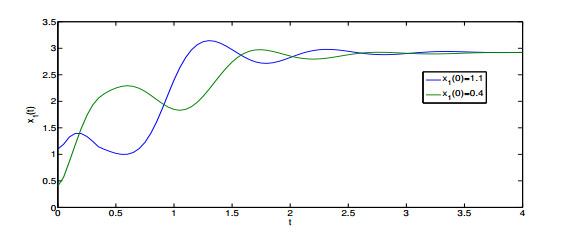
This paper was mainly concerned with the stability analysis of a class of fractional-order neural networks with S-type distributed delays. By using the properties of Riemann-Liouville fractional-order derivatives and integrals, along with the additivity of integration intervals and initial conditions, fractional-order integrals of the state function with S-type distributed delays were transformed into fractional-order integrals of the state function without S-type distributed delays. By virtue of the theory of contractive mapping and the Bellman-Gronwall inequality, the sufficient conditions for finite-time stability and global Mittag-Leffler stability were obtained when certain conditions were satisfied. Moreover, the correctness and realizability of the conclusion were verified through the presentation of two illustrative numerical simulation examples.
Citation: Wei Liu, Qinghua Zuo, Chen Xu. Finite-time and global Mittag-Leffler stability of fractional-order neural networks with S-type distributed delays[J]. AIMS Mathematics, 2024, 9(4): 8339-8352. doi: 10.3934/math.2024405
[1] | Huizhen Qu, Jianwen Zhou . $ S $-asymptotically $ \omega $-periodic dynamics in a fractional-order dual inertial neural networks with time-varying lags. AIMS Mathematics, 2022, 7(2): 2782-2809. doi: 10.3934/math.2022154 |
[2] | Yuehong Zhang, Zhiying Li, Wangdong Jiang, Wei Liu . The stability of anti-periodic solutions for fractional-order inertial BAM neural networks with time-delays. AIMS Mathematics, 2023, 8(3): 6176-6190. doi: 10.3934/math.2023312 |
[3] | Dan-Ning Xu, Zhi-Ying Li . Mittag-Leffler stabilization of anti-periodic solutions for fractional-order neural networks with time-varying delays. AIMS Mathematics, 2023, 8(1): 1610-1619. doi: 10.3934/math.2023081 |
[4] | Jingfeng Wang, Chuanzhi Bai . Global Mittag-Leffler stability of Caputo fractional-order fuzzy inertial neural networks with delay. AIMS Mathematics, 2023, 8(10): 22538-22552. doi: 10.3934/math.20231148 |
[5] | Ivanka Stamova, Gani Stamov . Impulsive control strategy for the Mittag-Leffler synchronization of fractional-order neural networks with mixed bounded and unbounded delays. AIMS Mathematics, 2021, 6(3): 2287-2303. doi: 10.3934/math.2021138 |
[6] | Mohammed D. Kassim . A fractional Halanay inequality for neutral systems and its application to Cohen-Grossberg neural networks. AIMS Mathematics, 2025, 10(2): 2466-2491. doi: 10.3934/math.2025115 |
[7] | Pratap Anbalagan, Evren Hincal, Raja Ramachandran, Dumitru Baleanu, Jinde Cao, Michal Niezabitowski . A Razumikhin approach to stability and synchronization criteria for fractional order time delayed gene regulatory networks. AIMS Mathematics, 2021, 6(5): 4526-4555. doi: 10.3934/math.2021268 |
[8] | Yang Xu, Zhouping Yin, Yuanzhi Wang, Qi Liu, Anwarud Din . Mittag-Leffler projective synchronization of uncertain fractional-order fuzzy complex valued neural networks with distributed and time-varying delays. AIMS Mathematics, 2024, 9(9): 25577-25602. doi: 10.3934/math.20241249 |
[9] | Hongmei Zhang, Xiangnian Yin, Hai Zhang, Weiwei Zhang . New criteria on global Mittag-Leffler synchronization for Caputo-type delayed Cohen-Grossberg Inertial Neural Networks. AIMS Mathematics, 2023, 8(12): 29239-29259. doi: 10.3934/math.20231497 |
[10] | Jiaqing Zhu, Guodong Zhang, Leimin Wang . Quasi-projective and finite-time synchronization of fractional-order memristive complex-valued delay neural networks via hybrid control. AIMS Mathematics, 2024, 9(3): 7627-7644. doi: 10.3934/math.2024370 |
This paper was mainly concerned with the stability analysis of a class of fractional-order neural networks with S-type distributed delays. By using the properties of Riemann-Liouville fractional-order derivatives and integrals, along with the additivity of integration intervals and initial conditions, fractional-order integrals of the state function with S-type distributed delays were transformed into fractional-order integrals of the state function without S-type distributed delays. By virtue of the theory of contractive mapping and the Bellman-Gronwall inequality, the sufficient conditions for finite-time stability and global Mittag-Leffler stability were obtained when certain conditions were satisfied. Moreover, the correctness and realizability of the conclusion were verified through the presentation of two illustrative numerical simulation examples.
The study of neural networks (NNs) with time delays is crucial for addressing practical problems. Time delays can be generally categorized as discrete delays, leakage delays, and distributed delays. Fractional-order calculus extends the order of nonlinear system models from integer-order to fractional-order. The control problem of fractional-order nonlinear systems has been a prominent and challenging research area in control theory and numerous research results have been obtained in this field. In [1], a novel decentralized non-integer order controller applied on the nonlinear fractional-order composite system was proposed, and some novel results for the asymptotic stabilization were obtained. In [2], a variable structure adaptive fuzzy control scheme was designed to solve the unknown dead-zone input nonlinearities which are considered in the Riemann-Liouville and Caputo fractional-order nonlinear systems. The authors in [3] proposed some novel stabilization methods and investigated the gradient control of a nonlinear fractional-order system. An adaptive composite dynamic surface control scheme was first proposed for nonlinear fractional-order systems subject to delayed input in [4]. In [5], the authors introduced a composite learning adaptive backstepping fuzzy control method for functional uncertainties of fractional-order nonlinear systems. In [6], the event-triggered predefined-time output feedback control problem was investigated for fractional-order nonlinear systems with input saturation. In the past decade, fractional-order neural networks (FNNs) with time delays have attracted wide and considerable attention because incorporating fractional-order derivatives into NNs can well describe the dynamical behavior of neurons, holding significant relevance across a broad spectrum of applications. Therefore, the study of the dynamical properties of FNNs with time delays has received considerable attention among many scholars and there have been numerous results, such as bifurcation properties [7,8], Mittag-Leffler synchronization [9,10], finite-time stability and synchronization[11,12,13,14,15], asymptotical stability [16,17,18,19,20,21], multistability [22], quasi-uniform stability [23], and synchronization control [24,25].
In 2002, Wang and Xu firstly introduced S-type distributed delays into bidirectional associative memory (BAM) NNs [26], which comprises discrete delays and continuously distributed delays in terms of Lebesgue-Stieltjes integral. The emergence of S-type distributed delays has aroused the interest of numerous scholars, leading investigations into the stability problems of NNs with S-type distributed delays (SDNNs), including global asymptotic stability [26], robust stability [27], global exponential robust stability [28,29], global exponential stability [30,31], and some solution problems of SDNNs, such as mild solution [32] and periodic solution [33].
However, to the best of our knowledge, research on the dynamical properties of fractional-order neural networks with S-type distributed delays (FSDNNs) has not been found. Therefore, the investigation of finite-time stability (FTS) and global Mittag-Leffler stability (GMLS) of FSDNNs in this paper is more interesting and meaningful in both theoretical development and practical application. The main contributions of this paper are summarized as follows.
● FSDNNs incorporating discrete delays and continuously distributed delays as S-type distributed delays in the sense of Lebesgue-Stieltjes integral are established.
● Fractional-order integrals of the state function with S-type distributed delays are transformed into fractional-order integrals of the state function without S-type distributed delays.
● Sufficient conditions for FTS and GMLS of FSDNNs are obtained when certain conditions are satisfied. This provides a new basis for further expanding NNs research and practical applications.
The paper is structured as follows: In Section 2, the FSDNNs formulation and some preliminaries contents are presented. In Section 3, some criteria for FTS and GMLS of FSDNNs are derived. In Section 4, two numerical simulations examples are presented to illustrate the correctness and realizability of our conclusion. In the end, a conclusion is drawn in Section 5.
We consider a class of FSDNNs described by
Dαtxi(t)=−dixi(t)+n∑j=1aijfj(xj(t))+n∑j=1bijfj(∫0−∞xj(t+θ)dwj(θ))+Ii,i=1,⋯,n | (2.1) |
with initial condition
xi(t)=φi(t),t∈(−∞,0]. |
In system (2.1), Dαt is an α-order (0<α<1) Riemann-Liouville derivative, fj(xj(t)) denotes activation function, aij and bij represent the weight between the jth neuron and the ith neuron, Ii is an input signal introduced from outside to the ith neuron, di>0 denotes the rate of the ith neuron returning to resting state without any connection, and the past effect of the jth neuron on the ith neuron is given by the Lebesgue-Stieltjes integral ∫0−∞xj(t+θ)dwj(θ) with nondecreasing bounded variation function wj(θ) and satisfies ∫0−∞dwj(θ)=kj>0,j=1,⋯,n.
The assumption that accompanies system (2.1) is given as follows:
Assumption A1: The output functions fi(⋅) satisfying Lipschitz condition, i.e., exists Fi>0, which satisfies
|fi(u)−fi(v)|≤Fi|u−v|,∀ u,v∈R,i=1,⋯,n. |
Definition 2.1. [34] The Riemann-Liouville fractional integral with order q of function f(t) is defined as
t0Iqtf(t)=1Γ(q)∫tt0(t−τ)q−1f(τ)dτ, |
for all t≥t0, q>0∈R, where Γ(τ)=∫+∞0tτ−1e−tdt.
Definition 2.2. [34] The Riemann-Liouville fractional derivative with order q is defined as
RLaDqxf(x)=Dn(aIn−qxf(x))=1Γ(n−q)dndxn∫xaf(t)(x−t)q−n+1dt, |
where n−1≤q<n, n∈Z+, q∈R.
Definition 2.3. [34] The Mittag-Leffler function with one parameter is defined as
Eα(z)=∞∑k=0zkΓ(kα+1), |
where α>0 and z∈C.
Lemma 2.4. [35] If u(t)∈C[0,+∞], and there exist c1>0,c2>0, which satisfy u(t)≤−c1Iqtu(t)+c2, then
u(t)≤c2Eq(−c1tq), |
where 0<q<1 and Eq(⋅) denotes the one-parameter Mittag-Leffler function.
Lemma 2.5. (Bellman-Gronwall inequality [36]) Assume that function y(t) satisfies
y(t)≤α(t)+∫t0β(τ)y(τ)dτ, |
with α(t) and β(t) being known real-valued positive functions. If α(t) is differentiable, then
y(t)≤α(0)exp[∫t0β(τ)dτ]+∫t0˙α(τ)exp[∫tτβ(r)dr]dτ. |
Definition 2.6. [37] The equilibrium point u∗=(u∗1,⋯,u∗n)T of system (2.1) is FTS with respect to {δ,ε,t0,J},J=[t0,t0+H],0<H<+∞,t0 denoting the initial time observation of the system, 0<δ<ε,δ,ε∈R, and any solution u(t,t0,φ) with initial condition ui(s)=φi(s),s∈(−∞,0],i=1,⋯,n, if and only if ‖φ−u∗‖<δ, implies
‖u(t)−u∗‖<ε,∀t∈J, |
where ‖φ−u∗‖=sup−∞≤s≤0n∑i=1|φi(s)−u∗i|, ‖u(t)−u∗‖=n∑i=1|ui(t)−u∗i|.
Definition 2.7. [35] If there exist constants ρ1≥0 and ρ2≥0, let x(t) and y(t) be two different solutions of system (2.1) with different initial values xi(s)=φi(s) and yi(s)=ψi(s), s∈(−∞,0]. The solution of system (2.1) is said to be GMLS if x(t) and y(t) satisfy
‖x−y‖≤[M(φ−ψ)Eq(−ρ1tρ1)]ρ2,t≥0, |
where x(t)=(x1(t),⋯,xn(t))T, y(t)=(y1(t),⋯,yn(t))T, φ(t)=(φ1(t),⋯,φn(t))T, ψ(t)=(ψ1(t),⋯,ψn(t))T, M(φ−ψ)≥0, M(0)=0, Eq is the Mittag-Leffler function with one parameter.
Theorem 3.1. For δ,ε,J is defined in Definition 2.6 and 0<δ<ε. If Assumption A1 and
n∑i=11dimax1≤j≤n{(|aij|+|bij|kj)Fi}<1, |
ηi=n∑j=1(|aji|+|bji|ki)Fi−di>0,i=1,⋯,n,ˉη=max1≤i≤n{ηi}, |
δn∑i=1n∑j=1|bij|FjkjtαΓ(α+1)eˉηΓ(α+1)tα<ε, |
hold, then there exists a unique equilibrium point in FSDNNs (2.1), which is FTS.
Proof. Let x(t)=(x1(t),⋯,xn(t))T∈Rn and define a mapping P:Rn→Rn by P(x)=(Px1,⋯,Pxn)T, where
Pxi=1di[n∑j=1aijfj(xj)+n∑j=1bijfj(∫0−∞xjdwj(θ))+Ii],i=1,⋯,n. |
We prove that the mapping P is contractive:
y(t)=(y1(t),⋯,yn(t))T∈Rn, which yields
‖P(x)−P(y)‖=n∑i=1|Pxi−Pyi|≤n∑i=11di[n∑j=1|aij||fj(xj)−fj(yj)|+n∑j=1|bij||fj(∫0−∞xjdwj(θ))−fj(∫0−∞yjdwj(θ))|]≤n∑i=11di[n∑j=1|aij|Fj|xj−yj|+n∑j=1|bij|Fj∫0−∞|xj−yj|dwj(θ)]≤n∑i=11dimax1≤j≤n{(|aij|+|bij|kj)Fi}n∑j=1|xj−yj|<‖x−y‖. | (3.1) |
Thus, P is the contractive mapping. In light of contractive mapping theory, there exists the unique point u∗=(u∗1,⋯,u∗n)T satisfying P(u∗)=u∗, Pu∗i=u∗i, i=1,⋯,n. Thus
u∗i=1di[n∑j=1aijfj(u∗j)+n∑j=1bijfj(∫0−∞u∗jdwj(θ))+Ii], |
and we have
−diu∗i+n∑j=1aijfj(u∗j)+n∑j=1bijfj(∫0−∞u∗jdwj(θ))+Ii=0, |
then u∗ is the unique equilibrium point in FSDNNs (2.1).
We prove the unique equilibrium point u∗ is finite-time stable as follows.
Let yi(t)=xi(t)−u∗i, for 0<δ<ε, and solution x(t,t0,φ) satisfies initial condition xi(s)=φi(s),s∈(−∞,0], i=1,⋯,n, such that ‖φ−u∗‖<δ. From FSDNNs (2.1), we have
Dαtyi(t)=−diyi(t)+n∑j=1aij[fj(xj(t))−fj(u∗j)]+n∑j=1bij[fj(∫0−∞xj(t+θ)dwj(θ))−fj(∫0−∞u∗jdwj(θ))],Dαt|yi(t)|≤sgn{yi(t)}Dαtyi(t)≤−di|yi(t)|+n∑j=1|aij|Fj|yj(t)|+n∑j=1|bij|Fj∫0−∞|yj(t+θ)|dwj(θ), | (3.2) |
|yi(t)|≤−diIαt|yi(t)|+n∑j=1|aij|FjIαt|yj(t)|+n∑j=1|bij|FjIαt(∫0−∞|yj(t+θ)|dwj(θ)),i=1,⋯,n. | (3.3) |
Case (a). When −∞<θ≤0, t>0, t+θ≤0,
Iαt(∫0−∞|yj(t+θ)|dwj(θ))=∫0−∞[1Γ(α)∫t0(t−τ)α−1|yj(τ+θ)|dτ]dwj(θ)z=τ+θ=∫0−∞[1Γ(α)∫t+θθ(t−z+θ)α−1|yj(z)|dz]dwj(θ)≤sup−∞<s≤0|φj(s)−u∗j|1Γ(α)∫0−∞[∫t+θθ(t−z+θ)α−1dz]dwj(θ)≤sup−∞<s≤0|φj(s)−u∗j|tαΓ(α+1)∫0−∞dwj(θ)=sup−∞<s≤0|φj(s)−u∗j|tαΓ(α+1)kj. | (3.4) |
Case (b). When −∞<θ≤0, t>0, t+θ≥0,
Iαt(∫0−∞|yj(t+θ)|dwj(θ))=∫0−∞[1Γ(α)∫t0(t−τ)α−1|yj(τ+θ)|dτ]dwj(θ)=∫0−∞[1Γ(α)∫t+θθ(t−z+θ)α−1|yj(z)|dz]dwj(θ)(3.3)=∫0−∞[1Γ(α)∫0θ(t−z+θ)α−1|yj(z)|dz]dwj(θ)+∫0−∞[1Γ(α)∫t+θ0(t−z+θ)α−1|yj(z)|dz]dwj(θ)≤sup−∞<s≤0|φj(s)−u∗j|tαΓ(α+1)ki+∫0−∞[1Γ(α)∫t0(t−s)α−1|yj(s)|ds]dwj(θ)=(sup−∞<s≤0|φj(s)−u∗j|tαΓ(α+1)+Iαt|yj(t)|)kj. | (3.5) |
When −∞<θ≤0, t>0, it follows from (3.4) and (3.5) that
Iαt(∫0−∞|yj(t+θ)|dwj(θ))≤(sup−∞<s≤0|φj(s)−u∗j|tαΓ(α+1)+Iαt|yj(t)|)kj,j=1,⋯,n. | (3.6) |
Substituting (3.6) into (3.3), we have
n∑i=1|yi(t)|≤n∑i=1(−diIαt|yi(t)|+n∑j=1|aij|FjIαt|yj(t)|+n∑j=1|bij|Fj(sup−∞<s≤0|φj(s)−u∗j|tαΓ(α+1)+Iαt|yj(t)|)kj)=n∑i=1(−diIαt|yi(t)|+n∑j=1|aji|FiIαt|yi(t)|+n∑j=1|bij|Fjkjsup−∞<s≤0|φj(s)−u∗j|tαΓ(α+1)+n∑j=1|bji|FikiIαt|yi(t)|)=n∑i=1([−di+n∑j=1(|aji|+|bji|ki)Fi]Iαt|yi(t)|+n∑j=1|bij|FjkjtαΓ(α+1)sup−∞<s≤0|φj(s)−u∗j|) |
≤max1≤i≤n{n∑j=1(|aji|+|bji|ki)Fi−di}1Γ(α)∫t0(t−s)α−1n∑i=1|yi(s)|ds+n∑i=1n∑j=1|bij|FjkjtαΓ(α+1)sup−∞<s≤0|φj(s)−u∗j|=ˉηΓ(α)∫t0(t−s)α−1n∑i=1|yi(s)|ds+M(φ−u∗), | (3.7) |
where
ˉη=max1≤i≤n{ηi},ηi=n∑j=1(|aji|+|bji|ki)Fi−di, |
M(φ−u∗)=n∑i=1n∑j=1|bij|FjkjtαΓ(α+1)sup−∞<s≤0|φj(s)−u∗j|. |
By Lemma 2.5 and from (3), we obtain
n∑i=1|yi(t)|≤n∑i=1n∑j=1|bij|Fjkjsup−∞<s≤0|φj(s)−u∗j|∫t0τα−1Γ(α)eηΓ(α)∫tτ(t−r)α−1drdτ=n∑i=1n∑j=1|bij||Fjkjsup−∞<s≤0|φj(s)−u∗j|∫t0τα−1Γ(α)eηΓ(α+1)(t−τ)αdτ≤n∑i=1n∑j=1|bij||Fjkjsup−∞<s≤0|φj(s)−u∗j|eηΓ(α+1)tα∫t0τα−1Γ(α)dτ=n∑i=1n∑j=1|bij||Fjkjsup−∞<s≤0|φj(s)−u∗j|eηΓ(α+1)tαtαΓ(α+1)≤δn∑i=1n∑j=1|bij||FjkjtαΓ(α+1)eηΓ(α+1)tα<ε. | (3.8) |
By Definition 2.6, the equilibrium point of FSDNNs (2.1) is FTS. This proves the theorem.
Theorem 3.2. Under the Assumption A1, for t∈[0,T], (0<T<+∞), if
ηi=n∑j=1(|aji|+|bji|ki)Fi−di<0,i=1,⋯,n, |
the solution of FSDNNs (2.1) is GMLS.
Proof. Let x(t)=(x1(t),⋯,xn(t))T and y(t)=(y1(t),⋯,yn(t))T be two solutions of FSDNNs (2.1), satisfying initial conditions xi(s)=φi(s) and yi(s)=ψi(s), s∈(−∞,0].
Let zi(t)=xi(t)−yi(t), i=1,⋯,n. From FSDNNs (2.1), we obtain
Dαtzi(t)=−dizi(t)+n∑j=1aij[fj(xj(t))−fj(yj(t))]+n∑j=1bij[fj(∫0−∞xj(t+θ)dwj(θ))−fj(∫0−∞yj(t+θ)dwj(θ))],Dαt|zi(t)|≤sgn{zi(t)}Dαtzi(t)≤−di|zi(t)|+n∑j=1|aij|Fj|zj(t)|+n∑j=1|bij|Fj∫0−∞|zj(t+θ)|dwj(θ), | (3.9) |
|zi(t)|≤−diIαt|zi(t)|+n∑j=1|aij|FjIαt|zj(t)|+n∑j=1|bij|FjIαt(∫0−∞|zj(t+θ)|dwj(θ)),i=1,⋯,n. | (3.10) |
Similar to the derivation of (3.6), we can have
Iαt(∫0−∞|zi(t+θ)|dwi(θ))≤(sup−∞<s≤0|φi(s)−ψi(s)|TαΓ(α+1)+Iαt|zi(t)|)ki,i=1,⋯,n. | (3.11) |
Substituting (3.11) into (3.10), we obtain
n∑i=1|zi(t)|≤n∑i=1(−diIαt|zi(t)|+n∑j=1|aij|FjIαt|zj(t)|+n∑j=1|bij|Fj(sup−∞<s≤0|φj(s)−ψj(s)|TαΓ(α+1)+Iαt|zj(t)|)kj)=n∑i=1(−diIαt|zi(t)|+n∑j=1|aji|FiIαt|zi(t)|+n∑j=1|bij|Fjkjsup−∞<s≤0|φj(s)−ψj(s)|TαΓ(α+1)+n∑j=1|bji|FikiIαt|zi(t)|)=n∑i=1([−di+n∑j=1(|aji|+|bji|ki)Fi]Iαt|zi(t)|+n∑j=1|bij|FjkjTαΓ(α+1)sup−∞<s≤0|φj(s)−ψj(s)|)≤−min1≤i≤n{di−n∑j=1(|aji|+|bji|ki)Fi}Iαt⋅n∑i=1|zi(t)|+n∑i=1n∑j=1|bij|FjkjTαΓ(α+1)sup−∞<s≤0|φj(s)−ψj(s)|=−η_⋅Iαtn∑i=1|zj(t)|+M(φ−ψ), | (3.12) |
where
η_=min1≤i≤n{−ηi},ηi=n∑j=1(|aji|+|bji|ki)Fi−di,i=1,⋯,n, |
M(φ−ψ)=n∑i=1n∑j=1|bij|FjkjTαΓ(α+1)sup−∞<s≤0|φj(s)−ψj(s)|. |
By Lemma 2.4, it follows from (3.12) that n∑i=1|zi(t)|≤M(φ−ψ)Eα(−η_tα), or ‖x−y‖≤M(φ−ψ)Eα(−η_tα). Moreover, M(φ−ψ)≥0, M(0)=0, where Eα(⋅) denotes the one-parameter Mittag-Leffler function. Thus, by Definition 2.7, the solution of FSDNNs (2.1) is GMLS.
Remark. Theorem 3.1 presents sufficient condition for FTS of FSDNNs when
ηi=n∑j=1(|aji|+|bji|ki)Fi−di>0, |
and Theorem 3.2 presents sufficient condition for GMLS of FSDNNs when
ηi=n∑j=1(|aji|+|bji|ki)Fi−di<0. |
Thus, the parameter
ηi=n∑j=1(|aji|+|bji|ki)Fi−di,i=1,⋯,n |
is the dividing quantity of two kinds of stability of FSDNNs, and we can choose the appropriate parameter and theorem to determine the stability of FSDNNs according to the requirements of practical applications and problems.
In this section, two numerical examples are presented to illustrate our theorems.
We consider a class of FSDNNs as follows:
Dαtxi(t)=−dixi(t)+2∑j=1aijfj(xj(t))+2∑j=1bijfj(∫0−∞xj(t+θ)dωj(θ))+Ii,i=1,2, | (4.1) |
where wj(θ) is a nondecreasing bounded variation function on the interval of (−∞,0], and we set
wj(θ)={−1,θ≤−τj,0,−τj<θ≤0.j=1,2.τj>0. | (4.2) |
Thus, we see that
∫0−∞dwj(θ)=1,∫0−∞xj(t+θ)dwj(θ))=xj(t−τj). |
Example 4.1. Let the parameters and the functions in FSDNNs (4.1) be: α=0.8, d1=0.1, d2=0.11, a11=0.01, a12=0.015, a21=−0.01, a22=0.015, b11=0.012, b12=0.02, b21=−0.0125, b22=0.015. I1=0.6, I2=0.4, fj(xj(t))=2.5sin(xj(t)), j=1,2.
|fj(vj)−fj(uj)|≤2.5|vj−uj|,j=1,2. |
Let Fj=2.5,kj=1,j=1,2, ε=0.02,δ=0.015, t0=2.5,H=1.5,t∈J=[t0,t0+H]=[2.5,4]. We can see Assumptions A1 are satisfied. It can be obtained by calculation:
ηi=2∑j=1(|aji|+|bji|ki)Fi−di>0,i=1,2, |
2∑i=11dimax1≤j≤n{(|aij|+|bij|kj)Fi}<1, |
δ2∑i=12∑j=1|bij|kjFjtαΓ(α+1)emax{ηi}Γ(α+1)tα<ε, t∈J=[2.5,4], |
which satisfies the conditions in Theorem 3.1. According to Theorem 3.1, there exists a unique equilibrium point in FSDNNs (4.1), which is FTS. According to simulation, we can see the trajectories of state variables in Example 4.1 (Figures 1 and 2).
Example 4.2. Let the parameters and the functions in FSDNNs (4.1) be: α=0.8, d1=2.5, d2=3, a11=0.3, a12=0.5, a21=−0.4, a22=0.15, b11=0.2, b12=0.2, b21=−0.25, b22=0.35. I1=0,3, I2=0,2, fj(xj(t))=110sin(xj(t)), j=1,2.
|fj(vj)−fj(uj)|≤110|vj−uj|,j=1,2. |
Let Fj=110, kj=1, j=1,2.
We can see Assumptions A1 are satisfied and the parameters are
n∑j=1(|aji|+|bji|ki)Fi−di<0,i=1,2, |
which satisfy the conditions in Theorem 3.2. According to Theorem 3.2, FSDNNs (4.1) are GMLS. According to simulation, we can see the trajectories of state variables in Example 4.2 (Figures 3 and 4). Obviously, the simulation and Theorem 3.2 are consistent.
In this paper, we mainly investigate the stability of a class of FSDNNs and obtain the sufficient conditions for FTS and GMLS of FSDNNs, i.e., Theorems 3.1 and 3.2, which comprise discrete and continously distributed delays. Specially, when the parameter satisfies n∑j=1(|aji|+|bji|ki)Fi−di>0,i=1,⋯,n, the solution of FSDNNs is FTS, and when the parameter satisfies n∑j=1(|aji|+|bji|ki)Fi−di<0,i=1,⋯,n, the solution of FSDNNs is GMLS. The results are complementary, which provides a new basis for further expanding NNs research and practical applications.
The conclusion is obtained by using Riemann-Liouville fractional-order derivatives in this paper. However, our next research endeavor will involve investigating FTS and GMLS with Caputo fraction-order derivatives. Moreover, this paper provides new useful tools and methods to investigate the stability problem of other types of NNs with S-type distributed delays, such as the stability of fractional-order Cohen-Grossberg NNs with S-type distributed delays and fractional-order BAM NNs with S-type distributed delays.
The authors declare they have not used Artificial Intelligence (AI) tools in the creation of this article.
The authors acknowledge The Ministry of Education's Cooperative Education Program for Industry-University Cooperation (220505876312732), The Science Research Key Project of Shaoxing University (2023LG017), The Science Research Project of Shaoxing University Yuanpei College (KY2022C03), and The Science Project of Zhejiang Educational Department (Y202145903).
The authors declare that there is no conflict of interests regarding the publication of this paper.
[1] |
Z. Zhang, Y. Wang, J. Zhang, Z. Ai, F. Y. Cheng, F. Liu, Novel fractional-order decentralized control for nonlinear fractional-order composite systems with time delays, ISA T., 128 (2022), 230–242. https://doi.org/10.1016/j.isatra.2021.11.044 doi: 10.1016/j.isatra.2021.11.044
![]() |
[2] |
S. Ha, L. Chen, H. Liu, Adaptive fuzzy variable structure control of fractional-order nonlinear systems with input nonlinearities, Int. J. Fuzzy Syst., 23 (2021), 2309–2323. https://doi.org/10.1007/s40815-021-01105-x doi: 10.1007/s40815-021-01105-x
![]() |
[3] |
G. F. Anaya, O. M. Fuentes, A. J. M. Vázquez, J. D. S. Torres, L. A. Q. Téllez, F. M. Vázquez, Passive decomposition and gradient control of fractional-order nonlinear systems, Nonlinear Dynam., 109 (2022), 1705–1722. https://doi.org/10.1007/s11071-022-07531-2 doi: 10.1007/s11071-022-07531-2
![]() |
[4] |
S. Liu, H. Wang, T. Li, Adaptive composite dynamic surface neural control for nonlinear fractional-order systems subject to delayed input, ISA T., 134 (2023), 122–133. https://doi.org/10.1016/j.isatra.2022.07.027 doi: 10.1016/j.isatra.2022.07.027
![]() |
[5] |
H. Qiu, H. Liu, X. Zhang, Historical data-driven composite learning adaptive fuzzy control of fractional-order nonlinear systems, Int. J. Fuzzy Syst., 25 (2022), 1156–1170. https://doi.org/10.1007/s40815-022-01430-9 doi: 10.1007/s40815-022-01430-9
![]() |
[6] |
M. Cui, S. Tong, Event-triggered predefined-time output feedback control for fractional-order nonlinear systems with input saturation, IEEE T. Fuzzy Syst., 31 (2023), 4397–4409. https://doi.org/10.1109/TFUZZ.2023.3283783 doi: 10.1109/TFUZZ.2023.3283783
![]() |
[7] |
C. Xu, M. Liao, P. Li, Y. Guo, Z. Liu, Bifurcation properties for fractional order delayed BAM neural networks, Cogn. Comput., 13 (2021), 322–356. https://doi.org/10.1007/s12559-020-09782-w doi: 10.1007/s12559-020-09782-w
![]() |
[8] |
L. Si, M. Xiao, G. Jiang, Z. Cheng, Q. Song, J. Cao, Dynamics of fractional-order neural networks with discrete and distributed delays, IEEE Access, 8 (2019), 46071–46080. https://doi.org/10.1109/ACCESS.2019.2946790 doi: 10.1109/ACCESS.2019.2946790
![]() |
[9] |
I. Stamova, Global Mittag-Leffler stability and synchronization of impulsive fractional-order neural networks with time-varying delays, Nonlinear Dynam., 77 (2014), 1251–1260. https://doi.org/10.1007/s11071-014-1375-4 doi: 10.1007/s11071-014-1375-4
![]() |
[10] |
B. Zheng, Z. Wang, Mittag-Leffler synchronization of fractional-order coupled neural networks with mixed delays, Appl. Math. Comput., 430 (2022), 127303. https://doi.org/10.1016/j.amc.2022.127303 doi: 10.1016/j.amc.2022.127303
![]() |
[11] |
Z. Zhang, J. Cao, Novel finite-time synchronization criteria for inertial neural networks with time delays via integral inequality method, IEEE T. Neur. Net. Lear., 30 (2019), 1476–1485. https://doi.org/10.1109/TNNLS.2018.2868800 doi: 10.1109/TNNLS.2018.2868800
![]() |
[12] |
F. Du, J. Lu, New results on finite-time stability of fractional-order Cohen-Grossberg neural networks with time delays, Asian J. Control, 24 (2022), 2328–2337. https://doi.org/10.1002/asjc.2641 doi: 10.1002/asjc.2641
![]() |
[13] |
F. Du, J. Lu, New approach to finite-time stability for fractional-order BAM neural networks with discrete and distributed delays, Chaos Soliton. Fract., 151 (2021), 111225. https://doi.org/10.1016/j.chaos.2021.111225 doi: 10.1016/j.chaos.2021.111225
![]() |
[14] |
Z. Zhang, J. Cao, Finite-time synchronization for fuzzy inertial neural networks by maximum value approach, IEEE T. Fuzzy Syst., 30 (2022), 1436–1446. https://doi.org/10.1109/TFUZZ.2021.3059953 doi: 10.1109/TFUZZ.2021.3059953
![]() |
[15] |
Z. Yang, J. Zhang, Z. Zhang, J. Mei, An improved criterion on finite-time stability for fractional-order fuzzy cellular neural networks involving leakage and discrete delays, Math. Comput. Simulat., 203 (2023), 910–925. https://doi.org/10.1016/j.matcom.2022.07.028 doi: 10.1016/j.matcom.2022.07.028
![]() |
[16] |
B. He, H. Zhou, Asymptotic stability and synchronization of fractional order Hopfield neural networks with unbounded delay, Math. Method. Appl. Sci., 46 (2023), 3157–3175. https://doi.org/10.1002/mma.8000 doi: 10.1002/mma.8000
![]() |
[17] |
Z. Yao, Z. Yang, Y. Fu, J. Li, Asymptotical stability for fractional-order Hopfield neural networks with multiple time delays, Math. Method. Appl. Sci., 45 (2022), 10052–10069. https://doi.org/10.1002/mma.8355 doi: 10.1002/mma.8355
![]() |
[18] |
F. Wang, J. Zhang, Y. Shu, X. G. Liu, Stability analysis for fractional-order neural networks with time-varying delay, Asian J. Control, 25 (2023), 1488–1498. https://doi.org/10.1002/asjc.2944 doi: 10.1002/asjc.2944
![]() |
[19] |
Z. Zhang, Z. Yang, Asymptotic stability for quaternion-valued fuzzy BAM neural networks via integral inequality approach, Chaos Soliton. Fract., 169 (2023), 113227. https://doi.org/10.1016/j.chaos.2023.113227 doi: 10.1016/j.chaos.2023.113227
![]() |
[20] |
J. Zhou, X. Ma, Z. Yan, S. Arik, Non-fragile output-feedback control for time-delay neural networks with persistent dwell time switching: A system mode and time scheduler dual-dependent design, Neural Networks, 169 (2024), 733–743. https://doi.org/10.1016/j.neunet.2023.11.007 doi: 10.1016/j.neunet.2023.11.007
![]() |
[21] |
Z. Yan, D. Zuo, T. Guo, J. Zhou, Quantized H∞ stabilization for delayed memristive neural, Neural Comput. Appl., 35 (2023), 16473–16486. https://doi.org/10.1007/s00521-023-08510-3 doi: 10.1007/s00521-023-08510-3
![]() |
[22] |
F. Zhang, Z. Zeng, Multistability of fractional-order neural networks with unbounded time-varying delays, IEEE T. Neur. Net. Lear., 32 (2020), 177–187. https://doi.org/10.1109/TNNLS.2020.2977994 doi: 10.1109/TNNLS.2020.2977994
![]() |
[23] |
F. Du, J. Lu, Improved quasi-uniform stability criterion of fractional-order neural networks with discrete and distributed delays, Asian J. Control, 25 (2023), 229–240. https://doi.org/10.1002/asjc.2758 doi: 10.1002/asjc.2758
![]() |
[24] |
R. Guo, S. Xu, J. Guo, Sliding-mode synchronization control of complex-valued inertial neural networks with Leakage delay and time-varying delays, IEEE T. Syst. Man Cy.-S., 53 (2023), 1095–1103. https://doi.org/10.1109/TSMC.2022.3193306 doi: 10.1109/TSMC.2022.3193306
![]() |
[25] |
X. Mao, X. Wang, Y. Lu, H. Qin, Synchronizations control of fractional-order multidimension-valued memristive neural networks with delays, Neurocomputing, 563 (2024), 126942. https://doi.org/10.1016/j.neucom.2023.126942 doi: 10.1016/j.neucom.2023.126942
![]() |
[26] |
L. Wang, D. Xu, Global asymptotic stability of bidirectional associative memory neural networks with S-type distributed delays, Int. J. Syst. Sci., 33 (2002), 869–877. https://doi.org/10.1080/00207720210161777 doi: 10.1080/00207720210161777
![]() |
[27] |
Z. Huang, X. Li, S. Mohamad, Z. Lu, Robust stability analysis of static neural network with S-type distributed delays, Appl. Math. Model., 33 (2009), 760–769. https://doi.org/10.1016/j.apm.2007.12.006 doi: 10.1016/j.apm.2007.12.006
![]() |
[28] |
W. Han, Y. Kao, L. Wang, Global exponential robust stability of static interval neural networks with S-type distributed delays, J. Franklin I., 348 (2011), 2072–2081. https://doi.org/10.1016/j.jfranklin.2011.05.023 doi: 10.1016/j.jfranklin.2011.05.023
![]() |
[29] |
H. Zheng, B. Wu, T. Wei, L. Wang, Y. Wang, Global exponential robust stability of high-order Hopfield neural networks with S-type distributed time delays, J. Appl. Math., 2014 (2014), 1–8. https://doi.org/10.1155/2014/705496 doi: 10.1155/2014/705496
![]() |
[30] | C. Ma, F. Zhou, Global exponential stability of high-order BAM neural networks with S-type distributed delays and reaction diffusion terms, WSEAS T. Math., 10 (2011), 333–345. |
[31] |
Y. Wang, C. Lu, G. Ji, L. Wang, Global exponential stability of high-order Hopfield-type neural networks with S-type distributed time delays, Commun. Nonlinear Sci., 16 (2011), 3319–3325. https://doi.org/10.1016/j.cnsns.2010.11.005 doi: 10.1016/j.cnsns.2010.11.005
![]() |
[32] |
Q. Yao, L. Wang, Y. Wang, Existence-uniqueness and stability of reaction-diffusion stochastic Hopfield neural networks with S-type distributed time delays, Neurocomputing, 275 (2018), 470–477. https://doi.org/10.1016/j.neucom.2017.08.060 doi: 10.1016/j.neucom.2017.08.060
![]() |
[33] |
Q. Yao, Y. F. Wang, L. S. Wang, Periodic solutions to stochastic reaction-diffusion neural networks with S-type distributed delays, IEEE Access, 7 (2019), 110905–110911. https://doi.org/10.1109/ACCESS.2019.2911962 doi: 10.1109/ACCESS.2019.2911962
![]() |
[34] | I. Podlubny, Fractional differential equations, New York: Academic Press, 1999. |
[35] |
L. Ke, Mittag-Leffler stability and asymptotic ω-periodicity of fractional-order inertial neural networks with time-delays, Neurocomputing, 465 (2021), 53–62. https://doi.org/10.1016/j.neucom.2021.08.121 doi: 10.1016/j.neucom.2021.08.121
![]() |
[36] | J. Slotine, W. Li, Applied nonlinear control, Englewood Cliffs: Prentice Hall, 1991. |
[37] |
Y. Ke, C. Miao, Stability analysis of fractional-order Cohen-Grossberg neural networks with time delay, Int. J. Comput. Math., 92 (2015), 1102–1113. https://doi.org/10.1080/00207160.2014.935734 doi: 10.1080/00207160.2014.935734
![]() |