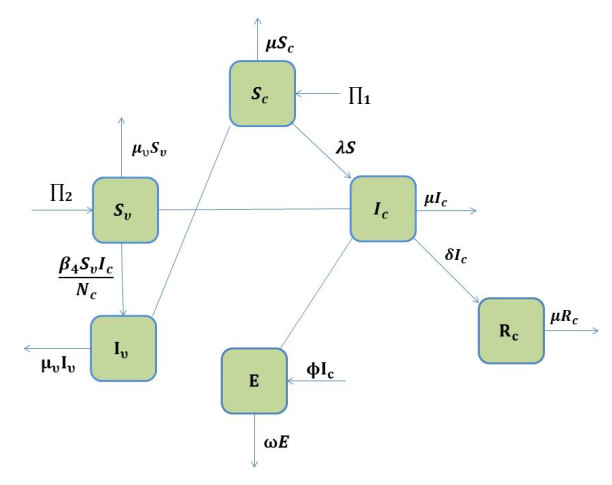
Let Fq be a finite field of q elements. For n∈N∗ with n≥2, let Mn:=Matn(Fq) be the ring of matrices of order n over Fq, Gn,1:=Sln(Fq) be the special linear group over Fq. In this paper, by using the technique of Fourier transformation, we obtain a formula for the number of representations of any element of Mn as the sum of k matrices in Gn,1. As a corollary, we give another proof of the number of the third power moment of the classic Kloosterman sum.
Citation: Yifan Luo, Qingzhong Ji. On the sum of matrices of special linear group over finite field[J]. AIMS Mathematics, 2025, 10(2): 3642-3651. doi: 10.3934/math.2025168
[1] | Liliana Guran, Khushdil Ahmad, Khurram Shabbir, Monica-Felicia Bota . Computational comparative analysis of fixed point approximations of generalized α-nonexpansive mappings in hyperbolic spaces. AIMS Mathematics, 2023, 8(2): 2489-2507. doi: 10.3934/math.2023129 |
[2] | Muhammad Waseem Asghar, Mujahid Abbas, Cyril Dennis Enyi, McSylvester Ejighikeme Omaba . Iterative approximation of fixed points of generalized αm-nonexpansive mappings in modular spaces. AIMS Mathematics, 2023, 8(11): 26922-26944. doi: 10.3934/math.20231378 |
[3] | Hamza Bashir, Junaid Ahmad, Walid Emam, Zhenhua Ma, Muhammad Arshad . A faster fixed point iterative algorithm and its application to optimization problems. AIMS Mathematics, 2024, 9(9): 23724-23751. doi: 10.3934/math.20241153 |
[4] | Zeynep Kalkan, Aynur Şahin, Ahmad Aloqaily, Nabil Mlaiki . Some fixed point and stability results in b-metric-like spaces with an application to integral equations on time scales. AIMS Mathematics, 2024, 9(5): 11335-11351. doi: 10.3934/math.2024556 |
[5] | Shahram Rezapour, Maryam Iqbal, Afshan Batool, Sina Etemad, Thongchai Botmart . A new modified iterative scheme for finding common fixed points in Banach spaces: application in variational inequality problems. AIMS Mathematics, 2023, 8(3): 5980-5997. doi: 10.3934/math.2023301 |
[6] | Buthinah A. Bin Dehaish, Rawan K. Alharbi . On fixed point results for some generalized nonexpansive mappings. AIMS Mathematics, 2023, 8(3): 5763-5778. doi: 10.3934/math.2023290 |
[7] | Austine Efut Ofem, Hüseyin Işik, Godwin Chidi Ugwunnadi, Reny George, Ojen Kumar Narain . Approximating the solution of a nonlinear delay integral equation by an efficient iterative algorithm in hyperbolic spaces. AIMS Mathematics, 2023, 8(7): 14919-14950. doi: 10.3934/math.2023762 |
[8] | Kifayat Ullah, Junaid Ahmad, Hasanen A. Hammad, Reny George . Iterative schemes for numerical reckoning of fixed points of new nonexpansive mappings with an application. AIMS Mathematics, 2023, 8(5): 10711-10727. doi: 10.3934/math.2023543 |
[9] | Dong Ji, Yao Yu, Chaobo Li . Fixed point and endpoint theorems of multivalued mappings in convex b-metric spaces with an application. AIMS Mathematics, 2024, 9(3): 7589-7609. doi: 10.3934/math.2024368 |
[10] | Chanchal Garodia, Izhar Uddin . A new fixed point algorithm for finding the solution of a delay differential equation. AIMS Mathematics, 2020, 5(4): 3182-3200. doi: 10.3934/math.2020205 |
Let Fq be a finite field of q elements. For n∈N∗ with n≥2, let Mn:=Matn(Fq) be the ring of matrices of order n over Fq, Gn,1:=Sln(Fq) be the special linear group over Fq. In this paper, by using the technique of Fourier transformation, we obtain a formula for the number of representations of any element of Mn as the sum of k matrices in Gn,1. As a corollary, we give another proof of the number of the third power moment of the classic Kloosterman sum.
Fractional calculus is an interesting and very famous branch of applied mathematics that deals with the computation of arbitrary order integrals and derivatives. It is noticed that there was limited discussion available on the applications of fractional calculus. However, over the past few years, it has become a most popular subject in academia due to its theoretical advancements and potential applications in various fields of engineering and science [1]. A system's conduct is dictated by its historical actions and mathematical models of real-world issues [2]. As a result, both the system's past and present conditions influence its future. Integer order differential operators do not capture such inherited properties of systems well. On the other hand, nonlocal fractional order derivatives provide valuable tools for describing nonlocal occurrences in space and time [3]. Non-integer order operators are suitable for characterizing a system's memory and genetic properties because they are accounted for at every level in the proposed model. The nonlocal character of non-integer order operators can explain the attraction of fractional calculus. When conceptually describing real-world systems, many fractional-order differential equation difficulties occur. These equations are typically impossible to solve analytically and are difficult to compute. Fractional-order systems are better suited to capture recall and long-range dependence phenomena than integer-order systems. These systems use fractional calculus to simulate complex dynamic behaviors that involve different time scales and past states that affect present and future ones. When modeling real-world occurrences, fractional-order differential equations provide a more adaptable framework. Fractional-order dynamics are present in many physical and natural systems. The Mittag-Leffler (M-L) function is a valuable weapon in solving such problems in fractional calculus. Different types of operators exist in the relevant literature, such as classical operators, operators derived from the power-law process, operators derived from the generalized M-L function, and operators derived from the exponential decay law. Recently, researchers have focused on developing new theories, proposing innovative ideas, and highlighting various applications for the different operators. Nowadays, research revolves around a novel fractal-fractional (F-F) integral and differential operator category. These novel operators are on the fractal operator combined with the notion of fractional differ integrals, where the three ways are Caputo, Caputo-Fabrizio (C-F), and Atangana-Baleanu [4,5,6]. The C-F derivative operator is commonly used in various scientific fields to represent systems with fractional-order dynamics. The term "fractal-fractional" in the context of C-F implies incorporating fractal geometry concepts into the study of fractional calculus using C-F derivatives. This approach involves integrating fractal characteristics or principles into formulating and analyzing fractional-order systems within the framework of C-F calculus.
Researchers have significantly developed for solving differential equations and systems. Due to the unavailability of analytical solutions or their complexity, numerical and approximative analytical methods are commonly used [7]. However, the range of numerical approaches for differential equations is limited, so the researchers have created new numerical techniques. They have found that the fractional derivatives of the Caputo and Riemann-Liouville (RL) types are particularly effective and classical [8]. Classical initial conditions in fractional differential equations involving the Caputo fractional derivative are understandable and can be measured accurately [9]. They have also helped develop the modified Predictor-Corrector technique for determining the numerical solution of fractional differential equations. It is an emphatic weapon for analyzing fractional differential equations the methodologies employed in the numerical simulation of chaotic fractional order systems on two dis-contiguous perspectives. "Time domain methods" are used to directly approximate the response of a fractional order system when solving differential equations numerically. The enhanced Adams-Bashforth Moulton algorithm, presented based on the predictor-corrector scheme, is one of the finest algorithms in this area. The approach can effectively address the stability of nonlinear systems of equations that are easy-to-handle chaotic problems, which is highly significant.
Incorporating strategic delays can enhance the stability of specific control systems. Delay systems can reduce instability and dampen oscillations by providing stabilizing mechanisms with additional time to take effect. In signal processing and communication systems, delays can be utilized to adjust for phase shifts. Controlled delays can help reduce phase distortion, align signals correctly, and improve overall signal quality. Delays are a common feature of many real-world systems, including biological, chemical, and transportation systems. Incorporating delay components into the modeling process can lead to more accurate representation and behavior prediction of these systems. Systems with delays can withstand some types of disruptions and serve as buffers, reducing oscillations and disruptions in the inputs or outputs of the system. Based on past performance or projections, adaptive control systems can modify control operations through delays. In dynamic situations, this adaptive behavior can enhance system responsiveness and performance. The benefits of a delay system depend on the problem and application. Delay systems are valuable instruments in many scientific and technical domains because, when employed correctly, they can improve system robustness, performance, and stability. Therefore, the primary difference between a general and a delay system is that the latter incorporates time delays as an essential component of its behavior. However, not all systems include delays; all delay systems are systems. The Ulam-Hyers stability is concerned with the sensitivity of the functional equation to small perturbations. It explains how fractional-order differential equations behave in systems with minor perturbations. These equations use fractional derivatives, which extend the concept of integer-order derivatives to non-integer orders and are used to describe fractional-order systems. These systems are helpful in many domains, including control theory, because they can store memory and long-range dependency events. It is crucial to examine how small changes or perturbations to the system characteristics or beginning circumstances impact the solutions of the fractional-order differential equations to understand Ulam-Hyer's stability of fractional-order systems [10]. This method helps to clarify the robustness and reliability of fractional-order systems in practical applications.
Science and engineering are seeing a rise in the use of disease modeling, as evidenced by the large number of research studies published in the literature. The main topics of recent research are analyzing the food chain system's global stability and modeling of medical resources, cell wall control, and gut microbiota [11,12,13]. Structurally, chemistry studies have also made use of modeling [14]. Lumpy skin disease (LSD) is a viral illness that harms cattle. The LSD virus causes the disease, and it also affects other ruminants, such as water buffalo. LSD leads to decreased milk production, weight loss, and, in severe cases, even death, resulting in significant financial losses. Cows and buffaloes are the main animals affected by the viral disease known as LSD, which can lead to elevated body temperatures, skin pimples, swelling in certain places, and overall depression. They may also experience bumps in their eyes and nose, swollen legs, and difficulty walking. Animals exposed to LSD will begin displaying symptoms of the sickness after about two to four weeks of incubation. The most effective way to avoid contracting LSD is to be immunized. Further vector control strategies that can be employed to stop the spread of LSD include using insecticides and eliminating environments that support the development of biting insects [15]. The effects of LSD on economies and cultures are well-established, extending beyond the health and productivity of animals. International trade in cattle and products generated from animals may be impacted if the disease leads to trade restrictions being put on affected nations. The clinical symptoms are more severe in young calves and lactating cows. The disease is widespread in Africa and the Middle East, with recent outbreaks reported in Turkey and Pakistan. Greece experienced epidemics in 2015 and 2016, which later spread to Bulgaria and the former yugoslav republic (FYR) of Macedonia. The virus spreads through insect vectors such as ticks, flies and mosquitoes, or contaminated needles. Although the specific vectors differ between nations, they are most active in warm and humid weather, especially during summers and falls when flies are most bothersome. Contaminated feed, water, and equipment can also transmit the disease. It is worth noting that humans cannot contract the virus. Remember that each animal may experience a different level of disease severity. Some may only display minor symptoms, while others may develop severe symptoms or even die as a result of the illness [16]. The death rate in infected herds can range from 1% to 20%, depending on the aggressiveness of the virus and the farming practices used. Early diagnosis and prompt action are crucial to reduce the harmful effects of LSD on animal health, welfare, and productivity. Effective preventive and control measures are necessary to stop the spread of LSD. Vaccination, eradicating the disease's vectors, and quarantining affected animals are some strategies [17]. To effectively control the spread of LSD and reduce its negative impacts, collaboration among veterinary authorities, farmers, and other stakeholders is essential.
When preventing the spread of bugs like flies and mosquitoes, we must be mindful of actions around where animals live. Experts advise against bringing vehicles near animal habitats unless they have been thoroughly cleaned with specialized products that are safe for animals. To keep insects from bothering animals, it's a good idea to use safe bug sprays or move the animals to areas with fewer biting flies, which can help reduce the risk of infection. It's also essential to use different needles when treating animals and to take general preventative measures such as disinfecting stable entrances and wearing disposable suits and boot covers when visiting. Cleaning and disinfecting vehicles before and after use is also crucial. To prevent infection from spreading, it's best to avoid contact with animals from different herds and grazing regions with a high fly population and share trucks, equipment, animals, and employees with other farms. The most effective way to prevent LSD in animals is through vaccination. In areas where the disease is prevalent, various immunization options are available. Mosquitoes, ticks, and other biting insects primarily transmit LSD. Implementing vector control measures such as using insecticides, managing the environment carefully, and creating physical barriers can help reduce the number of biting insects and prevent the spread of the disease. If an animal becomes ill, it should be quarantined and isolated to prevent the illness from spreading to other animals. Maintaining proper biosecurity is essential to prevent the disease from spreading externally. This involves cleaning and disinfecting all farm equipment to avoid contact between animals from different farms. Early detection and immediate response are crucial in preventing the spread of the disease.
Over 570 cows have died because of the LSD illness that has been detected in Pakistan, which is why it was first recognized in Pakistan. This disease has primarily affected Sindh province. At some time, animals throughout the province of Punjab were infected. LSD has been a major issue for Pakistani livestock. For several months, the nation struggled with an LSD virus and suffered significant financial losses as Eid-ul-Adha approached. The costs of other healthy animals were discovered to be exorbitant, rendering them unaffordable to the public. Farms run by small farmers with 50 animals or fewer are vulnerable to danger because of loss of income and poverty. There have been reports of LSD infections in Khyber Pakhtunkhwa districts, where multiple animals have perished. According to records, the Punjab livestock department notified them that they lacked the funds to vaccinate their livestock against the infection. The Pakistani government, particularly the livestock department, should step up and support farmers who have been unable to vaccinate their animals. Since farming is the sole source of income for these small farmers, finding solutions to their issues is essential.
Mathematical modeling plays a crucial role when studying disease epidemiology and disease control. According to these papers, the analysis of human disease dynamics and diseases of animals and plants uses mathematical models [18,19]. Based on the optimal control theory technique, the work's results demonstrate the elimination of sickness. In the literature, not much has been written about the dynamics of LSD. To discover various modes of transmission, a statistical model for the LSD virus was developed [20]. To illustrate their findings, the authors cited the 2006 outbreak in Ein-Zurim. Recently, researchers in [21] examined LSD infection throughout Europe, Asia, and Africa. The outcome was analyzed by the writers using statistical theory. For the dynamics of LSD and their controls, they employed an SEIR model (susceptible (S), exposed (E), infectious (I), and recovered (R)) [22]. The authors only addressed the population of cattle. Examining the 2021–2022 LSD outbreak [23], the authors gave their findings using an assortment of Gaussian mathematical models. By considering every potential pathway for transmission that could cause LSD, the current work examines a comprehensive mathematical model. Environmental transmission, vector transmission, and direct transmission from cattle to another are the three main transmission methods.
Since ancient times, people have grappled with illnesses and worked to find solutions within their communities. Despite the convenience and comfort that science and technology have brought us, they have also contributed to the deterioration of the natural environment worldwide [24]. While genetic modification of food products has become possible through technological advancements, humans' overuse of natural resources has led to the extinction of many plant and animal species. It wouldn't be fair to attribute all of the world's problems to pandemics alone, as seasonal changes can significantly impact different species populations. The research examines the link between the COVID-19 pandemic and Alzheimer's disease using a C-F fractional-order epidemiological model [25]. The authors discuss glucose-insulin levels in the human body in the paper, demonstrating how humans are connected [26]. This research focuses on the chaotic behavior of a fish farm model that includes the population of nutrients and mussels with varying kernels, focusing on the fractal-fractional operator insight [27]. To prevent the unpredictable behavior of the fractional-order Jerk system, the authors suggest a new system and create two control mechanisms [28]. The authors of this paper aim to study a fractional SHTR model (nondrinkers (S), heavy drinkers (H), drinkers in treatment (T), and recovered drinkers (R)) for a nonlinear drinking pandemic in the context of a constant proportional Caputo operator [29]. The authors of this paper have investigated the infectious chronic wasting disease model in deer [30]. In this paper, the authors analyze the spread of malaria and filariasis [31].
We divide the article into seven sections that summarize the whole paper logically. Basic definitions of fractional calculus are presented in Section 2, and the LSD non-integer model is covered in Section 3. Section 4, further broken down into Sections 4.1 and 4.2, explores the model solution's existence, uniqueness, and stability. Section 5 describes the numerical methodologies for a fractional-order LSD model. The graphical representation and comments are described in full in Section 6. Finally, the conclusion is given in Section 7.
In this section, we have discussed a few required definitions, theorems and results related to fractional operators.
Definition 2.1. [32,33] Let W(t) be a continuous and differentiable function in the open interval (a,b) with fractional order σ1 and fractal dimension σ2. Then, the fractal-fractional derivative with power law kernel of W(t) in RL sense is given as:
FFP0+Dσ1,σ2tW(t)=1Γ(k−σ1)ddtσ2∫t0(t−z)k−σ1−1W(z)dz, | (2.1) |
with k−1<σ1,σ2≤k∈N, and dW(z)dzσ2=limt→zW(t)−W(z)tσ2−zσ2.
Definition 2.2. [32,33] Let W(t) be a continuous and differentiable function in the open interval (a,b) with fractional order σ1 and fractal dimension σ2. Then, the fractal-fractional derivative with exponentially decaying kernel of W(t) in RL sense is given as:
FFE0+Dσ1,σ2tW(t)=M(σ1)1−σ1ddtσ2∫t0exp(−σ11−σ1(t−z))W(z)dz, | (2.2) |
and the normalized constant is M(0)=1 and M(1)=1.
Definition 2.3. [32,33] Let W(t) be a continuous and differentiable function in the open interval (a,b) with fractional order σ1 and fractal dimension σ2. Then, the fractal-fractional integral with power law kernel of W(t) in RL sense is given as:
FFP0+Iσ1,σ2tW(t)=σ2Γ(σ1)∫t0(t−z)σ1−1zσ2−1W(z)dz. | (2.3) |
Definition 2.4. [32,33] Let W(t) be a continuous and differentiable function in the open interval (a,b) with fractional order σ1 and fractal dimension σ2. Then, the fractal-fractional integral with exponential decay kernel of W(t) in RL sense is given as:
FFE0+Iσ1,σ2tW(t)=σ2(1−σ1)tσ2−1W(t)M(σ1)+σ1σ2M(σ1)∫t0zσ2−1W(z)dz. | (2.4) |
Definition 2.5. Let W(t) ∈ H1(a,b), a<b, and σ1∈[0,1], then the C-F fractional differential operator of W(t) is given as:
CFaDσ1tW(t)=M(σ1)1−σ1∫taW′(z)exp[−σ1t−z1−σ1]dz, | (2.5) |
where M(σ1) is the normalization function which satisfies the condition M(0)=M(1)=1.
But, if W ∉ H1(a,b) and W∈L1(−∞,b), then the derivative is given as
CFDσ1tW(t)=σ1M(σ1)1−σ1∫b−∞(W(t)−W(z))exp[−σ1t−z1−σ1]dz. | (2.6) |
Theorem 2.1. [34,35] Suppose that Ω:Θ→Θ is an operator and completely continuous. Let
![]() |
The operator Ω has at least one fixed point or the set is not bounded.
Lemma 2.1. [36] This perturbed problem has a solution
FFE0+Dσ1,σ2tW(t)=G(t,W(t))+κ(t),W(0)=W0, |
if the following condition is true:
|W(t)−{W(0)+(G(t,W(t))−κ0(t))σ2(1−σ1)M(σ1)tσ2−1+σ1σ2M(σ1)∫t0zσ1−1G(z,W(z))dz}|≤(σ2(1−σ1)Tσ2−1M(σ1)+σ2Tσ1M(σ1))NG. | (2.7) |
There have been early attempts to establish mathematical biology as a new field of study. Mathematical methods are used to create models of biological phenomena in this field. Despite the importance and necessity of mathematics in biological sciences, the number of research groups and individuals working in this area is currently limited. However, modern applications of mathematics in mathematical biology are fascinating, well-known, and rapidly increasing. As biology is becoming more quantitative, using mathematics in this field is crucial.
An infectious viral disease is called LSD, which affects cattle, causing lameness, swellings in the legs, nasal and ocular discharges, nodules on the mucous membranes, and skin edema and nodules. We are defining the total population of cattle as Nc at any given time t and further dividing it into susceptible cattle Sc(t), infected cattle Ic(t), and recovered cattle Rc(t) (i.e., Nc(t)=Sc(t)+Ic(t)+Rc(t)). The infection is caused by insect vectors such as other ticks, mosquitoes, or flies. These insect populations are denoted by Nν, and further divided into susceptible and infected insects represented by Sν and Iν, respectively (i.e., Nν(t)=Sν(t)+Iν(t)). The variable R(t) indicates the presence of a contaminated environment. Some viruses can spread through the wind or transportation in vehicles from one region to another. The virus can also be transmitted through contaminated needles, feed, equipment, and water. The spread of the illness is likely to happen rapidly during the warm and humid seasons of summer and fall, especially when flies are prevalent. Π1 represents the population growth of healthy cattle and μ represents the natural death rate of the cattle.
The parameter λ indicates the effective contact rate at which healthy cattle become infected. This rate comprises three factors: β1, which is the contact rate resulting from insects biting healthy animals and transmitting the virus; β2, which is the contact rate resulting from vehicles and other objects carrying the virus from the stable or environment and infecting other healthy cattle; and β3, which is the direct transmission of the virus from infected cattle to healthy ones. Because the virus can linger in the semen of infected bulls, it's important to remember that female cattle may also get infected through natural mating or artificial insemination. It is known that calves born to cows infected with this virus may have skin lesions. The virus can be passed to nursing calves through the skin sores in the teats or from contaminated milk. The recovery rate of the infected cattle is denoted by the symbol δ. The growth rate of the insects (which act as vectors for the virus) is denoted by the parameter Π2, while their natural death rate is shown by μν. The contact rate between healthy insects and infected cattle is given by β4. The rate of virus transmission from contaminated cattle, vehicles, and stables is denoted by ϕ, while ω represents virus disinfection. Figure 1 explains the system (3.1) flow rate.
The proposed model is described as [37] :
dScdt=Π1−λSc−μSc,dIcdt=λSc−μIc−δIc,dRcdt=δIc−μRc,dSνdt=Π2−μνSν−β4SνIcNc,dIνdt=β4SνIcNc−μνIν,dEdt=ϕIc−ωE, | (3.1) |
with the following initial conditions:
Sc(0)≥0,Ic(0)≥0,Rc(0)≥0,Sν(0)≥0,Iν(0)≥0,E(0)≥0, |
where λ(t)=β1Iν+β2E+β3IcNc.
Further, we are applying the F-F derivative in the C-F sense to the system (3.1), and then we get
FFE0+Dσ1,σ2tSc(t)=Π1−λSc−μSc,FFE0+Dσ1,σ2tIc(t)=λSc−μIc−δIc,FFE0+Dσ1,σ2tRc(t)=δIc−μRc,FFE0+Dσ1,σ2tSν(t)=Π2−μνSν−β4SνIcNc,FFE0+Dσ1,σ2tIν(t)=β4SνIcNc−μνIν,FFE0+Dσ1,σ2tE(t)=ϕIc−ωE. | (3.2) |
Now, we have calculated the fundamental reproduction number R0 for the LSD module (3.1) by applying the method described in paper [37]. The matrices below are acquired to accomplish this:
V=[δ+μ000μν0−ϕ0ω],F=[β3β1β2β4Π2μΠ1μν00000]. |
Once we calculated the ρ(FV−1), then we obtain
R0=R1+R2=β2ϕ+β3ω2ω(μ+δ)+√(β2ϕ+β3ω)24ω2(μ+δ)2+μΠ2β1β4μ2νΠ1(μ+δ), |
and
R⋆1=β2ϕ+β3ωω(μ+δ),R⋆2=μΠ2β1β4μ2νΠ1(μ+δ). |
In the following examination of the model (3.1), R0 is obtained by adjusting the expressions R1 and R2.
Equilibrium points:
This subsection analyzes the potential equilibrium points of the LSD model (3.1), including a disease-free equilibrium represented by W0. To find this equilibrium, follow these steps:
W0=(S0c,I0c,R0c,S0ν,I0ν,E0c)=(Π1μ,0,0,Π2μν,0,0). |
The theorem below demonstrates the local asymptotic stability (LAS) of the LSD model (3.1) at the equilibrium W0.
Theorem 3.1. The equilibrium point W0=(S0c,I0c,R0c,S0ν,I0ν,E0c) of the LSD system is LAS, if R⋆0<1.
Proof. The Jacobian matrix is obtained using the equilibrium point W0 of the LSD system (3.1),
J(W0)=[μ−β300−β1−β20−δ−μ+β300β1β20δ−μ0000−μβ4Π2Π1μν0−μν000μβ4Π2Π1μν00−μϵ00ϕ000−ω]. | (3.3) |
Therefore, the J(W0) characteristic equation is as follows:
(˜λ+μ)(˜λ+μ)(˜λ+μν)(˜λ3+b1˜λ2+b2˜λ+b3)=0, | (3.4) |
where
b1=μν+ω+(μ+δ)(1−β3μ+δ),b2=ω(μ+δ)(1−R⋆1)+μν(μ+δ)(1−R⋆2)+μν(ω−β3),b3=ωμν(μ+δ)(1−R⋆0), |
where
R⋆0=R20+2R1(1−R0). |
We clearly see in characteristic equation (3.4) that the three eigenvalues are −μ,−μ, and −μν. The cubic equation shows that for all related coefficients, bi>0 for i=1,2,3. Additionally, it is simple to demonstrate that b1b2−b3>0. Therefore, if R⋆0<1, the LSD system (3.1) at the disease-free equilibrium W0 is LAS.
Theorem 3.2. The equilibrium point W0=(S0c,I0c,R0c,S0ν,I0ν,E0c) of the LSD system is GAS (global asymptotically stability), if R⋆0<1.
Proof. We created the Lyapunov function that is displayed below,
L(t)=a1(Sc−S0c−S0lnScS0c)+a2Ic+a3(Sν−S0ν−S0lnSνS0ν)+a4Iν+a5E, | (3.5) |
where a1,a2,a3,a4,a5 are positive constants and differentiating the Eq (3.5), then we get
L′(t)=a1(1−S0cSc)S′c+a2I′c+a3(1−S0νSν)S′c+a4I′ν+a5E′. | (3.6) |
With help of system (3.1) in Eq (3.6), we get
L′(t)=a1(1−S0cSc)(Π1−λSc−μSc)+a2(λSc−μIc−δIc)+a3(1−S0νSν)(Π2−μνSν−β4SνIcNc)+a4(β4SνIcNc−μνIν)+a5(ϕIc−ωE). | (3.7) |
Rearranging the above equation a few times, we obtain
L′(t)=a1(1−S0cSc)(Π1−μSc)−λa1Sc+λa2Sc+λa1S0c−a2(μ+δ)Ic+a3(1−S0νSν)(Π2−μνSν)−a3β4SνIcNc+a3β4S0νIcNc+a4β4SνIcNc−a4μνIν+a5(ϕIc−ωE)=a1μ(1−S0cSc)(S0c−Sc)+a3μ(1−S0cSc)(S0c−Sc)+λ(a2−a1)Sc+(a4−a3)β4SνIcNc+(a1β3+a3β4Π2μμνΠ1−a2(δ+μ)+a5ϕ)Ic+(a1β1−a4μν)Iν+(a1β2−a5ω)E. |
Let a1=μν,a2=μν,a3=β1,a4=β1,a5=β2μνω, then we obtain
L′(t)=−μνμ(Sc−S0c)2Sc−β1μν(Sc−S0c)2Sc+μν(μ+δ)(R⋆0−1)Ic. |
It is evident that Sc=0, Ic=0 and Sν=0 if, and only if, L′(t)≤0, if R⋆0<1 and L′(t)=0. It appears that the outcomes are situated within the feasible region. Thus, the LSD system is GAS at W0, if R⋆0<1.
Here, we provide the existence and uniqueness theorem for the LSD model. We now rewrite system (3.2) using this structure, looking like this:
CF0+Dσ1tSc(t)=σ2tσ2−1[G1(t,Sc,Ic,Rc,Sν,Iν,E)],CF0+Dσ1tIc(t)=σ2tσ2−1[G2(t,Sc,Ic,Rc,Sν,Iν,E)],CF0+Dσ1tRc(t)=σ2tσ2−1[G3(t,Sc,Ic,Rc,Sν,Iν,E)],CF0+Dσ1tSν(t)=σ2tσ2−1[G4(t,Sc,Ic,Rc,Sν,Iν,E)],CF0+Dσ1tIν(t)=σ2tσ2−1[G5(t,Sc,Ic,Rc,Sν,Iν,E)],CF0+Dσ1tE(t)=σ2tσ2−1[G6(t,Sc,Ic,Rc,Sν,Iν,E)], | (4.1) |
where
{G1(t,Sc,Ic,Rc,Sν,Iν,E)=Π1−λSc−μSc,G2(t,Sc,Ic,Rc,Sν,Iν,E)=λSc−μIc−δIc,G3(t,Sc,Ic,Rc,Sν,Iν,E)=δIc−μRc,G4(t,Sc,Ic,Rc,Sν,Iν,E)=Π2−μνSν−β4SνIcNc,G5(t,Sc,Ic,Rc,Sν,Iν,E)=β4SνIcNc−μνIν,G6(t,Sc,Ic,Rc,Sν,Iν,E)=ϕIc−ωE. |
Further, we rewrite the system (4.1) as follows:
CF0+Dσ1tE(t)=σ2tσ2−1G(t,E(t)),E(0)=E0. | (4.2) |
We apply the F-F integral in the C-F sense, then we obtain
E(t)=E(0)+σ2tσ2−1(1−σ1)M(σ1)G(t,E(t))+σ1σ2M(σ1)∫t0zσ2−1G(z,E(z))dz, |
where
E(t)={Sc(t)Ic(t)Rc(t)Sν(t)Iν(t)E(t),G(t,E(t))={G1(t,Sc,Ic,Rc,Sν,Iν,E)G2(t,Sc,Ic,Rc,Sν,Iν,E)G3(t,Sc,Ic,Rc,Sν,Iν,E)G4(t,Sc,Ic,Rc,Sν,Iν,E)G5(t,Sc,Ic,Rc,Sν,Iν,E)G6(t,Sc,Ic,Rc,Sν,Iν,E). |
We are applying the F-F integral in the C-F sense in the system (4.1), then we get
Sc(t)=Sc(0)+σ2(1−σ1)M(σ1)tσ2−1G1(t,Sc,Ic,Rc,Sν,Iν,E)+σ1σ2M(σ1)∫t0zσ2−1G1(z,Sc,Ic,Rc,Sν,Iν,E)dz,Ic(t)=Ic(0)+σ2(1−σ1)M(σ1)tσ2−1G2(t,Sc,Ic,Rc,Sν,Iν,E)+σ1σ2M(σ1)∫t0zσ2−1G2(z,Sc,Ic,Rc,Sν,Iν,E)dz,Rc(t)=Rc(0)+σ2(1−σ1)M(σ1)tσ2−1G3(t,Sc,Ic,Rc,Sν,Iν,E)+σ1σ2M(σ1)∫t0zσ2−1G3(z,Sc,Ic,Rc,Sν,Iν,E)dz,Sν(t)=Sν(0)+σ2(1−σ1)M(σ1)tσ2−1G4(t,Sc,Ic,Rc,Sν,Iν,E)+σ1σ2M(σ1)∫t0zσ2−1G4(z,Sc,Ic,Rc,Sν,Iν,E)dz,Iν(t)=Iν(0)+σ2(1−σ1)M(σ1)tσ2−1G5(t,Sc,Ic,Rc,Sν,Iν,E)+σ1σ2M(σ1)∫t0zσ2−1G5(z,Sc,Ic,Rc,Sν,Iν,E)dz,R(t)=R(0)+σ2(1−σ1)M(σ1)tσ2−1G6(t,Sc,Ic,Rc,Sν,Iν,E)+σ1σ2M(σ1)∫t0zσ2−1G6(z,Sc,Ic,Rc,Sν,Iν,E)dz. | (4.3) |
In this section, we examine the existence and uniqueness of the solution in the LSD model. To show the proposed model's existence and uniqueness solution, consider defining a Banach space as follows: Θn = F[0,T] is the space of all functions Sc,Ic,Rc,Sν,Iν,E, respectively, to n=1,2,3,4,5,6. Θn generates a Banach space when provided with the norm ‖Sc‖=maxt∈[0,T]|Sc(t)|, ‖Ic‖=maxt∈[0,T]|Ic(t)|, ‖Rc‖=maxt∈[0,T]|R(t)|, ‖Sν‖=maxt∈[0,T]|Sν(t)|, ‖Iν‖=maxt∈[0,T]|Iν(t)|, and ‖E‖=maxt∈[0,T]|E(t)|, respectively, to n=1,2,3,4,5,6. Consequently, the norm is applied in the product space as
‖(Sc,Ic,Rc,Sν,Iν,E)‖=‖Sc‖+‖Ic‖+‖Rc‖+‖Sν‖+‖Iν‖+‖E‖. |
Banach spaces are defined as
Θ=(Θ1×Θ2×Θ3×Θ4×Θ5×Θ6,‖(Sc,Ic,Rc,Sν,Iν,E)‖). |
Assuming the system (4.3), which defines the operator Ω:Θ→Θ, we obtain
Ω(Sc,Ic,Rc,Sν,Iν,E)=(Ω1(Sc,Ic,Rc,Sν,Iν,E)(t)Ω2(Sc,Ic,Rc,Sν,Iν,E)(t)Ω3(Sc,Ic,Rc,Sν,Iν,E)(t)Ω4(Sc,Ic,Rc,Sν,Iν,E)(t)Ω5(Sc,Ic,Rc,Sν,Iν,E)(t)Ω6(Sc,Ic,Rc,Sν,Iν,E)(t)), | (4.4) |
where
Ω1(Sc,Ic,Rc,Sν,Iν,E)(t)=Sc(0)+σ2(1−σ1)M(σ1)tσ2−1G1(t,Sc,Ic,Rc,Sν,Iν,E)+σ1σ2M(σ1)∫t0zσ2−1G1(z,Sc,Ic,Rc,Sν,Iν,E)dz, |
Ω2(Sc,Ic,Rc,Sν,Iν,E)(t)=Ic(0)+σ2(1−σ1)M(σ1)tσ2−1G2(t,Sc,Ic,Rc,Sν,Iν,E)+σ1σ2M(σ1)∫t0zσ2−1G2(z,Sc,Ic,Rc,Sν,Iν,E)dz, |
Ω3(Sc,Ic,Rc,Sν,Iν,E)(t)=Rc(0)+σ2(1−σ1)M(σ1)tσ2−1G3(t,Sc,Ic,Rc,Sν,Iν,E)+σ1σ2M(σ1)∫t0zσ2−1G3(z,Sc,Ic,Rc,Sν,Iν,E)dz, | (4.5) |
Ω4(Sc,Ic,Rc,Sν,Iν,E)(t)=Sν(0)+σ2(1−σ1)M(σ1)tσ2−1G4(t,Sc,Ic,Rc,Sν,Iν,E)+σ1σ2M(σ1)∫t0zσ2−1G4(z,Sc,Ic,Rc,Sν,Iν,E)dz, |
Ω5(Sc,Ic,Rc,Sν,Iν,E)(t)=Iν(0)+σ2(1−σ1)M(σ1)tσ2−1G5(t,Sc,Ic,Rc,Sν,Iν,E)+σ1σ2M(σ1)∫t0zσ2−1G5(z,Sc,Ic,Rc,Sν,Iν,E)dz, |
Ω6(Sc,Ic,Rc,Sν,Iν,E)(t)=E(0)+σ2(1−σ1)M(σ1)tσ2−1G6(t,Sc,Ic,Rc,Sν,Iν,E)+σ1σ2M(σ1)∫t0zσ2−1G6(z,Sc,Ic,Rc,Sν,Iν,E)dz. |
Theorem 4.1. Let assume that Gr: J×R6→R are continuous functions and these constants are P1,Gr, P2,Gr,..., and P6,Gr>0, such that ∀ Sc, ˘Sc, Ic, ˘Ic, Rc, ˘Rc, Sν, ˘Sν, Iν, ˘Iν, E, ˘E ∈Θ, where J= [0,T] and r=1,2,3,4,5,6, then we get
‖Gr(t,Sc,Ic,Rc,Sν,Iν,E)−Gr(t,˘Sc,˘Ic,˘Rc,˘Sν,˘Iν,˘E)‖≤P1,Gr‖Sc−˘Sc‖+P2,Gr‖Ic−˘Ic‖+P3,Gr‖Rc−˘Rc‖+P4,Gr‖Sν−˘Sν‖+P5,Gr‖Iν−˘Iν‖+P6,Gr‖E−˘E‖. |
If the condition ΔΩΠG1+ΔΩΠG2+ΔΩΠG3+ΔΩΠG4+ΔΩΠG5+ΔΩΠG6<1 is fulfilled, then system (3.2) has a unique solution, where
ΔΩ={σ2(1−σ1)M(σ1)Tσ2−1+σ1Tσ2M(σ1)},ΠG1=P1,G1+P2,G1+P3,G1+P4,G1+P5,G1+P6,G1,ΠG2=P1,G2+P2,G2+P3,G2+P4,G2+P5,G2+P6,G2,ΠG3=P1,G3+P2,G3+P3,G3+P4,G3+P5,G3+P6,G3,ΠG4=P1,G4+P2,G4+P3,G4+P4,G4+P5,G4+P6,G4,ΠG5=P1,G5+P2,G5+P3,G5+P4,G5+P5,G5+P6,G5,ΠG6=P1,G6+P2,G6+P3,G6+P4,G6+P5,G6+P6,G6. |
Proof. We suppose that supt∈JG1(t,0,0,0,0,0,0) =ℵG1<∞, supt∈JG2(t,0,0,0,0,0,0) =ℵG2<∞, supt∈JG3(t,0,0,0,0,0,0) =ℵG3<∞, supt∈JG4(t,0,0,0,0,0,0) =ℵG4<∞, supt∈JG5(t,0,0,0,0,0,0) =ℵG5<∞, and supt∈JG6(t,0,0,0,0,0,0) =ℵG6<∞. First, we prove that and we assume that
is a closed convex ball (i.e.,
={(Sc,Ic,Rc,Sν,Iν,E)∈Θ:‖(Sc,Ic,Rc,Sν,Iν,E)‖≤κ}). We suppose that Sc,Ic,Rc,Sν,Iν,E∈
, and we have
‖Ω1(Sc,Ic,Rc,Sν,Iν,E)‖≤σ2(1−σ1)M(σ1)Tσ2−1maxt∈J(|G1(t,Sc,Ic,Rc,Sν,Iν,E)−G1(t,0,0,0,0,0,0)|+|G1(t,0,0,0,0,0,0)|)+σ1σ2M(σ1)maxt∈J∫t0zσ2−1(|G1(z,Sc,Ic,Rc,Sν,Iν,E)−G1(z,0,0,0,0,0,0)|+|G1(z,0,0,0,0,0,0)|)dz≤σ2(1−σ1)M(σ1)Tσ2−1(P1,G1‖Sc‖+P2,G1‖Ic‖+P3,G1‖Rc‖+P4,G1‖Sν‖+P5,G1‖Iν‖+P6,G1‖E‖+ℵG1)+σ1M(σ1)Tσ2(P1,G1‖Sc‖+P2,G1‖Ic‖+P3,G1‖Rc‖+P4,G1‖Sν‖+P5,G1‖Iν‖+P6,G1‖E‖+ℵG1)≤ΔΩΠG1(κ6+ℵG1)≤κ6. | (4.6) |
In this process, we apply the same steps to another one, and then we will obtain the outcome.
‖Ω2(Sc,Ic,Rc,Sν,Iν,E)‖≤κ6,‖Ω3(Sc,Ic,Rc,Sν,Iν,E)‖≤κ6,‖Ω4(Sc,Ic,Rc,Sν,Iν,E)‖≤κ6,‖Ω5(Sc,Ic,Rc,Sν,Iν,E)‖≤κ6,‖Ω6(Sc,Ic,Rc,Sν,Iν,E)‖≤κ6. | (4.7) |
We use the Θ definition and Eqs (4.6) and (4.7), then we get
‖Ω(Sc,Ic,Rc,Sν,Iν,E)‖≤κ, | (4.8) |
and when (Sc,Ic,Rc,Sν,Iν,E),(˘Sc,˘Ic,˘Rc,˘Sν,˘Iν,˘E)∈Θ, for each t∈J, we get
‖Ω1(Sc,Ic,Rc,Sν,Iν,E)−Ω1(˘Sc,˘Ic,˘Rc,˘Sν,˘Iν,˘E)‖≤σ2(1−σ1)M(σ1)Tσ2−1maxt∈J(|G1(t,Sc,Ic,Rc,Sν,Iν,E)−G1(t,˘Sc,˘Ic,˘Rc,˘Sν,˘Iν,˘E)|)+σ1σ2M(σ1)maxt∈J∫t0zσ2−1(|G1(z,Sc,Ic,Rc,Sν,Iν,E)−G1(z,˘Sc,˘Ic,˘Rc,˘Sν,˘Iν,˘E)|)dz≤ΔΩΠG1‖(Sc,Ic,Rc,Sν,Iν,E)−(˘Sc,˘Ic,˘Rc,˘Sν,˘Iν,˘E)‖. | (4.9) |
In this process, we apply the same steps to another one, and then we will obtain the outcome.
‖Ω2(Sc,Ic,Rc,Sν,Iν,E)−Ω2(˘Sc,˘Ic,˘Rc,˘Sν,˘Iν,˘E)‖≤ΔΩΠG2‖(Sc,Ic,Rc,Sν,Iν,E)−(˘Sc,˘Ic,˘Rc,˘Sν,˘Iν,˘E)‖,‖Ω3(Sc,Ic,Rc,Sν,Iν,E)−Ω3(˘Sc,˘Ic,˘Rc,˘Sν,˘Iν,˘E)‖≤ΔΩΠG3‖(Sc,Ic,Rc,Sν,Iν,E)−(˘Sc,˘Ic,˘Rc,˘Sν,˘Iν,˘E)‖,‖Ω4(Sc,Ic,Rc,Sν,Iν,E)−Ω4(˘Sc,˘Ic,˘Rc,˘Sν,˘Iν,˘E)‖≤ΔΩΠG4‖(Sc,Ic,Rc,Sν,Iν,E)−(˘Sc,˘Ic,˘Rc,˘Sν,˘Iν,˘E)‖,‖Ω5(Sc,Ic,Rc,Sν,Iν,E)−Ω5(˘Sc,˘Ic,˘Rc,˘Sν,˘Iν,˘E)‖≤ΔΩΠG5‖(Sc,Ic,Rc,Sν,Iν,E)−(˘Sc,˘Ic,˘Rc,˘Sν,˘Iν,˘E)‖,‖Ω6(Sc,Ic,Rc,Sν,Iν,E)−Ω6(˘Sc,˘Ic,˘Rc,˘Sν,˘Iν,˘E)‖≤ΔΩΠG6‖(Sc,Ic,Rc,Sν,Iν,E)−(˘Sc,˘Ic,˘Rc,˘Sν,˘Iν,˘E)‖. | (4.10) |
Using Eqs (4.9) and (4.10), we get
‖Ω(Sc,Ic,Rc,Sν,Iν,E)−Ω(˘Sc,˘Ic,˘Rc,˘Sν,˘Iν,˘E)‖≤(ΔΩΠG1+ΔΩΠG2+ΔΩΠG3+ΔΩΠG4+ΔΩΠG5+ΔΩΠG6)×‖(Sc,Ic,Rc,Sν,Iν,E)−(˘Sc,˘Ic,˘Rc,˘Sν,˘Iν,˘E)‖. | (4.11) |
Since ΔΩΠG1+ΔΩΠG2+ΔΩΠG3+ΔΩΠG4+ΔΩΠG5+ΔΩΠG6<1, then Ω(Sc,Ic,Rc,Sν,Iν,E) is a contraction operator. With the use of the Banach contraction theorem, Ω(Sc,Ic,Rc,Sν,Iν,E) has a unique fixed point. Hence, the proposed model (3.2) has a unique solution.
Theorem 4.2. We suppose that Ψ1,Gr, Ψ2,Gr, Ψ3,Gr, Ψ4,Gr, Ψ5,Gr, Ψ6,Gr, Ψ7,Gr, (r=1,2,3,4,5,6) :J→R+ such that ∀ Sc,Ic,Rc,Sν,Iν,E∈Θ, and we get
|Gr(t,Sc,Ic,Rc,Sν,Iν,E)|≤Ψ1,Gr(t)+Ψ2,Gr(t)|Sc(t)|+Ψ3,Gr(t)|Ic(t)|+Ψ4,Gr(t)|Rc(t)|+Ψ5,Gr(t)|Sν(t)|+Ψ6,Gr(t)|Iν(t)|+Ψ7,Gr(t)|E(t)|, |
with supt∈JΨ1,Gr(t)=~Ψ1,Gr, supt∈JΨ2,Gr(t)=~Ψ2,Gr, supt∈JΨ3,Gr(t)=~Ψ3,Gr, supt∈JΨ4,Gr(t)=~Ψ4,Gr, supt∈JΨ5,Gr(t)=~Ψ5,Gr, supt∈JΨ6,Gr(t)=~Ψ6,Gr, supt∈JΨ7,Gr(t)=~Ψ7,Gr and ~Ψ1,G1, ~Ψ1,G2, ~Ψ1,G3, ~Ψ1,G4, ~Ψ1,G5, ~Ψ1,G6>0.
Other assumptions are ΔΩ(~Ψr,G1+~Ψr,G2+~Ψr,G3+~Ψr,G4+~Ψr,G5+~Ψr,G6)<1 where r=2,3,...,7, and
Δ0=min{1−ΔΩ(~Ψ2,G1+~Ψ2,G2+~Ψ2,G3+~Ψ2,G4+~Ψ2,G5+~Ψ2,G6)1−ΔΩ(~Ψ3,G1+~Ψ3,G2+~Ψ3,G3+~Ψ3,G4+~Ψ3,G5+~Ψ3,G6)1−ΔΩ(~Ψ4,G1+~Ψ4,G2+~Ψ4,G3+~Ψ4,G4+~Ψ4,G5+~Ψ4,G6)1−ΔΩ(~Ψ5,G1+~Ψ5,G2+~Ψ5,G3+~Ψ5,G4+~Ψ5,G5+~Ψ5,G6)1−ΔΩ(~Ψ6,G1+~Ψ6,G2+~Ψ6,G3+~Ψ6,G4+~Ψ6,G5+~Ψ6,G6)1−ΔΩ(~Ψ7,G1+~Ψ7,G2+~Ψ7,G3+~Ψ7,G4+~Ψ7,G5+~Ψ7,G6)}, |
and the system (3.2) has at least one solution.
Proof. First, we suppose that Ω:Θ→Θ is an operator and completely continuous. We can say that the Ω operator is continuous because Gr,(r=1,2,3,4,5,6) is continuous. Let be a bounded set and there is ∃ constants
, such that maxt∈J|Gr(t,Sc,Ic,Rc,Sν,Iν,E)|≤
, ∀(Sc,Ic,Rc,Sν,Iν,E) ∈
. We have
![]() |
(4.12) |
In this process, we apply the same steps to another one, and then we will obtain the outcome.
![]() |
(4.13) |
We proved that Ω(Sc,Ic,Rc,Sν,Iν,E) is uniformly bounded with the use of Eqs (4.12) and (4.13).
Now, we show that Ω is equi-continuous. First, we suppose that 0≤t1≤t2≤T, therefore
![]() |
(4.14) |
In this process, we apply the same steps to another one, and then we will obtain the outcome.
![]() |
(4.15) |
![]() |
(4.16) |
![]() |
(4.17) |
![]() |
(4.18) |
![]() |
(4.19) |
Thus, Ω(Sc,Ic,Rc,Sν,Iν,E) is equi-continuous. Therefore, Ω(Sc,Ic,Rc,Sν,Iν,E) is completely continuous. Now, we prove that
![]() |
is bounded. Let (Sc,Ic,Rc,Sν,Iν,E)∈ , then (Sc,Ic,Rc,Sν,Iν,E)=ϱΩ(Sc,Ic,Rc,Sν,Iν,E). When t∈J, then Sc(t)=ϱΩ1(Sc,Ic,Rc,Sν,Iν,E)(t), Ic(t)=ϱΩ2(Sc,Ic,Rc,Sν,Iν,E)(t), Rc(t)=ϱΩ3(Sc,Ic,Rc,Sν,Iν,E)(t), Sν(t)=ϱΩ4(Sc,Ic,Rc,Sν,Iν,E)(t), Iν(t)=ϱΩ5(Sc,Ic,Rc,Sν,Iν,E)(t), and E(t)=ϱΩ6(Sc,Ic,Rc,Sν,Iν,E)(t). Then,
|Sc(t)|≤[σ2(1−σ1)M(σ1)Tσ2−1+σ1Tσ2M(σ1)](Ψ1,G1(t)+Ψ2,G1(t)|Sc(t)|+Ψ3,G1(t)|Ic(t)|+Ψ4,G1(t)|Rc(t)|+Ψ5,G1(t)|Sν(t)|+Ψ6,G1(t)|Iν(t)|+Ψ7,G1(t)|E(t)|). | (4.20) |
Simplifying Eq (4.20), we have
‖Sc‖≤ΔΩ(~Ψ1,G1+~Ψ2,G1‖Sc‖+~Ψ3,G1‖Ic‖+~Ψ4,G1‖Rc‖+~Ψ5,G1‖Sν‖+~Ψ6,G1‖Iν‖+~Ψ7,G1‖E‖). | (4.21) |
In this process, we apply the same steps to another one, and then we will obtain the outcome.
‖Ic‖≤ΔΩ(~Ψ1,G2+~Ψ2,G2‖Sc‖+~Ψ3,G2‖Ic‖+~Ψ4,G2‖Rc‖+~Ψ5,G2‖Sν‖+~Ψ6,G2‖Iν‖+~Ψ7,G2‖E‖),‖Rc‖≤ΔΩ(~Ψ1,G3+~Ψ2,G3‖Sc‖+~Ψ3,G3‖Ic‖+~Ψ4,G3‖Rc‖+~Ψ5,G3‖Sν‖+~Ψ6,G3‖Iν‖+~Ψ7,G3‖E‖),‖Sν‖≤ΔΩ(~Ψ1,G4+~Ψ2,G4‖Sc‖+~Ψ3,G4‖Ic‖+~Ψ4,G4‖Rc‖+~Ψ5,G4‖Sν‖+~Ψ6,G4‖Iν‖+~Ψ7,G4‖E‖),‖Iν‖≤ΔΩ(~Ψ1,G5+~Ψ2,G5‖Sc‖+~Ψ3,G5‖Ic‖+~Ψ4,G5‖Rc‖+~Ψ5,G5‖Sν‖+~Ψ6,G5‖Iν‖+~Ψ7,G5‖E‖),‖E‖≤ΔΩ(~Ψ1,G6+~Ψ2,G6‖Sc‖+~Ψ3,G6‖Ic‖+~Ψ4,G6‖Rc‖+~Ψ5,G6‖Sν‖+~Ψ6,G6‖Iν‖+~Ψ7,G6‖E‖). | (4.22) |
Now, we add the Eqs (4.21) and (4.22), then we get
‖Sc‖+‖Ic‖+‖Rc‖+‖Sν‖+‖Iν‖+‖E‖≤ΔΩ(~Ψ1,G1+~Ψ1,G2+~Ψ1,G3+~Ψ1,G4+~Ψ1,G5+~Ψ1,G6)+ΔΩ(~Ψ2,G1+~Ψ2,G2+~Ψ2,G3+~Ψ2,G4+~Ψ2,G5+~Ψ2,G6)‖Sc‖+ΔΩ(~Ψ3,G1+~Ψ3,G2+~Ψ3,G3+~Ψ3,G4+~Ψ3,G5+~Ψ3,G6)‖Ic‖+ΔΩ(~Ψ4,G1+~Ψ4,G2+~Ψ4,G3+~Ψ4,G4+~Ψ4,G5+~Ψ4,G6)‖Rc‖+ΔΩ(~Ψ5,G1+~Ψ5,G2+~Ψ5,G3+~Ψ5,G4+~Ψ5,G5+~Ψ5,G6)‖Sν‖+ΔΩ(~Ψ6,G1+~Ψ6,G2+~Ψ6,G3+~Ψ6,G4+~Ψ6,G5+~Ψ6,G6)‖Iν‖+ΔΩ(~Ψ7,G1+~Ψ7,G2+~Ψ7,G3+~Ψ7,G4+~Ψ7,G5+~Ψ7,G6)‖E‖. | (4.23) |
Thus, we obtain
‖Sc,Ic,Rc,Sν,Iν,E‖≤ΔΩ(~Ψ1,G1+~Ψ1,G2+~Ψ1,G3+~Ψ1,G4+~Ψ1,G5+~Ψ1,G6)Δ0. |
Hence, is bounded. Ω has at least one fixed point by the Theorem 2.1, therefore the proposed model (3.2) has a solution.
In this section, we establish stability conditions for the LSD model. Let κ(t) be a perturbed parameter,
(i)|κ(t)|≤ϵ,forϵ>0,(ii)FFE0+Dσ1,σ2tE(t)=G(t,E(t))+κ(t). |
Theorem 4.3. [36] If the following condition holds, Q<1, where Q=(σ2(1−σ1)Tσ2−1M(σ1)+σ2Tσ1M(σ1))NG, and with use of systems (4.1), (4.3), and Lemma 1, then Ulam-Hyers stability exists for the solution of the proposed model.
Proof. We have demonstrated that the LSD model has a unique solution. Let E∈Θ be a solution and ˉE∈Θ be a unique solution of the system (3.2). We have
|E(t)−ˉE(t)|=|E(t)−{¯E(0)+(G(t,ˉE(t))−G0(t))σ2(1−σ1)M(σ1)tσ2−1+σ1σ2M(σ1)∫t0zσ2−1G(z,ˉE(z))dz}|≤|E(t)−{E(0)+(G(t,E(t))−G0(t))σ2(1−σ1)M(σ1)tσ2−1+σ1σ2M(σ1)∫t0zσ2−1G(z,E(z))dz}|+|{E(0)+(G(t,E(t))−G0(t))σ2(1−σ1)M(σ1)tσ2−1+σ1σ2M(σ1)∫t0zσ2−1G(z,E(z))dz}|−|{ˉE(0)+(G(t,ˉE(t))−G0(t))σ2(1−σ1)M(σ1)tσ2−1+σ1σ2M(σ1)∫t0zσ2−1G(z,ˉE(z))dz}|≤ℶσ1,σ2+σ2(1−σ1)Tσ2−1NGM(σ1)‖E−ˉE‖+σ2Tσ1NGM(σ1)‖E−ˉE‖≤ℶσ1,σ2+Q‖E−ˉE‖. |
Based on the result mentioned above, we have
‖E−ˉE‖≤ℶσ1,σ21−Q‖E−ˉE‖. |
We can conclude that the system's solution is stable. So, the proof is finished.
Dealing with nonlinearity in a biological model using fractional derivatives is challenging. Researchers have recently developed some novel numerical algorithms to solve biological models. These numerical methods play a significant role in determining the numerical solution to our problem. This process is essential to dealing with nonlinearity in our models.
The LSD model in terms of F-F in the sense of C-F. Consequently, the numerical technique in the context of C-F is with the following structure:
\begin{align} & {}_{0+}^{CF}\mathfrak{D}^{{\sigma_1}}_{t}S_c(t) = {\sigma_2} t^{{\sigma_2} - 1}\left[ \mathbb{G}_1(t, S_c, I_c, R_c, S_\nu, I_\nu, E) \right] , \\ & {}_{0+}^{CF}\mathfrak{D}^{{\sigma_1}}_{t}I_c(t) = {\sigma_2} t^{{\sigma_2} - 1}\left[ \mathbb{G}_2(t, S_c, I_c, R_c, S_\nu, I_\nu, E) \right] , \\ & {}_{0+}^{CF}\mathfrak{D}^{{\sigma_1}}_{t}R_c(t) = {\sigma_2} t^{{\sigma_2} - 1}\left[ \mathbb{G}_3(t, S_c, I_c, R_c, S_\nu, I_\nu, E) \right] , \\ & {}_{0+}^{CF}\mathfrak{D}^{{\sigma_1}}_{t}S_\nu(t) = {\sigma_2} t^{{\sigma_2} - 1}\left[ \mathbb{G}_4(t, S_c, I_c, R_c, S_\nu, I_\nu, E) \right] , \\ & {}_{0+}^{CF}\mathfrak{D}^{{\sigma_1}}_{t}I_\nu(t) = {\sigma_2} t^{{\sigma_2} - 1}\left[ \mathbb{G}_5(t, S_c, I_c, R_c, S_\nu, I_\nu, E) \right] , \\ & {}_{0+}^{CF}\mathfrak{D}^{{\sigma_1}}_{t}E(t) = {\sigma_2} t^{{\sigma_2} - 1}\left[ \mathbb{G}_6(t, S_c, I_c, R_c, S_\nu, I_\nu, E) \right] . \end{align} | (5.1) |
We apply the C-F integral in system (5.1), then we get
\begin{align*} S_c(t) - S_c(0) = & \frac{{\sigma_2} t^{{\sigma_2} -1}(1-{\sigma_1})}{M({\sigma_1})}\mathbb{G}_1(t, S_c, I_c, R_c, S_\nu, I_\nu, E) \nonumber \\ & + \frac{{\sigma_1} {\sigma_2}}{M({\sigma_1})}\int_{0}^{t}z^{{\sigma_2} -1}\mathbb{G}_1(z, S_c, I_c, R_c, S_\nu, I_\nu, E)dz, \\ I_c(t) - I_c(0) = &\frac{{\sigma_2} t^{{\sigma_2} -1}(1-{\sigma_1})}{M({\sigma_1})}\mathbb{G}_2(t, S_c, I_c, R_c, S_\nu, I_\nu, E) \nonumber \\ & + \frac{{\sigma_1} {\sigma_2}}{M({\sigma_1})}\int_{0}^{t}z^{{\sigma_2} -1}\mathbb{G}_2(z, S_c, I_c, R_c, S_\nu, I_\nu, E)dz, \\ R_c(t) - R_c(0) = & \frac{{\sigma_2} t^{{\sigma_2} -1}(1-{\sigma_1})}{M({\sigma_1})}\mathbb{G}_3(t, S_c, I_c, R_c, S_\nu, I_\nu, E) \\ & + \frac{{\sigma_1} {\sigma_2}}{M({\sigma_1})}\int_{0}^{t}z^{{\sigma_2} -1}\mathbb{G}_3(z, S_c, I_c, R_c, S_\nu, I_\nu, E)dz, \\ S_{\nu}(t) - S_{\nu}(0) = &\frac{{\sigma_2} t^{{\sigma_2} -1}(1-{\sigma_1})}{M({\sigma_1})}\mathbb{G}_4(t, S_c, I_c, R_c, S_\nu, I_\nu, E) \nonumber \\ & + \frac{{\sigma_1} {\sigma_2}}{M({\sigma_1})}\int_{0}^{t}z^{{\sigma_2} -1}\mathbb{G}_4(z, S_c, I_c, R_c, S_\nu, I_\nu, E)dz, \\ I_{\nu}(t) - I_{\nu}(0) = &\frac{{\sigma_2} t^{{\sigma_2} -1}(1-{\sigma_1})}{M({\sigma_1})}\mathbb{G}_5(t, S_c, I_c, R_c, S_\nu, I_\nu, E) \nonumber \\ & + \frac{{\sigma_1} {\sigma_2}}{M({\sigma_1})}\int_{0}^{t}z^{{\sigma_2} -1}\mathbb{G}_5(z, S_c, I_c, R_c, S_\nu, I_\nu, E)dz, \\ E(t) - E(0) = &\frac{{\sigma_2} t^{{\sigma_2} -1}(1-{\sigma_1})}{M({\sigma_1})}\mathbb{G}_6(t, S_c, I_c, R_c, S_\nu, I_\nu, E) \nonumber \\ & + \frac{{\sigma_1} {\sigma_2}}{M({\sigma_1})}\int_{0}^{t}z^{{\sigma_2} -1}\mathbb{G}_6(z, S_c, I_c, R_c, S_\nu, I_\nu, E)dz. \end{align*} | (5.2) |
Initially, we merely solve the system (5.2) first equation. After that, other equations also follow similar analogous processes to the first equation.
\begin{align} S_c(t) - S_c(0) & = \frac{{\sigma_2} t^{{\sigma_2} -1}(1-{\sigma_1})}{M({\sigma_1})}\mathbb{G}_1(t, S_c, I_c, R_c, S_\nu, I_\nu, E) \\ & + \frac{{\sigma_1} {\sigma_2}}{M({\sigma_1})}\int_{0}^{t}z^{{\sigma_2} -1}\mathbb{G}_1(z, S_c, I_c, R_c, S_\nu, I_\nu, E)dz. \end{align} | (5.3) |
We put t = t_{n+1} in Eq (5.3), then we get
\begin{align} S_c(t_{n+1}) - S_c(0) = &\frac{{\sigma_2} t^{{\sigma_2} -1}_n(1-{\sigma_1})}{M({\sigma_1})}\mathbb{G}_1(t_n, S^n_c, I^n_c, R^n_c, S^n_\nu, I^n_\nu, E^n) \\ & + \frac{{\sigma_1} {\sigma_2}}{M({\sigma_1})}\int_{0}^{t_{n+1}}z^{{\sigma_2} -1}\mathbb{G}_1(z, S_c, I_c, R_c, S_\nu, I_\nu, E)dz. \end{align} | (5.4) |
Now, we simplified Eq (5.4), and we get
\begin{align} S_c(t_{n+1}) = & S_c(0) + \frac{{\sigma_2} t^{{\sigma_2} -1}_n(1-{\sigma_1})}{M({\sigma_1})}\mathbb{G}_1(t_n, S^n_c, I^n_c, R^n_c, S^n_\nu, I^n_\nu, E^n) \\ & - \frac{{\sigma_2} t^{{\sigma_2} -1}_{n-1}(1-{\sigma_1})}{M({\sigma_1})}\mathbb{G}_1(t_{n-1}, S^{n-1}_c, I^{n-1}_c, R^{n-1}_c, S^{n-1}_\nu, I^{n-1}_\nu, E^{n-1}) \\ & + \frac{{\sigma_1} {\sigma_2}}{M({\sigma_1})}\int_{t_n}^{t_{n+1}}z^{{\sigma_2} -1}\mathbb{G}_1(z, S_c, I_c, R_c, S_\nu, I_\nu, E)dz . \end{align} | (5.5) |
The following outcome is obtained using the Lagrange polynomial concept:
\begin{align} S_c(t_{n+1}) = &S_c(0) + \frac{{\sigma_2} t^{{\sigma_2} -1}_n(1-{\sigma_1})}{M({\sigma_1})}\mathbb{G}_1(t_n, S^n_c, I^n_c, R^n_c, S^n_\nu, I^n_\nu, E^n) \\ & - \frac{{\sigma_2} t^{{\sigma_2} -1}_{n-1}(1-{\sigma_1})}{M({\sigma_1})}\mathbb{G}_1(t_{n-1}, S^{n-1}_c, I^{n-1}_c, R^{n-1}_c, S^{n-1}_\nu, I^{n-1}_\nu, E^{n-1}) \\ & + \frac{{\sigma_1} {\sigma_2} h}{M({\sigma_1})}\left[ \frac{3 t^{{\sigma_2} -1}_n}{2} \mathbb{G}_1(t_n, S^n_c, I^n_c, R^n_c, S^n_\nu, I^n_\nu, E^n) \right. \\ & \left. - \frac{t^{{\sigma_2} -1}_{n-1}}{2}\mathbb{G}_1(t_{n-1}, S^{n-1}_c, I^{n-1}_c, R^{n-1}_c, S^{n-1}_\nu, I^{n-1}_\nu, E^{n-1}) \right] . \end{align} | (5.6) |
Further, we simplify the Eq (5.6), then we get
\begin{align} S^{n+1}_c = &S_c(t_{n+1}) \\ = & S_c(0) + {\sigma_2} t^{{\sigma_2} -1}_n\left( \frac{1 - {\sigma_1}}{M({\sigma_1})} + \frac{3 {\sigma_1} h}{2M({\sigma_1})}\right)\mathbb{G}_1(t_n, S^n_c, I^n_c, R^n_c, S^n_\nu, I^n_\nu, E^n) \\ & - {\sigma_2} t^{{\sigma_2} -1}_{n-1}\left( \frac{1 - {\sigma_1}}{M({\sigma_1})} + \frac{ {\sigma_1} h}{2M({\sigma_1})}\right)\mathbb{G}_1(t_{n-1}, S^{n-1}_c, I^{n-1}_c, R^{n-1}_c, S^{n-1}_\nu, I^{n-1}_\nu, E^{n-1}). \end{align} | (5.7) |
The previous similar process applies to other equations, then we get
\begin{align*} I^{n+1}_c = &I_c(t_{n+1}) \nonumber \\ = &I_c(0) + {\sigma_2} t^{{\sigma_2} -1}_n\left( \frac{1 - {\sigma_1}}{M({\sigma_1})} + \frac{3 {\sigma_1} h}{2M({\sigma_1})}\right)\mathbb{G}_2(t_n, S^n_c, I^n_c, R^n_c, S^n_\nu, I^n_\nu, E^n) \nonumber \\ & - {\sigma_2} t^{{\sigma_2} -1}_{n-1}\left( \frac{1 - {\sigma_1}}{M({\sigma_1})} + \frac{ {\sigma_1} h}{2M({\sigma_1})}\right)\mathbb{G}_2(t_{n-1}, S^{n-1}_c, I^{n-1}_c, R^{n-1}_c, S^{n-1}_\nu, I^{n-1}_\nu, E^{n-1}), \end{align*} |
\begin{align*} R^{n+1}_c = & R_c(t_{n+1}) \nonumber \\ = & R_c(0) + {\sigma_2} t^{{\sigma_2} -1}_n\left( \frac{1 - {\sigma_1}}{M({\sigma_1})} + \frac{3 {\sigma_1} h}{2M({\sigma_1})}\right)\mathbb{G}_3(t_n, S^n_c, I^n_c, R^n_c, S^n_\nu, I^n_\nu, E^n) \nonumber \\ & - {\sigma_2} t^{{\sigma_2} -1}_{n-1}\left( \frac{1 - {\sigma_1}}{M({\sigma_1})} + \frac{ {\sigma_1} h}{2M({\sigma_1})}\right)\mathbb{G}_3(t_{n-1}, S^{n-1}_c, I^{n-1}_c, R^{n-1}_c, S^{n-1}_\nu, I^{n-1}_\nu, E^{n-1}), \end{align*} |
\begin{align} S^{n+1}_{\nu} = &S_{\nu}(t_{n+1}) \\ = & S_{\nu}(0) + {\sigma_2} t^{{\sigma_2} -1}_n\left( \frac{1 - {\sigma_1}}{M({\sigma_1})} + \frac{3 {\sigma_1} h}{2M({\sigma_1})}\right)\mathbb{G}_4(t_n, S^n_c, I^n_c, R^n_c, S^n_\nu, I^n_\nu, E^n) \\ & - {\sigma_2} t^{{\sigma_2} -1}_{n-1}\left( \frac{1 - {\sigma_1}}{M({\sigma_1})} + \frac{ {\sigma_1} h}{2M({\sigma_1})}\right)\mathbb{G}_4(t_{n-1}, S^{n-1}_c, I^{n-1}_c, R^{n-1}_c, S^{n-1}_\nu, I^{n-1}_\nu, E^{n-1}), \end{align} | (5.8) |
\begin{align*} I^{n+1}_{\nu} & = I_{\nu}(t_{n+1}) \nonumber \\ & = I_{\nu}(0) + {\sigma_2} t^{{\sigma_2} -1}_n\left( \frac{1 - {\sigma_1}}{M({\sigma_1})} + \frac{3 {\sigma_1} h}{2M({\sigma_1})}\right)\mathbb{G}_5(t_n, S^n_c, I^n_c, R^n_c, S^n_\nu, I^n_\nu, E^n) \nonumber \\ &\quad - {\sigma_2} t^{{\sigma_2} -1}_{n-1}\left( \frac{1 - {\sigma_1}}{M({\sigma_1})} + \frac{ {\sigma_1} h}{2M({\sigma_1})}\right)\mathbb{G}_5(t_{n-1}, S^{n-1}_c, I^{n-1}_c, R^{n-1}_c, S^{n-1}_\nu, I^{n-1}_\nu, E^{n-1}), \end{align*} |
\begin{align*} E^{n+1} & = E(t_{n+1}) \nonumber \\ & = E(0) + {\sigma_2} t^{{\sigma_2} -1}_n\left( \frac{1 - {\sigma_1}}{M({\sigma_1})} + \frac{3 {\sigma_1} h}{2M({\sigma_1})}\right)\mathbb{G}_6(t_n, S^n_c, I^n_c, R^n_c, S^n_\nu, I^n_\nu, E^n) \nonumber \\ &\quad - {\sigma_2} t^{{\sigma_2} -1}_{n-1}\left( \frac{1 - {\sigma_1}}{M({\sigma_1})} + \frac{ {\sigma_1} h}{2M({\sigma_1})}\right)\mathbb{G}_6(t_{n-1}, S^{n-1}_c, I^{n-1}_c, R^{n-1}_c, S^{n-1}_\nu, I^{n-1}_\nu, E^{n-1}). \end{align*} |
We begin with a power-law scenario and use numerical techniques based on the proposed model.
\begin{align} {}_{\;\;0+}^{FFP}\mathfrak{D}^{{\sigma_1}, {{\sigma_2}}}_{t}S_c(t) & = \Pi_1 - \lambda S_c - \mu S_c, \\ {}_{\;\;0+}^{FFP}\mathfrak{D}^{{\sigma_1}, {{\sigma_2}}}_{t}I_c(t) & = \lambda S_c - \mu I_c - \delta I_c , \\ {}_{\;\;0+}^{FFP}\mathfrak{D}^{{\sigma_1}, {{\sigma_2}}}_{t}R_c(t) & = \delta I_c - \mu R_c , \\ {}_{\;\;0+}^{FFP}\mathfrak{D}^{{\sigma_1}, {{\sigma_2}}}_{t}S_\nu(t) & = \Pi_2 - \mu_\nu S_\nu - \frac{\beta_4 S_\nu I_c}{N_c} , \\ {}_{\;\;0+}^{FFP}\mathfrak{D}^{{\sigma_1}, {{\sigma_2}}}_{t}I_\nu(t) & = \frac{\beta_4 S_\nu I_c}{N_c} - \mu_\nu I_\nu , \\ {}_{\;\;0+}^{FFP}\mathfrak{D}^{{\sigma_1}, {{\sigma_2}}}_{t}E(t) & = \phi I_c - \omega E . \end{align} | (5.9) |
To express the LSD model before implementing the scheme, we employ the RL notion of Volterra representation [38],
\begin{align*} {}_{\;\;0+}^{FFP}\mathfrak{D}^{{\sigma_1}, {\sigma_2}}_{t}\mathfrak{E}(t) = \frac{1}{\Gamma(1-{\sigma_1})}\frac{d}{dt}\int_{0}^{t}\frac{(t - z)^{-{\sigma_1}}}{{\sigma_2} t^{{\sigma_2}-1}}{\mathfrak{E}(z)}dz . \end{align*} |
The fractional order system is under consideration, with the following results shown:
\begin{align} & {}_{0+}^{RL}\mathfrak{D}^{{\sigma_1}}_{t}S_c(t) = {\sigma_2} t^{{\sigma_2} - 1}\left[ \mathbb{G}_1(t, S_c, I_c, R_c, S_\nu, I_\nu, E) \right] , \\ & {}_{0+}^{RL}\mathfrak{D}^{{\sigma_1}}_{t}I_c(t) = {\sigma_2} t^{{\sigma_2} - 1}\left[ \mathbb{G}_2(t, S_c, I_c, R_c, S_\nu, I_\nu, E) \right] , \\ & {}_{0+}^{RL}\mathfrak{D}^{{\sigma_1}}_{t}R_c(t) = {\sigma_2} t^{{\sigma_2} - 1}\left[ \mathbb{G}_3(t, S_c, I_c, R_c, S_\nu, I_\nu, E) \right] , \\ & {}_{0+}^{RL}\mathfrak{D}^{{\sigma_1}}_{t}S_\nu(t) = {\sigma_2} t^{{\sigma_2} - 1}\left[ \mathbb{G}_4(t, S_c, I_c, R_c, S_\nu, I_\nu, E) \right] , \\ & {}_{0+}^{RL}\mathfrak{D}^{{\sigma_1}}_{t}I_\nu(t) = {\sigma_2} t^{{\sigma_2} - 1}\left[ \mathbb{G}_5(t, S_c, I_c, R_c, S_\nu, I_\nu, E) \right] , \\ & {}_{0+}^{RL}\mathfrak{D}^{{\sigma_1}}_{t}E(t) = {\sigma_2} t^{{\sigma_2} - 1}\left[ \mathbb{G}_6(t, S_c, I_c, R_c, S_\nu, I_\nu, E) \right], \end{align} | (5.10) |
where
\begin{align} \mathbb{G}_1(t, S_c, I_c, R_c, S_\nu, I_\nu, E) & = \Pi_1 - \lambda S_c - \mu S_c, \\ \mathbb{G}_2(t, S_c, I_c, R_c, S_\nu, I_\nu, E) & = \lambda S_c - \mu I_c - \delta I_c , \\ \mathbb{G}_3(t, S_c, I_c, R_c, S_\nu, I_\nu, E) & = \delta I_c - \mu R_c , \\ \mathbb{G}_4(t, S_c, I_c, R_c, S_\nu, I_\nu, E) & = \Pi_2 - \mu_\nu S_\nu - \frac{\beta_4 S_\nu I_c}{N_c} , \\ \mathbb{G}_5(t, S_c, I_c, R_c, S_\nu, I_\nu, E) & = \frac{\beta_4 S_\nu I_c}{N_c} - \mu_\nu I_\nu , \\ \mathbb{G}_6(t, S_c, I_c, R_c, S_\nu, I_\nu, E) & = \phi I_c - \omega E . \end{align} | (5.11) |
The F-F LSD model is numerically forged by applying the RL fractional integral to the system (5.10), which yields the following results:
\begin{align} & S_c(t) - S_c(0) = \frac{{\sigma_2}}{\Gamma({\sigma_1})} \int_{0}^{t}z^{{\sigma_2} -1}(t-z)^{{\sigma_1} -1}\mathbb{G}_1(z, S_c, I_c, R_c, S_\nu, I_\nu, E)dz, \\ & I_c(t) - I_c(0) = \frac{{\sigma_2}}{\Gamma({\sigma_1})} \int_{0}^{t}z^{{\sigma_2} -1}(t-z)^{{\sigma_1} -1}\mathbb{G}_2(z, S_c, I_c, R_c, S_\nu, I_\nu, E)dz, \\ & R_c(t) - R_c(0) = \frac{{\sigma_2}}{\Gamma({\sigma_1})} \int_{0}^{t}z^{{\sigma_2} -1}(t-z)^{{\sigma_1} -1}\mathbb{G}_3(z, S_c, I_c, R_c, S_\nu, I_\nu, E)dz, \\ & S_\nu(t) - S_\nu(0) = \frac{{\sigma_2}}{\Gamma({\sigma_1})} \int_{0}^{t}z^{{\sigma_2} -1}(t-z)^{{\sigma_1} -1}\mathbb{G}_4(z, S_c, I_c, R_c, S_\nu, I_\nu, E)dz , \\ & I_\nu(t) - I_\nu(0) = \frac{{\sigma_2}}{\Gamma({\sigma_1})} \int_{0}^{t}z^{{\sigma_2} -1}(t-z)^{{\sigma_1} -1}\mathbb{G}_5(z, S_c, I_c, R_c, S_\nu, I_\nu, E)dz , \\ & E(t) - E(0) = \frac{{\sigma_2}}{\Gamma({\sigma_1})} \int_{0}^{t}z^{{\sigma_2} -1}(t-z)^{{\sigma_1} -1}\mathbb{G}_6(z, S_c, I_c, R_c, S_\nu, I_\nu, E)dz . \end{align} | (5.12) |
Initially, we merely solve the system (5.12) first equation. After that, other equations also follow similar analogous processes to the first equation.
\begin{align} & S_c(t) - S_c(0) = \frac{{\sigma_2}}{\Gamma({\sigma_1})} \int_{0}^{t}z^{{\sigma_2} -1}(t-z)^{{\sigma_1} -1}\mathbb{G}_1(z, S_c, I_c, R_c, S_\nu, I_\nu, E)dz. \end{align} | (5.13) |
Put t = t_{n+1} in Eq (5.13), then we get
\begin{align} & S_c(t_{n+1}) - S_c(0) = \frac{{\sigma_2}}{\Gamma({\sigma_1})} \int_{0}^{t_{n+1}}z^{{\sigma_2} -1}(t_{n+1}-z)^{{\sigma_1} -1}\mathbb{G}_1(z, S_c, I_c, R_c, S_\nu, I_\nu, E)dz . \end{align} | (5.14) |
We simplify the Eq (5.14), and we have
\begin{align} S_c(t_{n+1}) & = S_c(0) + \frac{{\sigma_2}}{\Gamma({\sigma_1})} \sum\limits_{\mathfrak{r} = 0}^{n} \int_{t_\mathfrak{r}}^{t_{\mathfrak{r}+1}}z^{{\sigma_2} -1}(t_{n+1}-z)^{{\sigma_1} -1}\mathbb{G}_1(z, S_c, I_c, R_c, S_\nu, I_\nu, E)dz, \end{align} | (5.15) |
with the help of the Lagrangian interpolation technique for finding the approximate function z^{{\sigma_2} -1} \mathbb{G}_1(z, S_c, I_c, R_c, S_\nu, I_\nu, E) in the interval [t_{\mathfrak{r}}, t_{\mathfrak{r}+1}] into Eq (5.15), then we get
\begin{align} \mathscr{A}^1_\mathfrak{r}(z) = & \frac{z - t_{\mathfrak{r}-1}}{t_\mathfrak{r} - t_{\mathfrak{r}-1}}t^{{\sigma_2} -1}_{\mathfrak{r}}\mathbb{G}_1(t_{\mathfrak{r}}, S^{\mathfrak{r}}_c, I^{\mathfrak{r}}_c, R^{\mathfrak{r}}_c, S^{\mathfrak{r}}_{\nu}, I^{\mathfrak{r}}_{\nu}, E^{\mathfrak{r}}) \\ & - \frac{z - t_{\mathfrak{r}}}{t_\mathfrak{r} - t_{\mathfrak{r}-1}}t^{{\sigma_2} -1}_{\mathfrak{r}-1}\mathbb{G}_1(t_{\mathfrak{r}-1}, S^{\mathfrak{r}-1}_c, I^{\mathfrak{r}-1}_c, R^{\mathfrak{r}-1}_c, S^{\mathfrak{r}-1}_{\nu}, I^{\mathfrak{r}-1}_{\nu}, E^{\mathfrak{r}-1}). \end{align} | (5.16) |
We apply Eq (5.16) into Eq (5.15), then we get
\begin{align} S^{n+1}_c & = S_c(t_{n+1}) = S_c(0) + \frac{{\sigma_2}}{\Gamma({\sigma_1})} \sum\limits_{\mathfrak{r} = 0}^{n} \int_{t_\mathfrak{r}}^{t_{\mathfrak{r}+1}}z^{{\sigma_2} -1}(t_{n+1}-z)^{{\sigma_1} -1}\mathscr{A}^1_\mathfrak{r}(z)dz, \end{align} | (5.17) |
Eq (5.17) can be solved further to produce the following results,
\begin{align} S^{n+1}_c = & S_c(0) + \frac{h^{{\sigma_1}}{\sigma_2}}{\Gamma({\sigma_1} +2)} \sum\limits_{\mathfrak{r} = 1}^{n}\left[ t^{{\sigma_2} -1}_{\mathfrak{r}}\mathbb{G}_1(t_{\mathfrak{r}}, S^{\mathfrak{r}}_c, I^{\mathfrak{r}}_c, R^{\mathfrak{r}}_c, S^{\mathfrak{r}}_{\nu}, I^{\mathfrak{r}}_{\nu}, E^{\mathfrak{r}}){\mathfrak{a}_{n, \mathfrak{r}}} \right. \\ & \left. - t^{{\sigma_2} -1}_{\mathfrak{r}-1}\mathbb{G}_1(t_{\mathfrak{r}-1}, S^{\mathfrak{r}-1}_c, I^{\mathfrak{r}-1}_c, R^{\mathfrak{r}-1}_c, S^{\mathfrak{r}-1}_{\nu}, I^{\mathfrak{r}-1}_{\nu}, E^{\mathfrak{r}-1}){\mathfrak{b}_{n, \mathfrak{r}}} \right], \end{align} | (5.18) |
where
\begin{align*} \mathfrak{a}_{n, \mathfrak{r}} & = \left[ (n - \mathfrak{r} +1)^{{\sigma_1}}(n - \mathfrak{r} + {\sigma_1} + 2) - (n - \mathfrak{r})^{{\sigma_1}} (n-\mathfrak{r} + 2{\sigma_1} +2)\right], \nonumber \\ \mathfrak{b}_{n, \mathfrak{r}} & = \left[ (n - \mathfrak{r} +1)^{{\sigma_1}+1} - (n - \mathfrak{r})^{{\sigma_1}} (n-\mathfrak{r} + {\sigma_1} +1)\right], \end{align*} |
n = 0, 1, 2, ..., N , and \mathfrak{r} = 1, 2, 3, ..., n. The previous similar process applies to other equations, then we get
\begin{align} I^{n+1}_c = & I_c(0) + \frac{h^{{\sigma_1}}{\sigma_2}}{\Gamma({\sigma_1} +2)} \sum\limits_{\mathfrak{r} = 1}^{n}\left[ t^{{\sigma_2} -1}_{\mathfrak{r}}\mathbb{G}_2(t_{\mathfrak{r}}, S^{\mathfrak{r}}_c, I^{\mathfrak{r}}_c, R^{\mathfrak{r}}_c, S^{\mathfrak{r}}_{\nu}, I^{\mathfrak{r}}_{\nu}, E^{\mathfrak{r}}){\mathfrak{a}_{n, \mathfrak{r}}} \right. \\ & \left. - t^{{\sigma_2} -1}_{\mathfrak{r}-1}\mathbb{G}_2(t_{\mathfrak{r}-1}, S^{\mathfrak{r}-1}_c, I^{\mathfrak{r}-1}_c, R^{\mathfrak{r}-1}_c, S^{\mathfrak{r}-1}_{\nu}, I^{\mathfrak{r}-1}_{\nu}, E^{\mathfrak{r}-1}){\mathfrak{b}_{n, \mathfrak{r}}} \right], \\ R^{n+1}_c = & R_c(0) + \frac{h^{{\sigma_1}}{\sigma_2}}{\Gamma({\sigma_1} +2)} \sum\limits_{\mathfrak{r} = 1}^{n}\left[ t^{{\sigma_2} -1}_{\mathfrak{r}}\mathbb{G}_3(t_{\mathfrak{r}}, S^{\mathfrak{r}}_c, I^{\mathfrak{r}}_c, R^{\mathfrak{r}}_c, S^{\mathfrak{r}}_{\nu}, I^{\mathfrak{r}}_{\nu}, E^{\mathfrak{r}}){\mathfrak{a}_{n, \mathfrak{r}}} \right. \\ & \left. - t^{{\sigma_2} -1}_{\mathfrak{r}-1}\mathbb{G}_3(t_{\mathfrak{r}-1}, S^{\mathfrak{r}-1}_c, I^{\mathfrak{r}-1}_c, R^{\mathfrak{r}-1}_c, S^{\mathfrak{r}-1}_{\nu}, I^{\mathfrak{r}-1}_{\nu}, E^{\mathfrak{r}-1}){\mathfrak{b}_{n, \mathfrak{r}}} \right], \\ S^{n+1}_{\nu} = & S_{\nu}(0) + \frac{h^{{\sigma_1}}{\sigma_2}}{\Gamma({\sigma_1} +2)} \sum\limits_{\mathfrak{r} = 1}^{n}\left[ t^{{\sigma_2} -1}_{\mathfrak{r}}\mathbb{G}_4(t_{\mathfrak{r}}, S^{\mathfrak{r}}_c, I^{\mathfrak{r}}_c, R^{\mathfrak{r}}_c, S^{\mathfrak{r}}_{\nu}, I^{\mathfrak{r}}_{\nu}, E^{\mathfrak{r}}){\mathfrak{a}_{n, \mathfrak{r}}} \right. \\ & \left. - t^{{\sigma_2} -1}_{\mathfrak{r}-1}\mathbb{G}_4(t_{\mathfrak{r}-1}, S^{\mathfrak{r}-1}_c, I^{\mathfrak{r}-1}_c, R^{\mathfrak{r}-1}_c, S^{\mathfrak{r}-1}_{\nu}, I^{\mathfrak{r}-1}_{\nu}, E^{\mathfrak{r}-1}){\mathfrak{b}_{n, \mathfrak{r}}} \right], \\ I^{n+1}_{\nu} = & I_{\nu}(0) + \frac{h^{{\sigma_1}}{\sigma_2}}{\Gamma({\sigma_1} +2)} \sum\limits_{\mathfrak{r} = 1}^{n}\left[ t^{{\sigma_2} -1}_{\mathfrak{r}}\mathbb{G}_5(t_{\mathfrak{r}}, S^{\mathfrak{r}}_c, I^{\mathfrak{r}}_c, R^{\mathfrak{r}}_c, S^{\mathfrak{r}}_{\nu}, I^{\mathfrak{r}}_{\nu}, E^{\mathfrak{r}}){\mathfrak{a}_{n, \mathfrak{r}}} \right. \\ & \left. - t^{{\sigma_2} -1}_{\mathfrak{r}-1}\mathbb{G}_5(t_{\mathfrak{r}-1}, S^{\mathfrak{r}-1}_c, I^{\mathfrak{r}-1}_c, R^{\mathfrak{r}-1}_c, S^{\mathfrak{r}-1}_{\nu}, I^{\mathfrak{r}-1}_{\nu}, E^{\mathfrak{r}-1}){\mathfrak{b}_{n, \mathfrak{r}}} \right], \\ E^{n+1} = & E(0) + \frac{h^{{\sigma_1}}{\sigma_2}}{\Gamma({\sigma_1} +2)} \sum\limits_{\mathfrak{r} = 1}^{n}\left[ t^{{\sigma_2} -1}_{\mathfrak{r}}\mathbb{G}_6(t_{\mathfrak{r}}, S^{\mathfrak{r}}_c, I^{\mathfrak{r}}_c, R^{\mathfrak{r}}_c, S^{\mathfrak{r}}_{\nu}, I^{\mathfrak{r}}_{\nu}, E^{\mathfrak{r}}){\mathfrak{a}_{n, \mathfrak{r}}} \right. \\ & \left. - t^{{\sigma_2} -1}_{\mathfrak{r}-1}\mathbb{G}_6(t_{\mathfrak{r}-1}, S^{\mathfrak{r}-1}_c, I^{\mathfrak{r}-1}_c, R^{\mathfrak{r}-1}_c, S^{\mathfrak{r}-1}_{\nu}, I^{\mathfrak{r}-1}_{\nu}, E^{\mathfrak{r}-1}){\mathfrak{b}_{n, \mathfrak{r}}} \right]. \end{align} | (5.19) |
The integral and differential operators with an exponential kernel and a power law kernel are used instead of classical operators. The F-F derivative has been used to analyze and describe the revised LSD model, improving its understanding of qualitative and numerical components. To demonstrate the effectiveness of the suggested strategy, simulations have been performed using MATLAB [39]. The Adam-Bashborth scheme has been used to calculate the numerical solution for the proposed LSD model. The effectiveness and applicability of this technique have been established through the numerical solution of the LSD model using the F-F derivative. The parameter values and initial conditions used for simulation are derived as follows: S_c(0) = 5000, R_c(0) = 0, I_c(0) = 50, S_v(0) = 10000, E(0) = 500, I_v(0) = 100, \Pi_1 = 0.8, \delta = 0.008, \mu = \frac{1}{6\times 365}, \Pi_2 = 0.1, \beta_1 = 0.030013, \mu_v = 0.004, \beta_2 = 0.1010, \beta_3 = 0.01120, \beta_4 = 0.03, \omega = 0.001013 , and \phi = 0.001.
Table 1 represents a source of the parameters and stationary variables. Consideration has been given to significant factors and how they can contribute to the control of diseases. Figures 2 and 3 show the time series plot of the LSD model (3.2) at different fractional orders \sigma_1 and fixed fractal dimensions \sigma_2 = 1 using the F-F C-F operator. These graphs show a better understanding about the behavior of the proposed LSD model compared to the classical derivative. Figures 4 and 5 show the time series plot of the LSD model (3.2) at different fractal dimensions \sigma_2 and fixed fractional orders \sigma_1 = 1 using the F-F C-F operator. Figures 6 and 7 exhibit other time series diagrams of the LSD model (3.2) at different fractional orders \sigma_1 and fractal dimensions \sigma_2 using the F-F Caputo operator. Figures 2 through 5 demonstrate the numerical simulation of the proposed model, considering fractional order variations. It is important to reduce the vector to manage the infection in the cattle population, as the contact between the vector and cattle can further promote the spread of the virus in the community. Our data shows that reducing the fractional order number has led to a decrease in the population of infected classes. Furthermore, we have also investigated the effect of some parameters on the population of the LSD model such that we analyzed the effect of parameters in Figure 8 to Figure 15 in the susceptible cattle S_c , infected cattle I_c , recovered cattle R_c , susceptible insects S_v , infected insects I_v , and contaminated environment E populations. Figures 8 and 9 depict the population behavior of the LSD model at different parameter values \delta when \sigma_1, \sigma_2 = 0.99 . Figures 10 and 11 show the population behavior of the LSD model at different parameter values \beta_1 when \sigma_1, \sigma_2 = 0.99 . To illustrate this, Figures 10 and 11 demonstrate the dynamics of lumpy skin conditions with various \beta_1 values taken into account. To prevent the disease from spreading to other animals, it is important to make efforts to eliminate the mosquitoes, flies, and other ticks that cause the disease in cattle. As shown in Figure 10, reducing the value of \beta_1 (spraying on flies, mosquitoes, and ticks) has helped reduce the population of infected classes. Similarly, by lowering the value of \beta_1 (as shown in Figure 11), it is possible to reduce the infection in the environment and the infected vector. LSD, a disease that affects farm animals, is primarily spread through the bites of flies, mosquitoes, and ticks. To safeguard cattle, it is crucial to develop and execute an integrated insect control plan. This plan should include comprehensive measures to disrupt the breeding cycle of stable flies and mosquitoes at every stage of their life cycle. Tick control measures should also be taken into account. Figures 12 and 13 depict the population behavior of the LSD model at different parameter values \beta_2 when \sigma_1, \sigma_2 = 0.99 . Figures 12 and 13 show how the dynamics of the LSD vary with different values of the parameter \beta_2 . This disease has spread among animals because of viral pollution in the atmosphere. To reduce the infection rate among cattle, it's essential to decrease the level of contamination in their surroundings, such as factors and other areas they come into contact with. Proper environmental management can help achieve this by reducing the number of insect breeding grounds and roosting places. To prevent mosquito breeding, ensure that there is no standing water in containers and check that drains are not obstructed. You can also use techniques like residual spraying and fogging to prevent the use of adulthood. It's important to maintain documentation of chemical and mosquito control measures. Figures 14 and 15 describe the population behavior of the LSD model at different parameter values \beta_3 when \sigma_1, \sigma_2 = 0.98 . Figures 14 and 15 illustrate the changes in LSD dynamics because of the \beta_3 variant. The transmission of this disease directly from cattle to cattle is a significant threat to our cattle population. As we can observe from the figures, reducing the value of \beta_3 is crucial in minimizing the spread of the infected compartments.
Parameters | Source |
S_c(t) | [37] |
I_c(t) | [37] |
R_c(t) | [37] |
S_v(t) | [37] |
I_v(t) | [37] |
E(t) | [37] |
\Pi_1 | [37] |
\mu | [37] |
\delta | [37] |
\Pi_2 | [37] |
\mu_v | [37] |
\beta_1 | [37] |
\beta_2 | [37] |
\beta_3 | [37] |
\beta_4 | [37] |
\phi | [37] |
\omega | [37] |
In this research, we examine a fascinating mathematical model to examine the dynamics of the cattle population. The LSD model predominantly affects the cattle animal population more than the human population. We have identified the crucial pathway of transmission of LSD in cattle in the model. The primary routes of disease transmission from the environment are direct transmission from healthy cattle to diseased cattle and illness contracted from susceptible cattle through vectors. To establish the existence and uniqueness of the disease model, we used fixed point theory and its fundamentals. For the proposed model, we have the numerical results with the help of the fractal fractional operator. After considering sensitive parameters essential for treating diseases and factors such as \beta_{i}, i = 1, 2, 3 , graphical results are produced. An arbitrary-order model is capable of displaying more complex nonlinear dynamics. By examining the graphical representations of such models, we can observe the impact of derivative order and parameters on arbitrary-order LSD systems. Based on the graphical analysis, cattle-to-cattle, environmental, and reducing vector transmission can help effectively manage the spread of infection within the cattle herd. We found that sanitizing and washing the surroundings created by human foot traffic and moving vehicles can stop the virus from spreading across the community. In the future we will focus on analyzing the delay and Hopf bifurcation of the LSD system as well. It can provide valuable insights for future exploration and implementation of the proposed system.
Parveen Kumar: Formal analysis, methodology, writing-original draft, validation, software; Sunil Kumar: Investigation, conceptualization, supervision, writing-review and editing; Badr Saad T Alkahtani: Visualization, validation, conceptualization, writing-review and editing; Sara S Alzaid: Visualization, formal analysis, investigation, writing-original draft. All authors of this article have been contributed equally. All authors have read and approved the final version of the manuscript for publication.
The authors declare they have not used Artificial Intelligence (AI) tools in the creation of this article.
Researchers Supporting Project number (RSPD2024R526), King Saud University, Riyadh, Saudi Arabia.
Data available on request from the authors.
All authors declare no conflicts of interest.
[1] |
A. Abiad, B. Brimkov, S. Hayat, A. P. Khramova, J. H. Koolen, Extending a conjecture of Graham and Lovász on the distance characteristic polynomial, Linear Algebra Appl., 693 (2024), 63–82. https://doi.org/10.1016/j.laa.2023.03.027 doi: 10.1016/j.laa.2023.03.027
![]() |
[2] |
K. Balasubramanian, K. Kaipa, H. Khurana, On the cardinality of matrices with prescribed rank and partial trace over a finite field, Linear Algebra Appl., 704 (2025), 35–57. https://doi.org/10.1016/j.laa.2024.10.011 doi: 10.1016/j.laa.2024.10.011
![]() |
[3] |
M. Deaconescu, Adding units mod n, Elem. Math., 55 (2000), 123–127. https://doi.org/10.1007/s000170050078 doi: 10.1007/s000170050078
![]() |
[4] | P. Feinsilver, R. Schott, Algebraic structures and operator calculus: Volume II: special functions and computer science, Dordrecht: Springer, 1994. https://doi.org/10.1007/978-0-585-28003-5 |
[5] | F. R. Gantmacher, Matrizentheorie, Berlin, Heidelberg: Springer, 1986. https://doi.org/10.1007/978-3-642-71243-2 |
[6] |
S. Hayat, A. Khan, M. J. F. Alenazi, On some distance spectral characteristics of trees, Axioms, 13 (2024), 494. https://doi.org/10.3390/axioms13080494 doi: 10.3390/axioms13080494
![]() |
[7] | H. Iwaniec, Topics in classical automorphic forms, Providence: American Mathematical Society, 1997. http://doi.org/10.1090/gsm/017 |
[8] |
J. H. Koolen, M. Abdullah, B. Gebremichel, S. Hayat, Distance-regular graphs with exactly one positive q-distance eigenvalue, Linear Algebra Appl., 689 (2024), 230–246. https://doi.org/10.1016/j.laa.2024.02.030 doi: 10.1016/j.laa.2024.02.030
![]() |
[9] |
G. Landsberg, Ueber eine anzahlbestimmung und eine damit zusammenhängende reihe, J. Reine Angew. Math. 1893 (1893), 87–88. https://doi.org/10.1515/crll.1893.111.87 doi: 10.1515/crll.1893.111.87
![]() |
[10] |
Y. Li, S. Hu, Gauss sums over some matrix groups, J. Number Theory, 132 (2012), 2967–2976. http://doi.org/10.1016/j.jnt.2012.06.010 doi: 10.1016/j.jnt.2012.06.010
![]() |
[11] |
C. Miguel, On the sumsets of exceptional units in a finite commutative ring, Monatsh. Math., 186 (2018), 315–320. https://doi.org/10.1007/s00605-017-1070-x doi: 10.1007/s00605-017-1070-x
![]() |
[12] | J. S. Respondek, Fast matrix multiplication with applications, Cham: Springer, 2025. |
[13] |
J. W. Sander, On the addition of units and nonunits \pmod{m}, J. Number Theory, 129 (2009), 2260–2266. https://doi.org/10.1016/j.jnt.2009.04.010 doi: 10.1016/j.jnt.2009.04.010
![]() |
[14] |
J. W. Sander, T. Sander, Adding generators in cyclic groups, J. Number Theory, 133 (2013), 705–718. https://doi.org/10.1016/j.jnt.2012.08.021 doi: 10.1016/j.jnt.2012.08.021
![]() |
[15] |
C. F. Sun, Q. H. Yang, On the sumset of atoms in cyclic groups, Int. J. Number Theory, 10 (2014), 1355–1363. https://doi.org/10.1142/S1793042114500328 doi: 10.1142/S1793042114500328
![]() |
[16] |
Q. H. Yang, Q. Q. Zhao, On the sumsets of exceptional units in \mathbb{Z}_n, Monatsh. Math., 182 (2017), 489–493. https://doi.org/10.1007/s00605-015-0872-y doi: 10.1007/s00605-015-0872-y
![]() |
[17] |
X. Zhang, C. G. Ji, Sums of generators of ideals in residue class ring, J. Number Theory, 174 (2017), 14–25. https://doi.org/10.1016/j.jnt.2016.10.018 doi: 10.1016/j.jnt.2016.10.018
![]() |