48], achieving a video-level AUC of 92.74%, indicating its compatibility and positive impact on diverse backbone architectures. Similarly, the SwinV2 [49] backbone, coupled with our TFP, achieves an AUC of 90.67%, affirming the versatility of our approach across different model architectures. Integrating our TFP with BEiT [50] results in a 92.88% AUC score, demonstrating its ability to learn TFP among various models. In one of the most promising second choices as a backbone network for coherence analysis, PF [51] showcases effective detection performance with an overall average AUC score of 94.55% in all categories of intra- and cross-dataset evaluations. In comparison, our TCAN method serves as the baseline, achieving the best and most competitive video-level AUC scores across all evaluated backbones, as shown in Table 6, emphasizing its effectiveness compared to existing methods. In short, our ablation study illustrates that the proposed TFP mechanism within our framework can be adapted to other backbone networks as well. Additionally, without any tailored network design for deepfake detection tasks, it consistently maintains its performance across various state-of-the-art backbone models, emphasizing its adaptability and effectiveness in learning TFP representation for deepfake detection.
In this paper, we have presented a novel bi-level paradigm for deepfake video detection, which consists of temporal modeling and coherence analysis. In level one, we have employed the temporal modeling mechanism for generalized detection, which decomposes real or deep fake videos into TFP containing subtle and dynamic local-global temporal clues from the facial forgery-sensitive areas based on color variations. The second level is based on the TCAN to mine and capture vital dynamic inconsistencies. TCAN first employs dynamic feature extraction and aggregation mechanisms to integrate multi-level temporal features effectively, and then analyzes the local-global temporal perspectives within TFP by utilizing global temporal self-attention for robust incoherence identification. Our proposed approach dramatically enhances robustness and generalized performance corresponding to prior deepfake video detection techniques, including current video transformers. The empirical validation of our approach is conducted on a range of large-scale deepfake datasets, such as intra- and cross-dataset evaluations on FF++ subsets, DFD, DFDC, CDF-V2, and Deeper. TCAN outperformed all its competitors in the intra- and cross-evaluations with comprehensive margins on the FF++ subsets (DF by 1.1%, FS by 1.5%, F2F by 1.01%, NT by 0.96%), DFDC by 1.84%, CDF-V2 by 0.18%, and Deeper by 0.38%, which further provide evidence of our proposed method's effectiveness. In future research, we will explore different color spaces to refine the temporal modeling design and incorporate audio modality for enhanced deepfake video detection. We will also devise a visualization strategy that can help us better comprehend the mechanics behind deepfake video detection by transformer models.
The authors declare they have not used Artificial Intelligence (AI) tools in the creation of this article.
The Science and Technology Foundation of Guangzhou Huangpu Development District provided funding for this research work under Grant No. 2022GH15.
The authors declare there is no conflict of interest.
[1] |
A. E. Maxwell, T. A. Warner, F. Fang, Implementation of machine-learning classification in remote sensing: An applied review, Int. J. Remote Sens., 39 (2018), 2784–2817. https://doi.org/10.1080/01431161.2018.1433343 doi: 10.1080/01431161.2018.1433343
![]() |
[2] |
D. Maulud, A. M. Abdulazeez, A review on linear regression comprehensive in machine learning, J. Appl. Sci. Technol. Trends, 1 (2020), 140–147. https://doi.org/10.38094/jastt1457 doi: 10.38094/jastt1457
![]() |
[3] |
S. Sun, Z. Cao, H. Zhu, J. Zhao, A survey of optimization methods from a machine learning perspective, IEEE Trans. Cybern., 50 (2019), 3668–3681. https://doi.org/10.1109/TCYB.2019.2950779 doi: 10.1109/TCYB.2019.2950779
![]() |
[4] |
I. M. Ibrahim, A. M. Abdulazeez, The role of machine learning algorithms for diagnosing diseases, J. Appl. Sci. Technol. Trends, 2 (2021), 10–19. https://doi.org/10.38094/jastt20179 doi: 10.38094/jastt20179
![]() |
[5] |
J. Wang, M. Wang, Review of the emotional feature extraction and classification using EEG signals, Cognit. Rob., 1 (2021), 29–40. https://doi.org/10.1016/j.cogr.2021.04.001 doi: 10.1016/j.cogr.2021.04.001
![]() |
[6] |
A. I. Kadhim, Survey on supervised machine learning techniques for automatic text classification, Artif. Intell. Rev., 52 (2019), 273–292. https://doi.org/10.1007/s10462-018-09677-1 doi: 10.1007/s10462-018-09677-1
![]() |
[7] |
C. Jobanputra, J. Bavishi, N. Doshi, Human activity recognition: A survey, Procedia Comput. Sci., 155 (2019), 698–703. https://doi.org/10.1016/j.procs.2019.08.100 doi: 10.1016/j.procs.2019.08.100
![]() |
[8] |
O. Karabiber Cura, S. Kocaaslan Atli, H. S. Türe, A. Akan, Epileptic seizure classifications using empirical mode decomposition and its derivative, Biomed. Eng. Online, 19 (2020), 1–22. https://doi.org/10.1186/s12938-020-0754-y doi: 10.1186/s12938-020-0754-y
![]() |
[9] |
R. Zebari, A. Abdulazeez, D. Zeebaree, D. Zebari, J. Saeed, A comprehensive review of dimensionality reduction techniques for feature selection and feature extraction, J. Appl. Sci. Technol. Trends, 1 (2020), 56–70. https://doi.org/10.38094/jastt1224 doi: 10.38094/jastt1224
![]() |
[10] |
H. Namazi, A. Daneshi, H. Azarnoush, S. Jafari, F. Towhidkhah, Fractal-based analysis of the influence of auditory stimuli on eye movements, Fractals, 26 (2018), 1850040. https://doi.org/10.1142/S0218348X18500408 doi: 10.1142/S0218348X18500408
![]() |
[11] |
H. Alipour, F. Towhidkhah, S. Jafari, A. Menon, H. Namazi, Complexity-based analysis of the relation between fractal visual stimuli and fractal eye movements, Fluctuation Noise Lett., 18 (2019), 1950012. https://doi.org/10.1142/S0219477519500123 doi: 10.1142/S0219477519500123
![]() |
[12] |
H. Namazi, E. Aghasian, T. S. Ala, Fractal-based classification of electroencephalography (EEG) signals in healthy adolescents and adolescents with symptoms of schizophrenia, Technol. Health Care, 27 (2019), 233–241. https://doi.org/10.3233/THC-181497 doi: 10.3233/THC-181497
![]() |
[13] |
H. Namazi, R. Khosrowabadi, J. Hussaini, S. Habibi, A. Farid, V. V. Kulish, Analysis of the influence of memory content of auditory stimuli on the memory content of EEG signal, Oncotarget, 7 (2016), 56120–56128. https://doi.org/10.18632/oncotarget.11234 doi: 10.18632/oncotarget.11234
![]() |
[14] |
A. Narin, Y. Isler, M. Ozer, M. Perc, Early prediction of paroxysmal atrial fibrillation based on short-term heart rate variability, Physica A, 509 (2018), 56–65. https://doi.org/10.1016/j.physa.2018.06.022 doi: 10.1016/j.physa.2018.06.022
![]() |
[15] |
Y. Isler, A. Narin, M. Ozer, M. Perc, , Multi-stage classification of congestive heart failure based on short-term heart rate variability, Chaos, Solitons Fractals, 118 (2019), 145–151. https://doi.org/10.1016/j.chaos.2018.11.020 doi: 10.1016/j.chaos.2018.11.020
![]() |
[16] |
M. Mehrabbeik, S. Rashidi, A. Fallah, E. R. Khoshnood, Phonocardiography-based mitral valve prolapse detection with using fractional fourier transform, Biomed. Phys. Eng. Express, 7 (2020), 015003. https://doi.org/10.1088/2057-1976/abcaab doi: 10.1088/2057-1976/abcaab
![]() |
[17] |
S. Ansari Nasab, S. Panahi, F. Ghassemi, S. Jafari, K. Rajagopal, D. Ghosh, et al., Functional neuronal networks reveal emotional processing differences in children with ADHD, Cogn. Neurodyn., 16 (2022), 91–100. https://doi.org/10.1007/s11571-021-09699-6 doi: 10.1007/s11571-021-09699-6
![]() |
[18] |
Y. Merrikhi, K. Clark, B. Noudoost, Concurrent influence of top-down and bottom-up inputs on correlated activity of Macaque extrastriate neurons, Nat. Commun., 9 (2018), 5393. https://doi.org/10.1038/s41467-018-07816-4 doi: 10.1038/s41467-018-07816-4
![]() |
[19] |
Y. Merrikhi, K. Clark, E. Albarran, M. Parsa, M. Zirnsak, T. Moore, et al., Spatial working memory alters the efficacy of input to visual cortex, Nat. Commun., 8 (2017), 15041. https://doi.org/10.1038/ncomms15041 doi: 10.1038/ncomms15041
![]() |
[20] |
Y. Merrikhi, M. Shams-Ahmar, H. Karimi-Rouzbahani, K. Clark, R. Ebrahimpour, B. Noudoost, Dissociable contribution of extrastriate responses to representational enhancement of gaze targets, J. Cognit. Neurosci., 33 (2021), 2167–2180. https://doi.org/10.1162/jocn_a_01750 doi: 10.1162/jocn_a_01750
![]() |
[21] |
D. Zaksas, T. Pasternak, Directional signals in the prefrontal cortex and in area MT during a working memory for visual motion task, J. Neurosci., 26 (2006), 11726–11742. https://doi.org/10.1523/JNEUROSCI.3420-06.2006 doi: 10.1523/JNEUROSCI.3420-06.2006
![]() |
[22] |
J. W. Bisley, D. Zaksas, J. A. Droll, T. Pasternak, Activity of neurons in cortical area MT during a memory for motion task, J. Neurophysiol., 91 (2004), 286–300. https://doi.org/10.1152/jn.00870.2003 doi: 10.1152/jn.00870.2003
![]() |
[23] |
L. Chelazzi, E. K. Miller, J. Duncan, R. Desimone, Responses of neurons in macaque area V4 during memory-guided visual search, Cereb. Cortex, 11 (2001), 761–772. https://doi.org/10.1093/cercor/11.8.761 doi: 10.1093/cercor/11.8.761
![]() |
[24] |
M. Mehrabbeik, M. Shams-Ahmar, A. T. Levine, S. Jafari, Y. Merrikhi, Distinctive nonlinear dimensionality of neural spiking activity in extrastriate cortex during spatial working memory; a Higuchi fractal analysis, Chaos, Solitons Fractals, 158 (2022), 112051. https://doi.org/10.1016/j.chaos.2022.112051 doi: 10.1016/j.chaos.2022.112051
![]() |
[25] |
D. Mendoza-Halliday, S. Torres, J. C. Martinez-Trujillo, Sharp emergence of feature-selective sustained activity along the dorsal visual pathway, Nat. Neurosci., 17 (2014), 1255–1262. https://doi.org/10.1038/nn.3785 doi: 10.1038/nn.3785
![]() |
[26] |
K. M. Armstrong, M. H. Chang, T. Moore, Selection and maintenance of spatial information by frontal eye field neurons, J. Neurosci., 29 (2009), 15621–15629. https://doi.org/10.1523/JNEUROSCI.4465-09.2009 doi: 10.1523/JNEUROSCI.4465-09.2009
![]() |
[27] |
X. Zhou, F. Katsuki, X. L. Qi, C. Constantinidis, Neurons with inverted tuning during the delay periods of working memory tasks in the dorsal prefrontal and posterior parietal cortex, J. Neurophysiol., 108 (2012), 31–38. https://doi.org/10.1152/jn.01151.2011 doi: 10.1152/jn.01151.2011
![]() |
[28] |
Z. Bahmani, M. R. Daliri, Y. Merrikhi, K. Clark, B. Noudoost, Working memory enhances cortical representations via spatially specific coordination of spike times, Neuron, 97 (2018), 967–979. https://doi.org/10.1016/j.neuron.2018.01.012 doi: 10.1016/j.neuron.2018.01.012
![]() |
[29] |
R. Esteller, G. Vachtsevanos, J. Echauz, B. Litt, A comparison of waveform fractal dimension algorithms, IEEE Trans. Circuits. Syst. I Fundam. Theor. Appl., 48 (2001), 177–183. https://doi.org/10.1109/81.904882 doi: 10.1109/81.904882
![]() |
[30] |
T. Higuchi, Approach to an irregular time series on the basis of the fractal theory, Physica D, 31 (1988), 277–283. https://doi.org/10.1016/0167-2789(88)90081-4 doi: 10.1016/0167-2789(88)90081-4
![]() |
[31] |
M. J. Katz, Fractals and the analysis of waveforms, Comput. Biol. Med., 18 (1988), 145–156. https://doi.org/10.1016/0010-4825(88)90041-8 doi: 10.1016/0010-4825(88)90041-8
![]() |
[32] |
R. Morales, T. Di Matteo, R. Gramatica, T. Aste, Dynamical generalized Hurst exponent as a tool to monitor unstable periods in financial time series, Physica A, 391 (2012), 3180–3189. https://doi.org/10.1016/j.physa.2012.01.004 doi: 10.1016/j.physa.2012.01.004
![]() |
[33] |
C. Gómez, R. Hornero, Entropy and complexity analyses in Alzheimer's disease: An MEG study, Open Biomed. Eng. J., 4 (2010), 223–235. https://doi.org/10.2174/1874120701004010223 doi: 10.2174/1874120701004010223
![]() |
[34] |
P. Maragos, F. K. Sun, Measuring the fractal dimension of signals: Morphological covers and iterative optimization, IEEE Trans. Signal Process., 41 (1993), 108. https://doi.org/10.1109/TSP.1993.193131 doi: 10.1109/TSP.1993.193131
![]() |
[35] |
L. S. Liebovitch, T. Toth, A fast algorithm to determine fractal dimensions by box counting, Phys. Lett. A, 141 (1989), 386–390. https://doi.org/10.1016/0375-9601(89)90854-2 doi: 10.1016/0375-9601(89)90854-2
![]() |
[36] |
B. Klinkenberg, A review of methods used to determine the fractal dimension of linear features, Math. Geol., 26 (1994), 23–46. https://doi.org/10.1007/BF02065874 doi: 10.1007/BF02065874
![]() |
[37] |
K. Suganthi, G. Jayalalitha, Geometric brownian motion in stock prices, J. Phys. Conf. Ser., 1377 (2019), 012016. https://doi.org/10.1088/1742-6596/1377/1/012016 doi: 10.1088/1742-6596/1377/1/012016
![]() |
[38] | R. M. Rangayyan, Biomedical Signal Analysis, John Wiley & Sons, 2015. https://doi.org/10.1002/9781119068129 |
[39] |
H. H. Giv, Directional short-time Fourier transform, J. Math. Anal. Appl., 399 (2013), 100–107. https://doi.org/10.1016/j.jmaa.2012.09.053 doi: 10.1016/j.jmaa.2012.09.053
![]() |
[40] |
R. G. Stockwell, L. Mansinha, R. P. Lowe, Localization of the complex spectrum: the S transform, IEEE Trans. Signal Process., 44 (1996), 998–1001. https://doi.org/10.1109/78.492555 doi: 10.1109/78.492555
![]() |
[41] |
V. Kumar, S. Minz, Feature selection: a literature review, Smart Comput. Rev., 4 (2014), 211–229. https://doi.org/10.6029/smartcr.2014.03.007 doi: 10.6029/smartcr.2014.03.007
![]() |
[42] | L. Haldurai, T. Madhubala, R. Rajalakshmi, A study on genetic algorithm and its applications, Int. J. Comput. Sci. Eng., 4 (2016), 139. |
[43] |
B. Xue, M. Zhang, W. N. Browne, Particle Swarm Optimization for feature selection in classification: A multi-objective approach, IEEE Trans. Cybern., 43 (2013), 1656–1671. https://doi.org/10.1109/TSMCB.2012.2227469 doi: 10.1109/TSMCB.2012.2227469
![]() |
[44] |
B. Chen, L. Chen, Y. Chen, Efficient ant colony optimization for image feature selection, Signal Process., 93 (2013), 1566–1576. https://doi.org/10.1016/j.sigpro.2012.10.022 doi: 10.1016/j.sigpro.2012.10.022
![]() |
[45] |
A. A. B. Pessa, R. S. Zola, M. Perc, H. V. Ribeiro, Determining liquid crystal properties with ordinal networks and machine learning, Chaos, Solitons Fractals, 154 (2022), 111607. https://doi.org/10.1016/j.chaos.2021.111607 doi: 10.1016/j.chaos.2021.111607
![]() |
1. | Naveed Ur Rehman Ahmed, Afzal Badshah, Hanan Adeel, Ayesha Tajammul, Ali Daud, Tariq Alsahfi, Visual Deepfake Detection: Review of Techniques, Tools, Limitations, and Future Prospects, 2025, 13, 2169-3536, 1923, 10.1109/ACCESS.2024.3523288 | |
2. | Irene Amerini, Mauro Barni, Sebastiano Battiato, Paolo Bestagini, Giulia Boato, Vittoria Bruni, Roberto Caldelli, Francesco De Natale, Rocco De Nicola, Luca Guarnera, Sara Mandelli, Taiba Majid, Gian Luca Marcialis, Marco Micheletto, Andrea Montibeller, Giulia Orrù, Alessandro Ortis, Pericle Perazzo, Giovanni Puglisi, Nischay Purnekar, Davide Salvi, Stefano Tubaro, Massimo Villari, Domenico Vitulano, Deepfake Media Forensics: Status and Future Challenges, 2025, 11, 2313-433X, 73, 10.3390/jimaging11030073 |
Model modules | Information |
No. of encoder blocks | 4 |
No. of attention heads | [1, 2, 5, 8] |
Patch sizes | [7, 3, 3, 3] |
Strides | [4, 2, 2, 2] |
Classifier hidden size | 256 |
Parameters | 3.3 million |
FLOPs | 8.4 G |
Dataset | Manipulation methods | Real/Fake |
FF++ [35] | DF, F2F, FS, NT | 1000/4000 |
DFD [40] | Improved DF | 363/3068 |
DF-256, MM/NN, NTH, | ||
DFDC [41] | Audio swaps, DF-128, FSGAN, | 23,654/104,500 |
StyleGAN, refinement | ||
CDF-V2 [42] | Enhanced FaceSwap DF | 590/5639 |
Deeper [43] | DF-VAE with seven perturbations | 1000/9000 |
Type | Methods | Trained on remaining three | Avg. | #params. | |||
DF | FS | F2F | NT | ||||
Frame-level | LRLNet [7] | 91.37 | 89.44 | 90.22 | 85.83 | 89.22 | 46.3 M |
PEL [8] | 89.78 | 87.67 | 88.91 | 87.78 | 88.54 | 33.7 M | |
Video-level | S-MIL [9] | 87.68 | 85.26 | 85.85 | 84.49 | 85.82 | 42.2 M |
STIL [10] | 90.71 | 88.73 | 91.73 | 88.78 | 89.99 | 32.5 M | |
LipForensics [15] | 97.83 | 90.51 | 98.02 | 96.90 | 95.82 | 36.0 M | |
FTCN [16] | 96.71 | 96.02 | 96.07 | 96.24 | 96.26 | 26.6 M | |
DIL [17] | 94.36 | 94.77 | 94.81 | 93.24 | 94.3 | 41.4 M | |
ECGL [18] | 92.49 | 90.96 | 89.59 | 89.19 | 90.56 | 37.3 M | |
SeqFakeFormer [19] | 96.82 | 95.08 | 95.75 | 95.27 | 95.73 | 104 M | |
Two-branch [27] | 89.81 | 87.28 | 90.06 | 86.92 | 88.52 | 67.1 M | |
MSVT [28] | 98.06 | 97.43 | 96.68 | 97.19 | 97.34 | 26.5 M | |
VDF [29] | 96.20 | 86.31 | 89.60 | 94.25 | 91.62 | 40 M | |
Audio-DF [30] | 97.31 | 94.74 | 94.50 | 95.10 | 95.41 | 89 M | |
TCAN (Ours) | 99.16 | 98.93 | 99.03 | 98.15 | 98.82 | 3.3 M |
Type | Methods | Trained on all four subsets | Avg. | #params. | |||
DFD | DFDC | CDF-V2 | Deeper | ||||
Frame-level | LRLNet [7] | 77.61 | 76.61 | 78.37 | 85.86 | 79.61 | 46.3 M |
PEL [8] | 89.29 | 65.87 | 70.29 | 70.41 | 73.97 | 33.7 M | |
Video-level | S-MIL [9] | 86.39 | 66.79 | 75.86 | 83.07 | 78.03 | 42.2 M |
STIL [10] | 87.21 | 67.22 | 76.17 | 83.94 | 78.64 | 32.5 M | |
LipForensics [15] | 84.25 | 73.62 | 83.60 | 97.00 | 84.62 | 36.0 M | |
FTCN [16] | 90.46 | 74.17 | 86.95 | 97.95 | 87.38 | 26.6 M | |
DIL [17] | 88.15 | 72.52 | 82.82 | 86.09 | 82.40 | 41.4 M | |
ECGL [18] | 90.18 | 73.09 | 85.86 | 96.62 | 86.94 | 37.3 M | |
SeqFakeFormer [19] | 90.22 | 74.32 | 84.26 | 95.96 | 86.19 | 104 M | |
Two-branch [27] | 86.19 | 70.18 | 75.79 | 81.34 | 78.38 | 67.1 M | |
MSVT [28] | 91.36 | 76.79 | 88.81 | 98.42 | 88.85 | 26.5 M | |
VDF [29] | 90.50 | 85.10 | 80.70 | 84.30 | 85.15 | 40 M | |
Audio-DF [30] | 91.12 | 82.31 | 86.20 | 96.50 | 89.03 | 89 M | |
TCAN (Ours) | 91.09 | 86.94 | 88.99 | 98.80 | 91.46 | 3.3 M |
Compression level | Trained on remaining three | Avg. | |||
DF | FS | F2F | NT | ||
c23 | 85.23 | 79.67 | 76.84 | 74.14 | 78.97 |
c40 | 70.21 | 73.16 | 70.59 | 69.28 | 70.81 |
Methods | Trained on remaining three | Trained on all four | Avg. | #params. | |||||
DF | FS | F2F | NT | DFDC | CDF-V2 | Deeper | |||
ViT [20] | 96.30 | 98.34 | 98.31 | 95.25 | 86.28 | 82.15 | 97.61 | 93.46 | 85.8 M |
RegNet [48] | 97.71 | 97.30 | 98.55 | 97.98 | 82.51 | 78.69 | 96.50 | 92.74 | 43.9 M |
SwinV2 [49] | 97.99 | 96.53 | 96.91 | 93.09 | 77.76 | 78.89 | 93.53 | 90.67 | 27.5 M |
BEiT [50] | 96.21 | 98.21 | 97.20 | 96.82 | 77.19 | 87.69 | 96.88 | 92.88 | 85.7 M |
PF [51] | 98.98 | 98.78 | 98.02 | 98.08 | 84.04 | 85.18 | 98.77 | 94.55 | 11.4 M |
TCAN (Our) | 99.16 | 98.93 | 99.03 | 98.15 | 86.94 | 88.99 | 98.80 | 95.71 | 3.3 M |
Model modules | Information |
No. of encoder blocks | 4 |
No. of attention heads | [1, 2, 5, 8] |
Patch sizes | [7, 3, 3, 3] |
Strides | [4, 2, 2, 2] |
Classifier hidden size | 256 |
Parameters | 3.3 million |
FLOPs | 8.4 G |
Dataset | Manipulation methods | Real/Fake |
FF++ [35] | DF, F2F, FS, NT | 1000/4000 |
DFD [40] | Improved DF | 363/3068 |
DF-256, MM/NN, NTH, | ||
DFDC [41] | Audio swaps, DF-128, FSGAN, | 23,654/104,500 |
StyleGAN, refinement | ||
CDF-V2 [42] | Enhanced FaceSwap DF | 590/5639 |
Deeper [43] | DF-VAE with seven perturbations | 1000/9000 |
Type | Methods | Trained on remaining three | Avg. | #params. | |||
DF | FS | F2F | NT | ||||
Frame-level | LRLNet [7] | 91.37 | 89.44 | 90.22 | 85.83 | 89.22 | 46.3 M |
PEL [8] | 89.78 | 87.67 | 88.91 | 87.78 | 88.54 | 33.7 M | |
Video-level | S-MIL [9] | 87.68 | 85.26 | 85.85 | 84.49 | 85.82 | 42.2 M |
STIL [10] | 90.71 | 88.73 | 91.73 | 88.78 | 89.99 | 32.5 M | |
LipForensics [15] | 97.83 | 90.51 | 98.02 | 96.90 | 95.82 | 36.0 M | |
FTCN [16] | 96.71 | 96.02 | 96.07 | 96.24 | 96.26 | 26.6 M | |
DIL [17] | 94.36 | 94.77 | 94.81 | 93.24 | 94.3 | 41.4 M | |
ECGL [18] | 92.49 | 90.96 | 89.59 | 89.19 | 90.56 | 37.3 M | |
SeqFakeFormer [19] | 96.82 | 95.08 | 95.75 | 95.27 | 95.73 | 104 M | |
Two-branch [27] | 89.81 | 87.28 | 90.06 | 86.92 | 88.52 | 67.1 M | |
MSVT [28] | 98.06 | 97.43 | 96.68 | 97.19 | 97.34 | 26.5 M | |
VDF [29] | 96.20 | 86.31 | 89.60 | 94.25 | 91.62 | 40 M | |
Audio-DF [30] | 97.31 | 94.74 | 94.50 | 95.10 | 95.41 | 89 M | |
TCAN (Ours) | 99.16 | 98.93 | 99.03 | 98.15 | 98.82 | 3.3 M |
Type | Methods | Trained on all four subsets | Avg. | #params. | |||
DFD | DFDC | CDF-V2 | Deeper | ||||
Frame-level | LRLNet [7] | 77.61 | 76.61 | 78.37 | 85.86 | 79.61 | 46.3 M |
PEL [8] | 89.29 | 65.87 | 70.29 | 70.41 | 73.97 | 33.7 M | |
Video-level | S-MIL [9] | 86.39 | 66.79 | 75.86 | 83.07 | 78.03 | 42.2 M |
STIL [10] | 87.21 | 67.22 | 76.17 | 83.94 | 78.64 | 32.5 M | |
LipForensics [15] | 84.25 | 73.62 | 83.60 | 97.00 | 84.62 | 36.0 M | |
FTCN [16] | 90.46 | 74.17 | 86.95 | 97.95 | 87.38 | 26.6 M | |
DIL [17] | 88.15 | 72.52 | 82.82 | 86.09 | 82.40 | 41.4 M | |
ECGL [18] | 90.18 | 73.09 | 85.86 | 96.62 | 86.94 | 37.3 M | |
SeqFakeFormer [19] | 90.22 | 74.32 | 84.26 | 95.96 | 86.19 | 104 M | |
Two-branch [27] | 86.19 | 70.18 | 75.79 | 81.34 | 78.38 | 67.1 M | |
MSVT [28] | 91.36 | 76.79 | 88.81 | 98.42 | 88.85 | 26.5 M | |
VDF [29] | 90.50 | 85.10 | 80.70 | 84.30 | 85.15 | 40 M | |
Audio-DF [30] | 91.12 | 82.31 | 86.20 | 96.50 | 89.03 | 89 M | |
TCAN (Ours) | 91.09 | 86.94 | 88.99 | 98.80 | 91.46 | 3.3 M |
Compression level | Trained on remaining three | Avg. | |||
DF | FS | F2F | NT | ||
c23 | 85.23 | 79.67 | 76.84 | 74.14 | 78.97 |
c40 | 70.21 | 73.16 | 70.59 | 69.28 | 70.81 |
Methods | Trained on remaining three | Trained on all four | Avg. | #params. | |||||
DF | FS | F2F | NT | DFDC | CDF-V2 | Deeper | |||
ViT [20] | 96.30 | 98.34 | 98.31 | 95.25 | 86.28 | 82.15 | 97.61 | 93.46 | 85.8 M |
RegNet [48] | 97.71 | 97.30 | 98.55 | 97.98 | 82.51 | 78.69 | 96.50 | 92.74 | 43.9 M |
SwinV2 [49] | 97.99 | 96.53 | 96.91 | 93.09 | 77.76 | 78.89 | 93.53 | 90.67 | 27.5 M |
BEiT [50] | 96.21 | 98.21 | 97.20 | 96.82 | 77.19 | 87.69 | 96.88 | 92.88 | 85.7 M |
PF [51] | 98.98 | 98.78 | 98.02 | 98.08 | 84.04 | 85.18 | 98.77 | 94.55 | 11.4 M |
TCAN (Our) | 99.16 | 98.93 | 99.03 | 98.15 | 86.94 | 88.99 | 98.80 | 95.71 | 3.3 M |