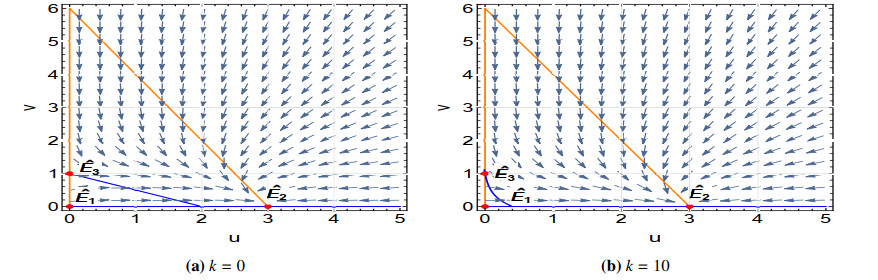
In this paper, we study the problem of optimal control of backward stochastic differential equations with three delays (discrete delay, moving-average delay and noisy memory). We establish the sufficient optimality condition for the stochastic system. We introduce two kinds of time-advanced stochastic differential equations as the adjoint equations, which involve the partial derivatives of the function f and its Malliavin derivatives. We also show that these two kinds of adjoint equations are equivalent. Finally, as applications, we discuss a linear-quadratic backward stochastic system and give an explicit optimal control. In particular, the stochastic differential equations with time delay are simulated by means of discretization techniques, and the effect of time delay on the optimal control result is explained.
Citation: Heping Ma, Hui Jian, Yu Shi. A sufficient maximum principle for backward stochastic systems with mixed delays[J]. Mathematical Biosciences and Engineering, 2023, 20(12): 21211-21228. doi: 10.3934/mbe.2023938
[1] | Xixia Ma, Rongsong Liu, Liming Cai . Stability of traveling wave solutions for a nonlocal Lotka-Volterra model. Mathematical Biosciences and Engineering, 2024, 21(1): 444-473. doi: 10.3934/mbe.2024020 |
[2] | Jinyu Wei, Bin Liu . Coexistence in a competition-diffusion-advection system with equal amount of total resources. Mathematical Biosciences and Engineering, 2021, 18(4): 3543-3558. doi: 10.3934/mbe.2021178 |
[3] | Jin Zhong, Yue Xia, Lijuan Chen, Fengde Chen . Dynamical analysis of a predator-prey system with fear-induced dispersal between patches. Mathematical Biosciences and Engineering, 2025, 22(5): 1159-1184. doi: 10.3934/mbe.2025042 |
[4] | Monika Joanna Piotrowska, Urszula Foryś, Marek Bodnar, Jan Poleszczuk . A simple model of carcinogenic mutations with time delay and diffusion. Mathematical Biosciences and Engineering, 2013, 10(3): 861-872. doi: 10.3934/mbe.2013.10.861 |
[5] | Junjing Xiong, Xiong Li, Hao Wang . The survival analysis of a stochastic Lotka-Volterra competition model with a coexistence equilibrium. Mathematical Biosciences and Engineering, 2019, 16(4): 2717-2737. doi: 10.3934/mbe.2019135 |
[6] | Jinyu Wei, Bin Liu . Global dynamics of a Lotka-Volterra competition-diffusion-advection system for small diffusion rates in heterogenous environment. Mathematical Biosciences and Engineering, 2021, 18(1): 564-582. doi: 10.3934/mbe.2021031 |
[7] | Ranjit Kumar Upadhyay, Swati Mishra . Population dynamic consequences of fearful prey in a spatiotemporal predator-prey system. Mathematical Biosciences and Engineering, 2019, 16(1): 338-372. doi: 10.3934/mbe.2019017 |
[8] | Soumitra Pal, Pankaj Kumar Tiwari, Arvind Kumar Misra, Hao Wang . Fear effect in a three-species food chain model with generalist predator. Mathematical Biosciences and Engineering, 2024, 21(1): 1-33. doi: 10.3934/mbe.2024001 |
[9] | Li-Jun Du, Wan-Tong Li, Jia-Bing Wang . Invasion entire solutions in a time periodic Lotka-Volterra competition system with diffusion. Mathematical Biosciences and Engineering, 2017, 14(5&6): 1187-1213. doi: 10.3934/mbe.2017061 |
[10] | Qianqian Li, Ankur Jyoti Kashyap, Qun Zhu, Fengde Chen . Dynamical behaviours of discrete amensalism system with fear effects on first species. Mathematical Biosciences and Engineering, 2024, 21(1): 832-860. doi: 10.3934/mbe.2024035 |
In this paper, we study the problem of optimal control of backward stochastic differential equations with three delays (discrete delay, moving-average delay and noisy memory). We establish the sufficient optimality condition for the stochastic system. We introduce two kinds of time-advanced stochastic differential equations as the adjoint equations, which involve the partial derivatives of the function f and its Malliavin derivatives. We also show that these two kinds of adjoint equations are equivalent. Finally, as applications, we discuss a linear-quadratic backward stochastic system and give an explicit optimal control. In particular, the stochastic differential equations with time delay are simulated by means of discretization techniques, and the effect of time delay on the optimal control result is explained.
Fear, is defined as, "An unpleasant emotion caused by the belief that someone or something is dangerous" [1].
It is a complex emotion, that is critical as a safety measure, and can trigger the "fight or flight" response [2] - in particular it can change the way one acts, even when there is no threat present [3]. In predator-prey systems, this is most naturally observed among prey, due to their perceived threat of depredation [4]. This perception can lead to non-consumptive effects or trait-mediated interactions, which are behavioral, morphological or physiological changes in prey phenotype, due to this threat [4,5]. Such effects are known to strongly influence predator-prey dynamics [6]. From a mathematical viewpoint, the effects of fear in predator-prey systems has been intensely investigated since the seminal work of Brown et al. [7], where optimal foraging theory is extended to consider a game theoretic setup, played out by predator and prey, exhibiting stealth and fear, in which an animal follows a map or a "landscape of fear" (LOF), which describes its predation risk while it navigates the physical landscape. In recent work, Wang et al. [8], model fear of depredation, as a (predator) density dependent effect, that negatively effects the prey population. In essence, the prey's growth rate is modeled as a monotonically decreasing function of predator density. Dynamically, a key finding in [8] is that under the parametric restrictions of a Hopf bifurcation, an increase in the fear parameter (and prey's birth rate parameter) can alter the direction of a Hopf bifurcation from supercritical to subcritical. Thus, fear enables both supercritical and subcritical Hopf bifurcations, contrary to only the supercritical bifurcations found in classical predator-prey systems. In essence, the fear effect can change the fundamental cyclical patterns of predator-prey dynamics, leading to large scale ecological consequences [9]. Note, age/stage structure in the model can also have influence on the dynamics. For example, in [10] anti-predator sensitivity, as a consequence of fear, is introduced. Higher values of anti-predator sensitivity may cause predator extinction, thus adult prey persistence. However, lower values lead to co-existence, thus bi-stability is observed.
These results have since initiated a host of activities in diverse ecological scenarios such as when refuges are present [11,12] or when the prey has tendencies to avoid predators [13]. Predator avoidance could cause its own cascade of dynamics, such as bats avoiding cave predators could fall prey to diurnal birds [14], or the green anolis lizard avoiding curly predatory tailed lizards could in turn introduce refuge competition [15]. A predator's response could also be influenced by interference pressures, for instance, via a Beddington-DeAngelis functional response [16]. Various works have considered the fear effect in case of group defense by the prey [10,17]. It has been investigated in the context of cooperative and competitive systems within the larger predator-prey context. These include the fear effect when predators are cooperating, as earlier mentioned [14,18] in the hunting process, or when they are hunting for competing prey [19]. These effects have been investigated in the three and multi-species settings as well [20,21] where fear can damp population explosions [22]. Various authors have considered the fear effect in a stochastic setting [23] as well as a spatially explicit setting, in the context of taxis type movements, as well as pattern formation [24,25]. It can also lead to chaotic dynamics [26]. However, the effect of fear has been far less investigated in classical monotone systems, such as purely cooperative or competitive two species systems - that are outside the predator-prey setting.
Competition among two species, typically modeled via the Lotka–Volterra competition model and its variants have been intensely investigated in the last few decades. These models take into account growth and inter/intraspecific competition [27], and predict well-observed states in biology of co-existence, competitive exclusion of one competitor, and bi-stability, and find diverse applications in ecology and invasion science [28,29,30,31,32]. There are several ecological motivations for competitors being fearful of each other. This is perhaps most naturally seen to occur with intraguild predation - a widespread phenomenon in many food webs, where competitors will kill and consume each other [33]. Recent evidence of non-consumptive effects exerted by intraguild predator mites (Blattisocius dentriticus) on their competitor (Neoseiulus cucumeris) show this can be an important factor in determining food web dynamics in biological control [34,35]. However, there is strong evidence for fear in purely competitive two species systems without predatory effects. Barred owls (Strix varia) are a species of owl, native to eastern North America. They have expanded their range westward over the last century and are considered invasive in western North America. Currently, their range overlaps with the spotted owl (Strix occidentalis), which is native to the north west and western North America. This has resulted in intense competition between the two species [36]. Barred owls exert a strong negative influence on spotted owls, threatening their possible competitive exclusion [37]. Field observations report frequent barred owl attacks on spotted owls, and even on surveyors imitating spotted owl calls [38]. There is also evidence of barred owls aggressively chasing spotted owls out of shared habitat - but not the opposite [39]. There is also other very recent empirical evidence to support such investigations. In [15] a series of 6 year long experiments are conducted in various Caribbean islands that aim to refute the theory of adaptive predation - which suggests that predators reduce dominant competitors, thus preventing competitive exclusion and enhancing coexistence in food webs. However, non-consumptive effects such as fear of depredation can have strongly influencing effects [15,40,41,42]. [15] considers a series of experiments with two competing species of lizards, brown anolis (Anolis sagrei) that dwells on tree trunks, and green anolis (Anolis smaragdinus) that dwells on tree canopies. The experiments show that typically these species co-exist - due to a clear niche separation. However, the introduction of an intraguild predator, the curly tailed lizard (Leiocephalus carinatus) that dwells on the ground, causes (non-consumptive) fear driven effects. The brown anolis being fearful of possible depredation (as the lower half of tree trunks are within striking distance of the curly tailed lizard) moves upwards into the canopy, which is occupied by the green anolis. Herein interspecific competition intensifies leading to a loss of co-existence. However, what is most crucial in this study, is that the fecal analysis of the curly tail lizard shows that its diet included the brown anolis, in only 2 out of 51 samples examined. Thus the new dispersal pattern of the brown anolis and the "refuge competition" is driven strongly by a non-consumptive fear effect and not a consumptive one. Thus the brown anolis and the curly tail lizards are really competitors, as they have a strong overlap in dietary niche for several insects. However, the brown anolis is clearly fearful of the curly tailed lizard [15]. There is further evidence of non consumptive effects such as fear among competing aphid species, as well as competitors that feed on aphids [43,44]. Such interplay between competition and predation has been investigated [45], where it is proposed that in many ecological processes, competition and predation are interlinked, and depending on niche overlap, one of them will dominate to drive the underlying dynamics. Such evidence clearly motivates considering fear dynamics into competitive two species model where one of the competitors is fearful of the other. Furthermore, one could look at more complex settings as well, consisting of several interacting predators and prey, across several trophic levels [46].
There are also several socio-economic-political settings, where pure competitors may be fearful of each other. Small/new businesses (as weaker competitors) may be fearful of large businesses (that are stronger/dominant competitors), due to their already large market share [47]. But large business may also be fearful of small local businesses, due to their familiarity with local nuances, that may yield competitive advantage at a small local scale [48] - Thus there could be strong spatial heterogeneity associated to the way in which competitors are fearful of each other. Fear is also conceivable among two competing political parties, where the weaker party on a national scale, may have a stronger voter bank at a regional scale [49] - This again is a clear example of spatial heterogeneity, in that a weaker competitor overall is dominant or exerts fear on a stronger competitor at a local/regional scale. Or perhaps two warring drug cartels, where the weaker cartel has certain local/territorial strongholds [50,51] - within which they might be able to induce fear among the stronger cartel [50]. Such phenomenon becomes even more interesting in the spatially explicit case where this fear could be heterogeneous in the spatial domain of interest. This connects back to the LOF concept, where the fear function is essentially the map that describes how the fear levels change as a species disperses over a physical landscape.
Consider the classical two species Lotka-Volterra ODE competition model,
{dudt=u(a1−b1u−c1v),dvdt=v(a2−b2v−c2u), | (2.1) |
where u and v are the population densities of two competing species, a1 and a2 are the intrinsic (per capita) growth rates, b1 and b2 are the intraspecific competition rates, c1 and c2 are the interspecific competition rates. All parameters considered are positive. The dynamics of this system are well studied [52]. We recap these briefly,
● E0=(0,0) is always unstable.
● Eu=(a1b1,0) is globally asymptotically stable if a1a2>max{b1c2,c1b2}. Herein u is said to competitively exclude v.
● Ev=(0,a2b2) is globally asymptotically stable if a1a2<min{b1c2,c1b2}. Herein v is said to competitively exclude u.
● E∗=(a1b2−a2c1b1b2−c1c2,a2b1−a1c2b1b2−c1c2) exists when b1b2−c1c2≠0. The positivity of the equilibrium holds if c2b1<a2a1<b2c1 and is globally asymptotically stable if b1b2−c1c2>0. This is said to be the case of weak competition.
● If b1b2−c1c2<0, then E∗=(a1b2−a2c1b1b2−c1c2,a2b1−a1c2b1b2−c1c2) is unstable as a saddle. In this setting, one has initial condition dependent attraction to either Eu(a1b1,0) or Ev(0,a2b2). This is the case of strong competition.
We proceed by considering the effects of fear on the classical model (2.1), when one of the competitors is fearful of the other.
Species diffusion is ubiquitous in spatial ecology [53]. Species disperse to find mates, food and shelter [54]. Such movement is modeled often via reaction diffusion systems [53]. The spatially explicit Lotka-Volterra model, particularly in the case of heterogeneity in spatial resources has been intensely investigated, [27,55,56,57,58,59,60,61,62,63,64]. Herein, we consider the spatially explicit version of the Lotka-Volterra competition model for two competing species u,v, where the competitor v is fearful of u. We model the fear effect as in [8], where the growth rate of the fearful competitor v, is not constant but rather density dependent. Essentially, the growth rate is decreased by a factor ≈11+ku, where k≥0 is a fear coefficient. Thus a higher density of the competitor u increases the fear in v. When k=0, the assumption is there is no fear and one recovers the classical spatially explicit model. If fear is present, we obtain the following spatially explicit model,
ut=d1Δu+a1u−b1u2−c1uv,x∈Ω,vt=d2Δv+a2v1+ku−b2v2−c2uv,x∈Ω,∂u∂ν=∂v∂ν=0,on∂Ω,u(x,0)=u0(x)>0,v(x,0)=v0(x)>0. | (2.2) |
Here u(x,t),v(x,t) are the densities of two competing species, where v is also fearful of u. The species diffuse in a bounded domain Ω⊂Rn, with dispersal speeds d1 and d2, respectively. We prescribe Neumann boundary conditions, modeling the effect that the species do not immigrate or emigrate from the domain Ω. Also the initial data is chosen to be positive. We now proceed to study the dynamics of the above model, when various forms of fear are considered. We divide the dynamical analysis into two components, d1=d2=0, the ODE case discussed next, after which we consider the PDE case d1>0,d2>0, where we allow a spatially heterogenous fear function as well, so k=k(x).
We consider the dynamics of (2.2), when d1=d2=0, and we are in a bonafide ODE setting, where the competitor v is fearful of u,
dudt=a1u−b1u2−c1uv,dvdt=a2v1+ku−b2v2−c2uv. | (3.1) |
The nullclines associated with the system (3.1) are
u(a1−b1u−c1v)=0andv(a21+ku−b2v−c2u). |
Hence, the boundary equilibrium points are obtained by substituting u=0 and v=0 in the above equations of the nullclines, respectively. Denote the boundary equilibrium points as ˆE1=(0,0), ˆE2=(a1b1,0) and ˆE3=(0,a2b2).
For the interior equilibrium, substitute u∗=a1b1−c1b1v∗ in the second nullcline equation, i.e.,
a21+k(a1b1−c1b1v∗)−b2v∗−c2(a1b1−c1b1v∗)=0. |
On simplification, we have that v∗ solves a quadratic equation of the form A1(v∗)2+B1v∗+C1=0, where
A1=c1k(b2b1−c1c2),B1=b1(c1c2−b2b1)−a1k(b2b1−2c1c2),C1=b1(a2b1−a1c2)−a21c2k. | (3.2) |
Let
v∗1,2=−B1±√B21−4A1C12A1 | (3.3) |
be the two roots of above quadratic equation. Define discriminant as Δ1=B21−4A1C1. WLOG assume v∗1<v∗2. Moreover, consider the following parametric restriction
b1(a1c2−a2b1)+a21c2k<[(b2b1−c1c2)(2a1k−b1)−a1kc1c2]a1c1. | (3.4) |
We can prove the existence of a positive equilibrium point ˆE4 with the choice of specific parameters. Let us use Descartes's rule of sign to establish some sufficient conditions for the existence of one or two positive equilibrium points.
Two positive equilibrium points: Under the assumption A1>0,B1<0,C1>0, i.e., b2b1>2c1c2>c1c2,k<1a21c2(b21a2−a1c2b1) and Δ1>0, we have two positive roots. In order to claim that these two roots correspond to two positive interior equilibria, we need some extra assumption given by:
v∗1<v∗2:=−B1±√B21−4A1C12A1<a1c1⟹−C1<(A1a1c1+B1)a1c1. |
Hence, if b1(a1c2−a2b1)+a21c2k<[(b2b1−c1c2)(2a1k−b1)−a1kc1c2]a1c1, we have two positive interior equilibrium points ˆE4=(u∗i,v∗i) for i=1,2.
Remark 1. The existence of two positive internal equilibrium points can also be observed under the assumption A1>0,B1<0,C1>0, and Δ1>0, which ensures that
v∗2=−B1+√Δ12A1<−B1A1<a1c1, |
and there must be two positive internal equilibria. Similarly, under the assumption A1<0,B1>0,C1<0, and Δ1>0, we have the existence of two positive internal equilibria.
One positive equilibrium point: Under the assumption A1>0,B1<0, i.e., b2b1>2c1c2>c1c2, we have at least one positive root of the quadratic equation. If C1<0, which is k>1a21c2(b21a2−a1c2b1) along with (3.4) gives existence of one positive equilibrium point ˆE4=(u∗,v∗). Moreover, if C1>0, which is k<1a21c2(b21a2−a1c2b1), Δ1>0, and v∗1<a1c1 and v∗2>a1c1, then we have existence of one positive equilibrium point ˆE4=(u∗,v∗).
We formulate all these restrictions as an existence theorem:
Theorem 3.1. For the given ODE system (3.1), we always have three boundary equilibrium points, namely ˆE1:=(0,0),ˆE2:=(a1b1,0) and ˆE3:=(0,a2b2). For the case of two positive interior equilibrium points ˆE4=(u∗i,v∗i)i=1,2, we have the following parametric restrictions:
b2b1>2c1c2>c1c2,k<1a21c2[b21a2−a1c2b1],Δ1>0,&(3.4)holds true. |
Lastly, for the case of one positive interior equilibrium point ˆE4=(u∗,v∗), we have either one of the following choices of parametric restrictions:
1) b2b1>2c1c2>c1c2,k>1a21c2(b21a2−a1c2b1) and (3.4) holds true.
2) b2b1>2c1c2>c1c2,k<1a21c2(b21a2−a1c2b1), Δ1>0 and v∗1<a1c1 and v∗2>a1c1.
3) b2b1<c1c2<2c1c2,k>1a21c2(b21a2−a1c2b1), Δ1>0 and v∗1<a1c1 and v∗2>a1c1,
where v∗i are the roots of the quadratic equation defined as (3.3).
We now provide several lemmas, so that we can compare the effect of fear to the classical competition case.
Lemma 3.2. Consider the given ODE system (3.1), with k=0, such that the system is in the weak competition setting with b2b1>2c1c2. Then for a fear coefficient k such that k>kc=1a21c2(b21a2−a1c2b1), (a1b1,0) is globally asymptotically stable. That is, u will competitively exclude v.
Remark 2. For the existence of one positive interior equilibrium point for the given system (3.1), we have either one of the following choices of parametric restrictions: For weak competition,
b2b1>2c1c2>c1c2&c2b1<a2a1<b2c1, | (3.5) |
and for strong competition,
b2b1<c1c2<2c1c2&c2b1>a2a1>b2c1. | (3.6) |
We next perform stability analysis on the equilibrium points of system (3.1). The Jacobian matrix of system (3.1) is given by
J(u∗,v∗)=(a1−2b1u∗−c1v∗−c1u∗−a2kv∗(ku∗+1)2−c2v∗a2ku∗+1−2b2v∗−c2u∗). | (3.7) |
We state the following lemmas:
Lemma 3.3. ˆE1 is unstable.
Proof. On evaluating (3.7) at ˆE1, we have
J(ˆE1)=(a100a2). |
Being a triangular matrix, we know that the above matrix has two positive eigenvalues a1 and a2. Hence, the equilibrium point ˆE1 is unstable.
Lemma 3.4. ˆE2 is locally stable iff k>a2b21−c2a1b1a21c2.
Proof. We again evaluate (3.7) at ˆE2 and obtain
J(ˆE2)=(−a1−c1a1b10a2b1b1+ka1−c2a1b1). |
Being a triangular matrix, the above matrix has two eigenvalues, λ1=−a1 and λ2=a2b1b1+ka1−c2a1b1. As λ1 is always negative, if we can show that λ2 is negative, we are done. We make the assumption that,
k>a2b21−c2a1b1a21c2⟺λ2=a2b1b1+ka1−c2a1b1<0. |
Therefore, the boundary equilibrium point ˆE2 is locally stable.
Local stability of ˆE2 actually implies global stability, we can see this via a simple geometric argument.
Lemma 3.5. ˆE2 is globally stable if k>kc=a2b21−c2a1b1a21c2.
Proof. Consider the nullclines of u and v, where,
v=1c1(a1−b1u)&v=1b2(a21+ku−c2u). |
In order to establish the global stability of ˆE2, via the geometry of the nullclines, it suffices to show that
a1−b1uc1>1b2(a21+ku−c2u) |
when u=a1b1, i.e., when k>a2b21−c2a1b1a21c2. Herein, the v-nullcline lies completely below the u-nullcline, and via the convexity of the v-nullcline, it lies completely below the straight line connecting its v and u intercepts - which lies completely below the u-nullcline. Now, via the standard theory of competition and a comparison argument, where v is compared to the ˜v that is a solution to the straight line nullcline connecting the v and u intercepts of the v-nullcline, we have the global stability of ˆE2.
Lemma 3.6. The equilibrium point ˆE3 is locally stable iff a1b2<c1a2.
Proof. Similar evaluation of (3.7) at ˆE3 yields
J(ˆE3)=(a1−c1a2b20−ka22b2−c2a2b2−a1). |
Being a triangular matrix, the above matrix has two eigenvalues, λ1=−a1 and λ2=a1−c1a2b2. Under the assumed parametric restriction,
a1b2<c1a2⟺λ2<0. |
Hence, the equilibrium point ˆE3 is locally stable.
Remark 3. This is essentially the parametric restriction for the case when v excludes u, see Figure 4. Thus under this parametric restriction, ˆE3 is locally stable for all k, but globally stable only for certain k values.
Lemma 3.7. The interior equilibrium ˆE4 exists and is locally stable if 0<k<1a2(b1b2c1−c2).
Proof. On evaluating (3.7) again at ˆE4, we have
J(ˆE4)=(−b1u∗−c1u∗−ka2v∗(1+ku∗)2−c2v∗−b2v∗). |
For the local stability of ˆE4, it is enough to show that Tr(J(ˆE4))<0 and det(J(ˆE4))>0. Simple computations yield
Tr(J(ˆE4))=−b1u∗−b2v∗<0, |
and
det(J(ˆE4))=b1b2u∗v∗−c1u∗(ka2v∗(1+ku∗)2+c2v∗)=u∗v∗{b1b2−c1(ka2(1+ku∗)2+c2)}. |
Note that
ka2>ka2(1+ku∗)2. |
Therefore, if k is chosen such that, 0<k<1a2(b1b2c1−c2), then,
b1b2>c1(ka2+c2)>c1[ka2(1+ku∗)2+c2]⟹det(J(ˆE4))>0, |
and the result follows.
Lemma 3.8. The interior equilibrium ˆE4 exists and is a saddle if
b2b1c1−c2<ka2b21(b1+ka1)2. |
Proof. In order to claim that the interior equilibrium ˆE4 is a saddle, it is enough to show that Tr(J(ˆE4))<0 and det(J(ˆE4))<0. We have that
Tr(J(ˆE4))=−b1u∗−b2v∗<0, |
and
det(J(ˆE4))=b1b2u∗v∗−c1u∗(ka2v∗(1+ku∗)2+c2v∗)=u∗v∗{b1b2−c1(ka2(1+ku∗)2+c2)}. |
Under the assumption and density of reals, we have
b2b1c1−c2<ka2(1+ka1b1)2<ka2(1+ku∗)2⟹det(J(ˆE4))<0. |
Hence, ˆE4 is a saddle.
Lemma 3.9. Consider the system (3.1). For any given k>0 such that, there exist two positive interior equilibria, a sink and a saddle.
Proof. Theorem 3.1 provides conditions under which two positive interior equilibria exists. Since stability has to alternate by standard theory [65] for planar systems, one of the equilibrium is stable while the other is unstable. Instability as a source is impossible due to the lack of periodic dynamics in the system via Lemma 3.10. Thus the unstable equilibrium must be a saddle. This proves the lemma.
Lemma 3.10. Consider the ODE system (3.1). There do not exist any periodic orbits for the system, for any values of the fear parameter k.
Proof. Consider the function ϕ(u,v)=1uv where u and v are both non-zero. Let,
F1(u,v)=a1u−b1u2−c1uv,F2(u,v)=a2v1+ku−b2v2−c2uv. |
Then we have
∂(F1ϕ)∂u+∂(F2ϕ)∂v=∂∂u(1uv(a1u−b1u2−c1uv))+∂∂v(1uv(a2v1+ku−b2v2−c2uv))=−b1v−b2u<0. |
The result follows by application of the Dulac criterion [65].
Corollary 1. Consider the ODE system (3.1), with the fear term modeled as a2v1+kF(u), where F(u)∈C0,1. There do not exist any periodic orbits for the system, for any values of the fear parameter k.
Proof. The result follows via direct application of the Dulac function from Lemma 3.10.
Remark 4. Some observations:
1) From Figure 4, we observe that when there is no fear, then (0,v∗) is globally stable. For a sufficiently large level of fear in species v, a bi-stability situation is created. That is, for a certain initial data, species u is completely excluded by v and initial data is attracted to the co-existence state (See Figure 4).
2) If (0,v∗) is globally attracting, a much higher level of fear in species v (≈200) can change the dynamics to a strong competition type case. However most data in this setting is attracted to (u∗,v∗). For initial data (u0,v0) to be attracted to (0,v∗), we would need v0≫1,u0≪1.
3) Lemma 3.10 shows the lack of periodic orbits in (3.1), which could be interpreted as the inability of the fear factor to destabilize a steady state through a Hopf bifurcation to cyclical dynamics. This result could be extended to a wider class of fear models if fear in the second competitor was modeled as a2v1+kF(u), where F(u) was a general density dependent fear effect, not necessarily linear. See Corollary 1 which shows non existence of periodic orbits. Thus the result can be extended to a more general class of models than (3.1).
4) One could consider the case of u fearing v, but since the fear model is symmetric, most of the results will be similar. Thus we do not consider this case in detail, rather relegate a few technicalities to the appendix Section B, that rigorously show that the case of u fearing v and v fearing u, are indeed models that are symmetric.
A bifurcation is said to occur in a dynamical system when the behavior of solutions changes when a parameter is varied. Bifurcation analysis is useful in understanding and measuring these qualitative changes as the system switches from stable to unstable and vice-versa.
The following theorem is connected to the existence of a saddle-node bifurcation for the growth rate a1 when there is no fear effect in competitor u.
Theorem 3.11. The ODE system (3.1) undergoes a saddle-node bifurcation around ^E4 at a1=a∗1=2(ku∗+1)2(b1u∗(2b2v∗+c2u∗)+b2c1v∗2)−a2(2b1u∗(ku∗+1)+c1v∗(2ku∗+1))(ku∗+1)((ku∗+1)(2b2v∗+c2u∗)−a2) and when c1>a2(2b2v∗+c2u∗)u∗v∗(ka2+(1+ku∗)2c2).
Proof. We shall use the Sotomayor's Theorem [65] to show the occurrence of a saddle-node bifurcation at a1=a∗1. At a1=a∗1, we can have det(J)=0 and for c1>a2(2b2v∗+c2u∗)u∗v∗(ka2+(1+ku∗)2c2), Tr(J)<0. This implies that, det(J) admits a zero eigenvalue. Now let P=(p1,p2)T and Q=(q1,q2)T be the eigenvectors of J and JT corresponding to the zero eigenvalue respectively.
We have that, P=(−BA,1)T and Q=(−AF,1)T
where A=a1−2b1u∗−c1v∗, B=−c1u∗, F=−a2kv∗(1+ku∗)2−c2v∗.
Now, let X=(X1,X2)T where
X1=a1u−b1u2−c1uv,X2=a2v1+ku−b2v2−c2uv. |
Furthermore,
QTXa1(^E4,a∗1)=(−AF,1)(u∗,0)T=−Au∗F≠0 |
and
QT[D2X(^E4,a∗1)(P,P)]=(−AF,1)(2BA(b1dfracBA+c1),2a2kBA(1+ku∗)2[1−kv∗1+ku∗]−BC2A−b2)T≠0. |
Therefore by the Sotomayor's theorem system (3.1) undergoes a saddle-node bifurcation at a1=a∗1 around ^E4.
Remark 5. Consider the case of two interior equilibria, such as in Figure 4. Then decreasing the fear coefficient k results in a saddle-node bifurcation, where the interior equilibrium (the saddle ^E4 and the node ^E5) collide and disappear, resulting in the boundary equilibrium ^E2=(u∗,0) becoming globally asymptotically stable. Thus in this setting, a certain critical level of "fear" can maintain a co-existence state, but fear less than this level takes the system back to a competitive exclusion type scenario. This transition occurs via a saddle-node bifurcation. This can be rigorously proven by adopting the methods of Theorem 3.11, to the parameter k instead of using a1.
Theorem 3.12. The model (3.1) undergoes a transcritical bifurcation around ˆE3 when a1=a∗1=c1a2b2 and c1≠b1b2ka2+c2.
Proof. The Jacobian matrix for system (3.1) evaluated at ˆE3 with a∗1=c1a2b2 is given as
J=(00−a2b2(a2k+c2)−a2). | (3.8) |
The corresponding eigenvalues to the Jacobian of (3.1) in Eq (3.8) are λ1=0 and λ2=−a2. Clearly, there is a zero eigenvalue at a1=a∗1=c1a2b2. Next, we let W=(w1,w2)T and Z=(z1,z2)T represent the eigenvectors related to the zero eigenvalue of the matrices J and JT respectively.
We obtain W=(−b2ka2+c2,1)T and Z=(1,0)T. Now, let X=(X1,X2)T as already defined. Presently, we validate the transversality conditions using the Sotomayor's theorem [65]. Now,
ZTXa1(ˆE3,a∗1)=(1,0)(0,0)T=0. |
Also,
ZT[DXa1(ˆE3,a∗1)W]=(10)(1000)(w1w2)=−b2ka2+c2≠0 |
and
ZT[D2X(ˆE3,a∗1)(W,W)]=(10)(2b2ka2+c2(c1−b1b2ka2+c2)2a22k2b2(ka2+c2)2)=2b2ka2+c2(c1−b1b2ka2+c2)≠0. |
Therefore by the Sotomayor's theorem system (3.1) experiences a transcritical bifurcation at a1=a∗1=c1a2b2 around ˆE3.
Remark 6. Consider the case of one interior equilibrium, such as in Figure 2-that is, we are in the weak competition case, when there is no fear or k=0. Now increasing the fear coefficient k, results in a transcritical bifurcation, where the interior equilibrium (the node ˆE4) and the boundary saddle equilibrium ˆE2=(u∗,0) collide, exchange stability, after which ˆE4 now moves to the 4th quadrant, while ˆE2 becomes globally asymptotically stable. Thus in this setting, a certain critical level of "fear" can move the system from a weak competition setting of coexistence to a competitive exclusion type scenario. This transition occurs via a transcritical bifurcation. This is rigorously proven in Theorem 3.12.
Classical case | k>0 | |
(i) | CE (u∗,0) | Species v is competitively excluded for both small and large k. |
(ii) | CE (0,v∗) | 1) If a2b21k(b1+ka1)2<b1b2−c1c2c1, then species u is competitively excluded. |
2) Possibility of one interior saddle equilibrium, with a large level of k. | ||
3) Possibility of two positive interior equilibria, one sink and one saddle, with an intermediate level of k. | ||
(iii) | weak competition | 1) If k<kc=b2b1−c2c1a2c1 via Thm 3.1 yields co-existence. |
2) If k>kc, then species v is competitively excluded. | ||
(iv) | strong competition | Interior equilibrium always exists and is a saddle. |
In this section we consider the dynamics of (2.2), when d1,d2>0, and we are in a bonafide PDE setting, where the competitor v is fearful of u.
To prove global existence of solutions to (2.2), it suffices to derive uniform estimate on the Lp norms of the R.H.S. of (2.2), for some p>n2. Classical theory will then yield global existence [66]. The usual norms in spaces Lp(Ω), L∞(Ω) and C(¯Ω) are respectively denoted by
‖u‖pp=∫Ω|u(x)|pdx, ‖u‖∞ = ess supx∈Ω|u(x)|. | (4.1) |
To this end, we use standard techniques [67]. We first recall classical results guaranteeing non-negativity of solutions, local and global existence [67,68]:
Lemma 4.1. Let us consider the following m×m - reaction diffusion system: for all i=1,...,m,
∂tui−diΔui=fi(u1,...,um)inR+×Ω,∂νui=0on∂Ω,ui(0)=ui0, | (4.2) |
where di∈(0,+∞), f=(f1,...,fm):Rm→Rm is C1(Ω) and ui0∈L∞(Ω). Then there exists a T>0 and a unique classical solution of (4.2) on [0,T). If T∗ denotes the greatest of these T′s, then
[supt∈[0,T∗),1≤i≤m||ui(t)||L∞(Ω)<+∞]⟹[T∗=+∞]. |
If the nonlinearity (fi)1≤i≤m is moreover quasi-positive, which means
∀i=1,...,m,∀u1,...,um≥0,fi(u1,...,ui−1,0,ui+1,...,um)≥0, |
then
[∀i=1,...,m,ui0≥0]⟹[∀i=1,...,m,∀t∈[0,T∗),ui(t)≥0]. |
Lemma 4.2. Using the same notations and hypotheses as in Lemma 4.1, suppose moreover that f has at most polynomial growth and that there exists b∈Rm and a lower triangular invertible matrix P with nonnegative entries such that
∀r∈[0,+∞)m,Pf(r)≤[1+m∑i=1ri]b. |
Then, for u0∈L∞(Ω,Rm+), the system (4.2) has a strong global solution.
Under these assumptions, the following local existence result is well known, see D. Henry [66].
Theorem 4.3. The system (4.4) admits a unique, classical solution (u,v) on [0,Tmax]×Ω. If Tmax<∞ then
limt↗Tmax{‖u(t,.)‖∞+‖v(t,.)‖∞}=∞, | (4.3) |
where Tmax denotes the eventual blow-up time in L∞(Ω).
The next result follows from the application of standard theory [69].
Theorem 4.4. Consider the reaction diffusion system (2.2). For spatially homogenous initial data u0≡c,v0≡d, with c,d>0, then the dynamics of (4.4) and its resulting kinetic (ODE) system, when d1=d2=0 in (2.2), are equivalent.
Our objective now is to consider the case of a fear function that may be heterogeneous in space. A motivation for this comes from several ecological and sociological settings. For example it is very common for prey to be highly fearful closer to a predators lair, but less fearful in a region of refuge [12], or in regions of high density due to group defense [70]. Furthermore, a conceivably weaker drug cartel, could have certain localized strongholds, within which they would be more feared by stronger groups. To these ends, it is conceivable that the fear coefficient k is not a constant, but actually varies in the spatial domain Ω, so k=k(x), which could take different forms depending on the application at hand. This is also in line with the LOF concept [7]. Thus we consider the following spatially explicit version of (3.1), with heterogeneous fear function k(x), resulting in the following reaction diffusion system,
ut=d1(u)xx+a1u−b1u2−c1uv,x∈Ω,vt=d2(v)xx+a2v1+k(x)u−b2v2−c2uv,x∈Ω,∂u∂ν=∂v∂ν=0,on∂Ω.u(x,0)=u0(x)≡c>0,v(x,0)=v0(x)≡d>0, | (4.4) |
where Ω⊂Rn. We assume Neumann boundary conditions. Also, we prescribe spatially homogeneous (flat) initial conditions u(x,0)=u0(x)≡c>0,v(x,0)=v0(x)≡d>0. Furthermore, we impose the following restrictions on the fear function k(x),
(i)k(x)∈C1(Ω),(ii)k(x)≥0,(iii)If k(x)≡0 on Ω1⊂Ω, then |Ω1|=0.(iv)If k(x)≡0 on ∪ni=1Ωi⊂Ω, then Σni=1|Ωi|=0. | (4.5) |
Remark 7. If k(x)≡0 on Ω1⊂Ω, with |Ω1|>δ>0, or k(x)≡0 on ∪ni=1Ωi⊂Ω, with Σni=1|Ωi|>δ>0, that is, on non-trivial parts of the domain, the analysis is notoriously difficult, as one now is dealing with a degenerate problem. See [71,72] for results on this problem. This case is not in the scope of the current manuscript.
Since the nonlinear right hand side of (4.4) is continuously differentiable on R+× R+, then for any initial data in C(¯Ω) or Lp(Ω),p∈(1,+∞), it is standard to estimate the Lp−norms of the solutions and thus deduce global existence. Standard theory will apply even in the case of a bonafide fear function k(x), because due to our assumptions on the form of k, standard comparison arguments will apply [73]. Thus applying the classical methods above, via Theorem 4.3, and Lemmas 4.1 and 4.2, we can state the following lemmas:
Lemma 4.5. Consider the reaction diffusion system (4.4), for k(x) such that the assumtions via (4.5) hold. Then solutions to (4.4) are non-negative, as long as they initiate from positive initial conditions.
Lemma 4.6. Consider the reaction diffusion system (4.4). For k(x) such that the assumtions via (4.5) hold, the solutions to (4.4) are classical, that is for (u0,v0)∈L∞(Ω), (u,v)∈C1(0,T;C2(Ω)), ∀T.
Our goal in this section is to investigate the dynamics of (4.4). Herein we will use the comparison technique, and compare to the ODE cases of classical competition, or the constant fear function case, where the dynamics are well known.
Remark 8. The analysis in this section are primarily focused on the choice of spatially homogenous (flat) initial data.
We begin by defining the following systems of PDEs,
¯ut=d1(¯u)xx+a1¯u−b1¯u2−c1¯u¯v,¯vt=d2(¯v)xx+a2¯v−b2¯v2−c2¯u¯v, | (4.6) |
^ut=d1(ˆu)xx+a1ˆu−b1ˆu2−c1ˆuˆv,ˆvt=d2(ˆv)xx+a2ˆv1+ˆkˆu−b2ˆv2−c2ˆuˆv, | (4.7) |
˜ut=d1(˜u)xx+a1˜u−b1˜u2−c1˜u˜v,˜vt=d2(˜v)xx+a2˜v1+˜k˜u−b2˜v2−c2˜u˜v, | (4.8) |
˜ut=d1(˜u)xx+a1˜u−b1˜u2−c1˜u˜v,˜vt=d2(˜v)xx+a2˜v1+˜ka1b1−b2˜v2−c2˜u˜v, | (4.9) |
where
ˆk=minx∈Ωk(x),˜k=maxx∈Ωk(x). | (4.10) |
We assume Neumann boundary conditions for all of the reaction diffusion systems (4.6)–(4.9). Also in each of the systems we prescribe spatially homogenous (flat) initial conditions u(x,0)=u0(x)≡c>0,v(x,0)=v0(x)≡d>0.
We now state the following lemma:
Lemma 4.7. Consider the reaction diffusion system (4.4), as well as the reaction diffusion systems (4.6)–(4.9). Then the following point wise comparison holds,
˜v≤˜v≤v≤ˆv≤¯v. |
Proof. Note via positivity of solutions to (4.4), (4.6)–(4.9), the definitions via (4.10), and the upper bound on u, which is a solution to (4.4) of a1b1, (derived via comparison to the logistic equation), we have
a21+˜ka1b1≤a21+˜ku(x)≤a21+k(x)u(x)≤a21+ˆku(x)≤a2,∀x∈Ω. | (4.11) |
Thus the result follows via standard comparison theory [73].
Theorem 4.8. Consider the reaction diffusion system (4.4), for a fear function k(x), such that the assumptions via (4.5) are met, and
˜k>a2b21−c2a1b1a21c2anda1a2>max{b1c2,c1b2}. |
Then the solution (u,v) to (4.4) converges uniformly to the spatially homogenous state (a1b1,0) as t→∞.
Proof. From the classical theory of competition [52], we know the dynamics for (4.6), that is in the competitive exclusion case, when
a1a2>max{b1c2,c1b2}, |
we have
(¯u,¯v)→(a1b1,0). |
Moreover, under the assumption
˜k>a2b21−c2a1b1a21c2, |
and making use of Lemma 3.5, along with the use of Theorem 4.4, we have (˜u,˜v)→(a1b1,0). Now using Lemma 4.7 we have,
˜v≤v≤¯v, |
which entails,
limt→∞(˜u,˜v)≤limt→∞(u,v)≤limt→∞(¯u,¯v), |
subsequently,
(a1b1,0)≤limt→∞(u,v)≤(a1b1,0). |
Now using a squeezing argument, in the limit that t→∞, we have uniform convergence of solutions of (4.4), i.e.,
(u,v)→(a1b1,0) |
as t→∞.
Using the positivity of solutions the requirement on k(x) and so in turn on ˜k, can be weakened to derive a stronger result.
Theorem 4.9. Consider the reaction diffusion system (4.4), for a fear function k(x), such that the assumptions via (4.5) are met, and a1a2>max{b1c2,c1b2}. Then the solution (u,v) to (4.4) converges uniformly to the spatially homogeneous state (a1b1,0) as t→∞.
Proof. From the classical theory of competition [52], we know the dynamics for (4.6), that is in the competitive exclusion case, when
a1a2>max{b1c2,c1b2}, |
we have
(¯u,¯v)→(a1b1,0). |
Now using Lemma 4.7, we have
v≤¯v. |
Using the non negativity of solutions to (4.4) via Lemma 4.5, entails,
0≤limt→∞v≤limt→∞(¯v)=0, |
subsequently,
limt→∞(u,v)→(a1b1,0). |
Theorem 4.10. Consider the reaction diffusion system (4.4), for a fear function k(x), such that the assumptions via (4.5) are met, and
a2b21˜k(b1+˜ka1)2<b1b2−c1c2c1anda1a2<min{b1c2,c1b2}. |
Then the solution (u,v) to (4.4) converges uniformly to the spatially homogeneous state (0,a2b2) as t→∞.
Proof. From the classical theory of competition [52], we know the dynamics for (4.6), that is in the competitive exclusion case, when
a1a2<min{b1c2,c1b2}, |
we have
(¯u,¯v)→(0,a2b2). |
Consider the nullclines of (4.8). If
ddu[a1−b1uc1]<ddu[1b2(a21+˜ku−c2u)], |
it follows via the geometry of the nullclines, that the v-nullcline remains above the u-nullcline, and thus (˜u,˜v)→(0,a2b2). On simplification, and using the upper bound estimate for u, we have
a2b21˜k(b1+˜ka1)2<a2˜k(1+˜ku)2<(b1b2−c1c2c1). |
Now using Lemma 4.7 we have,
˜v≤v≤¯v, |
which entails,
limt→∞(˜u,˜v)≤limt→∞(u,v)≤limt→∞(¯u,¯v), |
subsequently,
(0,a2b2)≤limt→∞(u,v)≤(0,a2b2). |
Now using a squeezing argument, in the limit that t→∞, we have uniform convergence of solutions of (4.4), i.e.,
(u,v)→(0,a2b2) |
as t→∞.
We state the following result:
Lemma 4.11. Consider the reaction diffusion system (4.4), for a fear function k(x), such that the assumptions via (4.5) are met, with
˜k>a2b21−c2a1b1a21c2andb1b2>2c1c2. |
Then the solution (u,v) to (4.4) converges uniformly to the spatially homogeneous state (u∗,0) as t→∞.
Proof. Via Lemma 3.2, and the parametric restrictions assumed we have,
˜v≤v≤ˆv, |
and the result follows via similar analysis as in Theorem 4.8.
Lemma 4.12. Consider the reaction diffusion system (4.4), such that system is in the weak competition case when k(x)≡0. Then given 0<ϵ≪1, there exists a fear function kϵ(x), for which the assumptions via (4.5) are met, such that the solution (u,v) to (4.4) with the fear function kϵ(x), converges uniformly to a spatially homogeneous state (u∗,v∗) as t→∞.
Proof. Given 0<ϵ≪1, we can always construct a kϵ, s.t kc−ϵ≤ˆkϵ, whereas ˜kϵ≤kc+ϵ. Thus via Lemma 4.7 we have,
˜v≤vϵ≤ˆv. |
Lemma 3.7 ensures we have (˜u,˜v)→(u∗,v∗) and (ˆu,ˆv)→(u∗∗,v∗∗), where the spatially homogeneous solutions may be different. Hence, via squeezing argument, we can take ϵ→0, to yield the uniform convergence of solutions, i.e.,
limϵ→0limt→∞(uϵ,vϵ)→(u∗,v∗). |
This proves the lemma.
The numerical simulations above motivate the following conjecture:
Conjecture 1. Consider the reaction diffusion system (4.4), for a fear function k(x), such that the assumptions via (4.5) are met, with
˜k<1a2(b1b2c1−c2), |
and the parameters follow (3.5) and Theorem 3.1, then the solution (u,v) to (4.4) converges uniformly to the spatially homogenous state (u∗,v∗) as t→∞.
Remark 9. We see via Lemma 3.5, and Lemma 3.7, that in the ODE case, if we are in the weak competition setting without fear, then a critical amount of fear kc is both sufficient and necessarily required to change the system's dynamics to a competitive exclusion type scenario. In the PDE case, where the fear function k(x) can be spatially heterogeneous, this requirement is certainly sufficient, as seen via Theorem 4.13, but not necessary, in a point wise sense.
This result is stated next:
Theorem 4.13. Consider the reaction diffusion system (4.4), with initial data u0(x)≥1, s.t u↗u∗(u increases to u∗), such that system is in the weak competition setting when k(x)≡0. For a fear function k(x), s.t the assumptions via (4.5) are met, the solution (u,v) converges uniformly to the spatially homogeneous state (a1b1,0) as t→∞, if the following condition holds,
C1|Ω|∫Ω11+k(x)dx<(11+kc(a1b1)), | (4.12) |
where kc is as defined in Lemma 3.5.
Proof. Consider (4.4), integrating the equation over Ω yields,
ddt∫Ωvdx=∫Ω(a2v1+k(x)u−b2v2−c2uv)dx≤∫Ω(a2v1+k(x)u0−b2v2−c2uv)dx=11+k(x∗)u0∫Ωa2vdx−∫Ω(b2v2+c2uv)dx≤11+k(x∗)∫Ωa2vdx−∫Ω(b2v2+c2uv)dx≤(C1∫Ω11+k(x)dx)∫Ωa2vdx−∫Ω(b2v2+c2uv)dx≤(11+kca1b1)∫Ωa2vdx−∫Ω(b2v2+c2uv)dx≤∫Ω(a21+kca1b1v−b2v2−c2uv)dx. | (4.13) |
This follows via the mean value theorem for integrals. We can now compare,
∫Ωvdx<∫Ω˜vdx, | (4.14) |
where ˜v solves d˜vdt=a21+kca1b1˜v−b2˜v2−c2u˜v. Thus using Theorem 4.4, Lemma 3.2 and the positivity of solutions, this entails
0≤limt→∞∫Ωvdx≤limt→∞∫Ω˜vdx=0. | (4.15) |
The bounds on v, standard Lebesgue convergence theorems, and squeezing argument entail,
∫Ω(limt→∞v)dx→0, | (4.16) |
which implies the uniform convergence,
limt→∞(u,v)→(a1b1,0). |
Remark 10. The C1 in Theorem 4.13 is a pure constant, that will depend on the size of the domain Ω, and the other problem parameters, but not on the initial data or the spatial variable x.
Remark 11. Clearly k(x) could be chosen such that it lies below kc for a portion of the domain, and above kc on some portion of the domain - thus minx∈Ωk(x) does not lie uniformly above kc. Yet via Theorem 4.13, we see that, one can change the system's dynamics and bring it to a competitive exclusion type scenario, from a coexistence situation.
The next result gives a lower estimate for the fear function k.
Lemma 4.14. Consider the fear function k(x) in Theorem 4.13, then we have,
∫Ωk(x)dx≥|Ω|(1−1C1(11+kc(a1b1))). |
Proof. We have that for any fear function k(x) satisfying (4.5),
11+k(x)>1−k(x)⟹∫Ω11+k(x)dx>|Ω|−∫Ωk(x)dx. | (4.17) |
Now using Theorem 4.13 the result follows.
Theorem 4.15. Consider the reaction diffusion system (4.4), for a fear function k(x), such that the assumptions via (4.5) are met, with b1b2<c1c2. Then there exists sufficiently large positive initial data [u0,v0] for which the solution (u,v) to (4.4) converges uniformly to the spatially homogenous state (0,a2b2) as t→∞, while there also exists sufficiently small positive initial data [u1,v1] for which solution (u,v) to (4.4) converges uniformly to the spatially homogenous state (a1b1,0) as t→∞.
Proof. Consider the system (4.6). From the classical strong competition parametric restrictions, b1b2<c1c2 that are assumed, as well as Lemma 4.7, we can make use of standard competition theory and use the stable manifold theorem, i.e., ∃Ws(E4)∈C1 separatrix, such that for initial data (¯u0,¯v0) chosen above Ws(E4) the solution (¯u,¯v)→(0,v∗) and for initial data chosen below Ws(E4), (¯u,¯v)→(u∗,0). Here E4 is the interior saddle equilibrium to the kinetic (ODE) system for (4.6). Moreover, since a21+˜ka1b1≤a2, and b1b2<c1c2, we have that for (4.10) we still remain in the strong competition case, and via standard theory again, W1s(E∗4)∈C1 separatrix, such that for initial data (ˆu0,ˆv0) chosen above W1s(E∗4), the solution (˜u,˜v)→(0,v∗) and for initial data chosen below W1s(E4), (˜u,˜v)→(u∗,0). Here E∗4 is the interior saddle equilibrium to the kinetic (ODE) system for (4.9). Now since a21+˜ka1b1≤a2, the v component of E∗4 is higher than the v component of E4. Now using the C1 property of the separatricies W1s(E∗4),Ws(E4), we have the existence of a wedge V emanating from E4, s.t within V we have W1s(E∗4)≥Ws(E4). Note via Lemma 4.7, we have ˜v≤v≤¯v. Let us consider positive initial data (u0,v0) chosen large enough, within V s.t. (u0,v0)>W1s(E∗4)>Ws(E4), we will have
{(0,v∗)}≤{(u,v)}≤{(0,v∗)}. |
On the other hand, for positive initial data (u1,v1) chosen small enough via an analogous construction we will have
{(u∗,0)}≤{(u,v)}≤{(u∗,0)}. |
This proves the theorem.
From the observations in the above numerical simulations, we can state a conjecture concerning positive interior equilibrium.
Conjecture 2. Consider the system (4.4). For any non-negative fear function k(x) such that
b2b1c1−c2<ka2b21(b1+ka1)2 | (4.18) |
and parametric restrictions given by (4.5) and Theorem 3.1 hold true, for k=˜k,ˆk. There exists some data [u0,v0], for which the solution (u,v)→(u∗,v∗), and for some choice of data [u1,v1], the solution converges to the boundary equilibrium (0,v∗).
All of our PDE simulations for the case of a spatially heterogeneous fear function were performed in MATLAB R2021b, using the MATLAB inbuilt function pdepe, which is used in solving 1−D parabolic and elliptic PDEs. The simulations were run on an 8−core CPU, Apple M1 pro-chip-based workstation. In this configuration, a typical simulation takes between 5–7 seconds when the spatial domain is taken to be [0,1], and is partitioned into 1000 sub-intervals. The simulations' parameters are chosen to meet the parametric constraints of the theorems. In the spatially explicit setting, we have used the comparison theory to determine point-wise restrictions on the fear functions such that competitive exclusion or strong competition-type dynamics abound. These are shown via Theorems 4.8, 4.10 and 4.15, whereas Figures 8, 9 and 12 can infer the numerical validation of this result. We also have numerically validated that in the spatially explicit setting, fear can change a situation of weak competition to a competitive exclusion type scenario for fear functions with certain L1 restrictions. This is proved in Theorem 4.13 and Lemma 4.14. Moreover, we have performed a time series analysis over a long period to numerically validate the result, see Figures 14–16. Various heterogeneous fear functions are constructed to demonstrate these results numerically; see Figure 17b. All the details about the parameters and their choice are explicitly mentioned in the captions of the figures of numerical simulation. Essentially in all of the PDE and ODE simulation, parametric values are chosen that meet the parametric restrictions of the Lemmas and Theorems that are being numerically demonstrated. Also we assume fairly competitive populations, and do not want one population exceeding more than three times the other. This (under scaling) restricts the choice of the parameters to lie in the range (0,3]. This also assists in easier visualization of the dynamics.
In this section we discuss several aspects of the lemmas and theorems described in the current manuscript. We first briefly recap our key results.
● Sufficiently large fear can change a situation of competitive exclusion, to a strong competition type scenario, where there is bi-stability between boundary equilibrium. Dynamically, this occurs via a transcritical bifurcation. This is shown via Lemma 3.12, see Figure 6.
● Fear in a certain parametric regime can change a situation of competitive exclusion to bi-stability between boundary equilibrium and interior equilibrium, see Figure 4. Dynamically, this occurs via a saddle-node bifurcation. This is shown via Lemma 3.11, see Figure 5. This is in sharp contrast with classical competition theory, where bi-stability occurs only between boundary equilibria.
● Sufficiently large fear can change a situation of weak competition to a competitive exclusion type scenario. This is shown via Lemma 3.5, see Figure 2.
● Fear cannot qualitatively change a strong competition type scenario. This is shown via Lemma 3.8, see Figure 3. Also, fear cannot produce periodic orbits. This is demonstrated via Lemma 3.10 and Corollary 1.
● In the spatially explicit setting, comparison theory is used to determine point-wise restrictions on the fear functions such that competitive exclusion or strong competition type dynamics abounds. These are shown via Theorems 4.8, 4.10 and 4.15, see Figures 8, 9 and 12.
● In the spatially explicit setting, fear can change a situation of weak competition to a competitive exclusion type scenario, for fear functions with certain L1 restrictions. This is shown via Theorem 4.13 and Lemma 4.14, see Figures [14,15,16]. In particular, the fear functions need not lie uniformly above the critical fear levels derived in the ODE case via Lemma 3.5.
● Various heterogeneous fear functions are constructed to demonstrate these results numerically, see Figure 17b. Applications of these to ecological as well as socio-political settings are discussed in Section 6.
We see that in the competitive exclusion case, where (u∗,0) is globally stable, a small amount of fear in u, can bring about a strong competition type situation - via a transcritical bifurcation. Where after bifurcation there appears an interior equilibrium, which is a saddle, and one has initial condition dependent attraction to (u∗,0) or (0,v∗). See Figures 4 and 6]. However, a slightly larger level of fear, can bring down the separatrix drastically see Figure 17a. Furthermore, here (depending on parametric restrictions) only a very small quantity of fear can create two interior equilibriums, see Figure 6, where there are both weak and strong competition dynamics at play, where most initial conditions are attracted to an interior equilibrium, but certain initial conditions (u0,v0) (where u0≪1,v0≫1), are attracted to (0,v∗). It is interesting to think about this in an invasion setting. If u were an invasive species, and v a resident species, then in the absence of fear in u, it can invade and thus exclude v. However, if the resident v can instill just a small amount of fear in the invader u, coexistence for most initial conditions is possible. If one plays devil's advocate, and switches the role of u and v, (u resident, v invader), then we see in the absence of fear in the resident, it will exclude the invader, but if it can instill some fear, then coexistence is possible, as earlier alluded to. However, having too much fear in either case, yields only one interior equilibrium, which is a saddle and we are in a strong competition type setting, with initial condition dependent attraction to either (u∗,0) or (0,v∗). Thus if coexistence (for most initial conditions) is sought after, it is advantageous to induce a little fear - but not too much fear. Dynamically, this is seen because in the former case we have a saddle-node bifurcation occurring first, followed by a transcritical bifurcation, see Figure 6b, as opposed to the later case, where only a transcritical bifurcation occurs, see Figure 6a. Also, a 2 parameter bifurcation in the k−c1 parameter space reveals a rich higher co-dimensional bifurcation structure. A Bogdanov Takens (BT) bifurcation, as well as a saddle node transcritical (SNTC) bifurcation is observed. Future work could entail further theoretical investigations of the SNTC [74], herein numerical investigations are also apt [75,76]. In this work we assume fear comes from the physical presence of a competitor. However even past presence such as by scent or other chemical cues can cause fear [77]. It is worthwhile considering modeling such a scenario in future work.
This phenomenon becomes even more interesting in sociological or political settings, particularly when we are in the spatially explicit scenario. Consider the case of law enforcement trying to control crime in high crime areas, where traditionally control efforts have failed, indicative of the competitive exclusion scenario, or a coexistence scenario, where the criminal groups have not been managed so coexist at high levels, and have not been eradicated. The question becomes, what is the optimal design of the fear function k(x), that could now yield a co-existence scenario–or one that can yield a competitive exclusion scenario. We see via Theorem 4.13, that this function could be very small in some areas of the spatial domain, and large enough in others, so that it would change dynamics - this is also seen numerically, where "hotspot" type fear functions have been utilized to generate a competitive exclusion type scenario, see Figures 14–16. Similar ideas for control and policing activities have been explored in [78,79]. Essentially, the fear function can be modeled through various functional types, that also preserve spatial features of the underlying domain. For instance, consider competition between two warring drug cartels, where the weaker cartel has certain territorial strongholds. This situation can be modeled through oscillatory functions like sin2(nx), where n∈N. Moreover, for complex ecological models, the result of these theorems also holds, where we need to model the fear responses by a sequence of functions or in the form of piece-wise functions. We display some of these functions in Figure 17b. Many of the relevant theorems have been tested by choosing such functions (See Figures 8, 10, 12 and 13). However, we have not incorporated non-smooth fear function or non-smooth density dependence herein [31,80,81,82], and this remains an apt direction for future work. In essence the fear model considered can be placed in a much broader context than ecological. Corollary 1 enables us to provide a mechanistic description of the fear function in much broader contexts. Also, in sociopolitical domains, the fear effect does not have to be thought of as consumptive vs non-consumptive. For example a new small business that is fearful of a larger established business might not invest in advertising or expanding clientele in established market, rather search for niche markets - which might hinder its growth. Interesting future work could consider competition models with a fear effect where the outcome of the competition whether contest or scramble was explicit.
In the weak competition case without fear, once there is a critical level of fear, in either u or v, a competitive exclusion type phenomenon will occur, see Lemma 3.5. This occurs again via a transcritical bifurcation, see Theorem 3.12 and Figure 6. This again is interesting in the spatially explicit setting, as via Theorem 4.8, where the fear function need not be above kc so as to induce competitive exclusion. We have explored certain types of fear functions herein, see Figures 8, 14–16, but the effect of functions such as in Figure 17b, remain to be explored. Here one could look at the effect of fear in fragmented domains vs domains that are not, via deriving or enforcing conditions relating the fear function k(x) to the resource function m(x), see similar ideas explored in [83]. The results are symmetric even if u is assumed to be fearful of v, and one considers the spatially explicit case, i.e., when a competitive exclusion situation, can change to a bi-stability type situation, where the bi-stability is between the (u∗,0) and a coexistence equilibrium. A further increase of the fear function causes a strong stability situation to occur. Similar dynamics are seen in changing a weak competition case to a competitive exclusion case. We are also motivated to studying the fear effect in competitive systems modeled differently - such as bringing in this effect in the competitive coefficients, rather than the birth rate. In addition, for competitive systems, one might intuitively think that the "dominant" population causes fear in the inferior population, which further weakens the inferior population. However, simulations show this is incorrect. Particularly if one considers fear in both competitors. The interplay of the heterogenous fear functions k(x),f(x), can actually lead to a co-existence scenario, even when one competitor is dominant with and without the fear effect. This counterintuitive situation warrants much further investigation.
An interesting question is the dynamical consequence of Allee effects on the fear models considered. Note, Allee effects in competition systems have been considered [84,85], and results show the possibility of alternate stable states, and even tri-stability situations possible, where the occurrence of two interior saddle equilibrium, cause the phase to be partitioned such that initial data can be attracted to (u∗,0), (0,v∗) or (0, 0). However, whether a weak or strong Allee effect is considered, periodic orbits have not been shown to occur [84,85,86]. The possibility of two interior saddles does not preclude the existence of heteroclinic orbits, say via a saddle-saddle collision–although such dynamics have not been rigorously proved to the best of our knowledge. The interplay of the fear effect with an Allee effect could well change this situation. For example with a simple strong Allee effect the Dulac function used in Lemma 3.10 would not be sufficient to claim non-existence of limit cycle dynamics. Thus such investigations would make for interesting future studies. It would be interesting to explore applications of these results to the case of competing political parties, where a nationally weaker party with smaller voter bank, could have several local strongholds. What levels of fear need to be instilled in those strongholds so as to enforce competitive advantage, is an apt question. Herein estimates on the L1(Ω) norm of k(x), would be interesting to derive. Currently Lemma 4.14, only gives lower estimates - but sharp upper estimates or even just upper estimates are unknown, and would make for interesting future work. The effect of fear on the strong competition setting is perhaps the least interesting dynamically. Herein, having fear in u or v, only shifts the interior saddle equilibrium - but qualitatively the dynamics remain the same, as in a strong competition type scenario persists, no matter what level of fear (f or k) is chosen. Also it would be of interest to rigorously prove Conjectures 1 and 2. Our current numerical evidence clearly motivates their validity. It would also be of interest to consider fractional derivative extensions of the fear model (3.1). Such problems have been much investigated recently [87,88,89,90].
Also, in [8], it is found that various monotonically decreasing fear functions essentially yield the same dynamics. This has not been tested in the current work, in the case of competitive systems. Neither have we investigated rigorously the case of both competitors being fearful of each other with possible different fear functions, indicative of different LOF for each competitor. Here again, upper estimates on the fear functions would be useful and we could allude to methods and techniques explored in [91] and in [92]. Here, one could consider a spatially dependent growth function as well, such as in [92], and attempt to derive conditions relating the function describing the resources to the fear function. Furthermore, it would make for interesting future work if certain choices of (density) dependence or fear functions which lead to degenerate dynamics, can cause periodic orbits. In the current scenario Lemma 3.10 and Corollary 1, do not allow limit cycle dynamics. All in all, we hope these questions lead to future investigations of the fear effect in competitive systems, as a host of rich applications exist.
VS and RP acknowledge valuable summer support from the National Science Foundation via DMS 1715044.
The author declare that they have no conflict of interest.
Here we shall consider the case when the competitor u is being fearful of v. Modeling as earlier yields,
dudt=a1u1+fv−b1u2−c1uv,dvdt=a2v−b2v2−c2uv. | (A.1) |
The system (A.1) can have one or two positive interior equilibria, but not three. This is established via the following lemma:
Lemma A.2.1. Consider the ODE system (A.1), with f=0. If we are in the competitive exclusion, weak-competition, or strong-competition setting, then for any f>0, under the parametric restrictions in (A.2), there do not exist three positive interior equilibria.
Proof. On substituting u∗=1b1(a11+fv∗−c1v∗) in the second nullcline equation, we get the following third order polynomial in v∗:
B2(v∗)3+C2(v∗)2+D2(v∗)+E2=0, |
where
B2=b1f2(c1c2−b1b2),C2=a2b21f2+2b1f(c1c2−b2b1),D2=b1f(−a1c2+2a2b1)+b1(c1c2−b2b1),E2=b1(−a1c2+a2b1). | (A.1) |
We will require the following conditions to satisfy Descartes's rule of signs, so as to obtain three positive roots to the cubic equation. These are:
c1c2−b1b2<0,a2b1f+2(c1c2−b2b1)>0,f(−a1c2+2a2b1)<−(c1c2−b2b1),−a1c2+a2b1>0. | (A.2) |
Let us prove this is an impossible claim by contradiction: First, assume the parameter set satisfies both competitive exclusion-state parametric restriction and (A.2). We know that competitive exclusion-state is asymptotically stable if a1a2>max{b1c2,c1b2}. On using these parametric restriction, −a1c2+a2b1<0, which is a contradiction to the last inequality in (A.2).
If the parameter set satisfies both the strong competition state parametric restriction and (A.2), then we have a contradiction because of the first inequality in (A.2), as under the strong competition state parametric restriction that inequality should be positive.
For the weak competition, recall the parametric restrictions:
c2b1<a2a1<b2c1. |
Let us re-write the third inequality in (A.2),
−(c1c2−b2b1)−f(−a1c2+2a2b1)>0. |
On adding the second and third inequality in (A.2), we have
(c1c2−b1b2)+f(a1c2−a2b1)>0, |
which is a contradiction as the added inequality should be negative by the parametric restrictions of weak-competition. This proves the lemma.
Also note:
Remark 12. The cubic equation obtained in (A.1) can be transformed to a quadratic polynomial by reducing (b1fv∗+b1) on both sides, i.e.,
~B2(v∗)2+~C2v∗+~D2=0, |
where
~B2=f(c1c2−b1b2),~C2=(c1c2−b1b2)+a2b1f,~D2=a2b1−a1c2. |
Thus in essence models (3.1) and (A.1) are symmetric, and it suffices to consider (3.1).
[1] |
E. Pardoux, S. G. Peng, Adapted solutions of backward differential equations, Syst. Control Lett., 14 (1990), 55–61. https://doi.org/10.1016/0304-4149(95)00024-2 doi: 10.1016/0304-4149(95)00024-2
![]() |
[2] |
N. El Karoui, S. G. Peng, M. Quenez, Backward stochastic differential equations in finance, Math. Finance, 7 (1997), 1–71. https://doi.org/10.1111/1467-9965.00022 doi: 10.1111/1467-9965.00022
![]() |
[3] |
D. Duffie, P. Geoffard, C. Skiadas, Efficient and equilibrium allocations with stochastic differential utility, J. Math. Econ., 23 (1994), 133–146. https://doi.org/10.1016/0304-4068(94)90002-7 doi: 10.1016/0304-4068(94)90002-7
![]() |
[4] |
G. Wang, H. Zhang, Mean-field backward stochastic differential equation with non-Lipschitz coefficient, Asian J. Control, 22 (2019), 1986–1994. https://doi.org/10.1002/asjc.2087 doi: 10.1002/asjc.2087
![]() |
[5] |
P. Huang, G. Wang, H. Zhang, An asymmetric information non-zero sum differential game of mean-field backward stochastic differential equation with applications, Adv. Differ. Equ., 236 (2019), 1–25. https://doi.org/10.1186/s13662-019-2166-5 doi: 10.1186/s13662-019-2166-5
![]() |
[6] | J. Yong, X. Zhou, Stochastic Controls: Hamiltonian Systems and HJB Equations, Springer-Verlag, New York, NY, USA, 1999. |
[7] | A. Bressan, B. Piccoli, Introduction to the Mathematical Theory of Control, Springfield: American Institute of Mathematical Sciences, 1 (2007). |
[8] |
N. Dokuchaev, X. Zhou, Stochastic controls with terminal contingent conditions, J. Math. Anal. Appl., 238 (1999), 143–165. https://doi.org/10.1006/JMAA.1999.6515 doi: 10.1006/JMAA.1999.6515
![]() |
[9] |
A. E. B. Lim, X. Zhou, Linear-quadratic control of backward stochastic differential equations, SIAM J. Control Optim., 40 (2001), 450–474. https://doi.org/10.1137/S0363012900374737 doi: 10.1137/S0363012900374737
![]() |
[10] |
J. Huang, G. Wang, J. Xiong, A maximum principle for partial information backward stochastic control problems with applications, SIAM J. Control Optim., 48 (2009), 2106–2117. https://doi.org/10.1137/080738465 doi: 10.1137/080738465
![]() |
[11] |
Z. Huang, Y. Wang, X. R. Wang, A mean-field optimal control for fully coupled forward-backward stochastic control systems with Lévy processes, J. Syst. Sci. Complex, 35 (2022), 205–220. https://doi.org/10.1007/s11424-021-0077-5 doi: 10.1007/s11424-021-0077-5
![]() |
[12] |
G. Wang, Z. Yu, A Pontryagin's maximum principle for non-zero sum differential games of BSDEs with applications, IEEE Trans. Autom. Control, 55 (2010), 1742–1747. https://doi.org/10.1109/TAC.2010.2048052 doi: 10.1109/TAC.2010.2048052
![]() |
[13] |
G. Wang, Z. Yu, A partial information non-zero sum differential game of backward stochastic differential equations with applications, Automatica, 48 (2012), 342–352. https://doi.org/10.1016/j.automatica.2011.11.010 doi: 10.1016/j.automatica.2011.11.010
![]() |
[14] |
G. Wang, H. Xiao, J. Xiong, A kind of linear quadratic non-zero differential game of backward stochastic differential equation with asymmetric information, Automatica, 63 (2018), 346–352. https://doi.org/10.1016/j.automatica.2018.08.019 doi: 10.1016/j.automatica.2018.08.019
![]() |
[15] |
S. Peng, Backward stochastic differential equations and applications to optimal control, Appl. Math. Optim., 27 (1993), 125–144. https://doi.org/10.1007/BF01195978 doi: 10.1007/BF01195978
![]() |
[16] |
W. Xu, Stochastic maximum principle for optimal control problem of forward and backward system, J. Aust. Math. Soc. Ser. B., 37 (1995), 172–185. https://doi.org/10.1017/S0334270000007645 doi: 10.1017/S0334270000007645
![]() |
[17] |
L. Chen, P. Zhou, H. Xiao, Backward Stackelberg games with delay and related forward-backward stochastic differential equaitons, Mathematics, 11 (2023), 2898. https://doi.org/10.3390/math11132898 doi: 10.3390/math11132898
![]() |
[18] |
Y. Zheng, J. Shi, A stackelberg game of backward stochastic differential equations with applications, J. Optim. Theory Appl., 10 (2019), 968–992. https://doi.org/10.1007/s13235-019-00341-z doi: 10.1007/s13235-019-00341-z
![]() |
[19] |
T. Wang, Backward stochastic volterra integro-differential equations and applications, SIAM J. Control Optim., 60 (2022), 2393–2419. https://doi.org/10.1137/20m1371464 doi: 10.1137/20m1371464
![]() |
[20] |
X. Li, J. Sun, J. Xiong, Linear quadratic optimal control problems for mean-field backward stochastic differential equations, Appl. Math. Optim., 80 (2019), 223–250. https://doi.org/10.1007/s00245-017-9464-7 doi: 10.1007/s00245-017-9464-7
![]() |
[21] |
L. Delong, P. Imleller, Backward stochastic differential equations with time delayed generators-results and counter examples, Ann. Appl. Probab., 20 (2010), 1512–1536. https://doi.org/10.1214/09-AAP663 doi: 10.1214/09-AAP663
![]() |
[22] | S. Tomasiello, L. Rarità, An approximation technique and a possible application for a class of delay differential equations, in Proceedings of the 34th European Modeling & Simulation Symposium (EMSS 2022), (2022), 45. https://doi.org/10.46354/i3m.2022.emss.045 |
[23] |
L. Rarità, C. D'Apice, B. Piccoli, D. Helbing, Sensitivity analysis of permeability parameters for flows on Barcelona networks, J. Differ. Equations, 249 (2010), 3110–3131. https://doi.org/10.1016/J.JDE.2010.09.006 doi: 10.1016/J.JDE.2010.09.006
![]() |
[24] |
L. Chen, J. H. Huang, Stochastic maximum principle for controlled backward delayed system via advanced stochastic differential equation, J. Optim. Theroy Appl., 167 (2015), 1112–1135. https://doi.org/10.1007/s10957-013-0386-5 doi: 10.1007/s10957-013-0386-5
![]() |
[25] |
N. Anakira, A. Alomari, I. Hashim, Optimal homotopy asymptotic method for solving delay differential equations, Math. Probl. Eng., 2013 (2013), 498902. https://doi.org/10.1155/2013/498902 doi: 10.1155/2013/498902
![]() |
[26] |
S. Wu, G. Wang, Optimal control problem of backward stochstic differential delay equation under partial information, Syst. Control Lett., 82 (2015), 71–78. https://doi.org/10.1016/j.sysconle.2015.05.008 doi: 10.1016/j.sysconle.2015.05.008
![]() |
[27] |
K. Dahl, S. E. A. Mohammed, B. Øksendal, E. Røse, Optimal control of systems with noisy memory and BSDEs with Malliavin derivatives, J. Funct. Anal., 271 (2016), 289–329. https://doi.org/10.1016/J.JFA.2016.04.031 doi: 10.1016/J.JFA.2016.04.031
![]() |
[28] | Y. Kazmerchuk, A. Swishchuk, J. Wu, A continuous-time GARCH model for stochastic volatility with delay, Can. Appl. Math. Q., 13 (2005), 123–149. |
[29] |
A. Delavarkhalafi, A. S. Fatemion Aghda, M. Tahmasebi, Maximum principle for infinite horizon optimal control of mean-field backward stochastic systems with delay and noisy memory, Int. J. Control, 95 (2020), 535–543. https://doi.org/10.1080/00207179.2020.1800822 doi: 10.1080/00207179.2020.1800822
![]() |
[30] |
J. Ma, P. Protter, J. M. Yong, Solving forward-backward stochastic differential equations explicitly–-a four step scheme, Probab. Theory Relat. Fields, 98 (1994), 339–359. https://doi.org/10.1007/BF01192258 doi: 10.1007/BF01192258
![]() |
[31] |
F. Zhang, Sufficient maximum prinicple for stochastic optimal control problems with general delays, J. Optim. Theory Appl., 192 (2022), 678–701. https://doi.org/10.1007/s10957-021-01987-9 doi: 10.1007/s10957-021-01987-9
![]() |
[32] | G. Di Nunno, B. Øksendal, F. Proske, Malliavin Calculus for Lévy Processes with Applications to Finance, Berlin: Springer, 2009. |
[33] | D. Nualart, The Malliavin Calculus and Related Topics, Berlin: Springer, 2006. |
[34] |
G. Di Nunno, T. Meyer-Brandis, B. Øksendal, F. Proske, Malliavin calculus and anticipative Itô formula for Lévy processes, Infin. Dimension. Anal. Quantum Probab. Relat. Top., 08 (2005), 235–258. https://doi.org/10.1142/S0219025705001950 doi: 10.1142/S0219025705001950
![]() |
[35] |
A. Delavarkhalafi, A. S. Fatemion Aghda, M. Tahmasebi, Maximum principle for infinite horizon optimal control of mean-field backward stochastic systems with delay and noisy memory, Int. J. Control, 95 (2022), 535–543. https://doi.org/10.1080/00207179.2020.1800822 doi: 10.1080/00207179.2020.1800822
![]() |
[36] |
H. Ma, L. Bin, Infinite horizon optimal control problem of mean-field backward stochastic delay differential equation under partial information, Eur. J. Control, 36 (2017), 43–50. https://doi.org/10.1016/j.ejcon.2017.04.001 doi: 10.1016/j.ejcon.2017.04.001
![]() |
[37] | S. Wu, Partially-observed maximum principle for backward stochastic differential delay equations, IEEE/CAA J. Autom. Sin., (2017), 1–6. https://doi.org/10.1109/JAS.2017.7510472 |
1. | Shangming Chen, Fengde Chen, Vaibhava Srivastava, Rana D. Parshad, Dynamical analysis of a Lotka–Volterra competition model with both Allee and fear effects, 2024, 17, 1793-5245, 10.1142/S1793524523500778 | |
2. | Yue Xia, Lijuan Chen, Vaibhava Srivastava, Rana D. Parshad, Stability and bifurcation analysis of a two-patch model with the Allee effect and dispersal, 2023, 20, 1551-0018, 19781, 10.3934/mbe.2023876 | |
3. | Qun Zhu, Fengde Chen, Impact of Fear on Searching Efficiency of First Species: A Two Species Lotka–Volterra Competition Model with Weak Allee Effect, 2024, 23, 1575-5460, 10.1007/s12346-024-01000-4 | |
4. | Benjamin Wacker, Jan Christian Schlüter, A non-standard finite-difference-method for a non-autonomous epidemiological model: analysis, parameter identification and applications, 2023, 20, 1551-0018, 12923, 10.3934/mbe.2023577 | |
5. | Shangming Chen, Fengde Chen, Vaibhava Srivastava, Rana D. Parshad, Dynamical Analysis of an Allelopathic Phytoplankton Model with Fear Effect, 2024, 23, 1575-5460, 10.1007/s12346-024-01047-3 | |
6. | Kwadwo Antwi-Fordjour, Sarah P. Westmoreland, Kendall H. Bearden, Dual fear phenomenon in an eco-epidemiological model with prey aggregation, 2024, 139, 2190-5444, 10.1140/epjp/s13360-024-05324-7 | |
7. | Benjamin Wacker, Qualitative Study of a Dynamical System for Computer Virus Propagation—A Nonstandard Finite‐Difference‐Methodological View, 2025, 0170-4214, 10.1002/mma.10798 |
Classical case | k>0 | |
(i) | CE (u∗,0) | Species v is competitively excluded for both small and large k. |
(ii) | CE (0,v∗) | 1) If a2b21k(b1+ka1)2<b1b2−c1c2c1, then species u is competitively excluded. |
2) Possibility of one interior saddle equilibrium, with a large level of k. | ||
3) Possibility of two positive interior equilibria, one sink and one saddle, with an intermediate level of k. | ||
(iii) | weak competition | 1) If k<kc=b2b1−c2c1a2c1 via Thm 3.1 yields co-existence. |
2) If k>kc, then species v is competitively excluded. | ||
(iv) | strong competition | Interior equilibrium always exists and is a saddle. |
Classical case | k>0 | |
(i) | CE (u∗,0) | Species v is competitively excluded for both small and large k. |
(ii) | CE (0,v∗) | 1) If a2b21k(b1+ka1)2<b1b2−c1c2c1, then species u is competitively excluded. |
2) Possibility of one interior saddle equilibrium, with a large level of k. | ||
3) Possibility of two positive interior equilibria, one sink and one saddle, with an intermediate level of k. | ||
(iii) | weak competition | 1) If k<kc=b2b1−c2c1a2c1 via Thm 3.1 yields co-existence. |
2) If k>kc, then species v is competitively excluded. | ||
(iv) | strong competition | Interior equilibrium always exists and is a saddle. |