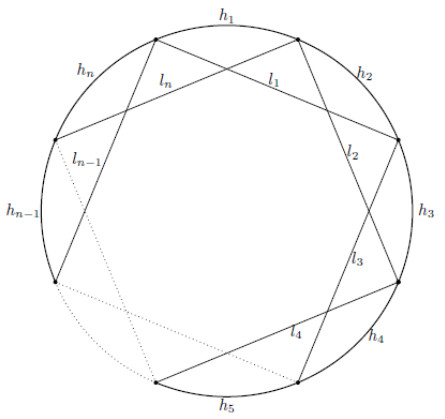
The focus of this article lies on the notion of the edge version of doubly resolving sets (EVDRSs) in circulant networks. EVDRSs refer to unique edge subsets that are necessary for identifying individual edges in a network and distinguishing them based on their edge distances to the elements of the EVDRS. The main objectives were to define the minimal size of EVDRSs for circulant networks Cn(1,2) and to investigate their basic properties. The systematic research helped to achieve a new understanding of the existence, construction, and characterization of EVDRSs in circulant networks Cn(1,2). It is established that the EVDRSs in the circulant network Cn(1,2) are finite and are bounded by the order of the network. Among the numerous implications of these findings are those that refer to the design and optimization of distributed sensor networks, improving communication and network protocols, as well as tracking the spread of infectious diseases and epidemics over social networks. The application of the identified methodology helps improve the process of network optimization which contributes to the development of more effective and robust circulant-based structures.
Citation: Ruby Nasir, Muhammad Ahmad, Zohaib Zahid, Sanaa A. Bajri, Hamiden Abd El-Wahed Khalifa. Characterizing edge-based doubly resolving sets within circulant networks Cn(1,2)[J]. AIMS Mathematics, 2024, 9(6): 15857-15874. doi: 10.3934/math.2024766
[1] | Ahmed Alamer, Hassan Zafar, Muhammad Javaid . Study of modified prism networks via fractional metric dimension. AIMS Mathematics, 2023, 8(5): 10864-10886. doi: 10.3934/math.2023551 |
[2] | Muhammad Ahmad, Muhammad Faheem, Sanaa A. Bajri, Zohaib Zahid, Muhammad Javaid, Hamiden Abd El-Wahed Khalifa . Optimizing SNARK networks via double metric dimension. AIMS Mathematics, 2024, 9(8): 22091-22111. doi: 10.3934/math.20241074 |
[3] | Chenggang Huo, Humera Bashir, Zohaib Zahid, Yu Ming Chu . On the 2-metric resolvability of graphs. AIMS Mathematics, 2020, 5(6): 6609-6619. doi: 10.3934/math.2020425 |
[4] | Ali N. A. Koam . Metric based resolvability of cycle related graphs. AIMS Mathematics, 2024, 9(4): 9911-9925. doi: 10.3934/math.2024485 |
[5] | Maryam Salem Alatawi, Ali Ahmad, Ali N. A. Koam, Sadia Husain, Muhammad Azeem . Computing vertex resolvability of benzenoid tripod structure. AIMS Mathematics, 2022, 7(4): 6971-6983. doi: 10.3934/math.2022387 |
[6] | Ali N. A. Koam, Sikander Ali, Ali Ahmad, Muhammad Azeem, Muhammad Kamran Jamil . Resolving set and exchange property in nanotube. AIMS Mathematics, 2023, 8(9): 20305-20323. doi: 10.3934/math.20231035 |
[7] | Mohamed Basher . On the reflexive edge strength of the circulant graphs. AIMS Mathematics, 2021, 6(9): 9342-9365. doi: 10.3934/math.2021543 |
[8] | Mohra Zayed, Ali Ahmad, Muhammad Faisal Nadeem, Muhammad Azeem . The comparative study of resolving parameters for a family of ladder networks. AIMS Mathematics, 2022, 7(9): 16569-16589. doi: 10.3934/math.2022908 |
[9] | Syed Ahtsham Ul Haq Bokhary, Zill-e-Shams, Abdul Ghaffar, Kottakkaran Sooppy Nisar . On the metric basis in wheels with consecutive missing spokes. AIMS Mathematics, 2020, 5(6): 6221-6232. doi: 10.3934/math.2020400 |
[10] | Ali N. A. Koam, Adnan Khalil, Ali Ahmad, Muhammad Azeem . Cardinality bounds on subsets in the partition resolving set for complex convex polytope-like graph. AIMS Mathematics, 2024, 9(4): 10078-10094. doi: 10.3934/math.2024493 |
The focus of this article lies on the notion of the edge version of doubly resolving sets (EVDRSs) in circulant networks. EVDRSs refer to unique edge subsets that are necessary for identifying individual edges in a network and distinguishing them based on their edge distances to the elements of the EVDRS. The main objectives were to define the minimal size of EVDRSs for circulant networks Cn(1,2) and to investigate their basic properties. The systematic research helped to achieve a new understanding of the existence, construction, and characterization of EVDRSs in circulant networks Cn(1,2). It is established that the EVDRSs in the circulant network Cn(1,2) are finite and are bounded by the order of the network. Among the numerous implications of these findings are those that refer to the design and optimization of distributed sensor networks, improving communication and network protocols, as well as tracking the spread of infectious diseases and epidemics over social networks. The application of the identified methodology helps improve the process of network optimization which contributes to the development of more effective and robust circulant-based structures.
The notion of resolving sets (RSs) in networks has been the subject of a great deal of research over the last few years, mostly because of its practical applications in a variety of domains, including pattern recognition, coding theory, error correction, network design, and distance labelling systems. The RSs are basically subsets of nodes in a network with the capacity to differentiate between each node according to their distances from each other. RSs are often referred to as locating sets and metric bases.
In [1], Slater presents the idea of locating sets, or RSs, first, and he also created the phrase location number to indicate the size of the smallest RS. By following the Slater's work, Melter and Harary expanded on the idea of locating sets and introduced the term metric dimension (MD). They further investigated the MD of trees [2], providing a comprehensive understanding of this idea. The application of the MD problem has gained significant attention in addressing various real-world issues in recent times. Its utilization spans several domains: establishing reliable sensor networks [3], authenticating network intrusions by leveraging the network's metric base, tackling coin weighing challenges [4], and connected joins in networks [5]. Moreover, MD is applied in different branches of navigation [6], and chemistry [7].
Murtaza et al. [8] estimated the constant MD of cycle-related networks. It was also discovered that finding the MD of a connected network is an NP-hard task [9]. Chartrand et al. calculated the MD of both unicyclic and path networks [7]. Furthermore, they provided a characterization of all connected networks of order p that possess MD 1, p−1, and p−2. For calculating the MD of networks, Murdiansyah and Adiwijaya [10] presented the particle swarm optimization technique. Fernau et al. [11] introduced an algorithm with linear-time complexity to calculate the MD of chain networks. These networks are bipartite and have vertices that can be arranged based on neighborhood inclusion. In [12], Mulyono et al. focused on establishing the MD for three types of networks: the friendship network Fn, the Petersen network P(n,m), and the lollipop network L(m,n). Moreover, the MD is thought to be paramount for studying many other structures, such as the RS of silicate stars [13], the exact values of MD and domination-related parameters of complete multipartite networks [14], and the sharp bounds on the MD of honeycomb networks studied in [15].
Determining the fault-tolerant MD of a network poses a complex combinatorial challenge, offering potential implications for sensor networks. In their exploration of the MD and fault-tolerant MD within various interconnection networks [16], Hayat et al. discovered that silicate, Benes, and butterfly networks have infinite fault-tolerant resolvability. Saha et al., in their research outlined in [17], delved into the k-MD of the circulant networks for a range of possible values of n and k, representing the required fault-tolerant MD. Investigations conducted by authors in [18,19] focused on the fault-tolerant MD within recognized families of convex polytope structures. Additionally, Saha et al. tackled the 2-MD problem regarding circulant networks specifically, contributing to the field of network resilience, as discussed in [20]. Raza et al. [21] demonstrate specific lower and upper limits regarding the FTMD for infinite of regular graphs. For more details, see [22].
Caceres et al. [23] have pioneered the exploration of doubly resolving sets (DRSs) within graph theory, showcasing the strong correlation between the MD of the Cartesian products and these minimal DRSs in network G. DRSs are essential in source localization because they help reduce ambiguity, improve accuracy, enhance robustness, and enable efficient algorithms for determining the locations of multiple sources [24,25]. DRSs are a valuable tool in various applications, including radar, sonar, wireless communication, and more, where source localization is a critical task.
In [26], Kratica et al. demonstrated that the minimal DRS problem is NP-hard and proposed the utilization of a genetic algorithm to address large-scale instances of the problem. The problem of determining the MDRSs has been investigated for various network families. Notably, prior research has delved into the investigation of minimal DRSs within prisms [27], convex polytopes [28], and Hamming networks [29]. In the work by Chen et al. [30], they established the upper and lower bounds for the DRS problem. Additionally, in the study by Ahmad et al. [31], it was noted that circulant networks in the same family exhibit identical MD and DRS properties.
Lately, Ahmad et al. have made significant strides in addressing the DRS problem within various network families, as evidenced in their work [32,33]. Jannesari [34] provided a characterization for networks G with a double metric dimension (DMD) of 2, utilizing 2-connected subnetworks within network G. In another recent research endeavor, Ahmad et al. [35] delved into the study of minimal DRSs specifically in the context of chordal ring networks.
There are many different kinds of network metric generators in use right now, and each kind is the subject of theoretical and practical research based on how useful and well-linked it is (for details see: [36]). However, while defining networks utilizing these metric generators, there are other viewpoints that have not yet been fully considered. Assume a network where vertices are accurately monitored and uniquely identified by a metric generator. However, if an intruder enters the network through its edges rather than its vertices, it is impossible to pinpoint their location, which makes surveillance impossible.
To address these kinds of issues, Ahmad et al. introduced a novel metric generator called EVDRSs in [37]. For the edge form of DRSs, only a few network families have been studied in the literature. The EVDRSs for the sunlet networks and prisms were found in [37]. The concept of the minimal EVDRSs has also been examined in [38] concerning the family of layer-sun networks. Moreover, the investigation of minimum-ordered EVDRSs in chorded cycles was carried out in [39], where the authors established that the least size of the EVDRSs for the chorded cycles is precisely one more than its edge version of MD. Additionally, the EVDRS problem for cocktail networks, jellyfish networks, and necklace networks has been examined in previous works, such as [40], and [41], respectively. In another study [42], Ahmad et al. tackled the problem of identifying the minimum EVDRSs for kayak paddle networks.
In this research article, we focus on EVDRSs in circulant networks Cn(1,2). A set is considered an EVDRS in a network if it has the ability to uniquely distinguish between any two edges of the network using any pair of edges from a subset of that network. The study of EVDRSs is relatively new and has gained attention as it provides additional information about the structure of a network. The main objective of this research is to investigate the existence, size, and structure of EVDRSs in circulant networks Cn(1,2). By providing insights into the EVDRSs of circulant networks Cn(1,2), we contribute to the broader understanding of this intriguing class of networks and expand the knowledge base of RSs. The research findings in this study hold implications for real-life situations in various domains; a few of them are as follows:
Communication Networks: The EVDRSs can help improve error detection and correction mechanisms in communication networks, which will increase the dependability of data transfer.
Distributed Sensor Networks: The design and optimization of distributed sensor networks, where effective data collection and processing are essential, may find applications from the insights gained from this study.
Furthermore, these findings may have an impact on the development of network navigation and localization algorithms. The characterization of EVDRSs in circulant networks Cn(1,2) provides the groundwork for the development of location-aware systems that are more reliable and accurate, influencing domains like tracking, surveillance, and autonomous navigation. To summarize, the study of EVDRSs in circulant networks Cn(1,2) has both theoretical and practical implications for enhancing the performance, efficiency, and reliability of different kinds of communication and sensing systems in real-world applications. The key contribution of the article is explained below:
● The Section 2 introduces the notation and preliminary definitions required for our study.
● Section 3 presents our main results on the existence and structure of EVDRSs in Cn(1,2).
● We discuss the implications and applications of these results in Section 4.
● Finally, we conclude the article in Section 5 by summarizing our findings, highlighting their significance and limitations, and suggesting directions for future research.
We take an undirected, connected, and simple network G consisting of the set of vertices V(G) and E(G), which is the set of edges. Let v1,v2 ∈ V(G), then the distance d(v1,v2) is the smallest path between v1 and v2. Let an ordered subset R={ri,1≤i≤l}⊆V(G), and for any v ∈ V(G), the representation of v with respect to R is the l-tuple (d(v,r1),d(v,r2),…,d(v,rl)) and written as r(v,R). The set R is said to be a RS, when different vertices of G have different representations with respect to R. The minimum number of vertices in subset R is called a basis for G, and the cardinality or size of the basis is known as the MD of G, represented by dim(G). For R⊂V(G), r(x,R) = 0, whenever x=ri, the ith component of R. Therefore, to prove that R is resolvable, we have to demonstrate that r(x,R)≠r(y,R), whenever x≠y ∈ V(G) ∖ R. Let w and x be any two vertices of G, where |V(G)|≥2. If for the vertices u,v∈G, d(u,w)−d(u,x)≠d(v,w)−d(v,x), i.e., the difference between their distances to w and x is not equal, then we say that u and v are doubly resolved by w and x. A subset D is said to be DRS, if some of its vertices doubly resolve every pair of the vertices in G. A DRS with the smallest possible size is said to be a minimal DRS, and its size is called as the double metric dimension (DMD), denoted by the ψ(G).
If e1 and e2 are edges of the network G, with the condition that |E(G)|≥2 and dE(f1,e1)−dE(f1,e2)≠dE(f2,e1)−dE(f2,e2), then f1 and f2 are said to be doubly resolved by e1 and e2. If an ordered subset DG={et,1≤t≤k} of edges in G doubly resolves any pair of edges f1 and f2 in E(G), then such a subset DG is called an EVDRS of G. The minimum size of an EVDRS of G is denoted as ψE(G). For more research on these basic concepts, see [37].
The family of circulant network, denoted by Cn(1,2) is the family of networks obtained by constructing a cycle of length n and connecting each vertex vλ with vλ+2 modulo n with a vertex set VCn(1,2)={v1,v2,…,vn} and edge set ECn(1,2)=H∪L, as shown in Figure 1, where H={h1,h2,…,hn} and L={l1,l2,…,ln}.
The edge version of MD for Cn(1,2) was studied in [43], and the result is given below:
Theorem 3.1. [43] Let Cn(1,2) be the circulant networks, then dimE(Cn(1,2))=4, ∀ n≥6.
The following proposition will be helpful in calculating the EVDRSs:
Proposition 3.1. [26] A subset DCn(1,2)={y1,y2,…yk}⊆E(Cn(1,2)) is an EVDRS of Cn(1,2) if, and only if, for every v,y∈E(Cn(1,2)), there exists λ∈{2,3,…,k} such that dE(v,yλ)−dE(v,y1) is not equal to dE(y,yλ)−dE(y,y1).
For every edge e∈E(Cn(1,2)), we define r′E(e,DCn(1,2))=(dE(e,y2)−dE(e,y1),…,dE(e,yk)−dE(e,y1)). The above proposition states that DCn(1,2) is a minimal EVDRS if and only if these distance vectors r′E(e,DCn(1,2)) are all distinct for every edge e∈E(Cn(1,2)).
The statement of the theorem for EVDRSs in circulant networks is as follows:
Theorem 3.2. Let Cn(1,2) be the circulant networks, then ψE(Cn(1,2))=4, for n≥6.
Proof. To compute EVDRSs for circulant networks, we will address the following four scenarios:
Case 1. When n ≡ 0(mod 4) or n=4k, where k≥2.
Consider the set of edges RCn(1,2)={h1,h2,hn2−1,hn2}, then the distance vectors of the edges in relation to RCn(1,2) are shown in Tables 1 and 2 as follows:
rE(h2λ−1,RCn(1,2)) | λ |
(0,1,n4,n4) | if λ=1; |
(λ,λ−1,n4−λ+1,n4−λ+1) | if 2≤λ≤n4−1; |
(n4,n4−1,0,1) | if λ=n4; |
(λ,n4,2,1) | if λ=n4+1; |
(n2−λ+2,n2−λ+2,λ−n4+1,λ−n4) | if n4+2≤λ≤n2. |
r′E(h2λ−1,RCn(1,2)) | λ |
(1,n4,n4) | if λ=1; |
(−1,n4−2λ+1,n4−2λ+1) | if 2≤λ≤n4−1; |
(−1,−n4,1−n4) | if λ=n4; |
(n4−λ,2−λ,1−λ) | if λ=n4+1; |
(0,2λ−3n4−1,2λ−3n4−2) | if n4+2≤λ≤n2. |
rE(h2λ,RCn(1,2)) | λ |
(1,0,n4−1,n4) | if λ=1; |
(λ,λ,n4−λ,n4−λ+1) | if 2≤λ≤n4−1; |
(n4,n4,1,0) | if λ=n4; |
(n2−λ+1,n2−λ+2,λ−n4+1,λ−n4+1) | if n4+1≤λ≤n2. |
r′E(h2λ,RCn(1,2)) | λ |
(−1,n4−2,n4−1) | if λ=1; |
(0,n4−2λ,n4−2λ+1) | if 2≤λ≤n4−1; |
(0,1−n4,−n4) | if λ=n4; |
(1,2λ−3n4,2λ−3n4) | if n4+1≤λ≤n2. |
rE(l2λ−1,RCn(1,2)) | λ |
(1,1,n4−1,n4) | if λ=1; |
(λ,λ−1,n4−λ,n4−λ+1) | if 2≤λ≤n4−1; |
(n4,λ−n4+4,λ−n4+1,1) | if n4≤λ≤n4+1; |
(n2−λ+1,n2−λ+2,λ−n4+1,λ−n4) | if n4+2≤λ≤n2−1; |
(1,2,n4,n4) | if λ=n2. |
r′E(l2λ−1,RCn(1,2)) | λ |
(0,n4−2,n4−1) | if λ=1; |
(−1,n4−2λ,n4−2λ+1) | if 2≤λ≤n4−1; |
(λ−n2+4,λ−n2+1,1−n4) | if n4≤λ≤n4+1; |
(1,2λ−3n4,2λ−3n4−1) | if n4+2≤λ≤n2−1; |
(1,n4−1,n4−1) | if λ=n2. |
rE(l2λ,RCn(1,2)) | λ |
(λ,λ,n4−λ,n4−λ) | if 1≤λ≤n4−1; |
(n4,n4,λ−n4+1,λ−n4+1) | if n4≤λ≤n4+1; |
(n2−λ+1,n2−λ+1,λ−n4+1,λ−n4+1) | if n4+2≤λ≤n2−1; |
(1,1,n4,n4) | if λ=n2. |
r′E(l2λ,RCn(1,2)) | λ |
(0,n4−2λ,n4−2λ) | if 1≤λ≤n4−1; |
(0,λ−n2+1,λ−n2+1) | if n4≤λ≤n4+1; |
(0,2λ−3n4,2λ−3n4) | if n4+2≤λ≤n2−1; |
(0,n4−1,n4−1) | if λ=n2. |
Case 2. When n ≡ 1(mod 4) or n=4k+1, where k≥2.
Consider the set of edges RCn(1,2)={h1,h⌊n2⌋,h⌊n2⌋+1,hn}, then the distance vectors of the edges in relation to RCn(1,2) are shown in Tables 3 and 4 as follows:
rE(h2λ−1,RCn(1,2)) | λ |
(0,n−14,n−14+1,1) | if λ=1; |
(λ,n−14−λ+1,n−14−λ+2,λ) | if 2≤λ≤n−14; |
(n−14+1,1,0,n−14+1) | if λ=n−14+1; |
(n−12−λ+2,λ−n−14,λ−n−14,n−12−λ+2) | if n−14+2≤λ≤n−12; |
(1,n−14+1,n−14+1,0) | if λ=n−12+1. |
r′E(h2λ−1,RCn(1,2)) | λ |
(n−14,n−14+1,1) | if λ=1; |
(n−14−2λ+1,n−14−2λ+2,0) | if 2≤λ≤n−14; |
(−n−14,−n−14−1,0) | if λ=n−14+1; |
(2λ−3n−34−2,2λ−3n−34−2,0) | if n−14+2≤λ≤n−12; |
(n−14,n−14,−1) | if λ=n−12+1. |
rE(h2λ,RCn(1,2)) | λ |
(λ,n−14−λ+1,n−14−λ+1,λ+1) | if 1≤λ≤n−14−1; |
(n−14,0,1,n−14+1) | if λ=n−14; |
(n−12−λ+2,λ−n−14+1,λ−n−14,n−12−λ+1) | if n−14+1≤λ≤n−12. |
r′E(h2λ,RCn(1,2)) | λ |
(n−14−2λ+1,n−14−2λ+1,1) | if 1≤λ≤n−14−1; |
(−n−14,1−n−14,1) | if λ=n−14; |
(2λ−3n−34−1,2λ−3n−34−2,−1) | if n−14+1≤λ≤n−12. |
rE(l2λ−1,RCn(1,2)) | λ |
(λ,n−14−λ+1,n−14−λ+1,λ) | if 1≤λ≤n−14; |
(n−12−λ+2,λ−n−14,λ−n−14,n−12−λ+1) | if n−14+1≤λ≤n−12; |
(1,n−14,n−14+1,1) | if λ=n−12+1. |
r′E(l2λ−1,RCn(1,2)) | λ |
(n−14−2λ+1,n−14−2λ+1,0) | if 1≤λ≤n−14; |
(2λ−3k−2,2λ−3k−2,−1) | if k+1≤λ≤2k; |
(n−14−1,n−14,0) | if λ=n−12+1. |
rE(l2λ,RCn(1,2)) | λ |
(λ,n−14−λ,n−14−λ+1,λ+1) | if 1≤λ≤n−14−1; |
(n−14,1,1,n−14+1) | if λ=n−14; |
(n−12−λ+1,λ−n−14+1,λ−n−14,n−12−λ+1) | if n−14+1≤λ≤n−12. |
r′E(l2λ,RCn(1,2)) | λ |
(n−14−2λ,n−14−2λ+1,1) | if 1≤λ≤n−14−1; |
(1−n−14,1−n−14,1) | if λ=n−14; |
(2λ−3n−34,2λ−3n−34−1,0) | if n−14+1≤λ≤n−12. |
Case 3. When n ≡ 2(mod 4) or n=4k+2, where k≥1.
Consider the set of edges RCn(1,2)={h1,hn2−1,hn2,hn}, then the distance vectors of the edges in relation to RCn(1,2) are shown in Tables 5 and 6 as follows:
rE(h2λ−1,RCn(1,2)) | λ |
(0,n−24,n−24+1,1) | if λ=1; |
(λ,n−24−λ+1,n−24−λ+2,λ) | if 2≤λ≤n−24; |
(n−24+1,1,0,n−24+1) | if λ=n−24+1; |
(n−22−λ+3,λ−n−24,λ−n−24,n−22−λ+2) | if n−24+2≤λ≤n−22+1. |
r′E(h2λ−1,RCn(1,2)) | λ |
(n−24,n−24+1,1) | if λ=1; |
(n−24−2i+1,n−24−2λ+2,0) | if 2≤λ≤n−24; |
(−n−24,−n−24−1,0) | if λ=n−24+1; |
(2λ−3n−64−3,2λ−3n−64−3,−1) | if n−24+2≤λ≤n−22+1. |
rE(h2λ,RCn(1,2)) | λ |
(λ,n−24−λ+1,n−24−λ+1,λ+1) | if 1≤λ≤n−24−1; |
(n−24,0,1,n−24+1) | if λ=n−24; |
(n−22−λ+2,λ−n−24+1,λ−n−24,n−22−λ+2) | if n−24+1≤λ≤n−22; |
(1,n−24+1,n−24+1,0) | if λ=n−22+1. |
r′E(h2λ,RCn(1,2)) | λ |
(n−24−2λ+1,n−24−2λ+1,1) | if 1≤λ≤n−24−1; |
(−n−24,1−n−24,1) | if λ=n−24; |
(2λ−3n−64−1,2λ−3n−64−2,0) | if n−24+1≤λ≤n−22; |
(n−24,n−24,−1) | if λ=n−22+1. |
rE(l2λ−1,RCn(1,2)) | λ |
(λ,n−24−λ+1,n−24−λ+1,λ) | if 1≤λ≤n−24; |
(n−22−λ+2,λ−n−24,λ−n−24,n−22−λ+2) | if n−24+1≤λ≤n−22+1. |
r′E(l2λ−1,RCn(1,2)) | λ |
(n−24−2λ+1,n−24−2λ+1,0) | if 1≤λ≤n−24; |
(2λ−3n−64−2,2λ−3n−64−2,0) | if n−24+1≤λ≤n−22+1. |
rE(l2λ,RCn(1,2)) | λ |
(λ,n−24−λ,n−24−λ+1,λ+1) | if 1≤λ≤n−24−1; |
(n−24,1,1,n−24+1) | if λ=n−24; |
(n−22−λ+2,λ−n−24+1,−n−24,n−22−λ+1) | if n−24+1≤λ≤n−22; |
(1,n−24,n−24+1,1) | if λ=n−22+1. |
r′E(l2λ,RCn(1,2)) | λ |
(n−24−2λ,n−24−2λ+1,1) | if 1≤λ≤n−24−1; |
(1−n−24,1−n−24,1) | if λ=n−24; |
(2λ−3n−64−1,2λ−3n−64−2,−1) | if n−24+1≤λ≤n−22; |
(n−24−1,n−24,0) | if λ=n−22+1. |
Case 4. When n ≡ 3(mod 4) or n=4k+3, where k≥1.
Consider the set of edges RCn(1,2)={h1,hn−2,l⌊n2⌋−1,l⌊n2⌋}, then the distance vectors of the edges in relation to RCn(1,2) are shown in Tables 7 and 8 as follows:
rE(h2λ−1,RCn(1,2)) | λ |
(0,2,n−34,n−34+1) | if λ=1; |
(λ,λ+1,n−34−λ+1,n−34−λ+2) | if 2≤λ≤n−34; |
(n−34+1,n−34+1,1,1) | if λ=n−34+1; |
(n−32−λ+3,n−32−λ+2,λ−n−34,n−34−λ−1) | if n−34+2≤λ≤n−32; |
(2,0,n−34+1,n−34) | if λ=n−32+1; |
(1,2,n−34+1,n−34+1) | if λ=⌊n−32+2⌋. |
r′E(h2λ−1,RCn(1,2)) | λ |
(2,n−34,n−34+1) | if λ=1; |
(1,n−34−2λ+1,n−34−2λ+2) | if 2≤λ≤n−34; |
(0,−n−34,−n−34) | if λ=n−34+1; |
(−1,2λ−3n−94−3,−n−34−4) | if n−34+2≤λ≤n−32; |
(−2,n−34−1,n−34−2) | if λ=n−32+1; |
(1,n−34,n−34) | if λ=⌊n−32+2⌋. |
rE(h2λ,RCn(1,2)) | λ |
(λ,λ+2,n−34−λ+1,n−34−λ+1) | if 1≤λ≤n−34−1; |
(λ,n−32−λ+1,1,1) | if n−34≤λ≤n−34+1; |
(n−32−λ+3,n−32−λ+1,λ−n−34,λ−n−34) | if n−34+2≤λ≤n−32; |
(2,1,n−34+1,n−34+1) | if λ=n−32+1. |
r′E(h2λ,RCn(1,2)) | λ |
(2,n−34−2λ+1,n−34−2λ+1) | if 1≤λ≤n−34−1; |
(n−32−2λ+1,1−λ,1−λ) | if n−34≤λ≤n−34+1; |
(−2,2λ−3n−94−3,2λ−3n−94−3) | if n−34+2≤λ≤n−32; |
(−1,n−34+1,n−34+1) | if λ=n−32+1. |
rE(l2λ−1,RCn(1,2)) | λ |
(λ,λ+1,n−34−λ+1,n−34−λ+1) | if 1≤λ≤n−34−1; |
(λ,n−32−λ+1,2,n−34−λ+1) | if n−34≤λ≤n−34+1; |
(n−32−λ+3,n−32−+1,λ−n−34,λ−n−34−1) | if n−34+2≤λ≤n−32; |
(−n−32+1,λ−n−32,3n−94−λ+2,−n−34−1) | if n−32+1≤λ≤⌊n−34+2⌋. |
r′E(l2λ−1,RCn(1,2)) | λ |
(1,n−34−2λ+1,n−34−2λ+1) | if 1≤λ≤n−34−1; |
(n−32−2λ+1,2−λ,n−34−2λ+1) | if n−34≤λ≤n−34+1; |
(−2,2λ−3n−94−3,2λ−3n−94−4) | if n−34+2≤λ≤n−32; |
(−1,5n−154−2λ−1,n−34−2) | if n−32+1≤λ≤⌊n+12⌋. |
rE(l2λ,RCn(1,2)) | λ |
(λ,λ+2,n−34−λ,n−34−λ+1) | if 1≤λ≤n−34−1; |
(λ,n−32−λ+1,λ−n−34,2) | if n−34≤λ≤n−34+1; |
(n−32−λ+2,n−32−λ+1,λ−n−34,λ−n−34) | if n−34+2≤λ≤n−32; |
(1,1,n−34+1,n−34+1) | if λ=n−32+1. |
r′E(l2λ,RCn(1,2)) | λ |
(2,n−34−2λ,n−34−2λ+1) | if 1≤λ≤n−34−1; |
(n−32−2λ+1,−n−34,2−λ) | if n−34≤λ≤n−34+1; |
(−1,2λ−3n−94−2,2λ−3n−94−2) | if n−34+2≤λ≤n−32; |
(0,n−34,n−34) | if λ=n−32+1. |
The distance vectors in the above four cases show that each distance vector r′E is unique, resulting in ψE(Cn(1,2)) = 4.
EVDRSs is a mathematical tool within the field of graph theory. They serve as a tool for locating particular edges inside a graph (network) by recognizing a collection of edges that are capable of precisely locating a different edge or set of related edges. In practical scenarios, this variant of graph theory finds numerous applications, including fault detection in various network types such as computer networks and network security systems, as well as tracing the origins of epidemics within social networks and contagious diseases, among other uses (for further information, see [44]).
An EVDRS essentially represents a set of measurements or tests that can be helpful in source localization to detect the origin of a viral disease outbreak or a virus source in complex networks. The term "doubly resolving" is used because it possesses the capability to not only identify the existence of contamination but also differentiate between various source categories. Let us explore the following example to better understand how an EVDRS might be used to identify the origins of epidemics during contagious disease outbreaks.
In a viral disease outbreak scenario, where the city is represented as a circulant network C8(1,2) with edges {h1,h2,h3,h4,h5,h6,h7,h8,l1,l2,l3,l4,l5,l6,l7,l8} as shown in Figure 2, we can employ the minimal EVDRSs to effectively track and control the spread of the virus.
Let us consider the following situation:
● The edges h1,h2,h3,h4,h5, and h6 represent residential areas.
● The edges l1,l2,l3,l4,l5, and l6 represent commercial areas.
● The edges h7,h8,l7, and l8 represent medical facilities.
A quick solution can be achieved by carefully placing observers across the city and ensuring exact and well-documented distances between these edges. However, executing this comprehensive procedure would incur substantial expenses and consume a significant amount of time. As a result, the question arises: how many observers must be deployed to trace the origin of infection when the initial time of commencement is unknown and transmission delays across edges vary? This demands a system in which each edge is distinguished by its minimal distance from strategically placed observers.
For this purpose, we need to identify a minimal set of edges (observers) that, when infection spreads, would uniquely determine the source of the infection and help in containing the spread. In the case where a city is represented by the circulant network C8(1,2), we can choose the following minimal EVDRS: RC8(1,2)={h1,h2h3,h4}.
Let us examine how this works:
Residential Areas: If anyone from the residential areas gets infected, then the source of infection would be uniquely identified from these residential areas using the information collected by the placed observers. This information can help in implementing targeted measures like quarantine, testing, and contact tracing within the residential areas.
Commercial Areas: If an infection spreads in any one of these commercial areas, similarly, by employing the collected data by the placed observer, the source of the infection would point to one of these commercial areas. This knowledge can be used to implement measures like temporary closure, sanitation, and testing within commercial areas.
Medical Facilities: If the infection has reached one of the medical facilities, then by finding the unknown starting time and edge-to-edge transmission delays using EVDRSs, the source of infection would be uniquely identified. This information is crucial for implementing measures to protect healthcare workers and patients and prevent further transmission within medical facilities.
By means of the careful monitoring and tracking of infection via these basic EVDRSs, health authorities and decision-makers can efficiently allocate resources, identify interventions, and make informed decisions to control the viral disease outbreak in the city.
There are numerous practical uses for circulant networks, particularly in distributed computing and computer networks. Particularly, circulant networks can be used for task assignments, load balancing, and data spreading in distributed computing systems. Circulant networks are beneficial for efficient routing and resource allocation due to their regular nature. Also, circulant networks offer an effective way to organize the communication architecture in sensor networks, where multiple sensors send data to a central node (or edge) or among one another.
Moreover, these networks can be utilized in wireless mesh networks, which expand network coverage by using wireless communication among nodes, or edges. Because of their regular structure, circulant networks are suitable for distributing computational workloads among multiple nodes (or edges) in an efficient manner. Moreover, they can be applied in social areas, in which coordination and a communication mechanism between distant units are needed. For instance, a social network or Internet of Things system.
The present paper has tackled the notion of EVDRSs in circulant networks Cn(1,2), EVDRSs being a set of edges in a network that ascertains that each edge has a unique representation in relation to the elements of an EVDRS. The main goals were to characterize the minimal size of EVDRS for circulant networks Cn(1,2) as well as to understand their structural properties. To sum up, the present study has focused on EVDRSs in circulant networks Cn(1,2) and has made significant advances in comprehending their structural features, determining their minimal size, and highlighting their independence from the parity of n. The results of the above investigations extend the understanding of graph theory and have practical implications in several fields, such as telecommunications and distributed computing, which rely on well-built and efficient networks. Our research findings are important for helping to create more robust and efficient networks, as well as for developing the theory of graph-based communication systems.
Because the obtained results are specific to circulant networks Cn(1,2), they may not hold true for other network topologies. It could be difficult to convert theoretical results into practical implementations, especially in complex and dynamic networks. Collaborating with specialists in related fields such as network engineering, epidemiology, and computer science may be necessary for fully comprehending the potential impact of the research in several domains. In fact, bridging the gap between theoretical research and practical applications can be greatly aided by disciplinary collaboration. Interdisciplinary collaboration can solve the shortcomings of specific disciplines by combining ideas and techniques from other domains, producing more trustworthy and useful research results. This could then lead to a greater impact from these discoveries in a variety of domains, eventually resulting in useful answers and breakthroughs in various disciplines of research.
Open Problem 5.1. Investigate the EVDRSs for the Chordal Ring network CRn(1,3,5), ∀ even integers n≥6.
Ruby Nasir and Muhammad Ahmad conducted the final review and made sure the results were validated at the problem discussion. Zohaib Zahid was helpful in initiating the problem, obtaining necessary information, and offering continuous supervision. Initiating the study's drafting process and contributing significantly to its analysis and computation was Sanaa A. Bajri. In addition to contributing to the methodology, Hamiden Abd El-Wahed Khalifa actively discussed the issue and carefully proofread the final draft.
The authors declare they have not used artificial intelligence (AI) tools in the creation of this article.
The Research is funded by Princess Nourah bint Abdulrahman University Researchers Supporting Project number (PNURSP2024R527), Princess Nourah bint Abdulrahman University, Riyadh, Saudi Arabia
The authors declared no potential conflicts of interest with respect to the research, authorship, and/or publication of this article.
[1] | P. J. Slater, Leaves of trees, Congr. Numer., 14 (1975), 549–559. |
[2] | F. Harary, R. A. Melter, On the metric dimension of a graph, Ars Combin., 2 (1976), 191–195. |
[3] |
M. Idrees, H. Ma, M. Wu, A. R. Nizami, M. Munir, S. Ali, Metric dimension of generalized Mobius ladder and its application to wsn localization, J. Adv. Comput. Intell., 24 (2020), 3–11. https://doi.org/10.20965/jaciii.2020.p0003 doi: 10.20965/jaciii.2020.p0003
![]() |
[4] |
S. Soderberg, H. S. Shapiro, A combinatory detection problem, Am. Math. Mon., 70 (1963), 1066–1070. https://doi.org/10.1080/00029890.1963.11992174 doi: 10.1080/00029890.1963.11992174
![]() |
[5] |
A. Sebo, E. Tannier, On metric generators of graphs, Math. Oper. Res., 29 (2004), 191–406. https://doi.org/10.1287/moor.1030.0070 doi: 10.1287/moor.1030.0070
![]() |
[6] |
S. Khuller, B. Raghavachari, A. Rosenfeld, Landmarks in graphs, Discrete Appl. Math., 70 (1996), 217–229. https://doi.org/10.1016/0166-218X(95)00106-2 doi: 10.1016/0166-218X(95)00106-2
![]() |
[7] |
G. Chartrand, L. Eroh, M. A. Johnson, O. R. Oellermann, Resolvability in graphs and the metric dimension of a graph, Discrete Appl. Math., 105 (2000), 99–113. https://doi.org/10.1016/S0166-218X(00)00198-0 doi: 10.1016/S0166-218X(00)00198-0
![]() |
[8] |
M. Ali, G. Ali, U. Ali, M. T. Rahim, On cycle related graphs with constant metric dimension, Open J. Discrete Math., 2 (2012), 21–23. https://doi.org/10.4236/ojdm.2012.21005 doi: 10.4236/ojdm.2012.21005
![]() |
[9] | M. R. Garey, D. S. Johnson, Computers and intractability: A guide to the theory of NP-completeness, 1990, 37–79. |
[10] | D. T. Murdiansyah, Adiwijaya, Computing the metric dimension of hypercube graphs by particle swarm optimization algorithms, In: Recent advances on soft computing and data mining: The second international conference on soft computing and data mining (SCDM-2016), Cham: Springer, 2016. https://doi.org/10.1007/978-3-319-51281-5_18 |
[11] |
H. Fernau, P. Heggernes, P. Van't Hof, D. Meistera, R. Saei, Computing the metric dimension for chain graphs, Inform. Process. Lett., 115 (2015), 671–676. https://doi.org/10.1016/j.ipl.2015.04.006 doi: 10.1016/j.ipl.2015.04.006
![]() |
[12] | M. Mulyono, W. Wulandari, The metric dimension of friendship graph Fn, lollipop graph L(m,n) and petersen graph P(n,m), Bull. Math., 8 (2016), 117–124. |
[13] | F. S. Raj, A. George, On the metric dimension of silicate stars, ARPN J. Eng. Appl. Sci., 10 (2015), 2187–2192. |
[14] |
S. Hayat, A. Khan, Y. Zhong, On resolvability- and domination-related parameters of complete multipartite graphs, Mathematics, 10 (2022), 1815. https://doi.org/10.3390/math10111815 doi: 10.3390/math10111815
![]() |
[15] |
P. Manuel, B. Rajan, I. Rajasingh, C. Monica, On minimum metric dimension of honeycomb networks, J. Discret. Algorit., 6 (2008), 20–27. https://doi.org/10.1016/j.jda.2006.09.002 doi: 10.1016/j.jda.2006.09.002
![]() |
[16] |
S. Hayat, A. Khan, M. Y. H. Malik, M. Imran, M. K. Siddiqui, Fault-tolerant metric dimension of interconnection networks, IEEE Access, 8 (2020), 145435–145445. https://doi.org/10.1109/ACCESS.2020.3014883 doi: 10.1109/ACCESS.2020.3014883
![]() |
[17] |
L. Saha, K. Tiwary, K. C. Das, Y. Shang, Optimal multi-level fault-tolerant resolving sets of circulant graph Cn(1,2), Mathematics, 11 (2023), 1896. https://doi.org/10.3390/math11081896 doi: 10.3390/math11081896
![]() |
[18] |
H. Raza, S. Hayat, X. F. Pan, On the fault-tolerant metric dimension of convex polytopes, Appl. Math. Comput., 339 (2018), 172–185. https://doi.org/10.1016/j.amc.2018.07.010 doi: 10.1016/j.amc.2018.07.010
![]() |
[19] |
H. M. A. Siddiqui, S. Hayat, A. Khan, M. Imran, A. Razzaq, J. B. Liu, Resolvability and fault-tolerant resolvability structures of convex polytopes, Theor. Comput. Sci., 796 (2019), 114–128. https://doi.org/10.1016/j.tcs.2019.08.032 doi: 10.1016/j.tcs.2019.08.032
![]() |
[20] |
L. Saha, R. Lama, K. Tiwary, K. C. Das, Y. Shang, Fault-tolerant metric dimension of circulant graphs, Mathematics, 10 (2022), 124. https://doi.org/10.3390/math10010124 doi: 10.3390/math10010124
![]() |
[21] |
H. Raza, S. Hayat, M. Imran, X. F. Pan, Fault-tolerant resolvability and extremal structures of graphs, Mathematics, 7 (2019), 78. https://doi.org/10.3390/math7010078 doi: 10.3390/math7010078
![]() |
[22] |
L. Saha, M. Basak, K. Tiwary, K. C. Das, Y. Shang, On the characterization of a minimal resolving set for power of paths, Mathematics, 10 (2022), 2445. https://doi.org/10.3390/math10142445 doi: 10.3390/math10142445
![]() |
[23] |
J. Caceres, C. Hernado, M. Mora, I. M. Pelayo, M. L. Puertas, C. Seara, et al., On the metric dimension of cartesian products of graphs, SIAM J. Discrete Math., 21 (2007), 423–441. https://doi.org/10.1137/050641867 doi: 10.1137/050641867
![]() |
[24] | B. Spinelli, L. E. Celis, P. Thiran, Observer placement for source localization: The effect of budgets and transmission variance, In: 2016 54th Annual Allerton Conference on Communication, Control, and Computing (Allerton), 2016,743–751. https://doi.org/10.1109/ALLERTON.2016.7852307 |
[25] | B. Spinelli, L. E. Celis, P. Thiran, How many sensors to localize the source? The double metric dimension of random networks, In: 2018 56th Annual Allerton Conference on Communication, Control, and Computing (Allerton), 2018, 1036–1043. https://doi.org/10.1109/ALLERTON.2018.8635897 |
[26] |
J. Kratica, M. Cangalovic, V. Kovacevic-Vujcic, Computing minimal doubly resolving sets of graphs, Comput. Oper. Res., 36 (2009), 2149–2159. https://doi.org/10.1016/j.cor.2008.08.002 doi: 10.1016/j.cor.2008.08.002
![]() |
[27] |
M. Cangalovic, J. Kratica, V. Kovacevic-Vujcic, M. Stojanovic, Minimal doubly resolving sets of prism graphs, Optimization, 62 (2013), 1037–1043. https://doi.org/10.1080/02331934.2013.772999 doi: 10.1080/02331934.2013.772999
![]() |
[28] |
J. Kratica, V. Kovacevic-Vujcic, M. Cangalovic, M. Stojanovic, Minimal doubly resolving sets and the strong metric dimension of some convex polytope, Appl. Math. Comput., 218 (2012), 9790–9801. https://doi.org/10.1016/j.amc.2012.03.047 doi: 10.1016/j.amc.2012.03.047
![]() |
[29] |
J. Kratica, V. Kovacevic-Vujcic, M. Cangalovic, M. Stojanovic, Minimal doubly resolving sets and the strong metric dimension of Hamming graphs, Appl. Anal. Discrete Math., 6 (2012), 63–71. https://doi.org/10.2298/AADM111116023K doi: 10.2298/AADM111116023K
![]() |
[30] |
X. Chen, X. Hu, C. Wang, Approximation for the minimum cost doubly resolving set problem, Theor. Comput. Sci., 609 (2016), 526–543. https://doi.org/10.1016/j.tcs.2015.03.048 doi: 10.1016/j.tcs.2015.03.048
![]() |
[31] |
A. Ahmad, S. Sultan, On minimal doubly resolving sets of circulant graphs, Acta Mech. Slovaca, 21 (2017), 6–11. https://doi.org/10.21496/ams.2017.002 doi: 10.21496/ams.2017.002
![]() |
[32] |
M. Ahmad, Z. Zahid, M. Javaid, M. A. Ashebo, A study on minimal doubly resolving sets of certain families of networks, IEEE Access, 11 (2023), 56344–56352. https://doi.org/10.1109/ACCESS.2023.3282701 doi: 10.1109/ACCESS.2023.3282701
![]() |
[33] | A. Ahmad, M. Baca, S. Sultan, Minimal doubly resolving sets of necklace graph, Math. Rep., 20 (2018), 123–129. |
[34] |
M. Jannesari, Graphs with doubly resolving number 2, Discrete Appl. Math., 339 (2023), 178–183. https://doi.org/10.1016/j.dam.2023.06.017 doi: 10.1016/j.dam.2023.06.017
![]() |
[35] |
M. Ahmad, Z. Zahid, M. Javaid, E. Bonyah, Studies of chordal ring networks via double metric dimensions, Math. Probl. Eng., 2022 (2022), 8303242. https://doi.org/10.1155/2022/8303242 doi: 10.1155/2022/8303242
![]() |
[36] |
H. Raza, S. Hayat, X. F. Pan, On the fault-tolerant metric dimension of certain interconnection networks, J. Appl. Math. Comput., 60 (2019), 517–535. https://doi.org/10.1007/s12190-018-01225-y doi: 10.1007/s12190-018-01225-y
![]() |
[37] |
M. Ahmad, Z. Zahid, S. Zafar, On minimal edge version of doubly resolving sets of a graph, J. Comb. Math. Comb. Comput., 119 (2024), 175–184. https://doi.org/10.61091/jcmcc119-18 doi: 10.61091/jcmcc119-18
![]() |
[38] |
J. B. Liu, A. Zafari, Computing minimal doubly resolving sets and the strong metric dimension of the layer sun graph and the line graph of the layer sun graph, Complexity, 2020 (2020), 6267072. https://doi.org/10.1155/2020/6267072 doi: 10.1155/2020/6267072
![]() |
[39] |
M. Ahmad, Z. Zahid, T. Rashid, J. L. G. Guirao, Computing edge version of resolvability and double resolvability of a graph, J. Chem., 2022 (2022), 2448032. https://doi.org/10.1155/2022/2448032 doi: 10.1155/2022/2448032
![]() |
[40] |
J. B. Liu, A. Zafari, H. Zarei, Metric dimension, minimal doubly resolving sets, and the strong metric dimension for jellyfish graph and cocktail party graph, Complexity, 2020 (2020), 9407456. https://doi.org/10.1155/2020/9407456 doi: 10.1155/2020/9407456
![]() |
[41] |
J. B. Liu, Z. Zahid, R. Nasir, W. Nazeer, Edge version of metric dimension and doubly resolving sets of the necklace graphs, Mathematics, 6 (2018), 243. https://doi.org/10.3390/math6110243 doi: 10.3390/math6110243
![]() |
[42] |
M. Ahmad, N. Ameen, Z. Zahid, S. Zafar, Computing edge version of metric and double metric dimensions of Kayak paddle graphs, Discret. Math. Algorit., 12 (2020), 2050070. https://doi.org/10.1142/S1793830920500706 doi: 10.1142/S1793830920500706
![]() |
[43] | J. Lv, X. Lv, R. Nasir, Z. Zahid, The edge version of metric dimension for the family of circulant graphs Cn(1,2), in IEEE Access, 9 (2021), 78165–78173. https://doi.org/10.1109/ACCESS.2021.3083182 |
[44] |
B. Spinelli, L. Brunella, E. Celis, P. Thiran, The effect of transmission variance on observer placement for source-localization, Appl. Netw. Sci., 2 (2017), 20. https://doi.org/10.1007/s41109-017-0040-5 doi: 10.1007/s41109-017-0040-5
![]() |
rE(h2λ−1,RCn(1,2)) | λ |
(0,1,n4,n4) | if λ=1; |
(λ,λ−1,n4−λ+1,n4−λ+1) | if 2≤λ≤n4−1; |
(n4,n4−1,0,1) | if λ=n4; |
(λ,n4,2,1) | if λ=n4+1; |
(n2−λ+2,n2−λ+2,λ−n4+1,λ−n4) | if n4+2≤λ≤n2. |
r′E(h2λ−1,RCn(1,2)) | λ |
(1,n4,n4) | if λ=1; |
(−1,n4−2λ+1,n4−2λ+1) | if 2≤λ≤n4−1; |
(−1,−n4,1−n4) | if λ=n4; |
(n4−λ,2−λ,1−λ) | if λ=n4+1; |
(0,2λ−3n4−1,2λ−3n4−2) | if n4+2≤λ≤n2. |
rE(h2λ,RCn(1,2)) | λ |
(1,0,n4−1,n4) | if λ=1; |
(λ,λ,n4−λ,n4−λ+1) | if 2≤λ≤n4−1; |
(n4,n4,1,0) | if λ=n4; |
(n2−λ+1,n2−λ+2,λ−n4+1,λ−n4+1) | if n4+1≤λ≤n2. |
r′E(h2λ,RCn(1,2)) | λ |
(−1,n4−2,n4−1) | if λ=1; |
(0,n4−2λ,n4−2λ+1) | if 2≤λ≤n4−1; |
(0,1−n4,−n4) | if λ=n4; |
(1,2λ−3n4,2λ−3n4) | if n4+1≤λ≤n2. |
rE(l2λ−1,RCn(1,2)) | λ |
(1,1,n4−1,n4) | if λ=1; |
(λ,λ−1,n4−λ,n4−λ+1) | if 2≤λ≤n4−1; |
(n4,λ−n4+4,λ−n4+1,1) | if n4≤λ≤n4+1; |
(n2−λ+1,n2−λ+2,λ−n4+1,λ−n4) | if n4+2≤λ≤n2−1; |
(1,2,n4,n4) | if λ=n2. |
r′E(l2λ−1,RCn(1,2)) | λ |
(0,n4−2,n4−1) | if λ=1; |
(−1,n4−2λ,n4−2λ+1) | if 2≤λ≤n4−1; |
(λ−n2+4,λ−n2+1,1−n4) | if n4≤λ≤n4+1; |
(1,2λ−3n4,2λ−3n4−1) | if n4+2≤λ≤n2−1; |
(1,n4−1,n4−1) | if λ=n2. |
rE(l2λ,RCn(1,2)) | λ |
(λ,λ,n4−λ,n4−λ) | if 1≤λ≤n4−1; |
(n4,n4,λ−n4+1,λ−n4+1) | if n4≤λ≤n4+1; |
(n2−λ+1,n2−λ+1,λ−n4+1,λ−n4+1) | if n4+2≤λ≤n2−1; |
(1,1,n4,n4) | if λ=n2. |
r′E(l2λ,RCn(1,2)) | λ |
(0,n4−2λ,n4−2λ) | if 1≤λ≤n4−1; |
(0,λ−n2+1,λ−n2+1) | if n4≤λ≤n4+1; |
(0,2λ−3n4,2λ−3n4) | if n4+2≤λ≤n2−1; |
(0,n4−1,n4−1) | if λ=n2. |
rE(h2λ−1,RCn(1,2)) | λ |
(0,n−14,n−14+1,1) | if λ=1; |
(λ,n−14−λ+1,n−14−λ+2,λ) | if 2≤λ≤n−14; |
(n−14+1,1,0,n−14+1) | if λ=n−14+1; |
(n−12−λ+2,λ−n−14,λ−n−14,n−12−λ+2) | if n−14+2≤λ≤n−12; |
(1,n−14+1,n−14+1,0) | if λ=n−12+1. |
r′E(h2λ−1,RCn(1,2)) | λ |
(n−14,n−14+1,1) | if λ=1; |
(n−14−2λ+1,n−14−2λ+2,0) | if 2≤λ≤n−14; |
(−n−14,−n−14−1,0) | if λ=n−14+1; |
(2λ−3n−34−2,2λ−3n−34−2,0) | if n−14+2≤λ≤n−12; |
(n−14,n−14,−1) | if λ=n−12+1. |
rE(h2λ,RCn(1,2)) | λ |
(λ,n−14−λ+1,n−14−λ+1,λ+1) | if 1≤λ≤n−14−1; |
(n−14,0,1,n−14+1) | if λ=n−14; |
(n−12−λ+2,λ−n−14+1,λ−n−14,n−12−λ+1) | if n−14+1≤λ≤n−12. |
r′E(h2λ,RCn(1,2)) | λ |
(n−14−2λ+1,n−14−2λ+1,1) | if 1≤λ≤n−14−1; |
(−n−14,1−n−14,1) | if λ=n−14; |
(2λ−3n−34−1,2λ−3n−34−2,−1) | if n−14+1≤λ≤n−12. |
rE(l2λ−1,RCn(1,2)) | λ |
(λ,n−14−λ+1,n−14−λ+1,λ) | if 1≤λ≤n−14; |
(n−12−λ+2,λ−n−14,λ−n−14,n−12−λ+1) | if n−14+1≤λ≤n−12; |
(1,n−14,n−14+1,1) | if λ=n−12+1. |
r′E(l2λ−1,RCn(1,2)) | λ |
(n−14−2λ+1,n−14−2λ+1,0) | if 1≤λ≤n−14; |
(2λ−3k−2,2λ−3k−2,−1) | if k+1≤λ≤2k; |
(n−14−1,n−14,0) | if λ=n−12+1. |
rE(l2λ,RCn(1,2)) | λ |
(λ,n−14−λ,n−14−λ+1,λ+1) | if 1≤λ≤n−14−1; |
(n−14,1,1,n−14+1) | if λ=n−14; |
(n−12−λ+1,λ−n−14+1,λ−n−14,n−12−λ+1) | if n−14+1≤λ≤n−12. |
r′E(l2λ,RCn(1,2)) | λ |
(n−14−2λ,n−14−2λ+1,1) | if 1≤λ≤n−14−1; |
(1−n−14,1−n−14,1) | if λ=n−14; |
(2λ−3n−34,2λ−3n−34−1,0) | if n−14+1≤λ≤n−12. |
rE(h2λ−1,RCn(1,2)) | λ |
(0,n−24,n−24+1,1) | if λ=1; |
(λ,n−24−λ+1,n−24−λ+2,λ) | if 2≤λ≤n−24; |
(n−24+1,1,0,n−24+1) | if λ=n−24+1; |
(n−22−λ+3,λ−n−24,λ−n−24,n−22−λ+2) | if n−24+2≤λ≤n−22+1. |
r′E(h2λ−1,RCn(1,2)) | λ |
(n−24,n−24+1,1) | if λ=1; |
(n−24−2i+1,n−24−2λ+2,0) | if 2≤λ≤n−24; |
(−n−24,−n−24−1,0) | if λ=n−24+1; |
(2λ−3n−64−3,2λ−3n−64−3,−1) | if n−24+2≤λ≤n−22+1. |
rE(h2λ,RCn(1,2)) | λ |
(λ,n−24−λ+1,n−24−λ+1,λ+1) | if 1≤λ≤n−24−1; |
(n−24,0,1,n−24+1) | if λ=n−24; |
(n−22−λ+2,λ−n−24+1,λ−n−24,n−22−λ+2) | if n−24+1≤λ≤n−22; |
(1,n−24+1,n−24+1,0) | if λ=n−22+1. |
r′E(h2λ,RCn(1,2)) | λ |
(n−24−2λ+1,n−24−2λ+1,1) | if 1≤λ≤n−24−1; |
(−n−24,1−n−24,1) | if λ=n−24; |
(2λ−3n−64−1,2λ−3n−64−2,0) | if n−24+1≤λ≤n−22; |
(n−24,n−24,−1) | if λ=n−22+1. |
rE(l2λ−1,RCn(1,2)) | λ |
(λ,n−24−λ+1,n−24−λ+1,λ) | if 1≤λ≤n−24; |
(n−22−λ+2,λ−n−24,λ−n−24,n−22−λ+2) | if n−24+1≤λ≤n−22+1. |
r′E(l2λ−1,RCn(1,2)) | λ |
(n−24−2λ+1,n−24−2λ+1,0) | if 1≤λ≤n−24; |
(2λ−3n−64−2,2λ−3n−64−2,0) | if n−24+1≤λ≤n−22+1. |
rE(l2λ,RCn(1,2)) | λ |
(λ,n−24−λ,n−24−λ+1,λ+1) | if 1≤λ≤n−24−1; |
(n−24,1,1,n−24+1) | if λ=n−24; |
(n−22−λ+2,λ−n−24+1,−n−24,n−22−λ+1) | if n−24+1≤λ≤n−22; |
(1,n−24,n−24+1,1) | if λ=n−22+1. |
r′E(l2λ,RCn(1,2)) | λ |
(n−24−2λ,n−24−2λ+1,1) | if 1≤λ≤n−24−1; |
(1−n−24,1−n−24,1) | if λ=n−24; |
(2λ−3n−64−1,2λ−3n−64−2,−1) | if n−24+1≤λ≤n−22; |
(n−24−1,n−24,0) | if λ=n−22+1. |
rE(h2λ−1,RCn(1,2)) | λ |
(0,2,n−34,n−34+1) | if λ=1; |
(λ,λ+1,n−34−λ+1,n−34−λ+2) | if 2≤λ≤n−34; |
(n−34+1,n−34+1,1,1) | if λ=n−34+1; |
(n−32−λ+3,n−32−λ+2,λ−n−34,n−34−λ−1) | if n−34+2≤λ≤n−32; |
(2,0,n−34+1,n−34) | if λ=n−32+1; |
(1,2,n−34+1,n−34+1) | if λ=⌊n−32+2⌋. |
r′E(h2λ−1,RCn(1,2)) | λ |
(2,n−34,n−34+1) | if λ=1; |
(1,n−34−2λ+1,n−34−2λ+2) | if 2≤λ≤n−34; |
(0,−n−34,−n−34) | if λ=n−34+1; |
(−1,2λ−3n−94−3,−n−34−4) | if n−34+2≤λ≤n−32; |
(−2,n−34−1,n−34−2) | if λ=n−32+1; |
(1,n−34,n−34) | if λ=⌊n−32+2⌋. |
rE(h2λ,RCn(1,2)) | λ |
(λ,λ+2,n−34−λ+1,n−34−λ+1) | if 1≤λ≤n−34−1; |
(λ,n−32−λ+1,1,1) | if n−34≤λ≤n−34+1; |
(n−32−λ+3,n−32−λ+1,λ−n−34,λ−n−34) | if n−34+2≤λ≤n−32; |
(2,1,n−34+1,n−34+1) | if λ=n−32+1. |
r′E(h2λ,RCn(1,2)) | λ |
(2,n−34−2λ+1,n−34−2λ+1) | if 1≤λ≤n−34−1; |
(n−32−2λ+1,1−λ,1−λ) | if n−34≤λ≤n−34+1; |
(−2,2λ−3n−94−3,2λ−3n−94−3) | if n−34+2≤λ≤n−32; |
(−1,n−34+1,n−34+1) | if λ=n−32+1. |
rE(l2λ−1,RCn(1,2)) | λ |
(λ,λ+1,n−34−λ+1,n−34−λ+1) | if 1≤λ≤n−34−1; |
(λ,n−32−λ+1,2,n−34−λ+1) | if n−34≤λ≤n−34+1; |
(n−32−λ+3,n−32−+1,λ−n−34,λ−n−34−1) | if n−34+2≤λ≤n−32; |
(−n−32+1,λ−n−32,3n−94−λ+2,−n−34−1) | if n−32+1≤λ≤⌊n−34+2⌋. |
r′E(l2λ−1,RCn(1,2)) | λ |
(1,n−34−2λ+1,n−34−2λ+1) | if 1≤λ≤n−34−1; |
(n−32−2λ+1,2−λ,n−34−2λ+1) | if n−34≤λ≤n−34+1; |
(−2,2λ−3n−94−3,2λ−3n−94−4) | if n−34+2≤λ≤n−32; |
(−1,5n−154−2λ−1,n−34−2) | if n−32+1≤λ≤⌊n+12⌋. |
rE(l2λ,RCn(1,2)) | λ |
(λ,λ+2,n−34−λ,n−34−λ+1) | if 1≤λ≤n−34−1; |
(λ,n−32−λ+1,λ−n−34,2) | if n−34≤λ≤n−34+1; |
(n−32−λ+2,n−32−λ+1,λ−n−34,λ−n−34) | if n−34+2≤λ≤n−32; |
(1,1,n−34+1,n−34+1) | if λ=n−32+1. |
r′E(l2λ,RCn(1,2)) | λ |
(2,n−34−2λ,n−34−2λ+1) | if 1≤λ≤n−34−1; |
(n−32−2λ+1,−n−34,2−λ) | if n−34≤λ≤n−34+1; |
(−1,2λ−3n−94−2,2λ−3n−94−2) | if n−34+2≤λ≤n−32; |
(0,n−34,n−34) | if λ=n−32+1. |
rE(h2λ−1,RCn(1,2)) | λ |
(0,1,n4,n4) | if λ=1; |
(λ,λ−1,n4−λ+1,n4−λ+1) | if 2≤λ≤n4−1; |
(n4,n4−1,0,1) | if λ=n4; |
(λ,n4,2,1) | if λ=n4+1; |
(n2−λ+2,n2−λ+2,λ−n4+1,λ−n4) | if n4+2≤λ≤n2. |
r′E(h2λ−1,RCn(1,2)) | λ |
(1,n4,n4) | if λ=1; |
(−1,n4−2λ+1,n4−2λ+1) | if 2≤λ≤n4−1; |
(−1,−n4,1−n4) | if λ=n4; |
(n4−λ,2−λ,1−λ) | if λ=n4+1; |
(0,2λ−3n4−1,2λ−3n4−2) | if n4+2≤λ≤n2. |
rE(h2λ,RCn(1,2)) | λ |
(1,0,n4−1,n4) | if λ=1; |
(λ,λ,n4−λ,n4−λ+1) | if 2≤λ≤n4−1; |
(n4,n4,1,0) | if λ=n4; |
(n2−λ+1,n2−λ+2,λ−n4+1,λ−n4+1) | if n4+1≤λ≤n2. |
r′E(h2λ,RCn(1,2)) | λ |
(−1,n4−2,n4−1) | if λ=1; |
(0,n4−2λ,n4−2λ+1) | if 2≤λ≤n4−1; |
(0,1−n4,−n4) | if λ=n4; |
(1,2λ−3n4,2λ−3n4) | if n4+1≤λ≤n2. |
rE(l2λ−1,RCn(1,2)) | λ |
(1,1,n4−1,n4) | if λ=1; |
(λ,λ−1,n4−λ,n4−λ+1) | if 2≤λ≤n4−1; |
(n4,λ−n4+4,λ−n4+1,1) | if n4≤λ≤n4+1; |
(n2−λ+1,n2−λ+2,λ−n4+1,λ−n4) | if n4+2≤λ≤n2−1; |
(1,2,n4,n4) | if λ=n2. |
r′E(l2λ−1,RCn(1,2)) | λ |
(0,n4−2,n4−1) | if λ=1; |
(−1,n4−2λ,n4−2λ+1) | if 2≤λ≤n4−1; |
(λ−n2+4,λ−n2+1,1−n4) | if n4≤λ≤n4+1; |
(1,2λ−3n4,2λ−3n4−1) | if n4+2≤λ≤n2−1; |
(1,n4−1,n4−1) | if λ=n2. |
rE(l2λ,RCn(1,2)) | λ |
(λ,λ,n4−λ,n4−λ) | if 1≤λ≤n4−1; |
(n4,n4,λ−n4+1,λ−n4+1) | if n4≤λ≤n4+1; |
(n2−λ+1,n2−λ+1,λ−n4+1,λ−n4+1) | if n4+2≤λ≤n2−1; |
(1,1,n4,n4) | if λ=n2. |
r′E(l2λ,RCn(1,2)) | λ |
(0,n4−2λ,n4−2λ) | if 1≤λ≤n4−1; |
(0,λ−n2+1,λ−n2+1) | if n4≤λ≤n4+1; |
(0,2λ−3n4,2λ−3n4) | if n4+2≤λ≤n2−1; |
(0,n4−1,n4−1) | if λ=n2. |
rE(h2λ−1,RCn(1,2)) | λ |
(0,n−14,n−14+1,1) | if λ=1; |
(λ,n−14−λ+1,n−14−λ+2,λ) | if 2≤λ≤n−14; |
(n−14+1,1,0,n−14+1) | if λ=n−14+1; |
(n−12−λ+2,λ−n−14,λ−n−14,n−12−λ+2) | if n−14+2≤λ≤n−12; |
(1,n−14+1,n−14+1,0) | if λ=n−12+1. |
r′E(h2λ−1,RCn(1,2)) | λ |
(n−14,n−14+1,1) | if λ=1; |
(n−14−2λ+1,n−14−2λ+2,0) | if 2≤λ≤n−14; |
(−n−14,−n−14−1,0) | if λ=n−14+1; |
(2λ−3n−34−2,2λ−3n−34−2,0) | if n−14+2≤λ≤n−12; |
(n−14,n−14,−1) | if λ=n−12+1. |
rE(h2λ,RCn(1,2)) | λ |
(λ,n−14−λ+1,n−14−λ+1,λ+1) | if 1≤λ≤n−14−1; |
(n−14,0,1,n−14+1) | if λ=n−14; |
(n−12−λ+2,λ−n−14+1,λ−n−14,n−12−λ+1) | if n−14+1≤λ≤n−12. |
r′E(h2λ,RCn(1,2)) | λ |
(n−14−2λ+1,n−14−2λ+1,1) | if 1≤λ≤n−14−1; |
(−n−14,1−n−14,1) | if λ=n−14; |
(2λ−3n−34−1,2λ−3n−34−2,−1) | if n−14+1≤λ≤n−12. |
rE(l2λ−1,RCn(1,2)) | λ |
(λ,n−14−λ+1,n−14−λ+1,λ) | if 1≤λ≤n−14; |
(n−12−λ+2,λ−n−14,λ−n−14,n−12−λ+1) | if n−14+1≤λ≤n−12; |
(1,n−14,n−14+1,1) | if λ=n−12+1. |
r′E(l2λ−1,RCn(1,2)) | λ |
(n−14−2λ+1,n−14−2λ+1,0) | if 1≤λ≤n−14; |
(2λ−3k−2,2λ−3k−2,−1) | if k+1≤λ≤2k; |
(n−14−1,n−14,0) | if λ=n−12+1. |
rE(l2λ,RCn(1,2)) | λ |
(λ,n−14−λ,n−14−λ+1,λ+1) | if 1≤λ≤n−14−1; |
(n−14,1,1,n−14+1) | if λ=n−14; |
(n−12−λ+1,λ−n−14+1,λ−n−14,n−12−λ+1) | if n−14+1≤λ≤n−12. |
r′E(l2λ,RCn(1,2)) | λ |
(n−14−2λ,n−14−2λ+1,1) | if 1≤λ≤n−14−1; |
(1−n−14,1−n−14,1) | if λ=n−14; |
(2λ−3n−34,2λ−3n−34−1,0) | if n−14+1≤λ≤n−12. |
rE(h2λ−1,RCn(1,2)) | λ |
(0,n−24,n−24+1,1) | if λ=1; |
(λ,n−24−λ+1,n−24−λ+2,λ) | if 2≤λ≤n−24; |
(n−24+1,1,0,n−24+1) | if λ=n−24+1; |
(n−22−λ+3,λ−n−24,λ−n−24,n−22−λ+2) | if n−24+2≤λ≤n−22+1. |
r′E(h2λ−1,RCn(1,2)) | λ |
(n−24,n−24+1,1) | if λ=1; |
(n−24−2i+1,n−24−2λ+2,0) | if 2≤λ≤n−24; |
(−n−24,−n−24−1,0) | if λ=n−24+1; |
(2λ−3n−64−3,2λ−3n−64−3,−1) | if n−24+2≤λ≤n−22+1. |
rE(h2λ,RCn(1,2)) | λ |
(λ,n−24−λ+1,n−24−λ+1,λ+1) | if 1≤λ≤n−24−1; |
(n−24,0,1,n−24+1) | if λ=n−24; |
(n−22−λ+2,λ−n−24+1,λ−n−24,n−22−λ+2) | if n−24+1≤λ≤n−22; |
(1,n−24+1,n−24+1,0) | if λ=n−22+1. |
r′E(h2λ,RCn(1,2)) | λ |
(n−24−2λ+1,n−24−2λ+1,1) | if 1≤λ≤n−24−1; |
(−n−24,1−n−24,1) | if λ=n−24; |
(2λ−3n−64−1,2λ−3n−64−2,0) | if n−24+1≤λ≤n−22; |
(n−24,n−24,−1) | if λ=n−22+1. |
rE(l2λ−1,RCn(1,2)) | λ |
(λ,n−24−λ+1,n−24−λ+1,λ) | if 1≤λ≤n−24; |
(n−22−λ+2,λ−n−24,λ−n−24,n−22−λ+2) | if n−24+1≤λ≤n−22+1. |
r′E(l2λ−1,RCn(1,2)) | λ |
(n−24−2λ+1,n−24−2λ+1,0) | if 1≤λ≤n−24; |
(2λ−3n−64−2,2λ−3n−64−2,0) | if n−24+1≤λ≤n−22+1. |
rE(l2λ,RCn(1,2)) | λ |
(λ,n−24−λ,n−24−λ+1,λ+1) | if 1≤λ≤n−24−1; |
(n−24,1,1,n−24+1) | if λ=n−24; |
(n−22−λ+2,λ−n−24+1,−n−24,n−22−λ+1) | if n−24+1≤λ≤n−22; |
(1,n−24,n−24+1,1) | if λ=n−22+1. |
r′E(l2λ,RCn(1,2)) | λ |
(n−24−2λ,n−24−2λ+1,1) | if 1≤λ≤n−24−1; |
(1−n−24,1−n−24,1) | if λ=n−24; |
(2λ−3n−64−1,2λ−3n−64−2,−1) | if n−24+1≤λ≤n−22; |
(n−24−1,n−24,0) | if λ=n−22+1. |
rE(h2λ−1,RCn(1,2)) | λ |
(0,2,n−34,n−34+1) | if λ=1; |
(λ,λ+1,n−34−λ+1,n−34−λ+2) | if 2≤λ≤n−34; |
(n−34+1,n−34+1,1,1) | if λ=n−34+1; |
(n−32−λ+3,n−32−λ+2,λ−n−34,n−34−λ−1) | if n−34+2≤λ≤n−32; |
(2,0,n−34+1,n−34) | if λ=n−32+1; |
(1,2,n−34+1,n−34+1) | if λ=⌊n−32+2⌋. |
r′E(h2λ−1,RCn(1,2)) | λ |
(2,n−34,n−34+1) | if λ=1; |
(1,n−34−2λ+1,n−34−2λ+2) | if 2≤λ≤n−34; |
(0,−n−34,−n−34) | if λ=n−34+1; |
(−1,2λ−3n−94−3,−n−34−4) | if n−34+2≤λ≤n−32; |
(−2,n−34−1,n−34−2) | if λ=n−32+1; |
(1,n−34,n−34) | if λ=⌊n−32+2⌋. |
rE(h2λ,RCn(1,2)) | λ |
(λ,λ+2,n−34−λ+1,n−34−λ+1) | if 1≤λ≤n−34−1; |
(λ,n−32−λ+1,1,1) | if n−34≤λ≤n−34+1; |
(n−32−λ+3,n−32−λ+1,λ−n−34,λ−n−34) | if n−34+2≤λ≤n−32; |
(2,1,n−34+1,n−34+1) | if λ=n−32+1. |
r′E(h2λ,RCn(1,2)) | λ |
(2,n−34−2λ+1,n−34−2λ+1) | if 1≤λ≤n−34−1; |
(n−32−2λ+1,1−λ,1−λ) | if n−34≤λ≤n−34+1; |
(−2,2λ−3n−94−3,2λ−3n−94−3) | if n−34+2≤λ≤n−32; |
(−1,n−34+1,n−34+1) | if λ=n−32+1. |
rE(l2λ−1,RCn(1,2)) | λ |
(λ,λ+1,n−34−λ+1,n−34−λ+1) | if 1≤λ≤n−34−1; |
(λ,n−32−λ+1,2,n−34−λ+1) | if n−34≤λ≤n−34+1; |
(n−32−λ+3,n−32−+1,λ−n−34,λ−n−34−1) | if n−34+2≤λ≤n−32; |
(−n−32+1,λ−n−32,3n−94−λ+2,−n−34−1) | if n−32+1≤λ≤⌊n−34+2⌋. |
r′E(l2λ−1,RCn(1,2)) | λ |
(1,n−34−2λ+1,n−34−2λ+1) | if 1≤λ≤n−34−1; |
(n−32−2λ+1,2−λ,n−34−2λ+1) | if n−34≤λ≤n−34+1; |
(−2,2λ−3n−94−3,2λ−3n−94−4) | if n−34+2≤λ≤n−32; |
(−1,5n−154−2λ−1,n−34−2) | if n−32+1≤λ≤⌊n+12⌋. |
rE(l2λ,RCn(1,2)) | λ |
(λ,λ+2,n−34−λ,n−34−λ+1) | if 1≤λ≤n−34−1; |
(λ,n−32−λ+1,λ−n−34,2) | if n−34≤λ≤n−34+1; |
(n−32−λ+2,n−32−λ+1,λ−n−34,λ−n−34) | if n−34+2≤λ≤n−32; |
(1,1,n−34+1,n−34+1) | if λ=n−32+1. |
r′E(l2λ,RCn(1,2)) | λ |
(2,n−34−2λ,n−34−2λ+1) | if 1≤λ≤n−34−1; |
(n−32−2λ+1,−n−34,2−λ) | if n−34≤λ≤n−34+1; |
(−1,2λ−3n−94−2,2λ−3n−94−2) | if n−34+2≤λ≤n−32; |
(0,n−34,n−34) | if λ=n−32+1. |