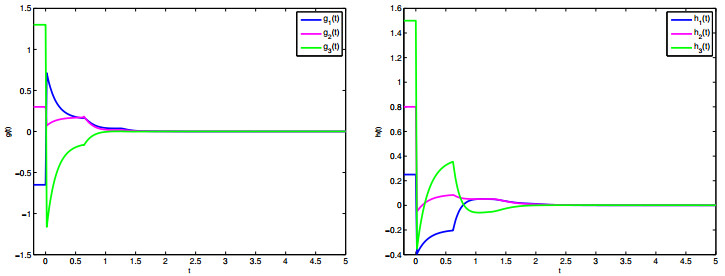
This manuscript is concerned with the stability and synchronization for fractional-order delayed gene regulatory networks (FODGRNs) via Razumikhin approach. First of all, the existence of FODGRNs are established by using homeomorphism theory, 2-norm based on the algebraic method and Cauchy Schwartz inequality. The uniqueness of this work among the existing stability results are, the global Mittag-Leffler stability of FODGRNs is explored based on the fractional-order Lyapunov Razumikhin approach. In the meanwhile, two different controllers such as linear feedback and adaptive feedback control, are designed respectively. With the assistance of fractional Razumikhin theorem and our designed controllers, we have established the global Mittag-Leffler synchronization and adaptive synchronization for addressing master-slave systems. Finally, three numerical cases are given to justify the applicability of our stability and synchronization results.
Citation: Pratap Anbalagan, Evren Hincal, Raja Ramachandran, Dumitru Baleanu, Jinde Cao, Michal Niezabitowski. A Razumikhin approach to stability and synchronization criteria for fractional order time delayed gene regulatory networks[J]. AIMS Mathematics, 2021, 6(5): 4526-4555. doi: 10.3934/math.2021268
[1] | Pratap Anbalagan, Evren Hincal, Raja Ramachandran, Dumitru Baleanu, Jinde Cao, Chuangxia Huang, Michal Niezabitowski . Delay-coupled fractional order complex Cohen-Grossberg neural networks under parameter uncertainty: Synchronization stability criteria. AIMS Mathematics, 2021, 6(3): 2844-2873. doi: 10.3934/math.2021172 |
[2] | Hongguang Fan, Jihong Zhu, Hui Wen . Comparison principle and synchronization analysis of fractional-order complex networks with parameter uncertainties and multiple time delays. AIMS Mathematics, 2022, 7(7): 12981-12999. doi: 10.3934/math.2022719 |
[3] | Rakkiet Srisuntorn, Wajaree Weera, Thongchai Botmart . Modified function projective synchronization of master-slave neural networks with mixed interval time-varying delays via intermittent feedback control. AIMS Mathematics, 2022, 7(10): 18632-18661. doi: 10.3934/math.20221025 |
[4] | Yang Xu, Zhouping Yin, Yuanzhi Wang, Qi Liu, Anwarud Din . Mittag-Leffler projective synchronization of uncertain fractional-order fuzzy complex valued neural networks with distributed and time-varying delays. AIMS Mathematics, 2024, 9(9): 25577-25602. doi: 10.3934/math.20241249 |
[5] | Tiecheng Zhang, Liyan Wang, Yuan Zhang, Jiangtao Deng . Finite-time stability for fractional-order fuzzy neural network with mixed delays and inertial terms. AIMS Mathematics, 2024, 9(7): 19176-19194. doi: 10.3934/math.2024935 |
[6] | Qike Zhang, Tao Xie, Wenxiang Fang . Fixed/predefined-time generalized synchronization for stochastic complex dynamical networks with delays. AIMS Mathematics, 2024, 9(3): 5482-5500. doi: 10.3934/math.2024266 |
[7] | Shuang Li, Xiao-mei Wang, Hong-ying Qin, Shou-ming Zhong . Synchronization criteria for neutral-type quaternion-valued neural networks with mixed delays. AIMS Mathematics, 2021, 6(8): 8044-8063. doi: 10.3934/math.2021467 |
[8] | Yinjie Qian, Lian Duan, Hui Wei . New results on finite-/fixed-time synchronization of delayed memristive neural networks with diffusion effects. AIMS Mathematics, 2022, 7(9): 16962-16974. doi: 10.3934/math.2022931 |
[9] | Saravanan Shanmugam, Mohamed Rhaima, Hamza Ghoudi . Exponential synchronization analysis for complex dynamical networks with hybrid delays and uncertainties under given control parameters. AIMS Mathematics, 2023, 8(12): 28976-29007. doi: 10.3934/math.20231484 |
[10] | Nayika Samorn, Kanit Mukdasai, Issaraporn Khonchaiyaphum . Analysis of finite-time stability in genetic regulatory networks with interval time-varying delays and leakage delay effects. AIMS Mathematics, 2024, 9(9): 25028-25048. doi: 10.3934/math.20241220 |
This manuscript is concerned with the stability and synchronization for fractional-order delayed gene regulatory networks (FODGRNs) via Razumikhin approach. First of all, the existence of FODGRNs are established by using homeomorphism theory, 2-norm based on the algebraic method and Cauchy Schwartz inequality. The uniqueness of this work among the existing stability results are, the global Mittag-Leffler stability of FODGRNs is explored based on the fractional-order Lyapunov Razumikhin approach. In the meanwhile, two different controllers such as linear feedback and adaptive feedback control, are designed respectively. With the assistance of fractional Razumikhin theorem and our designed controllers, we have established the global Mittag-Leffler synchronization and adaptive synchronization for addressing master-slave systems. Finally, three numerical cases are given to justify the applicability of our stability and synchronization results.
Fractional calculus, which is the general development of old calculus, has a remarkable ability to examine the world around us through the areas of both applied and pure mathematics, respectively. It has also gained considerable attention from the genetics, physics, chemistry, and computer research communities. The best way to make discoveries in mathematics is by adding some new theories to evaluate the current results. Frequently the results may fail. But, often it may pave a way to a new field of research works. Similarly, the fractional-order calculus is a perfect answer to the nonsensical question "what if the derivative order is non-integer?" by Leibnitz during the late sixteenth century. One of the most important properties of the differential fractional equation is its ability to track a motion of an object continuously and instantly of non-local nature. Besides, it contains more memory of the systems. The fractional-order models are easier to understand the complexity of the dynamic system with greater precision contrast to the integer-order differential models. Due to the memory properties, several researchers are integrating the memory properties into nonlinear dynamical systems, and lot of important results about fractional order nonlinear dynamical systems have been reported in recent literature, see Ref [3,11,12].
In an organism, gene expression is regulated by RNA, DNA, protein, and tiny molecules. Gene Regulatory Network (GRNs) defined the interconnections between these two. GRNs are viewed as complex networks. Each gene is regarded as a node, and the regulatory link between these genes is known as a relation between the nodes. To unleash the cure for deadly diseases such as cancer and AIDS, a greater understanding of the complex networks of GRNs is essential. Time delays in both biological and artificial neural networks are unavoidable because of the limited speed of information processing. Generally, there are typically two types of gene regulatory networks, such as the boolean model and the continuous model. The continuous model is commonly used for the study of GRNs, and several important results about GRNs with time delays had been well documented, see Ref [1,16,19].
On the other hand, stability theory is the flexible branch of science and engineering that deals with the behavioral effect for linear and nonlinear systems of dynamic structures. The investigation on various stability problems of time-delayed GRNs (TDGRNs) are accounted [20,24,26,36]. Paper by Luo et al., has analyzed the existence and Lagrange stability of TDGRNs in Lyapunov's sense based on novel algebraic method and stability theory [20]. In [24], the authors demonstrated the delay-dependent finite time stability issues of TDGRNs with impulses based on the LMI approach and Lyapunov stability theory. In [26], the problem of the stability criterion of TDGRNs with impulsive effects was analyzed. By employing the LMI techniques, convex combination approach, and Lyapunov-Krasovskii functional, the sufficient conditions to assure the global asymptotic stability analysis of the proposed TDGRNs model. In [36], the authors researched the global exponential delay-dependent stability criterion of TDGRNs under distributed delays based on LMI techniques and Lyapunov-Krasovskii functional approach.
Nowadays, the synchronization of dynamic systems is advancing as a dominant research field and has drawn a great deal of interest from researchers of diverse field. Its application found in many fields like secure communication, image, and signaling process. Many types of synchronization results are available in recent works including Mittag-Leffler, asymptotic, quasi and pinning synchronization, and so forth [7,8,9,10,17,18,30,33]. GRN synchronization is essential for knowing the synergic behavior between the more than one gene networks through the connections of gene signals and their products. The advantages of researching GRN synchronization are acquiring knowledge about a gene's internal processes even at cellular levels. Some significant results about synchronization for time-delayed GRNs (TDGRNs) had been studied in recent years. For example, by means of observer-based non-fragile and linear feedback control, LMI techniques, and Lyapunov-Krasovskii method, Ali et al. demonstrate some sufficient criteria for the global asymptotic synchronization issues of TDGRNs under uncertainty [2]. By exploiting finite-time control techniques, robust analysis, and theory of finite-time stability, Jiang et al. investigate stochastic synchronization in finite time analysis for TDGRNs under parameter uncertainties [14]. Depending on the pinning control strategy, some famous inequality approaches, matrix theory, and event-triggered condition, Yue et al.analyze the cluster synchronization analysis for GRNs with coupling terms [35]. The research works in fractional order gene regulatory with delay arguments has been undergone exciting development in recent years, and some meaningful scientific results had been obtained. By using a hybrid control approach, the authors experimented with the bifurcation analysis for FODGRNs [15]. By utilizing the principle of Banach contraction mapping and absolute Lyapunov functional with 1-norm, the authors exhibited the several stability criteria of fractional order GRNs [25]. Depending on the principle of Banach contraction, Lyapunov functional with 1-norm, linear feedback, and adaptive feedback techniques, the sufficient criteria to ensure the finite time delay-independent synchronization problem of considered FODGRNs via Razumikhin approach [23]. By employing the diffusion and stability theory, the authors have demonstrated with the issues of local stability and instability criteria for bifurcation diffusion FODGRNs [29]. Unfortunately, there is no work done on the existence, stability, and synchronization for FODGRNs via Razumikhin approach and quadratic Lyapunov approach, this situation motivates further discussion for global Mittag-Leffler stability and adaptive synchronization of FODGRNs. The essential theme of this manuscript lies in the following aspects:
1) By means of homeomorphism theory and Cauchy Schwartz inequality, a sufficient condition is presented to ascertain the existence and uniqueness of the equilibrium point for FODGRNs.
2) Based on fractional Lyapunov method, fractional-order Razumikhin theorem, and some traditional inequality techniques, a sufficient condition is established for global Mittag-Leffler stability of the proposed networks.
3) According to feedback control technique, two kinds feedback controllers are designed to guarantee the synchronization of a class of master-slave fractional order time delayed gene regulatory networks. One is linear feedback control, which is better and simpler to execute over the other controls. Another one is adaptive feedback control, which is designed to prevent the high feedback gains and it is regarded as the more versatile one. Since, it can adjust the coupling weights by itself.
4) The proposed results in this paper are still true for global exponential stability and synchronization of integer-order GRNs with time delay effects, and these results do not discuss in the previous works of literature.
The scheme of this paper is as planned out as follows. We present the key concepts about the calculus of fractional order, essential lemmas, and the system description in Section 2. In Section 3 and Section 4, we present the main results of this manuscript. In Section 5, we include the numerical results and its simulations. Lastly, we draw some conclusions in Section 6.
Notations: The required notations are displayed as follows: Rm refers to the space of m-dimensional space. A set of all m×m real matrix is described by Rm×m. sign(⋅) indicate the signum function. Eλ,μ and Eλ,1 refers to the two parameter and one parameter Mittag-Leffler functions, respectively. For any matrix B=(bpq)m×m, |B|=(|bpq|)m×m. The greatest and smallest eigenvalues of matrix B is represented by ΦM and Φm, respectively. The symmetric term in a matrix is displayed by ✠. The operator norm of a matrix B is denoted by ‖B‖=√ΦM(BTB). Γ(⋅) is the gamma function. C([−τ,0],Rm) indicate the group of continuous functions from [−τ,0] to Rm, where time lag τ>0 and the signum function is referred by sign(⋅).
This section comprises of the rudimentary fractional-order definitions, lemmas which are further employed in the subsequent section.
Definition 2.1 [22] The λ−th fractional order for integral function ℓ(t) is denoted as:
C0D−λtℓ(t)=1Γ(λ)∫tt0(t−θ)λ−1ℓ(θ)dθ. |
Definition 2.2 [22] The λ−th Caputo type fractional order for a function ℓ(t) is denoted as:
C0Dλtℓ(t)=1Γ(m−λ)∫tt0ℓ(m)(θ)(t−θ)λ−m+1dθ, |
where t≥t0 and m−1<λ<m∈Z+.
Lemma 2.3 [13] For 0<λ<1, ℓ(t)∈Rm be a continuously vector valued differentiable function, then for any t≥t0
C0Dλt{ℓT(t)Xℓ(t)}≤2ℓT(t)X{Dλtℓ(t)}, |
where X∈Rm×m is a positive definite symmetric matrix.
Lemma 2.4 [22] If ℓ(t)∈Cm([0,+∞),R), then
C0D−λt(C0Dλtℓ(t))=ℓ(t)−m−1∑x=0txx!ℓ(x)(t0). |
where m−1<λ<m,(m∈Z+,m≥1).
If 0<λ<1, then C0D−λt(C0Dλtℓ(t))=ℓ(t)−ℓ(t0).
Lemma 2.5 [31] For 0<λ<1, if ℓ(t) is continuously derivable function on [0,+∞), then there exist a constants ϑ1>0 and ϑ2>0 such that
C0Dλtℓ(t)≤−ϑ1ℓ(t)+ϑ2,t≥t0, |
then
ℓ(t)≤ℓ(t0)Eλ(−ϑ1(t−t0)λ)+ϑ2tλEλ,λ+1(−ϑ1(t−t0)λ),t≥t0. |
Lemma 2.6 [34] For 0<λ<1, a nondecreasing derivable function ℓ(t) is defined on positive, then there exist a constant ϑ>0 such that
C0Dλt[ℓ(t)−ϑ]2≤2[ℓ(t)−ϑ]C0Dλtℓ(t). |
Lemma 2.7 [37] For 0<λ<1, ℓ(t) be a continuously vector valued differentiable function, then
C0Dλt|ℓ(t)|≤sgn(ℓ(t))C0Dλtℓ(t). |
We consider a class of Caputo-sense FODGRNs in this manuscript as follows:
{C0Dλtgp(t)=−apgp(t)+∑mq=1bpqfq(hq(t−σ1))+Fp,C0Dλthp(t)=−cphp(t)+dpgp(t−σ2), | (2.1) |
where p=1,2,..,m, 0<λ<1 signifies the fractional order, gp(t)∈Rm and hp(t)∈Rm indicate the concentrations of mRNA and protein of pth node at time t, respectively. ap and cp are degradation velocities of mRNA and protein molecule, respectively. Moreover, dp represents the translation rate. The time lags are denoted as σ1>0 and σ2>0. bpq is coupling matrix. Besides, the functions fq(⋅) represents the nonlinear protein feedback regulation, which are commonly indicated in the Hill form as
fq(ν)=(νςq)Hq[1+(νςq)Hq], |
where Hq is the Hill coefficients and αy signifies non-negative constants. The coupling matrix of the network B=(bpq)m×m∈Rm×m are represents as follows:
bpq={−φpq,qis a repressor of genep0,qdoes not regulate genepφpq,qis a initiator of genep. |
Presently, we define Gp as Gp=∑y∈ˆGbpq, where ˆG indicate the set of all repressor of gene p. It's significant to mention that Caputo's definition was the most celebrated definition due to its properties such as derivative of constant is zero. Further, the Cauchy problem defined in the sense of Caputo's definition has an interpretation of integer-order initial values. Therefore, the initial values combined with FODGRNs (2.1) in the sense of Caputo type can be described as:
gp(t)=ωp(t),hp(t)=ϖp(t),t∈[−τ=max{σ1,σ2},0], |
where ωp(t),ϖp(t)∈C([−τ,0],Rm) and its norm is defined by
‖ϖ‖=m∑p=1sup−σ1≤θ≤0{|ϖp(θ)|},‖ω‖=m∑p=1sup−σ2≤θ≤0{|ωp(θ)|}. |
The vector form of FODGRNs (2.1) is given as
{C0Dλtg(t)=−Ag(t)+Bf(h(t−σ1))+FC0Dλth(t)=−Ch(t)+Dg(t−σ2), | (2.2) |
where g(t)=(g1(t),...,gm(t))T, h(t)=(h1(t),..,hm(t))T, A=diag{a1,...,am}, B=(bpq)m×m, C=diag{c1,...,cm}, D=diag{d1,...,dm}, f(h(t))=(f1(h1(t),...,fm(hm(t))T and F=(F1,...,Fm)T.
In the development of main results, the following Assumption and Lemma's are important.
Assumption1. The feedback function fq(⋅) is monotonically increasing, it is fulfilled that
0≤fq(v)−fq(w)v−w≤βq,q=1,2,…,m, |
for all v,w∈R with v≠w.
Lemma 2.8 [5] A uniformly continuous function ℓ(t) is defined on positive interval and ∫tt0ℓ(θ)dθ exists and is bounded, then limt→+∞ℓ(t)=0.
Lemma 2.9 [6] For any v,w∈Rm and R∈Rm×m is a positive definite matrix, then
vTw≤12vTRv+12wTR−1w. |
Lemma 2.10 [21] If a continuous map Υ:Rm→Rm holds the following conditions:
(1). Υ(g) is injective on Rm, that is Υ(g)≠Υ(h)∀g≠h.
(2). ‖Υ(g)‖→+∞ as ‖g‖→+∞.
Then, Υ(g) is homeomorphism of Rm.
In this part, we will derive the existence and stability of FODGRNs (2.1) by using the following Definitions.
Definition 3.1 The vectors g⋆=(g⋆1,...,g⋆m)T and h⋆=(h⋆1,...,h⋆m)T is an equilibrium points of FODGRNs (2.1), if and only if
{−apg⋆p+∑mq=1bpqfq(h⋆q)+Fp=0,−cph⋆p+dpg⋆p=0,p=1,2,..m. |
Definition 3.2 The equilibrium point of FODGRNs (2.1) is said to be global Mittag-Leffler stable, if there exists two positive constants ζ1>0 and ζ2>0 such that for any solution (g(t)−g⋆,h(t)−h⋆) of FODGRNs (2.1) with initial conditions (ω(t)−g⋆,ϖ(t)−h⋆) such that
m∑p=1(gp(t)−g⋆p)2+m∑p=1(hp(t)−h⋆p)2≤{N(ω(t)−g⋆,ϖ(t)−h⋆)Eλ,1(ζ1(t−t0)λ)}ζ2, |
for t≥t0, where t0 is starting time, N(0,0)=0, N(ω,ϖ)≥0, and N(ω,ϖ) refers to locally Lipschitz with respect to ϖ∈C([−σ1,0],Rm) and ω∈C([−σ2,0],Rm).
Theorem 3.3 Under Assumption 1, the existence of equilibrium point (g⋆,h⋆) of FODGRNs (2.1) is unique, where g⋆=(g⋆1,...,g⋆m)T and h⋆=(h⋆1,...,h⋆m)T if there exist positive constants αp>0 for p=1,2,..m such that the condition is established:
ξp=2apcpdp−m∑q=1|bpq|βq−m∑q=1αqαp|bqp|βp>0,p=1,2,..,m. | (3.1) |
Proof. According to Definition 3.1, it easy to obtain
g⋆p=cpdph⋆p,p=1,2,..,m, |
which prove that if the existence of equilibrium point (g⋆p,h⋆p) of FODGRNs (2.1) is unique, so we only to establish the existence of unique equilibrium point h⋆p.
Define Υ(h)=(Υ1(h),...,Υm(h))T, h=(h1,...,hm)T∈Rm, where
Υp(hp)=−apcpdph⋆p+m∑q=1bpqfq(h⋆q)+Fp,p=1,2,..,m. | (3.2) |
In the following, we will demonstrate that Υ(h) is homeomorphism of Rm onto itself based on Lemma 2.10. That is (i). If hp≠kp, then Υp(hp)≠Υp(kp),p=1,2,..,m. (ii). ‖Υ(h)‖→+∞ as ‖h‖→+∞.
Firstly, we show (ⅰ). If there exist hp,kp∈Rm holds hp≠kp such that Υp(hp)≠Υp(kp) for p=1,2,..,m. Then,
apcpdp(hp)−apcpdp(kp)=m∑q=1bpq[fq(hq)−fq(kq)] |
According to Assumption 1, it follows that
apcpdp|hp−kp|≤m∑q=1|bpq|βq|hq−kq|. | (3.3) |
From (3.3), we have
m∑p=12αpapcpdp|hp−kp|2=m∑p=12αp|hp−kp|(apcpdp|hp−kp|)≤m∑p=12αp|hp−kp|(m∑q=1|bpq|βq|hq−kq|)≤m∑p=1m∑q=12αp|bpq|βq|hp−kp||hq−kq|≤m∑p=1m∑q=1αp|bpq|βq(|hp−kp|2+|hq−kq|2)=m∑p=1m∑q=1[αp|bpq|βq+αq|bqp|βp]|hp−kp|2. | (3.4) |
Together with (3.1) and (3.4), we sustain
m∑p=1ξp|hp−kp|2≤0. | (3.5) |
Based on inequality (3.2) and (3.5), it follows that |hp−kp|=0, which leads to a contradiction with our assumption.
Next, we show (ⅱ). From (3.2), we get
∑mp=12αp(Υp(hp)−Υp(0))sign(hp)|hp|
≤−m∑p=12αpapcpdp|hp|2+m∑p=1m∑q=12αp|bpq|βq|hq||hp|≤m∑p=1[−2apcpdp+m∑q=1|bpq|βq+m∑q=1αqαp|bqp|βp]|hp|2≤−ξmin‖h‖2, |
where ξmin=min1≤p≤m{ξp}. Then, we have
‖h‖2≤−2ξminm∑p=1αp(Υp(hp)−Υp(0))sign(hp)|hp|≤2αMξminm∑p=1|Υp(hp)−Υp(0)||hp|, | (3.6) |
αM=max1≤p≤m{αp}. By means of famous Cauchy-Schwartz inequality and the above inequality (3.6), we obtain
‖h‖2≤2αMξmin(m∑p=1|Υp(hp)−Υp(0)|2)12(m∑p=1|hp|2)12, |
which implies to
‖h‖≤2αMξmin‖Υ(h)−Υ(0)‖≤2αMξmin(‖Υ(h‖+‖Υ(0)‖). |
Based on above discussions, we see that ‖Υ(h)‖→+∞ as ‖h‖→+∞. In view of Lemma 2.10, Υ(h) is homeomorphism on Rm, which indicates, the existence of equilibrium point (g⋆p,h⋆p) of FODGRNs (2.1) is unique, and the proof of Theorem 3.3 is ended.
Remark 3.4 There exist other methods to obtain the existence of equilibrium, such as Schauder's fixed point theorem, Banach fixed point theorem, Browner's fixed point theorem and Krasnoselskii fixed point theorem, and Homotopy invariance theorem. In [23,25], based on the theory of fractional calculus, the contraction mapping principle and the norm-1 properties, the existence and uniqueness of the equilibrium point of the fractional order genetic regulatory networks is discussed. Different from above mentioned references [23,25], we have discussed the existence and uniqueness by homeomorphism theory.
Transform (g⋆p,h⋆p) of FODGRNs (2.1) to origin via the transformation vp(t)=gp(t)−g⋆p and wp(t)=hp(t)−h⋆p for p=1,2,..,m. Then, the FODGRNs error system is:
{C0Dλtvp(t)=−apvp(t)+∑mq=1bpq˜fq(wq(t−σ1))C0Dλtwp(t)=−cpwp(t)+dpvp(t−σ2), | (3.7) |
for p=1,2,..,m and ˜fq(wq(t−σ1))=(fq(wq(t−σ1)+h⋆q)−h⋆q). The vector form of FODGRNs (3.7) is given as
{C0Dλtv(t)=−Av(t)+B˜f(w(t−σ1))C0Dλtw(t)=−Cw(t)+Dv(t−σ2), | (3.8) |
where v(t)=(v1(t),...,vm(t))T, w(t)=(w1(t),..,wm(t))T, ˜f(w(t))=(˜f1(w1(t),...,˜fm(wm(t))T.
Theorem 3.5 Under Assumption 1, the existence of equilibrium point (g⋆,h⋆) of FODGRNs (2.1) is globally Mittag-Leffler stable if there exist two positive diagonal matrices X∈Rm×m and Y∈Rm×m such that
ΦM(X)(−2‖A‖+‖B‖‖S‖ΦM(X)+Φm(Y)Φm(Y)+ΦM(Y)Φm(X)‖D‖)<0, | (3.9) |
ΦM(Y)(−2‖C‖+‖D‖Φm(X)+ΦM(Y)Φm(X)+ΦM(X)Φm(Y)‖B‖‖S‖)<0, | (3.10) |
where S=diag{β1,...,βm}.
Proof. According to conditions (3.9) and (3.10) from Theorem 3.5 that there exist two positive scalars γ1>0 and γ2>0 such that
ΦM(X)(−2‖A‖+‖B‖‖S‖ΦM(X)+Φm(Y)Φm(Y)+ΦM(Y)Φm(X)‖D‖)<−γ1, | (3.11) |
ΦM(Y)(−2‖C‖+‖D‖Φm(X)+ΦM(Y)Φm(X)+ΦM(X)Φm(Y)‖B‖‖S‖)<−γ2. | (3.12) |
Consider the following subsequent Lyapunov-Razumikhin functional:
H(v(t),w(t))=vT(t)Xv(t)+wT(t)Yw(t) | (3.13) |
Noting that
Φm(X)‖v(t)‖2+Φm(Y)‖w(t)‖2≤H(v(t),w(t))≤ΦM(X)‖v(t)‖2+ΦM(Y)‖w(t)‖2. | (3.14) |
Then, based on Lemma 2.3, and the Caputo-derivative of H(v(t),w(t)) with respect to FODGRNs error system (3.7), we have
C0DλtH(v(t),w(t))≤2ΦM(X)‖v(t)‖C0Dλt‖v(t)‖+2ΦM(Y)‖w(t)‖C0Dλt‖w(t)‖ |
From Assumption 1 and Lemma 2.7, we sustain
C0DλtH(v(t),w(t))≤2ΦM(X)‖v(t)‖sign(v(t))C0Dλt{v(t)}+2ΦM(Y)‖w(t)‖sign(w(t))C0Dλt{w(t)}≤2ΦM(X)‖v(t)‖sign(v(t)){−Av(t)+BSw(t−σ1)}+2ΦM(Y)‖w(t)‖sign(w(t)){−Cw(t)+Dv(t−σ2)}≤−2ΦM(X)‖A‖‖v(t)‖2−2ΦM(Y)‖C‖‖w(t)‖2+2ΦM(X)‖B‖‖S‖‖v(t)‖‖w(t−σ1)‖+2ΦM(Y)‖D‖‖w(t)‖‖v(t−σ2)‖≤−2ΦM(X)‖A‖‖v(t)‖2−2ΦM(Y)‖C‖‖w(t)‖2+2ΦM(X)‖B‖‖S‖supt−σ1≤θ≤t{‖v(t)‖‖w(θ)‖}+2ΦM(Y)‖D‖supt−σ2≤θ≤t{‖w(t)‖‖v(θ)‖}. |
By using famous inequality 2|μ1||μ2|≤μ21+μ22, for supt−σ1≤θ≤t{‖v(t)‖‖w(θ)‖} and supt−σ2≤θ≤t{‖w(t)‖‖v(θ)‖}, we sustain
C0DλtH(v(t),w(t))≤−2ΦM(X)‖A‖‖v(t)‖2−2ΦM(Y)‖C‖‖w(t)‖2+ΦM(X)‖B‖‖S‖[‖v(t)‖2+supt−σ1≤θ≤t‖w(θ)‖2]+ΦM(Y)‖D‖[supt−σ2≤θ≤t‖v(θ)‖2+‖w(t)‖2]. | (3.15) |
For any function v(t) and w(t) that hold the following Razumikhin criteria, see Ref [27,32]
H(v(θ),w(θ))≤H(v(t),w(t)),t−τ≤θ≤t, |
we get
Φm(X)‖v(θ)‖2+Φm(Y)‖w(θ)‖2≤vT(θ)Xv(θ)+wT(θ)Yw(θ)≤vT(t)Xv(t)+wT(t)Yw(t)≤ΦM(X)‖v(t)‖2+ΦM(Y)‖w(t)‖2, |
and hence
‖v(θ)‖2≤ΦM(X)‖v(t)‖2+ΦM(Y)‖w(t)‖2Φm(X) | (3.16) |
‖w(θ)‖2≤ΦM(X)‖v(t)‖2+ΦM(Y)‖w(t)‖2Φm(Y) | (3.17) |
for t−τ≤θ≤t. From (3.15)-(3.17), one can obtain
C0DλtH(v(t),w(t))≤−2ΦM(X)‖A‖‖v(t)‖2−2ΦM(Y)‖C‖‖w(t)‖2+ΦM(X)‖B‖‖S‖[‖v(t)‖2+ΦM(X)‖v(t)‖2+ΦM(Y)‖w(t)‖2Φm(Y)]+ΦM(Y)‖D‖[ΦM(X)‖v(t)‖2+ΦM(Y)‖w(t)‖2Φm(X)+‖w(t)‖2]≤ΦM(X)(−2‖A‖+‖B‖‖S‖ΦM(X)+Φm(Y)Φm(Y)+ΦM(Y)Φm(X)‖D‖)×‖v(t)‖2+ΦM(Y)(−2‖C‖+‖D‖Φm(X)+ΦM(Y)Φm(X)+ΦM(X)Φm(Y)‖B‖‖S‖)‖w(t)‖2≤−γ1‖v(t)‖2−γ2‖w(t)‖2≤−γm(‖v(t)‖2+‖w(t)‖2) | (3.18) |
where γm=min{γ1,γ2}. According to inequality (3.14), we get
C0DλtH(v(t),w(t))≤−γmδM(‖v(t)‖2+‖w(t)‖2) | (3.19) |
where δM=max{ΦM(X),ΦM(Y)}. According to inequality (3.19) and based on Lemma 2.5, we have
H(v(t),w(t))≤sup−τ≤θ≤0{H(ω(θ)−g⋆,ϖ(θ)−h⋆)}×Eλ,1(−γmδM(t−t0)λ)∀t∈[t0,+∞). |
Then, by using inequality (3.14), we get
δm(‖v(t)‖2+‖w(t)‖2)≤H(v(t),w(t))≤sup−τ≤θ≤0{H(ω(θ)−g⋆,ϖ(θ)−h⋆)}Eλ,1(−γmδM(t−t0)λ)≤δM[m∑p=1sup−σ2≤θ≤0(ωp(θ)−g⋆p)2+m∑p=1sup−σ1≤θ≤0(ϖp(θ)−h⋆p)2]×Eλ,1(−γmδM(t−t0)λ) |
where δm=min{Φm(X),Φm(Y)}. Let
N(ω−g⋆,ϖ−h⋆)=δMδm[m∑p=1sup−σ2≤θ≤0(ωp(θ)−g⋆p)2+m∑p=1sup−σ1≤θ≤0(ϖp(θ)−h⋆p)2], |
then
‖v(t)‖2+‖w(t)‖2≤N(ω−g⋆,ϖ−h⋆)Eλ,1(−γmδM(t−t0)λ)∀t≥t0, |
where N≥0 and N=0 satisfy only if ω(θ)=g⋆ for −σ2≤θ≤0 and ϖ(θ)=h⋆ for −σ1≤θ≤0, respectively. Therefore, based on Definition 3.2, the existence of equilibrium point (g⋆,h⋆) of FODGRNs (2.1) is globally Mittag-Leffler stable, and the proof of Theorem 3.5 is ended.
If σ1=σ2=0, then system (2.1) becomes the following form:
{C0Dλtgp(t)=−apgp(t)+m∑q=1bpqfq(hq(t))+Fp,C0Dλthp(t)=−cphp(t)+dpgp(t),p=1,2..,m. | (3.20) |
Corollary 3.6 Under Assumption 1, the existence of equilibrium point (g⋆,h⋆) of (3.20) is globally Mittag-Leffler stable if there exist two positive diagonal matrices X∈Rm×m and Y∈Rm×m such that
ΦM(X)(−2‖A‖+‖B‖‖S‖+ΦM(Y)ΦM(X)‖D‖)<0ΦM(Y)(−2‖C‖+‖D‖+ΦM(X)ΦM(Y)‖B‖‖S‖)<0. |
Remark 3.7 This study is a first attempt on the global Mittag-Leffler stability criterion FODGRNs. This analysis takes into account for feedback regulation time delay σ1 and translation time delay σ2. The main difficulties of this study is how to deal with time-delay terms. To overcome this difficulty, we adopt Razumikhin condition. In [25,28], many sufficient criteria guaranteeing the global Mittag-Leffler stability of FODGRNs are obtained in terms of algebraic inequalities. Compared with other researches by employing algebraic inequalities method to obtain the global Mittag-Leffler stability criteria in [25,28], our results, in terms of norm matrices, are very easy to verified with help of MATLAB toolbox in practice.
FODGRNs (2.1) acts as the master system and the slave system is
{C0Dλtup(t)=−apup(t)+m∑q=1bpqfq(zq(t−σ1))+Fp+xp(t),C0Dλtzp(t)=−cpzp(t)+dpup(t−σ2)+yp(t), | (4.1) |
where p=1,2,..,m, up(t)∈Rm and vp(t)∈Rm indicate the concentrations of mRNA and protein of p-node at time t, respectively. xp(t) and yp(t) are suitable controller and all others are same as one of system (2.1). The initial values of FODGRNs (4.1) can be described as:
up(t)=˜ωp(t),zp(t)=˜ϖp(t),t∈[−τ=max{σ1,σ2},0], |
where ˜ωp(t),˜ϖp(t)∈C([−τ,0],Rm) and its norm is defined by
‖˜ϖ‖=m∑p=1sup−σ1≤θ≤0{|˜ϖp(θ)|},‖˜ω‖=m∑p=1sup−σ2≤θ≤0{|˜ωp(θ)|}. |
Two types control like linear feedback control and adaptive feedback control, respectively, are designed as follows:
{xp(t)=−kp(up(t)−gp(t))yp(t)=−lp(zp(t)−hp(t)) | (4.2) |
and
{xp(t)=−ξp(t)(up(t)−gp(t))yp(t)=−ηp(t)(zp(t)−hp(t)) | (4.3) |
with adaptive rule
{C0Dλtξp(t)=˜kp|up(t)−gp(t)|2C0Dλtηp(t)=˜lp|zp(t)−hp(t)|2 |
for p=1,2,..,m, kp>0,lp>0,˜kp>0,˜lp>0 are suitable constants, ξp(t) and ηp(t) are adaptive coupling weights.
Let vp(t)=up(t)−gp(t) and wp(t)=zp(t)−hp(t), then based on (2.1) and (4.1), the synchronization error system is described by
{C0Dλtvp(t)=−apvp(t)+m∑q=1bpq˜fq(wq(t−σ1))+xp(t),C0Dλtwp(t)=−cpwp(t)+dpvp(t−σ2)+yp(t), | (4.4) |
where p=1,2,..,m and ˜fq(wq(t−σ1))=fq(zq(t−σ1))−fq(hq(t−σ1)).
In the development of synchronization criteria, the following Definition's are significant.
Definition 4.1 Master-slave FODGRNs systems (2.1) and (4.1) are said to realize global Mittag-Leffler synchronization under linear feedback control (4.2), if there exists two positive constants ζ1>0 and ζ2>0 such that for any solution (u(t)−g(t),z(t)−h(t)) of FODGRNs systems (2.1) and (4.1) with initial conditions (˜ω(t)−ω(t),˜ϖ(t)−ϖ(t)) such that
∑mp=1(up(t)−gp(t))2+∑mp=1(zp(t)−hp(t))2≤{N(˜ω(t)−ω(t),˜ϖ(t)−ϖ(t))Eλ,1(ζ1(t−t0)λ)}ζ2 |
for t≥t0, where t0 is starting time, N(0,0)=0, N(˜ω−ω,˜ϖ−ϖ)≥0, and N(˜ω−ω,˜ϖ−ϖ) refers to locally Lipschitz with respect to ˜ϖ,ϖ∈C([−σ1,0],Rm) and ˜ω,ω∈C([−σ2,0],Rm).
Definition 4.2 Master-slave FODGRNs systems (2.1) and (4.1) are said to realize global asymptotical synchronization under adaptive feedback control (4.3), if
limt→+∞m∑p=1(up(t)−gp(t))2+limt→+∞m∑p=1(zp(t)−hp(t))2=0. |
Remark 4.3 Global synchronization in the sense of Mittag-Leffler leads to global asymptotic synchronization.
Theorem 4.4 Under Assumption 1, master-slave FODGRNs systems (2.1) and (4.1) realize globally Mittag-Leffler synchronization under linear feedback control (4.2) if there exist two positive diagonal matrices X∈Rm×m and Y∈Rm×m such that
ΦM(X)(−2‖A‖−2‖K‖+‖B‖‖S‖ΦM(X)+Φm(Y)Φm(Y)+ΦM(Y)Φm(X)‖D‖)<0,ΦM(Y)(−2‖C‖−2‖L‖+‖D‖Φm(X)+ΦM(Y)Φm(X)+ΦM(X)Φm(Y)‖B‖‖S‖)<0, |
where S=diag{β1,...,βm}, K=diag{k1,...,km} and L=diag{l1,...,lm}.
Proof. The proof of Theorem 4.4 is similar to the proof Theorem 3.5. Hence the proof of the above Theorem 4.4 is skipped.
Corollary 4.5 Under Assumption 1, master-slave FODGRNs systems (2.1) and (4.1) with σ1=σ2=0 realize globally Mittag-Leffler synchronization under linear feedback control (4.2) if there exist two positive diagonal matrices X∈Rm×m and Y∈Rm×m such that
ΦM(X)(−2‖A‖−2‖K‖+‖B‖‖S‖+ΦM(Y)ΦM(X)‖D‖)<0ΦM(Y)(−2‖C‖−2‖L‖+‖D‖+ΦM(X)ΦM(Y)‖B‖‖S‖)<0. |
Theorem 4.6 Under Assumption 1, master-slave FODGRNs systems (2.1) and (4.1) realize global asymptotic synchronization under adaptive feedback control (4.3) if there exist positive diagonal matrices X>0,Y>0,R1>0 and R2>0 such that the following LMIs are holds:
(ⅰ). There exist two constants ζ1>0 and ζ2>0 such that
[−2XA−2X˜K+ζ1XXB✠−R1]<0,[−2YC−2Y˜L+ζ2YY✠−R2]<0. |
(ⅱ). There exist two constants ζ3>0 and ζ4>0 such that
R1S2−ζ3Y<0,R2D2−ζ4X<0. |
(ⅲ). For some ς>1 and ϱ>1 such that
Ω>ςζ3+ϱζ4, |
where Ω=min{ζ1,ζ2}, S=diag{β1,...,βm}, X=diag{x1,...,xm}, Y=diag{y1,...,ym}, ˜K=diag{˜k⋆1,...,˜k⋆m} and ˜L=diag{˜l⋆1,...,˜l⋆m}.
Proof. Consider the following subsequent Lyapunov-Razumikhin functional:
H(v(t),w(t))=|v(t)|TX|v(t)|+|w(t)|TY|w(t)|⏟H1(v(t),w(t))+m∑p=1xp˜kp[ξp(t)−˜k⋆p]2+m∑p=1yp˜lp[ηp(t)−˜l⋆p]2⏟H2(v(t),w(t)) | (4.5) |
where ˜k⋆p and ˜l⋆p holds the LMI of condition (i) in Theorem 4.6. Based on Lemma 2.3, Lemma 2.6, Lemma 2.9 and Assumption 1, we obtain
C0DλtH(v(t),w(t))≤2|v(t)|TXC0Dλt|v(t)|+2|w(t)|TYC0Dλt|w(t)|+m∑p=12xp˜kp[ξp(t)−˜k⋆p]C0Dλtξp(t)+m∑p=12yp˜lp[ηp(t)−˜l⋆p]C0Dλtηp(t)≤2m∑p=1xp|vp(t)|C0Dλt|vp(t)|+2m∑p=1yp|wp(t)|C0Dλt|wp(t)|+m∑p=12xp˜kp[ξp(t)−˜k⋆p]{˜kp|vp(t)|2}+m∑p=12yp˜lp[ηp(t)−˜l⋆p]{˜lp|wp(t)|2}≤2m∑p=1xp|vp(t)|sign{−apvp(t)+m∑q=1bpq˜fq(wq(t−σ1))−ξp(t)vp(t)}+2m∑p=1yp|wp(t)|sign{−cpwp(t)+dpvp(t−σ2)−ηp(t)wp(t)}+2m∑p=1xp[ξp(t)−˜k⋆p]|vp(t)|2+2m∑p=1yp[ηp(t)−˜l⋆p]|wp(t)|2≤−2m∑p=1|vp(t)|(xp(ap+k⋆p))|vp(t)|−2m∑p=1|wp(t)|(yp(cp+l⋆p))|wp(t)|+2m∑p=1m∑q=1|vp(t)|xp|bpq|βq|wq(t−σ1)|+2m∑p=1|wp(t)|ypdp|vp(t−σ2)|=−2|v(t)|TX(A+˜K)|v(t)|−2|w(t)|TY(C+˜L)|w(t)|+2|v(t)|TXBS|w(t−σ1)|+2|w(t)|TYD|v(t−σ2)|≤−2|v(t)|TX(A+˜K)|v(t)|−2|w(t)|TY(C+˜L)|w(t)|+|v(t)|TXBR−11BTXT|v(t)|+|w(t−σ1)|TR1S2|w(t−σ1)|+|w(t)|TYR−12YT|w(t)|+|v(t−σ2)|TR2D2|v(t−σ2)|=|v(t)|T[−2XA−2X˜K+XBR−11BTXT+ζ1X]|v(t)|+|w(t)|T[−2YC−2Y˜L+YR−12YT+ζ2Y]|w(t)|−ζ1|v(t)|TX|v(t)|+|w(t−σ1)|T[R1S2−ζ3Y]|w(t−σ1)|−ζ2|w(t)|TY|w(t)|+|v(t−σ2)|T[R2D2−ζ4X]|v(t−σ2)|+ζ3|w(t−σ1)|TY|w(t−σ1)|+ζ4|v(t−σ2)|TX|v(t−σ2)| | (4.6) |
Combined with condition (i) and (ii) from Theorem 4.6 of (4.6) and Razumikhin theorem for fractional systems, see Ref [4] that
C0DλtH(v(t),w(t))≤−ΩH1(v(t),w(t))+ζ3H1(v(t−σ1),w(t−σ1))+ζ4H1(v(t−σ2),w(t−σ2))≤−[Ω−ζζ3−ρζ3]H1(v(t),w(t))=−γH1(v(t),w(t)), | (4.7) |
where γ=Ω−ζζ3−ρζ3>0. Taking integer order integration of (4.7) on both sides, we have
−γ∫tt0H1(v(θ),w(θ))dθ≥∫tt0C0DλθH(v(ℓ),w(ℓ))dℓdθ=1Γ(1−λ)∫tt0∫θt0(θ−ℓ)−λH′(v(ℓ),w(ℓ))dℓdθ=1Γ(1−λ)∫tt0∫tℓ(θ−ℓ)−λH′(v(ℓ),w(ℓ))dθdℓ=1Γ(2−λ)∫tt0(t−ℓ)1−λH′(v(ℓ),w(ℓ))dℓ=1Γ(1−λ)∫tt0(t−ℓ)−λH(v(ℓ),w(ℓ))dℓ−1Γ(2−λ)(t−t0)1−λH(v(t0),w(t0))≥−1Γ(2−λ)(t−t0)1−λH(v(t0),w(t0)) |
which leads to
∫tt0H1(v(θ),w(θ))dθ≤H(v(t0),w(t0))γΓ(2−λ)(t−t0)1−λ. | (4.8) |
Noting that
δm(‖v(t)‖2+‖w(t)‖2)≤H1(v(t),w(t))≤δM(‖v(t)‖2+‖w(t)‖2), | (4.9) |
where δm=min{Φm(X),Φm(Y)} and δM=max{ΦM(X),ΦM(Y)}. From (4.8) and (4.9), we sustain
∫tt0(‖v(θ)‖2+‖w(θ)‖2)dθ≤H(v(t0),w(t0))δmγΓ(2−λ)(t−t0)1−λ |
hence
limt→+∞∫tt0(‖v(θ)‖2+‖w(θ)‖2)dθ(t−t0)1−λ≤H(v(t0),w(t0))δmγΓ(2−λ). |
If limt→+∞∫tt0(‖v(θ)‖2+‖w(θ)‖2)dθ<+∞, then
limt→+∞(‖v(t)‖2+‖w(t)‖2)=0, |
where Lemma 2.8 has been used.
If limt→+∞∫tt0(‖v(θ)‖2+‖w(θ)‖2)dθ=+∞, then by using well known L'Hospital rule, we get
limt→+∞(t−t0)λ(‖v(t)‖2+‖w(t)‖2)≤H(v(t0),w(t0))δmγΓ(2−λ). | (4.10) |
Based on Lemma 2.4, we take the fractional integral of both sides of (4.7) from t0 to t, one can get
H(v(t),w(t))≤H(v(t0),w(t0))−ΩΓ(λ)∫tt0(t−θ)λ−1H1(v(θ),w(θ))dθ. | (4.11) |
Combined with (4.5) and (4.11), we establish
δm(‖v(t)‖2+‖w(t)‖2)≤H1(v(t),w(t))≤H(v(t),w(t))≤H(v(t0),w(t0)), |
which proves that (‖v(t)‖2+‖w(t)‖2) must be bounded, then it follows from (4.10), there exist a T>0 such that
(‖v(t)‖2+‖w(t)‖2)≤H(v(t0),w(t0))δmγΓ(2−λ)(t−t0)λ,∀t≥T, | (4.12) |
which means that
limt→+∞(‖v(t)‖2+‖w(t)‖2)=0. |
Therefore, master-slave FODGRNs systems (2.1) and (4.1) can realize global asymptotic synchronization under adaptive feedback control (4.3) and the proof of Theorem 4.6 is ended.
Corollary 4.7 Under Assumption 1, master-slave FODGRNs systems (2.1) and (4.1) with σ1=σ2=0 realize global asymptotic synchronization under adaptive feedback control (4.3) if there exist positive diagonal matrices X>0,Y>0,R1>0 and R2>0 such that the following LMIs are holds:
(ⅰ). There exist two constants ζ1>0 and ζ2>0 such that
[−2XA−2X˜K+R2D2+ζ1XXB✠−R1]<0,[−2YC−2Y˜L+R1S2+ζ2YY✠−R2]<0, |
where X, Y, ˜K and ˜L are already defined in Theorem 4.6.
Remark 4.8 For time delayed GRNs, there are many findings regarding stability and synchronization criteria, see Ref [10,20,26,30,33]. Yet these results are discussed mainly in the case of integer-order. Consequently, the stability and synchronization of FODGRNs via 2-norm method properties are not studied by anyone. Therefore our research work, in the sense of innovation, is completely distinct from previous ones.
Remark 4.9 When λ=1, the FODGRNs model (2.1) reduces into global exponential stability and synchronization for integer-order time-delayed gene regulatory networks.
Remark 4.10 In Theorem 3.5, Theorem 4.4, and Theorem 4.6, the sufficient condition ensuring global stability in sense of Mittag-Leffler, global synchronization in sense of Mittag-Leffler, and adaptive synchronization for Caputo sense FODGRNs are derived in form of LMIs. These results can be easily checked with the MATLAB LMI Control Toolbox.
This section, providing three numerical simulations to verify the superiority and benefits of the presented main results.
Example 5.1 Consider a class of three dimensional FODGRNs:
{C0Dλtgp(t)=−apgp(t)+3∑q=1bpqfq(hq(t−σ1))+Fp,C0Dλthp(t)=−cphp(t)+dpgp(t−σ2),p=1,2,3, | (5.1) |
where λ=0.98, g(t)=(g1(t),g2(t),g3(t))T, h(t)=(h1(t),h2(t),h3(t))T, a1=a2=a3=6, c1=c2=c3=5, d1=d2=d3=1.5, σ1=σ2=0.6, fq(hq(t))=h2q(t)1+h2q(t), F1=F2=F3=1.5, and
B=(bpq)3×3=[1.4−0.81.6−0.70.651.22−1.5−0.5]. |
From Assumption 1, we have β1=β2=β3=0.3. Choose α1=α1=α3=1. According to the conditions of Theorem 3.3, it is easy to obtain ξ1=37.63,ξ2=38.35 and ξ3=37.69. Then, let us take X=diag{2,2,2} and Y=diag{1.5,1.5,1.5}. Based on the conditions of Theorem 3.5, we have
ΦM(X)(−2‖A‖+‖B‖‖S‖ΦM(X)+Φm(Y)Φm(Y)+ΦM(Y)Φm(X)‖D‖)=−17.3844<0ΦM(Y)(−2‖C‖+‖D‖Φm(X)+ΦM(Y)Φm(X)+ΦM(X)Φm(Y)‖B‖‖S‖)=−8.0625<0. |
Thus, all conditions of Theorem 3.3 and Theorem 3.5 holds. Therefore, the existence of the equilibrium point of FODGRNs (5.1) is globally Mittag-Leffler stable. Furthermore, the simulation results for FODGRNs (5.1) narrates in Figures 1 and 2 under that initial values ω(t)=(−0.65,0.3,1.3)T, ϖ(t)=(0.25,0.8,1.5)T. The state trajectories for concerned FODGRNs (5.1) are displayed in Figure 1. Figure 2 indicates the state norm trajectories for the concerned system (5.1).
where λ=0.99, g(t)=(g1(t),g2(t),g3(t))T, h(t)=(h1(t),h2(t),h3(t))T, a1=a2=a3=1, c1=c2=c3=2.5, d1=d2=d3=3, σ1=σ2=0.4, fq(hq(t))=h2q(t)1+h2q(t), F1=F2=F3=0, and
B=(bpq)3×3[−2.53.21.51.31.6−2.5−2.41.6−1.8]. |
The three-dimensional slave system is given as:
{C0Dλtup(t)=−apup(t)+3∑q=1bpqfq(zq(t−σ1))+Fp+xp(t),C0Dλtzp(t)=−cpzp(t)+dpup(t−σ2)+yp(t),p=1,2,3 | (5.2) |
where λ=0.99, xp(t) and yp(t) are linear feedback control, and others are same as FODGRNs (5.3). The initial conditions are chosen as: ω(t)=(1.7,−1.3,2.5)T, ϖ(t)=(−2,−1.4,−1.9)T, ˜ω(t)=(−2,−1.6,−1.7)T and ˜ϖ(t)=(1.4,2.5,2)T. When the controllers are not applied, the state trajectories of FODGRNs (5.3) and FODGRNs (5.2) are shown in Figure 3. In Figure 4 depicts the synchronization error trajectories of FODGRNs (5.3) and FODGRNs (5.2), without applying any control inputs. The chaotic behavior of FODGRNs (5.3) and FODGRNs (5.2), without using controller are shown in Figure 5.
Example 5.2 Consider a class of three-dimensional FODGRNs:
{C0Dλtgp(t)=−apgp(t)+∑3q=1bpqfq(hq(t−σ1))+Fp,C0Dλthp(t)=−cphp(t)+dpgp(t−σ2),p=1,2,3, | (5.3) |
One simply to verify that Assumption 1 holds with β1=β2=β3=0.75. By Theorem 4.4, we select X=diag{1.75,1.75,1.75} and Y=diag{2,2,2}. If we choose the control gains of linear feedback controller (4.2) as: k1=k2=k3=14 and l1=l2=l3=12. Based on the above parameters, it is simply to get
ΦM(X)(−2‖A‖−2‖K‖+‖B‖‖S‖ΦM(X)+Φm(Y)Φm(Y)+ΦM(Y)Φm(X)‖D‖)=−34.37<0,ΦM(Y)(−2‖C‖−2‖L‖+‖D‖Φm(X)+ΦM(Y)Φm(X)+ΦM(X)Φm(Y)‖B‖‖S‖)=−40.75<0. |
Hence, all the conditions of Theorem 4.4 hold. Therefore, the master system FODGRNs (5.3) synchronizes with the slave system FODGRNs (5.2) under linear feedback control.
When the linear feedback controllers are applied to the slave system, the state trajectories of FODGRNs (5.3) and FODGRNs (5.2) are exhibits in Figure 6. Figure 7 depicts the synchronization error trajectories of FODGRNs (5.3) and FODGRNs (5.2). The chaotic behavior of FODGRNs (5.3) and FODGRNs (5.2) with control inputs are shown in Figure 8. Synchronization error norm of FODGRNs (5.3) and FODGRNs (5.2) are displayed in Figure 9.
Example 5.3 Consider a class of two dimensional FODGRNs:
{C0Dλtgp(t)=−apgp(t)+∑2q=1bpqfq(hq(t−σ1))+Fp,C0Dλthp(t)=−cphp(t)+dpgp(t−σ2),p=1,2, | (5.4) |
where λ=0.98, g(t)=(g1(t),g2(t))T, h(t)=(h1(t),h2(t))T, a1=a2=2, c1=c2=2.5, d1=d2=2.5, σ1=σ2=0.9, fq(hq(t))=h2q(t)1+h2q(t), F1=F2=2.4, and
B=(bpq)2×2=[−3.52.2−2.3−3.6]. |
The two-dimensional slave system is given as:
{C0Dλtup(t)=−apup(t)+2∑q=1bpqfq(zq(t−σ1))+Fp+xp(t),C0Dλtzp(t)=−cpzp(t)+dpup(t−σ2)+yp(t),p=1,2, | (5.5) |
where λ=0.98, xp(t) and yp(t) are adaptive feedback control, the initial values are selected as: ω(t)=(1.8,−1.22)T, ϖ(t)=(−1.14,1.6)T, ˜ω(t)=(−1.1,1.6)T and ˜ϖ(t)=(1.2,−2)T, and others are same as FODGRNs (5.4).
When the control inputs are not applied in FODGRNs (5.5), the state trajectories of (5.3) and (5.2) are shown in Figure 10. In Figure 11 depicts the time evolution of synchronization error, without using control inputs. The phase plot of FODGRNs (5.4) and FODGRNs (5.5), without using the controller is depicted in Figure 12. In Figures 10–12 demonstrate that FODGRNs (5.4) and FODGRNs (5.5) did not achieve synchronization if the control inputs are not applied.
Through simple computation, we have β1=β2=0.1. If the adaptive feedback controller xp(t) and yp(t) in (4.3) is designed as, select ˜k1=˜k2=0.8, ˜k1=˜k2=0.5, ξ1(0)=ξ2(0)=0.05 and η1(0)=η2(0)=0.08. Let ζ1=3.5, ζ2=2.8, ζ3=0.5, ζ4=0.4, k⋆1=k⋆2=l⋆1=l⋆2=0.9. Then it is clear to find that the given LMIs (i) and (ii) of Theorem 4.6 is feasible, and these solutions are given as below:
X=[0.769000.796],Y=[0.1875000.1937],R1=[4.5662004.7104],R2=[0.0232000.0241]. |
Therefore, all conditions of Theorem 4.6 are holds, which indicates that master-slave FODGRNs systems (5.4) and (5.5) realize global asymptotic synchronization under adaptive feedback control (4.3). The time responses of states (5.3) and (5.2) with control inputs are displayed in Figure 13. The time evolution of synchronization errors with control inputs is illustrated in Figure 14. The phase plot of FODGRNs (5.4) and FODGRNs (5.5) with control inputs are shown in Figure 15. Synchronization error norm of (5.3) and (5.2) with control inputs are presented in Figure 16. The adaptive control strengths are demonstrated in Figure 17, which it tends to some positive constants.
Remark 5.4 As we can see that the linear feedback controller (4.2) is simpler than the adaptive feedback controller (4.3), but the control strengths of the adaptive feedback controller (4.3) is smaller than those of linear feedback controller (4.2). Adaptive synchronization is superior to the synchronization in general.
In this manuscript, the stability and synchronization for fractional-order gene regulatory networks wit time-delay effects has been investigated in brief. Under some inequality techniques, Razumikhin approach and fractional order Lyapunov method, the globally Mittag-Leffler stability of proposed FODGRNs is proved. Moreover, the suitable controllers were designed to ensure the several synchronization for addressing FODGRNs in terms of LMIs. Further, three numerical simulations are provided. Our future research work will be generalized to state estimator design for fuzzy non-integer order gene regulatory networks with time delays and impulsive effects.
This article has been written with the joint partial financial support of RUSA Phase 2.0 Grant No. F 2451/2014-U, Policy (TN Multi-Gen), Dept.of Edn. Govt. of India, UGC-SAP (DRS-I) Grant No. F.510/8/DRS-I/2016(SAP-I), DST-PURSE 2nd Phase programme vide letter No. SR/ PURSE Phase 2/38 (G), DST (FIST - level I) 657876570 Grant No.SR/FIST/MS-I/ 2018/17 and the National Science Centre in Poland Grant DEC-2017/25/ B/ST7/02888.
The authors declare no conflict of interest.
[1] | R. Anbuvithya, K. Mathiyalagan, R. Sakthivel, P. Prakash, Sampled-data state estimation for genetic regulatory networks with time varying delays, Neurocomputing, 15 (2015), 737–744. |
[2] |
M. S. Ali, R. Agalya, K. S. Hong, Non-fragile synchronization of genetic regulatory networks with randomly occurring controller gain fluctuation, Chin. J. Phys., 62 (2019), 132–143. doi: 10.1016/j.cjph.2019.09.019
![]() |
[3] |
D. Baleanu, A. Jajarmi, H. Mohammadi, S. Rezapour, A new study on the mathematical modelling of human liver with Caputo-Fabrizio fractional derivative, Chaos, Solitons Fractals, 134 (2020), 109705. doi: 10.1016/j.chaos.2020.109705
![]() |
[4] | D. Baleanu, S. Sadati, R. Ghaderi, A. Ranjbar, T. Abdeljawad, F. Jarad, Razumikhin stability theorem for fractional systems with delay, Abstract Appl. Anal., 9 (2010), 124812. |
[5] | I. Barbalat, Systems d'equations differential d'oscillations nonlinearities, Revue Roumaine De Math. Pures Et Appl., 4 (1959), 267–270. |
[6] |
J. Cao, D. W. Ho, X. Huang, LMI-based criteria for global robust stability of bidirectional associative memory networks with time delay, Nonlinear Anal., 66 (2007), 1558–1572. doi: 10.1016/j.na.2006.02.009
![]() |
[7] |
S. Ding, Z. Wang, Event-triggered synchronization of discrete-time neural networks: A switching approach, Neural Networks., 125 (2020), 31–40. doi: 10.1016/j.neunet.2020.01.024
![]() |
[8] |
S. Ding, Z. Wang, N. Rong, Intermittent control for quasisynchronization of delayed discrete-time neural networks, IEEE Trans. Cybern., 51 (2021), 862–873. doi: 10.1109/TCYB.2020.3004894
![]() |
[9] | S. Ding, Z. Wang, Synchronization of coupled neural networks via an event-dependent intermittent pinning control, IEEE Trans. Syst., Man, Cybern.: Syst., (2020). Available from: Doi: 10.1109/TSMC.2020.3035173. |
[10] |
Z. Ding, Y. Shen, L. Wang, Global Mittag-Leffler synchronization of fractional order neural networks with discontinuous activations, Neural Networks., 73 (2016), 77–85. doi: 10.1016/j.neunet.2015.10.010
![]() |
[11] |
F. Du, J. G. Lu, New criterion for finite-time stability of fractional delay systems, Appl. Math. Lett., 104 (2020), 106248. doi: 10.1016/j.aml.2020.106248
![]() |
[12] | F. Du, J. G. Lu, Finite-time stability of neutral fractional order time delay systems with Lipschitz nonlinearities, Appl. Math. Comput., 375 (2020), 125079. |
[13] |
M. A. Duarte-Mermoud, N. Aguila-Camacho, J. A, Gallegos, R. Castro-Linares, Using general quadratic Lyapunov functions to prove Lyapunov uniform stability for fractional order systems, Comm. Nonlinear Sci. Numer. Simul., 22 (2015), 650–659. doi: 10.1016/j.cnsns.2014.10.008
![]() |
[14] |
N. Jiang, X. Liu, W. Yu, J. Shen, Finite-time stochastic synchronization of genetic regulatory networks, Neurocomputing, 167 (2015), 314–321. doi: 10.1016/j.neucom.2015.04.064
![]() |
[15] |
C. Huang, J. Cao, M. Xiao, Hybrid control on bifurcation for a delayed fractional gene regulatory network, Chaos, Solitons Fractals, 87 (2016), 19–29. doi: 10.1016/j.chaos.2016.02.036
![]() |
[16] |
S. Lakshmanan, J. H. Park, H. Y.Jung, P. Balasubramaniam, S. M. Lee, Design of state estimator for genetic regulatory networks with time-varying delays and randomly occurring uncertainties, Biosystems, 111 (2013), 51–70. doi: 10.1016/j.biosystems.2012.11.001
![]() |
[17] |
H. Li, J. Cao, H. Jiang, A. Alsaedi, Graph theory-based finite-time synchronization of fractional-order complex dynamical networks, J. Franklin Inst., 355 (2018), 5771–5789. doi: 10.1016/j.jfranklin.2018.05.039
![]() |
[18] |
S. Liang, R. Wu, L. Chen, Adaptive pinning synchronization in fractional-order uncertain complex dynamical networks with delay, Physica A., 444 (2016), 49–62. doi: 10.1016/j.physa.2015.10.011
![]() |
[19] |
P. L. Liu, Robust stability analysis of genetic regulatory network with time delays, ISA Trans., 52 (2013), 326–334. doi: 10.1016/j.isatra.2013.01.003
![]() |
[20] |
Q. Luo, R. Zhang, X. Liao, Unconditional global exponential stability in Lagrange sense of genetic regulatory networks with SUM regulatory logic, Cogn Neurodyn., 4 (2010), 251–261. doi: 10.1007/s11571-010-9113-1
![]() |
[21] | S. Mohamad, Exponential stability in Hopfield type neural networks with impulses, Chaos Solitions Fractals, 32 (2007), 457–467. |
[22] | I. Podlubny, Fractional differential equations, San Diego California: Academic Press, 1999. |
[23] |
Y. Qiao, H. Yan, L. Duan, J. Miao, Finite-time synchronization of fractional-order gene regulatory networks with time delay, Neural Networks, 126 (2020), 1–10. doi: 10.1016/j.neunet.2020.02.004
![]() |
[24] |
J. Qiu, K. Sun, C. Yang, X. Chen, C. Xiang, A. Zhangyong, Finite-time stability of genetic regulatory networks with impulsive effects, Neurocomputing, 219 (2017), 9–14. doi: 10.1016/j.neucom.2016.09.017
![]() |
[25] |
F. Ren, F. Cao, J. Cao, Mittag-Leffler stability and generalized Mittag–Leffler stability of fractional-order gene regulatory networks, Neurocomputing., 160 (2015), 185–190. doi: 10.1016/j.neucom.2015.02.049
![]() |
[26] |
S. Senthilraj, R. Raja, Q. Zhu, R. Samidurai, H. Zhou, Delay-dependent asymptotic stability criteria for genetic regulatory networks with impulsive perturbations, Neurocomputing, 214 (2016), 981–990. doi: 10.1016/j.neucom.2016.07.018
![]() |
[27] | I. Stamova, Stability of impulsive fractional differential equations, Berlin: Walter de Gruyter. |
[28] |
T. Stamov, I. Stamova, Design of impulsive controllers and impulsive control strategy for the Mittag–Leffler stability behavior of fractional gene regulatory networks, Neurocomputing, 424 (2021), 54–62. doi: 10.1016/j.neucom.2020.10.112
![]() |
[29] |
B. Tao, M. Xiao, Q. Sun, J. Cao, Hopf bifurcation analysis of a delayed fractional-order genetic regulatory network model, Neurocomputing, 275 (2018), 677–686. doi: 10.1016/j.neucom.2017.09.018
![]() |
[30] |
X. Yang, T. Huang, Q. Song, J. Huang, Synchronization of fractional-order memristor-based complex valued neural networks with uncertain parameters and time delays, Chaos, Solitons Fractals, 110 (2018), 105–123. doi: 10.1016/j.chaos.2018.03.016
![]() |
[31] |
H. Wu, X. Zhang, S. Xue, L. Wang, Y. Wang, LMI conditions to global Mittag-Leffler stability of fractional-order neural networks with impulses, Neurocomputing, 193 (2016), 148–154. doi: 10.1016/j.neucom.2016.02.002
![]() |
[32] |
J. Yan, J. Shen, Impulsive stabilization of impulsive functional differential equations by Lyapunov-Razumikhin functions, Nonlinear Anal., 37 (1999), 245–255. doi: 10.1016/S0362-546X(98)00045-5
![]() |
[33] |
X. Yang, C. Li, T. Huang, Q. Song, X. Chen, Quasi-uniform synchronization of fractional-order memristor-based neural networks with delay, Neurocomputing, 234 (2017), 205–215. doi: 10.1016/j.neucom.2017.01.014
![]() |
[34] |
J. Yu, C. Hu, H. Jiang, Projective synchronization for fractional neural networks, Neural Networks, 49 (2014), 87–95. doi: 10.1016/j.neunet.2013.10.002
![]() |
[35] |
D. Yue, Z. H. Guan, T. Li, R. Q. Liao, F. Liu, Q. Lai, Event-based cluster synchronization of coupled genetic regulatory networks, Physica A., 482 (2017), 649–665. doi: 10.1016/j.physa.2017.04.024
![]() |
[36] | L. Zhang, X. Zhang, Y. Xue, X. Zhang, New method to global exponential stability analysis for switched genetic regulatory networks with mixed delays, IEEE Trans. NanoBiosci., 19 (2020), 308–314. |
[37] |
S. Zhang, Y. Yu, H. Wang, Mittag-Leffler stability of fractional-order Hopfield neural networks, Nonlinear Anal.: Hybrid Syst., 16 (2015), 104–121. doi: 10.1016/j.nahs.2014.10.001
![]() |
1. | Mani Mallika Arjunan, Thabet Abdeljawad, Pratap Anbalagan, Impulsive effects on fractional order time delayed gene regulatory networks: Asymptotic stability analysis, 2022, 154, 09600779, 111634, 10.1016/j.chaos.2021.111634 | |
2. | Ivanka Stamova, Gani Stamov, Lyapunov Approach for Almost Periodicity in Impulsive Gene Regulatory Networks of Fractional Order with Time-Varying Delays, 2021, 5, 2504-3110, 268, 10.3390/fractalfract5040268 | |
3. | M. Hymavathi, Tarek F. Ibrahim, M. Syed Ali, Gani Stamov, Ivanka Stamova, B. A. Younis, Khalid I. Osman, Synchronization of Fractional-Order Neural Networks with Time Delays and Reaction-Diffusion Terms via Pinning Control, 2022, 10, 2227-7390, 3916, 10.3390/math10203916 | |
4. | Xuemei Li, Xinge Liu, Shuailei Zhang, New criteria on the finite-time stability of fractional-order BAM neural networks with time delay, 2022, 34, 0941-0643, 4501, 10.1007/s00521-021-06605-3 | |
5. | J. L. Echenausía-Monroy, C. A. Rodríguez-Martíne, L. J. Ontañón-García, J. Alvarez, J. Pena Ramirez, Atila Bueno, Synchronization in Dynamically Coupled Fractional-Order Chaotic Systems: Studying the Effects of Fractional Derivatives, 2021, 2021, 1099-0526, 1, 10.1155/2021/7242253 | |
6. | S. Aadhithiyan, R. Raja, J. Alzabut, Q. Zhu, M. Niezabitowski, Robust non‐fragile Mittag‐Leffler synchronization of fractional order non‐linear complex dynamical networks with constant and infinite distributed delays, 2022, 45, 0170-4214, 2166, 10.1002/mma.7915 | |
7. | Mani Mallika Arjunan, Pratap Anbalagan, Qasem Al‐Mdallal, Robust uniform stability criteria for fractional‐order gene regulatory networks with leakage delays, 2022, 0170-4214, 10.1002/mma.8255 | |
8. | Ricardo Almeida, Ravi P. Agarwal, Snezhana Hristova, Donal O’Regan, Stability of Gene Regulatory Networks Modeled by Generalized Proportional Caputo Fractional Differential Equations, 2022, 24, 1099-4300, 372, 10.3390/e24030372 | |
9. | Hongyun YAN, Yuanhua QIAO, Lijuan DUAN, Jun MIAO, Synchronization of fractional-order gene regulatory networks mediated by miRNA with time delays and unknown parameters, 2022, 359, 00160032, 2176, 10.1016/j.jfranklin.2022.01.028 | |
10. | Zhong Ji, Zhihao Li, Yan Zhang, Haoran Wang, Yanwei Pang, Xuelong Li, Hierarchical matching and reasoning for multi-query image retrieval, 2024, 173, 08936080, 106200, 10.1016/j.neunet.2024.106200 | |
11. | Hasan S. Panigoro, Nursanti Anggriani, Emli Rahmi, Understanding the Role of Intraspecific Disease Transmission and Quarantine on the Dynamics of Eco-Epidemiological Fractional Order Model, 2023, 7, 2504-3110, 610, 10.3390/fractalfract7080610 | |
12. | 兰 Duolan, Linying Xiang, Guanrong Chen, Synchronization of stochastic complex networks with time-delayed coupling, 2023, 32, 1674-1056, 060502, 10.1088/1674-1056/acc44e | |
13. | A. Stephen, R. Raja, Xiaoshan Bai, J. Alzabut, R. Swaminathan, G. Rajchakit, Asymptotic pinning synchronization of nonlinear multi-agent systems: Its application to tunnel diode circuit, 2023, 49, 1751570X, 101366, 10.1016/j.nahs.2023.101366 | |
14. | S. Arivumani, P. Vadivel, G. Rajchakit, T. Saravanakumar, Non-fragile tracking controller design for fractional order systems against active disturbance rejection, 2024, 1951-6355, 10.1140/epjs/s11734-024-01217-z | |
15. | Jiamin Zhou, Chunmei Zhang, Huiling Chen, Exponential stability of stochastic multi-layer complex network with regime-switching diffusion via aperiodically intermittent control, 2024, 662, 00200255, 120241, 10.1016/j.ins.2024.120241 | |
16. | Qing Hu, Bing Zheng, A novel two-layer fuzzy neural network for solving inequality-constrained ℓ1-minimization problem with applications, 2024, 178, 08936080, 106491, 10.1016/j.neunet.2024.106491 |