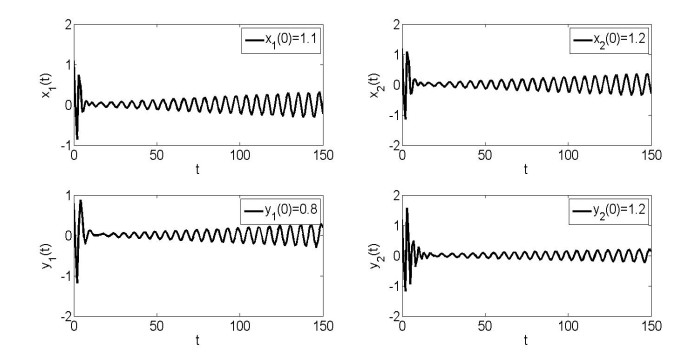
The dynamic signal transmission process can be regarded as an anti-periodic process, and fractional-order inertial neural networks are widely used in signal processing and other fields, so anti-periodicity is also regarded as an important dynamic feature of inertial neural networks. This paper mainly studies the existence and Mittag-Leffler stability of anti-periodic solutions for a class of fractional-order inertial BAM neural networks with time-delays. By introducing variable substitution, the model with two different fractional-order derivatives is transformed into a model with only one fractional-order derivative of the same order. Using the properties of fractional-order calculus, the relationship between the fractional-order integral of the state function with and without time-delays is given. Firstly, the sufficient conditions for the boundedness and the Mittag-Leffler stability of the solutions for the system are derived. Secondly, by constructing the sequence solution of the function for the system and applying Ascoli-Arzela theorem, the sufficient conditions for the existence and Mittag-Leffler stability of the anti-periodic solution are given. Finally, the correctness of the conclusion is verified by a numerical example.
Citation: Yuehong Zhang, Zhiying Li, Wangdong Jiang, Wei Liu. The stability of anti-periodic solutions for fractional-order inertial BAM neural networks with time-delays[J]. AIMS Mathematics, 2023, 8(3): 6176-6190. doi: 10.3934/math.2023312
[1] | Dan-Ning Xu, Zhi-Ying Li . Mittag-Leffler stabilization of anti-periodic solutions for fractional-order neural networks with time-varying delays. AIMS Mathematics, 2023, 8(1): 1610-1619. doi: 10.3934/math.2023081 |
[2] | Zhiying Li, Wei Liu . The anti-periodic solutions of incommensurate fractional-order Cohen-Grossberg neural network with inertia. AIMS Mathematics, 2025, 10(2): 3180-3196. doi: 10.3934/math.2025147 |
[3] | Huizhen Qu, Jianwen Zhou . S-asymptotically ω-periodic dynamics in a fractional-order dual inertial neural networks with time-varying lags. AIMS Mathematics, 2022, 7(2): 2782-2809. doi: 10.3934/math.2022154 |
[4] | Qian Cao, Xiaojin Guo . Anti-periodic dynamics on high-order inertial Hopfield neural networks involving time-varying delays. AIMS Mathematics, 2020, 5(6): 5402-5421. doi: 10.3934/math.2020347 |
[5] | Jingfeng Wang, Chuanzhi Bai . Global Mittag-Leffler stability of Caputo fractional-order fuzzy inertial neural networks with delay. AIMS Mathematics, 2023, 8(10): 22538-22552. doi: 10.3934/math.20231148 |
[6] | Zhiying Li, Wangdong Jiang, Yuehong Zhang . Dynamic analysis of fractional-order neural networks with inertia. AIMS Mathematics, 2022, 7(9): 16889-16906. doi: 10.3934/math.2022927 |
[7] | Yanshou Dong, Junfang Zhao, Xu Miao, Ming Kang . Piecewise pseudo almost periodic solutions of interval general BAM neural networks with mixed time-varying delays and impulsive perturbations. AIMS Mathematics, 2023, 8(9): 21828-21855. doi: 10.3934/math.20231113 |
[8] | Qingyi Cui, Changjin Xu, Wei Ou, Yicheng Pang, Zixin Liu, Jianwei Shen, Muhammad Farman, Shabir Ahmad . Further study on Hopf bifurcation and hybrid control strategy in BAM neural networks concerning time delay. AIMS Mathematics, 2024, 9(5): 13265-13290. doi: 10.3934/math.2024647 |
[9] | Ailing Li, Xinlu Ye . Finite-time anti-synchronization for delayed inertial neural networks via the fractional and polynomial controllers of time variable. AIMS Mathematics, 2021, 6(8): 8173-8190. doi: 10.3934/math.2021473 |
[10] | Jin Gao, Lihua Dai . Anti-periodic synchronization of quaternion-valued high-order Hopfield neural networks with delays. AIMS Mathematics, 2022, 7(8): 14051-14075. doi: 10.3934/math.2022775 |
The dynamic signal transmission process can be regarded as an anti-periodic process, and fractional-order inertial neural networks are widely used in signal processing and other fields, so anti-periodicity is also regarded as an important dynamic feature of inertial neural networks. This paper mainly studies the existence and Mittag-Leffler stability of anti-periodic solutions for a class of fractional-order inertial BAM neural networks with time-delays. By introducing variable substitution, the model with two different fractional-order derivatives is transformed into a model with only one fractional-order derivative of the same order. Using the properties of fractional-order calculus, the relationship between the fractional-order integral of the state function with and without time-delays is given. Firstly, the sufficient conditions for the boundedness and the Mittag-Leffler stability of the solutions for the system are derived. Secondly, by constructing the sequence solution of the function for the system and applying Ascoli-Arzela theorem, the sufficient conditions for the existence and Mittag-Leffler stability of the anti-periodic solution are given. Finally, the correctness of the conclusion is verified by a numerical example.
As a special case of periodic solutions, the existence and stability of anti-periodic solutions are extremely important in the dynamic behavior of nonlinear differential equations. Their phenomena are widely found in various physical phenomena [1,2,3,4,5]. In recent years, the scholars have obtained many results on the existence and stability. For example, [6,7,8] studied the stability of inertial BAM neural networks and Cohen-Grossberg BAM neural networks. [9,10] studied the global exponential stability of anti-periodic solutions for BAM neural networks. The global exponential stability of the anti-periodic solution of Cohen-Grossberg neural networks is determined by[11]. The existence and stability of anti-periodic solutions of BAM type Cohen-Grossberg neural networks are studied by[12]. The existence and stability of anti-periodic solutions of inertial neural networks are studied by[13]. Almost anti-periodic solution of inertial neural networks with leakage and time-varying delays on timescales is studied in[14]. The paper [15] discussed the anti-periodic dynamics on high-order inertial Hopfield neural networks with time-varying delays. The paper [16] studied the anti-periodicity on high-order inertial Hopfield neural networks with mixed delays.
From [9,10,11,12,13,14,15,16], it can be seen that the models are all models with integer derivative. In recent years, the study of periodic solutions of fractional-order neural networks has been attracted, some important results have been obtained, such as: asymptotic periodic solutions, s-asymptotic periodic solutions, almost periodic solutions of fractional-order neural networks in[17,18,19,20,21].
Fractional-order calculus is a generalization of integer-order. Fractional-order differential equations are considered as a powerful tool for modeling practical problems in biology, chemistry, physics, medicine, economics and other sciences.
With the further study of the periodicity of nonlinear differential equations, many scholars have paid attention to the anti-periodic problem, which is another characteristic of periodic changes, such as[22,23,24]. The phenomena are widely seen in biology, economics, medicine, physics and many other disciplines. Because the fractional-order inertial neural networks models are the nonlinear differential equations, which are widely used in signal processing, fluid mechanics, biology and other fields. According to the data we have consulted, there have been many achievements in the research on the global asymptotic stability, Mittag-Leffler stability, single stability and multi stability of periodic solutions of fractional-order inertial neural networks, such as[17,18,19,20,21]. However, the research results on the anti-periodic dynamic behavior of fractional-order inertial neural networks have not been seen yet. In this paper, we study the stability of the anti-periodic solutions of a class of fractional-order inertial BAM neural networks. It is a new topic worthy of study, which will provide a new criterion for theoretical analysis in the exploration of dynamic behavior and practical application. The following are the main innovations of this article:
1) The fractional-order inertial BAM neural networks model with two different fractional-order derivatives is transformed into a model with only one fractional-order derivative with the same order through appropriate variable replacement;
2) When the time variable changes in the finite interval with less than or equal to time-delays and in the infinite interval with more than or equal to time-delays, the relationship between the fractional-order integral of the state function with and without time-delay is derived;
3) By constructing the function sequence solutions of the system, we can derive that it converges uniformly to a continuous function by applying Ascoli-Arzela theorem, then the sufficient conditions for judging the existence and Mittag-Leffler stability of the anti-periodic solutions for the system are given;
4) The results given provides a new theoretical reference for further research on the theory and practical application of fractional-order inertial BAM neural networks with time-delays.
We Consider a class of fractional-order inertial BAM neural networks with time-delays:
{D2αtxi(t)=−ciDαtxi(t)−αixi(t)+n∑j=1aijfj(yj(t))+n∑j=1bijfj(yj(t−τij))+Ii(t),D2αtyj(t)=−djDαtyj(t)−βjyj(t)+n∑i=1gjifi(xi(t))+n∑i=1hjifi(xi(t−σji))+Jj(t), | (1.1) |
where t≥0, i,j=1,2,⋯,n. Dαt is the Riemann-Liouville fractional-order derivative of order α, 0<α<1, xi(t) and yj(t) represent the state of ith and jth neurons of t. ci>0, dj>0, αi>0, βj>0, aij, bij, gji, hji respectively represent the connection weights between neurons, fi(⋅) represents the excitation function of the ith neuron, Ii(t) and Jj(t) represent the external input of the ith and jth neurons at time t, τij and σji represent the signal transmission delay of the ith neuron and the jth neuron at the time t and satisfies 0<τij≤τ, 0<σji≤σ.
The initial conditions of (1.1) are given as follows:
{xi(s)=φ1i(s), Dαtxi(s)=φ2i(s), −δ≤s≤0,yj(s)=ψ1j(s), Dαtyj(s)=ψ2j(s), −δ≤s≤0, | (1.2) |
where i,j=1,2,⋯,n, and φ1i(s), φ2i(s), ψ1j(s), ψ2j(s) are continuous and bounded in [−δ,0], δ=max{σ,τ}.
Definition 2.1. [25] Let q>0 be any positive real number, the fractional-order integral (Riemann- Liourille integral) of f(t) with q order is defined as
D−qtf(t)=1Γ(q)∫t0(t−r)q−1f(r)dr, |
where Γ(⋅) is a Γ function, that is Γ(s)=∫+∞0e−xxs−1dx, s>0.
Definition 2.2. [25] Let q>0 be any positive real number, n−1≤q<n, Riemann-Liourille fractional-order derivative is defined as
Dqtf(t)=1Γ(n−q)dndtn∫t0f(s)(t−s)q−n+1ds. |
Definition 2.3. [25] The Mittag-Leffler function with a parameter q is defined as
Eq(z)=+∞∑k=0zkΓ(kq+1), |
where the real part Re(q)>0 of the complex number q, z is a complex number, and Γ(⋅) is a Γ function.
Definition 2.4. Let XT(t), ¯XT(t) be two solutions of system (1.1) with initial conditions
{xi(s)=φ1i(s), Dαtxi(s)=φ2i(s), −σ≤s≤0,yj(s)=ψ1j(s), Dαtyj(s)=ψ2j(s), −τ≤s≤0, |
and
{¯xi(s)=¯φ1i(s), Dαt¯xi(s)=¯φ2i(s), −σ≤s≤0,¯yj(s)=¯ψ1j(s), Dαt¯yj(s)=¯ψ2j(s), −τ≤s≤0. |
If there are two normal numbers ρ1>0 and ρ2>0, which satisfy
‖X(t)−¯X(t)‖≤[M(φ−¯φ)Eq(−ρ1tq)]ρ2, t≥0, |
then, the solution of system (1.1) is said to be globally Mittag-Leffler stable, where
X(t)=(x1(t),x2(t),⋯,xn(t),y1(t),y2(t),⋯,yn(t))T, |
¯X(t)=(¯x1(t),¯x2(t),⋯,¯xn(t),¯y1(t),¯y2(t),⋯,¯yn(t))T, |
φ(t)=(φ11(t),φ12(t),⋯,φ1n(t),ψ11(t),ψ12(t),⋯,ψ1n(t))T, |
¯φ(t)=(¯φ11(t),¯φ12(t),⋯,¯φ1n(t),¯ψ11(t),¯ψ12(t),⋯,¯ψ1n(t))T, |
M(φ−¯φ)≥0,M(0)=0. |
Eq(⋅) is a Mittag-Leffler function with one parameter.
Definition 2.5. If u(t)∈C(R), u(t+ω)=−u(t) for t∈R, then, u(t) is an anti-periodic function, where ω is a positive number.
Lemma 2.1. [26] If x(t)∈R is continuous and differentiable in [0,δ](δ>0), and 0<q<1, n−1<p<n, n∈Z+, then,
(1) DptDqtx(t)=Dp+qtx(t);
(2) D−qtDqtx(t)=x(t).
Lemma 2.2. [27] If r(t) is derivable and r′(t) is continuous, then,
12Dqtr2(t)≤r(t)Dqtr(t),0<q≤1. |
Lemma 2.3. [26] Let u(t) be a continuous function which is defined in [0,+∞), if there exist constants d1>0 and d2>0, such that u(t)≤−d1D−qtu(t)+d2,t≥0, then,
u(t)≤d2Eq(−d1tq), |
where 0<q<1 and Eq(⋅) is the Mittag-Leffler function with one parameter.
The discussion is based on the assumptions below for i,j=1,2,...,n.
H1. The function fj(⋅) is continuous and bounded which satisfies the Lipschitz condition, that is, there exist constants lj>0, ˉfj>0, satisfing
|fj(u)−fj(v)|≤lj|u−v|,|fj(u)|≤ˉfj,u,v∈R, |
and
fj(u)=−fj(−u),u∈R. |
H2. Ii(t) and Jj(t) are bounded, that is, there exist constants ˉIi>0 and ˉJj>0, satisfing
|Ii(t)|≤ˉIi,|Jj(t)|≤ˉJj. |
H3. There exists a constant ω>0, satisfing Ii(t+ω)=−Ii(t),Jj(t+ω)=−Jj(t).
For system (1.1), we introduce variable replacement:
{ui(t)=Dαtxi(t)+γixi(t), vj(t)=Dαtyj(t)+γjyj(t), γi>0,γj>0, i,j=1,2,⋯,n. |
From Lemma 2.1, system (1.1) can be transformed into
{Dαtxi(t)=−γixi(t)+ui(t),Dαtui(t)=−(αi+γ2i−γici)xi(t)−(ci−γi)ui(t)+n∑j=1aijfj(yj(t))+n∑j=1bijfj(yj(t−τij))+Ii(t),Dαtyj(t)=−γjyj(t)+vj(t),Dαtvj(t)=−(βj+γ2j−γjdj)yj(t)−(dj−γj)vj(t)+n∑i=1gjifi(xi(t))+n∑i=1hjifi(xi(t−σji))+Jj(t). | (2.1) |
Theorem 3.1. For the system (1.1), assume that H1 and H2 are true, if
η1=min1≤i≤n{γi−|αi+γ2i−γici|,ci−γi−1}>0,η2=min1≤j≤n{γj−|βj+γ2j−γjdj|,dj−γj−1}>0, |
then, the solutions xi(t), yj(t) of (1.1) and Dαtxi(t), Dαtyj(t)(i,j=1,2,⋯,n) are bounded in [0,T](T<+∞).
Proof. According to the first two formulas in (2.1), we can get
{Dαt|xi(t)|≤−γi|xi(t)|+|ui(t)|,Dαt|ui(t)|≤|αi+γ2i−γici||xi(t)|−(ci−γi)|ui(t)|+n∑j=1(|aij|+|bij|)ˉfj+ˉIi. | (3.1) |
According to Lemma 2.1, we can get from (3.1) that
|xi(t)|+|ui(t)|≤−γiD−αt|xi(t)|+D−αt|ui(t)|+|αi+γ2i−γici|D−αt|xi(t)|−(ci−γi)D−αt|ui(t)|+D−αt[n∑j=1(|aij|+|bij|)ˉfj+ˉIi]≤−(γi−|αi+γ2i−γici|)D−αt|xi(t)|−(ci−γi−1)D−αt|ui(t)|+[n∑j=1(|aij|+|bij|)ˉfj+ˉIi]TαΓ(α+1)≤−η1D−αt[|xi(t)|+|ui(t)|]+m1, | (3.2) |
where m1=[n∑j=1(|aij|+|bij|)ˉfj+ˉIi]TαΓ(α+1). According to Lemma 2.3 and (3.2), we have
|xi(t)|+|ui(t)|≤m1Eα(−η1tα),t>0, |
so xi(t) and ui(t) are bounded. Due to Dαtxi(t)=−γixi(t)+ui(t), when xi(t) and ui(t) are bounded, and
Dαt|xi(t)|≤−γi|xi(t)|+|ui(t)|≤(γi+1)m1Eα(−η1tα), |
so Dαtxi(t) are bounded.
Similarly, it can be deduced from the last two formulas of (2.1) that
|yj(t)|+|vj(t)|≤m2Eα(−η2tα),Dαt|yj(t)|≤(γj+1)m2Eα(−η2tα), |
where m2=[n∑i=1(|gji|+|hji|)ˉfi+ˉJj]TαΓ(α+1). So yj(t) and Dαtyj(t) are bounded.
Theorem 3.2. Assume that the condition H1 is true, if
η= min{min1≤i≤n{2γi−|1−αi−γ2i+γici|−n∑j=1(|gji|+|hji|)li, 2ci−2γi−|1−αi−γ2i+γici|− n∑j=1(|aij|+|bij|)lj}, min1≤j≤n{2γj−|1−βj−γ2j+γjdj|−n∑i=1(|aij|+|bij|)lj, 2dj−2γj−|1−βj−γ2j+γjdj|− n∑i=1(|gji|+|hji|)li}}>0, then the solutions of system (1.1) are globally Mittag-Leffler stable.
Proof. Let X(t)=(x1(t),x2(t),⋯,xn(t),y1(t),y2(t),⋯,yn(t))T and ¯X(t)=(¯x1(t),¯x2(t),⋯,¯xn(t),¯y1(t), ¯y2(t),⋯,¯yn(t))T be two solutions of system (1.1) with the initial value xi(s)=φ1i(s), Dαtxi(s)=φ2i(s), yi(s)=ψ1i(s), Dαtxi(s)=ψ2i(s) and ¯xi(s)=¯φ1i(s), Dαt¯xi(s)=¯φ2i(s), ¯yi(s)=¯ψ1i(s), Dαt¯yi(s)=¯ψ2i(s). Let zi(t)=xi(t)−¯xi(t), wi(t)=yi(t)−¯yi(t), pi(t)=ui(t)−¯ui(t), qi(t)=vi(t)−¯vi(t), i=1,2,⋯,n. According to (2.1), we get
{Dαtzi(t)=−γizi(t)+pi(t),Dαtpi(t)=−(αi+γi2−γici)zi(t)−(ci−γi)pi(t)+n∑j=1aij(fj(yj(t))−fj(¯yj(t)))+n∑j=1bij(fj(yj(t−τij))−fj(¯yj(t−τij))),Dαtwj(t)=−γjwj(t)+qj(t),Dαtqj(t)=−(βj+γj2−γjdj)wj(t)−(dj−γj)qj(t)+n∑i=1gji(fi(xi(t))−fi(¯xi(t)))+n∑i=1hji(fi(xi(t−σji))−fi(¯xi(t−σji))). | (3.3) |
According to Lemma 2.2 and (3.3), we have
{Dαtz2i(t)≤−2γiz2i(t)+2zi(t)pi(t),Dαtp2i(t)≤−2(αi+γi2−γici)zi(t)pi(t)−2(ci−γi)p2i(t)+2|pi(t)|[n∑j=1|aij|lj|wj(t)|+n∑j=1|bij|lj|wj(t−τij)|],Dαtw2j(t)≤−2γjw2j(t)+2wj(t)qj(t),Dαtq2j(t)≤−2(βj+γj2−γjdj)qj(t)wj(t)−2(dj−γj)q2j(t)+2|qj(t)|[n∑i=1|gji|li|zi(t)|+n∑i=1|hji|li|zi(t−σji)|]. | (3.4) |
From (3.4), we have
Dαtn∑i=1[z2i(t)+p2i(t)]+Dαtn∑j=1[w2j(t)+q2j(t)]≤n∑i=1{−2γiz2i(t)+2(1−αi−γ2i+γici)zi(t)pi(t)−2(ci−γi)p2i(t)+n∑j=1|aij|lj(p2i(t)+w2j(t))+n∑j=1|bij|lj(p2i(t)+w2j(t−τij))}+n∑j=1{−2γjw2j(t)+2(1−βj−γ2j+γjdj)wj(t)qj(t)−2(dj−γj)q2j(t)+n∑i=1|gji|li(z2i(t)+q2j(t))+n∑i=1|hji|li(q2j(t)+z2i(t−σji))}=n∑i=1{−[2γi−|1−αi−γ2i+γici|−n∑j=1|gji|li]z2i(t)−[2ci−2γi−|1−αi−γ2i+γici|−n∑j=1(|aij|+|bij|)lj]p2i(t)}+n∑j=1{−[2γj−|1−βj−γ2j+γjdj|−n∑i=1|aij|lj]w2j(t)−[2dj−2γj−|1−βj−γ2j+γjdj|−n∑i=1(|gji|+|hji|)li]q2j(t)}+n∑i=1n∑j=1|bij|ljw2j(t−τij)+n∑j=1n∑i=1|hji|liz2i(t−σji). | (3.5) |
From (3.5), we can obtain
n∑i=1[z2i(t)+p2i(t)]+n∑j=1[w2j(t)+q2j(t)]≤n∑i=1{−[2γi−|1−αi−γ2i+γici|−n∑j=1|gji|li]D−αtz2i(t)−[2ci−2γi−|1−αi−γ2i+γici|−n∑j=1(|aij|+|bij|)lj]D−αtp2i(t)}+n∑j=1{−[2γj−|1−βj−γ2j+γjdj|−n∑i=1|aij|lj]D−αtw2j(t)−[2dj−2γj−|1−βj−γ2j+γjdj|−n∑i=1(|gji|+|hji|)li]D−αtq2j(t)}+n∑i=1n∑j=1|bij|ljD−αtw2j(t−τij)+n∑j=1n∑i=1|hji|liD−αtz2i(t−σji). | (3.6) |
When t∈[0,τij], we have t−τij≤0, then,
D−αtw2j(t−τij)=1Γ(α)∫t0(t−s)α−1w2j(s−τij)ds=1Γ(α)∫t−τij−τij(t−u−τij)α−1w2j(u)du≤w∗jΓ(α)∫t−τij−τij(t−u−τij)α−1du=w∗jtαΓ(α+1)≤w∗jταΓ(α+1), | (3.7) |
where w∗j=sup−τ≤s≤0{|ψ1j(s)−¯ψ1j(s)|},j=1,2,⋯,n.
When t∈[τij,+∞), we have t−τij≥0, then
D−αtw2j(t−τij)=1Γ(α)∫t0(t−s)α−1w2j(s−τij)ds=1Γ(α)∫t−τij−τij(t−u−τij)α−1w2j(u)du=1Γ(α)∫0−τij(t−u−τij)α−1w2j(u)du+1Γ(α)∫t−τij0(t−u−τij)α−1w2j(u)du≤w∗jταΓ(α+1)+1Γ(α)∫t0(t−u)α−1w2j(u)du=w∗jταΓ(α+1)+D−αtw2j(t). | (3.8) |
From (3.7) and (3.8), we get
D−αtw2j(t−τij)≤w∗jταΓ(α+1)+D−αtw2j(t), | (3.9) |
where w∗j=sup−τ≤s≤0{|ψ1j(s)−¯ψ1j(s)|},j=1,2,⋯,n. The same can be deduced
D−αtz2i(t−σji)≤z∗iσαΓ(α+1)+D−αtz2i(t), | (3.10) |
where z∗i=sup−σ≤s≤0{(φ1i(s)−¯φ1i(s))2},i=1,2,⋯,n.
We substitute (3.9) and (3.10) into (3.6) to get
n∑i=1[z2i(t)+p2i(t)]+n∑j=1[w2j(t)+q2j(t)]≤n∑i=1{−[2γi−|1−αi−γ2i+γici|−n∑j=1(|gji+|hji|)li]D−αtz2i(t)−[2ci−2γi−|1−αi−γ2i+γici|−n∑j=1(|aij|+|bij|)lj]D−αtp2i(t)}+n∑j=1{−[2γj−|1−βj−γ2j+γjdj|−n∑i=1(|aij|+|bij|)lj]D−αtw2j(t)−[2dj−2γj−|1−βj−γ2j+γjdj|−n∑i=1(|gji|+|hji|)li]D−αtq2j(t)}+1Γ(α+1)n∑i=1n∑j=1[|bij|ljw∗jτα+|hji|liz∗iσα]≤−ηD−αt[n∑i=1(z2i(t)+p2i(t))+n∑j=1(w2j(t)+q2j(t))]+M(φ−¯φ), |
where
M(φ−¯φ)=1Γ(α+1)n∑i=1n∑j=1[|bij|ljw∗jτα+|hji|liz∗iσα], |
φ=(φ11(t),φ12(t),⋯,φ1n(t),ψ11(t),ψ12(t),⋯,ψ1n(t))T, |
¯φ=(¯φ11(t),¯φ12(t),⋯,¯φ1n(t),¯ψ11(t),¯ψ12(t),⋯,¯ψ1n(t))T. |
According to Lemma 2.3, we have
n∑i=1[z2i(t)+p2i(t)]+n∑j=1[w2j(t)+q2j(t)]≤M(φ−¯φ)Eα(−ηtα). |
Then,
n∑i=1z2i(t)+n∑i=1w2i(t)≤M(φ−¯φ)Eα(−ηtα). |
Using the inequality n∑i=1|ai|≤(nn∑i=1a2i)12, we have
‖X(t)−¯X(t)‖=n∑i=1[|zi(t)|+|wi(t)|]≤2[nM(φ−¯φ)Eα(−ηtα)]12. | (3.11) |
Obviously, M(0)=0, M(φ−¯φ)≥0. According to Definition 2.4, the solutions of the system (1.1) are globally Mittag-Leffler stable.
Theorem 3.3. Suppose that H1–H3 hold, if the conditions of Theorems 3.1 and 3.2 are satisfied, then the system (1.1) has an anti-periodic solution which is globally Mittag-Leffler stable.
Proof. Let X(t)=(x1(t),x2(t),⋯,xn(t),y1(t),y2(t),⋯,yn(t))T be any solution of system (1.1). For any natural number k and ω>0 which is given in H3, we can get from (2.1) that
{Dαt[(−1)k+1xi(t+(k+1)ω)]=−γi(−1)k+1xi(t+(k+1)ω)+(−1)k+1ui(t+(k+1)ω),Dαt[(−1)k+1ui(t+(k+1)ω)]=−(αi+γi2−γici)(−1)k+1xi(t+(k+1)ω)−(ci−γi)(−1)k+1ui(t+(k+1)ω)+n∑j=1aijfj((−1)k+1yj(t+(k+1)ω))+n∑j=1bij(fj((−1)k+1yj(t+(k+1)ω−τij))+Ii(t),Dαt[(−1)k+1yj(t+(k+1)ω)]=−γj(−1)k+1yj(t+(k+1)ω)+(−1)k+1vj(t+(k+1)ω),Dαt[(−1)k+1vj(t+(k+1)ω)]=−(βj+γj2−γjdj)(−1)k+1yj(t+(k+1)ω)−(dj−γj)(−1)k+1vj(t+(k+1)ω)+n∑i=1gjifi((−1)k+1xi(t+(k+1)ω))+n∑i=1hji(fi((−1)k+1xi(t+(k+1)ω−σji))+Jj(t). | (3.12) |
It can be seen that for any natural number k,
(−1)k+1xi(t+(k+1)ω),(−1)k+1ui(t+(k+1)ω),(−1)k+1yj(t+(k+1)ω),(−1)k+1vj(t+(k+1)ω) |
are the solutions of (2.1), then,
(−1)kxi(t+kω),(−1)kui(t+kω),(−1)kyj(t+kω),(−1)kkvj(t+kω)(i,j=1,2,⋯,n) |
are also the solutions of (2.1). This means (−1)kxi(t+kω),(−1)kyj(t+kω) are the solution of system (1.1). From Theorem 3.1, the solutions of system (1.1) xi(t),yj(t) and Dαtxi(t),Dαtyj(t) are bounded, due to
ui(t)=Dαtxi(t)+γixi(t),vj(t)=Dαtyj(t)+γjyj(t), |
we get (−1)kxi(t+kω),(−1)kui(t+kω),(−1)kyj(t+kω),(−1)kvj(t+kω) are also bounded, i,j=1,2,⋯,n.
On the other hand, due to
RLaDαtg(t)=CaDαtg(t)+n−1∑k=1(t−a)k−αΓ(k−α+1)fk(a), |
where RLaDαtg(t) is Riemann-Liourille fractional-order derivative, CaDαtg(t) is Caputo fractional-order derivative, that is
CaDαtg(t)=1Γ(n−α)∫taf(n)(τ)(t−τ)α+1−ndτ,n−1<α<n. |
So that we can get xi(t),yj(t),ui(t), vj(t)(i,j=1,2,⋯,n) are differentiable, then we obtain the function sequence (−1)kxi(t+kω), (−1)kui(t+kω), (−1)kyj(t+kω),(−1)kvj(t+kω) is equicontinuity and uniform boundedness. Using the Ascoli-Arzela theorem, we can select a subsequence {kω}k∈N, such that {(−1)kxi(t+kω)}k∈N, {(−1)kyj(t+kω)}k∈N, {(−1)kui(t+kω)}k∈N, {(−1)kvj(t+kω)}k∈N(N=1,2,⋯,n,⋯) uniformly converge to continuous functions x∗i(t),y∗j(t), u∗i(t), v∗j(t) on any compact set in [0,+∞), that is,
limk→+∞(−1)kxi(t+kω)=x∗i(t),limk→+∞(−1)kyj(t+kω)=y∗j(t), |
limk→+∞(−1)kui(t+kω)=u∗i(t),limk→+∞(−1)kvj(t+kω)=v∗j(t). |
Let
X∗(t)=(x∗1(t),x∗2(t),⋯,x∗n(t),y∗1(t),y∗2(t),⋯,y∗n(t))T, |
U∗(t)=(u∗1(t),u∗2(t),⋯,u∗n(t),v∗1(t),v∗2(t),⋯,v∗n(t))T. |
We can prove that X∗(t) and U∗(t) are anti-periodic functions. In fact,
X∗(t)=limk→+∞(−1)kX(t+ω+kω)=−limk→+∞(−1)k+1X(t+(k+1)ω)=−X∗(t), |
U∗(t)=limk→+∞(−1)kU(t+ω+kω)=−limk→+∞(−1)k+1U(t+(k+1)ω)=−U∗(t), |
from this, X∗(t) and U∗(t) are the ant-periodic functions. Because
limk→+∞(−1)k+1xi(t+(k+1)ω)=x∗i(t),limk→+∞(−1)k+1yj(t+(k+1)ω)=y∗j(t), |
limk→+∞(−1)k+1ui(t+(k+1)ω)=u∗i(t),limk→+∞(−1)k+1vj(t+(k+1)ω)=v∗j(t). |
When fi(⋅) is continuous, it can be inferred from (3.12) that
{Dαtx∗i(t)=−γix∗i(t)+u∗i(t),Dαtu∗i(t)=−(αi+γ2i−γici)x∗i(t)−(ci−γi)u∗i(t)+n∑j=1aijfj(y∗j(t))+n∑j=1bijfj(y∗j(t−τij))+Ii(t),Dαty∗j(t)=−γjy∗j(t)+v∗j(t),Dαtv∗j(t)=−(βj+γ2j−γjdj)y∗j(t)−(dj−γj)v∗j(t)+n∑i=1gjifi(x∗i(t))+n∑i=1hjifi(x∗i(t−σji))+Jj(t). | (3.13) |
That is, X∗(t) and U∗(t) are the anti-periodic solutions of (2.1). This means X∗(t) is the anti-periodic solution of system (1.1). According to (3.11), we have
‖X(t)−X∗(t)‖≤2[nM(φ−φ∗)Eα(−ηtα)]12, |
where X∗(t) is with the initial value φ∗=(φ∗11(s),φ∗12(s),⋯,φ∗1n(s),ψ∗11(s),ψ∗12(s),⋯,ψ∗1n(s))T, −δ≤s≤0,δ=max{τ,σ},M(φ−φ∗)≥0,M(0)=0. Therefore, the system (1.1) has an anti-periodic solution which is Mittag-Leffler stable.
Example 1. Consider the following fractional-order inertial BAM neural networks with time-delays:
{D2αtxi(t)=−ciDαtxi(t)−αixi(t)+2∑j=1aijfj(yj(t))+2∑j=1bijfj(yj(t−τij))+Ii(t),D2αtyj(t)=−djDαtyj(t)−βjyj(t)+2∑i=1gjifi(xi(t))+2∑i=1hjifi(xi(t−σji))+Jj(t), | (4.1) |
for t\geq0 , i, j = 1, 2 . The parameters settings are as follows: \alpha = 0.85, \; f_i(x_i) = \frac{1}{50}(|x_i+1|-|x_i-1|), \; \tau_{ij} = \sigma_{ij} = 1, \; i, j = 1, 2. \; I_{1}(t) = 0.01\cos(t), \; I_{2}(t) = 0.01\cos(t), \; J_{1}(t) = 0.03\sin(t), \; J_{2}(t) = 0.01\sin(t), \alpha_1 = 2.5, \; \alpha_2 = 2.6, \; \beta_1 = 2.5, \; \beta_2 = 3, \; c_1 = 2.5, \; c_2 = 2.6, \; d_1 = 2.8, \; d_2 = 2.6, a_{11} = 0.4, \; a_{12} = 0.5, \; a_{21} = 0.5, \; a_{22} = 0.8, \; b_{11} = -0.1, \; b_{12} = 0.1, \; b_{21} = 0.2, \; b_{22} = 0.2 , g_{11} = 0.6, \; g_{12} = 0.5, \; g_{21} = 0.5, \; g_{22} = 0.6, \; h_{11} = 0.1, \; h_{12} = 0.5, \; h_{21} = 0.4, \; h_{22} = 0.2 .
We choose l_i = 1, \; i = 1, 2 , \omega = \pi, \; \gamma_1 = 1.25, \; \gamma_2 = 1.35 . After calculation, we get
\eta_1 = \min\limits_{1\leq i\leq n}\{\gamma_{i}- |\alpha_i+\gamma_i^{2}-\gamma_ic_i|, c_{i}-\gamma_{i}-1\} = 0.25 > 0, |
\eta_2 = \min\limits_{1\leq j\leq n}\{\gamma_{j}- |\beta_j+\gamma_j^{2}-\gamma_jd_j|, d_{j}-\gamma_{j}-1\} = 0.0375 > 0, |
\eta = \min\{ \min\limits_{1\leq i\leq n}\{2\gamma_{i}-|1-\alpha_i-\gamma_{i}^2+\gamma_{i}c_i|-\sum\limits_{j = 1}^n(|g_{ji}|+|h_{ji}|)l_{i}, \\ 2c_{i}-2\gamma_{i}-|1-\alpha_i-\gamma_{i}^2+\gamma_{i}c_i|-\sum\limits_{j = 1}^n(|a_{ij}|+|b_{ij}|)l_{j}\}, |
\min\limits_{1\leq j\leq n}\{2\gamma_{j}-|1-\beta_j-\gamma_{j}^2+\gamma_{j}d_j|-\sum\limits_{i = 1}^n(|a_{ij}|+|b_{ij}|)l_{j}, \\ 2d_{j}-2\gamma_{j}-|1-\beta_j-\gamma_{j}^2+\gamma_{j}d_j|-\sum\limits_{i = 1}^n(|g_{ji}|+|h_{ji}|)l_{i}\}\} = 0.4875 > 0. |
That is to say, the conditions in Theorem 3.3 are satisfied. It can be seen that system (4.1) has an anti- periodic solution with a period of \pi and it is Mittag-Leffler stable.
On the other hand, we get the state of the example through numerical simulation in Figure 1. It can be seen from the figure that it is consistent with the theoretical result of Theorem 3.3.
The fractional-order inertial neural networks considers the damping factor and can simulate more complex networks systems. Therefore, compared with fractional-order neural networks without inertia term, it has more research value in theory and application. The anti-periodic phenomena of nonlinear differential equations appear widely in biology, physics and many other fields. The fractional-order inertial BAM neural networks model is a system of nonlinear differential equations, which has a wide application background. It is important to study the existence and stability of the anti-periodic solution for the system in dynamic behaviors.
In this paper, we study the existence and Mittag-Leffler stability of anti-periodic solutions for fractional-order inertial BAM neural networks with time-delays. It is a new topic. Studying the existence and stability of the anti-periodic solution for the system has certain research value in theoretical exploration. By introducing variable substitution, the BAM neural networks model with two fractional-order derivatives of different orders is transformed into a model with only one fractional-order neural networks of the same order. Using the fractional-order calculus properties, the boundedness and Mittag-Leffler stability of the system solution are given. By constructing the sequence solution of the function for the system and applying the Ascoli-Arzela theorem, the sufficient conditions for the existence and Mittag-Leffler stability of anti-periodic solutions are obtained, and the validity of the conclusions derived in this paper is verified by numerical simulation. We give the sufficient conditions for the existence and stability of anti-periodic solutions for a class of fractional-order inertial BAM neural networks, that is, the results of Theorems 3.1–3.3. From the data we have consulted, there are no results of the study on the stability of anti-periodic solutions for fractional-order inertial BAM neural networks. Therefore, our research topic is new, and the results obtained are also new. The results provide theoretical criteria for further research on innovation and practical application of system dynamic behavior.
Using the research ideas and methods in this paper, we can further study other types of fractional-order inertial neural networks with time-delays, such as the existence and Mittag-Leffler stability of anti-periodic solutions for fractional-order inertial Cohen-Grossberg type BAM neural networks with time-delays.
The work was supported by Science Project of Zhejiang Educational Department (No. Y202145903), the Ministry of Education's Cooperative Education Project (220603284143545), Science Project of Shaoxing University (No. 2020LG1009) and Science Project of Shaoxing University Yuanpei College (KY2021C04).
The authors declare that there is no conflict of interest regarding the publication of this article.
[1] |
A. R. Aftabizadeh, S. Aizicovici, N. H. Pavel, On a class of second-order anti-periodic boundary value problems, J. Math. Anal. Appl., 171 (1992), 301–320. https://doi.org/10.1016/0022-247X(92)90345-E doi: 10.1016/0022-247X(92)90345-E
![]() |
[2] |
S. Aizicovici, M. Mckibben, S. Reich, Anti-periodic solutions to nonmonotone evolution equations with discontinuous nonlinearities, Nonlinear Anal., 43 (2001), 233–251. https://doi.org/10.1016/S0362-546X(99)00192-3 doi: 10.1016/S0362-546X(99)00192-3
![]() |
[3] |
Y. Q. Chen, J. J. Nieto, D. O'Regan, Anti-periodic solutions for fully nonlinear first-order differential equations, Math. Comput. Model., 46 (2007), 1183–1190. https://doi.org/10.1016/j.mcm.2006.12.006 doi: 10.1016/j.mcm.2006.12.006
![]() |
[4] | H. L. Chen, Antiperiodic wavelets, J. Comput. Math., 14 (1996), 32–39. |
[5] |
Y. Q. Li, L. H. Huang, Anti-periodic solutions for a class of Liénard-type systems with continuously distributed delays, Nonlinear Anal. Real World Appl., 10 (2009), 2127–2132. https://doi.org/10.1016/j.nonrwa.2008.03.020 doi: 10.1016/j.nonrwa.2008.03.020
![]() |
[6] |
Z. Q. Zhang, Z. Y. Quan, Global exponential stability via inequality technique for inertial BAM neural networks with time delays, Neurocomputing, 151 (2015), 1316–1326. https://doi.org/10.1016/j.neucom.2014.10.072 doi: 10.1016/j.neucom.2014.10.072
![]() |
[7] |
Z. Q. Zhang, W. B. Liu, D. M. Zhou, Global asymptotic stability to a generalized Cohen-Grossberg BAM neural networks of neutral type delays, Neural Networks, 25 (2012), 94–105. https://doi.org/10.1016/j.neunet.2011.07.006 doi: 10.1016/j.neunet.2011.07.006
![]() |
[8] |
Z. Q. Zhang, J. D. Cao, D. M. Zhou, Novel LMI-based condition on global asymptotic stability for a class of Cohen-Grossberg BAM networks with extended activation functions, IEEE Trans. Neural Net. Learn. Syst., 25 (2014), 1161–1172. https://doi.org/10.1109/TNNLS.2013.2289855 doi: 10.1109/TNNLS.2013.2289855
![]() |
[9] |
X. Y. Fu, F. C. Kong, Global exponential stability analysis of anti-periodic solutions of discontinuous bidirectional associative memory (BAM) neural networks with time-varying delays, Int. J. Nonlinear Sci. Numer. Simul., 21 (2020), 807–820. https://doi.org/10.1515/ijnsns-2019-0220 doi: 10.1515/ijnsns-2019-0220
![]() |
[10] |
N. Radhakrishnan, R. Kodeeswaran, R. Raja, C. Maharajan, A. Stephen, Global exponential stability analysis of anti-periodic of discontinuous BAM neural networks with time-varying delays, J. Phys. Conf. Ser., 1850 (2021), 1–20. https://doi.org/10.1088/1742-6596/1850/1/012098 doi: 10.1088/1742-6596/1850/1/012098
![]() |
[11] |
C. F. Xu, F. C. Kong, Global exponential stability of anti-periodic solutions for discontinuous Cohen-Grossberg neural networks with time-varying delays, J. Exp. Theor. Artif. Intell., 33 (2021), 263–281. https://doi.org/10.1080/0952813X.2020.1737244 doi: 10.1080/0952813X.2020.1737244
![]() |
[12] |
P. Cui, Z. B. Li, Anti-periodic solutions for BAM-type Cohen-Grossberg neural networks with time delays, J. Nonlinear Sci. Appl., 10 (2017), 2171–2180. https://doi.org/10.22436/jnsa.010.04.69 doi: 10.22436/jnsa.010.04.69
![]() |
[13] |
Y. Q. Ke, C. F. Miao, Anti-periodic solutions of inertial neural networks with time delays, Neural Process. Lett., 45 (2017), 523–538. https://doi.org/10.1007/s11063-016-9540-z doi: 10.1007/s11063-016-9540-z
![]() |
[14] |
A. Arbi, N. Tahri, C. Jammazi, C. X. Huang, J. D. Cao, Almost anti-periodic solution of inertial neural networks with leakage and time-varying delays on timescales, Circuits Syst. Signal Process., 41 (2022), 1940–1956. https://doi.org/10.1007/s00034-021-01894-4 doi: 10.1007/s00034-021-01894-4
![]() |
[15] |
Q. Cao, X. J. Guo, Anti-periodic dynamics on high-order inertial Hopfield neural networks involving time-varying delays, AIMS Math., 5 (2020), 5402–5421. https://doi.org/10.3934/math.2020347 doi: 10.3934/math.2020347
![]() |
[16] |
L. G. Yao, Q. Cao, Anti-periodicity on high-order inertial Hopfield neural networks involving mixed delays, J. Inequal. Appl., 2020 (2020), 182. https://doi.org/10.1186/s13660-020-02444-3 doi: 10.1186/s13660-020-02444-3
![]() |
[17] |
B. S. Chen, J. J. Chen, Global asymptotical \omega-periodicity of a fractional-order non-autonomous neural networks, Neural Networks, 68 (2015), 78–88. https://doi.org/10.1016/j.neunet.2015.04.006 doi: 10.1016/j.neunet.2015.04.006
![]() |
[18] |
Y. Y. Hou, L. H. Dai, S-asymptotically \omega-periodic solutions of fractional-order complex-valued recurrent neural networks with delays, IEEE Access, 9 (2021), 37883–37893. https://doi.org/10.1109/ACCESS.2021.3063746 doi: 10.1109/ACCESS.2021.3063746
![]() |
[19] |
A. L. Wu, Z. G. Zeng, Boundedness, Mittag-Leffler stability and asymptotical \omega-periodicity of fractional-order fuzzy neural networks, Neural Networks, 74 (2016), 73–84. https://doi.org/10.1016/j.neunet.2015.11.003 doi: 10.1016/j.neunet.2015.11.003
![]() |
[20] |
Y. K. Li, M. Huang, B. Li, Besicovitch almost periodic solutions for fractional-order quaternion-valued neural networks with discrete and distributed delays, Math. Methods Appl. Sci., 45 (2022), 4791–4808. https://doi.org/10.1002/mma.8070 doi: 10.1002/mma.8070
![]() |
[21] |
P. Wan, D. H. Sun, M. Zhao, H. Zhao, Monostability and multistability for almost-periodic solutions of fractional-order neural networks with unsaturating piecewise linear activation functions, IEEE Trans. Neural Net. Learn. Syst., 31 (2020), 5138–5152. https://doi.org/10.1109/TNNLS.2020.2964030 doi: 10.1109/TNNLS.2020.2964030
![]() |
[22] |
C. J. Xu, P. L. Li, On anti-periodic solutions for neutral shunting inhibitory cellular neural networks with time-varying delays and D operator, Neurocomputing, 275 (2018), 377–382. https://doi.org/10.1016/j.neucom.2017.08.030 doi: 10.1016/j.neucom.2017.08.030
![]() |
[23] |
C. X. Huang, S. G. Wen, L. H. Huang, Dynamics of anti-periodic solutions on shunting inhibitory cellular neural networks with multi-proportional delays, Neurocomputing, 357 (2019), 47–52. https://doi.org/10.1016/j.neucom.2019.05.022 doi: 10.1016/j.neucom.2019.05.022
![]() |
[24] |
Y. K. Li, J. L. Xiang, Existence and global exponential stability of anti-periodic solution for Clifford-valued inertial Cohen-Grossberg neural networks with delays, Neurocomputing, 332 (2019), 259–269. https://doi.org/10.1016/j.neucom.2018.12.064 doi: 10.1016/j.neucom.2018.12.064
![]() |
[25] | I. Podlubny, Fractional differential equations, New York: Academic Press, 1998. |
[26] |
L. Ke, Mittag-Leffler stability and asymptotic \omega-periodicity of fractional-order inertial neural networks with time-delays, Neurocomputing, 465 (2021), 53–62. https://doi.org/10.1016/j.neucom.2021.08.121 doi: 10.1016/j.neucom.2021.08.121
![]() |
[27] |
Y. J. Gu, H. Wang, Y. G. Yu, Stability and synchronization for Riemann-Liouville fractional-order time-delayed inertial neural networks, Neurocomputing, 340 (2019), 270–280. https://doi.org/10.1016/j.neucom.2019.03.005 doi: 10.1016/j.neucom.2019.03.005
![]() |
1. | Hongyun Yan, Yuanhua Qiao, Jun Miao, Zhihua Ren, Lijuan Duan, Fixed-time synchronization of delayed BAM neural networks via new fixed-time stability results and non-chattering quantized controls, 2023, 360, 00160032, 10251, 10.1016/j.jfranklin.2023.07.044 | |
2. | M. Syed Ali, Gani Stamov, Ivanka Stamova, Tarek F. Ibrahim, Arafa A. Dawood, Fathea M. Osman Birkea, Global Asymptotic Stability and Synchronization of Fractional-Order Reaction–Diffusion Fuzzy BAM Neural Networks with Distributed Delays via Hybrid Feedback Controllers, 2023, 11, 2227-7390, 4248, 10.3390/math11204248 | |
3. | Mohra Zayed, Shahid Wani, Exploring the versatile properties and applications of multidimensional degenerate Hermite polynomials, 2023, 8, 2473-6988, 30813, 10.3934/math.20231575 | |
4. | Zhiying Li, Wei Liu, The anti-periodic solutions of incommensurate fractional-order Cohen-Grossberg neural network with inertia, 2025, 10, 2473-6988, 3180, 10.3934/math.2025147 | |
5. | Wentao Wang, Wei Zeng, Wei Chen, Global exponential stability of periodic solutions for inertial delayed BAM neural networks, 2025, 145, 10075704, 108728, 10.1016/j.cnsns.2025.108728 |