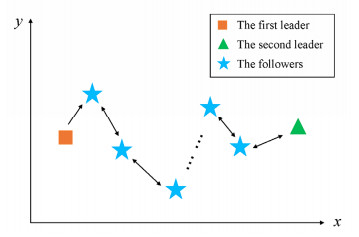
In this paper, the formation control problem of PDE-based multi-agent systems (MASs) is discussed. Firstly, the MASs are developed on a one-dimensional chain topology based on the polar coordinate system, and the dynamics of MASs is simulated using the spatial-varying coefficient wave equation. Secondly, a boundary control scheme is proposed by combining PDE-backstepping technique and the Volterra integral transformation. The well-posedness of kernel function is proved by using the iterative and inductive methods. Then, the stability of the closed-loop system is proved by using Lyapunov direct method. Finally, the PDE model is discretized using the finite difference method, and the distributed cooperative control protocol is obtained, in which the followers only need to know the location information of themselves and their neighbors. With this control protocol, leaders drive the MAS to stabilize in the desired formation. Both theoretical analysis and numerical simulation prove that the proposed control scheme is effective.
Citation: Sai Zhang, Li Tang, Yan-Jun Liu. Formation deployment control of multi-agent systems modeled with PDE[J]. Mathematical Biosciences and Engineering, 2022, 19(12): 13541-13559. doi: 10.3934/mbe.2022632
[1] | Meiqiao Wang, Wuquan Li . Distributed adaptive control for nonlinear multi-agent systems with nonlinear parametric uncertainties. Mathematical Biosciences and Engineering, 2023, 20(7): 12908-12922. doi: 10.3934/mbe.2023576 |
[2] | Xinxin Zhang, Huaiqin Wu . Bipartite consensus for multi-agent networks of fractional diffusion PDEs via aperiodically intermittent boundary control. Mathematical Biosciences and Engineering, 2023, 20(7): 12649-12665. doi: 10.3934/mbe.2023563 |
[3] | Siyu Li, Shu Li, Lei Liu . Fuzzy adaptive event-triggered distributed control for a class of nonlinear multi-agent systems. Mathematical Biosciences and Engineering, 2024, 21(1): 474-493. doi: 10.3934/mbe.2024021 |
[4] | Na Zhang, Jianwei Xia, Tianjiao Liu, Chengyuan Yan, Xiao Wang . Dynamic event-triggered adaptive finite-time consensus control for multi-agent systems with time-varying actuator faults. Mathematical Biosciences and Engineering, 2023, 20(5): 7761-7783. doi: 10.3934/mbe.2023335 |
[5] | Jinxin Du, Lei Liu . Adaptive fuzzy fixed time formation control of state constrained nonlinear multi-agent systems against FDI attacks. Mathematical Biosciences and Engineering, 2024, 21(3): 4724-4741. doi: 10.3934/mbe.2024207 |
[6] | Tong Guo, Jing Han, Cancan Zhou, Jianping Zhou . Multi-leader-follower group consensus of stochastic time-delay multi-agent systems subject to Markov switching topology. Mathematical Biosciences and Engineering, 2022, 19(8): 7504-7520. doi: 10.3934/mbe.2022353 |
[7] | Qiushi Wang, Hongwei Ren, Zhiping Peng, Junlin Huang . Dynamic event-triggered consensus control for nonlinear multi-agent systems under DoS attacks. Mathematical Biosciences and Engineering, 2024, 21(2): 3304-3318. doi: 10.3934/mbe.2024146 |
[8] | Tongyu Wang, Yadong Chen . Event-triggered control of flexible manipulator constraint system modeled by PDE. Mathematical Biosciences and Engineering, 2023, 20(6): 10043-10062. doi: 10.3934/mbe.2023441 |
[9] | Duoduo Zhao, Fang Gao, Jinde Cao, Xiaoxin Li, Xiaoqin Ma . Mean-square consensus of a semi-Markov jump multi-agent system based on event-triggered stochastic sampling. Mathematical Biosciences and Engineering, 2023, 20(8): 14241-14259. doi: 10.3934/mbe.2023637 |
[10] | Jianxin Yang, Zhipeng Qiu, Xue-Zhi Li . Global stability of an age-structured cholera model. Mathematical Biosciences and Engineering, 2014, 11(3): 641-665. doi: 10.3934/mbe.2014.11.641 |
In this paper, the formation control problem of PDE-based multi-agent systems (MASs) is discussed. Firstly, the MASs are developed on a one-dimensional chain topology based on the polar coordinate system, and the dynamics of MASs is simulated using the spatial-varying coefficient wave equation. Secondly, a boundary control scheme is proposed by combining PDE-backstepping technique and the Volterra integral transformation. The well-posedness of kernel function is proved by using the iterative and inductive methods. Then, the stability of the closed-loop system is proved by using Lyapunov direct method. Finally, the PDE model is discretized using the finite difference method, and the distributed cooperative control protocol is obtained, in which the followers only need to know the location information of themselves and their neighbors. With this control protocol, leaders drive the MAS to stabilize in the desired formation. Both theoretical analysis and numerical simulation prove that the proposed control scheme is effective.
In recent years, as the development of complex systems theory and its wide application in the fields of transportation systems, UAVs, satellites, vehicles and control engineering, the cooperative control of MASs has been extensively researched [1,2,3]. Consensus control and formation control are the primary issues studied in cooperative control. Consensus control emphasizes multiple agents converging to the same target state [4,5,6,7], while formation control is the design of a cooperative control scheme to move the agents to multiple target states, which in turn form the desired formation. Hence, formation control technology is widely applied to radar, navigation, aerial photography, military exercises, and other fields [8,9].
In formation studies, the majority of MASs are modeled by ordinary differential equations (ODEs) based on graph theoretic Laplace consistent action [10,11,12,13,14]. However, when the number of agents approaches infinity, it is difficult to achieve the control goal using ODEs modeling. In addition, since the Laplace operator in PDEs has the same consistent action as the Laplace consistent control law, more and more scholars have applied the continuum model based on PDEs to describe MASs with interactions in recent years [15,16,17,18]. In [19], the dynamics of a MAS described by a Burgers-type PDE was considered and an extended Luenberger state observer was designed. It is also combined with motion planning and stability feedback control to enable the MASs to perform the desired formation in finite time. In [20], the MAS was modeled by the reaction-advection-diffusion equation, and the control laws of the agents were designed along with the PDE-backstepping method, which stabilizes the agents in the desired formation under a fixed communication topology. In [21], a modeling, analysis and control design approach for large MASs deployed in three-dimensional space was presented. Based on two reaction-convection-diffusion two-dimensional PDEs, the issue of stabilizing PDEs by boundary control on a disk was innovatively solved using a new explicit backstepping kernel containing a Poisson kernel. In [22], the MASs in finite time were deployed into planar formation profiles along predefined spatio-temporal paths with use of formal power series, appropriate summation and generalized sequence transformations.
The commonly adopted control methods for formation control problems of the MASs are the leader-follower method [23], the behavior-based method [24], and the virtual leader method [25]. In this paper, the leader-follower method will be used, in which followers only need to know the relative position information of itself and its neighbors, and leaders hold the global information and drive followers to the position of the desired formation in combination with the controller, so that the MASs stabilizes in the desired formation [26]. There are two main types of desired formations, static and time-varying formations. Static formation refers to the formation of agents in a fixed formation under the action of a control law [27]. In particular, time-varying formation means that the agents form a specified formation and move along a certain trajectory [28]. The static formation deployment issue under the grid-grid two-dimensional cylindrical surface topology in polar coordinates was studied in [29]. In addition, the formation of MASs under arbitrarily large boundary input delays was innovatively investigated in [30]. The leader agents were affected by input delays caused by communication, measurement, and actuator constraints, so the authors designed boundary control laws to compensate for them and to drive all agents into the desired formation. In [31], the time-varying formation control for MASs was discussed. The PDE-backstepping technique was applied to design the boundary controller and observer, which transform it into a leader-driven model using only local information, thus converging the MASs to the desired time-varying formation. In [32], a large-scale MASs formation tracking control based on wave equations was studied. Employing the leader-following model, a control algorithm was developed and the robustness of the control law to velocity measurement disturbances was discussed.
The main idea of dealing with the formation control problem for large-scale MASs modeled by PEDs is that [33]: 1) The discrete agents are modeled as a continuum and the MASs dynamic equation are established based on PDEs; 2) The control is designed based on PDEs; 3) The PDE system and controller are discretized to realize the revivification from PDEs to the agents themselves. Theoretically, provided the number of agents is large enough, then this approach is independent of the number of agents and the communication structure.
In this paper, the wave equation with spatial-varying coefficient is adopted to describe the MASs and the formation control problem of MASs in polar coordinates is discussed. A boundary controller is proposed by combining the PDE-backstepping technique, Lyapunov stability theory and state feedback control. The Lyapunov direct method is employed to prove the stability of the closed-loop system. In the closed-loop system, the first leader arrives directly at the desired position, the followers only need to know the position information of themselves and their neighbors, and the second leader drives the MASs to stabilize in the desired formation under the action of this control law. The main contributions of this paper are as follows: 1) The PDEs framework considered in this paper is a second-order hyperbolic equation with spatial-varying coefficient. The parameters of the reaction term are a space-dependent function that describe more general and extensive formations of MASs. 2) A collaborative control law for the second leader at the boundary is designed by selecting the appropriate target system using the PDE-backstepping technique. 3) The existence uniqueness of the kernel function is analyzed using the variable substitution method and the iterative method, which further shows that the closed-loop system satisfies the well-posedness.
Notations: (Δ)s and (Δ)ss mean the first and second order derivatives of (Δ) in terms of the variable s, i.e., (Δ)s=∂(Δ)/∂(Δ)∂s∂s, (Δ)ss=∂2(Δ)/∂2(Δ)∂s2∂s2. Similarly, for the variable t, (Δ)t=∂(Δ)/∂(Δ)∂t∂t, (Δ)tt=∂2(Δ)/∂2(Δ)∂t2∂t2.
A chain communication structure with M nodes is considered, which is shown in Figure 1. The label k denotes the node, which is the position of the kth agent. The agents at the ends of the chain structure are leader agents, labeled 1 and M, and the others are follower agents, labeled k, (k=2,...,M−1). From [20], it is clear that the Laplace operator in PDEs has the same consensus action as the Laplace consensus control laws in ODEs. Therefore, the number of agents can be represented as continuous variables and view the cluster of agents as a continuum. When M→∞, The discrete chain topology approximates s=[G1,G2], i.e., k→sk→s. Let z(s,t)=x+iy denote the position coordinates of the agent s.
In this paper, a leader-follower MAS is studied based on wave equation with spatial-varying coefficient, whose dynamics are described as follows
ztt(s,t)=Δz+β(s)z(s,t) | (1) |
where (s,t)∈[G1,G2]×R+, with the boundary conditions
z(G1,t)=Q1(t),z(G2,t)=Q2(t)+Γ(t) | (2) |
and the initial conditions
z(s,0)=z0(s),zt(s,0)=z1(s) | (3) |
In the above equations, s and t are the independent spatial and time variables, respectively. z(s,t) represents the position of agents, and z0(s)∈H1(G1,G2),z1(s)∈L2(G1,G2). Δz=(zs(s,t)⋅s)ss=zss+zss is the Laplace operator, β(s) is a spatial-varying bounded complex function, and β(s)z(s,t) serves to describe the different formations. Q1(t),Q2(t)∈C[0,∞] are complex functions, which designate the dynamic trajectories that agents should follow. Moreover, s=G1 is the position of the first leader. During the formation changing process, it arrives directly at the desired position and sends information to its neighbors unidirectionally. At the same time, the control force Γ(t) on the second leader s=G2 is applied to communicate bilaterally with its neighbors and drive the MAS to stabilize in the desired formation.
Similarly to (1), the desired formation of the MAS is described as
zdtt(s,t)=Δzd+β(s)zd(s,t) | (4) |
with the boundary conditions
zd(G1,t)=Q1(t),zd(G2,t)=Q2(t) | (5) |
and the initial conditions
zd(s,0)=zd0(s),zdt(s,0)=zd1(s) | (6) |
where zd0(s)∈H1(G1,G2),zd1(s)∈L2(G1,G2).
Definition 1. Introducing the space H1L(G1,G2):={y∈H1(G1,G2);y(G1)=0}, H=H1(G1,G2)×L2(G1,G2) and the domain Ω={(s,α)∈R2:G1⩽α⩽s⩽G2}. The L2-norm and H1-norm are defined by ‖z‖L2=(∫G2G1z(s,t)2sds)1/122, ‖z‖H1=(∫G2G1z(s,t)2sds+∫G2G1zs(s,t)2sds)1/122.
It is important to note that for positive function β(s), the MAS (1)–(3) may be open-loop unstable. In this case, the agents will not spontaneously move to the desired position. Therefore, it is essential to design a leader-driven state feedback boundary controller.
Firstly, the error variable of the system is defined as q(s,t)=z(s,t)−zd(s,t). Then the following error system is obtained
{qtt(s,t)=Δq+β(s)q(s,t)q(G1,t)=0,q(G2,t)=Φ(t)=Γ(t)q(s,0)=q0(s),qt(s,0)=q1(s) | (7) |
The primary method applied for the control design in this paper is the PDE-backstepping method. The main idea is to first design a Volterra transformation that enables to transform the q-system (7) into a suitable stable target system (g-system). Then substituting the g-system into the Volterra transformation, the PDEs with respect to kernel functions are obtained. To proceed, using the variable substitution method and the iterative method, the existence and the uniqueness of the solution of the PDEs is proved, and then derive the boundary control law. Finally, by the exponential stability of the g-system and the invertibility of the Volterra transform, the stability of the closed-loop system q-system is guaranteed. The clearer control design process chart is presented in Figures 2 and 3, respectively.
Define the following Volterra integral transformation
g(s,t)=q(s,t)−∫sG1F(s,α)q(α,t)dα | (8) |
where F(s,α) is a kernel function defined on Ω. The kernel function F(s,α) will be suitably selected later to realize converting the unstable q-system (7) into the following target system (g-system) by using the defined Volterra integral transformation (8).
{gtt(s,t)=Δg−c(s)g(s,t)g(G1,t)=0,g(G2,t)=0g(s,0)=g0(s),gt(s,0)=g1(s) | (9) |
where c(s) satisfies c−⩽‖c(s)‖L2⩽ˉc for any s∈[G1,G2]. c−,ˉc are positive constants [34]. With simple computation, it is easy to obtain the boundary control
Φ(t)=q(G2,t)=∫G2G1F(G2,α)q(α,t)dα | (10) |
In the remainder of this section, the PDEs satisfied by the kernel F(s,α) are derived, and the existence and uniqueness of the kernel F(s,α) are analyzed.
To start with, we compute gs(s,t), gss(s,t) and gtt(s,t) respectively based on the transformation (8). Substituting (8) into (9), one gets
gs(s,t)=qs(s,t)−F(s,s)q(s,t)−∫sG1Fs(s,α)q(α,t)dα | (11) |
gss(s,t)=qss(s,t)−Fs(s,s)q(s,t)−F(s,s)qs(s,t)−dF(s,s)dsq(s,t)−∫sG1Fss(s,α)q(α,t)dα | (12) |
where dF(s,s)ds=Fs(s,s)+Fα(s,s). Calculating the second time derivative of g(s,t), one yields
gtt(s,t)=qss(s,t)+[β(s)+Fα(s,s)−F(s,s)s]q(s,t)+[1s−F(s,s)]qs(s,t)+F(s,G1)qs(G1,t)+[−Fρ(s,G1)+F(s,G1)G1]q(G1,t)+∫sG1[−Fαα(s,α)+Fα(s,α)α−F(s,α)α2−F(s,α)β(α)]q(α,t)dα | (13) |
Considering Δg−c(s)g(s,t)=gss(s,t)+gss(s,t)−c(s)g(s,t), and combining (8), (9), (11), (12) with (13), one obtains
[dF(s,s)ds+Fs(s,s)+Fα(s,s)+β(s)+c(s)]q(s,t)+F(s,G1)qs(G1,t)+[−Fα(s,G1)+F(s,G1)G1]q(G1,t)+∫sG1{Fss(s,α)−Fαα(s,α)+Fs(s,α)s+Fα(s,α)α−F(s,α)α2−[β(α)+c(s)]F(s,α)}q(α,t)dα=0 | (14) |
Hence, the following Eqs (15)–(18) hold
dF(s,s)ds+Fs(s,s)+Fα(s,s)+β(s)+c(s)=0 | (15) |
F(s,G1)=0 | (16) |
−Fα(s,G1)+F(s,G1)G1=0 | (17) |
Fss(s,α)−Fαα(s,α)+Fs(s,α)s+Fα(s,α)α−F(s,α)α2−[β(α)+c(s)]F(s,α)=0 | (18) |
To simplify the expression, define (·)(s,α)=(·) and a(s)=β(s)+c(s). Then, from (15)–(18), the following equations are derived
{Fss+Fss−Fαα+Fαα−Fα2=[β(α)+c(s)]FF(s,G1)=0F(s,s)=−12∫sG1a(τ)dτ | (19) |
Next, the well-posedness of (19) will be proved by using the variable substitution method and the iterative method, and then the boundary control law is obtained.
To prove the existence of the kernel function F(s,α), a PDE equivalent to the system (19) is derived. Firstly, the functional transformation F(s,α)=√αsw(s,α) is introduced, and the variables replacement are defined
γ=s+α,φ=s−α | (20) |
which is equivalent to
s=γ+φ2,α=γ−φ2 | (21) |
Further, define function Ψ=Ψ(γ,φ) relevant to variables γ and φ as
Ψ(γ,φ)=w(γ+φ2,γ−φ2) | (22) |
Meanwhile, let λ(γ)=−12∫γ/γ22G1a(τ)dτ. Next, based on F(s,α)=√αsw(s,α), the first and second order derivatives of the variables s and α are obtained, respectively.
{Fs=−12⋅1s√αsw+√αswsFss=34⋅1s2√αsw−1s√αsws+√αswssFα=12⋅1ρ√αsw+√αswαFαα=−14⋅1α2√αsw+1α√αswα+√αswαα | (23) |
In addition, the derivative relationship between w and Ψ can be calculated from (22)
{ws=Ψγ+Ψφwss=Ψγγ+2Ψγφ+Ψφφwα=Ψγ−Ψφwαα=Ψγγ−2Ψγφ+Ψφφ | (24) |
Substituting (24) into (23), and combining (21) with (22), the left-hand side of (18) can be rewritten as
Fss+Fss−Fαα+Fαα−Fα2=4√αsΨγφ−4γφ(γ2−φ2)2√αsΨ | (25) |
Furthermore, one obtains
[β(α)+c(s)]F=[β(α)+c(s)]√αsw=b1(γ,φ)√αsΨ | (26) |
where b1(γ,φ)=β(γ−φ2)+c(γ+φ2). Thereby, in light of (25) and (26), (19) with respect to the kernel F(s,α) is converted to
4Ψγφ−4γφ(γ2−φ2)2Ψ=b1(γ,φ)Ψ | (27) |
when γ=γ,φ=0, one has
Ψ(γ,0)=w(γ2,γ2)=F(γ2,γ2)=λ(γ) | (28) |
and when γ=φ+2G1,φ=0, one has
Ψ(φ+2G1,0)=w(φ+G1,G1)=0 | (29) |
Synthesis of (27)–(29), the PDEs equivalent to (19) is given
{4Ψγφ=b(γ,φ)ΨΨ(γ,0)=g(γ)Ψ(φ+2G1,0)=0 | (30) |
where b(γ,φ)=4γφ(γ2−φ2)2+b1(γ,φ). Next, integrating (30), first from 0 to φ for variable φ, and then from φ+2G1 to γ for variable γ, then Ψ(γ,φ) is obtained
Ψ(γ,φ)=λ(γ)−g(φ+2G1)+14∫γφ+2G1∫φ0b(τ,μ)Ψ(τ,μ)dμdτ | (31) |
Combining the above analysis, it is concluded that (31) has a solution. Further, according to the variable and function substitution (20)–(22), one yields that (31) is equivalent to (19) satisfied by the kernel function. Thus, the PDEs (19) satisfied by the kernel function has a solution. In the next step, we will focus on the uniqueness of the PDEs (19).
In the following, the iterative method is applied to prove that the PDEs (19) has a unique solution. Firstly, the function Ψ0 is defined as
Ψ0(ξ,η)=λ(ξ)−λ(η+2G1) | (32) |
and set the following recursive formula, for n∈N:
Ψn+1(γ,φ)=14∫γφ+2G1∫φ0b(τ,μ)Ψ(τ,μ)dμdτ | (33) |
Then, one obtains
|Ψ0(γ,φ)|=|λ(γ)−λ(φ+2G1)|⩽‖λ′‖L∞|φ−(φ+2G1)|⩽2G2‖λ′‖L∞ | (34) |
Next, let N=2G2‖λ′‖L∞, we assume that the following equation holds for some n∈N:
|Ψn(γ,φ)|⩽NPn(γ+φ)nn! | (35) |
Given the recursive formula (33), Ψn+1 satisfies the following relationship
|Ψn+1(γ,φ)|⩽14‖b‖L∞∫γφ+2G1∫φ0|Ψn(τ,μ)|dμdτ⩽‖b‖L∞4NPnn!∫γφ+2G1∫η0(τ+μ)ndμdτ⩽‖b‖L∞2(G2−G1)NPn(n+1)!(γ+φ)n+1 | (36) |
Therefore, it can be shown that (35) holds for a given constant P, and P=(G2−G1)‖b‖L∞2. Then, combining (36) with it, one obtains the solution of (31) as
Ψ(γ,φ)=∞∑n=0Ψn(γ,φ) | (37) |
which is a continuous function. From (31), it follows that if b is a continuous function, then Ψ∈C2. Therefore, the following conclusion is drawn.
Theorem 1. Let β(s)∈C0([G1,G2]) and c(s)∈C0([G1,G2]). Then (19) has a unique solution F∈C2(Ω).
By making s=G2 in (8), and combining the boundary conditions in (7) and (9), the control law Φ(t) of the q-system as is derived as
Φ(t)=∫G2G1F(G2,α)Q(α,t)dα | (38) |
and the control law Γ(t) of the MAS (1)–(3) is
Γ(t)=∫G2G1F(G2,α)(z(α,t)−zd(α,t))dα | (39) |
This completes the formation control design for the MAS. Next we will pay attention to the stability of MAS under the action of the formation control. Before that, it is necessary to analyze the equivalence of the original system q-system and the target system g-system. Then, it further shows that the stability of the original system q-system is ensured by proving that the target system g-system is stable.
Define the following map [35]
Ξ:H1L(G1,G2)×L2(G1,G2)→H1L(G1,G2)×L2(G1,G2)(q1,q2)↦Π(q1,q2)=(y1,y2) | (40) |
where y1,y2 are respectively defined as
y1(s)=q1(s,t)−∫SG1F(s,α)q1(α,t)dα | (41) |
y2(s)=q2(s,t)−∫SG1F(s,α)q2(α,t)dα | (42) |
The defined map is linear and continuous, thus there exists a constant K1>0, such that
‖Ξ(q1,q2)‖H1×L2⩽K1‖(q1,q2)‖H1×L2 | (43) |
The map Ξ maps solutions (q(t),qt(t)) of (44) to solutions (y(t),yt(t)):=Ξ(q(t),qt(t)) of (45).
{qtt(s,t)=qss(s,t)+qs(s,t)s+β(s)q(s,t)q(G1,t)=0,q(G2,t)=∫G2G1F(G2,α)q(α,t)dα | (44) |
{ytt(s,t)=yss(s,t)+ys(s,t)s−c(s)y(s,t)y(G1,t)=0,y(G2,t)=0 | (45) |
The map Ξ−1 that convert q-system into g-system is reversible, and the inverse transformation is
q(s,t)=y(s,t)−∫sG1J(s,α)y(α,t)dα | (46) |
Similar to the integral transformation (8), the kernel functions J(s,α) in (46) can be computed. The existence of J(s,α) can be shown by a method similar to that in Section 3.2. Therefore, the inverse map exists
Ξ−1:H1L(G1,G2)×L2(G1,G2)→H1L(G1,G2)×L2(G1,G2)(y1,y2)↦Ξ−1(y1,y2)=(q1,q2) | (47) |
Furthermore, there exists a constant K2>0 such that
‖Ξ−1(y1,y2)‖H1×L2⩽K2‖(y1,y2)‖H1×L2 | (48) |
Consequently, the norm of the q-system is equivalent to the norm of the g-system. Therefore, it is only to prove the stability of the g-system (9), which can guarantee the stability of the q-system (7).
This section will discuss the stability of g-system (9). The candidate Lyapunov function is chosen as
Π(t)=Π1(t)+Π2(t) | (49) |
where
Π1(t)=12‖gs‖2+12‖gt‖2+12‖g‖2 | (50) |
Π2(t)=δ2∫G2G1(gg∗t+g∗gt)sds | (51) |
with 0<δ<1 being a constant. To prove the stability of g-system, the following lemma is firstly presented.
Lemma 1. The upper and lower bounds of the candidate Lyapunov function Π(t) are η1Π1(t) and η2Π1(t), respectively. This means that
η1Π1(t)⩽Π(t)⩽η2Π1(t) | (52) |
where η1 and η2 are two positive constants, whose definition will be given later.
Proof. Applying Young's inequality to |Π2(t)|, one gets
|Π2(t)|=δ2∫G2G1|gg∗t+g∗gt|sds⩽δξ12∫G2G1|g|2sds+δ2ξ1∫G2G1|gt|2sds | (53) |
where ξ1>0 is a constant. Let C0=max{δξ1,δ/δξ1ξ1}, and one gets |Π2(t)|⩽C0Π1(t). Therefore, it is satisfied that C0<1 by choosing δ small enough, and then
(1−C0)Π1(t)⩽Π(t)⩽(1+C0)Π1(t) | (54) |
Define η1=1−C0,η2=1+C0, then η1Π1(t)⩽Π(t)⩽η2Π1(t) holds.
This completes the proof of Lemma 1. Following, the time derivatives for Π1(t) and Π2(t) are caculated, respectively.
Firstly, taking the derivative of Π1(t) along time, one has
˙Π1(t)=12∫G2G1(gsg∗st+g∗sgst)sds+12∫G2G1(gtg∗tt+g∗tgtt)sds+12∫G2G1(gg∗t+g∗gt)sds | (55) |
To proceed, integrating the first term by parts and substituting the target system (9) into the second term of the above equation, one gets
˙Π1(t)=−12∫G2G1(gssg∗t+g∗ssgt)sds−12∫G2G1(gsg∗t+g∗sgt)ds+12∫G2G1(gg∗t+g∗gt)sds+12∫G2G1(gtg∗ss+gtg∗ss−c(s)gtg∗)sds+12∫G2G1(g∗tgss+g∗tgss−c(s)g∗tg)sds | (56) |
Then, it is simplified to obtain that
˙Π1(t)=12∫G2G1[1−c(s)]gtg∗sds+12∫G2G1[1−c(s)]gg∗tsds | (57) |
By utilizing Young's inequality yields
˙Π1(t)⩽∫G2G1[1−c(s)]g2sds+∫G2G1[1−c(s)]g2tsds | (58) |
Taking the time derivative of Π2(t) and substituting (9) into ˙Π2(t), one has
˙Π2(t)=δ2∫G2G1(gg∗tt+g∗gtt)sds+δ∫G2G1g2tsds | (59) |
Performing integration by parts on the second term, one obtains
˙Π2(t)=12∫G2G1(gg∗ss+gg∗ss−c(s)g2)sds+δ∫G2G1g2tsds+12∫G2G1(g∗gss+g∗gss−c(s)g2)sds | (60) |
Then, simplifying it, one gets
˙Π2(t)=−δ∫G2G1g2ssds−δ∫G2G1c(s)g2sds+δ∫G2G1g2tsds | (61) |
The time derivative of (49) yields ˙Π(t)=˙Π1(t)+˙Π2(t). Combining (58) with (61), one obtains
˙Π(t)⩽−δ∫G2G1g2ssds−∫G2G1[c(s)−1−δ]g2tsds−∫G2G1[(1+δ)c(s)−1]g2sds | (62) |
Based on the assumptions of c(s) and d(s), it holds that
˙Π(t)⩽−η3Π(t) | (63) |
where η3=min{2δ,2ˉc(1+δ)−2,2(ˉc−1−δ)}>0 is a constant.
Finally, according to (52) and (63), ˙Π(t) is further expressed as
˙Π(t)⩽−ηΠ(t) | (64) |
where η=η3/η3η2η2>0.
Through the above analysis, the following lemma for the g-system is obtained.
Lemma 2. There exist constants ε1>0 and K3>0, such that for any initial condition (g0,g1)∈H1L×L2, the g-system (9) with the boundary compatibility g0(G1)=0, g1(G1)=0, g0(G2)=0 and g1(G2)=0 is exponentially stable and its solution satisfies
‖g(⋅,t),gt(⋅,t)‖H1L×L2⩽K3e−ε1t‖g0,g1‖H1L×L2 | (65) |
Proof. Multiplying eηt on both sides of (64), one has
˙Π(t)eηt⩽−ηΠ(t)eηt | (66) |
The above equation is equivalent to
ddt(Π(t)eηt)⩽0 | (67) |
Then, integrating (67) with respect to t from 0 to t, one gets
Π(t)⩽e−ηtΠ(0) | (68) |
Thus, (65) holds.
This completes the proof of Lemma 2.
Based on equivalence between the q-system and the g-system in Section 3.3, it is concluded that the q-system is also exponentially stable. Detailed conclusion is stated as follows.
Theorem 2. There exists a unique solution of the closed-loop system (7) with the control (38) and the initial condition (q0,q1)∈H1L×L2 satisfying the compatibility condition
q0(G2)=∫G2G1F(G2,α)q0(α,t)dα | (69) |
Moreover, there exists positive constants ε2 and K4, such that the q-system (7) is exponentially stable and its solution satisfies
‖q(⋅,t),qt(⋅,t)‖H1L×L2⩽K4e−ε2t‖q0,q1‖H1L×L2 | (70) |
Together with the above analysis, it is obtained that the MASs (1)–(3) are stable under the action of the boundary control law (39).
Remark 1. The differences and advantages of using PDEs to model multi-agent systems versus ODEs modeling focus on two main points. Firstly, if the ODEs are used for modeling, each agent corresponds to one ODE. As the number of agents increases, the number of ODEs also increases. Therefore, when MASs have a large number of nodes, it is difficult to add control inputs to each agent. While PDEs can model large-scale MASs based on a continuum model perspective. Secondly, the dynamics of the agent actually has spatio-temporal distribution characteristics. PDEs can not only describe the temporal characteristics, but also simulate the spatial characteristics. Thus, the PDEs-based approach has the advantage of large-scale evolution of MASs compared with the ODEs model.
Remark 2. It is worth noting that the modeling of MASs using the PDE framework must focus on the well-posedness problem of the closed-loop system (i.e., the existence of uniqueness of the system solution). In this paper, the considered MASs satisfies the well-posedness as a prerequisite for its control design. Compared with the ODE model, the PDE model involves spatial and temporal variables and is more difficult to analyze for well-posedness. In this paper, the problem of the well-posedness of MASs is properly handled and solved by using the variable substitution method and the iterative method, and the existence of uniqueness of the closed-loop system solution is guaranteed.
To verify the effectiveness of the proposed distributed control law, 31 agents are simulated on a chain topology. We consider the space step as Δs=(G2−G1)/(G2−G1)(M−1)(M−1). Thus, each agent on the chain topology can be represented as sk=G1+(k−1)Δs, k=1,2,…,M. The positions of the first leader, second leader, and followers are denoted as z1, zM and zk,k=2,…,M−1, respectively. Next, the PDE model (1) is discretized using the finite difference approximation method, which yields the control laws of leaders and followers.
The control law of the first leader is
z1=zd1 | (71) |
The control laws for followers are
¨zk(t)=zk+1−2zk+zk−1(Δs)2+1skzk+1−zk−12Δs+β(k)zk,k=2,…,M−1 | (72) |
Using Simpson's numerical integration rule, the second leader's control law is obtained as
zM=zdM+M−1∑k=1akFk(zk−zdk)+aMFM(zM−zdM) | (73) |
where ak is the Simpson coefficients, and according to Simpson's rule of numerical integration, ak is
ak={Δs3,k=1,M4Δs3,k=2,4,…,M−12Δs3,k=3,5,…,M−2 | (74) |
in which M must be chosen as an odd number according to Simpson's rule.
The topology parameters are selected as: G1=0.2 and G2=1. The spatial-varying functions are chosen as β(s)=0.154+0.075cos(5.14s)−0.0317i and c(s)=−0.042−0.075cos(5.1s)−0.03i The finite difference method is applied to discretize the hyperbolic PDE (21) satisfied by the kernel F(s,α), which leads to the kernel gain F(G2,α) of the controller, as shown in Figure 4. Then, under the distributed control laws (71)–(73), the evolution of the agents from the initial formation (circular) to the desired formation (V-shaped) is simulated. The initial position is the circle z0(s)=3cos(2πs/2πs(G2−0.18)(G2−0.18))+3isin(2πs/2πs(G2−0.18)(G2−0.18)) and the desired position is V-shaped zd(s)=(−7−3.67i)J2((17−6i)1/122s)+(5−11i)Y0((17−6i)1/122s)+3i. The first leader reaches the desired position directly. The second leader communicates with its neighbors in both directions under the action of the actuator, and thus gradually reaches the desired position with the followers. The transient evolution process is shown in Figure 5. The above analysis and the simulation results show that the proposed control scheme is effective.
In this paper, a distributed cooperative deployment scheme was constructed using the Volterra integral transformation, which is used for two-dimensional spatial deployment control of the MAS on a one-dimensional chain topology. The communication graph of agents is determined by the discretization of the PDE space derivatives, which leads to a distributed protocol in which each agent only needs to exchange information with its neighbors. The well-posedness of the kernel function in the control scheme is proved using the iterative and inductive methods, so that the feasibility of the control protocol was guaranteed. Then, the stability of the closed-loop system is illustrated by a rigorous mathematical analysis combined with Lyapunov stability theory. Finally, the effectiveness of the proposed control protocol is verified by simulation. Moreover, considering finite-time control, event-triggered control and bipartite cooperative control of MASs based on the PDE continuum model will be significant and interesting. All these issues will be our future work.
This work is supported in part by the National Natural Science Foundation of China under Grants 61973147, 62273171 and 62025303, and in part by the LiaoNing Revitalization Talents Program under Grant XLYC2007177, in part by the Natural Science Foundation of Liaoning Province under Grant 2021-MS-318.
The authors declare that there is no conflict of interest.
[1] |
Y. Liu, Y. M. Jia, An iterative learning approach to formation control of multi-agent systems, Syst. Control Lett., 61 (2012), 148-154. https://doi.org/10.1016/j.sysconle.2011.10.011 doi: 10.1016/j.sysconle.2011.10.011
![]() |
[2] |
R. Himo, M. Ogura, N. Wakamiya, Iterative shepherding control for agents with heterogeneous responsivity, Math. Biosci. Eng., 19 (2022), 3509-3525. https://doi.org/10.3934/mbe.2022162 doi: 10.3934/mbe.2022162
![]() |
[3] |
C. Wang, J. Li, H. D. Rao, A. W. Chen, J. Jiao, N. F. Zou, et al., Multi-objective grasshopper optimization algorithm based on multi-group and co-evolution, Math. Biosci. Eng., 18 (2021), 2527-2561. https://doi.org/10.3934/mbe.2021129 doi: 10.3934/mbe.2021129
![]() |
[4] |
Y. N. Chen, Z. Q. Zuo, Y. J. Wang, Bipartite consensus for a network of wave equations with time-varying disturbances, Syst. Control Lett., 136 (2020), 104604. https://doi.org/10.1016/j.sysconle.2019.104604 doi: 10.1016/j.sysconle.2019.104604
![]() |
[5] |
Y. N. Chen, Z. Q. Zuo, Y. J. Wang, Bipartite consensus for a network of wave PDEs over a signed directed graph, Automatica, 129 (2021), 109640. https://doi.org/10.1016/j.automatica.2021.109640 doi: 10.1016/j.automatica.2021.109640
![]() |
[6] |
T. Guo, J. Han, C. C. Zhou, J. P. Zhou, Multi-leader-follower group consensus of stochastic time-delay multi-agent systems subject to Markov switching topology, Math. Biosci. Eng., 19 (2022), 7504-7520. https://doi.org/10.3934/mbe.2022353 doi: 10.3934/mbe.2022353
![]() |
[7] |
S. Q. Zheng, P. Shi, S. Y. Wang, Y. Shi, Adaptive neural control for a class of nonlinear multiagent systems, IEEE Trans. Neural Netw. Learn. Syst., 32 (2020), 763-776. https://doi.org/10.1109/TNNLS.2020.2979266 doi: 10.1109/TNNLS.2020.2979266
![]() |
[8] |
Y. Wang, Z. S. Cheng, M. Xiao, UAVs' formation keeping control based on Multi-Agent system consensus, IEEE Access, 8 (2020), 49000-49012. https://doi.org/10.1109/ACCESS.2020.2979996 doi: 10.1109/ACCESS.2020.2979996
![]() |
[9] |
S. Kalantar, U. R. Zimmer, Distributed shape control of homogeneous swarms of autonomous underwater vehicles, Autonomous Robots, 22 (2007), 37-53. https://doi.org/10.1007/s10514-006-9002-y doi: 10.1007/s10514-006-9002-y
![]() |
[10] |
Z. Y. Lin, L. L. Wang, Z. M. Han, M. Y. Fu, Distributed formation control of multi-agent systems using complex Laplacian, IEEE Trans. Autom. Control, 59 (2014), 1765-1777. https://doi.org/10.1109/TAC.2014.2309031 doi: 10.1109/TAC.2014.2309031
![]() |
[11] |
L. W. Yang, L. X. Fu, P. Li, J. L. Mao, N. Guo, L. H. Du, LF-ACO: An effective formation path planning for multi-mobile robot, Math. Biosci. Eng., 19 (2022), 225-252. https://doi.org/10.3934/mbe.2022012 doi: 10.3934/mbe.2022012
![]() |
[12] |
X. W. Dong, G. Q. Hu, Time-varying formation control for general linear multi-agent systems with switching directed topologies, Automatica, 73 (2016), 47-55. https://doi.org/10.1016/j.automatica.2016.06.024 doi: 10.1016/j.automatica.2016.06.024
![]() |
[13] |
Z. Q. He, L. Yao, Improved successive approximation control for formation flying at libration points of solar-earth system, Math. Biosci. Eng., 18 (2021), 4084-4100. https://doi.org/10.3934/mbe.2021205 doi: 10.3934/mbe.2021205
![]() |
[14] |
T. Han, Z. H. Guan, M. Chi, B. Hu, T. Li, X. H. Zhang, Multi-formation control of nonlinear leader-following multi-agent systems, ISA Trans., 69 (2017), 140-147. https://doi.org/10.1016/j.isatra.2017.05.003 doi: 10.1016/j.isatra.2017.05.003
![]() |
[15] | P. Frihauf, M. Krstic, Multi-agent deployment to a family of planar arcs, in The 2010 American Control Conference. IEEE, (2010), 4109-4114. https://doi.org/10.1109/ACC.2010.5530623 |
[16] | J. Q. Wei, E. Fridman, A. Selivanov, K. H. Johansson, Multi-agent deployment under the leader displacement measurement: a PDE-based approach, in The 18th European Control Conference, IEEE, (2019), 2424-2429. https://doi.org/10.23919/ECC.2019.8796132 |
[17] |
Y. S. Zhou, N. L. Wu, H. D. Yuan, F. Pan, Z. Y. Shan, C. Wu, PDE formation and iterative docking control of USVs for the straight-line-shaped mission, J. Mar. Sci. Eng., 10 (2022), 478. https://doi.org/10.3390/jmse10040478 doi: 10.3390/jmse10040478
![]() |
[18] |
J. Kim, K. D. Kim, V. Natarajan, S. D. Kelly, J. Bentsman, PDE-based model reference adaptive control of uncertain heterogeneous multiagent networks, Nonlinear Anal. Hybr. Syst., 2 (2008), 1152-1167. https://doi.org/10.1016/j.nahs.2008.09.008 doi: 10.1016/j.nahs.2008.09.008
![]() |
[19] |
G. Freudenthaler, F. Göttsch, T. Meurer, Backstepping-based extended Luenberger observer design for a Burgers-type PDE for multi-agent deployment, in The 20th IFAC world congress, 50 (2017), 6780-6785. https://doi.org/10.1016/j.ifacol.2017.08.1196 doi: 10.1016/j.ifacol.2017.08.1196
![]() |
[20] |
P. Frihauf, M. Krstic, Leader-enabled deployment onto planar curves: A PDE-based approach, IEEE Trans. Autom. Control, 56 (2011), 1791-1806. https://doi.org/10.1109/TAC.2010.2092210 doi: 10.1109/TAC.2010.2092210
![]() |
[21] |
J. Qi, R. Vazquez, M. Krstic, Multi-agent deployment in 3-D via PDE Control, IEEE Trans. Autom. Control, 60 (2015), 891-906. https://doi.org/10.1109/TAC.2014.2361197 doi: 10.1109/TAC.2014.2361197
![]() |
[22] |
T. Meurer, M. Krstic, Finite-time multi-agent deployment: A nonlinear PDE motion planning approach, Automatica, 47 (2011), 2534-2542. https://doi.org/10.1016/j.automatica.2011.08.045 doi: 10.1016/j.automatica.2011.08.045
![]() |
[23] |
Z. J. Ji, Z. D. Wang, H. Lin, Z. Wang, Interconnection topologies for multi-agent coordination under leader-follower framework, Automatica, 45 (2009), 2857-2863. https://doi.org/10.1016/j.automatica.2009.09.002 doi: 10.1016/j.automatica.2009.09.002
![]() |
[24] |
S. El Ferik, M. T. Nasir, U. Baroudi, A behavioral adaptive fuzzy controller of multi robots in a cluster space, Appl. Soft Comput., 44 (2016), 117-127. https://doi.org/10.1016/j.asoc.2016.03.018 doi: 10.1016/j.asoc.2016.03.018
![]() |
[25] |
Q. Fu, L. L. Du, G. Z. Xu, J. R. Wu, Consensus control for multi-agent systems with distributed parameter models via iterative learning algorithm, J. Franklin Institute, 355 (2018), 4453-4472. https://doi.org/10.1016/j.jfranklin.2018.04.033 doi: 10.1016/j.jfranklin.2018.04.033
![]() |
[26] | S. Barawkar, Collaborative Transportation of A Common Payload Using Two UAVs Based on Force Feedback Control, MS thesis, University of Cincinnati, 2017. |
[27] |
T. Meurer, M. Krstic, Nonlinear PDE-based motion planning for the formation control of mobile agents, IFAC Proc. Vol., 43 (2010), 599-604. https://doi.org/10.3182/20100901-3-IT-2016.00072 doi: 10.3182/20100901-3-IT-2016.00072
![]() |
[28] |
D. Aeyels, F. De Smet, Cluster formation in a time-varying multi-agent system, Automatica, 47 (2011), 2481-2487. https://doi.org/10.1016/j.automatica.2011.08.036 doi: 10.1016/j.automatica.2011.08.036
![]() |
[29] |
J. Qi, S. X. Tang, C. Wang, Parabolic PDE-based multi-agent formation control on a cylindrical surface, Int. J. Control, 92 (2017), 1-34. https://doi.org/10.1080/00207179.2017.1308556 doi: 10.1080/00207179.2017.1308556
![]() |
[30] |
J. Qi, S. S. Wang, J. A. Fang, Control of multi-agent systems with input delay via PDE-based method, Int. J. Control, 106 (2019), 91-100. https://doi.org/10.1016/j.automatica.2019.04.032 doi: 10.1016/j.automatica.2019.04.032
![]() |
[31] |
J. Qi, J. Zhang, Y. S. Ding, Wave equation-based time-varying formation control of multi-agent systems, IEEE Trans. Control Syst. Tech., 26 (2018), 1578-1591. https://doi.org/10.1109/TCST.2017.2742985 doi: 10.1109/TCST.2017.2742985
![]() |
[32] |
S. X. Tang, J. Qi, J. Zhang, Formation tracking control for multi-agent systems: A wave-equation based approach, Int. J. Control, Autom. Syst., 15 (2017), 2704-2713. https://doi.org/10.1007/s12555-016-0562-0 doi: 10.1007/s12555-016-0562-0
![]() |
[33] | G. Freudenthaler, T. Meurer, PDE-based multi-agent formation control using flatness and backstepping: Analysis, design and robot experiments, Automatica, 115 (2020), 108897. https://doi.org/10.1016/j.automatica.2020.108897 |
[34] | X. D. Feng, Z. F. Zhang, Boundary stabilization of coupled wave system with spatially-varying coefficients and internal anti-damping, in The 39th Chinese Control Conference, (2020), 791-796. https://doi.org/10.23919/CCC50068.2020.9188546 |
[35] |
A. Smyshlyaev, E. Cerpa, M. Krstic, Boundary stabilization of a 1-D wave equation with in-domain antidamping, SIAM J. Control Optim., 48 (2010), 4014-4031. https://doi.org/10.1137/080742646 doi: 10.1137/080742646
![]() |
1. | Yukun Song, Yue Song, Yongjun Wu, Adaptive boundary control of an axially moving system with large acceleration/deceleration under the input saturation, 2023, 20, 1551-0018, 18230, 10.3934/mbe.2023810 | |
2. | Hao Chen, Zhengjie Wang, Haoran Yue, Yue Wang, Qiyuan Cheng, PDE-Based 2-D Surface Formation-Containment Control Multi-Agent Systems With α- Leader Delay-Compensated Input, 2024, 12, 2169-3536, 131458, 10.1109/ACCESS.2024.3429177 | |
3. | Jinxin Du, Lei Liu, Adaptive fuzzy fixed time formation control of state constrained nonlinear multi-agent systems against FDI attacks, 2024, 21, 1551-0018, 4724, 10.3934/mbe.2024207 |