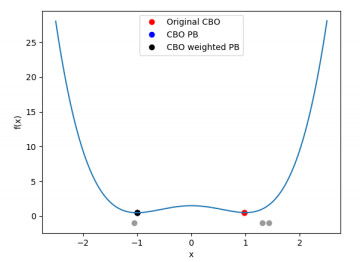
This study aimed to identify potential circular RNA (circRNA), microRNA (miRNA) and mRNA biomarkers as well as their underlying regulatory mechanisms in papillary thyroid carcinoma (PTC). Three microarray datasets from the Gene Expression Omnibus database as well as expression data and clinical phenotype from The Cancer Genome Atlas (TCGA) were downloaded, followed by differential expression, functional enrichment, protein–protein interaction (PPI), and module analyses. The support vector machine (SVM)-recursive feature elimination (RFE) algorithm was used to screen the key circRNAs. Finally, the mRNA-miRNA-circRNA regulatory network and competitive endogenous RNA (ceRNA) network were constructed. The prognostic value and clinical correlations of key mRNAs were investigated using TCGA dataset, and their expression was validated using the UALCAN database. A total of 1039 mRNAs, 18 miRNAs and 137 circRNAs were differentially expressed in patients with PTC. A total of 37 key circRNAs were obtained using the SVM-RFE algorithm, whereas 46 key mRNAs were obtained from significant modules in the PPI network. A total of 11 circRNA-miRNA pairs and 40 miRNA-mRNA pairs were predicted. Based on these interaction pairs, 46 circRNA-miRNA-mRNA regulatory pairs were integrated, of which 8 regulatory pairs in line with the ceRNA hypothesis were obtained, including two circRNAs (circ_0004053 and circ_0028198), three miRNAs (miR-199a-5p, miR-199b-5p, and miR-7-5p), and five mRNAs, namely APOA2, CCL20, LPAR5, MFGE8, and TIMP1. Survival analysis showed that LPAR5 expression was associated with patient survival. APOA2 expression showed significant differences between metastatic and non-metastatic tumors, whereas CCL20, LPAR5, MFGE8 and TIMP1 showed significant differences between metastatic and non-metastatic lymph nodes. Overall, we identified several potential targets and regulatory mechanisms involved in PTC. APOA2, CCL20, LPAR5, MFGE8, and TIMP1 may be correlated with PTC metastasis.
Citation: Jie Qiu, Maolin Sun, Chuanshan Zang, Liwei Jiang, Zuorong Qin, Yan Sun, Mingbo Liu, Wenwei Zhang. Five genes involved in circular RNA-associated competitive endogenous RNA network correlates with metastasis in papillary thyroid carcinoma[J]. Mathematical Biosciences and Engineering, 2021, 18(6): 9016-9032. doi: 10.3934/mbe.2021444
[1] | Fei Chen, Yanmin Liu, Jie Yang, Meilan Yang, Qian Zhang, Jun Liu . Multi-objective particle swarm optimization with reverse multi-leaders. Mathematical Biosciences and Engineering, 2023, 20(7): 11732-11762. doi: 10.3934/mbe.2023522 |
[2] | Xuepeng Zheng, Bin Nie, Jiandong Chen, Yuwen Du, Yuchao Zhang, Haike Jin . An improved particle swarm optimization combined with double-chaos search. Mathematical Biosciences and Engineering, 2023, 20(9): 15737-15764. doi: 10.3934/mbe.2023701 |
[3] | Xiaoding Meng, Hecheng Li, Anshan Chen . Multi-strategy self-learning particle swarm optimization algorithm based on reinforcement learning. Mathematical Biosciences and Engineering, 2023, 20(5): 8498-8530. doi: 10.3934/mbe.2023373 |
[4] | Yuhang Yao, Jiaxin Yuan, Tao Chen, Xiaole Yang, Hui Yang . Distributed convex optimization of bipartite containment control for high-order nonlinear uncertain multi-agent systems with state constraints. Mathematical Biosciences and Engineering, 2023, 20(9): 17296-17323. doi: 10.3934/mbe.2023770 |
[5] | Minna Shao, Hongyong Zhao . Dynamics and optimal control of a stochastic Zika virus model with spatial diffusion. Mathematical Biosciences and Engineering, 2023, 20(9): 17520-17553. doi: 10.3934/mbe.2023778 |
[6] | Pengpeng Wang, Xu Wang, Yumin Shen, Jinlong Wang, Xiaoyun Xiong . PBFT optimization algorithm based on community contributions. Mathematical Biosciences and Engineering, 2023, 20(6): 10200-10222. doi: 10.3934/mbe.2023447 |
[7] | Jiangtao Dai, Ge Guo . A leader-following consensus of multi-agent systems with actuator saturation and semi-Markov switching topologies. Mathematical Biosciences and Engineering, 2024, 21(4): 4908-4926. doi: 10.3934/mbe.2024217 |
[8] | Yuting Zhu, Wenyu Zhang, Junjie Hou, Hainan Wang, Tingting Wang, Haining Wang . The large-scale group consensus multi-attribute decision-making method based on probabilistic dual hesitant fuzzy sets. Mathematical Biosciences and Engineering, 2024, 21(3): 3944-3966. doi: 10.3934/mbe.2024175 |
[9] | Heping Ma, Hui Jian, Yu Shi . A sufficient maximum principle for backward stochastic systems with mixed delays. Mathematical Biosciences and Engineering, 2023, 20(12): 21211-21228. doi: 10.3934/mbe.2023938 |
[10] | H. J. Alsakaji, F. A. Rihan, K. Udhayakumar, F. El Ktaibi . Stochastic tumor-immune interaction model with external treatments and time delays: An optimal control problem. Mathematical Biosciences and Engineering, 2023, 20(11): 19270-19299. doi: 10.3934/mbe.2023852 |
This study aimed to identify potential circular RNA (circRNA), microRNA (miRNA) and mRNA biomarkers as well as their underlying regulatory mechanisms in papillary thyroid carcinoma (PTC). Three microarray datasets from the Gene Expression Omnibus database as well as expression data and clinical phenotype from The Cancer Genome Atlas (TCGA) were downloaded, followed by differential expression, functional enrichment, protein–protein interaction (PPI), and module analyses. The support vector machine (SVM)-recursive feature elimination (RFE) algorithm was used to screen the key circRNAs. Finally, the mRNA-miRNA-circRNA regulatory network and competitive endogenous RNA (ceRNA) network were constructed. The prognostic value and clinical correlations of key mRNAs were investigated using TCGA dataset, and their expression was validated using the UALCAN database. A total of 1039 mRNAs, 18 miRNAs and 137 circRNAs were differentially expressed in patients with PTC. A total of 37 key circRNAs were obtained using the SVM-RFE algorithm, whereas 46 key mRNAs were obtained from significant modules in the PPI network. A total of 11 circRNA-miRNA pairs and 40 miRNA-mRNA pairs were predicted. Based on these interaction pairs, 46 circRNA-miRNA-mRNA regulatory pairs were integrated, of which 8 regulatory pairs in line with the ceRNA hypothesis were obtained, including two circRNAs (circ_0004053 and circ_0028198), three miRNAs (miR-199a-5p, miR-199b-5p, and miR-7-5p), and five mRNAs, namely APOA2, CCL20, LPAR5, MFGE8, and TIMP1. Survival analysis showed that LPAR5 expression was associated with patient survival. APOA2 expression showed significant differences between metastatic and non-metastatic tumors, whereas CCL20, LPAR5, MFGE8 and TIMP1 showed significant differences between metastatic and non-metastatic lymph nodes. Overall, we identified several potential targets and regulatory mechanisms involved in PTC. APOA2, CCL20, LPAR5, MFGE8, and TIMP1 may be correlated with PTC metastasis.
Interacting particle systems play an important role in many applications in science - on the one hand as a modeling framework for social and biological systems, on the other as a tool for computational algorithms used in data science. In the latter case the collective behavior of interacting particle systems is used to solve high-dimensional problems, often resulting from non-convex optimization tasks in data science. Well known algorithms include particle swarm optimization (PSO) [1], ant colony optimization [2] or evolutionary [3] and genetic algorithms [4].
PSO was first introduced in [1] and has been successfully used in engineering applications [5]. Each particle in a PSO algorithm adjusts its position due to information of the global best, personal best and a noise term that allows for exploration of its neighborhood. Consensus-based optimization (CBO) [6,7] combines the idea of swarm intelligence with consensus formation techniques [8,9,10] to obtain a global optimization algorithm for non-convex high-dimensional problems. On the one hand particles explore the state space via an amplitude modulated random walk. On the other a drift term convects them towards the weighted global best. The method was first introduced in [6] and analyzed at the mean-field level in [7]. Recent developments of CBO include component-wise diffusion and utilize random mini-batch ideas to reduce the computational cost of calculating the weighted average [11]. Other contributions investigate a CBO dynamic that is restricted to the sphere [12,13]. Also, convergence and error estimates for time-discrete consensus-based optimization algorithms have been discussed [14]. CBO-type systems are related to large interacting particle systems, in which the dynamics are driven by weighted average quantities, see [15,16,17].
The model proposed in the work is based on the component-wise diffusion variant introduced in [11] and combines it with personal best information. This adjustment is motivated by the original work on PSO by Eberhart and Kennedy [1], where the particles move towards a (stochastic) linear combination of their personal best or the common global best position. The new information leads to an additional drift term in the dynamics. We investigate two types of memory effects - either using a weighted personal best over time or the personal best value in the past. The latter corresponds to record processes, see [18] for an overview. The former is used in the presented analysis and approximates the personal best of each particle. We expect by arguments similar to the Laplace principle that the weighted mean converges towards the personal best.
The proposed stochastic dynamics with weighted personal best fall into the class of stochastic functional differential equations. These equations are in general non-Markovian and their mean-field limit has been investigated in special cases only. For example, Gadat and Panloup [19] investigated a non-Markovian process with memory, which corresponds to the weighted average of the drift all along the particle's trajectory. This memory term is of a special form allowing them to rewrite the system as a 2-dimensional non-homogeneous Markovian dynamical system. Moreover, they exploit this special structure to analyze the existence and long time behavior of solutions as well as the mean-field limit. The strategy of increasing the dimension to get around the non-Markovian nature goes back to the Mori-Zwanzig formalism, see [20]. This strategy was recently adapted for non-Markovian interacting dynamics by Duong and Pavliotis in [21]. Another interesting work by Kuntzmann, see [22], investigates the ergodic behavior of self-interacting diffusions depending on the empirical mean of the process. The proposed generalization of CBO with weighted personal best does not fall into this category, hence the derivation and analysis of the respective mean-field dynamics, which often give useful insights into the dynamics, is to the best of the authors' knowledge open. This applies as well for personal best, where the update of the best function value corresponds to a record process. Hence, we focus on the well-posedness of the stochastic system as well as a detailed computational investigation of the dynamics.
This paper is organized as follows: we introduce the particle dynamics with (weighted) personal best in Section 2 and illustrate its dynamics with first toy examples. Section 3 discusses well-posedness and existence of solutions to the SDE model with weighted personal best. Section 4 presents extensive computational experiments of various benchmark optimization problems.
In this section we discuss how personal best information can be included in consensus based optimization algorithms as proposed by Carrillo and co-workers in [6,7,11]. We start by introducing the notation before continuing with the modeling.
We refer the euclidean norm by |x|=(x21+⋯+x2d)1/2 for x∈Rd and |Y|=(∑dNi,j=1Y2ij)1/2 for matrices Y∈RdN×dN. The set of natural numbers without 0 is denoted by N∗=1,2,3,… and the half-line [0,∞) by R+. A vector valued function or vector x∈RdN is assumed to be of the form x=(x1,…,xN) with xi∈Rd. When discussing the stochastic systems we follow the notation of [23]: (Ω,F,P,{Ft}t≥0) corresponds to the stochastic basis with sample space Ω, filtration F and probability function P. Moreover, Spd[0,T] is the space of (equivalence classes of) P -measurable continuous stochastic processes X:Ω×[0,T]→RdN such that
Esupt∈[0,T]|Xt|p<+∞ if p>0. |
Two processes X,Y are called equivalent if (Xt=Yt∀t∈[0,T])P-almost surely (P-a.s.). Furthermore, Spd is the space of (equivalence classes of) P-measurable continuous stochastic processes X:Ω×R+→Rd such that for all T>0 the restriction X|[0,T] of X to [0,T] belongs to Spd[0,T]. Analogously, we define Λpd(0,T) as the space of (equivalent classes) of P-measurable processes X:[0,T]→Rd such that
∫T0|Xt|2dt<+∞P-a.s.ω∈Ω if p=0andE(∫T0|Xt|2dt)p/2<+∞ if p>0. |
We refer to Λpd as the space of (equivalence classes of) P-measurable continuous stochastic processes X:Ω×(0,+∞)→Rd for which for all T>0 the restriction X|[0,T] of X to [0,T] belongs to Λpd(0,T). Moreover, for any ϕ∈C(R+,RdN) we define
‖ϕ‖t:=sup0≤s≤t|ϕ(s)|=sup0≤s≤t(ϕ1(s)2+⋯+ϕdN(s)2)1/2. |
We wish to approximate the global minimum
minx∈Rdf(x), | (2.1) |
of a given non-negative, continuous objective function f:Rd→R. In doing so we consider N∈N particles and denote the position of the i-th particle at time t by Xit:=Xi(t)∈Rd, i=1,…N. Note that we use Xt=X(t)=(X1(t),…XN(t))∈RdN, when referring to the positions of all particles at time t. In CBO particles compare their current function value with a weighted mean value based on the current information of the whole system. A particle moves towards the position of the weighted mean, if the function value of the weighted mean is lower. Following the ideas of [6,7], we use the weighted average
vf(t)=vf[Xt]=∑Ni=1Xi(t)exp(−αf(Xi(t)))∑Ni=1exp(−αf(Xi(t))), | (2.2) |
with α>0, to approximate the global best, that is, the particle with the lowest function value. Note that even though the weighted average uses only information of the current time step, it is assumed to approximate the global best over time as well, since a particle that is close to the weighed average experiences only small drift and diffusion. The parameter α scales the influence of local and global minima in the weighted mean. In fact, for α=0 the weights are independent of the function values, and all particles are weighted equally. For α>0 the particle with the best function value has the largest weight. Moreover, the Laplace principle from large deviations theory [24] assures that vf(t) converges to the global best, as α→∞. For more details on the Laplace principle in the CBO context, we refer to [6,7].
In the original version of PSO, see [25], particles compare their current position with the global best as well as their personal best value up to that time. We propose two different approaches how to include the personal best pi of the i-th particle. First, we consider the true personal best by setting
Pif(t)=argminY∈{Xi(s):s∈[0,t]}f(Y). | (2.3) |
Moreover, the personal best can be approximated similarly to the global best, vf(t), defined in (2.2). Hereby, we use the entire trajectory in the past and refer to this trajectory by X=(X1,…,XN) with Xi∈C(R+,Rd) for all i=1,…,N. Let Xi0 denote the initial position of the i-th particle at time t=0, the weighted mean over time of the i-th particle is defined by
pif(t)={Xi0,t=0,∫t0Xisexp(−βf(Xis))ds/∫t0exp(−βf(Xis))ds,otherwise, | (2.4) |
with β>0. Note that the well-posedness result presented in Section 3 holds for the weighted personal best (2.4) only. Again, by the Laplace principle, we expect that pif(t)→Pif as β→∞.
We recall that particles either move towards the global or personal best state. The respective CBO dynamics for the i-th particle, i=1,…N are then given by the following SDE:
dXi(t)=[−λ(t,X)(Xi(t)−vf)−μ(t,X)(Xi(t)−pif)]dt+√2σdiag(Xi(t)−vf)dBit, | (2.5) |
where
λ(t,X)=H(f(Xi(t))−f(vf))H(f(pif)−f(vf)),μ(t,X)=H(f(Xi(t))−f(pif))H(f(vf)−f(pif)). |
The function H corresponds to the Heaviside function and σ>0 denotes the standard deviation. System (2.5) is supplemented with the initial condition Xi0=ξi, i=1,…,N. The drift and diffusion are motivated by the following considerations:
1. If the global best vf is better than the current position Xit and the personal best pif, the particle moves towards the current global best vf.
2. If the personal best pif is better than the current position Xit and the global best vf, the particle moves towards the personal best pif.
3. If none of the above holds, the particle still explores the function landscape via Brownian motion until it reaches the global best vf.
Note that the drift coefficients depend on the past of each particle, hence system (2.5) is non-Markovian. The form of the memory does not allow us to use existing results, such as [23] to rewrite the system. Hence the existence and form of the respective mean-field model is, up to the authors' knowledge, not known.
Remark 1. (1) The CBO version proposed in [11] can be recoverd by setting
λ(t,X)≡λ,μ(t,X)≡0. | (2.6) |
(2) Note that the personal best (2.3) and weighted personal best (2.4) can be computed very efficiently due to their accumulative structure; this does not significantly increase the computational cost.
Throughout this manuscript we will refer to the dynamics defined by (2.5) with (2.6) as CBO, and to (2.5) with (2.3) or (2.4) as personal best (PB) or weighted personal best (wPB), respectively.
In the following we will illustrate the differences between CBO and (w)PB using a 1D toy objective function f and 3 particles. We consider a double well-type f of the form:
f(x)=(x2−1)2+0.01x+0.5. |
For this function, shown in Figure 1 the global and local minimum, located at x=−1.00125 and x=0.998748 respectively, are very close. In the following we perform 1000 Monte Carlo (MC) simulations with deterministic initial conditions ξ. We count a run as run successful, if the final position of the particles satisfy |vf(T)−Xi(T)|<0.4 for all i=1,…,N. The final time is set to T=100, the time step size dt=10−3 and β in (2.4) to β=30. We study the dynamics for the following two initial conditions:
(IC1) Initialize 2 particles near the local minimizer and 1 particle near the global minimizer.
(IC2) Initialize 1 particle near the local minimizer and 2 particles near the global minimizer.
The initial positions (IC1) and (IC2) of the particles correspond to the gray dots in Figure 1 and in Figure 3, respectively. We discuss (IC1) first. In this situation the weighted average, vf(0), is located near x=0.9, thus, the Heaviside functions are zero and the system would be in a stationary state for σ=0.
For σ>0, the diffusion term drives the dynamic and the particles are exploring their neighborhood. Due to the multiplicative factor, the particle on the left is exposed to more diffusion than the particles on the right. In case of the CBO scheme, the particle on the left has a high probability of jumping out of the basin of the global minimum. Then, all particles concentrate near the local minimum. For one run, this behavior is illustrated by the positions of vf(T) shown in Figure 1 (left). This alone does not reflect the concentration which becomes apparent in Figure 2. In fact, the orange lines show fluctuations for small times but stabilize quickly indicating that no diffusion is present and thus that all particles are concentrated. This behavior changes when personal best information is included. Here, particles still explore their neighborhood, however at some point their current positions are worse than their personal best, and hence the drift starts pulling them back towards their personal best. This behavior is also illustrated by the success rates stated in Table 1. We see that (w)PB outperform CBO for large and small values of α. The 'pull-back' effect slows down the convergence of (w)PB - we observe that the respective energies decrease slower than for CBO in Figure 2. Nevertheless, they find the global minimum.
scheme | success rate | success rate |
α=10 | α=30 | |
CBO | 30 % | 60, 9% |
PB | 100 % | 100 % |
wPB | 100 % | 100 % |
Next we consider initial condition (IC2). Again, in the deterministic case σ=0 the initial configuration is stationary. For σ>0 the particles on the left are less diffusive than the particle on the right. Therefore, it is more likely that the particle on the right jumps into the basin of the global minimum. This is illustrated in Figure 3 and confirmed by the success rates in Table 2. Again, CBO converges faster than (w)PB, see Figure 4. Nevertheless, the function values at the point of concentration are smaller for (w)PB which means that the slower algorithms find better approximations. Note that the scale of the time step-axis is much smaller than in Figure 2.
scheme | success rate | success rate |
α=10 | α=30 | |
CBO | 91, 6 % | 98, 1 % |
PB | 100 % | 100 % |
wPB | 100 % | 100 % |
In the following we discuss well-posedness of the wPB model. We begin by considering CBO with component-wise diffusion, which was proposed in [11].
Theorem 1. Let f be locally Lipschitz and N∈N. Then system (2.5) with λ(t,X)≡λ,μ(t,X)≡0 admits a unique strong solution for any initial condition ξ=(ξ1,…,ξN) satisfying E|ξ|2<∞.
A detailed proof can be found in the Appendix. Let us just emphasize that the estimates in the proof of Theorem 1 are independent of the dimension, d, as was already highlighted in [11] for the mean-field setting. This is in contrast to [6,7], where the estimates depend on d.
Next, we present an existence and uniqueness result for the proposed SDE model with weighted personal best and smoothed Heaviside functions. Note that the structure of the weighted personal best suggests the idea of introducing new variables for the numerator and the denominator. This re-formulation converts the non-Markovian process into a Markovian system for times t>0, but violates the initial condition. We therefore use different proofs for properties of SDEs with local Lipschitz conditions as well as path-dependent SDEs that can be found in the literature [23]. To the authors' knowledge none of them covers the case of path-dependent SDEs with local Lipschitz conditions. In the following we present a proof which combines the two techniques to obtain a well-posedness result.
We assume that the regularized Heaviside function Hϵ satisfies the following conditions:
(A1) Let 0≤Hϵ(x)≤1 for all x∈R.
(A2) There exists a constant C>0 such that
|Hϵ(x)−Hϵ(y)|≤Cϵ|x−y| for all x,y∈R. | (3.1) |
This corresponds to the following regularized problem
dX(t)=[−λϵ(t,X)(X(t)−vf)−μϵ(t,X)(X(t)−pif)]dt+√2σdiag(X(t)−vf)dBt, | (3.2a) |
with
λϵ(t,X)=diag(Hϵ(f(Xi(t))−f(vf))Hϵ(f(pif)−f(vf)))i=1,…,N∈RdN×dN, | (3.2b) |
μϵ(t,X)=diag(Hϵ(f(Xi(t))−f(pif))Hϵ(f(vf)−f(pif)))i=1,…,N∈RdN×dN. | (3.2c) |
Moreover, we assume that the objective function f satisfies the following properties:
(A3) Positivity: it holds 0≤f(x) for all x∈Rd,
(A4) Quasi-local Lipschitz condition: for any n<∞ and |x|,|y|≤n it holds
|f(x)−f(y)|≤Lf|x−y|, |
with a constant Lf>0 depending on n only.
Remark 2. The well-known regularization of the Heaviside function
Hϵ(x)=12+12tanh(xϵ) |
satisfies the assumptions (A1) and (A2). Note that in the context of optimization problems, the positivity assumption on f is not too restrictive. Since f corresponds to a minimization functional it is naturally bounded from below and can be shifted to satisfy the positivity constraint.
The following proof is based on a combination of arguments of Theorem 3.17 and Theorem 3.27 in [23] - this yields well-posedness of (3.2). We begin with two lemmata providing necessary estimates. The first lemma is concerned with properties of the weighed averages. Note that the global best vf depends on the current state of the process and the personal best pf on the whole trajectory. We therefore write vf[φ(t)]=vf(t) and pf[φ]=pf.
Lemma 1. Let f satisfy (A3) and (A4), N∈N and φ=(φ1,…φN)∈C(R+,RdN). Then
vf[φ(t)]∈Rd,|vf[φ(t)]|≤|φ(t)|for everyt,pf[φ]∈C(R+,RdN),|pf[φ](t)|≤|φ|t. | (3.3) |
Moreover, the averages satisfy the local Lipschitz conditions:
|pf[φ](t)−pf[ˆφ](t)|2=N∑i=1|pif[φ](t)−pif[ˆφ](t)|2≤C1‖φ−ˆφ‖2t, | (3.4) |
|vf[φ(t)]−vf[ˆφ(t)]|2≤C2|φ(t)−ˆφ(t)|2 | (3.5) |
for all t∈[0,∞) with |φ|t,|ˆφ|t≤n with constants
C1=(1+(1+2Lf)βneβ(¯f−f_)),andC2=(1+αnLfe−αf_N+neα(¯f−f_)(1N+αnLf))22N−1. | (3.6) |
Here Lf is the Lipschitz constant of f in Bn={x:|x|≤n} and f_,¯f correspond, respectively, to the minimal and maximal values of f on Bn.
The proof of Lemma 1 can be found in the Appendix. Using Lemma 1 we show that the drift and diffusion terms satisfy local Lipschitz and linear growth conditions. These properties allow us to apply the existence and uniqueness result later on.
Lemma 2. Let (A1)–(A4) hold. Then
b:[0,+∞)×C(R+,RdN)→RdNandΣ:[0,+∞)×C(R+,RdN)→RdN×dN |
given by
b(t,φ)=−λϵ(t,φ)(φ(t)−vf)−μϵ(t,φ)(φ(t)−pf) |
and
Σ(t,φ)=diag((φi(t)−vf)i=1,…,N)∈RdN×dN |
with φi=(φ(i−1)d+1,…,φ(i−1)d+d) satisfy the following conditions for all φ,ψ∈C(R+,RdN) and all R>0:
(i) |b(t,φ)−b(t,ψ)|≤LR‖φ−ψ‖t, (iii) |Σ(t,φ(t))−Σ(t,ψ(t))|≤ℓR|φ(t)−ψ(t)|,
(ii) |b(t,φ)|≤aR‖φ‖t, (iv) |Σ(t,φ(t))|≤bR|φ(t)|,
where LR,ℓR,aR,bR∈R.
Proof. To show (ⅰ) we calculate
|bi(t,φ)−bi(t,ψ)|2≤2(I1+I2+I3+I4), | (3.7) |
where
I1:=|(λϵ,i(t,φ)−λϵ,i((t,ψ))(φi(t)−vf[φ(t)])|2≤12ϵLf(|φi(t)|+|vf[φ(t)]|)(2|φi(t)−ψi(t)|2+8|vf[ψ(t)]−vf[φ(t)]|2+4|pif[φ](t)−pif[ψ](t)|2),I2:=|λϵ,i((t,ψ)(|φi(t)−ψi(t)|+|vf[ψ(t)]−vf[φ(t)]|)|2≤2|φi(t)−ψi(t)|2+2|vf[ψ(t)]−vf[φ(t)|2,I3:=|(μϵ,i((t,φ)−μϵ,i((t,ψ))(φi(t)−pif[φ](t))|2≤12ϵLf(|φ(t)−pif[φ](t)|)(2|φi(t)−ψi(t)|2+4|vf[ψ(t)]−vf[φ(t)]|2+8|pif[φ](t)−pif[ψ](t)|2),I4:=|μϵ,i((t,ψ)(φi(t)−ψi(t)+pif[ψ](t)−pif[φ](t))|2≤2|φi(t)−ψi(t)|2+2|pif[φ](t)−pif[ψ](t)|2. |
From Lemma 1 we know that |vf[ψ(t)]−vf[φ(t)]|2≤C1|φ(t)−ψ(t)|2, and |pif[φ](t)−pif[ψ](t)|2≤C2‖φi−ψi‖2t with constants C1 and C2 given by (3.6) with n=R. This yields (ⅰ) since
|b(t,φ)−b(t,ψ)|=(N∑i=1|bi(t,φ)−bi(t,ψ)|)1/2≤LR‖φ−ψ‖t. |
The Lipschitz bound (ⅲ) follows from similar arguments using the diagonal structure of Σ:
|Σ(t,φ(t))−Σ(t,ψ(t))|=(N∑i=1|Σii(t,φ(t))−Σii(t,ψ(t))|2)1/2≤(N∑i=12|φi(t)−ψi(t)|2+2|vf[φ(t)]−vf[ψ(t)]|2)1/2≤ℓR|φ(t)−ψ(t)|. |
The last two inequalities hold due to
|b(t,φ)|=(N∑i=1bi(t,φ)2)1/2≤(N∑i=18|φi(t)|2+4|vf[φ(t)]|2+2|pif[φ](t)|2)1/2≤aR‖φ‖t,|Σ(t,φ)|=(N∑i=1Σii(t,φ)2)1/2≤(N∑i=12φi(t)2+2|vf[φ(t)]|2)1/2≤bR|φ(t)|. |
Equipped with this lemma, we have everything at hand to prove the main theorem.
Theorem 2. Let (A1)–(A4) be satisfied and ξ∈L0(Ω,F0,P,RdN) with E|ξ|p<∞ for each p>0. Then, there exists a unique strong global solution to (3.2). Moreover, there exists a constant Cp,T,Lr,ℓR such that
Esupt∈[0,T]|X(t)|p≤Cp,T,Lr,ℓRE|ξ|p. |
The proof combines arguments of Theorem 3.17 (path-dependent SDE) and Theorem 3.27 (SDE with local Lipschitz coefficients) in [23].
Proof. We start by proving uniqueness. Let X,ˆX∈S0dN be two solutions to (3.2) corresponding to initial data ξ,ˆξ∈L0(Ω,F0,P,RdN), respectively. Then it holds Eξ=Eˆξ. Define the stopping time τn(ω)=inf{t≥0:|Xt(ω)|+|ˆXt(ω)|≥n}. Then the two solutions satisfy
Xt∧τn=ξ+∫t01[0,τn](s)b(s∧τn,X)ds+∫t01[0,τn](s)σ(s∧τn,Xs∧τn)dBs,ˆXt∧τn=ˆξ+∫t01[0,τn](s)b(s∧τn,ˆX)ds+∫t01[0,τn](s)σ(s∧τn,ˆXs∧τn)dBs, |
and τn→∞ for n→∞. Note that we have global Lipschitz constants for b and σ for all times t∈[0,τn] with n arbitrary but fixed. This allows us to use Theorem 3.8 in [23], see proof of Theorem 3.27 in [23] for more details, to obtain
E‖e−VR(X⋅∧τn−ˆX⋅∧τn)‖pT(1+‖e−VR(X⋅∧τn−ˆX⋅∧τn)‖2T)p/2≤CpE|X0−ˆX0|p(1+|X0−ˆX0|2)p/2, |
for some VR depending on the local Lipschitz constants LR,ℓR,aR,bR and p≥2 arbitrary. Hence, the uniqueness of the solution on [0,τn]. As τn→∞ for n→∞, this allows us to conclude the global uniqueness of X∈S0dN.
Next, we show the existence of solutions. Let M∈N∗ and 0=T0<T1<⋯<TM=τn with Ti=iτnM. It holds
α(τnM):=sup0<s−t<τnM(∫stLRdr)p+(∫stℓ2Rdr)p/2⟶0 as M→∞. |
We employ a fixed point argument for the mapping Γ:SpdN[0,T1]→SpdN[0,T1] given by
Γ(U)t=ξ+∫t0b(s,U)ds+∫t0σ(s,Us)dBs, |
where we need no stopping times due to t<τn on [0,T1]. Indeed, the mapping Γ is well-defined since for all φ∈C(R+,RdN)
|b(t,φ)|≤LR‖φ‖t,and|σ(t,φ)|≤ℓR‖φ‖t, |
is satisfied. Because of the Lipschitz continuity, both stochastic processes b(⋅,U) and σ(⋅,Us) are progressively measurable for all U∈SpdN[0,τn] and b(⋅,U)∈Lp(Ω,L1(0,τn)) and σ(⋅,U)∈ΛpdN×dN(0,τn). Therefore,
∫∙0b(r,U)dr,∫∙0σ(r,Ur)dBr∈SpdN[0,τn]. |
We will show that the operator Γ is a strict contraction on the complete metric space SpdN[0,T1] for sufficiently large M (where SpdN[0,T1] is equipped with the usual distance dp,M(U,V)=(E‖U−V‖pT1)1/p∨1). Let U,V∈SpdN[0,T1]. By the Burkholder-Davis-Gundy inequality we have
E‖Γ(U)−Γ(V)‖pT1≤(1∨2p−1)Esups∈[T0,T1]|∫sT0b(r,U)−b(r,V)dr|p+(1∨2p−1)Esups∈[T0,T1]|∫sT0σ(r,Ur)σ(r,Vr)dBr|p≤(1∨2p−1)[E(∫T1T0LR‖U−V‖rdr)p+E(∫T1T0ℓ2R|Ur−Vr|dr)p/2]≤(1∨2p−1)α(τnM)E(‖U−V‖pT1). |
Let M0∈N∗ such that (1∨2p−1)α(τnM0)≤(12)1∨p. Then Γ is a strict contraction in SpdN[0,T1] and thus (3.2) has a unique solution X∈SpdN[0,T1]. We extend the solution to the interval [0,T2] by defining a mapping, again, called Γ:SpdN[0,T2]→SpdN[0,T2]:
Γ(U)t={Xt,if t∈[0,T1],XT1+∫tT1b(s,U)ds+∫tT1σ(s,Us)ds,if t∈(T1,T2]. |
We repeat the argument M0 times to be valid the whole interval [0,τn]. Since τn→∞ almost surely, the uniqueness of the solution implies that
[Xn+1t(ω)−Xnt(ω)]1[0,τn(ω)](t)1[0,∞)(τn(ω))=0. |
Hence, the process X∈S0d is defined by Xt(ω)=Xnt(ω) if 0≤t≤τn(ω) and τn(ω)>0 is the unique solution to the regularized problem (3.2).
The numerical simulations are based on the direct simulation of system (2.5) using the Euler-Maruyama scheme, in which we do not approximate the Heaviside function H. Note that the smoothing of H was only needed for analytic considerations. In practise, we want only one of the drift terms to effect the particles dynamics, which is why we have not pursued this option any further. The final time is set to T=15, discretized into 3×104 time steps. All presented results are averaged over M=5000 realizations. The standard deviation is set to σ=0.5, while the number of agents depends on the dimension of the function space. In particular, we set the number of agents to 3,5 or 10 times the space dimension. The initial positions of particles are drawn from a uniform distribution within a specific domain for each function. The parameters α and β to compute the global and personal best are set to
α=10 and β=10. |
A realization is successful if the average mean is close to the function minimum fmin, in particular
|f(vf(T))−f(xmin)|<0.1. |
We compare the performance of the CBO scheme with μ=0, PB and wPB for the following benchmark problems:
1. Alpine [26]: This non-convex differentiable function has a global minimum at xmin=(0,…,0)
f(x)=d∑i=1|xisin(xi)+0.1xi|. | (4.1) |
2. Ackley [27]: This function is continuous, non-differentiable and non-convex and has its global minimum at xmin=(0,…,0).
f(x)=−20exp(0.2√1d|x|2)−exp(1dd∑i=1cos(2πxi))+20+exp(1). | (4.2) |
3. Rastrigin [28]: The Rastrigin function is continuous, differentiable and convex, has lots of local minima and a global minimum at xmin=(0,…0).
f(x)=10d+d∑i=1(x2i−10cos(2πxi)). | (4.3) |
4. Xinsheyang2: [26] This function is continuous but not differentiable and non-convex with a global minimum at xmin=(0,…0).
f(x)=d∑i=1|xi|exp(−d∑i=1sin(x2i)). | (4.4) |
The choice of these functions is based on the different characteristics they have, see Figure 5 for plots in 2D. Table 3 shows the results for the Alpine function (4.1) and the Ackley function (4.2). We observe that the success rate increases with the number of particles, and decreases for higher space dimension. Weighted personal best and personal best give comparable results, which is not surprising since wPB approximates PB for large values of β. A similar behavior can be seen in the case of the Rastrigin function (4.3) and the Xinsheyang function (4.4) in Table 4.
d | # par. | CBO | PB | wPB | d | # par. | CBO | PB | wPB | |
1 | 3 | 0.8372 | 0.7184 | 0.8456 | 1 | 3 | 0.8138 | 0.8164 | 0.8144 | |
1 | 5 | 0.9626 | 0.8424 | 0.968 | 1 | 5 | 0.9598 | 0.9602 | 0.9598 | |
1 | 10 | 0.9986 | 0.9292 | 0.999 | 1 | 10 | 0.9986 | 0.999 | 0.9986 | |
3 | 9 | 0.4868 | 0.4904 | 0.485 | 3 | 9 | 0.902 | 0.917 | 0.9008 | |
3 | 15 | 0.6294 | 0.6754 | 0.6458 | 3 | 15 | 0.9908 | 0.9934 | 0.99 | |
3 | 30 | 0.847 | 0.8858 | 0.8404 | 3 | 30 | 1 | 1 | 1 | |
5 | 15 | 0.3246 | 0.3266 | 0.3194 | 5 | 15 | 0.9886 | 0.9928 | 0.9908 | |
5 | 25 | 0.4206 | 0.4352 | 0.4226 | 5 | 25 | 0.9996 | 1 | 0.9998 | |
5 | 50 | 0.5748 | 0.6092 | 0.5778 | 5 | 50 | 1 | 1 | 1 |
d | # par. | CBO | PB | wPB | d | # par. | CBO | PB | wPB | |
1 | 3 | 0.7924 | 0.7992 | 0.8006 | 1 | 3 | 0.8732 | 0.8914 | 0.8762 | |
1 | 5 | 0.9528 | 0.953 | 0.9522 | 1 | 5 | 0.9786 | 0.9892 | 0.9802 | |
1 | 10 | 0.9984 | 0.999 | 0.9988 | 1 | 10 | 0.9996 | 1 | 0.9998 | |
3 | 9 | 0.4634 | 0.4726 | 0.4624 | 3 | 9 | 0.8538 | 0.8634 | 0.8548 | |
3 | 15 | 0.6844 | 0.7112 | 0.6914 | 3 | 15 | 0.9268 | 0.9412 | 0.9298 | |
3 | 30 | 0.9138 | 0.9278 | 0.9204 | 3 | 30 | 0.9766 | 0.984 | 0.9792 | |
5 | 15 | 0.5012 | 0.4944 | 0.5 | 5 | 15 | 0.481 | 0.45 | 0.4742 | |
5 | 25 | 0.7074 | 0.7296 | 0.7144 | 5 | 25 | 0.4996 | 0.4724 | 0.498 | |
5 | 50 | 0.908 | 0.9172 | 0.9106 | 5 | 50 | 0.5318 | 0.4918 | 0.5216 |
We conclude by investigating a 2D version of the toy problem considered in Section 2.3:
f(x1,x2)=(x21−1)2+0.01x1+0.5+x22. |
This function has a global minimum at xmin=(−1.00125,0) and a local minimum at (0.998748,0). We wish to explore the dynamics of this 2D version for different number of particles. In doing so we consider 4, 8 or 16 particles and start the particle schemes with 2, 4 and 8 placed in each of the two wells with a random perturbation. Furthermore we set α=10 and β=20. Table 5 shows the results as the number of particles increases. We observe that CBO and (w)PB perform equally well for large numbers of particles, and that (w)PB outperform CBO for few particles. This could be explained by the fact that the probability of all particles deviating from the global minimum decreases as their number increases.
d | # par. | CBO | PB | wPB |
2 | 4 | 0.6572 | 0.8212 | 0.7596 |
2 | 8 | 0.7168 | 0.78 | 0.7684 |
2 | 16 | 0.7768 | 0.7684 | 0.7736 |
In this paper we introduced a consensus based global optimization scheme, which includes the personal best information of each particle. The proposed generalization is motivated by the original works on particle swarm algorithms, in which particles adjust their position as a linear combination of moving towards the current global best and their personal best value.
We discussed how information about the personal best can be included in consensus based optimization schemes, leading to a system of functional stochastic differential equations. A well-posedness result for the respective regularized non-Markovian SDEs was presented. New features of the algorithm with personal best were illustrated and compared in computational experiments. The numerical results indicate that information about the personal best leads to higher success rates in the case of few particles and that the corresponding weighted means are better approximations of the global function minima.
The authors would like to thank Christoph Belak (TU Berlin), Stefan Grosskinsky (University of Warwick), Greg Pavliotis (Imperial College London) and Oliver Tse (TU Eindhoven) for the helpful discussions and constructive input. CT was partly supported by the European Social Fund and by the Ministry Of Science, Research and the Arts Baden-Württemberg. MTW was partly supported by the New Frontier's grant NST-0001 of the Austrian Academy of Sciences ÖAW.
All authors declare no conflicts of interest in this paper.
Proof. [Theorem 1] In the following we denote in abuse of notation by vf[X] the vector (vf,…,vf)∈RdN. We rewrite (2.5) with λ(Xi(t),vf,pif)≡λ,μ(Xi(t),vf,pif)≡0 as
dX(t)=−λ(X(t)−vf[X(t)])dt+√2σdiag(X(t)−vf[X(t)])dBt |
with diag(X(t)−vf[X(t)])∈RdN×dN being the diagonal matrix with dk=diag(Xk(t)−vf)∈Rd×d for k=1,…,N and dBt a dN-dimensional Brownian motion. The argument follows the lines of the well-posedness in [7]. In fact, let M[X(t)]=diag(X(t)−vf[X(t)]) and n∈N arbitrary. We have to check that there exists a constant Cn such that
−2λX(t)⋅(X(t)−vf[X(t)])+2σ2trace(M[X(t)]M[X(t)]T)≤Cn|X(t)|2, | (5.1) |
for every |X(t)|≤n. Note that f(X(t)) is bounded for |X(t)|≤n due to its local Lipschitz continuity. Hence, the estimate for the first term on the left-hand side is identical to the one in [7]. Indeed, we have
−2λX(t)⋅(X(t)−vf[X(t)])≤2λ√N|X(t)|2. |
For the component-wise drift we obtain
2σ2trace(M[X(t)]M[X(t)]T)=2σ2N∑j=1d∑k=1[(Xj(t)−vf)k]2≤4σ2(1+N)|X(t)|2. |
Combining the two preceding estimates we obtain Eq (5.1). Now, employing [29,Ch 5.3,Thm 3.2] yields the desired result.
[Lemma 1] We start by showing continuity. For pf[φ] we need to check continuity as t→0. In fact, l'Hospital's rule yields
limt→0∫t0φi(s)e−βf(φi(s))ds∫t0e−βf(φi(s))ds=limt→0φi(t)e−βf(φi(t))e−βf(φi(t))=limt→0φi(t)=φi0. |
This directly implies the continuity of the vector pf[φ]. Moreover, it is easy to see that
|vf[φ(t)]|2=|∑Ni=1φi(t)e−αf(φi(t))∑Ni=1e−αf(φi(t))|2≤|φ(t)|2,|pf[φ](t)|2=N∑i=1|pif[φ](t)|2=N∑i=1|∫t0φi(s)e−βf(φi(s))ds∫t0e−βf(φi(s))ds|2≤‖φ‖2t. |
We are left to show the local Lipschitz continuity. Therefore, we estimate
|pif[φ](t)−pif[ˆφ](t)|≤|∫t0(φi(s)−ˆφi(s))e−βf(φi(s))ds∫t0e−βf(φi(s))ds|+|∫t0ˆφi(s)(e−βf(φi(s))−e−βf(ˆφi(s)))ds∫t0e−βf(φi(s))ds|+|∫t0ˆφi(s)e−βf(ˆφi(s))ds(∫t0ˆφi(s)e−βf(ˆφi(s))ds−∫t0φi(s)e−βf(φi(s))ds)∫t0e−βf(φi(s))ds∫t0e−βf(ˆφi(s))ds|=:I1+I2+I3, |
to obtain
I1≤‖φi−ˆφi‖t,I2≤βeβ(¯f−f_)Lfn‖φi−ˆφi‖t, and I3≤βeβ(¯f−f_)(1+Lfn)‖ˆφi‖t‖ˆφi−φi‖t, |
with Lf being the global Lipschitz constant of f on Bn={x∈Rd:|x|≤n} and f_,¯f are the minimal and maximal value of f on Bn, respectively. Altogether, this yields the estimate
|pif[φ](t)−pif[ˆφ](t)|≤(1+(1+2Lf)βneβ(¯f−f_))‖ˆφi−φi‖t. |
For the vectors pf(t)=(pif[φ](t))i=1,…,N and ˆpf(t)=(ˆpif[φ](t))i=1,…,N this implies
|pf(t)−ˆpf(t)|2=N∑i=1|pif[φ](t)−pif[ˆφ](t)|2≤(1+(1+2Lf)βneβ(¯f−f_))‖φ−ˆφ‖2t. |
Similarly, we have
|vf[φ(t)]−vf[ˆφ(t)]|≤|∑Ni=1(φi(t)−ˆφi(t))e−αf(φi(t))∑Ni=1e−αf(φi(t))|+|∑Ni=1ˆφi(t)(e−αf(φi(t))−e−αf(ˆφi(t)))∑Ni=1e−αf(φi(t))|+|∑Ni=1ˆφi(t)e−αf(ˆφi(t))(∑Ni=1ˆφi(t)e−αf(ˆφi(t))−∑Ni=1φi(t)e−αf(φi(t)))(∑Ni=1e−αf(φi(t)))(∑Ni=1e−αf(ˆφi(t)))|=:J1+J2+J3, |
which satisfy
J1≤|φ(t)−ˆφ(t)|1,J2≤αnLfe−αf_N|φ(t)−ˆφ(t)|1, and J3≤neα(¯f−f_)(1N+αnLf)|ˆφ(t)−φ(t)|1. |
Thus, we get
|vf[φ(t)]−vf[ˆφ(t)]|≤(1+αnLfe−αf_N+neα(¯f−f_)(1N+αnLf))|ˆφ(t)−φ(t)|1. |
Taking squares leads to the estimate
|vf[φ(t)]−vf[ˆφ(t)]|2≤(1+αnLfe−αf_N+neα(¯f−f_)(1N+αnLf))22N−1|ˆφ(t)−φ(t)|2. |
[1] |
B. Jankovic, K. T. Le, J. M. Hershman, Hashimoto's thyroiditis and papillary thyroid carcinoma: is there a correlation?, J. Clin. Endocrinol. Metab., 98 (2013), 474-482. doi: 10.1210/jc.2012-2978
![]() |
[2] |
D. S. McLeod, A. M. Sawka, D. S. Cooper, Controversies in primary treatment of low-risk papillary thyroid cancer, The Lancet, 381 (2013), 1046-1057. doi: 10.1016/S0140-6736(12)62205-3
![]() |
[3] |
A. Cummings, M. Goldfarb, Thyroid carcinoma metastases to axillary lymph nodes: report of two rare cases of papillary and medullary thyroid carcinoma and literature review, Endocr. Pract., 20 (2014), e34-e37. doi: 10.4158/EP13339.CR
![]() |
[4] |
G. H. Sakorafas, D. Sampanis, M. Safioleas, Cervical lymph node dissection in papillary thyroid cancer: current trends, persisting controversies, and unclarified uncertainties, Surg. Oncol., 19 (2010), e57-e70. doi: 10.1016/j.suronc.2009.04.002
![]() |
[5] |
L. L. Chen, The biogenesis and emerging roles of circular RNAs, Nat. Rev. Mol. Cell Bio., 17 (2016), 205-211. doi: 10.1038/nrm.2015.32
![]() |
[6] |
H. L. Sanger, G. Klotz, D. Riesner, H. J. Gross, A. K. Kleinschmidt, Viroids are single-stranded covalently closed circular RNA molecules existing as highly base-paired rod-like structures, Proc. Natl. Acad. Sci., 73 (1976), 3852-3856. doi: 10.1073/pnas.73.11.3852
![]() |
[7] | J. Li, J. Yang, P. Zhou, Y. Le, C. Zhou, S. Wang, et al., Circular RNAs in cancer: novel insights into origins, properties, functions and implications, Am. J. Cancer Res., 5 (2015), 472-480. |
[8] |
Y. Zhang, X. O. Zhang, T. Chen, J. F. Xiang, Q. F. Yin, Y. H. Xing, et al., Circular intronic long noncoding RNAs, Mol. Cell, 51 (2013), 792-806. doi: 10.1016/j.molcel.2013.08.017
![]() |
[9] |
W. R. Jeck, N. E. Sharpless, Detecting and characterizing circular RNAs, Nat. Biotechnol., 32 (2014), 453-461. doi: 10.1038/nbt.2890
![]() |
[10] |
Z. Zhong, M. Huang, M. Lv, Y. He, C. Duan, L. Zhang, et al., Circular RNA MYLK as a competing endogenous RNA promotes bladder cancer progression through modulating VEGFA/VEGFR2 signaling pathway, Cancer Lett., 403 (2017), 305-317. doi: 10.1016/j.canlet.2017.06.027
![]() |
[11] |
L. Lü, J. Sun, P. Shi, W. Kong, K. Xu, B. He, et al., Identification of circular RNAs as a promising new class of diagnostic biomarkers for human breast cancer, Oncotarget, 8 (2017), 44096-44107. doi: 10.18632/oncotarget.17307
![]() |
[12] |
K. Y. Hsiao, Y. C. Lin, S. K. Gupta, N. Chang, L. Yen, H. S. Sun, et al., Noncoding effects of circular RNA CCDC66 promote colon cancer growth and metastasis, Cancer Res., 77 (2017), 2339-2350. doi: 10.1158/0008-5472.CAN-16-1883
![]() |
[13] |
D. Han, J. Li, H. Wang, X. Su, J. Hou, Y. Gu, et al., Circular RNA circMTO1 acts as the sponge of microRNA-9 to suppress hepatocellular carcinoma progression, Hepatology, 66 (2017), 1151-1164. doi: 10.1002/hep.29270
![]() |
[14] | G. K. Smyth, LIMMA: linear models for microarray data, in Bioinformatics and Computational Biology Solutions Using R and Bioconductor, Spring, (2005), 397-420. |
[15] |
M. Ashburner, C. A. Ball, J. A. Blake, D. Botstein, H. Butler, J. M. Cherry, et al., Gene ontology: tool for the unification of biology, Nat. Genet., 25 (2000), 25-29. doi: 10.1038/75556
![]() |
[16] |
M. Kanehisa, S. Goto, KEGG: kyoto encyclopedia of genes and genomes, Nucleic Acids Res., 28 (2000), 27-30. doi: 10.1093/nar/28.1.27
![]() |
[17] |
D. Szklarczyk, A. Franceschini, S. Wyder, K. Forslund, D. Heller, J. Huerta-Cepas, et al., STRING v10: protein–protein interaction networks, integrated over the tree of life, Nucleic Acids Res., 43 (2015), D447-D452. doi: 10.1093/nar/gku1003
![]() |
[18] |
P. Shannon, A. Markiel, O. Ozier, N. S. Baliga, J. T. Wang, D. Ramage, et al., Cytoscape: a software environment for integrated models of biomolecular interaction networks, Genome Research, 13 (2003), 2498-2504. doi: 10.1101/gr.1239303
![]() |
[19] |
W. P. Bandettini, P. Kellman, C. Mancini, O. J. Booker, S. Vasu, S. W. Leung, et al., MultiContrast delayed enhancement (MCODE) improves detection of subendocardial myocardial infarction by late gadolinium enhancement cardiovascular magnetic resonance: a clinical validation study, J. Cardiovasc. Magn. Reson., 14 (2012), 83. doi: 10.1186/1532-429X-14-83
![]() |
[20] |
H. D. Zhang, L. H. Jiang, D. W. Sun, J. C. Hou, Z. L. Ji, et al., CircRNA: a novel type of biomarker for cancer, Breast Cancer, 25 (2018), 1-7. doi: 10.1007/s12282-017-0793-9
![]() |
[21] |
G. Wu, W. Zhou, X. Pan, Z. Sun, Y. Sun, H. Xu, et al., Circular RNA profiling reveals exosomal circ_0006156 as a novel biomarker in papillary thyroid cancer, Mol. Ther. Nucleic. Acids, 19 (2020), 1134-1144. doi: 10.1016/j.omtn.2019.12.025
![]() |
[22] |
Q. Liu, L. Z. Pan, M. Hu, J. Y. Ma, Molecular network-based identification of circular RNA-associated cerna network in papillary thyroid cancer, Pathol. Oncol. Res., 26 (2020), 1293-1299. doi: 10.1007/s12253-019-00697-y
![]() |
[23] |
S. Borran, G. Ahmadi, S. Rezaei, M. M. Anari, M. Modabberi, Z. Azarash, et al., Circular RNAs: new players in thyroid cancer, Pathol. Res. Pract., 216 (2020), 153217. doi: 10.1016/j.prp.2020.153217
![]() |
[24] |
X. Li, J. Ding, X. Wang, Z. Cheng, Q. Zhu, NUDT21 regulates circRNA cyclization and ceRNA crosstalk in hepatocellular carcinoma, Oncogene, 39 (2020), 891-904. doi: 10.1038/s41388-019-1030-0
![]() |
[25] |
P. F. Tussy, I. R. Maldonado, C. F. Hernando, MicroRNAs and circular RNAs in lipoprotein metabolism, Curr. Atheroscler. Rep., 23 (2021), 33. doi: 10.1007/s11883-021-00934-3
![]() |
[26] |
G. Yu, Z. Yang, T. Peng, Y. Lv, Circular RNAs: rising stars in lipid metabolism and lipid disorders, J. Cell Physiol., 236 (2021), 4797-4806. doi: 10.1002/jcp.30200
![]() |
[27] |
L. Wang, Z. Zheng, X. Feng, X. Zang, W. Ding, F. Wu, et al., circRNA/lncRNA-miRNA-mRNA network in oxidized, low-density, lipoprotein-induced foam cells, DNA Cell Biol., 38 (2019), 1499-1511. doi: 10.1089/dna.2019.4865
![]() |
[28] | H. Y. Zhang, C. H. Li, X. C. Wang, Y. Q.Luo, X. D. Cao, J. J. Chen, MiR-199 inhibits EMT and invasion of hepatoma cells through inhibition of Snail expression, Eur. Rev. Med. Pharmacol. Sci., 23 (2019), 7884-7891. |
[29] |
K. Koshizuka, T. Hanazawa, N. Kikkawa, T. Arai, A. Okato, A. Kurozumi, et al., Regulation of ITGA3 by the anti-tumor miR-199 family inhibits cancer cell migration and invasion in head and neck cancer, Cancer Sci., 108 (2017), 1681-1692. doi: 10.1111/cas.13298
![]() |
[30] |
T. B. Hansen, J. Kjems, C. K. Damgaard, Circular RNA and miR-7 in cancer, Cancer Res., 73 (2013), 5609-5612. doi: 10.1158/0008-5472.CAN-13-1568
![]() |
[31] |
H. Xiao, MiR-7-5p suppresses tumor metastasis of non-small cell lung cancer by targeting NOVA2, Cell Mol. Biol. Lett., 24 (2019), 60. doi: 10.1186/s11658-019-0188-3
![]() |
[32] |
K. Zeng, X. Chen, M. Xu, X. Liu, X. Hu, T. Xu, et al., CircHIPK3 promotes colorectal cancer growth and metastasis by sponging miR-7, Cell Death Dis., 9 (2018), 417. doi: 10.1038/s41419-018-0454-8
![]() |
[33] |
T. Hong, J. Ding, W. Li, miR-7 reverses breast cancer resistance to chemotherapy by targeting MRP1 and BCL2, Onco. Targets Ther., 12 (2019), 11097-11105. doi: 10.2147/OTT.S213780
![]() |
[34] |
J. Xia, T. Cao, C. Ma, Y. Shi, Y. Sun, Z. P. Wang, et al., MiR-7 suppresses tumor progression by directly targeting MAP3K9 in pancreatic cancer, Mol. Ther. Nucleic Acids, 13 (2018), 121-132. doi: 10.1016/j.omtn.2018.08.012
![]() |
[35] |
W. Shi, J. Song, Z. Gao, X. Liu, W. Wang, Downregulation of miR-7-5p Inhibits the Tumorigenesis of esophagus cancer via Targeting KLF4, Onco. Targets Ther., 13 (2020), 9443-9453. doi: 10.2147/OTT.S251508
![]() |
[36] | Y. Duan, Y. Zhang, W. Peng, P. Jiang, Z. Deng, C. Wu, MiR-7-5pand miR-451 as diagnostic biomarkers for papillary thyroid carcinoma in formalin-fixed paraffin-embedded tissues, Pharmazie, 75 (2020), 266-270. |
[37] | J. Y. Han, S. Guo, N. Wei, R. Xue, W. Li, G. Dong, et al, CiRS-7 promotes the proliferation and migration of papillary thyroid cancer by negatively regulating the miR-7/epidermal growth factor receptor axis, Biomed Res. Int., 2020 (2020), 9875636. |
[38] |
K. Honda, V. A. Katzke, A. Hüsing, S. Okaya, H. Shoji, K. Onidani, et al., CA19-9 and apolipoprotein-A2 isoforms as detection markers for pancreatic cancer: a prospective evaluation, Int. J. Cancer, 144 (2019), 1877-1887. doi: 10.1002/ijc.31900
![]() |
[39] |
Y. Sato, T. Kobayashi, S. Nishiumi, A. Okada, T. Fujita, T. Sanuki, et al., Prospective study using plasma apolipoprotein a2-isoforms to screen for high-risk status of pancreatic cancer, Cancers (Basel), 12 (2020), 2625. doi: 10.3390/cancers12092625
![]() |
[40] |
W. Zeng, H. Chang, M. Ma, Y. Li, CCL20/CCR6 promotes the invasion and migration of thyroid cancer cells via NF-kappa B signaling-induced MMP-3 production, Exp Mol. Pathol., 97 (2014), 184-190. doi: 10.1016/j.yexmp.2014.06.012
![]() |
[41] | C. Y. Wu, C. Zheng, E. J. Xia, R. Quan, J. Hu, X. Zhang, et al., Lysophosphatidic acid receptor 5 (LPAR5) plays a significance role in papillary thyroid cancer via phosphatidylinositol 3-Kinase/Akt/Mammalian target of rapamycin (mTOR) pathway, Med. Sci. Monit., 26 (2020), e919820. |
[42] |
W. J. Zhao, L. L. Zhu, W. Q. Yang, S. J. Xu, J. Chen, X. F. Ding, et al., LPAR5 promotes thyroid carcinoma cell proliferation and migration by activating class IA PI3K catalytic subunit p110β, Cancer Sci., 112 (2021), 1624-1632. doi: 10.1111/cas.14837
![]() |
[43] | L. Yu, R. Hu, C. Sullivan, R J. Swanson, S. Oehninger, Y. P. Sun, et al., MFGE8 regulates TGF-β-induced epithelial mesenchymal transition in endometrial epithelial cells in vitro, Reproduction, 152 (2016), 225-233. |
[44] |
B. Wang, Z. Ge, Y. Wu, Y. Zha, X. Zhang, Y. Yan, et al., MFGE8 is down-regulated in cardiac fibrosis and attenuates endothelial-mesenchymal transition through Smad2/3-Snail signalling pathway, J. Cell. Mol. Med., 24 (2020), 12799-12812. doi: 10.1111/jcmm.15871
![]() |
[45] |
H. W. Jackson, V. Defamie, P. Waterhouse, R. Khokha, TIMPs: versatile extracellular regulators in cancer, Nat. Rev. Cancer, 17 (2017), 38-53. doi: 10.1038/nrc.2016.115
![]() |
[46] |
W. Q. Zhang, W. Sun, Y. Qin, C. Wu, L. He, T. Zhang, et al., Knockdown of KDM1A suppresses tumour migration and invasion by epigenetically regulating the TIMP1/MMP9 pathway in papillary thyroid cancer, J. Cell. Mol. Med., 23 (2019), 4933-4944. doi: 10.1111/jcmm.14311
![]() |
![]() |
![]() |
1. | Martin Burger, Lisa Maria Kreusser, Claudia Totzeck, Mean-field optimal control for biological pattern formation, 2021, 27, 1292-8119, 40, 10.1051/cocv/2021034 | |
2. | Alessandro Benfenati, Giacomo Borghi, Lorenzo Pareschi, Binary Interaction Methods for High Dimensional Global Optimization and Machine Learning, 2022, 86, 0095-4616, 10.1007/s00245-022-09836-5 | |
3. | Hui Huang, Jinniao Qiu, On the mean‐field limit for the consensus‐based optimization, 2022, 45, 0170-4214, 7814, 10.1002/mma.8279 | |
4. | Massimo Fornasier, Hui Huang, Lorenzo Pareschi, Philippe Sünnen, Anisotropic Diffusion in Consensus-Based Optimization on the Sphere, 2022, 32, 1052-6234, 1984, 10.1137/21M140941X | |
5. | Sara Grassi, Lorenzo Pareschi, From particle swarm optimization to consensus based optimization: Stochastic modeling and mean-field limit, 2021, 31, 0218-2025, 1625, 10.1142/S0218202521500342 | |
6. | Claudia Totzeck, 2022, Chapter 6, 978-3-030-93301-2, 201, 10.1007/978-3-030-93302-9_6 | |
7. | Konstantin Riedl, Leveraging memory effects and gradient information in consensus-based optimisation: On global convergence in mean-field law, 2024, 35, 0956-7925, 483, 10.1017/S0956792523000293 | |
8. | Leon Bungert, Tim Roith, Philipp Wacker, Polarized consensus-based dynamics for optimization and sampling, 2024, 0025-5610, 10.1007/s10107-024-02095-y | |
9. | Giacomo Borghi, Michael Herty, Lorenzo Pareschi, An Adaptive Consensus Based Method for Multi-objective Optimization with Uniform Pareto Front Approximation, 2023, 88, 0095-4616, 10.1007/s00245-023-10036-y | |
10. | Giacomo Albi, Federica Ferrarese, Claudia Totzeck, Kinetic-based optimization enhanced by genetic dynamics, 2023, 33, 0218-2025, 2905, 10.1142/S0218202523500641 | |
11. | Massimo Fornasier, Timo Klock, Konstantin Riedl, Consensus-Based Optimization Methods Converge Globally, 2024, 34, 1052-6234, 2973, 10.1137/22M1527805 | |
12. | Claudia Schillings, Claudia Totzeck, Philipp Wacker, Ensemble-Based Gradient Inference for Particle Methods in Optimization and Sampling, 2023, 11, 2166-2525, 757, 10.1137/22M1533281 | |
13. | Massimo Fornasier, Peter Richtárik, Konstantin Riedl, Lukang Sun, Consensus-based optimisation with truncated noise, 2024, 0956-7925, 1, 10.1017/S095679252400007X | |
14. | Hui Huang, Jinniao Qiu, Konstantin Riedl, On the Global Convergence of Particle Swarm Optimization Methods, 2023, 88, 0095-4616, 10.1007/s00245-023-09983-3 | |
15. | Hui Huang, Hicham Kouhkouh, Self-interacting CBO: Existence, uniqueness, and long-time convergence, 2025, 161, 08939659, 109372, 10.1016/j.aml.2024.109372 | |
16. | Kathrin Klamroth, Michael Stiglmayr, Claudia Totzeck, Consensus-based optimization for multi-objective problems: a multi-swarm approach, 2024, 89, 0925-5001, 745, 10.1007/s10898-024-01369-1 | |
17. | Stefano Almi, Marco Morandotti, Francesco Solombrino, Optimal control problems in transport dynamics with additive noise, 2023, 373, 00220396, 1, 10.1016/j.jde.2023.07.010 | |
18. | Massimo Fornasier, Lukang Sun, A PDE framework of consensus-based optimization for objectives with multiple global minimizers, 2025, 0360-5302, 1, 10.1080/03605302.2025.2459194 |
scheme | success rate | success rate |
α=10 | α=30 | |
CBO | 30 % | 60, 9% |
PB | 100 % | 100 % |
wPB | 100 % | 100 % |
scheme | success rate | success rate |
α=10 | α=30 | |
CBO | 91, 6 % | 98, 1 % |
PB | 100 % | 100 % |
wPB | 100 % | 100 % |
d | # par. | CBO | PB | wPB | d | # par. | CBO | PB | wPB | |
1 | 3 | 0.8372 | 0.7184 | 0.8456 | 1 | 3 | 0.8138 | 0.8164 | 0.8144 | |
1 | 5 | 0.9626 | 0.8424 | 0.968 | 1 | 5 | 0.9598 | 0.9602 | 0.9598 | |
1 | 10 | 0.9986 | 0.9292 | 0.999 | 1 | 10 | 0.9986 | 0.999 | 0.9986 | |
3 | 9 | 0.4868 | 0.4904 | 0.485 | 3 | 9 | 0.902 | 0.917 | 0.9008 | |
3 | 15 | 0.6294 | 0.6754 | 0.6458 | 3 | 15 | 0.9908 | 0.9934 | 0.99 | |
3 | 30 | 0.847 | 0.8858 | 0.8404 | 3 | 30 | 1 | 1 | 1 | |
5 | 15 | 0.3246 | 0.3266 | 0.3194 | 5 | 15 | 0.9886 | 0.9928 | 0.9908 | |
5 | 25 | 0.4206 | 0.4352 | 0.4226 | 5 | 25 | 0.9996 | 1 | 0.9998 | |
5 | 50 | 0.5748 | 0.6092 | 0.5778 | 5 | 50 | 1 | 1 | 1 |
d | # par. | CBO | PB | wPB | d | # par. | CBO | PB | wPB | |
1 | 3 | 0.7924 | 0.7992 | 0.8006 | 1 | 3 | 0.8732 | 0.8914 | 0.8762 | |
1 | 5 | 0.9528 | 0.953 | 0.9522 | 1 | 5 | 0.9786 | 0.9892 | 0.9802 | |
1 | 10 | 0.9984 | 0.999 | 0.9988 | 1 | 10 | 0.9996 | 1 | 0.9998 | |
3 | 9 | 0.4634 | 0.4726 | 0.4624 | 3 | 9 | 0.8538 | 0.8634 | 0.8548 | |
3 | 15 | 0.6844 | 0.7112 | 0.6914 | 3 | 15 | 0.9268 | 0.9412 | 0.9298 | |
3 | 30 | 0.9138 | 0.9278 | 0.9204 | 3 | 30 | 0.9766 | 0.984 | 0.9792 | |
5 | 15 | 0.5012 | 0.4944 | 0.5 | 5 | 15 | 0.481 | 0.45 | 0.4742 | |
5 | 25 | 0.7074 | 0.7296 | 0.7144 | 5 | 25 | 0.4996 | 0.4724 | 0.498 | |
5 | 50 | 0.908 | 0.9172 | 0.9106 | 5 | 50 | 0.5318 | 0.4918 | 0.5216 |
d | # par. | CBO | PB | wPB |
2 | 4 | 0.6572 | 0.8212 | 0.7596 |
2 | 8 | 0.7168 | 0.78 | 0.7684 |
2 | 16 | 0.7768 | 0.7684 | 0.7736 |
scheme | success rate | success rate |
α=10 | α=30 | |
CBO | 30 % | 60, 9% |
PB | 100 % | 100 % |
wPB | 100 % | 100 % |
scheme | success rate | success rate |
α=10 | α=30 | |
CBO | 91, 6 % | 98, 1 % |
PB | 100 % | 100 % |
wPB | 100 % | 100 % |
d | # par. | CBO | PB | wPB | d | # par. | CBO | PB | wPB | |
1 | 3 | 0.8372 | 0.7184 | 0.8456 | 1 | 3 | 0.8138 | 0.8164 | 0.8144 | |
1 | 5 | 0.9626 | 0.8424 | 0.968 | 1 | 5 | 0.9598 | 0.9602 | 0.9598 | |
1 | 10 | 0.9986 | 0.9292 | 0.999 | 1 | 10 | 0.9986 | 0.999 | 0.9986 | |
3 | 9 | 0.4868 | 0.4904 | 0.485 | 3 | 9 | 0.902 | 0.917 | 0.9008 | |
3 | 15 | 0.6294 | 0.6754 | 0.6458 | 3 | 15 | 0.9908 | 0.9934 | 0.99 | |
3 | 30 | 0.847 | 0.8858 | 0.8404 | 3 | 30 | 1 | 1 | 1 | |
5 | 15 | 0.3246 | 0.3266 | 0.3194 | 5 | 15 | 0.9886 | 0.9928 | 0.9908 | |
5 | 25 | 0.4206 | 0.4352 | 0.4226 | 5 | 25 | 0.9996 | 1 | 0.9998 | |
5 | 50 | 0.5748 | 0.6092 | 0.5778 | 5 | 50 | 1 | 1 | 1 |
d | # par. | CBO | PB | wPB | d | # par. | CBO | PB | wPB | |
1 | 3 | 0.7924 | 0.7992 | 0.8006 | 1 | 3 | 0.8732 | 0.8914 | 0.8762 | |
1 | 5 | 0.9528 | 0.953 | 0.9522 | 1 | 5 | 0.9786 | 0.9892 | 0.9802 | |
1 | 10 | 0.9984 | 0.999 | 0.9988 | 1 | 10 | 0.9996 | 1 | 0.9998 | |
3 | 9 | 0.4634 | 0.4726 | 0.4624 | 3 | 9 | 0.8538 | 0.8634 | 0.8548 | |
3 | 15 | 0.6844 | 0.7112 | 0.6914 | 3 | 15 | 0.9268 | 0.9412 | 0.9298 | |
3 | 30 | 0.9138 | 0.9278 | 0.9204 | 3 | 30 | 0.9766 | 0.984 | 0.9792 | |
5 | 15 | 0.5012 | 0.4944 | 0.5 | 5 | 15 | 0.481 | 0.45 | 0.4742 | |
5 | 25 | 0.7074 | 0.7296 | 0.7144 | 5 | 25 | 0.4996 | 0.4724 | 0.498 | |
5 | 50 | 0.908 | 0.9172 | 0.9106 | 5 | 50 | 0.5318 | 0.4918 | 0.5216 |
d | # par. | CBO | PB | wPB |
2 | 4 | 0.6572 | 0.8212 | 0.7596 |
2 | 8 | 0.7168 | 0.78 | 0.7684 |
2 | 16 | 0.7768 | 0.7684 | 0.7736 |