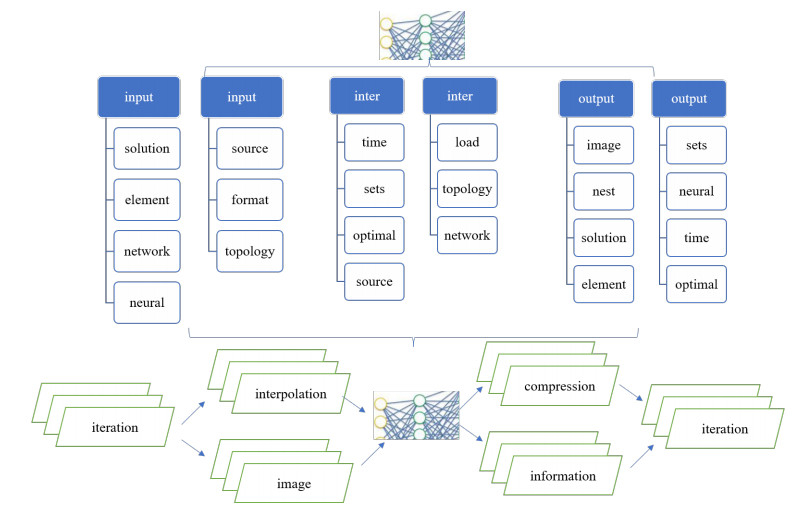
With increasingly mature commercial operations, football has become the most popular sport in the world. As the main body of football, athletes are prone to injury due to an increasing degree of competition intensity. Their health determines the length of these athletes careers, especially regarding the lower limbs that are mainly used. Therefore, the smart visualization approaches that can realize such function are in urgent demand in the area of sports healthcare. Benefitted by the strong ability of perception and analysis, a convolutional neural network (CNN) is utilized to construct an intelligent data analysis-based medical management method for the lower limb health of football athletes. First, the CNN is formulated as the main backbone, and its parameters are optimized for multiple rounds during the training stage. Then, a statistical analysis software named SPSS is introduced to assess the effect mechanism of different postures on lower limbs. Some experiments are carried out on simulative data to evaluate the proposed method, and results show a good performance of the proposed method.
Citation: Xiang Wang, Yongcheng Wang, Limin He. An intelligent data analysis-based medical management method for lower limb health of football athletes[J]. Mathematical Biosciences and Engineering, 2023, 20(8): 14005-14022. doi: 10.3934/mbe.2023624
[1] | Jiayu Fu, Haiyan Wang, Risu Na, A JISAIHAN, Zhixiong Wang, Yuko OHNO . Recent advancements in digital health management using multi-modal signal monitoring. Mathematical Biosciences and Engineering, 2023, 20(3): 5194-5222. doi: 10.3934/mbe.2023241 |
[2] | Bo An . Construction and application of Chinese breast cancer knowledge graph based on multi-source heterogeneous data. Mathematical Biosciences and Engineering, 2023, 20(4): 6776-6799. doi: 10.3934/mbe.2023292 |
[3] | Ivan Izonin, Nataliya Shakhovska . Special issue: Informatics & data-driven medicine. Mathematical Biosciences and Engineering, 2021, 18(5): 6430-6433. doi: 10.3934/mbe.2021319 |
[4] | Jiayu Fu, Haiyan Wang, Risu Na, A Jisaihan, Zhixiong Wang, Yuko Ohno . A novel "five-in-one" comprehensive medical care framework for rehabilitation and nursing. Mathematical Biosciences and Engineering, 2023, 20(3): 5004-5023. doi: 10.3934/mbe.2023232 |
[5] | Ivan Izonin, Nataliya Shakhovska . Special issue: informatics & data-driven medicine-2021. Mathematical Biosciences and Engineering, 2022, 19(10): 9769-9772. doi: 10.3934/mbe.2022454 |
[6] | Marco Roccetti . Predictive health intelligence: Potential, limitations and sense making. Mathematical Biosciences and Engineering, 2023, 20(6): 10459-10463. doi: 10.3934/mbe.2023460 |
[7] | Yinhua Su . Visualization design of health detection products based on human-computer interaction experience in intelligent decision support systems. Mathematical Biosciences and Engineering, 2023, 20(9): 16725-16743. doi: 10.3934/mbe.2023745 |
[8] | Delong Cui, Hong Huang, Zhiping Peng, Qirui Li, Jieguang He, Jinbo Qiu, Xinlong Luo, Jiangtao Ou, Chengyuan Fan . Next-generation 5G fusion-based intelligent health-monitoring platform for ethylene cracking furnace tube. Mathematical Biosciences and Engineering, 2022, 19(9): 9168-9199. doi: 10.3934/mbe.2022426 |
[9] | Tingting Yang, Yi He . Design of intelligent robots for tourism management service based on green computing. Mathematical Biosciences and Engineering, 2023, 20(3): 4798-4815. doi: 10.3934/mbe.2023222 |
[10] | Qinwang Niu, Haoyue Li, Yu Liu, Zhibo Qin, Li-bo Zhang, Junxin Chen, Zhihan Lyu . Toward the Internet of Medical Things: Architecture, trends and challenges. Mathematical Biosciences and Engineering, 2024, 21(1): 650-678. doi: 10.3934/mbe.2024028 |
With increasingly mature commercial operations, football has become the most popular sport in the world. As the main body of football, athletes are prone to injury due to an increasing degree of competition intensity. Their health determines the length of these athletes careers, especially regarding the lower limbs that are mainly used. Therefore, the smart visualization approaches that can realize such function are in urgent demand in the area of sports healthcare. Benefitted by the strong ability of perception and analysis, a convolutional neural network (CNN) is utilized to construct an intelligent data analysis-based medical management method for the lower limb health of football athletes. First, the CNN is formulated as the main backbone, and its parameters are optimized for multiple rounds during the training stage. Then, a statistical analysis software named SPSS is introduced to assess the effect mechanism of different postures on lower limbs. Some experiments are carried out on simulative data to evaluate the proposed method, and results show a good performance of the proposed method.
There are many factors that cause football player injuries, which can be roughly divided into internal and external factors [1]. Internal factors include the athlete's age, joint instability, muscle strength, muscle density, injury history, and psychological state, etc [2]. The asymmetry of the lower limbs on both sides of football players is also considered to be a factor leading to injury [3]. The asymmetry of the lower limbs can also be called laterality [4]. Football players usually choose one side as the kicking leg according to their own habits, mainly to complete actions such as shooting and dribbling the ball [5,6]. This difference in function may lead to asymmetry in strength and movement strategy [7,8,9] between the kicking leg and the supporting leg after prolonged training and competition [10].
At present, the measurement of muscle strength of lower limbs is generally carried out by an isokinetic test [11,12]. An isokinetic test can measure the joint muscle torque at different speeds, and the peak torque is often used to evaluate the asymmetry on both sides [13,14,15]. At present, neural network database systems can access existing data in the database, and the amount of information obtained from these data is only a part of the information contained in the entire database [16]. The traditional methods of analyzing and processing lateral shear dynamic data only analyze the local or surface characteristics of the data, and cannot obtain the description of the overall characteristics of these data hidden behind them nor predict their development trends [17,18]. This difference in function may lead to asymmetry in the strength of the muscles on both sides of the lower limbs [19]. Bigler [20] tested the lower limb muscle strength of football players with different training years and showed that the lower limb muscle strength of the athletes who had undergone 8–10 years of professional training reached a peak, and the athletes who had undergone short-term and medium-time training had asymmetrical muscle strength on both sides of the lower limbs. Montanino [21] conducted an isokinetic test on 41 outstanding football players on both sides of the knee flexor and extensor muscles, and the results showed that the knee flexor and extensor muscle strength of the kicking leg was lower than that of the support leg, which was due to the form of action on both sides during exercise, resulting in different training methods on both sides and subsequent asymmetry on both sides.
However, there are also studies that suggest that the strength of the lower body muscles on both sides of the kicking leg and the supporting leg is not completely asymmetric. Kaur [22] studied the flexion and extension of the left and right knee joints of amateur football players under different loads and showed that the strength of the flexion and extension muscles of the knee joints on both sides was asymmetrical only at 40–60% of the maximum load. The absence of bilateral asymmetry at maximum load is due to the fact that the maximum load occurs in very slow movements, which are rarely seen in football. Li [23] divided subjects into flanks according to the position of the court and it was found that the position on the court also affected the asymmetry of the lower body. The asymmetry of the left and right extensor muscles of the wing players is not obvious, which may be because the wing players need to do more sprinting movements in the game, and the left and right extensor muscles work in a more balanced fashion [24]. Compared with the wing players, the middle players need more take-offs in the game, and the take-off requires more work of the knee flexors on both sides, resulting in a smaller asymmetry of the knee flexors on both sides of the middle players [25,26,27]. Visual based recognition of football player's lateral shear behavior is the process of annotating, recognizing, and analyzing the technical actions of football players in a video; through neural network training, this can have good generalization. At present, a lot of research on behavior recognition has been done both domestically and internationally, and widely applied in athlete assisted training and operational processes.
In this paper, a dynamic analysis model of lateral shear direction was constructed, the isokinetic muscle torque was tested on the knee and ankle joints of the kicking leg and supporting leg of football players, and the peak torque of the knee and ankle flexor and extensor muscles of the kicking leg was compared with the knee and ankle of the supporting leg. To test whether there is asymmetry in the peak torque of the joint flexor and extensor groups, 14 semi-professional adult male soccer players were selected as subjects, and the subjects wore long spike soccer shoes (SG), short spike soccer shoes (AG), and broken spike soccer shoes (TF) under natural turf conditions for straight acceleration and 45∘ left sidestep cutting, with a control speed of 4.5±0.2 m/s. This chapter provides an introduction to some details of our experiment, such as the experimental environment and parameter settings. We conducted extensive experiments on the dataset to demonstrate the effectiveness of the methods we proposed in this chapter. Then, the athletes completed a kinematics and dynamics test of sidecut, sudden stop, and take-off for the following reasons: 1) to compare whether there were differences in the kinematics and dynamics of the lower limbs on both sides of the injury to understand whether the two sides of the kicking leg and the supporting leg cause asymmetry due to the difference in the main motor functions in sports; 2) to understand the impact of the asymmetry of the lower limbs on both sides of the sports injury and competition level; 3) to formulate effective preventive measures for football players' injuries caused by bilateral lower limb asymmetry; and 4) provide a theoretical basis for coaches and athletes to further understand bilateral asymmetry and improve their training methods.
In terms of the basic network structure, the basic network is built by dense convolutional neural network (CNN) layers based on different principles, which is used to extract features. The network proposed in this paper enables CNNs to extract richer feature information without increasing network parameters, thus improving the accuracy of the algorithm in classification and regression. The spatial transformation network layer is added in front of the basic network layer so that the target detection algorithm can be used in the lateral shear dynamics analysis task of football players. In this paper, the characteristics proposed by the basic network are used to predict the rectangular box target. This paper mainly solves the special limitation of rotation tilt in the side shear dynamics of football players in real scenarios.
Each neuron that constitutes a CNN may be bidirectionally connected to each other, and all neurons are used both as an input and output. This network handles information differently than a forward network. When they perform a convolution, unlike normal convolutions, it is a graph convolution. Different from an ordinary convolution operation, although the parameters of the convolution operation are fixed in the graph, the adjacent key points of each key point are not. In the feedforward network, the information processing is from the input layer through the intermediate layer (hidden layer) to the output, and the processing ends. In this kind of network f(x), if an input is applied outside the bran neural network at a certain time, each neuron interacts with each other and the information processing r(asis) until the output values of all the meridians in the network c(x,y) converge to a certain value exp(x−y). The processing of the information is not finished until the average falls.
f(x)=r( asis )−mid( asis )∣r( asis )−mid( asis )−x∣ | (2.1) |
c(x,y)=1−exp(x−y)1+exp(x−y) | (2.2) |
It can make the score segments corresponding to the scores of x (sparse distribution of scores) and y (such as javelin, dense distribution of scores), and then use the scores of these items as the input vector of the CNN and the corresponding score as the output vector. Among them, a represents the sequence of continuous video image frames, b represents the optical flow extracted by the continuous video frames in the direction of axis x, and c represents the optical flow extracted by continuous video frames in the direction of axis y. Once the model is trained and learned, it can be used to generate a scoring table that changes with the times and the development of science and technology, and has generalization. Because the parameters u(k) required in the neural network are obtained from training w(k) and learning, it is more objective and reasonable than the production of the original score table, and can achieve better accuracy max[k].
u(k)=μ(k)−θ(k), if {μ(k)>θ(k)} | (2.3) |
w(k)∈max[θ(k)−n+1,θ(k)] | (2.4) |
The classification task based on neural network mainly includes two parts: one is the function to calculate the classification score and the other is the loss function. The main operation of calculating the classification score function is to map the data obtained from the fully connected layer into the category score through a linear classifier. The usual methods to assess the asymmetry of muscle strength on both sides of the lower extremities include the isokinetic test and the jump test. Regardless of the form of the mapping between the evaluation indicators and the evaluation results, the neural network can automatically learn any previous experience from the provided training samples and simulate it through training, thereby replacing the cumbersome query and presentation process by the review experts. For those cases where the influencing factors are more complex and the degree 1−e(x) of nonlinearity is higher, the advantage of the neural network is more obvious, because the neural network u(x,y) has the characteristics of high adaptability, self-organization, self-learning, etc.
u(x,y)=1−e(x)e(x)−1−e(y)e(y) | (2.5) |
A(i,j)=t∑i=1w(i,j)∗v(i,j)+b | (2.6) |
In the forward propagation A(i,j), if the network output w(i,j) at the output layer differs greatly from the expected output v(i,j), the backpropagation process is started, and each connection weight between network nodes is modified according to the signal error between the network output and the expected output. In this way, the error u(t) between the actual output of the network and the expected output ku(t) is reduced.
f(u+kt)=ku(t)−aku(t−1)−b | (2.7) |
v(x,y)=12v(sa−x)v(x)−13mid(sa−y)mid(y) | (2.8) |
An element is randomly selected from each set v(x,y) according to the information state mid(sa−y) of each element in the set mid(y), and the pheromone corresponding to the selected element is adjusted. The selection of elements is completed in all sets, that is, a set of neural network weights is selected in all sets, even if the source is reached, the nest is returned to the nest along the path just traveled, and the corresponding selected elements are adjusted at the same time. After the continuous iteration of Figure 1, the application of neural networks in exercise load prediction can finally find the optimal solution of the parameters. The optical flow here only calculates the difference between successive video frames and does not filter by threshold. The filtering operation in the experiment in this paper will be carried out during the training of the model, and the filtered results will be mapped to the range of [0,255].
If the compression factor is 8, there is no obvious relationship between the influence of the image compression operation on the estimated skeleton point coordinates. The conclusion when the compression factor is 8 is inconsistent with 2 and 4, mainly because the core principle of image compression is a bilinear interpolation. When the compression factor is 2, 4, and 8, the side-length ratios in the compression process are 2, 4, and 8, respectively. When the side-length ratio is 8, it means that each pixel after the image compression operation is is the sum of the weights of the 4 pixels closest to the coordinates on the source image. Therefore, compared with the compression factors 2 and 4, the compression factor 8 will lose more image features during the compression process, which cannot occur in the image compression operation.
We have to adapt the weighted mix of the convolution of the channel features and establish the dependency between the features, which inevitably involves mixing operations. Our basic idea is to use a gate like mechanism to restrict the feature information from different branches and different convolution kernel sizes to the next layer of the network.To achieve this goal, the gate mechanism needs to aggregate the characteristic information of all branches. We demonstrate this with a simple two-branch case, using element-by-element addition for feature aggregation.
The muscle joint angle is defined as the Euler angle rotated between the coordinate system of the distal link and the coordinate system of the proximal link, that is, the rotation of the distal link relative to the proximal link. The ankle joint angle is obtained by rotating the foot coordinate system relative to the calf coordinate system, the knee joint angle is obtained by rotating the calf coordinate system relative to the thigh coordinate system, and the hip joint angle is obtained by rotating the thigh coordinate system relative to the pelvis coordinate system. The right hip joint angle is defined as the first rotation around the x-axis to obtain the flexion and extension angle (negative angle is flexion) and the second rotation around the y-axis to obtain the abduction angles (positive angle is adduction, negative angle is abduction); you can then rotate around the z-axis for the third time and obtain the internal and external rotation angle (positive angle is external rotation, negative angle is internal rotation). The right knee and ankle joint angles are defined as the first rotation around the x-axis, obtaining extension angle (positive angle is flexion) and the second rotation around the y-axis to obtain adduction and abduction angles (positive angle is adduction, negative angle is abduction). The joint angle is defined as the first rotation around the x-axis to obtain the flexion and extension angle (positive angle is flexion) and the second rotation around the y-axis to obtain the abduction angles (positive angle is abduction, negative angle is adduction). you can then rotate around the z-axis for the third time and obtain the internal and external rotation angle (positive angle is internal rotation, negative angle is external rotation).
According to the grouping situation in Table 1, the three groups of data on the vigilance network efficiency were compared in pairs, and the method of variance analysis was used to check the significance. For a three-dimensional convolution, if the receptive field in the time dimension is smaller than that in the space dimension, the behavior information may not be captured; otherwise, the edge information of different objects will coincide. The average response time of the alertness network in the professional group was 41.95 ms, with a standard deviation of 26.568 ms; the average response time of the alertness network in the school team was 48.25 ms, with a standard deviation of 25.729 ms; the average response time of the alertness network in the general group was 61.75 ms, with a standard deviation of 37.230 ms. If the compression factor is 2 or 4, the effect of the image compression operation on the estimated skeleton point coordinates is linear. In the model estimation function of each video data, the error between the estimated and the compression coefficient is below 0.5, and the error between the correction value b and the offset a is about 1. For the data distribution in Figure 2, the representative value x of the compressed human pose coordinate set and the representative value y of the human pose coordinate set of the original image are distributed around the estimation function.
Marker point name | Sign point symbol | index Location description |
Head forward | 0.74349 | The highest point of the human body |
Back of head | 0.02226 | Human body upright midpoint |
Front arm | 0.59422 | Mid point of arm |
Back of arm | 0.37465 | Arm golden ratio |
Front thigh | 0.38224 | Mid-thigh point |
Back of thigh | 0.12831 | Thigh golden ratio |
A one-way analysis of variance (ANOVA) was performed to test the significance between the three groups. The test obtained F=5.300, p=0.008, and the difference was significant, indicating that the efficiency of the executive control network of the professional group, the school team group, and the ordinary group was significant. In a further multiple comparison analysis, the results found that the executive control network efficiency between the professional group and the school team group was 46.45±36.046, 64.80±56.357, p=0.225. The executive control network efficiency between the two groups was 46.45±36.046, 94.70±47.327, p=0.002, with very significant difference; the executive control network efficiency between the school team group and the ordinary group was 64.80±56.357, 94.70±47.327, p=0.05.
The dynamic analysis system will provide two forms of knowledge expression: chart form and conditional expression form. Experimental personnel can intuitively see the association relationships and credibility of the rules among the shear test items on each side of the rules. The lateral cut and the jump-off and landing process were divided into the buffer stage and the extension stage according to the flexion angle of the knee joint. The buffer stage refers to the moment from when the foot touches the ground to the moment when the knee joint flexion angle z(a,b) is at the maximum. The moment of touchdown is defined as the moment asis(bra−a) when the vertical ground reaction force is greater than 10N, and the moment when it is less than 10N is the moment of lifting off the ground.
z(a,b)=0.21 asis ( bra-a )+0.33 asis ( bra-b) | (2.9) |
theta (x,y)=cos−1[x∗y|x|∗|y|] | (2.10) |
In order to establish the dependency relationship of channel features, we carry out two full-join operations after global mean pooling to increase the nonlinear relationship of features. Our operations adhere to two guidelines: 1) they must be flexible and be able to experiment with different situations (because we only use two branches here); and 2) it should not interfere with the original dependence and spatial relationship between channel features. To satisfy the two above requirements, we use the sigmoid function as the activation function. The activation function theta(x,y) can simulate the frequency cos(x,y) and amplitude of the signal by adjusting the parameters, and at the same time, it can simulate the state that multiple signals work together, making the activation function more suitable for the structure of the human brain. The step size is the super parameter - learning rate in deep learning. The main purpose of setting the learning rate is to get the minimum value of the gradient, which is the optimal solution in the function. The results show that the activation function is not prone to problems such as gradient disappearance, and it can also simulate the signals j(a,b) with different frequency k(a,b) and amplitude i(a,b) and their effect on the neural network.
j(a,b)=k(a,b)⊗i(a,b)(11) | (2.11) |
∇stage(w,t)−∇stage(t)1−∇stage(w,t)−∇stage(t)<1 | (2.12) |
The input t of the model is the representative value stage(t) of the human body pose coordinate set after the compression operation, and the output w(t) is the representative value of the human body pose coordinate set before the compression operation. The soft saturation of the activation function means that it is derivable in the definition domain loss(k), and when the input signal tends to infinity or infinitely small, the slope w(k)/w(t) of the activation function will approach 0, and the problem of gradient disappearance will occur.
loss(k)=1/(∑Δk−Δt+∑Δk+Δt) | (2.13) |
miu(k,i,j)=1−k/(∑w(k)/w(i)+∑w(k)/w(j)) | (2.14) |
Compared with a single variable frequency sine function, the superposition of two sine functions with different frequencies and phases makes the whole function closer to the biological mechanism of brain waves. The new activation function miu(k,i,j) is monotonic as a whole, but non-monotonic locally.
The model in Figure 3 is trained using a stochastic gradient descent. After training, the model will display the data distribution based on the input data and estimate the possible linear relationship between these data. The representative value distribution and model estimation function of the video data before and after the image compression operation are as described in the text, where the x-axis is the representative value of the compressed human pose coordinate set, the y-axis is the representative value of the human pose coordinate set of the original image, and the compression coefficient is 2.
Isokinetic muscle torque test results are as in the text, where there is no significant difference in the peak torque of the kicking leg and the supporting leg knee flexor and extensor at the coordinate rotation speed of the different movement stages. When the test speed was 60o/s, the flexor symmetry index was −0.8 and the extensor symmetry index was −1.6, while when the test speed was 180o/s, the flexor symmetry index was 1.8% and the extensor symmetry index was −1.3. According to the grouping situation, the three groups of data of execution control network efficiency are compared in pairs, and the one-way ANOVA method is used to check the significance. Before pairwise comparisons, the mean reaction times and standard deviations of the executive control networks for the three groups were calculated. The average response time of the executive control network in the professional group was 46.45 ms, with a standard deviation of 36.046 ms; the average response time of the school team group's executive control network was 64.80 ms, with a standard deviation of 56.357 ms; the average response time of the general group's executive control network was 94.70 ms, with a standard deviation is 47.327 ms.
There is a single item in Figure 4 that reflects the superiority of the neural network to formulate the score; however, the results of the pass item in the exponential fitting score and the neural network score are almost the same. The results of multiple comparison analyses found that the alertness network efficiency between the professional group and the school team group was 41.95±26.568, 48.25±25.729, p=0.834. The alertness network efficiency between the professional group and the general group was 41.95±26.568, 61.75±37.230, p=0.172, which was not statistically significant; the alertness network efficiency between the school team and the general group was 48.25±25.729, 61.75±37.230, p=0.471, and also not statistically significant. The exponential fit scale only considers the progressiveness, and the scales developed with the neural network model comprehensively consider various factors, including progressiveness and equilibrium. If the learning rate is set too large, it may lead to a failure local minimum value, but if the setting is small, it may cause the model convergence is slow, which will consume more time to train the model. Taking the future development of science into account, the scoring table needs to be constantly revised. The scoring model developed by the neural network model is used to establish a scoring model. It can be seen that the developed scoring table can be used for future training.
The experimental data of kinetic indicators were statistically analyzed using Microsoft Excel 2018 and the SPSS 19 software, and the statistical results were expressed in the form of mean standard deviation. The right leg support at the beginning of the side cut is the foot contact period (0–10%) and the end of the support is the foot off the ground period. A grip coefficient value of (90–100%) is unstable, so this study selects the period of 10 to 90% of the support period when the grip coefficient is relatively stable to calculate the average grip coefficient value for comparison. The results of the lower limb joint angles at the first peak of the ground horizontal backward reaction force between the kicking leg and the supporting leg are shown. The hip flexion angle of the kicking leg is smaller than that of the supporting leg in the lateral cut (p=0.002), while other joint angles have no significant difference. There is a significant difference in the internal and external rotation angles of the kicking leg and the supporting leg in the sudden stop and take-off (p=0.027). There were no significant differences in other joint angles.
Table 2 shows that if the balance of the scores of each project is to be guaranteed, it should be ensured that the corresponding scores of the starting and ending points of each project should be the lowest and highest scores in the entire table; that is, when each project is of equal difficulty, absolute equality cannot be guaranteed because the decision of the starting and ending points is still determined by the opinions of experts and the average sports performance of the current top international athletes, so this equality can only be relative. The algorithm implementation process is as follows. First, generate a candidate project set C, scan the database for all transactions, calculate the support of these candidate sub project sets, and generate sub frequent sets L. At the same time, a hash table H for fast statistics is established to construct the candidate itemset (H is defined as the first hash table for programming convenience), and each read row of transactions is combined and decomposed based on the length of the candidate itemset to be constructed. It should not be the middle score segment on the grading table. Therefore, based on this, the starting and ending points of each item are limited to the lowest and highest scores of the entire score. The usual methods to assess the asymmetry of muscle strength on both sides of the lower extremities include the isokinetic test and the jump test. Research on lower limb asymmetry exists in many sports, especially the functional asymmetry of lower limb is a potential risk factor for athlete injury in youth training.
Image node | Number to be measured | Sampling ratio | Mean square error |
Test 1 | 0.65671 | 0.44514 | 0.44005 |
Test 2 | 0.03253 | 0.48486 | 0.37435 |
Test 3 | 0.7749 | 0.42601 | 0.96316 |
Test 4 | 0.99037 | 0.3317 | 0.54197 |
Test 5 | 0.1638 | 0.02419 | 0.50538 |
Test 6 | 0.44968 | 0.37192 | 0.10029 |
Test 7 | 0.87167 | 0.04717 | 0.60792 |
According to the grouping situation in Figure 5, the three groups of data of the directional network efficiency are compared in pairs, and the method of variance analysis of factors is used to check the significance. Before pairwise comparisons, mean reaction times and standard deviations were calculated for the three groups of directional networks. The average response time of the directional network in the professional group was 55.15 ms, with a standard deviation of 41.923 ms; the average response time of the directional network in the school team was 74.30 ms, with a standard deviation of 51.087 ms; the average response time of the directional network in the general group was 86.50 ms, with a standard deviation of 49.653 ms. Non-contact knee injury in football is often caused by improper movement design, which leads to excessive abduction and external rotation of the knee joint in the side cut, quick stop and turn and so on. In this paper, football players were tested on side incision and sharp turn. Therefore, this system proposes a new deep neural network model for estimating the two-dimensional coordinates of the human skeleton points of football players in a single frame image, referring to the corrected human posture model. During training, the model monitors the loss function of each stage to reduce the overall loss during training.
This research takes football players as research samples. These include national Division I and Division II athletes. In order to ensure the accuracy and scientificity of the experimental results, when selecting samples, this paper specially communicated with coaches and other relevant personnel one-on-one to conduct detailed exploration and record of the physical conditions of the experimental samples.
There is no significant difference in the peak torque of the ankle flexor of the kicking leg and the supporting leg at different speeds, while the peak torque of the ankle extensor of the kicking leg is at the test speed. At a test speed of 60 o/s, the ankle flexor symmetry index was 0.3% and the extensor symmetry index was 18%. At a test speed of 180o/s, the ankle flexor symmetry index was 0.4% and the extensor symmetry index was 13.6%. This paper utilizes Matlab6.5 and Delphi7.0, which can easily complete the interaction between Matlab and the external environment, that is, when Delphi is used as a front-end development tool for application development maps, combined with the neural network toolbox in Matlab, the complex program is integrated. During the modeling process in MATLAB, by mining and analyzing the physical fitness status of football players, we found that in addition to the network model, the number of hidden nodes and the number of training samples can affect the performance of the CNN model, modifying parameters such as the learning step size and number of learning times for weights and thresholds also affects the learning algorithm to a certain extent.
In the training process of the optimized model in Figure 6, the lateral shear direction dataset is first used for training, and further training is performed with the collected football player moving images. Training with the lateral shear direction data set can ensure that the overall estimation of the optimization model is accurate. On the basis of the lateral shear direction data set training, it can use the collected football player moving images to train again to ensure that the optimization model can accurately estimate the football player's body posture. The results of the multiple comparison analysis of the alertness network test showed that the alertness network efficiency between the professional group and the school team group was 41.95±26.568, 48.25±25.729, p=0.834. The vigilance network efficiency was 41.95±26.568, 61.75±37.230, p=0.172, and the vigilance network efficiency between the school team group and the normal group was 48.25±25.729, 61.75±37.230, p=0.4715, which was not statistically significant. There was no substantial difference in motor type, which may be the reason why there were no significant differences in the results of the vigilance network test among the three groups.
In this paper, bypass and gate units are introduced. The two-branch architecture reduces the difficulty of training hundred-layer networks. This idea is also used in ResNet, except that in ResNet the bypass path is simply an identity map. GoogleNet carefully configured each branch using custom kernel filters to aggregate more information and diverse characteristics. In comparison, our SKENets follows the GoogleNets idea of using multiple convolution cores on multiple branches, but our SKENets is more concise and does not have redundant custom structures. We use a Resnet-like topology that has been proven to improve model accuracy while reducing parameters.
The content of the dynamic simulation is to select the video collected from the left side of the net and the right side of the net, estimate the coordinates of the human body posture skeleton point in each frame of the video through the basic model, and record the time when the (0, 0) point appears in each frame. The video collected from the left side of the net (video A) has a total of 365 frames, and the video collected from the right side of the net (video B) has a total of 468 frames. The experimental strategy is to use the estimation model to estimate the human pose coordinate sets before and after compression, and use the representative values to reflect the estimated skeletal point coordinate changes before and after the compression operation. The test indicators include kinematic indicators and kinetic indicators. The kinematic test indicators include the following: contact time, three-dimensional range of motion (ROM) of hip, knee, and ankle joints, and three-dimensional peak of joint angle (PA). The specific indicators are the peak flexion angle and flexion range of motion of the hip joint, the peak abduction angle and abduction range of the hip joint, the peak internal rotation angle and internal rotation range of motion of the hip joint. The experiment of Figure 7 is expected to obtain the relationship with the compression factor by comparing the change of the representative value before and after the compression operation.
The frame rate itself does not affect the image quality, and it mainly determines the fineness of the athlete's pose extraction. The higher the frame rate of the camera, the more images of the athlete's posture are collected per second, so that the posture changes of the athlete's posture during vigorous exercise will not be missed, that is, there will be no motion blur in the collected images. For example, in the jump ball, athletes usually complete the action of jumping the ball at the highest point in the 3 to 4 frames of images. Therefore, during the continuous sampling process, if the frame rate of the camera is too low, there may be motions of human limbs in the collected images. The human body pose is evaluated from the overall point of view, and the average value of the summation is used to ensure that each skeleton point has the same weight in the human body pose representative value of the frame. By setting the priority of the human body posture skeleton points, the evaluation is performed according to the priority, which can amplify the role of the skeleton points with high priority in the human body posture evaluation. The simulation gives the biochemical results, where the ankle extensor peak torque displays significant asymmetry on both sides of the football player's kicking leg and the supporting leg and the kicking leg is higher than the supporting leg. The asymmetry of muscle strength between the kicking leg and the supporting leg is not obvious.
In the isokinetic test of lower limb muscle strength, there was no significant difference in the peak torque of the knee flexors and extensors of the kicking leg and the supporting leg at both 60o/s and 180o/s respectively, and the peak torque of the knee flexors and extensors were not significantly different. The ratio was not significantly different; the peak ankle plantar flexor torque was not significantly different at both 60o/s and 180o/s, while the ankle extensor peak torque was significantly higher than the supporting leg. In the kinematics and dynamics tests, there was no significant difference in the peak ground reaction force between the kicking leg and the supporting leg at the first peak moment of the ground horizontal backward and vertical reaction forces; the hip flexion angle of the kicking leg in the lateral cut was significantly smaller than that of the supporting leg, the ankle plantar flexion angle is significantly greater than that of the supporting leg. During the emergency stop and take-off, the hip of the kicking leg is externally rotated while the supporting leg is internally rotated; in the lateral cut, the ankle of the kicking leg presents an external rotation moment while the supporting leg presents an internal rotation moment. During the stop-and-go, the knee and ankle joints of the kicking leg show external rotation torque, while the knee and ankle joints of the supporting leg show internal rotation torque. Experiments have shown that our scheme can better learn the features of the middle layer and the relationships between features, especially when the number of sample frames is small. It not only outperforms the baseline method, but also improves more significantly than when the number of sample frames is large.
Figure 8 uses a comparison of multiple score segments and using a comparison of representative items on the existing scale: slower-growing items and faster-growing items. It can be seen that in the existing scoring table, the score increases rapidly with the performance, such as shooting attitude, etc, and the items whose score is almost linearly related to the performance are the passing attitude and so on. In this process, the conversion from the camera coordinate system to the image pixel coordinate system only uses the camera's internal parameter matrix and distortion correction, so the change of the human posture in the camera coordinate system is the same as that in the image pixel coordinate system. In the camera coordinate system, if the human body makes the same pose but the distance from the camera lens is different, the pose will show two poses in the camera coordinate system. Among them, the growth rate of points is equal to the difference of points/the difference of athletic performance. The practical application proves that the CNN model constructed is used to predict and analyze the exercise load of football players by comprehensively analyzing the athletes' physiology, biochemistry and related indicators.
Based on the CNN theory, this paper constructs a dynamic analysis model of the lateral shear direction. The research and analysis show that the kinematic results at the first peak of the vertical reaction force on the ground are only different on the two sides during the lateral cut, and the hip joint of the kicking leg is flexed during the lateral cut. The larger plantar flexion angle of the ankle of the kicking leg indicates that the kicking leg is more inclined to land on the front foot when doing a sidecut, so a smaller hip flexion angle is required. The greater plantar flexion of the ankle indicates that the kicking-leg ankle has a longer time to absorb the impact of the vertical reaction force on the ground. At the same time, the higher grip coefficient of SG during the side cutting action can also provide athletes with better sports performance, while the excessively high grip coefficient between the shoes and the ground can easily lead to the fixation effect of the foot and the ground, thereby increasing the soft tissue of the lower limbs and knees. In the isokinetic muscle torque test, only two test speeds are selected, which cannot fully reflect the maximum muscle strength of the lower limbs at different speeds. It is suggested that future research can use the isokinetic test and the lower limb jump function.
The authors declare they have not used Artificial Intelligence (AI) tools in the creation of this article.
The authors declare there is no conflict of interest.
[1] | Z. Guo, K. Yu, A. Jolfaei, G. Li, F. Ding, A. Beheshti, Mixed graph neural network-based fake news detection for sustainable vehicular social networks, IEEE Trans. Intell. Trans. Syst., 2022 (2022), forthcoming. https://doi.org/10.1109/TITS.2022.3185013 |
[2] |
Q. Li, L. Liu, Z. Guo, P. Vijayakumar, F. Taghizadeh-Hesary, K. Yu, Smart assessment and forecasting framework for healthy development index in urban cities, Cities, 131 (2022), 103971. https://doi.org/10.1016/j.cities.2022.103971 doi: 10.1016/j.cities.2022.103971
![]() |
[3] |
Z. Guo, K. Yu, N. Kumar, W. Wei, S. Mumtaz, M. Guizani, Deep distributed learning-based poi recommendation under mobile edge networks, IEEE Int. Things J., 10 (2023), 303–317. https://doi.org/10.1109/JIOT.2022.3202628 doi: 10.1109/JIOT.2022.3202628
![]() |
[4] |
L. Zhao, Z. Yin, K. Yu, X. Tang, L. Xu, Z. Guo, et al., A fuzzy logic based intelligent multi-attribute routing scheme for two-layered sdvns, IEEE Trans. Network Ser. Manage., 19 (2022), 4189–4200. https://doi.org/10.1109/TNSM.2022.3202741 doi: 10.1109/TNSM.2022.3202741
![]() |
[5] |
C. Chen, Z. Liao, Y. Ju, C. He, K. Yu, S. Wan, Hierarchical domain-based multi-controller deployment strategy in sdn-enabled space-air-ground integrated network, IEEE Trans. Aerosp. Electron. Syst., 58 (2022), 4864–4879. https://doi.org/10.1109/TAES.2022.3199191 doi: 10.1109/TAES.2022.3199191
![]() |
[6] | L. Yang, Y. Li, S. X. Yang, Y. Lu, T. Guo, K. Yu, Generative adversarial learning for intelligent trust management in 6g wireless networks, IEEE Network, 36 (2022), 134–140. https://doi.org/10.1109/MNET.003.2100672 |
[7] | Q. Zhang, Z. Guo, Y. Zhu, P. Vijayakumar, A. Castiglione, B. B. Gupta, A deep learning-based fast fake news detection model for cyber-physical social services, Pattern Recognit. Lett., 168 (2023), 31–38. |
[8] | S. J. Raymond, N. J. Cecchi, H. V. Alizadeh, A. A. Callan, E. Rice, Y. Liu, et al., Physics-informed machine learning improves detection of head impacts, Ann. Biomed. Eng., 2022 (2022), 1–12. |
[9] | Z. Guo, K. Yu, Z. Lv, K. K. R. Choo, P. Shi, J. J. P. C. Rodrigues, Deep federated learning enhanced secure poi microservices for cyber-physical systems, IEEE Wireless Commun., 29 (2022), 22–29. |
[10] | Y. Li, H. Ma, L. Wang, S. Mao, G. Wang, Optimized content caching and user association for edge computing in densely deployed heterogeneous networks, IEEE Trans. Mobile Comput., 21 (2022), 2130–2142, 2022. |
[11] | Z. Guo, Y. Shen, S. Wan, W. Shang, K. Yu, Hybrid intelligence-driven medical image recognition for remote patient diagnosis in internet of medical things, IEEE J. Biomed. Health Inf., 26 (2022), 5817–5828. |
[12] | Z. Zhou, Y. Li, J. Li, K. Yu, G. Kou, M. Wang, et al., Gan-siamese network for cross-domain vehicle re-identification in intelligent transport systems, IEEE Trans. Network Sci. Eng., 2022 2022, forthcoming. |
[13] |
S. Xia, Z. Yao, G. Wu, Y. Li, Distributed offloading for cooperative intelligent transportation under heterogeneous networks, IEEE Trans. Intell. Transp. Syst., 23 (2022), 16701–16714. https://doi.org/10.1109/TITS.2022.3190280 doi: 10.1109/TITS.2022.3190280
![]() |
[14] | G. Tierney, Concussion biomechanics, head acceleration exposure and brain injury criteria in sport: a review, Sports Biomechan., 2022 (2022), 1–29. |
[15] | L. Zhao, Z. Bi, A. Hawbani, K. Yu, Y. Zhang, M. Guizani, Elite: An intelligent digital twin-based hierarchical routing scheme for softwarized vehicular networks, IEEE Trans. Mobile Comput., 2022 (2022). |
[16] | Z. Zhou, Y. Su, J. Li, K. Yu, Q. M. J. Wu, Z. Fu, et al., Secret-to-image reversible transformation for generative steganography, IEEE Trans. Dependable Secure Comput., 2022 (2022), forthcoming. |
[17] | J. Zhang, Q. Yan, X. Zhu, K. Yu, Smart industrial iot empowered crowd sensing for safety monitoring in coal mine, Dig. Commun. Networks, 2022 (2022). |
[18] | J. Yang, F. Lin, C. Chakraborty, K. Yu, Z. Guo, A.-T. Nguyen, et al., A parallel intelligence-driven resource scheduling scheme for digital twins-based intelligent vehicular systems, IEEE Trans. Intell. Veh., 2023 (2023). |
[19] |
Y. Lu, L. Yang, S. X. Yang, Q. Hua, A. K. Sangaiah, T. Guo, et al., An intelligent deterministic scheduling method for ultra-low latency communication in edge enabled industrial internet of things, IEEE Trans. Ind. Inf., 19 (2023), 1756–1767. https://doi.org/10.1109/TII.2022.3186891 doi: 10.1109/TII.2022.3186891
![]() |
[20] | E. D. Bigler, Volumetric mri findings in mild traumatic brain injury (mtbi) and neuropsychological outcome, Neuropsychol. Rev., 2021 (2021), 1–37. |
[21] | A. Montanino, X. Li, Z. Zhou, M. Zeineh, D. Camarillo, S. Kleiven, Subject-specific multiscale analysis of concussion: from macroscopic loads to molecular-level damage, Brain Multiphys., 2 (2021), 100027. |
[22] | P. Kaur, S. Harnal, V. Gautam, M. P. Singh, S. P. Singh, A novel transfer deep learning method for detection and classification of plant leaf disease, J. Ambient Intell. Humanized Comput., 2022 (2022), 1–18. |
[23] | H. Li, R. Hu, Analysis of athletes' training characteristics based on action statistics of image processing, Wireless Commun. Mobile Comput., 2022 (2022), forthcoming. |
[24] |
T. Wu, M. Hajiaghamemar, J. S. Giudice, A. Alshareef, S. S. Margulies, M. B. Panzer, Evaluation of tissue-level brain injury metrics using species-specific simulations, J. Neurotrauma, 38 (2021), 1879–1888. https://doi.org/10.1089/neu.2020.7445 doi: 10.1089/neu.2020.7445
![]() |
[25] |
B. B. Tripathi, S. Chandrasekaran, G. F. Pinton, Super-resolved shear shock focusing in the human head, Brain Multiphys., 2 (2021), 100033. https://doi.org/10.1016/j.brain.2021.100033 doi: 10.1016/j.brain.2021.100033
![]() |
[26] | G. Weir, Anterior cruciate ligament injury prevention in sport: biomechanically informed approaches, Sports Biomechan., 2022 (2022), 1–21. |
[27] |
W. Zhao, S. Ji, White matter anisotropy for impact simulation and response sampling in traumatic brain injury, J. Neurotrauma, 36 (2019), 250–263. https://doi.org/10.1089/neu.2018.5634 doi: 10.1089/neu.2018.5634
![]() |
Marker point name | Sign point symbol | index Location description |
Head forward | 0.74349 | The highest point of the human body |
Back of head | 0.02226 | Human body upright midpoint |
Front arm | 0.59422 | Mid point of arm |
Back of arm | 0.37465 | Arm golden ratio |
Front thigh | 0.38224 | Mid-thigh point |
Back of thigh | 0.12831 | Thigh golden ratio |
Image node | Number to be measured | Sampling ratio | Mean square error |
Test 1 | 0.65671 | 0.44514 | 0.44005 |
Test 2 | 0.03253 | 0.48486 | 0.37435 |
Test 3 | 0.7749 | 0.42601 | 0.96316 |
Test 4 | 0.99037 | 0.3317 | 0.54197 |
Test 5 | 0.1638 | 0.02419 | 0.50538 |
Test 6 | 0.44968 | 0.37192 | 0.10029 |
Test 7 | 0.87167 | 0.04717 | 0.60792 |
Marker point name | Sign point symbol | index Location description |
Head forward | 0.74349 | The highest point of the human body |
Back of head | 0.02226 | Human body upright midpoint |
Front arm | 0.59422 | Mid point of arm |
Back of arm | 0.37465 | Arm golden ratio |
Front thigh | 0.38224 | Mid-thigh point |
Back of thigh | 0.12831 | Thigh golden ratio |
Image node | Number to be measured | Sampling ratio | Mean square error |
Test 1 | 0.65671 | 0.44514 | 0.44005 |
Test 2 | 0.03253 | 0.48486 | 0.37435 |
Test 3 | 0.7749 | 0.42601 | 0.96316 |
Test 4 | 0.99037 | 0.3317 | 0.54197 |
Test 5 | 0.1638 | 0.02419 | 0.50538 |
Test 6 | 0.44968 | 0.37192 | 0.10029 |
Test 7 | 0.87167 | 0.04717 | 0.60792 |