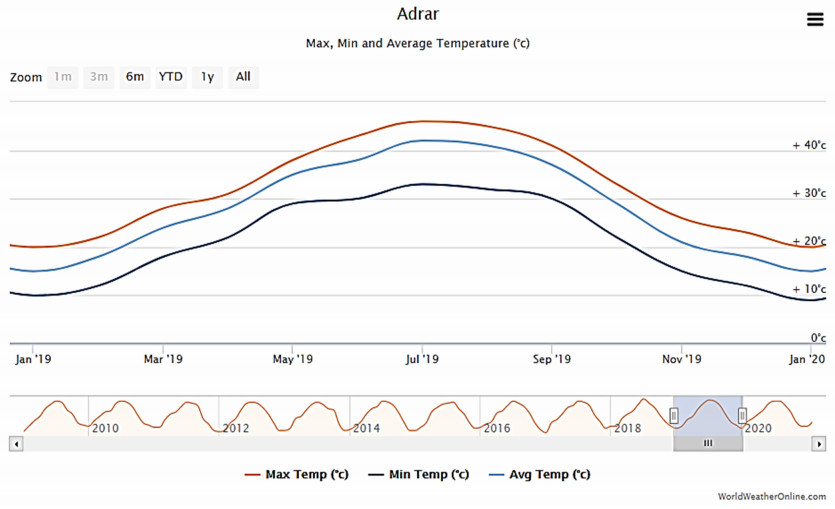
In this study, we explore the precise trajectory tracking control problem of autonomous underwater vehicle (AUV) under the disturbance of the underwater environment. First, a model-free adaptive control (MFAC) is designed based on data-driven ideology and a full-form dynamic linearization (FFDL) method is utilized to online estimate time-varying parameter pseudo gradient (PG) to establish an equivalent data model of AUV motion. Second, the iterative extended state observer (IESO) scheme is designed to combine with FFDL-MFAC. Because the proposed novel controller is able to learn from repeated iterations, the proposed novel controller can estimate and compensate the model approximation error produced by external environmental unknown disturbance. Third, three-dimensional motion is decoupled into horizontal and vertical and a multi closed-loop control structure is designed that exhibits faster convergence rate and reduces sensitivity to parameter jumps than single closed-loop system. Finally, two simulation scenarios are designed featuring non external disturbance and Gaussian noise of signal-to-noise ratio of 90 dB. The simulation results reveal the superiority of FFDL. Furthermore, we adpot the technical parameters data of T-SEA I AUV to conduct numerical simulation, aunderwater trajectory as the tracking scenario and set waves to 0.5 m and current to 0.2 m/s to simulate Lv.2 ocean conditions of "International Ocean State Standard". The simulation results demonstrate the effectiveness and robustness of the proposed tracking control algorithm.
Citation: Chengxi Wu, Yuewei Dai, Liang Shan, Zhiyu Zhu, Zhengtian Wu. Data-driven trajectory tracking control for autonomous underwater vehicle based on iterative extended state observer[J]. Mathematical Biosciences and Engineering, 2022, 19(3): 3036-3055. doi: 10.3934/mbe.2022140
[1] | Kong Fah Tee, Sayedali Mostofizadeh . Numerical and experimental investigation of concrete with various dosages of fly ash. AIMS Materials Science, 2021, 8(4): 587-607. doi: 10.3934/matersci.2021036 |
[2] | Ketut Aswatama Wiswamitra, Sri Murni Dewi, Moch. Agus Choiron, Ari Wibowo . Heat resistance of lightweight concrete with plastic aggregate from PET (polyethylene terephthalate)-mineral filler. AIMS Materials Science, 2021, 8(1): 99-118. doi: 10.3934/matersci.2021007 |
[3] | Abdul Saboor Karzad, Moussa Leblouba, Zaid A. Al-Sadoon, Mohamed Maalej, Salah Altoubat . Modeling the flexural strength of steel fibre reinforced concrete. AIMS Materials Science, 2023, 10(1): 86-111. doi: 10.3934/matersci.2023006 |
[4] | G. Sri Harsha, P. Poluraju, Veerendrakumar C. Khed . Computation of shear strength equation for shear deformation of reinforced concrete deep beams using finite element method. AIMS Materials Science, 2021, 8(1): 42-61. doi: 10.3934/matersci.2021004 |
[5] | Rein Terje Thorstensen . Preventing early age chloride migration into low-carbon concrete. AIMS Materials Science, 2019, 6(6): 1020-1032. doi: 10.3934/matersci.2019.6.1020 |
[6] | Ashhad Imam, Shashank Mishra, Yeetendra Kumar Bind . Review study towards corrosion mechanism and its impact on the durability of concrete structures. AIMS Materials Science, 2018, 5(2): 276-300. doi: 10.3934/matersci.2018.2.276 |
[7] | Marcílio M. A. Filho, Paulo A. G. Piloto, Carlos Balsa . The load-bearing of composite slabs with steel deck under natural fires. AIMS Materials Science, 2022, 9(1): 150-171. doi: 10.3934/matersci.2022010 |
[8] | Ayumu Yasue, Keita Hayashi, Shogo Yamamoto, Toshitsugu Inukai, Shigeru Fujimori . Influence of concrete bleeding due to mix proportion on the drilling speed of hardened surface layer. AIMS Materials Science, 2021, 8(3): 486-500. doi: 10.3934/matersci.2021030 |
[9] | Masanori Kikuchi . Study on multiple surface crack growth and coalescence behaviors. AIMS Materials Science, 2016, 3(4): 1623-1631. doi: 10.3934/matersci.2016.4.1623 |
[10] | Elisa Padovano, Francesco Trevisan, Sara Biamino, Claudio Badini . Processing of hybrid laminates integrating ZrB2/SiC and SiC layers. AIMS Materials Science, 2020, 7(5): 552-564. doi: 10.3934/matersci.2020.5.552 |
In this study, we explore the precise trajectory tracking control problem of autonomous underwater vehicle (AUV) under the disturbance of the underwater environment. First, a model-free adaptive control (MFAC) is designed based on data-driven ideology and a full-form dynamic linearization (FFDL) method is utilized to online estimate time-varying parameter pseudo gradient (PG) to establish an equivalent data model of AUV motion. Second, the iterative extended state observer (IESO) scheme is designed to combine with FFDL-MFAC. Because the proposed novel controller is able to learn from repeated iterations, the proposed novel controller can estimate and compensate the model approximation error produced by external environmental unknown disturbance. Third, three-dimensional motion is decoupled into horizontal and vertical and a multi closed-loop control structure is designed that exhibits faster convergence rate and reduces sensitivity to parameter jumps than single closed-loop system. Finally, two simulation scenarios are designed featuring non external disturbance and Gaussian noise of signal-to-noise ratio of 90 dB. The simulation results reveal the superiority of FFDL. Furthermore, we adpot the technical parameters data of T-SEA I AUV to conduct numerical simulation, aunderwater trajectory as the tracking scenario and set waves to 0.5 m and current to 0.2 m/s to simulate Lv.2 ocean conditions of "International Ocean State Standard". The simulation results demonstrate the effectiveness and robustness of the proposed tracking control algorithm.
In an arid climate, and hot weather, and very low relative humidity, significant additional stresses and strains can occur in the elements of a concrete structure due to shrinkage and temperature variation. Concrete cracking is expected to occur when these stresses' combined effects exceed the concrete's tensile strength. Moreover, the presence of cracks is not only undesirable from the aesthetic point of view; but more significantly. Moreover, it could adversely affect the durability of concrete members and their structural integrity in some situations.
The region of Adrar, located in southern Algeria, is an arid area where the concrete construction system has already replaced the old clay-based adobe construction system to improve structures' mechanical strength. However, worrying problems, such as cracking in most constructions, even in recent ones, were observed.
Researchers have provided different perspectives on classifying the early-age crack types in hardened concrete [1]. Cracking in concrete structures, if not avoided, may present one of the most severe problems in construction because it can reduce their durability and lifetime [2]. It is worth noting that the durability of concrete structures depends on their behavior in the face of severe climatic and environmental conditions [3] Shrinkage and thermal stresses experienced during concrete curation and hydration often lead to severe early-age cracks [4]. These problems may become even more severe when cracking occurs before the concrete begins to harden [5].
It is well known that the excessive evaporation of the mixing water dramatically influences the durability of concrete. It should be remembered that the temperature in these southern regions can reach significant values in the summer period; it can even exceed 50 ℃ [6]. Compressive concrete strength at the initial days was deficient and increased with an increase in the age up to 28 days [7].
Furthermore, very low relative humidity can also be responsible for cracking in concrete [8]. Reinforced concrete structures are often exposed to numerous undesirable physicochemical attacks, increasing the risk of cracking [9]. Moreover, the concrete formulation may also affect plastic cracking from chemical and physical points of view [10,11]. It is worth citing, by way of example, the constituents' chemical composition, the amount of cement, the ratio (W/C), the quantity, shape, and size of fines, and the distribution of agammaegates [12,13]. Cracks can be also developed due to various factors like internal/external pressure or loading criteria, curing, material, mixing, environmental effects, improper compaction, etc. [14]. Therefore, to improve the durability of concrete structures in engineering, cracks, wildly penetrating cracks, caused by early-age shrinkage must be mitigated [15]. However, the concrete mixed workability is increased and maintained using more superplastic is added to increase the concrete's durability [16].
The most significant negative impact of hot weather on fresh concrete is cracking due to plastic shrinkage [17]. Almusallam et al. studied the effect of mixing proportions on plastic shrinkage cracking of concrete in hot environments, the cumulative effect of these parameters on the plastic shrinkage of concrete was evaluated by measuring the bleeding rate, water evaporation, time and cracking intensity. The results indicate that cement content and water-cement ratio significantly affect the parameters controlling the plastic shrinkage of concrete. The mix design studied can be advantageously used in hot environments to minimize plastic shrinkage cracking [17].
Also, the effects of a hot climate on the cooling and curing properties of self-hardening concrete and normal conventional concrete in hot weather were investigated by Rizzuto et al., They used a water-soluble self-hardening polymer, polyethylene glycol, was added to the self-curing mixes. They tested the properties, as workability, compressive strength, tensile strength and flexural strength, but the results could not be simply attributed to the mix's water retention by the self-hardening admixture [18]. A large span of floor slabs, exposed to dry environmental conditions, promotes high evaporation rates [19].
With this in mind, and to study the impact of arid climatic conditions on concrete cracking, it decided to conduct the following experimental study. For this, concrete mixtures, similar to those prepared on local sites, were prepared using the same local materials and to respect the same method of formulation of the concrete, while maintaining the same severe climatic conditions.
The first step consisted of identifying and characterizing the materials brought from the most recognized quarries in the region. The preparation of concrete mixes in the laboratory was carried out following the Faury method [20], which is generally applied in the region of Adrar. Then, the concrete was placed in standard molds. After demolding, the specimens were exposed to the summer climate during which the temperature could reach 48 ℃, with a humidity level lower than 10%. Two conservation methods were adopted; some samples were stored inside the laboratory, and the others were kept outside, in the open air, according to the American Standard ASTM C 1579 [21].
The region of Adrar is located in the southwestern part of Algeria, where the climate is desert and hot, according to the Köppen BWh classification [22]. Adrar is known for its high temperatures during the summer season (spread over more than 6 months) and shallow temperatures in winter, in addition to its year-round drought (few mm of annual precipitation), and a deficient humidity level in summer [23].
The average maximum temperatures vary between 46 and 50 ℃, which confirms that Adrar is one of the world's hottest cities.
The World Weather Online site, dedicated to providing global weather forecasts and weather content [24], gives the variations in temperature and relative humidity recorded during the year 2019. These variations are shown in Figures 1 and 2. It is easy to see that July and August are the hottest months of the year. It is for this reason that the present experimental study was carried out during that period.
It is worth noting that all the constituents of our concretes come from locally sourced materials, which are virtually used in the majority of private or public construction sites in the region of Adrar.
The cement used is type CEMII, CPJ 42.5, and comes from the Aoulef Cement Plant located 150 km south of the capital; its commercial name is STG. Its composition is given in Table 1.
Element | Content (%) |
SiO2 | 21.30 |
Al2O3 | 4.83 |
Fe2O3 | 3.31 |
CaO | 64.53 |
SO3 | 2.28 |
MgO | 1.43 |
Free CaO | 0.61 |
The sand is generally brought from dunes in the locality of Bouda, 20 km west of Adrar.
Particle-size analysis of dune sand:
The analysis of the agammaegates used, including sand, is displayed below (Figure 3), following Standard NF P 18-560 [25].
The water content is 1.6% for dune sand.
The absolute volumetric mass density of dune sand is of the order of 1.63 g/cm3.
The sand equivalent is an indicator that characterizes the cleanliness of sand. It also indicates the content of fines, which are elements with a diameter smaller than 0.5 mm.
The tests were carried out according to Standard NF EN 933-8/IN1[26]. The values obtained, illustrated in Figure 4, allow concluding that the dune sand from Bouda is spotless.
Fineness modulus of dune sand: according to Standard NF P 18 304 [27], is equal to one-hundredth of the sum of rejects, expressed as a percentage by weight, on the various sieves in the series, except the 0.08 sieve. The sand of the dune of Bouda is a little too fine, with a modulus of fineness Mf = 1.90.
The first step consisted of physically and mechanically characterizing the materials used. Note that the gravel comes from the Koussane quarry, located about 8 km from Adrar.
The absolute volumetric mass of the agammaegates:
According to Standard NF EN 12620+A1[28], the absolute volumetric mass densities are around 2.5 g/cm3 for agammaegates 3/8 and 8/15. However, the apparent volumetric mass densities are 1.39 g/cm3 for the agammaegate 3/8 and 1.48 g/cm3 for the agammaegate 8/15.
The water content of agammaegates is between 1% to 2% for the agammaegates 3/8 and 8/15.
Coefficient of absorption of agammaegates is defined as the ratio of an increase in the mass of a water-soaked sample to that sample's dry mass. Table 2 shows the coefficient values of the different agammaegates.
Granular class | Ab (%) |
3/8 | 2.68 |
8/15 | 2.88 |
The hardness and abrasion of agammaegates were evaluated using the Los-Angeles (LA) and Micro-Deval (MDE) tests, and the results obtained are reported in Figure 5.
Note that the sample is more resistant to wear when the Micro-Deval coefficient is low.
The absorption coefficient of agammaegates is defined as the ratio of an increase in the mass of a water-soaked sample to that sample's dry mass.
As for the mixing water used, it comes from the drinking water supply network from the Oued-Ezzine (Adrar) water table.
The adjuvant, namely MEDAFLOW 3041 R, a high-water reducer and setting retarder, was used by standard NF EN 934-2 [29]. It is prepared based on synthetic and combined polymers. The adjuvant MEDAFLOW 3041 R has a setting retarding effect as a secondary function. This allows us to achieve also a sharp increase in compressive strength [30].
Following Standard NF P 18-370 [31], and for the sake of protecting the prepared concrete against desiccation, a curing product named MEDACURE was used. This product comes in the form of a whitish liquid, intended to cover fresh concretes and mortars.
The Faury method for concrete mix design was selected for this study as it is the most widely used in the region of Adrar. It is essential to mention that some project developers use adjuvants and even curing agents to protect the concrete. Indeed, several studies have focused on the incorporation of adjuvants in concrete [25,26,27,28,31].
The mixing curves: based on the granular curves of Figure 6, it was deduced that the percentages obtained for each constituent, namely (dune sand + cement), gravel 3/8, and gravel 8/15, were respectively 44%, 24%, and 32%. The dosages of the various constituents for 1 m3 of concrete are summarized in Table 3.
Materials | Masses for control concrete and with curing (kg/m3) | Masses for concretes with adjuvant (kg/m3) |
Cement | 350 | 350 |
Dune sand | 581 | 602 |
Gravel 3/8 | 471 | 497 |
Gravel 8/15 | 628 | 661 |
Water | 255 | 198 |
Adjuvant | 0 | 5.8 |
Several tests were carried out on slabs to study the potential of plastic shrinkage cracking in concrete. Each set of three slabs was subjected to two types of preservation, i.e., one inside the laboratory and the other outside.
According to ASTM C 1579, cracking usually; occurs in the middle of the specimens [32].
Among the crack mitigation methods previously investigated by some researchers, the one relating to the introduction of adjuvants into concrete proved to be interesting [33].
It is worth specifying that these adjuvants can reduce the surface tension of water in concrete, which in turn; reducing water evaporation, settlement of fresh concrete, and capillary pressures. These are the main effects of reducing plastic shrinkage [11,34]. Also, the curing compounds used on construction sites can effectively prevent rapid water loss from concrete and minimizing cracking risk. To this end, mixtures of concrete with adjuvants similar to those used in the region's construction sites were examined.
After determining the various percentages and quantities of the components making up our concretes, it was decided to proceed with the preparation of the mixtures and the casting of small slabs, according to Standard ASTM 1579 [21].
Since many other parameters, such as granulometry, concrete formulation procedures, slump, concrete temperature, can also influence potential cracking, care should be taken to ensure that they are very similar.
Molds were then made according to Standard ASTM C1579, as shown in Figure 7, and the concrete mixtures were prepared according to the method of Faury [20].
The indicator showed a temperature around 37 ℃ inside the laboratory. The unfavorable climatic conditions, generally encountered in summer (high temperature, low relative humidity, dry air, wind, and sun) during the placement of exposed concrete can contribute to the rapid loss of water and may therefore give rise to crazing and cracking problems, particularly during the setting period and at the onset of concrete hardening.
The adjuvants were incorporated during the mixing of concrete at a rate of 0.5% of the cement mass in the concrete mix, according to Standard NF EN 934-2/A2 [35].
Curing was also used to protect concrete against rapid drying and minimize the risk of cracking. Following the disruption of hydration due to lack of water in early concrete, a curing product was applied by spraying to the surface of fresh concrete according to Standard NF P 18-370 [31]. In certain cases, this curing product is compulsory (Standard NF EN 13670) [36].
Three sets of specimens were adopted, with each set containing six slabs; three were kept outside the laboratory, in the open air, and the other three were stored inside the laboratory. The various concrete mixtures produced are:
-Concrete without adjuvant (control mixture);
-Concrete with adjuvant;
-Concrete treated with a curing product.
The first step was to control the variation in fresh concrete temperatures in the three concretes, as clearly shown in Figure 8.
The measurement of the temperature of fresh concrete with the measuring instrument, i.e., the concrete thermometer. The recorded values are shown in the histogram shown in Figure 9.
The temperature of fresh control concrete without adjuvant was equal to 37 ℃; it is identical to that of the concrete treated with the curing agent. On the other hand, for the concrete with adjuvant, the indicator registered on average the temperature of 36 ℃. This was due to the introduction of the adjuvant into the concrete.
The slump is determined by using the Abrams cone (Standard NF P18-451) which measures concrete slump [37]. We proceeded to mix the concrete so that the workability is maintained constant at plastic consistency [38].
The slump estimates the concrete's consistency, as shown in Figure 10. The value of the Abrams cone slump for all concretes is given in Figure 11. These values obtained for the three types of concretes are divided into two classes; the first is that of the control and cured concretes, and the second is that of the concrete containing the admixture.
The control slabs and those treated with the curing agent showed an average sag of 8 cm, which means that it is plastic concrete. However, the adjuvant's slabs exhibited a sag of about 10 cm, making it very plastic concrete.
In studies on cracking, especially cracking of fresh concrete, it is essential to have appropriate methods to quantify free shrinkage: the mechanism and cracking: the consequence. Several disadvantages and limitations of the available methods are as follows.
The breaking mechanism of plastic shrinkage (cracking) in the dry state can be summarized as follows. The rapid evaporation of water from the surface pores, while the entire body is still saturated, leads to large deformations. The evaporation from the surface pores causes the formation of menisci in these pores and increases capillary pressure. The capillary pressure causes a dramatic increase in rigidity, which leads to a corresponding increase in stress. When these stresses exceed the breaking limit, cracking begins [39].
Three types of concrete were made and divided into two sets; the first was stored inside the laboratory, and the second was outside, in the open air. To simplify the reading, the following names were given to the various slabs:
CSO: concretes without adjuvant (control), slabs stored outside the laboratory;
CSI: concretes without adjuvant (control), slabs stored inside the laboratory;
SAO: concretes with adjuvant, slabs stored outside the laboratory;
SAI: concretes with adjuvant, slabs stored inside the laboratory;
SCO: concretes treated with a curing product, slabs stored outside the laboratory;
SCI: concretes treated with a curing product, slabs stored inside the laboratory.
After each casting of the slabs, the cracks' evolution and their development in lengths and widths were monitored from their first appearance as shown in Figure 12. To better interpret the results, this progression the standard deviation (S.D) is given in the form of graphs.
The performance of passive plastic shrinkage crack mitigation methods was compared according to the ASTM standard, comparing final crack sizes.
Figure 13a shows that, after the second hour, cracks suddenly appeared remarkably, in the middle of the three slabs, with significant lengths (from 10 to over 20 cm). After about two hours, this crack length increased by about 10 cm for all slabs. After 5 hours of casting, these values kept increasing and stabilized around 33 cm. It should be noted that the crack's width could reach even 0.6 mm (Figure 13a).
On the other hand, for the same formulation, the control slabs stored inside the laboratory, sheltered from the sun and wind (Figure 13b), presented the same aspect of progression over time. However, after the sixteenth hour, the cracks' initiation was not observed; by the nineteenth hour, these cracks' progression stopped altogether, for an average length of 17 cm. In this case, the progression of the cracks lasted only three hours. It should be noted that the length of these cracks was significantly smaller than that of cracks on the slabs kept outside the laboratory. Their widths did not exceed 0.2 mm (Figure 14a), which is three times less than those observed on slabs kept outside the laboratory. This difference in crack width proves the strong impact of the climate on concrete structures in this region. This phenomenon is mainly due to the rapid evaporation of water.
For the concretes incorporating the adjuvant and the three slabs cast and kept in the open air outside the laboratory (Figure 14c), the curve shows cracks in the third hour. In contrast, the length of cracks remained constant in all the slabs throughout the observation period. Indeed, the recorded crack lengths varied between 12 and 14 cm. Furthermore, it was found that the crack widths were about 0.2 mm. They are smaller than those of concretes without adjuvant. This is undoubtedly due to the effect of the adjuvant.
Cracks appeared on the slabs with the adjuvant and were stored in the laboratory (Figure 13d). The cracks' progression rate was nearly the same; the average length value was about 32 cm. After three hours, the progression of cracks in all the slabs stopped completely. It should also be noted that the width of cracks was smaller than that observed on the slabs kept outside the laboratory (Figure 14b); indeed, it did not exceed 0.1 mm, which supports the climate impact on concrete.
The concrete treatment results with a curing product are shown in (Figure 13e), where small cracks appeared from the first hour. Under the curing agent treatment effect, which prevented the rapid evaporation of water present in the concrete, the evolution of cracking became slower until the sixteenth hour of hardening. Note that the crack length increased from 5 to about 20 cm, with a crack width varying between 0.2 and 0.3 mm. This represents a value less than half of that observed in the control slabs (without curing treatment).
On the other hand, concerning the slabs treated with a curing product and stored outside the laboratory, cracks appeared within the first two hours (Figure 13f); their length was constant and about 26 cm. These cracks did not exceed 0.1 mm in width (Figure 14c). It is worth noting that the curing effect aided in the retention of the mixed water inside concrete.
High ambient temperature, low humidity, and high-wind speed increase water evaporation from concrete surfaces with the possibility of plastic shrinkage cracking.
This evaporation is all the more critical when the ambient air is dry (low RH), when the temperature is high and when the wind speed is essential.
Particularly in arid areas, chemical reactions accelerate and modify the crystallization of hydrates. Also, the hydration of cement is an exothermic reaction. The resulting plastic deformations are a complex combination of mechanisms appearing at different scales whose effect is cumulative to two types of drying; drying at high ambient temperatures with the effect of the and drying at high ambient temperatures without effect the wind.
Air moisture is of paramount importance to the water balance of concrete. When the concrete's moisture content is higher than that of the air (as in arid areas), water tends to evaporate into the air.
These phenomena lead, in particular, to an increase in shrinkage and risks of early cracking.
According to the results obtained and shown in the Figures 13 and 14, the appearance of cracks and their development is also mentioned in Table 4.
Slabs | Average onset of cracking (h) | Average initial crack length (cm) | Average final crack length (cm) | Average crack width (mm) |
CSO | 2.68 | 163.3 | 337.0 | 0.57 |
CSI | 16.53 | 102.7 | 173.0 | 0.20 |
SAO | 2.30 | 116.0 | 120.0 | 0.23 |
SAI | 2.68 | 130.0 | 234.7 | 0.10 |
SCO | 1.21 | 45.0 | 199.0 | 0.24 |
SCI | 2.33 | 244.3 | 266.7 | 0.10 |
Based on the analysis of the values presented in Table 4, and by comparing the experimental results of slabs kept inside the laboratory with those kept outside, we found that the first crack always appears in slabs kept outside the laboratory (CSO, SAO, and SCO). Also, the cracks' lengths and widths' critical values are observed in the slabs kept outside the laboratory (SCI, SAI, and SCI), which is explained by the fact that outside the laboratory, the climate is hot and dry. The development of cracks in slabs stored outside the laboratory is faster and more critical than that of slabs stored indoors.
Consequently, the appearance of cracks in this hot and arid climate can start to appear, in general, from the second hour of pouring and then accelerate. However, the addition of the admixture reduces the water's surface tension in the concrete, and treatment with the curing agent prevents the water loss from the concrete. These treatments reduce plastic shrinkage, delay the appearance of cracks and slow their development. They considerably reduce their widths.
The standard deviation for concrete is the method for determining the reliability of a batch of concrete. The standard deviation is the basis for controlling the variability of concrete test results for the same concrete batch.
The maximum standard deviations obtained were 8 and 10 mm, in crack length for all slabs and various formulations outside the laboratory. The same goes for those inside the laboratory (Figure 13a, c, e). Figure 13b, d, f show the lowest value for that inside the laboratory.
The standard deviation is between 0 and 0.1 mm width cracks. These values gave a good indication of the presence of crack and the values correlated.
The crack was visible at an exact position indicated by a characteristic response of the crack and its location. Further, the confidence interval obtained from the standard deviation was very low, which proved the repeatability and feasibility of these results.
This study aimed to investigate the effect of severe climatic conditions, which characterize Adrar's region (arid zone), on the cracking of concrete constructions in that region, using local materials. It was decided to introduce an adjuvant on the one hand, and on the other hand, a curing product into the concretes under study.
The first step consisted of carrying out experimental tests to characterize of materials, calculate subsidence, and measure the temperature of fresh concrete. The second step focused on the evolution of cracks and their dimensions (length and width of the crack).
The main conclusions drawn from this study are as follows:
● Crack initiation always occurs first on slabs stored outside the laboratory, with more rapid evolution. On the other hand, the cracks are slightly delayed on slabs kept inside the laboratory;
● Comparison of crack widths on slabs kept outside the laboratory and on those kept inside the laboratory confirmed the significant impact of the arid climate of this region on concrete;
● The findings indicated that the crack widths on slabs kept inside the laboratory were about 66% smaller than those kept outside the laboratory;
● The introduction of the adjuvant into the mixture significantly reduced the progress of cracking. A cracking reduction rate of approximately 50% in length and 66% in width was recorded;
● The treatment with a curing product contributed to slowing down the evolution of cracks by protecting the concrete against rapid drying, by approximately 40% and 50% in length and width, respectively, compared with the control slabs kept outside the laboratory. This drop was approximately 50% for the crack width than that observed on slabs kept outside the laboratory;
● Cracking due to plastic shrinkage is significant in this arid region. It would be interesting to develop low-shrinkage concretes that minimize cracking. It is therefore essential to cure the surface of the concrete during the first two or three days. It can also be achieved by using a shrinkage-reducing admixture;
● Based on the results obtained from our experimental program and in comparison, with control slabs (without addition and treatment), it is recommended to improve the concrete formulation based on local materials with better thermo-mechanical properties.
All authors declare no conflicts of interest in this paper.
[1] |
A. Tonacci, G. Lacava, M. A. Lippa, L. Lupi, M. Cocco, C. Domenici, Electronic nose and AUV: A novel perspective in marine pollution monitoring, Mar. Technol. Soc. J., 49 (2015), 18–24. https://doi.org/10.4031/MTSJ.49.5.4 doi: 10.4031/MTSJ.49.5.4
![]() |
[2] | B. Christ, J. Klara, 2016, A new approach to wide area survey multiple AUV application, in OCEANS 2016 MTS/IEEE Monterey, IEEE, Monterey, CA, USA, (2016), 1–6. https://doi.org/10.1109/OCEANS.2016.7761449 |
[3] |
X. Cao, H. Sun, L. Guo, Potential field hierarchical reinforcement learning approach for target search by multi-AUV in 3-d underwater environments, Int. J. Control, 93 (2020), 1677–1683. https://doi.org/10.1080/00207179.2018.1526414 doi: 10.1080/00207179.2018.1526414
![]() |
[4] |
A. Sultan, M. L. Ricardo, T. Thomas, H. Ibrahim, M. K. Omar, Optimal 3D time-energy trajectory planning for AUVs using ocean general circulation models, Ocean Eng., 218 (2020), 108057. https://doi.org/10.1016/j.oceaneng.2020.108057 doi: 10.1016/j.oceaneng.2020.108057
![]() |
[5] | K. Teo, E. An, P. P. J. Beaujean, A robust fuzzy autonomous underwater vehicle (AUV) docking approach for unknown current disturbances, IEEE J. Ocean. Eng., 37 (2), 143–155. https: //doi.org/10.1109/JOE.2011.2180058 |
[6] | C. Samson, Path following and time-varying feedback stabilization of a wheeled mobile robot, in International Conference on Advanced Robotics and Computer Vision, Singapore, 13 (1992). |
[7] |
Z. Chu, D. Zhu, S. X. Yang, Observer-based adaptive neural network trajectory tracking control for remotely operate dvehicle, IEEE Trans. Neural. Netw. Learn. Syst., 28 (2017), 1633–1645. https://doi.org/10.1109/TNNLS.2016.2544786 doi: 10.1109/TNNLS.2016.2544786
![]() |
[8] |
R. Da Silva Tchilian, E. Rafikova, S. A. Gafurov, M. Rafikov, Optimal control of an underwater glider vehicle, Procedia Eng., 176 (2017), 732–740. https://doi.org/10.1016/j.proeng.2017.02.322 doi: 10.1016/j.proeng.2017.02.322
![]() |
[9] |
T. I. Fossen, S. I. Sagatun, Adaptive control of nonlinear systems: A case study of underwater robotic systems, J. Robot. Syst., 8 (1991), 393–412. https://doi.org/10.1002/rob.4620080307 doi: 10.1002/rob.4620080307
![]() |
[10] |
T. Elmokadem, M. Zribi, K. Youcef-Toumi, Terminal sliding mode control for the trajectory tracking of under actuated autonomous underwater vehicles, Ocean Eng., 129 (2017), 613–625. https://doi.org/10.1016/j.oceaneng.2016.10.032 doi: 10.1016/j.oceaneng.2016.10.032
![]() |
[11] |
Y. Zhang, X. Liu, M. Luo, C. Yang, MPC-based 3-D trajectory tracking for an autonomous underwater vehicle with constraints in complex ocean environments, Ocean Eng., 189 (2019), 106309. https://doi.org/10.1016/j.oceaneng.2019.106309 doi: 10.1016/j.oceaneng.2019.106309
![]() |
[12] |
A. A. R. Al Makdah, N. Daher, D. Asmar, E. Shammas, Three-dimensional trajectory tracking of a hybrid autonomous underwater vehicle in the presence of underwater current, Ocean Eng., 185 (2019), 115–132. https://doi.org/10.1016/j.oceaneng.2019.05.030 doi: 10.1016/j.oceaneng.2019.05.030
![]() |
[13] | Z. S. Hou, The Parameter Identification, Adaptive Control and Model Free Learning Adaptive Control for Nonlinear Systems, Ph.D thesis, Northeastern University, China, 1994. |
[14] |
Z. Hou, R. Chi, H. Gao, An overview of dynamic-linearizationbased data-driven control and applications, IEEE Trans. Ind. Electron., 64 (2016), 4076–4090. https://doi.org/10.1109/TIE.2016.2636126 doi: 10.1109/TIE.2016.2636126
![]() |
[15] |
Z. Peng, J. Hu, B. K. Ghosh, Data-driven containment control of discrete-time multi-agent systems via value iteration, Sci. China Inf. Sci., 63 (2020), 1–3. https://doi.org/10.1007/s11432-018-9671-2 doi: 10.1007/s11432-018-9671-2
![]() |
[16] |
Z. Peng, R. Luo, J. Hu, K. Shi, S. K. Nguang, B. K. Ghosh, Optimal tracking control of nonlinear multiagent systems using internal reinforce Q-learning, IEEE Trans. Neural. Netw. Learn. Syst., 2021. https://doi.org/10.1109/TNNLS.2021.3055761 doi: 10.1109/TNNLS.2021.3055761
![]() |
[17] |
Y. Liao, T. Du, Q. Jiang, Model-free adaptive control method with variable forgetting factor for unmanned surface vehicle control, Appl. Ocean Res., 93 (2019), 101945. https://doi.org/10.1016/j.apor.2019.101945 doi: 10.1016/j.apor.2019.101945
![]() |
[18] |
Y. Jiang, X. Xu, L. Zhang, Heading tracking of 6WID/4WIS unmanned ground vehicles with variable wheel base based on model free adaptive control, Mech. Syst. Signal Process., 159 (2021), 107715. https://doi.org/10.1016/j.ymssp.2021.107715 doi: 10.1016/j.ymssp.2021.107715
![]() |
[19] |
C. Zhu, B. Huang, B. Zhou, Y. Su, E. Zhang, Adaptive model-parameter-free fault-tolerant trajectory tracking control for autonomous underwater vehicles, ISA Trans., 114 (2021), 57–71. https://doi.org/10.1016/j.isatra.2020.12.059 doi: 10.1016/j.isatra.2020.12.059
![]() |
[20] |
B. Chen, J. Hu, Y. Zhao, B. K. Ghosh, Finite-time velocity-free observer-based consensus tracking for heterogeneous uncertain AUVs via adaptive sliding mode control, Ocean Eng., 237 (2021), 109565. https://doi.org/10.1016/j.oceaneng.2021.109565 doi: 10.1016/j.oceaneng.2021.109565
![]() |
[21] |
X. Li, C. Ren, S. Ma, X. Zhu, Compensated model-free adaptive tracking control scheme for autonomous underwater vehicles via extended state observer, Ocean Eng., 217 (2020), 107976. https://doi.org/10.1016/j.oceaneng.2020.107976 doi: 10.1016/j.oceaneng.2020.107976
![]() |
[22] |
L. Hou, P. He, W. Wang, X. Yan, N. Tu, Research on model-free adaptive sliding mode control for PMSM based on ESO, Control Eng., 2021. http://doi.org/10.14107/j.cnki.kzgc.20200590 doi: 10.14107/j.cnki.kzgc.20200590
![]() |
[23] |
A. Saleki, M. M. Fateh, Model-free control of electrically driven robot manipulators using an extended state observer, Comput. Electr. Eng., 87 (2020), 106768. https://doi.org/10.1016/j.compeleceng.2020.106768 doi: 10.1016/j.compeleceng.2020.106768
![]() |
[24] |
Z. S. Hou, S. D. Liu, T. Tian, Lazy-learning-based data driven model-free adaptive predictive control for a class of discrete-time nonlinear systems, IEEE Trans. Neural. Netw. Learn. Syst., 28 (2017), 1914–1928. https://doi.org/10.1109/TNNLS.2016.2561702 doi: 10.1109/TNNLS.2016.2561702
![]() |
[25] | T. I. Fossen, Handbook of Marine Craft Hydrodynamics and Motion Control, John Wiley & Sons, New York, 2011. https://doi.org/10.1002/9781119994138 |
[26] |
Z. S. Hou, S. T. Jin, A novel data-driven control approach for a class of discrete-time nonlinear systems, IEEE Trans. Control Syst. Technol., 19 (2011), 1549–1558. https://doi.org/10.1109/TCST.2010.2093136 doi: 10.1109/TCST.2010.2093136
![]() |
[27] | Z. Hou, S. Jin, Model Free Adaptive Control: Theory and Application, Science Press, Beijing, 2013. https://doi.org/10.1201/b15752 |
[28] | W. Rudin, Principles of Mathematical Analysis, McGraw-Hill, New York, 1976. |
[29] |
Z. S. Hou, S. T. Jin, Data-driven model-free adaptive control for a class of MIMO nonlinear discrete-time systems, IEEE Trans. Neural. Netw., 22 (2011), 2173–2188. https://doi.org/10.1109/TNN.2011.2176141 doi: 10.1109/TNN.2011.2176141
![]() |
[30] | Q. Zhou, Research on Dynamic Positioning Methods of Autonomous of Docking and Recovery Technology, Mast.D. thesis, Jiangsu University of Science and Technology, China, 2019. |
[31] | L. Jia, Research on Attitude Control of Autonomous of Docking and Recovery Technology, Mast.D. thesis, Jiangsu University of Science and Technology, China, 2021. |
1. | Talal O. Alshammari, Maurizio Guadagnini, Kypros Pilakoutas, The Effect of Harsh Environmental Conditions on Concrete Plastic Shrinkage Cracks: Case Study Saudi Arabia, 2022, 15, 1996-1944, 8622, 10.3390/ma15238622 |
Element | Content (%) |
SiO2 | 21.30 |
Al2O3 | 4.83 |
Fe2O3 | 3.31 |
CaO | 64.53 |
SO3 | 2.28 |
MgO | 1.43 |
Free CaO | 0.61 |
Granular class | Ab (%) |
3/8 | 2.68 |
8/15 | 2.88 |
Materials | Masses for control concrete and with curing (kg/m3) | Masses for concretes with adjuvant (kg/m3) |
Cement | 350 | 350 |
Dune sand | 581 | 602 |
Gravel 3/8 | 471 | 497 |
Gravel 8/15 | 628 | 661 |
Water | 255 | 198 |
Adjuvant | 0 | 5.8 |
Slabs | Average onset of cracking (h) | Average initial crack length (cm) | Average final crack length (cm) | Average crack width (mm) |
CSO | 2.68 | 163.3 | 337.0 | 0.57 |
CSI | 16.53 | 102.7 | 173.0 | 0.20 |
SAO | 2.30 | 116.0 | 120.0 | 0.23 |
SAI | 2.68 | 130.0 | 234.7 | 0.10 |
SCO | 1.21 | 45.0 | 199.0 | 0.24 |
SCI | 2.33 | 244.3 | 266.7 | 0.10 |
Element | Content (%) |
SiO2 | 21.30 |
Al2O3 | 4.83 |
Fe2O3 | 3.31 |
CaO | 64.53 |
SO3 | 2.28 |
MgO | 1.43 |
Free CaO | 0.61 |
Granular class | Ab (%) |
3/8 | 2.68 |
8/15 | 2.88 |
Materials | Masses for control concrete and with curing (kg/m3) | Masses for concretes with adjuvant (kg/m3) |
Cement | 350 | 350 |
Dune sand | 581 | 602 |
Gravel 3/8 | 471 | 497 |
Gravel 8/15 | 628 | 661 |
Water | 255 | 198 |
Adjuvant | 0 | 5.8 |
Slabs | Average onset of cracking (h) | Average initial crack length (cm) | Average final crack length (cm) | Average crack width (mm) |
CSO | 2.68 | 163.3 | 337.0 | 0.57 |
CSI | 16.53 | 102.7 | 173.0 | 0.20 |
SAO | 2.30 | 116.0 | 120.0 | 0.23 |
SAI | 2.68 | 130.0 | 234.7 | 0.10 |
SCO | 1.21 | 45.0 | 199.0 | 0.24 |
SCI | 2.33 | 244.3 | 266.7 | 0.10 |