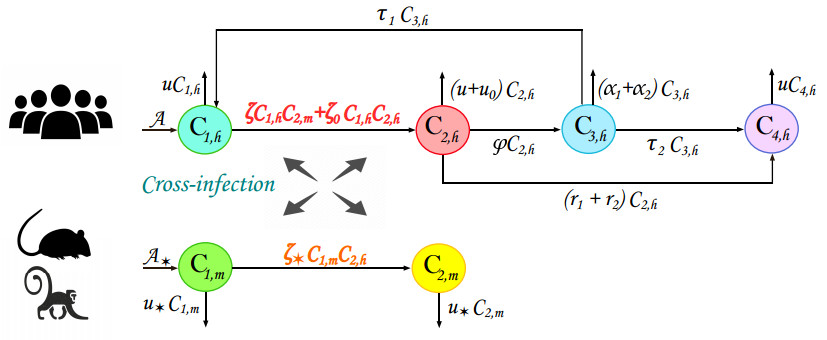
Monkeypox 2022, a new re-emerging disease, is caused by the Monkeypox virus. Structurally, this virus is related to the smallpox virus and infects the host in a similar way; however, the symptoms of Monkeypox are more severe. In this research work, a mathematical model for understanding the dynamics of Monkeypox 2022 is suggested that takes into account two modes of transmission: horizontal human dissemination and cross-infection between animals and humans. Due to lack of substantial knowledge about the virus diffusion and the effect of external perturbations, the model is extended to the probabilistic formulation with Lévy jumps. The proposed model is a two block compartmental system that requires the form of Itô-Lévy stochastic differential equations. Based on some assumptions and nonstandard analytical techniques, two principal asymptotic properties are proved: the eradication and continuation in the mean of Monkeypox 2022. The outcomes of the study reveals that the dynamical behavior of the proposed Monkeypox 2022 system is chiefly governed by some parameters that are precisely correlated with the noise intensities. To support the obtained theoretical finding, examples based on numerical simulations and real data are presented at the end of the study. The numerical simulations also exhibit the impact of the innovative adopted mathematical techniques on the findings of this work.
Citation: Asad Khan, Yassine Sabbar, Anwarud Din. Stochastic modeling of the Monkeypox 2022 epidemic with cross-infection hypothesis in a highly disturbed environment[J]. Mathematical Biosciences and Engineering, 2022, 19(12): 13560-13581. doi: 10.3934/mbe.2022633
[1] | J. Amador, D. Armesto, A. Gómez-Corral . Extreme values in SIR epidemic models with two strains and cross-immunity. Mathematical Biosciences and Engineering, 2019, 16(4): 1992-2022. doi: 10.3934/mbe.2019098 |
[2] | Yun Kang, Sourav Kumar Sasmal, Amiya Ranjan Bhowmick, Joydev Chattopadhyay . Dynamics of a predator-prey system with prey subject to Allee effects and disease. Mathematical Biosciences and Engineering, 2014, 11(4): 877-918. doi: 10.3934/mbe.2014.11.877 |
[3] | Yongli Cai, Yun Kang, Weiming Wang . Global stability of the steady states of an epidemic model incorporating intervention strategies. Mathematical Biosciences and Engineering, 2017, 14(5&6): 1071-1089. doi: 10.3934/mbe.2017056 |
[4] | Carlos M. Hernández-Suárez, Carlos Castillo-Chavez, Osval Montesinos López, Karla Hernández-Cuevas . An application of queuing theory to SIS and SEIS epidemic models. Mathematical Biosciences and Engineering, 2010, 7(4): 809-823. doi: 10.3934/mbe.2010.7.809 |
[5] | Pierre Degond, Maxime Herda, Sepideh Mirrahimi . A Fokker-Planck approach to the study of robustness in gene expression. Mathematical Biosciences and Engineering, 2020, 17(6): 6459-6486. doi: 10.3934/mbe.2020338 |
[6] | Jehad Alzabut, Ghada Alobaidi, Shah Hussain, Elissa Nadia Madi, Hasib Khan . Stochastic dynamics of influenza infection: Qualitative analysis and numerical results. Mathematical Biosciences and Engineering, 2022, 19(10): 10316-10331. doi: 10.3934/mbe.2022482 |
[7] | Jie Wang, Jian Wang, Jiafeng Cao . A heterogeneous parasitic-mutualistic model of mistletoes and birds on a periodically evolving domain. Mathematical Biosciences and Engineering, 2020, 17(6): 6678-6698. doi: 10.3934/mbe.2020347 |
[8] | Jinzhe Suo, Bo Li . Analysis on a diffusive SIS epidemic system with linear source and frequency-dependent incidence function in a heterogeneous environment. Mathematical Biosciences and Engineering, 2020, 17(1): 418-441. doi: 10.3934/mbe.2020023 |
[9] | Yongxin Gao, Shuyuan Yao . Persistence and extinction of a modified Leslie-Gower Holling-type Ⅱ predator-prey stochastic model in polluted environments with impulsive toxicant input. Mathematical Biosciences and Engineering, 2021, 18(4): 4894-4918. doi: 10.3934/mbe.2021249 |
[10] | Zongwei Ma, Hongying Shu . Viral infection dynamics in a spatial heterogeneous environment with cell-free and cell-to-cell transmissions. Mathematical Biosciences and Engineering, 2020, 17(3): 2569-2591. doi: 10.3934/mbe.2020141 |
Monkeypox 2022, a new re-emerging disease, is caused by the Monkeypox virus. Structurally, this virus is related to the smallpox virus and infects the host in a similar way; however, the symptoms of Monkeypox are more severe. In this research work, a mathematical model for understanding the dynamics of Monkeypox 2022 is suggested that takes into account two modes of transmission: horizontal human dissemination and cross-infection between animals and humans. Due to lack of substantial knowledge about the virus diffusion and the effect of external perturbations, the model is extended to the probabilistic formulation with Lévy jumps. The proposed model is a two block compartmental system that requires the form of Itô-Lévy stochastic differential equations. Based on some assumptions and nonstandard analytical techniques, two principal asymptotic properties are proved: the eradication and continuation in the mean of Monkeypox 2022. The outcomes of the study reveals that the dynamical behavior of the proposed Monkeypox 2022 system is chiefly governed by some parameters that are precisely correlated with the noise intensities. To support the obtained theoretical finding, examples based on numerical simulations and real data are presented at the end of the study. The numerical simulations also exhibit the impact of the innovative adopted mathematical techniques on the findings of this work.
Monkeypox is a contagious disease caused by an Orthopoxvirus. This zoonotic infection was initially transmitted to humans in the forest areas of central and western Africa by wild rodents; however, human-to-human spread (horizontal transmission) is also possible in this infection. The human-to-human spread is particularly observed within the family or in the context of care [1]. The Monkeypox virus can be diffused by immediate contact with lesions on the skin or mucous membranes of a sick person, as well as by droplets (sneezing, saliva, sputters, etc.) [2]. Generally, an individual can get the infection whenever he/she comes into the surrounding environment of a Monkeypox infected patient. It is therefore important to isolate such a patient throughout the duration of his/her illness. In many cases, humans are getting the infection due to their active contact with animals, rodents or monkeys [3].
Infection with the Monkeypox virus begins with a fever, often high, accompanied by headaches, body aches and weakness [4]. After about two days, a blistering rash appears, consisting of fluid-filled blisters that progress to dryness and crusting, then scarring and itching. The bubbles are most concentrated on the face, the forehands and the soles of the feet. The mucous membranes of the mouth and genital area are also affected [5], with swollen and painful lymph nodes under the jaw and neck. The disease is more severe in children and immunocompromised people. It can be complicated by secondary infection of skin lesions or damage to the respiratory, digestive, ophthalmic or nervous systems [2].
Historically, the earliest cases of the disease were discovered in the 1970s in DR Congo. The next outbreak of Monkeypox outside of Africa was detected in the United States, 2003 [6]. On 14 May 2022, the United Kingdom Health Security Agency (UKHSA) reported two cases of Monkeypox. Since then, new cases have been identified in Belgium, France, Italy, Portugal, Spain, Sweden, Austria, Canada and the United States [1]. In total, 80 mild cases were recorded in ten countries, as of May 20, according to the World Health Organization. While transmission from animals to humans is acceptable in Africa, these emerging cases are associated with human-to-human contamination, most often observed in homosexual or bisexual men with skin lesions of the genitals and face [6].
The European Center for Disease Prevention and Control (ECDPC) recently announced that there is a potentially significant risk of human-to-animal spread of Monkeypox in Europe [7]. This has already been confirmed during recent years in West Africa, in particular, when a relationship exists between infected humans and susceptible domestic animals [8]. Actually, the hypothesis of cross-infection between human and animals cannot be neglected.
In this research, we propound a mathematical formulation of Monkeypox that simulates its strong spread at two levels: human-human transmission and animal-human infection. Our model is composed of two blocks with possible cross-infection between animals and humans. The first block describes the evolution of Monkeypox in the human population, which is divided into four classes: sensitive class C1,h, infected class C2,h, the isolated class C3,h and the recovered class C4,h. The last block describes the evolution of the virus between animals, which are divided into two sub-populations: susceptible animals C1,m and infected animals C2,m. Movements between these classes are characterized by the following deterministic system:
{dC1,h(t)={A−ζC1,h(t)C2,m(t)−ζ∘C1,h(t)C2,h(t)−uC1,h(t)+τ1C3,h(t)}dt,dC2,h(t)={ζC1,h(t)C2,m(t)+ζ∘C1,h(t)C2,h(t)−(u+u0+φ+r1+r2)C2,h(t)}dt,dC3,h(t)={φC2,h(t)−(τ1+τ2+α1+α2)C3,h(t)}dt,dC4,h(t)={(r1+r2)C2,h(t)+τ2C3,h(t)−uC4,h(t)}dt,−−−−−−−−−−−−−−−−−−−−−−−−−−dC1,m(t)={A⋆−ζ⋆C1,m(t)C2,h(t)−u⋆C1,m(t)}dt,dC2,m(t)={ζ⋆C1,m(t)C2,h(t)−u⋆C2,m(t)}dt, | (1.1) |
where A designates the recruitment rate of C1,h. ζ indicates the cross infection transmission rate between C1,h and C2,m. ζ∘ is the horizontal human-human dissemination rate. τ1 is the transfer rate from C3,h to C1,h. u and u0 are, respectively, the natural and the Monkeypox-induced death rates of the human individuals. φ is the quarantine rate of infected human individuals. r1 is the human recuperation rate. r2 is the the medical treatment rate. τ2 is cure rate of isolated individuals. α1 and α2 are, respectively, the natural and the infection-induced death rates of the isolated human individuals. For the animal population, A⋆ designates the recruitment rate of C1,m. ζ⋆ is the the cross contamination rate between C1,m and C2,h. u⋆ is the animal natural death rates. For ease of reading the remainder of this manuscript, we illustrate the transmission mechanisms of the above-mentioned model by the flow diagram shown in Figure 1.
Epidemiologically, the basic reproduction ratio S0 is the number of secondary cases produced by one infected individual in an entirely susceptible population during its period as an infective. In the literature, several techniques have been proposed for the calculation of S0 ([9], chapter 5), but the most known is that of the next generation approach introduced by van den Driessche and Watmough in [10]. According to this method, which will be used in our case, S0 is the spectral radius ρ of the next generation matrix defined by M=FV−1 where F and V are, respectively, the matrices expressing the infection transition and the emergence of new infected cases in the different contaminated compartments of the model. On account of the mathematical results presented in [6], the spread behavior of the aforesaid Monkeypox model is entirely determined by the deterministic sill S0, which is expressed in this case by
S0=ζAζ⋆A⋆+ζ∘Au2⋆u(u+u0+φ+r1+r2)u2⋆. |
In fact, external environmental disturbances affect the spread of the epidemic and make its behavior difficult to predict [11,12,13,14,15,16]. In such cases, deterministic systems, while capable of making very useful predictions, are not sufficiently adequate [17,18]. Thus, there is an urgent need for a sophisticated and general mathematical model that takes into account the effect of randomness, especially in the context of studying the prevalence of a highly prevalent infectious disease such as Monkeypox [19,20]. In this context, a large number of authors have proposed and developed several stochastic models that describe the dynamics of epidemics from different angles and projections [21,22,23]. In all these previously mentioned works, the transition from the deterministic to the stochastic formula is carried out by assuming that the solution of this first naturally fluctuates around its value, which is often expressed by obfuscating some parameters of the system with white noise [24]. The addition of the latter is one of the most logical and remarkable ways of modeling any phenomenon in which a given quantity undergoes slight and continuous fluctuations [25]. For example, in the context of the spread of infectious diseases, environmental uncertainty is often represented in this way. Unfortunately, this approach is not adequate to model the impact of massive and sudden disturbances such as climate changes, economic crises, human interventions, etc. [26]. For this reason, we turn to Lévy processes, which are famous for their ability to properly formulate this type of issue. There are several reasons to enhance the classical stochastic paradigm. Models based on Lévy processes make it possible to deal with situations leading to heavy-tailed distributions. Moreover, they make it possible to exploit the full force of Markovian modeling because the most general Markov processes are solutions of stochastic differential equations driven by Lévy processes. As the Lévy processes admit jumps, they are well suited to describe complex problems in a discontinuous manner. In addition, several fractal patterns associated with white noise and Lévy jumps are observed in the living world. They seem, for example, to be present in domains as varied as biology and the economy. If the Lévy process acted at the individual level, these adaptation mechanisms could then also have effects at higher levels of organization and dynamics, even at the scale of ecosystems [27,28]. So, we can expand the model (1.1) to the probabilistic formulation bellow:
![]() |
(1.2) |
where
{dΣ1(t)=Υ1C1,h(t)dP1(t)+∫JΞ1(u)C1,h(t−)˜C[dt,du],dΣ2(t)=Υ2C2,h(t)dP2(t)+∫JΞ2(u)C2,h(t−)˜C[dt,du],dΣ3(t)=Υ3C3,h(t)dP3(t)+∫JΞ3(u)C3,h(t−)˜C[dt,du],dΣ4(t)=Υ4C4,h(t)dP4(t)+∫JΞ4(u)C4,h(t−)˜C[dt,du],dΣ5(t)=Υ5C1,m(t)dP5(t)+∫JΞ5(u)C1,m(t−)˜C[dt,du],dΣ6(t)=Υ6C2,m(t)dP6(t)+∫JΞ6(u)C2,m(t−)˜C[dt,du]. |
Here, Pℓ(ℓ=1,⋯,6), are mutually independent Wiener processes of intensities Υℓ>0(ℓ=1,⋯,6), respectively. These processes are defined on a filtered probability space (Ω,F,(Ft)t⩾0,P) endowed with a filtration that satisfies the habitual conditions. C1,h(t−),C2,h(t−),C3,h(t−),C4,h(t−),C1,m(t−) and C2,m(t−) denote the left limits of C1,h(t),C2,h(t),C3,h(t),C4,h(t),C1,m(t) and C2,m(t). C is a Poisson counting measure independent of Pℓ(ℓ=1,⋯,6) with a finite characteristic measure ϖ defined on a measurable set J⊂R+. ˜C is a compensating martingale, and it is presumed that ϖ is a specific Lévy measure that verifies ϖ(du)dt=C[dt,du]−˜C[dt,du]. Lastly, Ξℓ:J→R are the jumps, magnitude functions, which are supposed to be continuous on J.
Regarding the present paper, our principal intention is to find sufficient criteria for eradication and continuation in the mean of the Monkeypox system (1.2). These two asymptotic properties are regarded to be sufficient for having an excellent view of the Monkeypox future pandemic situation. Our new approach presented in this research improves some standard methods presented, for example, in [29,30]. Also, we adopt some new analytic techniques to get a more precise threshold for Monkeypox eradication. The analysis presented in this work seems to be very promising for studying other epidemic models, especially those which are perturbed with Lévy jumps.
The remainder of this research is ordered as follows: In Section 2, we start by giving some necessary lemmas and techniques. In Section 3, we treat the dynamical bifurcation by giving the acute criteria of eradication and continuation of the Monkeypox. Section 4 numerically validates the mathematical outcomes and explores the effect of leaps on the long-run attitude of the Monkeypox infection model (1.2).
We start this section by providing the hypothetical framework of our analysis. During this research, we presume the following.
⋆c1:∀ℓ∈{1,⋯,6}, Ξℓ(u)>−1, and the quantities
{max1⩽ℓ⩽6{∫JΞ2ℓ(u)ϖ(du)},max1⩽ℓ⩽6{∫J(Ξℓ(u)−ln(1+Ξℓ(u)))ϖ(du)},max1⩽ℓ⩽6{∫J((1+Ξℓ(u))2−1)2ϖ(du)},max1⩽ℓ⩽6{∫J(ln(1+Ξℓ(u)))2ϖ(du)}, |
are finite.
∙c2:∃v>2 such that Vv=min{u,u⋆}−0.5(v−1)U−1vWv>0, where U=max{Υ2ℓ∣ℓ∈{1,⋯,6}}, ˜Ξ(u)=max{Ξℓ(u)∣ℓ∈{1,⋯,6}}, Ξ∼(u)=min{Ξℓ(u)∣ℓ∈{1,⋯,6}}, ˜Θv(u)=(1+˜Ξ(u))v−vטΞ(u)−1, Θ∼v(u)=(1+Ξ∼(u))v−v×Ξ∼(u)−1, Θv(u)=max{˜Θv(u),Θ∼v(u)}, Wv=∫JΘv(u)ϖ(du).
Now, the first question in exploring the dynamics of an epidemic model is whether it admits a unique and positive global solution over time. The following lemma shows these properties and ensures the well-posedness of the proposed probabilistic system (1.2).
Lemma 2.1. Let c1 holds. Then, the stochastic system (1.2) is biologically and mathematically well-posed in the sense thatit has a single solution which is positive and global in time.
We define the total human population as follows: T(t)=C1,h(t)+C2,h(t)+C3,h(t)+C4,h(t). Then, we explore some long-run characteristics of the boundary equations associated with model (1.2) in the case of C2,h(t)=0 and C2,m(t)=0 (absence of Monkeypox). Because of that reason, we use the following two-block auxiliary system with quadratic Lévy noise:
{{dD(t)=(A−uD(t))dt+4∑ℓ=1dΣℓ(t),D(t)=T(0),{dD⋆(t)=(A⋆−u⋆D⋆(t))dt+Υ5D⋆(t)dP5(t)+∫JΞ5(u)D⋆(t−)˜C[dt,du],D(t)=C1,m(0). | (2.1) |
Lemma 2.2. Assume that c1 and c2 hold and let (D(t),D⋆(t)) be two Markov processes that verify the two-block auxiliary system (2.3). Then, we have thefollowing properties:
1) Θ:=2u−U−∫Jmax{˜Ξ2(u),Ξ∼2(u)}ϖ(du)>0.
2) limt→∞1t∫t0D(s)ds=Aua.s
3) limt→∞1t∫t0D2(s)ds≤2A2uΘa.s
4) Θ⋆:=2u⋆−Υ25−∫JΞ25(u)ϖ(du)>0.
5) limt→∞1t∫t0D⋆(s)ds=A⋆u⋆a.s
6) limt→∞1t∫t0D2⋆(s)ds≤2A2⋆u⋆Θ⋆a.s
The proof of this result is almost analogous to that of Lemma 2.1. in [12].
In this part, we probe the eradication condition of the Monkeypox infection. For simplicity and comfort in reading the next results, we define the following quantities:
⋆z∘,†:=max{0,z}=0.5(z+|z|), ∀z∈R.
⋆g_(u)={min{Ξ2(u),Ξ5(u)}−ln(1+min{Ξ2(u),Ξ5(u)})}×1{min{Ξ2(u),Ξ5(u)}>0}.
⋆g_(u)={max{Ξ2(u),Ξ5(u)}−ln(1+max{Ξ2(u),Ξ5(u)})}×1{max{Ξ2(u),Ξ5(u)}≤0}.
⋆H1:=∫J(¯g(u)+g_(u))ϖ(du).
⋆H2=0.5(Υ2Υ5)2Υ22+Υ25.
⋆Δ⋆=max{u+u0+φ+r1+r2,u⋆}×{S0.50−1}∘,†.
⋆Δ⋆=min{u+u0+φ+r1+r2,u⋆}×{1−S0.50}∘,†.
⋆H3=Δ⋆−Δ⋆.
Definition 3.1. The population X is said to be exponentially extinct if lim supt→∞t−1lnX(t)<0 a.s.
Theorem 3.1. The solution of the probabilistic system (1.2) verifies the following estimate:
lim supt→∞t−1ln(ζ⋆A⋆u2⋆(u+u0+φ+r1+r2)C2,h(t)+S0.50u⋆C2,m(t))⩽ςa.s, |
where
ς=H1−H2−H3+ζ∘Au+0.5u⋆S0.50(2uΘ−1)0.5+0.5(u+u0+φ+r1+r2)S0.50(2u⋆Θ⋆−1)0.5. |
Specifically, the eradication of Monkeypox infection with full probability occurs when ς<0.
Proof. First, we define the following function:
W(C2,h,C2,m)=ln(ζ⋆A⋆u2⋆(u+u0+φ+r1+r2)⏟=Z1C2,h+S0.50u⋆⏟=Z2C2,m). |
By the use of Itô's rule, we obtain
dW(C2,h(t),C2,m(t))=LItôW(C2,h(t),C2,m(t))dt+{Z1Υ2C2,h(t)dP2(t)+Z2Υ5C2,m(t)dP5(t)}Z1C2,h(t)+Z2C2,m(t)+∫Jln(1+Z1Ξ2(u)C2,h(t)+Z2Ξ5(u)C2,m(t)Z1C2,h(t)+Z2C2,m(t))˜C[dt,du], |
where
LItôW(C2,h(t),C2,m(t))=Z1(ζC1,h(t)C2,m(t)+ζ∘C1,h(t)C2,h(t)−(u+u0+φ+r1+r2)C2,h(t))Z1C2,h(t)+Z2C2,m(t)+Z2(ζ⋆C1,m(t)C2,h(t)−u⋆C2,m(t))Z1C2,h(t)+Z2C2,m(t)−0.5Z21Υ22C22,h(t)+0.5Z22Υ25C22,m(t)(Z1C2,h(t)+Z2C2,m(t))2+∫J{ln(1+Z1Ξ2(u)C2,h(t)+Z2Ξ5(u)C2,m(t)Z1C2,h(t)+Z2C2,h(t))−Z1Ξ2(u)C2,h(t)+Z2Ξ5(u)C2,m(t)Z1C2,h(t)+Z2C2,m(t)}ϖ(du). | (3.1) |
Obviously, we can see that
(Z1C2,h(t)+Z2C2,m(t))2=(1Υ2Z1Υ2C2,h(t)+1Υ5Z2Υ5C2,m(t))2⩽(1Υ22+1Υ25)(Z21Υ22C22,h(t)+Z22Υ25C22,h(t)). |
Thus,
−1(Z1C2,h(t)+Z2C2,h(t))2(Z21Υ22C22,h(t)+Z22Υ25C22,m(t))⩽−(Υ2Υ5)2Υ22+Υ25. | (3.2) |
Furthermore, we can show that
∫J{ln(Z1Ξ2(u)C2,h(t)+Z2Ξ5(u)C2,m(t)Z1C2,h(t)+Z2C2,m(t)+1)−Z1Ξ2(u)C2,h(t)+Z2Ξ5(u)C2,m(t)Z1C2,h(t)+Z2C2,m(t)}ϖ(du)⩽−H1. | (3.3) |
We amalgamate (3.2) and (3.3) with (3.1), and then
![]() |
(3.4) |
Then, we have
![]() |
Consequently, we infer that
![]() |
A simple integration leads to
W(C2,h(t),C2,m(t))t−W(C2,h(0),C2,m(0))t⩽H3−H1−H2+ζ∘t∫t0D(s)ds+Z1ζZ2t∫t0{D(s)−Au}∘,†ds+Z2ζ⋆Z1t∫t0{D⋆(s)−A⋆u⋆}∘,†ds+1t(∫t0Z1Υ2C2,h(s)Z1C2,h(s)+Z2C2,m(s)dP2(s)+∫t0Z2Υ5C2,m(s)Z1C2,h(s)+Z2C2,m(s)dP5(s))⏟:=O1(t)+1t∫t0∫Jln{1+max{Ξ2(u),Ξ5(u)}}˜C[ds,du]⏟:=O2(t). | (3.5) |
In line with Hölder's inequality, we acquire that
1t∫t0{D(s)−Au}∘,†ds=0.5t∫t0(D(s)−Au)ds+0.5t∫t0|D(s)−Au|ds⩽0.5t∫t0(D(s)−Au)ds+0.5t0.5(∫t0(D(s)−Au)2ds)0.5⩽0.5(1t∫t0D(s)ds−Au)+0.5(1t∫t0(D2(s)−2AuD(s)+A2u2)ds)0.5. |
As a result, we get
limt→∞1t∫t0{D(s)−Au}∘,†ds⩽0.5(2A2uΘ−2A2u2+A2u2)0.5=A2u(−1+2uΘ)0.5a.s. | (3.6) |
Using the same analytical treatment, we obtain
limt→∞1t∫t0{D⋆(s)−A⋆u⋆}∘,†ds⩽0.5(2A2⋆u⋆Θ⋆−2A2⋆u2⋆+A2⋆u2⋆)0.5=A⋆2u⋆(−1+2u⋆Θ⋆)0.5a.s. | (3.7) |
Now, by employing the well-known strong law of large numbers (for local martingales) and condition c1, we have
limt→∞t−1O1(t)=0andlimt→∞t−1O2(t)=0a.s. | (3.8) |
Eventually, we conclude that
lim supt→∞W(C2,h(t),C2,m(t))t⩽H3−H1−H2+ζ∘Au+Z1ζZ2A2u(2uΘ−1)0.5+Z2ζ⋆Z1A⋆2u⋆(2u⋆Θ⋆−1)0.5=H3−H1−H2+ζ∘Au+0.5u⋆S0.50(2uΘ−1)0.5+0.5(u+u0+φ+r1+r2)S0.50(2u⋆Θ⋆−1)0.5:=ς. |
In accordance with the definition of the probabilistic eradication of the infection [12], we can say that when ς is strictly negative, the Monkeypox epidemic will disappear in the population.
Remark 3.1. Needless to say, the preceding theorem implies the stochastic extinction of infected individuals, which implies in turn (by the positivity of the solution) that limt→∞C2,h(t)=0 and limt→∞C2,m(t)=0 a.s. Compared to the deterministic framework, we notice that the extinction criterion is mainly determined by the parameters closely related to the intensities of the disturbances. From the structure of the constant ς, we show that the stochastic extinction can occur although the deterministic solution is extinguishing (i.e., S0<1). Therefore, we say that the Lévy noise has negative effects on the prevalence of Monkeypox. This means that jumps can change the asymptotic behavior of the Monkeypox model significantly.
In this part, we seek to explore the sufficient criteria for the continuation of the Monkeypox epidemic. For this target, we index the following notations:
⋆I1=u+Υ212+∫J(Ξ1(u)−ln(1+Ξ1(u)))ϖ(du).
⋆I2=(u+u0+φ+r1+r2)+Υ222+∫J(Ξ2(u)−ln(1+Ξ2(u)))ϖ(du).
⋆I3=u⋆+Υ252+∫J(Ξ5(u)−ln(1+Ξ5(u)))ϖ(du).
⋆I4=u⋆+Υ262+∫J(Ξ6(u)−ln(1+Ξ6(u)))ϖ(du).
⋆˜S0:=ζζ⋆AA⋆I1I2I3I4.
Definition 3.2. The population X is said to be strongly persistent in the mean if lim inft→∞t−1∫t0X(s)ds>0 a.s.
Theorem 3.2. The sufficient condition of the Monkeypox persistence is ˜S0>1.
Proof. We define the following function:
W⋆⋆(C1,h,C2,h,C1,m,C2,m)=−Z1ln[C1,h]−Z2ln[C2,h]−Z3ln[C1,m]−Z4ln[C2,m], |
where Z1,Z3,Z4>0 are three quantities to be determined later, and Z2=1. By employing Itô's rule, we get
dW⋆⋆(C1,h(t),C2,h(t),C1,m(t),C2,m(t))=LItôW⋆⋆(C1,h(t),C2,h(t),C1,m(t),C2,m(t))dt−Z1Υ1dP1(t)−Υ2dP2(t)−Z3Υ5dP5(t)−Z4Υ6dP6(t)−Z1∫Jln(1+Ξ1(u))˜C[dt,du]−∫Jln(1+Ξ2(u))˜C[dt,du]−Z3∫Jln(1+Ξ5(u))˜C[dt,du]−Z4∫Jln(1+Ξ6(u))˜C[dt,du], |
where LItôW⋆⋆(C1,h(t),C2,h(t),C1,m(t),C2,m(t)) is expressed as follows:
![]() |
Then, we obtain
LItôW⋆⋆(C1,h(t),C2,h(t),C1,m(t),C2,m(t))⩽−Z1AC1,h(t)−ζC1,h(t)C2,m(t)C2,h−Z3A⋆C1,m(t)−Z4ζ⋆C1,m(t)C2,h(t)C2,m(t)+Z1ζC2,m(t)+(Z1ζ∘+Z3ζ⋆)C2,h(t)+Z1{u+Υ212+∫J(Ξ1(u)−ln(1+Ξ1(u)))ϖ(du)}+{(u+u0+φ+r1+r2)+Υ222+∫J(Ξ2(u)−ln(1+Ξ2(u)))ϖ(du)}+Z3(u⋆+Υ252+∫J(Ξ5(u)−ln(1+Ξ5(u)))ϖ(du))+Z4{u⋆+Υ262+∫J(Ξ6(u)−ln(1+Ξ6(u)))ϖ(du)}. |
Hence, we derive that
LItôW⋆⋆(C1,h(t),C2,h(t),C1,m(t),C2,m(t))⩽−44√Z1Z3Z4ζζ⋆AA⋆+Z1ζC2,m(t)+(Z1ζ∘+Z3ζ⋆)C2,h(t)+Z1{u+Υ212+∫J(Ξ1(u)−ln(1+Ξ1(u)))ϖ(du)}⏟=I1+{(u+u0+φ+r1+r2)+Υ222+∫J(Ξ2(u)−ln(1+Ξ2(u)))ϖ(du)}⏟=I2+Z3{u⋆+Υ252+∫J(Ξ5(u)−ln(1+Ξ5(u)))ϖ(du)}⏟=I3+Z4{u⋆+Υ262+∫J(Ξ6(u)−ln(1+Ξ6(u)))ϖ(du)}⏟=I4. |
Now, we select Z1=ζζ⋆AA⋆I21I3I4, Z3=ζζ⋆AA⋆I1I23I4 and Z4=ζζ⋆AA⋆I1I3I24, and then
LItôW⋆⋆(C1,h(t),C2,h(t),C1,m(t),C2,m(t))⩽Z1ζC2,m(t)+Z3ζ⋆C2,h(t)+I2−ζζ⋆AA⋆I1I3I4=(Z1ζC2,m(t)+(Z1ζ∘+Z3ζ⋆)C2,h(t))−I2{(ζζ⋆AA⋆I1I2I3I4⏟˜S0−1}. |
Hence,
dW⋆⋆(C1,h(t),C2,h(t),C1,m(t),C2,m(t))⩽{(Z1ζC2,m(t)+(Z1ζ∘+Z3ζ⋆)C2,h(t))−I2(˜S0−1)}dt−Z1Υ1dP1(t)−Υ2dP2(t)−Z3Υ5dP5(t)−Z4Υ6dP6(t)−Z1∫Jln(1+Ξ1(u))˜C[dt,du]−∫Jln(1+Ξ2(u))˜C[dt,du]−Z3∫Jln(1+Ξ5(u))˜C[dt,du]−Z4∫Jln(1+Ξ6(u))˜C[dt,du]. | (3.9) |
An integration from 0 to t gives
W⋆⋆(C1,h(t),C2,h(t),C1,m(t),C2,m(t))t−W⋆⋆(C1,h(0),C2,h(0),C1,m(0),C2,m(0))t⩽Z1ζt∫t0C2,m(s)ds+(Z1ζ∘+Z3ζ⋆)t∫t0C2,h(s)ds−I2(˜S0−1)−Z1Υ1P1(t)t−Υ2P2(t)t−Z3Υ5P5(t)t−Z4Υ6P6(t)t−Z1t∫t0∫Jln(1+Ξ1(u))˜C[ds,du]−∫t0∫Jln(1+Ξ2(u))˜C[ds,du]−Z3t∫t0∫Jln(1+Ξ5(u))˜C[ds,du]−Z4t∫t0∫Jln(1+Ξ6(u))˜C[ds,du]. |
So,
W⋆⋆(C1,h(t),C2,h(t),C1,m(t),C2,m(t))t−W⋆⋆(C1,h(0),C2,h(0),C1,m(0),C2,m(0))t⩽max{Z1ζ,Z1ζ∘+Z3ζ⋆}t∫t0(C2,m(s)+C2,h(s))ds−I2(˜S0−1)⩽−Z1Υ1P1(t)t−Υ2P2(t)t−Z3Υ5P5(t)t−Z4Υ6P6(t)t−Z1t∫t0∫Jln(1+Ξ1(u))˜C[ds,du]−∫t0∫Jln(1+Ξ2(u))˜C[ds,du]−Z3t∫t0∫Jln(1+Ξ5(u))˜C[ds,du]−Z4t∫t0∫Jln(1+Ξ6(u))˜C[ds,du]. |
Consequently,
max{Z1ζ,Z1ζ∘+Z3ζ⋆}t∫t0(C2,m(s)+C2,h(s))ds⩾I2(˜S0−1)+Z1Υ1P1(t)t+Υ2P2(t)t+Z3Υ5P5(t)t+Z4Υ6P6(t)t+Z1t∫t0∫Jln(1+Ξ1(u))˜C[ds,du]+∫t0∫Jln(1+Ξ2(u))˜C[ds,du])+Z3t∫t0∫Jln(1+Ξ5(u))˜C[ds,du]+Z4t∫t0∫Jln(1+Ξ6(u))˜C[ds,du]−W⋆⋆(C1,h(t),C2,h(t),C1,m(t),C2,m(t))t+W⋆⋆(C1,h(0),C2,h(0),C1,m(0),C2,m(0))t. | (3.10) |
By taking the inferior limit, we infer that
lim inft→∞1t∫t0(C2,m(s)+C2,h(s))ds⩾I2(˜S0−1)max{Z1ζ,Z1ζ∘+Z3ζ⋆}>0a.s., |
that is, the continuation of the Monkeypox infection occurs when ˜S0>1.
Remark 3.2. Persistence in the mean is an important concept in mathematical epidemiology. It captures the long-term survival of Monkeypox even when the population size is quite low at t=0. Moreover, the persistence of the model refers to a situation where Monkeypox is endemic in a population.
In order to check and support the diverse analytical outcomes presented in this research, we implement some numerical examples by taking the deterministic and probabilistic parameter values from the simulated data presented in Table 1. All trajectories of the analyzed Monkeypox model are numerically simulated in our case with the following initial information: C1,h(0)=120,C2,h(0)=70,C3,h(0)=20,C4,h(0)=10,C1,m(0)=130 and C2,m(0)=50. In the following, the unit of time is 1 day, and the number of human and animal individuals is 1 million.
Parameter | Test 1 | Test 2 | Test 3 |
A | 10 | 10 | 10 |
ζ | 0.0001 | 0.0009 | 0.001 |
ζ∘ | 0.0002 | 0.0002 | 0.0002 |
u | 0.05 | 0.05 | 0.05 |
τ1 | 0.32 | 0.32 | 0.32 |
τ2 | 0.2 | 0.2 | 0.2 |
u0 | 0.0003 | 0.0003 | 0.0003 |
φ | 0.5 | 0.5 | 0.5 |
r1 | 0.041 | 0.041 | 0.041 |
r2 | 0.043 | 0.043 | 0.043 |
α1 | 0.004 | 0.004 | 0.004 |
α2 | 0.002 | 0.002 | 0.002 |
A⋆ | 10 | 10 | 10 |
ζ⋆ | 0.00027 | 0.00027 | 0.00027 |
u⋆ | 0.02 | 0.02 | 0.02 |
Υ1 | 0.2 | 0.2 | 0.2 |
Υ2 | 0.4 | 0.4 | 0.4 |
Υ3 | 0.1 | 0.1 | 0.1 |
Υ4 | 0.2 | 0.2 | 0.2 |
Υ5 | 0.1 | 0.1 | 0.1 |
Υ6 | 0.2 | 0.2 | 0.2 |
Ξ1(u) | 0.3 | 0.3 | 0.3 |
Ξ2(u) | 0.3 | 0.3 | 0.3 |
Ξ3(u) | 0.3 | 0.3 | 0.3 |
Ξ4(u) | 0.3 | 0.3 | 0.3 |
Ξ5(u) | 0.3 | 0.3 | 0.3 |
Ξ6(u) | 0.3 | 0.3 | 0.3 |
In this example, by choosing the parameter values presented in the second column, Test 1, in Table 1, and also taking J=R+ with ϖ(J)=1, we will examine the theoretical results of Theorem 3.1. By performing some calculations, we get S0=0.2759<1 and ς=−0.0478<0. So, by the virtue of Theorem 3.1, the Monkeypox pandemic will be eradicated almost surely, which is exactly shown in Figure 2. Now, we select the parameters from the third column, Test 2, in Table 1. Then, we obtain S0=1.9786>1 and ς=−0.0013<0. From Theorem 3.1, we confirm the eradication of Monkeypox, which is depicted in Figure 3. We note from this figure that Poisson jumps lead to a lack of infection spreading, while the deterministic system predicts steady continuation. So, heavy jumps have passive influence on the diffusion of Monkeypox. This indicates that random fluctuations can notably alter the future of the epidemiological situation.
In this part, we will illustrate the continuation of the Monkeypox infection. By selecting the parameters from Test 3 in Table 1, we can directly get ˜S0=1.2370>1. Consequently, by Theorem 3.2, the Monkeypox epidemic is persistent in the mean, which agrees well with the results shown in Figure 4.
In this paper, we analyzed a generalized stochastic Monkeypox epidemic model with cross-infection between animals and humans. Due to the complexity of disease, we captured the dynamics of the Monkeypox virus as a compartmental system that assumed the form of an Itô-Lévy stochastic differential equations system. Initially, we developed a deterministic system based on some assumptions and characteristics of the disease. We applied the nonstandard analytical techniques for proving two principal asymptotic properties: the eradication and continuation in the mean of Monkeypox 2022. The system is then converted to a stochastic model, and a number of sophisticated mathematical analyse are carried out that offer many insights related to Monkeypox propagation, including, notably, the results related to the long-run behavior. The main mathematical and biological results of this paper are as follows:
1) We have provided sufficient and threshold results for the Monkeypox eradication (ς<0).
2) We have proved results which ensure the continuation of the Monkeypox illness (˜S0>1).
Roughly speaking, our obtained outcomes show that the eradication and permanence conditions rely fundamentally on white noise and leaps' intensities. Analytically, it was noticed that the obtained results enhance our comprehension related to the dynamics of the Monkeypox virus, which makes this paper more appropriate in providing a rich ground for further studies, especially in understanding the reappearance of the Monkeypox disease in many countries around the globe.
One limitation of this paper is that we obtained two separate critical conditions for extinction and persistence, which is less than the ideal when it comes to epidemiological models. Therefore, there is a significant gap between the defined criteria and the corresponding threshold value, which is still considered an open question. The authors are curious to deal with this problem in future work.
This research was sponsored by the Guangzhou Government Project under, Grant No: 62216235.
The authors declare no conflicts of interest.
[1] | WHO, Monkeypox, 2022. Abstract from: https://www.who.int/news-room/fact-sheets/detail/monkeypox. |
[2] | N. P. Singh, S. Sharma, G. Ghai, A. Singh, A systematic review on epidermology of human Monkeypox virus, Ann. Rom. Soc. Cell Biol., 25 (2021), 602–610. |
[3] |
M. J. Oladoye, Monkeypox: A neglected viral zoonotic disease, Electron. J. Med. Edu. Technol., 14 (2021), 2108. https://doi.org/10.30935/ejmets/10911 doi: 10.30935/ejmets/10911
![]() |
[4] | S. Deresinski, A case of Monkeypox in a returned traveler, Infect. Dis. Alert, 41 (2022). |
[5] | V. Costello, M. Sowash, A. Gaur, M. Cardis, H. Pasieka, G. Wortmann, et al., Imported Monkeypox from international traveler, Maryland, USA, 2021, Emerg. Infect. Dis., 28 (2022), 1002–1005. https://doi.org/10.3201%2Feid2805.220292 |
[6] |
O. J. Peter, S. Kumar, N. Kumari, F. A. Oguntolu, K. Oshinubi, R. Musa, Transmission dynamics of Monkeypox virus: A mathematical modelling approach, Model. Earth Syst. Environ., 8 (2022), 3423–3434. https://doi.org/10.1007/s40808-021-01313-2 doi: 10.1007/s40808-021-01313-2
![]() |
[7] | WHO, Risk assessment: Monkeypox multi-country outbreak, 2022. Available from: https://www.ecdc.europa.eu/en/publications-data/risk-assessment-monkeypox-multi-country-outbreak. |
[8] |
E. Petersen, A. Kantele, M. Koopmans, D. A. A. Yinka-Ogunleye, C. Ihekweazu, A. Zumla, Human Monkeypox: Epidemiologic and clinical characteristics, diagnosis, and prevention, Infect. Dis. Clin., 33 (2019), 1027–1043. https://doi.org/10.1016/j.idc.2019.03.001 doi: 10.1016/j.idc.2019.03.001
![]() |
[9] | M. Martcheva, An Introduction to Mathematical Epidemiology, Springer, 2015. |
[10] |
P. Van den Driessche, J. Watmough, Reproduction numbers and sub-threshold endemic equilibria for compartmental models of disease transmission, Math. Biosci., 180 (2002), 29–48. https://doi.org/10.1016/S0025-5564(02)00108-6 doi: 10.1016/S0025-5564(02)00108-6
![]() |
[11] |
D. Kiouach, Y. Sabbar, Stability and threshold of a stochastic SIRS epidemic model with vertical transmission and transfer from infectious to susceptible individuals, Discrete Dyn. Nat. Soc., 2018 (2018), 7570296. https://doi.org/10.1155/2018/7570296 doi: 10.1155/2018/7570296
![]() |
[12] |
D. Kiouach, Y. Sabbar, S. E. A. El-idrissi, New results on the asymptotic behavior of an SIS epidemiological model with quarantine strategy, stochastic transmission, and Levy disturbance, Math. Methods Appl. Sci., 44 (2021), 13468–13492. https://doi.org/10.1002/mma.7638 doi: 10.1002/mma.7638
![]() |
[13] |
Y. Sabbar, D. Kiouach, New method to obtain the acute sill of an ecological model with complex polynomial perturbation, Math. Methods Appl. Sci., 2022 (2022), forthcoming. https://doi.org/10.1002/mma.8654 doi: 10.1002/mma.8654
![]() |
[14] |
Y. Sabbar, A. Zeb, D. Kiouach, N. Gul, T. Sitthiwirattham, D. Baleanu et al., Dynamical bifurcation of a sewage treatment model with general higher-order perturbation, Results Phys., 39 (2022), 105799. https://doi.org/10.1016/j.rinp.2022.105799 doi: 10.1016/j.rinp.2022.105799
![]() |
[15] |
Y. Sabbar, A. Din, D. Kiouach, Predicting potential scenarios for wastewater treatment under unstable physical and chemical laboratory conditions: A mathematical study, Results Phys., 39 (2022), 105717. https://doi.org/10.1016/j.rinp.2022.105717 doi: 10.1016/j.rinp.2022.105717
![]() |
[16] |
Y. Sabbar, A. Khan, A. Din, Probabilistic analysis of a marine ecological system with intense variability, Mathematics, 10 (2022), 2262. https://doi.org/10.3390/math10132262 doi: 10.3390/math10132262
![]() |
[17] | D. Kiouach, Y. Sabbar, Developing new techniques for obtaining the threshold of a stochastic SIR epidemic model with 3-dimensional Lévy process, preprint, arXiv: 2002.09022. |
[18] |
D. Kiouach, Y. Sabbar, The long-time behaviour of a stochastic SIR epidemic model with distributed delay and multidimensional Lévy jumps, Int. J. Biomath., 15 (2022), 2250004. https://doi.org/10.1142/S1793524522500048 doi: 10.1142/S1793524522500048
![]() |
[19] |
D. Kiouach, Y. Sabbar, Dynamic characterization of a stochastic SIR infectious disease model with dual perturbation, Int. J. Biomath., 14 (2021), 2150016. https://doi.org/10.1142/S1793524521500169 doi: 10.1142/S1793524521500169
![]() |
[20] |
D. Kiouach, Y. Sabbar, Ergodic stationary distribution of a stochastic hepatitis B epidemic model with interval-valued parameters and compensated poisson process, Comput. Math. Methods Med., 2020 (2020), 9676501. https://doi.org/10.1155/2020/9676501 doi: 10.1155/2020/9676501
![]() |
[21] |
A. Din, A. Khan, D. Baleanu, Stationary distribution and extinction of stochastic coronavirus (COVID-19) epidemic model, Chaos Solitons Fractals, 139 (2020), 110036. https://doi.org/10.1016/j.chaos.2020.110036 doi: 10.1016/j.chaos.2020.110036
![]() |
[22] |
A. Din, Y. Li, T. Khan, G. Zaman, Mathematical analysis of spread and control of the novel corona virus (COVID-19) in China, Chaos Solitons Fractals, 141 (2020), 110286. https://doi.org/10.1016/j.chaos.2020.110286 doi: 10.1016/j.chaos.2020.110286
![]() |
[23] |
A. Din and Y. Li and A. Yusuf, Delayed hepatitis B epidemic model with stochastic analysis, Chaos Solitons Fractals, 146 (2020), 110839. https://doi.org/10.1016/j.chaos.2021.110839 doi: 10.1016/j.chaos.2021.110839
![]() |
[24] |
D. Kiouach, Y. Sabbar, The threshold of a stochastic siqr epidemic model with Lévy jumps, Trends Biomath. Math. Model. Health Harvesting Popul. Dyn., 2019 (2019), 87–105. https://doi.org/10.1007/978-3-030-23433-1_7 doi: 10.1007/978-3-030-23433-1_7
![]() |
[25] |
D. Zhao, S. Yuan, Sharp conditions for the existence of a stationary distribution in one classical stochastic chemostat, Appl. Math. Comput., 339 (2018), 199–205. https://doi.org/10.1016/j.amc.2018.07.020 doi: 10.1016/j.amc.2018.07.020
![]() |
[26] |
N. Privault, L. Wang, Stochastic SIR Lévy jump model with heavy tailed increments, J. Nonlinear Sci., 31 (2021), 1–28. https://doi.org/10.1007/s00332-020-09670-5 doi: 10.1007/s00332-020-09670-5
![]() |
[27] | D. Kiouach, Y. Sabbar, Threshold analysis of the stochastic hepatitis b epidemic model with successful vaccination and Lévy jumps, in 2019 4th World Conference on Complex Systems (WCCS), IEEE, (2019). https://doi.org/10.1109/ICoCS.2019.8930709 |
[28] |
Y. Sabbar, D. Kiouach, S. Rajasekar, S. E. A. El-idrissi, The influence of quadratic Lévy noise on the dynamic of an SIC contagious illness model: New framework, critical comparison and an application to COVID-19 (SARS-CoV-2) case, Chaos Solitons Fractals, 2022 (2022), 112110. https://doi.org/10.1016/j.chaos.2022.112110 doi: 10.1016/j.chaos.2022.112110
![]() |
[29] |
Y. Zhou, W. Zhang, Threshold of a stochastic SIR epidemic model with Levy jumps, Phys. A, 446 (2016), 204–216. https://doi.org/10.1016/j.physa.2015.11.023 doi: 10.1016/j.physa.2015.11.023
![]() |
[30] |
D. Zhao, S. Yuan, H. Liu, Stochastic dynamics of the delayed chemostat with Levy noises, Int. J. Biomath., 12 (2019), 1950056. https://doi.org/10.1142/S1793524519500566 doi: 10.1142/S1793524519500566
![]() |
1. | Ian B. Augsburger, Grace K. Galanthay, Jacob H. Tarosky, Jan Rychtář, Dewey Taylor, Mabel Carabali, Voluntary vaccination may not stop monkeypox outbreak: A game-theoretic model, 2022, 16, 1935-2735, e0010970, 10.1371/journal.pntd.0010970 | |
2. | Alia M. Alzubaidi, Hakeem A. Othman, Saif Ullah, Nisar Ahmad, Mohammad Mahtab Alam, Analysis of Monkeypox viral infection with human to animal transmission via a fractional and Fractal-fractional operators with power law kernel, 2023, 20, 1551-0018, 6666, 10.3934/mbe.2023287 | |
3. | Marwan Al-Raeei, The study of human monkeypox disease in 2022 using the epidemic models: herd immunity and the basic reproduction number case, 2023, 85, 2049-0801, 316, 10.1097/MS9.0000000000000229 | |
4. | Anwarud Din, Asad Khan, Yassine Sabbar, Long-Term Bifurcation and Stochastic Optimal Control of a Triple-Delayed Ebola Virus Model with Vaccination and Quarantine Strategies, 2022, 6, 2504-3110, 578, 10.3390/fractalfract6100578 | |
5. | Zihao Guo, Shi Zhao, Shengzhi Sun, Daihai He, Ka Chun Chong, Eng Kiong Yeoh, Estimation of the serial interval of monkeypox during the early outbreak in 2022, 2023, 95, 0146-6615, 10.1002/jmv.28248 | |
6. | Yassine Sabbar, Anwar Zeb, Nadia Gul, Driss Kiouach, S. P. Rajasekar, Nasim Ullah, Alsharef Mohammad, Stationary distribution of an SIR epidemic model with three correlated Brownian motions and general Lévy measure, 2023, 8, 2473-6988, 1329, 10.3934/math.2023066 | |
7. | Bing Guo, Asad Khan, Anwarud Din, Numerical Simulation of Nonlinear Stochastic Analysis for Measles Transmission: A Case Study of a Measles Epidemic in Pakistan, 2023, 7, 2504-3110, 130, 10.3390/fractalfract7020130 | |
8. | Ishtiaq Ali, Sami Ullah Khan, Dynamics and simulations of stochastic COVID-19 epidemic model using Legendre spectral collocation method, 2023, 8, 2473-6988, 4220, 10.3934/math.2023210 | |
9. | Humera Batool, Weiyu Li, Zhonggui Sun, Extinction and Ergodic Stationary Distribution of COVID-19 Epidemic Model with Vaccination Effects, 2023, 15, 2073-8994, 285, 10.3390/sym15020285 | |
10. | Mouhcine Naim, Yassine Sabbar, Mostafa Zahri, Behzad Ghanbari, Anwar Zeb, Nadia Gul, Salih Djilali, Fouad Lahmidi, The impact of dual time delay and Caputo fractional derivative on the long-run behavior of a viral system with the non-cytolytic immune hypothesis, 2022, 97, 0031-8949, 124002, 10.1088/1402-4896/ac9e7a | |
11. | Yassine Sabbar, Asad Khan, Anwarud Din, Mouhcine Tilioua, New Method to Investigate the Impact of Independent Quadratic α-Stable Poisson Jumps on the Dynamics of a Disease under Vaccination Strategy, 2023, 7, 2504-3110, 226, 10.3390/fractalfract7030226 | |
12. | Yassine Sabbar, José Luis Diaz Palencia, Mouhcine Tilioua, Abraham Otero, Anwar Zeb, Salih Djilali, A general chemostat model with second-order Poisson jumps: asymptotic properties and application to industrial waste-water treatment, 2023, 8, 2473-6988, 13024, 10.3934/math.2023656 | |
13. | Mohammed Lakhal, Regragui Taki, Mohamed El Fatini, Tarik El Guendouz, Quarantine alone or in combination with treatment measures to control COVID-19, 2023, 0971-3611, 10.1007/s41478-023-00569-4 | |
14. | Shuo Li, Saif Ullah, Salman A. AlQahtani, Sayed M. Tag, Ali Akgül, Mathematical assessment of Monkeypox with asymptomatic infection: Prediction and optimal control analysis with real data application, 2023, 51, 22113797, 106726, 10.1016/j.rinp.2023.106726 | |
15. | Mohamed Mehdaoui, Well-posedness results for a new class of stochastic spatio-temporal SIR-type models driven by proportional pure-jump Lévy noise, 2024, 126, 0307904X, 543, 10.1016/j.apm.2023.11.017 | |
16. | Changtong Li, Saif Ullah, Rashid Nawaz, Salman A AlQahtani, Shuo Li, Mathematical modeling and analysis of monkeypox 2022 outbreak with the environment effects using a Cpauto fractional derivative, 2023, 98, 0031-8949, 105239, 10.1088/1402-4896/acf88e | |
17. | Kottakkaran Sooppy Nisar, Yassine Sabbar, Long-run analysis of a perturbed HIV/AIDS model with antiretroviral therapy and heavy-tailed increments performed by tempered stable Lévy jumps, 2023, 78, 11100168, 498, 10.1016/j.aej.2023.07.053 | |
18. | Waleed Adel, Amr Elsonbaty, A. Aldurayhim, A. El-Mesady, Investigating the dynamics of a novel fractional-order monkeypox epidemic model with optimal control, 2023, 73, 11100168, 519, 10.1016/j.aej.2023.04.051 | |
19. | Amr Elsonbaty, Waleed Adel, A. Aldurayhim, A. El-Mesady, Mathematical modeling and analysis of a novel monkeypox virus spread integrating imperfect vaccination and nonlinear incidence rates, 2024, 15, 20904479, 102451, 10.1016/j.asej.2023.102451 | |
20. | Pushpendra Kumar, M. Vellappandi, Zareen A. Khan, Sivalingam S M, Anthony Kaziboni, V. Govindaraj, A case study of monkeypox disease in the United States using mathematical modeling with real data, 2023, 213, 03784754, 444, 10.1016/j.matcom.2023.06.016 | |
21. | Abdelalim A. Elsadany, Yassine Sabbar, Waleed Adel, Ahmed El-Mesady, Dynamics of a novel discrete fractional model for maize streak epidemics with linear control, 2025, 13, 2195-268X, 1, 10.1007/s40435-024-01509-1 | |
22. | Nasser H. Sweilam, Seham M. Al-Mekhlafi, Saleh M. Hassan, Nehaya R. Alsenaideh, Abdelaziz E. Radwan, A Novel Hybrid Crossover Dynamics of Monkeypox Disease Mathematical Model with Time Delay: Numerical Treatments, 2024, 8, 2504-3110, 185, 10.3390/fractalfract8040185 | |
23. | S. Olaniyi, F. M. Chuma, Lyapunov Stability and Economic Analysis of Monkeypox Dynamics with Vertical Transmission and Vaccination, 2023, 9, 2349-5103, 10.1007/s40819-023-01572-w | |
24. | Ahmed Alshehri, Saif Ullah, Mathematical analysis of monkeypox infection with optimal control analysis: A case study with a new outbreak in the United States, 2023, 0170-4214, 10.1002/mma.9505 | |
25. | Li Zhang, Jianping Huang, Wei Yan, Yingjie Zhao, Danfeng Wang, Bin Chen, Global prediction for mpox epidemic, 2024, 243, 00139351, 117748, 10.1016/j.envres.2023.117748 | |
26. | Guyue Liu, Huilai Li, Dynamical analysis of a class of Monkeypox epidemic model, 2024, 28, 0354-9836, 3367, 10.2298/TSCI2404367L | |
27. | N.H. Sweilam, S.M. Al-Mekhlafi, W.S. Abdel Kareem, G. Alqurishi, A new crossover dynamics mathematical model of monkeypox disease based on fractional differential equations and the Ψ-Caputo derivative: Numerical treatments, 2025, 111, 11100168, 181, 10.1016/j.aej.2024.10.019 | |
28. | O.A. Adepoju, H.O. Ibrahim, An optimal control model for monkeypox transmission dynamics with vaccination and immunity loss following recovery, 2024, 6, 27724425, 100355, 10.1016/j.health.2024.100355 | |
29. | Botao Liu, Samreen Farid, Saif Ullah, Mohamed Altanji, Rashid Nawaz, Shewafera Wondimagegnhu Teklu, Mathematical assessment of monkeypox disease with the impact of vaccination using a fractional epidemiological modeling approach, 2023, 13, 2045-2322, 10.1038/s41598-023-40745-x | |
30. | Wilfredo Meza Cuba, Juan Carlos Huaman Alfaro, Hasnain Iftikhar, Javier Linkolk López-Gonzales, Modeling and Analysis of Monkeypox Outbreak Using a New Time Series Ensemble Technique, 2024, 13, 2075-1680, 554, 10.3390/axioms13080554 | |
31. | Jeta Molla, Idriss Sekkak, Ariel Mundo Ortiz, Iain Moyles, Bouchra Nasri, Mathematical modeling of mpox: A scoping review, 2023, 16, 23527714, 100540, 10.1016/j.onehlt.2023.100540 | |
32. | N.H. Sweilam, Z.N. Mohammed, W.S. Abdel Kareem, Numerical approaches for solving complex order monkeypox mathematical model, 2024, 90, 11100168, 170, 10.1016/j.aej.2024.01.061 | |
33. | Jan Rychtář, Dewey Taylor, Oyita Udiani, 2025, 9780443221231, 187, 10.1016/B978-0-443-22123-1.00001-6 | |
34. | Wei Zhang, Juan Zhang, Quan-Hui Liu, Shi Zhao, Wei-Qiang Li, Jun-Jie Ma, Xin Lu, Stefano Boccaletti, Gui-Quan Sun, Attila Szolnoki, Behavior changes influence mpox transmission in the United States, 2022–2023: Insights from homogeneous and heterogeneous models, 2025, 4, 2752-6542, 10.1093/pnasnexus/pgaf025 |
Parameter | Test 1 | Test 2 | Test 3 |
A | 10 | 10 | 10 |
ζ | 0.0001 | 0.0009 | 0.001 |
ζ∘ | 0.0002 | 0.0002 | 0.0002 |
u | 0.05 | 0.05 | 0.05 |
τ1 | 0.32 | 0.32 | 0.32 |
τ2 | 0.2 | 0.2 | 0.2 |
u0 | 0.0003 | 0.0003 | 0.0003 |
φ | 0.5 | 0.5 | 0.5 |
r1 | 0.041 | 0.041 | 0.041 |
r2 | 0.043 | 0.043 | 0.043 |
α1 | 0.004 | 0.004 | 0.004 |
α2 | 0.002 | 0.002 | 0.002 |
A⋆ | 10 | 10 | 10 |
ζ⋆ | 0.00027 | 0.00027 | 0.00027 |
u⋆ | 0.02 | 0.02 | 0.02 |
Υ1 | 0.2 | 0.2 | 0.2 |
Υ2 | 0.4 | 0.4 | 0.4 |
Υ3 | 0.1 | 0.1 | 0.1 |
Υ4 | 0.2 | 0.2 | 0.2 |
Υ5 | 0.1 | 0.1 | 0.1 |
Υ6 | 0.2 | 0.2 | 0.2 |
Ξ1(u) | 0.3 | 0.3 | 0.3 |
Ξ2(u) | 0.3 | 0.3 | 0.3 |
Ξ3(u) | 0.3 | 0.3 | 0.3 |
Ξ4(u) | 0.3 | 0.3 | 0.3 |
Ξ5(u) | 0.3 | 0.3 | 0.3 |
Ξ6(u) | 0.3 | 0.3 | 0.3 |
Parameter | Test 1 | Test 2 | Test 3 |
A | 10 | 10 | 10 |
ζ | 0.0001 | 0.0009 | 0.001 |
ζ∘ | 0.0002 | 0.0002 | 0.0002 |
u | 0.05 | 0.05 | 0.05 |
τ1 | 0.32 | 0.32 | 0.32 |
τ2 | 0.2 | 0.2 | 0.2 |
u0 | 0.0003 | 0.0003 | 0.0003 |
φ | 0.5 | 0.5 | 0.5 |
r1 | 0.041 | 0.041 | 0.041 |
r2 | 0.043 | 0.043 | 0.043 |
α1 | 0.004 | 0.004 | 0.004 |
α2 | 0.002 | 0.002 | 0.002 |
A⋆ | 10 | 10 | 10 |
ζ⋆ | 0.00027 | 0.00027 | 0.00027 |
u⋆ | 0.02 | 0.02 | 0.02 |
Υ1 | 0.2 | 0.2 | 0.2 |
Υ2 | 0.4 | 0.4 | 0.4 |
Υ3 | 0.1 | 0.1 | 0.1 |
Υ4 | 0.2 | 0.2 | 0.2 |
Υ5 | 0.1 | 0.1 | 0.1 |
Υ6 | 0.2 | 0.2 | 0.2 |
Ξ1(u) | 0.3 | 0.3 | 0.3 |
Ξ2(u) | 0.3 | 0.3 | 0.3 |
Ξ3(u) | 0.3 | 0.3 | 0.3 |
Ξ4(u) | 0.3 | 0.3 | 0.3 |
Ξ5(u) | 0.3 | 0.3 | 0.3 |
Ξ6(u) | 0.3 | 0.3 | 0.3 |