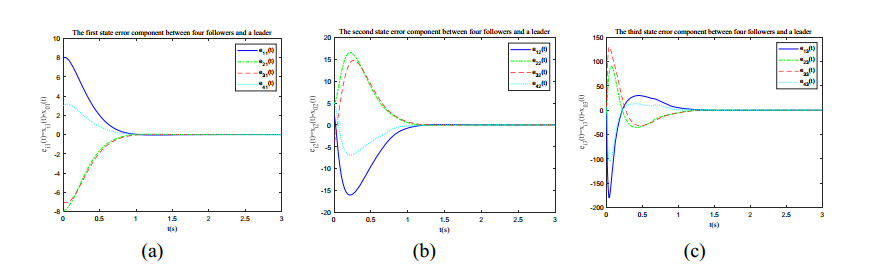
The leader-following consensus (LFC) issue is investigated in this paper for multi-agent systems (MASs) subject to actuator saturation with semi-Markov switching topologies (SMST). A new consensus protocol is proposed by using a semi-Markov process to model the switching of network topologies. Compared to the traditional Markov switching topologies, the SMST is more general and practical because the transition rates are time-varying. By using the local sector conditions and a suitable Lyapunov-Krasovskii functional, some sufficient conditions are proposed such that the leaderfollowing mean-square consensus is locally achieved. Based on the derived sufficient conditions, an optimization problem is analyzed to determine the consensus feedback gains and to find a maximal estimate of the domain of consensus attraction (DOCA) of a closed-loop model. At the end, a numerical case is presented to verify the performance of the design method.
Citation: Jiangtao Dai, Ge Guo. A leader-following consensus of multi-agent systems with actuator saturation and semi-Markov switching topologies[J]. Mathematical Biosciences and Engineering, 2024, 21(4): 4908-4926. doi: 10.3934/mbe.2024217
[1] | Tong Guo, Jing Han, Cancan Zhou, Jianping Zhou . Multi-leader-follower group consensus of stochastic time-delay multi-agent systems subject to Markov switching topology. Mathematical Biosciences and Engineering, 2022, 19(8): 7504-7520. doi: 10.3934/mbe.2022353 |
[2] | Duoduo Zhao, Fang Gao, Jinde Cao, Xiaoxin Li, Xiaoqin Ma . Mean-square consensus of a semi-Markov jump multi-agent system based on event-triggered stochastic sampling. Mathematical Biosciences and Engineering, 2023, 20(8): 14241-14259. doi: 10.3934/mbe.2023637 |
[3] | Na Zhang, Jianwei Xia, Tianjiao Liu, Chengyuan Yan, Xiao Wang . Dynamic event-triggered adaptive finite-time consensus control for multi-agent systems with time-varying actuator faults. Mathematical Biosciences and Engineering, 2023, 20(5): 7761-7783. doi: 10.3934/mbe.2023335 |
[4] | Qiushi Wang, Hongwei Ren, Zhiping Peng, Junlin Huang . Dynamic event-triggered consensus control for nonlinear multi-agent systems under DoS attacks. Mathematical Biosciences and Engineering, 2024, 21(2): 3304-3318. doi: 10.3934/mbe.2024146 |
[5] | Xiaofeng Chen . Double-integrator consensus for a switching network without dwell time. Mathematical Biosciences and Engineering, 2023, 20(7): 11627-11643. doi: 10.3934/mbe.2023516 |
[6] | Wangming Lu, Zhiyong Yu, Zhanheng Chen, Haijun Jiang . Prescribed-time cluster practical consensus for nonlinear multi-agent systems based on event-triggered mechanism. Mathematical Biosciences and Engineering, 2024, 21(3): 4440-4462. doi: 10.3934/mbe.2024196 |
[7] | Siyu Li, Shu Li, Lei Liu . Fuzzy adaptive event-triggered distributed control for a class of nonlinear multi-agent systems. Mathematical Biosciences and Engineering, 2024, 21(1): 474-493. doi: 10.3934/mbe.2024021 |
[8] | Mingxia Gu, Zhiyong Yu, Haijun Jiang, Da Huang . Distributed consensus of discrete time-varying linear multi-agent systems with event-triggered intermittent control. Mathematical Biosciences and Engineering, 2024, 21(1): 415-443. doi: 10.3934/mbe.2024019 |
[9] | Sai Zhang, Li Tang, Yan-Jun Liu . Formation deployment control of multi-agent systems modeled with PDE. Mathematical Biosciences and Engineering, 2022, 19(12): 13541-13559. doi: 10.3934/mbe.2022632 |
[10] | Meiqiao Wang, Wuquan Li . Distributed adaptive control for nonlinear multi-agent systems with nonlinear parametric uncertainties. Mathematical Biosciences and Engineering, 2023, 20(7): 12908-12922. doi: 10.3934/mbe.2023576 |
The leader-following consensus (LFC) issue is investigated in this paper for multi-agent systems (MASs) subject to actuator saturation with semi-Markov switching topologies (SMST). A new consensus protocol is proposed by using a semi-Markov process to model the switching of network topologies. Compared to the traditional Markov switching topologies, the SMST is more general and practical because the transition rates are time-varying. By using the local sector conditions and a suitable Lyapunov-Krasovskii functional, some sufficient conditions are proposed such that the leaderfollowing mean-square consensus is locally achieved. Based on the derived sufficient conditions, an optimization problem is analyzed to determine the consensus feedback gains and to find a maximal estimate of the domain of consensus attraction (DOCA) of a closed-loop model. At the end, a numerical case is presented to verify the performance of the design method.
Distributed coordinated control of MASs has drawn vast attention in the past two decades because of its broad applications in unmanned aerial vehicles [1], unicycle-like nonholonomic robots [2], distributed sensor networks [3,4,5] and so on. Among these, consensus is often recognized as one of the most fundamental problems, whose aim is to establish a distributed protocol where all agents in the system agree on the same final state only through local information exchange [6]. In recent years, for MASs with or without leaders, consensus related issues have been studied by a large number of scholars from various perspectives [7,8,9,10]. Overall analysis indicates that the research focus in this area is primarily on the issue of leader-following consensus, and many models have been proposed to address this issue [11,12,13]. The main purpose of LFC-related research is to determine an optimized consensus protocol, enabling all followers to track the leader's status, thereby helping to reduce energy consumption and control costs [14].
In practical control systems, the actuator saturation phenomenon occurs frequently because of physical limitations and safety constraints, which may lead to closed-loop system instability or poor performance due to high sensitivity to system parameters. Therefore, studying the consensus problem of MASs with actuator saturation is of significant value. The following is a brief description of this research. Recently, a series of progress has been made for the research regarding the consensus of this type of multi-agent system. For example, [15] selected second-order MASs with input saturation and general directed communication graphs for research and analyzed its consensus. A distributed anti-windup strategy was implemented to mitigate the effects of systems with multiple saturated inputs. [16] developed a frequency-domain approach to deal with the global consensus problem for a class of general second-order MASs subject to actuator saturations. The consensus criterion with actuator saturation was established for the first time using the generalized Nyquist method. It should be noted that the above literature considers second-order MASs. This potentially limits their applicability to higher-order or different types of dynamical systems. For MASs with general linear dynamics, [17] conducted research on uncertain MASs with input saturation via a meta-morphic low gain feedback approach, and analyzed the corresponding semi-global robust tracking consensus. Based on the convex hull algorithm, [18] studied linear MASs with actuator saturation and external disturbances, and discussed the optimization modes of consensus control under different conditions. To overcome the limitation that the leader-following error cannot be applied globally, [18] used the neighbor's tracking error as an invariant ellipsoid to estimate the consensus attraction domain. Using generalized sector bounded conditions, [19] studied the network-based practical set consensus of MASs subject to input saturation. They provided an optimization algorithm to design the consensus controller gain and estimate the region of attraction. However, these results have considered consensus with actuator saturation only for fixed topology. Therefore, investigating consensus with actuator saturation and switching topology is one of the primary motivations.
Owing to the constraint of obstacles, communication zone and transmission delay, etc, the communication network of MASs is generally time-varying. Therefore, the consensus for multi-agent systems with time-varying topology attracts a great deal of attention. For example, [20] considered the leader-following consensus of linear time-varying multi-agent systems under switching topologies, and showed that the protocol using local information had better robustness under a certain degree of link failure. [21] developed an approach to solve the distributed consensus tracking problem for linear MASs with directed switching topologies. [22] considered the positive consensus for a class of MASs with average dwell time (ADT) switching. [23] investigated the guaranteed cost positive consensus for linear MASs with multiple time-varying delays and mode-dependent average dwell time (MDADT). It should be emphasized that most existing literature usually assumes that switching ways follow some specific rules, such as ADT or MDADT [21,22,23]. However, in real systems, numerous disturbances and random abrupt changes can occur, including time delays and connection failures, among others. These disturbances may result in random switching patterns. To describe these switching mechanisms, Markov processes were introduced in [24,25]. In recent years, the consensus of MASs with Markov switching topology has attracted a great deal of attention. [26,27] analyzed the consensus of multiple types of MASs and established models. Theoretical analysis shows that the dwell time in this type of switching topology follows an exponential distribution in the continuous time domain. However, research has found that in practical situations, dwell time may obey more general probability distribution, such as Weibull or Gaussian distributions. However, Markov processes cannot be used to characterize these random switching topologies [28,29]. To overcome this shortcoming, semi-Markov processes are used to model general stochastic time-varying communication topologies [30,31,32], where the sojourn-time can follow Weibull, Gaussian distributions, among others [33,34]. Owing to the relaxed restrictions on the probability distributions, semi-Markov switching topologies have significantly wider applicability than traditional Markov switching topologies. Yet, research on the consensus of MASs with SMST has been relatively scarce [35,36]. In particular, the leader-following consensus within systems featuring actuator saturation and semi-Markov switching topologies has not been studied to data, which inspired the current study.
We addresse the LFC problem of MASs with actuator saturation and SMST. The main objective is to reveal the effect of semi-Markov switching topologies and actuator saturation on consensus. First, a novel consensus protocol is proposed. Second, with this protocol and the sector bound condition, the consensus issue is transformed into the stability issue of nonlinear semi-Markov jump systems. Then, by introducing Lyapunov-Krasovskii functional and using the sector bounded condition, three sufficient conditions are obtained, which can be cast in optimization problems for the purpose of enlarging estimates of the DOCA. Our work includes three points.
1) A new consensus protocol based on SMST is put forward. Compared with conventional Markov switching topologies, the restriction that dwell time must follow an exponential distribution is removed. The SMST considered here is more general and can be used to describe more time-varying topologies.
2) A multiple Lyapunov functions approach is introduced to reduce conservatism, where each individual Lyapunov function is dependent on the system mode. This means that the matrices in the multiple Lyapunov functions have more freedom than those in a single common Lyapunov matrix.
3) In order to provide a more accurate and realistic description of the dynamics of MASs, both actuator saturation and switching topology factors were taken into account during the research process.
This research includes the following sections. In Section 2, some research on graph theory and problem formulation is presented. The major findings are presented in Section 3. Section 4 deals with the estimate of the DOCA. Finally, an example is offered in Section 5 to verify the performance of the theoretical results.
Throughout this paper, let Rn and Rm×n be the n dimensional Euclidean space and the m×n real matrix space, respectively.
In denotes an n-order identity matrix, 0m×n represents a zero matrix with m rows and n columns, and 0n denotes an n dimensional zero vector. P>0(P⩾0) means that P is positive definite (semi-definite) and symmetric. diag{P1,P2,…,Pn} stands for a block-diagonal matrix whose diagonal elements are P1,P2,…,Pn. For a symmetric matrix Q, λmin(Q) and λmax(Q) indicate the smallest and the largest eigenvalues of matrix Q, respectively. QT is the transposition of the matrix Q.A⊗B is Kronecker product of matrices A and B. In a matrix, ∗ is used to represent the term that is induced by symmetry. E{X(t)} denotes the mathematical exception of the random variable X(t). ‖x‖ is the Euclidean norm of vector x. The symbol He{X} represents X+XT. Given the vectors xi∈Rni, i=1,2,⋯,m, we use col{x1,x2,…,xm} to denote the column vector [xT1xT2⋯xTm]T.
In this paper, G=(V,E,A) is a directed graph, corresponding to the information interaction between agents. V={v1,v2,…,vN} and E denote the set of N follower agents and directed edges, respectively. A=(aij)N×N represents the relevant weighted adjacency matrix. εij∈E refers to that vi can receive data from vj, and aij>0, otherwise aij=0. In this case, vj is said to be a neighbor of vi, and the neighbor index of vi is represented by Ni={j|εij∈E}. The degree matrix of G in this paper is marked as D=diag{d1,d2,…,dN} and di=∑j∈Niaij. Hence, the Laplacian matrix of G is described as L=D−A, or L=(lij)N×N with lii=∑j∈Niaij and lij=−aij, for i≠j.
In order to facilitate the following research, a directed graph ˉG is applied to characterize the communication topology between followers and a leader agent (labeled by v0), where ˉG is based on graph G, v0 and relevant edges from v0 to the other nodes in G. The adjacency matrix of ˉG is a diagonal matrix B=diag{b1,b2,…,bN}, where bi>0 under condition of v0 is a neighbor of node vi and or else bi=0. For more graph theory, please refer to [37].
The SMST in this paper is represented a G(γ(t))=(V,E(γ(t)),A(γ(t))), where G(γ(t))∈ {G(1),G(2),⋯,G(s)}, γ(t):R+→S={1,2,…,s} stands for the semi-Markov switching signal. For any α∈S,A(α) and L(α) represent the adjacency matrix and Laplacian matrix, respectively. The probability transitions of the semi-Markov switching signal γ(t) is as follows:
Pr{γ(t+h)=β|γ(t)=α}={λαβ(h)h+o(h),α≠β,1+λαβ(h)h+o(h),α=β, | (2.1) |
in which o(h) is the higher order infinitesimal of h as h approaches zero, λαβ(h)⩾0 denotes the transition rate from mode α at time t to mode β at time t+h, and λαα(h)=−s∑β=1,α≠βλαβ(h).
Remark 1. The λαβ(h) in Eq (2.1) is a function of the sojourn-time h, in which h represents the time duration between this jump and the next jump. The term o(h) is the higher order infinitesimal of h as h approaches zero, which means that as h approaches 0, the limit of o(h)/h is 0. When λαβ(h) is equal to a constant, SMST becomes Markov switching topologies. Therefore, Markov switching topologies can be regarded as a special case of SMST.
Consider a leader-following MASs with the following dynamic model:
{˙xi(t)=Axi(t)+Bsat(ui(t)),i=1,2,…,N,˙x0(t)=Ax0(t) | (2.2) |
in which x0∈Rn represents the leader agent state, xi(t)∈Rn and ui(t)∈Rp are the ith follower agent state and control input, respectively, A and B refer to matrices with constant coefficients, sat(ui)=(sat(ui1),sat(ui2),…,sat(uip))T is a saturation function with sat(uij)=sign(uij)min{|uij|,v0}, where v0>0 is the known saturation level.
Next, the consensus protocol with SMST is considered in present research.
ui(t)=−K(γ(t)){∑j∈Niaij(γ(t))[xi(t)−xj(t)]+bi(γ(t))(xi(t)−x0(t))}, | (2.3) |
where γ(t) is the semi-Markov switching signal, the feedback gain matrix K(γ(t)) is calculated subs-equently, aij(γ(t)) is the (i,j)th element of the relevant adjacency matrix A(γ(t)), bi(γ(t))⩾0 is the coupling weight, which satisfies bi(γ(t))>0 if the data of the leader is available to the ith follower agent, or else, bi(γ(t))=0.
Denoting the error state of ith by δi(t)=xi(t)−x0(t), i=1,2,…N, then (2.3) are rewritten as
ui(t)=−K(γ(t)){∑j∈Niaij(γ(t))[δi(t)−δj(t)]+bi(γ(t))δi(t)}. | (2.4) |
Substituting (2.4) into (2.2) yields
˙δi(t)=Aδi(t)+Bsat(−K(γ(t)){∑j∈Niaij(γ(t))[δi(t)−δj(t)]+bi(γ(t))δi(t)}). | (2.5) |
Let δ(t)=col{δ1(t),δ2(t),…,δN(t)}. For γ(t)=α,α∈S, Eq (2.5) are expressed in matrix form as
˙δ(t)=(IN⊗A)δ(t)+(IN⊗B)sat(−(H(α)⊗K(α))δ(t)) | (2.6) |
where H(α)=L(α)+B(α), B(α)=diag{b1(α),b2(α),…,bN(α)}, and L(α) is the Laplace matrix.
Through the above analysis, the mean-square consensus issue of MASs (2.2) with consensus protocol (2.3) are transformed into the mean-square stability problem of semi-Markov switching system (2.6). Similar to [30], we give the following Definition 1.
Definition 1. The local LFC of MASs (2.2) with SMST is said to be achieved when for any γ0∈S and any δ(0)∈X, there is a protocol (2.3) such that the states of agents satisfy
limt→∞E‖xi(t)−x0(t)‖=0,i=1,2,…,N, | (2.7) |
where δ(0)=col{δ1(0),…,δN(0)}, and xj(0),j=0,1,2,…,N, represents the initial state of jth agent. The set X is called the DOCA in mean square sense of the MASs (2.2).
Remark 2. From Definition 1, it can be seen that the DOCA of the origin is X:={δ(0)∈RNn:limt→∞E‖xi(t)−x0(t)‖=0,i=1,2,…,N}. In general, it is very difficult to find the exact DOCA. However, one can determine a set Xρ as large as possible, such that Xρ is contained in the DOCA. In the sequel, the estimate of the DOCA is defined as follows: Xρ={δ(0)∈RNn:‖δ(0)‖⩽ρ}, where Xρ⊂X, scalar ρ>0 that will be maximized in what follows.
In order to facilitate the following discussion, we define a decentralized deadzone nonlinearity:
φ(v)=v−sat(v) | (2.8) |
and a polyhedral set:
S(v0)={v∈RNp,w∈RNp:−v0⩽v(i)−w(i)⩽v0}. | (2.9) |
Using the Eq (2.8), the system (2.6) are described as
˙δ(t)=(IN⊗A)δ(t)−(H(α)⊗BK(α))δ(t)−(IN⊗B)φ(vα) | (2.10) |
where vα=−(H(α)⊗K(α))δ(t), α=1,2,…,s.
Initially, we propose the following assumption and lemma, which can be applied in the proof of our main results.
Assumption 1 [38]. Every communication topology ˉGi, i∈S, has a directed spanning tree with the root node 0.
Lemma 1 [39]. Consider the function φ(v) defined in (2.8). If v and w belong to S(v0), then φ(v) satisfies the following inequality:
φ(v)TT(φ(v)−w)⩽0 | (2.11) |
for any diagonal positive definite matrix T∈RNp×Np.
Theorem 1. Under Assumption 1, for any initial condition belonging to the set
Xρ={δ(0)∈RNn:[maxα∈Sλmax(IN⊗P(α))]ρ2⩽1}, |
the local consensus for the MASs (2.2) with consensus protocol (2.3) can be achieved under conditons that there has a diagonal matrix T>0, matrices P(α)>0,α=1,2,…,s, and matrices G, K(α) such that the following matrix inequalities hold:
[Ξ1(α)Ξ2(α)∗−2T]<0, | (3.1) |
[IN⊗P(α)GTj∗v20]⩾0,j∈{1,2,…,Np},α∈S, | (3.2) |
where Ξ1(α)=s∑β=1λαβ(h)(IN⊗P(β))+He{IN⊗(P(α)A)−H(α)⊗(P(α)BK(α))},
Ξ2(α)=[GT−HT(α)⊗KT(α)]T−IN⊗(P(α)B). |
Proof. By setting vα=−(H(α)⊗K(α))δ(t), wα=vα+Gδ(t), where G represents a matrix of suitable dimension. According to Lemma 1, if δ(t) belongs to the following set
S(G,v0)={δ(t)∈RNp;−v0⩽Gjδ(t)⩽v0},j∈{1,2,…,Np} | (3.3) |
then, we have
φ(vα)TT(φ(vα)−vα−Gδ(t))⩽0, | (3.4) |
where Gj is the jth row of G. Define a set of ellipsoids as
ζ(P(α))={δ(t)∈RNn:δT(t)(IN⊗P(α))δ(t)⩽1}, |
where P(α), α∈S, are positive definite matrices. The ellipsoids ζ(P(α)), α∈S, are included in set (3.3) if the inequality (3.2) holds. Hence, φ(vα) satisfies the sector condition (3.4) for any δ(t)∈ζ(P(α)), α∈S.
Establish Lyapunov functional candidate as
V(δ(t),γ(t),t)=δT(t)(IN⊗P(γ(t)))δ(t), | (3.5) |
where P(γ(t))>0. Define the weak infinitesimal operator ℑ of V(δ(t),γ(t),t) as
ℑV(δ(t),γ(t),t)=limΔ→0+1Δ{E{V(δ(t+Δ),γ(t+Δ),t+Δ)|δ(t),γ(t)}−V(δ(t),γ(t),t)} | (3.6) |
For γ(t)=α, α∈S, using total probability formula and conditional expectation formula, we have
ℑV(δ(t),γ(t),t)=limΔ→0+1Δ[s∑β=1,β≠αPr{γ(t+Δ)=β|γ(t)=α}δT(t+Δ)(IN⊗P(β))δ(t+Δ)+ Pr{γ(t+Δ)=α|γ(t)=α}δT(t+Δ)(IN⊗P(α))δ(t+Δ)−δT(t)(IN⊗P(α))δ(t)] =limΔ→0+1Δ[s∑β=1,β≠αqαβ(Fα(h+Δ)−Fα(h))1−Fα(h)δT(t+Δ)(IN⊗P(β))δ(t+Δ) +1−Fα(h+Δ)1−Fα(h)δT(t+Δ)(IN⊗P(α))δ(t+Δ)−δT(t)(IN⊗P(α))δ(t)] | (3.7) |
where h is sojourn-time, qαβ is the probability intensity from mode α to mode β, and Fα(h) is the relevant distribution function of h of the system staying in mode α. If Δ is infinitely close to 0, then δ(t+Δ) are expressed as
δ(t+Δ)=δ(t)+˙δ(t)Δ+o(Δ) | (3.8) |
Applying the property of the cumulative probability distribution function [40], we get
limΔ→0Fα(h+Δ)−Fα(h)(1−Fα(h))Δ=λα(h), limΔ→0Fα(h+Δ)−Fα(h)1−Fα(h)=0 and limΔ→01−Fα(h+Δ)1−Fα(h)=1 | (3.9) |
where λα(h) means corresponding jumping from mode α.
Substituting Eqs (3.8) and (3.9) into (3.7), we obtain
ℑV(δ(t),γ(t),t)=limΔ→0+1Δ[s∑β=1,β≠αqαβ(Fα(h+Δ)−Fα(h))1−Fα(h)[Δ2˙δT(t)(IN⊗P(β))˙δ(t)+Δ˙δT(t)(IN⊗P(β)) |
×δ(t)+ΔδT(t)(IN⊗P(β))˙δ(t)+δT(t)(IN⊗P(β))δ(t)]+1−Fα(h+Δ)1−Fα(h)[Δ2˙δT(t)(IN⊗P(α))˙δ(t)+ |
Δ˙δT(t)(IN⊗P(α))δ(t)+ΔδT(t)(IN⊗P(α))˙δ(t)+δT(t)(IN⊗P(α))δ(t)]δT(t)(IN⊗P(α))δ(t)] |
=limΔ→0+[s∑β=1,β≠αqαβ(Fα(h+Δ)−Fα(h))(1−Fα(h))ΔδT(t)(IN⊗P(β))δ(t)+1−Fα(h+Δ)1−Fα(h)[˙δT(t)(IN⊗P(α))δ(t) |
+δT(t)(IN⊗P(α))˙δ(t)]+Fα(h)−Fα(h+Δ)(1−Fα(h))ΔδT(t)(IN⊗P(α))δ(t)] |
=s∑β=1,β≠αqαβλα(h)δT(t)(IN⊗P(β))δ(t)+˙δT(t)(IN⊗P(α))δ(t)+δT(t)(IN⊗P(α))˙δ(t)−λα(h)δT(t) |
×(IN⊗P(α))δ(t) |
Define λαβ(h)=qαβλα(h) for α≠β and λαα(h)=−s∑β=1,β≠αλαβ(h), then it can be obtained that
ℑV(δ(t),γ(t),t)=s∑β=1λαβ(h)δT(t)(IN⊗P(β))δ(t)+˙δT(t)(IN⊗P(α))δ(t)+δT(t)(IN⊗P(α))˙δ(t). | (3.10) |
Thus, combining (3.10) with (3.4), we obtain
ℑV(δ(t),γ(t),t)⩽s∑β=1λαβ(h)δT(t)(IN⊗P(β))δ(t)+˙δT(t)(IN⊗P(α))δ(t)+δT(t)(IN⊗P(α))˙δ(t) |
−2φ(vα)TT(φ(vα)−vα−Gδ(t)). |
Based on the matrix inequalities (3.1) and (3.2), the following inequalities can be derived
ℑV(δ(t),γ(t),t)<0. | (3.11) |
Thus, we have
ℑV(δ(t),γ(t),t)<−εδT(t)δ(t) | (3.12) |
for a sufficiently small ε>0. Integrating both sides of the inequality (3.12) from 0 to t yields the following inequality:
E{V(δ(t),γ(t))}−V(δ(0),γ(0))=E{∫t0ℑV(δ(s),γ(s))ds}<−εE{∫t0δT(s)δ(s)ds}. |
Then
E{∫t0δT(s)δ(s)ds}<1ε[V(δ(0),γ(0))−E{V(δ(t),γ(t))}]<1εV(δ(0),γ(0))⩽δT(0)Υδ(0), |
where Υ=1εmaxα∈S[λmax(IN⊗P(α))].
Taking the limit as Tf→∞ gives
limTf→∞E{∫Tf0δT(s)δ(s)ds}<δT(0)Υδ(0), |
which indicates that limTf→∞δT(s)δ(s)=0. Accordingly, Eq (2.7) holds.
From (3.5) and (3.11), it follows that
δT(t)(IN⊗P(α))δ(t)=V(t)⩽V(0)=δT(0)(IN⊗P(α))δ(0) |
Hence, for any initial condition δ(0) in Xρ, it can be infered that δT(t)(IN⊗P(α))δ(t)⩽1. This ensures that all the trajectories of δ(t) which starts from the set Xρ always remain in the domain ζ(P(α)). As a consequence, the result derived using the sector condition is valid. Based on above analysis, if conditions (3.1) and (3.2) are satisfied, one obtains ℑV(δ(t),γ(t),t)<0,∀t⩾0 for all initial condition belonging to the set Xρ. Thus, Under Assumption 1, for any initial condition in set Xρ, the local consensus for the MASs (2.2) with consensus protocol (2.3) can be obtained.
It should be emphasized that the above result concerning the consensus is valid only in a local sense. If the matrix A in the MASs (2.2) is Hurwitz, the global consensus issue can be addressed by considering G=0. The following corollary gives a sufficient condition for the MASs (2.2) to meet the requirement of global consensus.
Corollary 1. Suppose that under Assumption 1, the global consensus for the MASs (2.2) with consensus protocol (2.3) can be achieved under conditions that there has a diagonal matrix T>0, matrices P(α)>0, α=1,2,…,s, and matrices K(α) such that the following matrix inequalities hold:
[ˆΞ1(α)ˆΞ2(α)∗−2T]<0,α∈S, |
where ˆΞ1(α)=s∑β=1λαβ(h)(IN⊗P(β))+He{IN⊗(P(α)A)−H(α)⊗(P(α)BK(α))},
ˆΞ2(α)=−[HT(α)⊗KT(α)]T−IN⊗(P(α)B). |
In the next theorem, we will design a consensus controller to ensure that the local consensus for the MASs (2.2) can be reached.
Theorem 2. Under Assumption 1, if there exists a diagonal matrix ˜T>0, matrices ˜P(α)>0, α∈S, and ˜G(α), ˜K(α), such that the matrix inequalities (3.13) and (3.14) hold:
[˜Ξ1(α)˜Ξ2(α)Σ(α,h)∗−2˜T0∗∗−P2(α)]<0, | (3.13) |
[IN⊗˜P(α)˜GTj(α)∗v20]⩾0,j∈{1,2,…,Np},α∈S, | (3.14) |
where
˜Ξ1(α)=λαα(h)(IN⊗˜P(α))+He{IN⊗(A˜P(α))−H(α)⊗(B˜K(α))}, |
˜Ξ2(α)=[˜GT(α)−HT(α)⊗˜KT(α)]−(IN⊗B)˜T,Σ(α,h)=λ(α,h)P1(α),P1(α)=diag{(IN⊗˜P(α)),…,(IN⊗˜P(α))}s−1, |
λ(α,h)=[√λα1(h)…√λα,α−1(h)√λα,α+1(h)…√λα,s(h)], |
P2(α)=diag{(IN⊗˜P(1)),…,(IN⊗˜P(α−1)),(IN⊗˜P(α+1)),…,(IN⊗˜P(s))}s−1. |
Then, under Definition 1, for any initial condition in the set
Xρ={δ(0)∈RNn:[maxα∈S(λmax(IN⊗˜P−1(α)))]ρ2⩽1}, | (3.15) |
the local consensus for the MASs (2.2) with consensus protocol (2.3) can be reached. Moreover, the consensus feedback gains can be given by
K(α)=˜K(α)˜P−1(α),α=1,2,…,s. |
Proof. Defining matrix variables
˜P(α)=P−1(α),˜T=T−1,˜G(α)=G(IN⊗˜P(α)),˜K(α)=K(α)˜P(α). |
Pre- and post-multiplying (3.1) with
diag{IN⊗P−1(α),T−1}, |
we can yield
[˜Ξ1(α)+Ξ0(α)˜Ξ2(α)∗−2˜T]<0, |
that is,
[˜Ξ1(α)˜Ξ2(α)∗−2˜T]+[Σ(α,h)0]P−12(α)[ΣT(α,h)0]<0, | (3.16) |
where
˜Ξ1(α)=λαα(h)(IN⊗˜P(α))+He{IN⊗(A˜P(α))−H(α)⊗(B˜K(α))},˜Ξ2(α)=[˜GT(α)−HT(α)⊗˜KT(α)]−(IN⊗B)˜T,Ξ0(α)=s∑β=1,β≠αλαβ(h)(IN⊗˜P(α))(IN⊗P(β))(IN⊗˜P(α)). |
The inequality (3.16) is equivalent to the inequality (3.13) using the Schur complement. Pre- and post-multiplying the matrix inequalities (3.2) by diag{IN⊗˜P(α),IN⊗In}, we can get (3.14), and the proof is finished.
Due to the fact that λαβ(h) is time-varying, the inequality (3.13) is very hard to treat. Subsequenty, based on the bounds of the transition rate, the following Theorem 3 is proposed to determine the solvable conditions.
Theorem 3. Under Assumption 1, if there has a diagonal matrix ˜T>0, matrices ˜P(α)>0,α∈S, and matrices ˜G(α),˜K(α), such that (3.14) and the following LMIs hold:
[Ξ_1(α)˜Ξ2(α)Σ_(α)∗−2˜T0∗∗−P2(α)]<0, | (3.17) |
[ˉΞ1(α)˜Ξ2(α)ˉΣ(α)∗−2˜T0∗∗−P2(α)]<0, | (3.18) |
where
Ξ_1(α)=λ_αα(h)(IN⊗˜P(α))+He{IN⊗(A˜P(α))−H(α)⊗(B˜K(α))},ˉΞ1(α)=ˉλαα(h)×(IN⊗˜P(α))+He{IN⊗(A˜P(α))−H(α)⊗(B˜K(α))}, |
Σ_(α)=λ_(α)P1(α),λ_(α)=[√λ_α1…√λ_α,α−1√λ_α,α+1…√λ_α,s], |
ˉΣ(α)=ˉλ(α)P1(α),ˉλ(α)=[√ˉλα1…√ˉλα,α−1√ˉλα,α+1…√ˉλα,s], |
˜Ξ2(α),P1(α) and P2(α) are defined as in Theorem 2.
Then, under Definition 1, for all initial condition in set Xρ, the local consensus for the MASs (2.2) with (2.3) can be reached. Moreover, the consensus feedback gains can be given by
K(α)=˜K(α)˜P−1(α),α=1,2,…,s. |
Proof. The proof of Theorem 3 is similar to Theorem 2 in [40], which is omitted here.
Remark 3. In Theorem 3, a consensus controller to ensure the local consensus of the MASs (2.2) with (2.3) is designed based on the linear matrix inequality (LMI) form. From (3.14), (3.17) and (3.18), we noticed that there are (2+Np)s LMIs and 3s+1 matrix variables. By analytical calculation, the number of decision variables in Theorem 3 is [ns(n+1+2p)/2]+Np(Nns+1), where N,s,n and p denote the number of follower agents, semi-Markov switching topologies, the dimension of the agent state vector and control input vector, respectively. Obviously, as N,s,n and p increase, the computational complexity increases accordingly.
Subsequently, a less conservative result can be obtained in Corollary.
Corollary 2. Under Assumption 1, if there exists a diagonal matrix ˜T>0, matrices ˜P(α,k)>0, and matrices ˜G(α,k),˜K(α,k),α∈S, k=1,2,…,K, such that the LMIs (3.19), (3.20) and (3.21) hold:
[Ξ_1(α,k)˜Ξ2(α,k)Σ_(α,k)∗−2˜T0∗∗−P2(α,k)]<0, | (3.19) |
[ˉΞ1(α,k)˜Ξ2(α,k)ˉΣ(α,k)∗−2˜T0∗∗−P2(α,k)]<0, | (3.20) |
[IN⊗˜P(α,k)˜GTj(α,k)∗v20]⩾0,j∈{1,2,…,Np},α∈S,k=1,2,…,K, | (3.21) |
where
Ξ_1(α,k)=λ_αα,k(h)(IN⊗˜P(α,k))+He{IN⊗(A˜P(α,k))−H(α)⊗(B˜K(α,k))}, |
ˉΞ1(α,k)=ˉλαα,k(h)(IN⊗˜P(α,k))+He{IN⊗(A˜P(α,k))−H(α)⊗(B˜K(α,k))}, |
˜Ξ2(α,k)=[˜GT(α,k)−HT(α)⊗˜KT(α,k)]−(IN⊗B)˜T,Σ_(α,k)=λ_(α,k)P1(α,k), |
λ_(α,k)=[√λ_α1,k…√λ_αα−1,k√λ_αα+1,k…√λ_αs,k],ˉΣ(α,k)=ˉλ(α,k)P1(α,k), |
ˉλ(α,k)=[√ˉλα1,k…√ˉλαα−1,k√ˉλαα+1,k…√ˉλαs,k],P1(α,k)=diag{(IN⊗˜P(α,k)), |
…,(IN⊗˜P(α,k))}s−1,P2(α,k)=diag{(IN⊗˜P(1,k)),…,(IN⊗˜P(α−1,k)),(IN⊗˜P(α+1,k)), |
…,(IN⊗˜P(s,k))}s−1. |
Then, under Definition 1, for all initial condition in set
Xρ(α,k)={δ(0)∈RNn:[maxα∈S(λmax(IN⊗˜P−1(α,k)))]ρ2⩽1}, | (3.22) |
the local consensus for the MASs (2.2) with (2.3) can be reached. Moreover, the consensus feedback gains are determined by
K(α,k)=˜K(α,k)˜P−1(α,k),α∈S,k=1,2,…,K. |
Next, we will discuss the estimation and optimization of the domain of consensus attraction Xρ.
By solving the inequality (3.19), (3.20) and (3.21), we can get a set of feedback matrices K(α,k), α∈S, k=1,2,…,K, and a consensus attraction domain Xρ(α,k). However, we are more interested in finding a set of solutions such that the set of admissible initial conditions is as large as possible. In the following, an optimization procedure is proposed to maximize the set of the initial conditions, i.e., to find a maximal estimate of the DOCA. According to the Corollary 2, an optimization problem of the estimate of that were stated as follows:
min σ0 subject to{(a) LMIs (3.19) , (3.20) and (3.21) hold,(b)[σ0INnINnINnIN⊗˜P(α,k)]⩾0. | (4.1) |
Similar to the literature [41], the scalars {\sigma _0} are introduced to bound the items {\lambda _{\max }}({I_N} \otimes {\tilde P^{ - 1}}(\alpha, k)) in (3.22) for getting a maximal estimate of the DOCA for system (2.6). In fact, the satisfaction of LMI (b) implies that {\lambda _{\max }}({I_N} \otimes {\tilde P^{ - 1}}(\alpha, k)) \leqslant {\sigma _0} . Combining this inequality with (3.22), we obtain that
\rho _{\max }^2 = {1 / {(ma{x_{\alpha \in \mathcal{S}}}({\lambda _{\max }}({I_N} \otimes {{\tilde P}^{ - 1}}(\alpha , k))))}} \geqslant {1 / {{\sigma _0}}}. |
Therefore, if we minimize {\sigma _0} , then we maximize the bound {1 / {{\sigma _0}}} and thus {\rho _{\max }} tends to be larger. In other words, by solving the optimal problem (4.1), we can obtain a maximal estimate of the consensus attraction domain.
In this part, a practical example on the position tracking of multiple wheeled mobile robots [42,43] is provided to prove the validity of the proposed algorithm.
Consider the following MASs (2.2), including four followers and a leader:
\left\{ {\begin{array}{*{20}{l}} {{{\dot x}_i}(t) = A{x_i}(t) + Bsat({u_i}(t)), i = 1, 2, 3, 4, } \\ {{{\dot x}_0}(t) = A{x_0}(t)} \end{array}} \right. |
whose system matrices are given as
A = \left[ {\begin{array}{*{20}{c}} 0&1&0 \\ 0&0&{{c_i}} \\ 0&{ - {d_i}}&{ - {a_i}} \end{array}} \right], B = \left[ {\begin{array}{*{20}{c}} 0 \\ 0 \\ {{b_i}} \end{array}} \right], {x_i}(t) = \left[ {\begin{array}{*{20}{c}} {{x_{i1}}(t)} \\ {{x_{i2}}(t)} \\ {{x_{i3}}(t)} \end{array}} \right] \in {R^3}, i = 0, 1, 2, 3, 4, |
where the system parameter {f_i} = [\begin{array}{*{20}{c}} {{a_i}} & {{b_i}} & {{c_i}} & {{d_i}} \end{array}] are selected as {f_i} = [\begin{array}{*{20}{c}} 2 & {10} & 1 & {10} \end{array}] .
It is assumed that the communication topology of MASs (2.2) is semi-Markov switching and has three modes \{ \mathcal{G}(1), \mathcal{G}(2), \mathcal{G}(3)\} . For convenience, the communication topology graph is omitted here. In fact, it is also easy to get this graph from the following matrix. The corresponding Laplacian matrix and leader adjacency matrix are given directly as follows:
L(1) = \left[ {\begin{array}{*{20}{c}} 0&0&0&0 \\ { - 1}&1&0&0 \\ 0&{ - 1}&1&0 \\ { - 1}&0&0&1 \end{array}} \right], L(2) = \left[ {\begin{array}{*{20}{c}} 1&{ - 1}&0&0 \\ 0&0&0&0 \\ { - 1}&0&1&0 \\ 0&0&{ - 1}&1 \end{array}} \right], L(3) = \left[ {\begin{array}{*{20}{c}} 1&0&{ - 1}&0 \\ 0&0&0&0 \\ 0&0&0&0 \\ { - 1}&0&0&1 \end{array}} \right], |
\mathcal{B}({\text{1}}) = diag\{ 1, 1, 0, 0\} , \mathcal{B}({\text{2}}) = diag\{ 1, 1, 0, 0\} , \mathcal{B}({\text{3}}) = diag\{ 0, 1, 1, 0\} . |
The transition rates in that are time-varying, and we can assume that they have the following upper and lower bounds:
{\lambda _{11}}(h) \in ( - 7.4, - 1.3), {\lambda _{12}}(h) \in (0.5, 3), {\lambda _{13}}(h) \in (0.8, 4.5), {\lambda _{21}}(h) \in (0.6, 3.8), {\lambda _{22}}(h) \in ( - 9, - 1.6), |
{\lambda _{23}}(h) \in (1, 5), {\lambda _{31}}(h) \in (1.6, 5.6), {\lambda _{32}}(h) \in (0.7, 7), {\lambda _{33}}(h) \in ( - 12, - 2). |
By solving optimization (33) with {v_0} = 100 , we can obtain {\sigma _{\min }} = {\text{0}}{\text{.8892}} and consensus feedback matrices
{K_1} = \left[ {\begin{array}{*{20}{c}} {{\text{75}}{\text{.7}}}&{{\text{26}}{\text{.5}}}&{{\text{3}}{\text{.3}}} \end{array}} \right], {K_2} = \left[ {\begin{array}{*{20}{c}} {{\text{61}}{\text{.0}}}&{{\text{21}}{\text{.3}}}&{{\text{2}}{\text{.7}}} \end{array}} \right], {K_3} = \left[ {\begin{array}{*{20}{c}} {{\text{88}}{\text{.1}}}&{{\text{30}}{\text{.7}}}&{{\text{3}}{\text{.9}}} \end{array}} \right] . |
Thus, the corresponding estimation of the DOCA is {\rho _{\max }} = {1 / {\sqrt {{\sigma _{\min }}} }} = 1.0605 . The initial states of every agent as following as {x_0}(0) = {(0, 0, 0)^T}, {x_1}(0) = {(8, 2, - 3)^T}, {x_2}(0) = {(- 8, 5, - 8)^T}, {x_3}(0) = {(- 7, - 4, 5)^T}, {x_4}(0) = {(3, 5, 7)^T} . According to the above analysis and assumptions, we can plot the tracking error trajectories between the leader and the followers (see Figure (1)). The control input of four follower agents is also shown below (see Figure (2)).
As we can see from Figure 1, under the consensus protocol with SMST (2.3), the tracking errors approach zero asymptotically which means that the local consensus for the MASs (2.2) with consensus protocol (2.3) can be obtained. The semi-Markov switching signal is presented in Figure 3.
We researched the consensus issue for MASs subject to actuator saturation with SMST. By constructing a suitable mode-dependent Lyapunov-Krasovskii functional, some sufficient conditions were presented to confirm that the LFC can be locally achieved in mean-square sense. Then, with the derived sufficient condition, the design issue of the consensus controller was converted into an optimization problem aiming at enlarging the estimation the DOCA of the origin. Then, a numerical example was presented to verify the performance of the design method. In future work, there are problems that need to be solved, such as consensus of singular MASs with SMST, cooperative control of fractional order MASs with SMST, and so on.
The authors declare they have not used Artificial Intelligence (AI) tools in the creation of this article.
This work was supported by The Science & Technology Development Fund of Tianjin Education Commission for Higher Education (2019KJ142).
The authors declare there is no conflict of interest.
[1] |
J. Wang, Z. Zhou, C. Wang, Z. Ding, Cascade structure predictive observer design for consensus control with applications to UAVs formation flying, Automatica, 121 (2020), 109200. https://doi.org/10.1016/j.automatica.2020.109200 doi: 10.1016/j.automatica.2020.109200
![]() |
[2] |
X. Chen, H. Yu, F. Hao, Prescribed-time event-triggered bipartite consensus of multiagent systems, IEEE Trans. Cybern., 52 (2022), 2589–2598. https://doi.org/10.1109/TCYB.2020.3004572 doi: 10.1109/TCYB.2020.3004572
![]() |
[3] |
L. An, G. H. Yang, Distributed secure state estimation for cyber-physical systems under sensor attacks, Automatica, 107 (2019), 526–538. https://doi.org/10.1016/j.automatica.2019.06.019 doi: 10.1016/j.automatica.2019.06.019
![]() |
[4] |
X. Li, H.Wu, J. Cao, Prescribed-time synchronization in networks of piecewise smooth systems via a nonlinear dynamic event-triggered control strategy, Math. Comput. Simul., 203 (2023), 647–668. https://doi.org/10.1016/j.matcom.2022.07.010 doi: 10.1016/j.matcom.2022.07.010
![]() |
[5] |
X. Li, H.Wu, J. Cao, A new prescribed-time stability theorem for impulsive piecewise-smooth systems and its application to synchronization in networks, Appl. Math. Modell., 115 (2023), 385–397. https://doi.org/10.1016/j.apm.2022.10.051 doi: 10.1016/j.apm.2022.10.051
![]() |
[6] |
J. Jeong, Y. Lim, A. Parivallal, An asymmetric Lyapunov-Krasovskii functional approach for event-triggered consensus of multi-agent systems with deception attacks, Appl. Math. Comput., 439 (2023), 127584. https://doi.org/10.1016/j.amc.2022.127584 doi: 10.1016/j.amc.2022.127584
![]() |
[7] |
D. Li, L. Su, H. Shen, J. Wang, Leader-following consensus of semi-Markov jump nonlinear multi-agent systems under hybrid cyber-attacks, J. Frankl. Inst., 360 (2023), 5878–5891. https://doi.org/10.1016/j.jfranklin.2023.03.017 doi: 10.1016/j.jfranklin.2023.03.017
![]() |
[8] |
R. Wang, Adaptive output-feedback time-varying formation tracking control for multi-agent systems with switching directed networks, J. Frankl. Inst., 357 (2020), 551–568. https://doi.org/10.1016/j.jfranklin.2019.11.077 doi: 10.1016/j.jfranklin.2019.11.077
![]() |
[9] |
J. Yang, G. Cao, W. X. Chen, W. D. Zhang, Finite-time formation control of second-order linear multi-agent systems with relative state constraints: A barrier function sliding mode control approach, IEEE Trans. Circuits Syst. II Express Briefs, 69 (2022), 1253–1256. https://doi.org/10.1109/TCSII.2021.3098031 doi: 10.1109/TCSII.2021.3098031
![]() |
[10] |
Y. Tian, S. Tian, H. Li, Q. Han, X. Wang, Event-triggered security consensus for multi-agent systems with Markov switching topologies under DoS attacks, Energies, 15 (2022), 5353. https://doi.org/10.3390/en15155353 doi: 10.3390/en15155353
![]() |
[11] |
J. Shao, W. X. Zheng, L. Shi, Y. Cheng, Leader-follower flocking for discrete-time Cucker-Smale models with lossy links and general weight functions, IEEE Trans. Autom. Control, 66 (2020), 4945–4951. https://doi.org/10.1109/TAC.2020.3046695 doi: 10.1109/TAC.2020.3046695
![]() |
[12] |
J. Wang, Y. Wang, H. Yan, J. Cao, H. Shen, Hybrid event-based leader-following consensus of nonlinear multiagent systems with semi-Markov jump parameters, IEEE Syst. J., 16 (2020), 397–408. https://doi.org/10.1109/JSYST.2020.3029156 doi: 10.1109/JSYST.2020.3029156
![]() |
[13] |
J. L. Liu, T. T. Yin, D. Yue, H. R. Karimi, J. D. Cao, Event-based secure leader-following consensus control for multiagent systems with multiple cyber attacks, IEEE Trans. Cybern., 51 (2021), 162–173. https://doi.org/10.1109/TCYB.2020.2970556 doi: 10.1109/TCYB.2020.2970556
![]() |
[14] |
N. H. A. Nguyen, S. H. Kim, Leader-following consensus for multi-agent systems with asynchronous control modes under nonhomogeneous Markovian jump network topology, IEEE Access, 8 (2020), 203017–203027. https://doi.org/10.1109/ACCESS.2020.3036447 doi: 10.1109/ACCESS.2020.3036447
![]() |
[15] |
J. Fu, Y. Lv, T. Huang, Distributed anti-windup approach for consensus tracking of second-order multi-agent systems with input saturation, Syst. Control Lett., 130 (2019), 1–6. https://doi.org/10.1016/j.sysconle.2019.06.002 doi: 10.1016/j.sysconle.2019.06.002
![]() |
[16] |
C. Gao, Z. Wang, X. He, Q. L. Han, On consensus of second-order multiagent systems with actuator saturations: A generalized-Nyquist-criterion-based approach, IEEE Trans. Cybern., 52 (2020), 9048–9058. https://doi.org/10.1109/TCYB.2020.3025824 doi: 10.1109/TCYB.2020.3025824
![]() |
[17] |
B. Wang, W. Chen, B. Zhang, Semi-global robust tracking consensus for multi-agent uncertain systems with input saturation via metamorphic low-gain feedback, Automatica, 103 (2019), 363–373. https://doi.org/10.1016/j.automatica.2019.02.002 doi: 10.1016/j.automatica.2019.02.002
![]() |
[18] |
C. Deng, G. H. Yang, Consensus of linear multiagent systems with actuator saturation and external disturbances, IEEE Trans. Circuits Syst. II Express Briefs, 64 (2017), 284–288. https://doi.org/10.1109/TCSII.2016.2551549 doi: 10.1109/TCSII.2016.2551549
![]() |
[19] |
L. Ding, W. X. Zheng, G. Guo, Network-based practical set consensus of multi-agent systems subject to input saturation, Automatica, 89 (2018), 316–324. https://doi.org/10.1016/j.automatica.2017.12.001 doi: 10.1016/j.automatica.2017.12.001
![]() |
[20] |
J. Jiang, Y. Jiang, Leader-following consensus of linear time-varying multi-agent systems under fixed and switching topologies, Automatica, 113 (2020), 108804. https://doi.org/10.1016/j.automatica.2020.108804 doi: 10.1016/j.automatica.2020.108804
![]() |
[21] |
G. Wen, W. X. Zheng, On constructing multiple Lyapunov functions for tracking control of multiple agents with switching topologies, IEEE Trans. Autom. Control, 64 (2018), 3796–3803. https://doi.org/10.1109/TAC.2018.2885079 doi: 10.1109/TAC.2018.2885079
![]() |
[22] |
X. Cao, Y. Li, Positive consensus for multi-agent systems with average dwell time switching, J. Frankl. Inst., 358 (2021), 8308–8329. https://doi.org/10.1016/j.jfranklin.2021.08.024 doi: 10.1016/j.jfranklin.2021.08.024
![]() |
[23] |
X. Cao, C. Zhang, D. Zhao, Y. Li, Guaranteed cost positive consensus for multi-agent systems with multiple time-varying delays and MDADT switching, Nonlinear Dyn., 107 (2022), 3557–3572. https://doi.org/10.1007/s11071-021-07157-w doi: 10.1007/s11071-021-07157-w
![]() |
[24] |
H. Shen, X. Hu, J. Wang, J. Cao, W. Qian, Non-fragile H∞ synchronization for Markov jump singularly perturbed coupled neural networks subject to double-layer switching regulation, IEEE Trans. Neural Networks Learn. Syst., 34 (2021), 2682–2692. https://doi.org/10.1109/TNNLS.2021.3107607 doi: 10.1109/TNNLS.2021.3107607
![]() |
[25] |
F. Li, S. Xu, B. Zhang, Resilient asynchronous H∞ control for discrete-time Markov jump singularly perturbed systems based on hidden Markov model, IEEE Trans. Syst., Man, Cybern., Syst., 50 (2018), 2860–2869. https://doi.org/10.1109/TSMC.2018.2837888 doi: 10.1109/TSMC.2018.2837888
![]() |
[26] |
B. Li, G.Wen, Z. Peng, S. Wen, T. Huang, Time-varying formation control of general linear multi-agent systems under Markovian switching topologies and communication noises, IEEE Trans. Circuits Syst. II Express Briefs, 68 (2021), 1303–1307. https://doi.org/10.1109/TCSII.2020.3023078 doi: 10.1109/TCSII.2020.3023078
![]() |
[27] |
H. Guo, M. Meng, G. Feng, Mean square leader-following consensus of heterogeneous multi-agent systems with Markovian switching topologies and communication delays, Int. J. Robust Nonlinear Control, 33 (2023), 355–371. https://doi.org/10.1002/rnc.6456 doi: 10.1002/rnc.6456
![]() |
[28] |
K. Liang, W. He, J. Xu, F. Qian, Impulsive effects on synchronization of singularly perturbed complex networks with semi-Markov jump topologies, IEEE Trans. Syst., Man, Cybern., Syst., 52 (2022), 3163–3173. https://doi.org/10.1109/TSMC.2021.3062378 doi: 10.1109/TSMC.2021.3062378
![]() |
[29] |
D. Zhao, F. Gao, J. Cao, X. Li, X. Ma, Mean-square consensus of a semi-Markov jump multi-agent system based on event-triggered stochastic sampling, Math. Biosci. Eng., 20 (2023), 14241–14259. https://doi.org/10.3934/mbe.2023637 doi: 10.3934/mbe.2023637
![]() |
[30] |
H. Shen, Y. Wang, J. Xia, J. H. Park, Z. Wang, Fault-tolerant leader-following consensus for multi-agent systems subject to semi-Markov switching topologies: An event-triggered control scheme, Nonlinear Anal. Hybrid. Syst., 34 (2019), 92–107. https://doi.org/10.1016/j.nahs.2019.05.003 doi: 10.1016/j.nahs.2019.05.003
![]() |
[31] |
X. Xiao, X. Wu, X. Hou, Y. He, Almost sure exponential consensus of linear and nonlinear multi-agent systems under semi-markovian switching topologies and application to synchronization of chaos systems, IEEE Access, 11 (2023), 126775–126781. https://doi.org/10.1109/ACCESS.2023.3331745 doi: 10.1109/ACCESS.2023.3331745
![]() |
[32] |
R. Sakthivel, A. Parivallal, N. Huy Tuan, S. Manickavalli, Nonfragile control design for consensus of semi‐Markov jumping multiagent systems with disturbances, Int. J. Adapt. Control Signal Process., 35 (2021), 1039–1061. https://doi.org/10.1002/acs.3245 doi: 10.1002/acs.3245
![]() |
[33] |
H. Shen, M. Xing, H. Yan, J. Cao, Observer-based L2-L∞ control for singularly perturbed semi-Markov jump systems with an improved weighted TOD protocol, Sci. China Inf. Sci., 65 (2022), 199204. https://doi.org/10.1007/s11432-021-3345-1 doi: 10.1007/s11432-021-3345-1
![]() |
[34] |
F. Li, W. X. Zheng, S. Xu, Stabilization of discrete-time hidden semi-Markov jump singularly perturbed systems with partially known emission probabilities, IEEE Trans. Autom. Control, 67 (2021), 4234–4240. https://doi.org/10.1109/TAC.2021.3113471 doi: 10.1109/TAC.2021.3113471
![]() |
[35] |
J. Dai, G. Guo, Exponential consensus of non-linear multi-agent systems with semi-Markov switching topologies, IET Control Theory Appl., 11 (2017), 3363–3371. https://doi.org/10.1049/iet-cta.2017.0562 doi: 10.1049/iet-cta.2017.0562
![]() |
[36] |
J. Dai, G. Guo, Event-triggered leader-following consensus for multi-agent systems with semi-Markov switching topologies, Inf. Sci., 459 (2018), 290–301. https://doi.org/10.1016/j.ins.2018.04.054 doi: 10.1016/j.ins.2018.04.054
![]() |
[37] | C. Godsil, G. Royle, Algebraic Graph Theory, Springer-Verlag, New York, 2001. https://doi.org/10.1007/978-1-4613-0163-9 |
[38] |
Z. Hu, X. Mu, Impulsive consensus of stochastic multi-agent systems under semi-Markovian switching topologies and application, Automatica, 150 (2023), 110871. https://doi.org/10.1016/j.automatica.2023.110871 doi: 10.1016/j.automatica.2023.110871
![]() |
[39] |
M. G. da Silva, S. Tarbouriech, Antiwindup design with guaranteed regions of stability: An LMI-based approach, IEEE Trans. Autom. Control, 50 (2005), 106–111. https://doi.org/10.1109/TAC.2004.841128 doi: 10.1109/TAC.2004.841128
![]() |
[40] |
J. Huang, Y. Shi, Stochastic stability and robust stabilization of semi-Markov jump linear systems, Int. J. Robust Nonlinear Control, 23 (2013), 2028–2043. https://doi.org/10.1002/rnc.2862 doi: 10.1002/rnc.2862
![]() |
[41] |
D. Zhang, L.Yu, Fault-tolerant control for discrete-time switched linear systems with time-varying delay and actuator saturation, J. Optim. Theory Appl., 153 (2012), 157–176. https://doi.org/10.1007/s10957-011-9955-7 doi: 10.1007/s10957-011-9955-7
![]() |
[42] |
Y. Cai, H. Zhang, Z. Gao, L. Yang, Q. He, Adaptive bipartite event-triggered time-varying output formation tracking of heterogeneous linear multi-agent systems under signed directed graph, IEEE Trans. Neural Netw. Learn. Syst., 34 (2023), 7049–7058. https://doi.org/10.1109/TIE.2017.2772196 doi: 10.1109/TIE.2017.2772196
![]() |
[43] |
D. Zhang, Z. Xu, H. R. Karimi, Q. G. Wang, L. Yu, Distributed H∞ output-feedback control for consensus of heterogeneous linear multiagent systems with aperiodic sampled-data communications, IEEE Trans. Ind. Electron., 65 (2017), 4145–4155. https://doi.org/10.1109/TIE.2017.2772196 doi: 10.1109/TIE.2017.2772196
![]() |