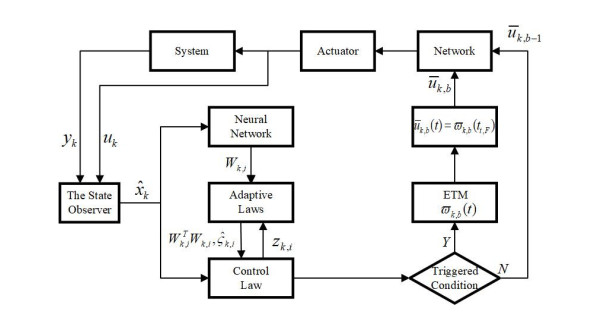
An adaptive neural network event-triggered consensus control method incorporating a state observer was proposed for a class of uncertain nonlinear multi-agent systems (MASs) with actuator failures. To begin, a state observer was constructed in an adaptive backstepping framework to estimate the MASs' unmeasurable states, and a radial basis function neural network (RBFNN) was employed to approximate the unknown nonlinear function of MASs. Meanwhile, to reduce the impact of actuator failure on the performance of MASs, the adaptive event-triggered mechanism (ETM) was designed to dynamically compensate for actuator failures, which alleviated the communication burden among individual agents by decreasing the update frequency of the control signals. Furthermore, all followers can track the leader's output signal with the synchronization errors converging to zero. Finally, simulation examples were used to verify the effectiveness of the proposed control strategy.
Citation: Kairui Chen, Yongping Du, Shuyan Xia. Adaptive state observer event-triggered consensus control for multi-agent systems with actuator failures[J]. AIMS Mathematics, 2024, 9(9): 25752-25775. doi: 10.3934/math.20241258
[1] | Zuo Wang, Hong Xue, Yingnan Pan, Hongjing Liang . Adaptive neural networks event-triggered fault-tolerant consensus control for a class of nonlinear multi-agent systems. AIMS Mathematics, 2020, 5(3): 2780-2800. doi: 10.3934/math.2020179 |
[2] | Le You, Chuandong Li, Xiaoyu Zhang, Zhilong He . Edge event-triggered control and state-constraint impulsive consensus for nonlinear multi-agent systems. AIMS Mathematics, 2020, 5(5): 4151-4167. doi: 10.3934/math.2020266 |
[3] | Jiaqi Liang, Zhanheng Chen, Zhiyong Yu, Haijun Jiang . Fixed-time consensus of second-order multi-agent systems based on event-triggered mechanism under DoS attacks. AIMS Mathematics, 2025, 10(1): 1501-1528. doi: 10.3934/math.2025070 |
[4] | Hongjie Li . H-infinity bipartite consensus of multi-agent systems with external disturbance and probabilistic actuator faults in signed networks. AIMS Mathematics, 2022, 7(2): 2019-2043. doi: 10.3934/math.2022116 |
[5] | Yuming Chen, Jie Gao, Luan Teng . Distributed adaptive event-triggered control for general linear singular multi-agent systems. AIMS Mathematics, 2023, 8(7): 15536-15552. doi: 10.3934/math.2023792 |
[6] | Hongjie Li . Event-triggered bipartite consensus of multi-agent systems in signed networks. AIMS Mathematics, 2022, 7(4): 5499-5526. doi: 10.3934/math.2022305 |
[7] | Xiaohang Su, Peng Liu, Haoran Jiang, Xinyu Yu . Neighbor event-triggered adaptive distributed control for multiagent systems with dead-zone inputs. AIMS Mathematics, 2024, 9(4): 10031-10049. doi: 10.3934/math.2024491 |
[8] | Lu Zhi, Jinxia Wu . Adaptive constraint control for nonlinear multi-agent systems with undirected graphs. AIMS Mathematics, 2021, 6(11): 12051-12064. doi: 10.3934/math.2021698 |
[9] | Qiushi Wang, Hongwei Ren, Zhiping Peng . Event-triggered impulsive control for second-order nonlinear multi-agent systems under DoS attacks. AIMS Mathematics, 2024, 9(6): 13998-14011. doi: 10.3934/math.2024680 |
[10] | Zhaohui Chen, Jie Tan, Yong He, Zhong Cao . Decentralized observer-based event-triggered control for an interconnected fractional-order system with stochastic Cyber-attacks. AIMS Mathematics, 2024, 9(1): 1861-1876. doi: 10.3934/math.2024091 |
An adaptive neural network event-triggered consensus control method incorporating a state observer was proposed for a class of uncertain nonlinear multi-agent systems (MASs) with actuator failures. To begin, a state observer was constructed in an adaptive backstepping framework to estimate the MASs' unmeasurable states, and a radial basis function neural network (RBFNN) was employed to approximate the unknown nonlinear function of MASs. Meanwhile, to reduce the impact of actuator failure on the performance of MASs, the adaptive event-triggered mechanism (ETM) was designed to dynamically compensate for actuator failures, which alleviated the communication burden among individual agents by decreasing the update frequency of the control signals. Furthermore, all followers can track the leader's output signal with the synchronization errors converging to zero. Finally, simulation examples were used to verify the effectiveness of the proposed control strategy.
Currently, many engineering problems are complex and dynamic, posing challenges for traditional single-agent systems. Multi-agent systems (MASs) address these challenges through distributed problem-solving via collaboration, competition, and division of labor. Consequently, the coherent cooperative control of MASs has become a key research focus [1,2,3,4,5,6,7]. In [1], the authors studied the coherent adaptive cooperative control of uncertain nonlinear MASs from the three uncertainties of dead zone, disturbances, and uncertain time-varying control directions. Similarly, [3] extends the investigation to include uncertainties such as unknown control directions, input unmodeled dynamics, sensor failures, and prescribed performance. In [5], a distributed control protocol is formulated through the integration of adaptive control techniques and matrix theory. The design incorporates Fourier series expansion and neural networks (NNs) to approximate uncertain nonlinearities with unmeasured periodic time-varying perturbations. A variety of industrial field agents are further reorganized into new heterogeneous MASs for cooperative control, and a review and outlook of the latest results are presented in [6]. However, all the above control methods presuppose the assumption that all states throughout the system can be measured.
The acquisition of state information is limited during the operational phase due to equipment constraints, various other variables, and environmental conditions [8,9,10,11,12,13,14]. These limitations hinder the system's tracking performance and stability. This constraint ultimately hinders the system's tracking performance and stability. In [8], heterogeneous MASs comprising numerous unmanned ground vehicles (UGVs) and unmanned aerial vehicles (UAVs) are investigated to design a fixed-time observer aimed at estimating the mismatch interference and set total uncertainty.
In [9], the aid of a fuzzy observer for estimating the unavailable states is accompanied by utilizing the characteristics of fuzzy logic systems (FLSs) to address mismatches among agents and uncertainties within the MASs. In [12], the combination of the designed NNs state observer with the introduced adaptive control algorithm addresses the system's steady-state control and transient performance. To deal with the problems arising from unknown perturbations, a low-coupling, simple structure and easy-to-implement nonlinear perturbation observer is designed in [14].
In addition, nonlinearities widely exist in engineering control systems, for instance, actuator failures are unavoidable problems that may negatively affect the stability, performance, safety, and lifetime of the system. Therefore, it is especially critical for systems that require a high degree of controllability and robustness. To cope with this problem, many scholars have proposed a multitude of innovative control schemes with adaptive backstepping control techniques as the research background [15,16,17,18,19,20,21]. In [15], an adaptive fuzzy fixed-time fault-tolerant controller was designed by utilizing the inverse step control technique and the fixed-time stabilization theory. In [16], an adaptive output feedback compensation method is proposed by considering both actuator and sensor failure. Compared with [16] Authors in [17] relaxes the assumption requirement on the nonlinear functions, and proposes a new compensation mechanism to compensate for the impact of actuator failures by employing the approach of cubic absolute value Lyapunov functions and the novel (σ,σf)-modification.
On the contrary, the aforementioned research accomplishments rely on a traditional time-triggered mechanism (TTM). The fixed sample frequency and limitations in communication bandwidth associated with TTM lead to data redundancy in network signal transmission, thus occupying communication resources. Consequently, there has been a growing interest among scholars in exploring the event-triggered mechanism (ETM)[22,23,24,25,26,27,28,29,30]. In [22], an event-triggered output feedback control method incorporating adaptive dynamic programming (ADP) with a state observer is proposed to reduce the signal transmissions on network channels, and thus control signals are updated only at specific moments where the triggering conditions are violated. In[25], researchers construct an adaptive progressive tracking control strategy with a temporal control mechanism and an event triggering mechanism with variable thresholds, and comparative experiments indicate better robustness with the latter. In [27], the combination of command filtering backstepping and adaptive event-triggered communication (e.g., when a certain threshold is reached) solves the virtual controller complexity problem while effectively avoiding the waste of communication resources. While [29] is opposite to [27,29], the preset triggered condition is established by utilizing the negative semi-definiteness of the Lyapunov function's derivative, aiming to alleviate the communication burden and conserve computational resources.
In summary, with regard to a category of uncertain nonlinear MASs with actuator failures, an adaptive NNs event-triggered consensus control strategy incorporating the state observer is proposed to reduce the impact of the system by actuator failures. In comparison to existing findings, the main innovations of the proposed control method include:
(1) Different from the existing algorithms, such as [15,16,27] an event-triggered adaptive states observation consistency control strategy is proposed for a class of uncertain nonlinear MASs with actuator failures.
(2) With regard to the actuator failures that are common in the actual industrial production system, the existing compensation programs, such as [17,18,19,20], need to occupy a large amount of communication resources. Thus, the design of an adaptive ETM to achieve dynamic compensation for actuator failures while reducing the update frequency of control signals, which reduces the occupation of communication resources, is more widespread in practical engineering.
(3) Due to the actual systems, the states of the system are often unmeasurable and the system is characterized by nonlinearities. Therefore, an adaptive NNs control method incorporating a state observer is proposed, which utilizes radial basis function neural networks (RBFNNs) to approximate MASs' unknown nonlinear functions, and the state observer is formulated for estimating the states of MASs.
The rest is presented in the following sections. Section 2 contains the problem description and preliminaries. Section 3 designs an adaptive NNs event-triggered consensus control scheme. Section 4 gives two simulation examples to verify the effectiveness of the designed scheme. Section 5 is the conclusion.
Consider the following category of uncertain nonlinear MASs [31]
{˙xk,i=xk,i+1+fk,i(Xk,i),i=1,2,…,n−1˙xk,n=uk+fk,n(Xk,n)yk=xk,1 | (2.1) |
where Xk,i=[xk,1,xk,2,…,xk,i]T and Xk,n=[xk,1,xk,2,…,xk,n]T are the state vectors, which are not measurable; fk,i(⋅) is the unknown smooth nonlinear function; uk represents the control input, while yk∈[yk,1,yk,2,…,yk,n]T denotes system output. The system consists of a leader labeled G and k(k=1,2,…,N) followers.
Assume each agent is equipped with b=1,2,…,Z,(Z>1) actuators, where uk=∑Zb=1lk,buk,b and lk,b denotes the known constant control gain. During actual operation, agents will inevitably suffer from actuator failures. The actuator failure model of b−th (1,2,…,Z) is given as follows:
{uk,b=ρk,bˉuk,b+ck,b,∀t≥tbwρk,bck,b=0 | (2.2) |
where the health factor is ρk,b∈[0,1]; ck,b and tbw are unknown constants, tbw indicates the moment of the bth actual failure. The crucial working states of the actuator operation are categorized into three situations:
1) ρk,b=0, uk,b=ck,b. The actuator is in a state of complete failure.
2) ρk,b∈(0,1), uk,b=ρk,bˉuk,b. The partial failure will occur during actuator working.
3) ρk,b=1, uk,b=ˉuk,b. The actuator works properly.
To facilitate the subsequent analysis, the work situations of the three actuators mentioned above are further summarized. Thus, two sets Lm1 and Lm2 are set and Lm1∪Lm2={1,2,…,Z}. Here, Lm1 indicates that the actuator is in a set of partial failure and normal operating states; Lm2 denotes the set in which the actuator is in a complete failure state.
Then, the MASs (2.1) is transformed into the following equation:
{˙Xk=AeXk+Hyk+n∑i=1Rk,ifk,i(Xk,i)+Rk,nukyk=QTXk | (2.3) |
where
Ae=[−hk,11⋯0⋮⋮⋱⋮−hk,n−10⋯1−hk,n0⋯0]n×nH=[hk,1⋮hk,n−1hk,n]Rk,i=[0⋯1⏟i⋯0]TRk,n=[0⋯1⏟n]TQ=[1,0,⋯,0]T |
where Ae is a strict Hurwitz matrix. Given a positive definite matrix P=PT>0, there exists a positive definite matrix O=OT>0, which satisfies ATeO+OAe=−P.
Assumption 1 ([32]). The desired trajectory ys=[ys1,ys2,…,ysn]T∈Rn has the derivative of the (n+1)−th order. There are constants s0>0 and s1>0 such that s1>s0 and |ys|≤s0≤s1.
Assumption 2 ([33]). During the operation, the system can stand Z−1 actuator failures at the same time to the greatest extent.
Lemma 1 ([34]). For any υ, ϱ∈R, the following inequality holds for
0≤|υ|−υtanh(υϱ)≤0.2785ϱ. | (2.4) |
Through a directed graph Q(F,D), the communication topology among agents including leaders and followers is presented, in which F={G,1,2,…,N} represents the set of agents and D⊆F×F denotes the set of edges. A=[akj]N×N signifies the adjacency matrix of the graph Q between agents. If there is an edge (j,k)⊆D (from agent j to agent k), which signifies that agent k can receive information from agent j, then akj>0; contrarily, akj=0. In addition, a matrix D=diag{s1,s2,.,sN}∈RN×N is obtained, in which si=∑Nj=1akj, i=1,2,…,N, and a Laplacian matrix is L=D−A∈RN×N.
By using the characteristic that NNs can arbitrarily approximate the unknown nonlinear function, the unknown nonlinear function of Q(θ) is processed, and there are
Q(θ)=ψTW(θ)+ε(θ) | (2.5) |
where W(θ)=[W1(θ),W2(θ),…,Wn(θ)]T is expressed as the basis function vector and ψ=[ψ1,ψ2,…,ψn]T signifies the ideal weight vector, in which n>0 is the number of neural nodes; there exists a positive constant ˉε denoted as the approximation error such that |ε(θ)|≤ˉε. Generally, the following basis function is selected as
Wi(θ)=−exp((θ−pi)T(θ−pi)κ2i) | (2.6) |
where i=1,2,…,n; κi signifies the width, while pi=[pi1,pi2,…,pin]T denotes the center of the Gaussian function.
Remark 1. ψ∈Rι encompasses ι unknown constants, represented by an unknown constant value ζ for ||ψ||2=ζ. By updating the norm of ψ instead of directly estimating it, thus, only the parameter ζ is estimated. This is done to minimize the estimation error ˜ζ=ζ−ˆζ, thereby reducing computational load and simplifying controller design.
Since the states of the MASs are unmeasurable, the following state observer is designed for its estimation and its expression is
{˙ˆxk,i=ˆxk,n+hi(yk−ˆxk,1)˙ˆxk,n=Z∑b=1lk,buk,b+hn(yk−ˆxk,1) | (3.1) |
where ˆXk,i=[ˆxk,1,ˆxk,2,⋯,ˆxk,i]T is the estimated value of Xk,i and ˆXk,n=[ˆxk,1,ˆxk,2,⋯,ˆxk,n]T is the estimated value of Xk,n. The observer error is ek=Xk,n−ˆXk,n, and its derivative is obtained as
˙ek=Aek+n∑q=1Bk,qfk,q. | (3.2) |
Select the following Lyapunov function:
Vk,0=eTkOkek. | (3.3) |
By taking the derivative of Vk,0, one has
˙Vk,0=eTk(ATeOk+OkAe)ek+eTkOk(n∑q=1Bk,qfk,q)=−eTkPkek+2eTkOk(n∑q=1Bk,qfk,q). | (3.4) |
According to Young's inequality and ∑ni=1WTk,0Wk,0≤1. Additionally, ζ is defined as ζ=max{n‖ψ0‖2,‖ψi‖2,i=1,…,n} and n is the number of neural nodes. Therefore, there will be ‖ψ0‖2≤ζ/n, and |εk,0|≤ˉεk,0 represents the approximate error, ˉεk,0>0.
2eTkOkn∑q=1Bk,qfk,q=2eTkOkn∑q=1Bk,q(˜ψTk,0Wk,0+εk,0)≤2λ2max(Ok)‖ek‖2+‖Ok‖2ζk+‖Ok‖2ˉε2k,0. | (3.5) |
Then, substitute (3.5) into (3.4) to get
˙Vk,0≤−Δ‖ek‖2+‖Ok‖2(ζk+ˉε2k,0) | (3.6) |
where Δ=−2(λmax(Ok))2+λmin(Pk).
The construction of virtual control laws within the backstepping control framework follows. Initially, the error system is expressed as
{zk,1=υk(yk−ys)+N∑j=1akj(yk−yj)zk,n=ˆxk,n−αk,n−1(n≥2) | (3.7) |
where zk,1 and zk,n represent a synchronization error of agent k and a virtual error, respectively; ys is the leader's output signal; υk≥0 denotes the weight vector associated with the edge from agent k to leader G. In particular, when υk=0, it means that there is no direct information exchange between agent k and leader G. Moreover, at least one agent will receive the synchronization information from leader G.
Step 1: The first Lyapunov function is constructed as follows:
Vk,1=Vk,0+12z2k,1+12rk,1˜ζ2k,1 | (3.8) |
where rk,1>0 is a constant. ˜ζk,1=ζk,1−ˆζk,1 signifies the estimation error, and ˆζk,1 represents the estimation of the uncertain parameter ζk,1. Then, from (2.1) and (3.7), the derivation of zk,1 is
˙zk,1=(υk+sk)(ek,2+zk,2+αk,1+fk,1)−˙ysυk−N∑j=1akj(xj,2+fj,1). | (3.9) |
According to (3.9), the ˙Vk,1 has
˙Vk,1=˙Vk,0+zk,1(υk+sk)(ek,2+zk,2+αk,1+fk,1)−˙ysυk−N∑j=1akj(xj,2+fj,1)−1rk,1˜ζk,1˙ˆζk,1. | (3.10) |
Utilizing Young's inequality yields
zk,1(υk+sk)ek,2≤14z2k,1(υk+sk)2+‖ek‖2. | (3.11) |
Let Qk,1(θk,1)=(υk+sk)fk,1+14zk,1(υk+sk)2−˙ysυk−∑Nj=1akj(xj,2+fj,1), the unknown nonlinear function Qk,1(θk,1) is approximated by RBFNN, and it obtained that
Qk,1(θk,1)=ψTk,1Wk,1(θk,1)+εk,1(θk,1) | (3.12) |
where θk,1=[ˆxk,1,ˆxj,1,ˆxj,2,˙ys]T. Utilizing Young's inequality yields
zk,1Qk,1(θk,1)=zk,1ψTk,1Wk,1(θk,1)+zk,1εk,1(θk,1)≤12σ2k,1z2k,1ζk,1‖Wk,1‖2+σ2k,12+z2k,12+ˉε2k,12 | (3.13) |
where ζk,1=‖ψk,1‖2, σk,1 is a positive constant to be designed, and |εk,1|≤ˉεk,1 represents the approximate error, ˉεk,1>0.
By incorporating (3.11)–(3.13) into (3.10) yields
˙Vk,1≤˙Vk,0+zk,1((υk+sk)αk,1+12σ2k,1zk,1ˆζk,1‖Wk,1‖2)+z2k,12+σ2k,12+ˉε2k,12−1rk,1˜ζk,1(˙ˆζk,1−12σ2k,1rk,1z2k,1‖Wk,1‖2)+(υk+sk)zk,1zk,2. | (3.14) |
αk,1 and ˙ˆζk,1 are designed as
αk,1=−1(υk+sk)(βk,1zk,1+12σ2k,1zk,1ˆζk,1‖Wk,1‖2), | (3.15) |
˙ˆζk,1=12σ2k,1rk,1z2k,1‖Wk,1‖2−ξk,1ˆζk,1, | (3.16) |
where βk,1>0 and ξk,1>0 are constants.
By incorporating (3.15)-(3.16) into (3.14) yields
˙Vk,1≤−Δ1‖ek‖2+M1−βk,1z2k,1+1rk,1ξk,1˜ζk,1ˆζk,1+(υk+sk)zk,1zk,2+σ2k,12+ˉε2k,12+z2k,12 | (3.17) |
where Δ1=Δ−1 and M1=‖Ok‖2(ζk+ˉε2k,0).
Step i (i=2,3,…,n−1): The Lyapunov function is constructed as
Vk,i=Vk,i−1+12z2k,i+12rk,i˜ζ2k,i, | (3.18) |
where ˜ζk,i=ζk,i−ˆζk,i signifies the estimation error, and ˆζk,i represents the estimation of the uncertain parameter ζk,i. According to (3.7), one has
˙zk,i=zk,i+1+αk,i+hi(yk−ˆxk,1)−˙αk,i−1 | (3.19) |
where
˙αk,i−1=i−1∑q=1∂αk,i−1∂ˆxk,q(ˆxk,q+1+hqek,1)+i−1∑q=1∂αk,i−1∂ˆζk,q˙ˆζk,q+∂αk,i−1∂xk,1(ek,2+ˆxk,2+fk,1)+i−1∑q=1N∑j=1∂αk,i−1∂ˆxj,q˙xj,q+i∑q=1∂αk,i−1∂y(q−1)s˙y(q)s. | (3.20) |
According to (3.19)-(3.20), one has
˙Vk,i=˙Vk,i−1+zk,i(zk,i+1+αk,i+hi(yk−ˆxk,1)−i−1∑q=1∂αk,i−1∂ˆxk,q(ˆxk,q+1+hqek,1)−i−1∑q=1∂αk,i−1∂ˆζk,q˙ˆζk,q−∂αk,i−1∂xk,1(ek,2+ˆxk,2+fk,1)−i−1∑q=1N∑j=1∂αk,i−1∂ˆxj,q˙xj,q−i∑q=1∂αk,i−1∂y(q−1)s˙y(q)s)−1rk,1˜ζk,i˙ˆζk,i. | (3.21) |
Utilizing the Young's inequality yields
−zk,i∂αk,i−1∂k,iek,2≤14z2k,i(∂αk,i−1∂k,i)2+‖ek‖2. | (3.22) |
If i=2, let Qk,i(θk,i)=hi(yk−ˆxk,1)−∑i−1q=1∂αk,i−1∂ˆxk,q(ˆxk,q+1+hqek,1) - ∑i−1q=1∂αk,i−1∂ˆζk,q˙ˆζk,q−∂αk,i−1∂xk,1(ˆxk,2+fk,1) - ∑i−1q=1∑Nj=1∂αk,i−1∂ˆxj,q˙xj,q - ∑iq=1∂αk,i−1∂y(q−1)s˙y(q)s + 14z2k,i(∂αk,i−1∂k,i)2+z2k,i−12+(υk+sk)zk,i−1. If i≥3, let Qk,i(θk,i)=hi(yk−ˆxk,1)−∑i−1q=1∂αk,i−1∂ˆxk,q (ˆxk,q+1+hqek,1)−∑i−1q=1∂αk,i−1∂ˆζk,q˙ˆζk,q− ∂αk,i−1∂xk,1(ˆxk,2+fk,1)−∑i−1q=1 ∑Nj=1∂αk,i−1∂ˆxj,q˙xj,q− ∑iq=1∂αk,i−1∂y(q−1)s˙y(q)s+14z2k,i(∂αk,i−1∂k,i)2+z2k,i−12+zk,i−1. Then, the unknown nonlinear function Qk,i(θk,i) is approximated by an RBFNN, and it is obtained that
Qk,i(θk,i)=ψTk,iWk,i(θk,i)+εk,i(θk,i) | (3.23) |
where θk,i=[ˆxTk,i,ˆxTj,i,˙ys]T. Applying Young's inequality yields
zk,iQk,i(θk,i)=zk,iψTk,iWk,i(θk,i)+zk,iεk,i(θk,i)≤12σ2k,iz2k,iζk,i‖Wk,i‖2+σ2k,i2+z2k,i2+ˉε2k,i2 | (3.24) |
where ζk,i=‖ψk,i‖2, σk,i is a positive constant to be designed, and |εk,i|≤ˉεk,i represents the approximate error, ˉεk,i>0.
By incorporating (3.22)–(3.24) into (3.21) yields
˙Vk,i≤−Δi‖ek‖2+M1+zk,1(αk,i+12σ2k,izk,1ˆζk,i‖Wk,i‖2)+z2k,i2+zk,izk,i+1−1rk,i˜ζk,i(˙ˆζk,i−12σ2k,irk,iz2k,i‖Wk,i‖2)+i∑q=1(σ2k,q2+ˉε2k,q2). | (3.25) |
αk,i and ˙ˆζk,i are designed as
αk,i=−βk,izk,i−12σ2k,izk,iˆζk,i‖Wk,i‖2, | (3.26) |
˙ˆζk,i=12σ2k,irk,iz2k,i‖Wk,i‖2−ξk,iˆζk,i, | (3.27) |
where βk,i and ξk,i are positive constants to be designed.
Further, by incorporating (3.26)-(3.27) into (3.25) yields
˙Vk,i≤−Δi‖ek‖2+M1−βk,iz2k,i+z2k,i2+zk,izk,i+1+1rk,iξk,i˜ζk,iˆζk,i+i∑q=1(σ2k,q2+ˉε2k,q2). | (3.28) |
Step n: Construct the Lyapunov function as
Vk,n=Vk,n−1+12z2k,n+12rk,n˜ζ2k,n+Z∑b=1,b∈Lm112|lk,b|ρk,b˜KTk,bΓ−1k,b˜Kk,b | (3.29) |
where rk,n is a positive constant to be designed. ˜ζk,n=ζk,n−ˆζk,n signifies the estimation error, and ˆζk,n represents the estimation of the uncertain parameter ζk,n. Γ−1k,b is the inverse matrix of Γk,b, which moreover denotes a positive definite matrix.
Since the ˙zk,n=∑Zb=1lk,b(ˉuk,bρk,b+ck,b)+hn(yk−ˆxk,1)−˙αk,n−1, it is the same as Step i.
˙αk,n−1=n−1∑q=1∂αk,n−1∂ˆxk,q(ˆxk,q+1+hqek,1)+n−1∑q=1∂αk,n−1∂ˆζk,q˙ˆζk,q+∂αk,n−1∂xk,1(ek,2+ˆxk,2+fk,1)+n−1∑q=1N∑j=1∂αk,n−1∂ˆxj,q˙xj,q+n∑q=1∂αk,n−1∂y(q−1)s˙y(q)s. | (3.30) |
The expression for Vk,n after derivation is
˙Vk,n=˙Vk,n−1+zk,n(Z∑b=1lk,b(ˉuk,bρk,b+ck,b)+hn(yk−ˆxk,1)−n−1∑q=1∂αk,n−1∂ˆxk,q(ˆxk,q+1+hqek,1)−n−1∑q=1∂αk,n−1∂ˆζk,q˙ˆζk,q−∂αk,n−1∂xk,1(ek,2+ˆxk,2+fk,1)−n−1∑q=1N∑j=1∂αk,n−1∂ˆxj,q˙xj,q−n∑q=1∂αk,n−1∂y(q−1)s˙y(q)s)−1rk,1˜ζk,n˙ˆζk,n−Z∑b=1|lk,b|ρk,b˜K−1k,bΓ−1k,b˙ˆKk,b. | (3.31) |
Utilizing Young's inequality yields
−zk,n∂αk,n−1∂k,nek,2≤14z2k,n(∂αk,n−1∂k,n)2+‖ek‖2. | (3.32) |
Let Qk,n(θk,n)=hn(yk−ˆxk,1)−∑n−1q=1∂αk,n−1∂ˆxk,q(ˆxk,q+1+hqek,1)− ∑n−1q=1∂αk,n−1∂ˆζk,q˙ˆζk,q− ∂αk,n−1∂xk,1(ˆxk,2+fk,1)−∑n−1q=1∑Nj=1∂αk,n−1∂ˆxj,q ˙xj,q−∑nq=1∂αk,n−1∂y(q−1)s˙y(q)s +14z2k,n(∂αk,n−1∂k,n)2+z2k,n−12+zk,n−1. Then, the unknown nonlinear function Qk,n(θk,n) is approximated by an RBFNN, and it is obtained that
Qk,n(θk,n)=ψTk,nWk,n(θk,n)+εk,n(θk,n) | (3.33) |
where θk,n=[ˆxTk,n,ˆxTj,n,˙ys]T. Applying Young's inequality, one has
zk,nQk,n(θk,n)=zk,nψTk,nWk,n(θk,n)+zk,nεk,n(θk,n)≤12σ2k,nz2k,nζk,n‖Wk,n‖2+σ2k,n2+z2k,n2+ˉε2k,n2 | (3.34) |
where ζk,n=‖ψk,n‖2, σk,n is a positive constant to be designed, and |εk,n|≤ˉεk,n represents the approximate error, ˉεk,n>0.
By incorporating (3.32)–(3.34) into (3.31) yields
˙Vk,n≤˙Vk,n−1+zk,nZ∑b=1lk,b(ˉuk,bρk,b+ck,b)+12σ2k,nzk,nˆζk,n‖Wk,n‖2+z2k,n2−z2k,n−12−1rk,n˜ζk,n(˙ˆζk,n−12σ2k,nrk,nz2k,n‖Wk,n‖2)+σ2k,n2+ˉε2k,n2−zk,nzk,n−1. | (3.35) |
The ETM of relative threshold is designed as follows
ϖk,b=−(1+η)[˜uk,btanh(zk,nsgn(lk,b)˜uk,bλ)+γtanh(zk,nsgn(lk,b)γλ)], | (3.36) |
{ˉuk,b(t)=ϖk,b(tk,F),tk,F≤t<tk,F+1ti,F+1=inf{t∈R||Ek,b(t)|≥η|ˉuk,b(t)|+η1} | (3.37) |
where 0<η<1, λ>0, and γ>0 are design constants; Ek,b(t)=ˉuk,b(t)−ϖk,b(t), in which ˉuk,b(t) denotes the control signal and ϖk,b(t) is the event-triggered control input; and γ>η1/(1−η) and m∈Z+. When the trigger condition |Ek,b(t)|≥η|ˉuk,b(t)|+η1 holds true, ˉuk,b(t)=ϖk,b(tk,F) is updated and keeps this value until the next event is triggered. tk,F denotes the moment when the event is triggered, tk,F>0, and F∈Z+. The control law ˜uk,b will be designed later.
For any t∈[tk,F,tk,F+1), ϖk,b(t)=(1+ηϕ1(t))ˉuk,b(t)+η1ϕ2(t), in which |ϕ1(t)|≤1 and |ϕ2(t)|≤1. Thereby, it can be further articulated as
ˉuk,b(t)=ϖk,b(t)−η1ϕ2(t)1+ηϕ1(t). | (3.38) |
Based on the uncertain actuator model and ETM, the control law ˜uk,b is constructed as follows
˜uk,b=sgn(lk,b)KTk,bH | (3.39) |
where Kk,b=[Kk,b11,Kk,b21,…,Kk,b2Z]T, Kk,b11=1∑Zb=1,b∈Lm1|lk,b|ρk,b, and H=[αk,n,1,…,1]T. Especially, if b∈Lm2, one has Kk,b2Z=−|lk,b|ck,b∑Zb=1,b∈Lm1|lk,b|ρk,b. In contrast, if b∈Lm1, one has Kk,b2Z=0.
Further, it can be inferred that
Z∑b=1,b∈Lm1zk,n|lk,b|ρk,bKTk,bHk,b+Z∑b=1,b∈Lm2zk,n|lk,b|ck,b=zk,nαk,n. | (3.40) |
Remark 2. It is noteworthy that, from Equations (3.39)–(3.40), it can be observed that ρk,b and ck,b are all unknown constants. Therefore, the value of Kk,b cannot be directly measured. To achieve the feasibility of the intermediate control law ˜uk,b, the estimation ˆKk,b is utilized to estimate the value of Kk,b, resulting in an estimation error ˜Kk,b=Kk,b−ˆKk,b.
Then, ˜uk,b is re-expressed as
˜uk,b=sgn(lk,b)ˆKTk,bH. | (3.41) |
According to (3.36), (3.38), and Lemma 1, it can be obtained that
zk,nsgn(lk,b)ˉuk,b=−zk,nsgn(lk,b)(1+η1+ϕ1η(˜uk,btanh(zk,nsgn(lk,b)˜uk,bλ)+γtanh(zk,nsgn(lk,b)γλ))+ϕ2η11+ϕ1η1)≤|zk,nsgn(lk,b)˜uk,b|−zk,nsgn(lk,b)˜uk,btanh(zk,nsgn(lk,b)˜uk,bλ)+|zk,nsgn(lk,b)γ|−zk,nsgn(lk,b)γtanh(zk,nsgn(lk,b)γλ)−|zk,nsgn(lk,b)˜uk,b|≤zk,nsgn(lk,b)˜uk,b+0.557λ. | (3.42) |
By incorporating (3.38)–(3.42) into (3.35) yields
˙Vk,n≤˙Vk,n−1+Z∑b=1,b∈Lm1zk,n|lk,b|sng(lk,b)ρk,b˜uk,b+0.557Z∑b=1,b∈Lm1|lk,b|ρk,bλ+Z∑b=1,b∈Lm2zk,nlk,bck,b−zk,nzk,n−1+12σ2k,nzk,nˆζk,n‖Wk,n‖2+z2k,n2−z2k,n2−1rk,n˜ζk,n(˙ˆζk,n−12σ2k,nrk,nz2k,n‖Wk,n‖2)+n∑q=1(σ2k,q2+ˉε2k,q2)≤˙Vk,n−1+zk,n(αk,n+12σ2k,nzk,nˆζk,n‖Wk,n‖2)−z2k,n2−zk,nzk,n−1−1rk,n˜ζk,n(˙ˆζk,n−12σ2k,nrk,nz2k,n‖Wk,n‖2)+z2k,n2+n∑q=1(σ2k,q2+ˉε2k,q2)−Z∑b=1,b∈Lm1|lk,b|˜KTk,bρk,bΓ−1k,b(˙ˆKk,b+zk,nΓk,bH)+0.557Z∑b=1,b∈Lm1|lk,b|ρk,bλ. | (3.43) |
˙ˆζk,n, ˙ˆKk,b, and αk,n are designed as
˙ˆζk,n=12σ2k,nrk,nz2k,n‖Wk,n‖2−ξk,nˆζk,n, | (3.44) |
˙ˆKk,b=−zk,nΓk,bH−gk,bΓk,bˆKk,b, | (3.45) |
αk,n=−βk,nzk,n−12σ2k,nzk,nˆζk,n‖Wk,n‖2. | (3.46) |
Substituting (3.44)–(3.46), one has
˙Vk,n≤−Δn‖ek‖2+M1−n∑q=1βk,qz2k,q+n∑q=11rk,qξk,q˜ζk,qˆζk,q+n∑q=1(σ2k,q2+ˉε2k,q2)+Z∑b=1,b∈Lm1|lk,b|ρk,bgk,b˜KTk,bˆKk,b+0.557Z∑b=1,b∈Lm1|lk,b|ρk,bλ+z2k,n2 | (3.47) |
where Δn=Δn−1−1.
Utilizing Young's inequality yields
n∑q=11rk,qξk,q˜ζk,qˆζk,q≤−n∑q=112rk,qξk,q˜ζ2k,q+n∑q=112rk,qξk,qζ2k,q, | (3.48) |
|lk,b|ρk,bgk,b˜KTk,bˆKk,b≤−12|lk,b|ρk,bgk,b˜KTk,b˜Kk,b+12|lk,b|ρk,bgk,bKTk,bKk,b. | (3.49) |
Further, combining (3.47)–(3.49) yields
˙Vk,n≤−Δn‖ek‖2−n∑q=1βk,qz2k,q−n∑q=112rk,qξk,q˜ζ2k,q−Z∑b=1,b∈Lm112|lk,b|ρk,bgk,b˜KTk,b˜Kk,b+z2k,n2+Ω | (3.50) |
where Ω=M1+∑nq=112rk,qξk,qζ2k,q+ ∑Zb=1,b∈Lm112|lk,b|ρk,bgk,bKTk,bKk,b +0.557∑Zb=1,b∈Lm1|lk,b|ρk,bλ+∑nq=1 (σ2k,q2+ˉε2k,q2).
Ultimately, it can be given as follows
˙Vk,n≤−μ1eTkOkek−μ2n−1∑q=112z2k,q−μ312z2k,n−μ4n∑q=112rk,q˜ζ2k,q−μ5Z∑b=1,b∈Lm112|lk,b|ρk,b˜KTk,bΓ−1k,b˜Kk,b+Ω, | (3.51) |
where μ1=min{2Δnλmax(Ok)}, μ2=min{2βk,q}, μ3=min{2βk,n−1}, μ4=min{ξk,q}, and μ5=min{gk,bλmax(Γ−1k,b)}. λmax(O−1k) and λmax(Γ−1k,b) are the maximum eigenvalues of O−1k and Γ−1k,b, respectively.
Hence, it could be obtained that
˙Vk,n≤−μ6Vk,n+Ω | (3.52) |
where μ6=min{μ1,μ2,μ3,μ4,μ5}. The proposed control method can be showed by Figure 1.
Theorem 1. Under the Lemma 1, Assumption 1 and Assumption 2, combining the MASs (2.1) with virtual control laws (3.15), (3.26), (3.46) and adaptive laws (3.16), (3.27), (3.44), (3.45) designed based on the ETM (3.36), (3.37) and the state observer (3.1), the following conditions are satisfied:
1) All signals within the closed-loop system remain bounded, with each agents' output following the trajectory of the virtual leader;
2) The occurrence of Zeno's phenomenon can be successfully prevented.
Proof: Multiplying both sides of Equation (3.52) by eμ6t simultaneously yields
d(Vk,neμ6t)dt≤Ωeμ6t. | (3.53) |
Then, solving the differential equation gives
12z2k,1≤Vk,n(t)≤e−μ6tVk,n(0)+Ωμ6(1−e−μ6t). | (3.54) |
Thus, z2k,1 converges exponentially to the tight set Ξ={zk,1|z2k,1≤2Ωμ6} at the rate of μ6 and can be tuned by adjusting the design parameters for Ξ.
According to the tight set Ξ it follows that the error signals are all bounded, and ys is bounded, and ζk,1 is a constant so that xk,1, ˜ζk,1, and ek are also bounded. Further, the bound of (3.7), (3.15), (3.26), and (3.46) yields that αl,1, and xk,2 are all bounded. Similarly, xk,i, ˆζk,1, ˆxk,i and αk,i are all bounded. Moreover, because ˜Kk,b=Kk,b−ˆKk,b and ˜Kk,b are bounded, ˆKk,b is also bounded. Thus, all signals within the closed-loop system are semi-global consistent and eventually bounded.
Based on the literature [35], defining the synchronization error vector Θk=[z1,1,z2,1,...,zk,1]T, one has:
‖ˉΘk‖≤‖Θk‖λmax(L+A) | (3.55) |
where ˉΘk=[ˉz1,1,ˉz2,1,...,ˉzk,1]T=Yk−ys, Yk=[y1,1,y2,1,...,yk,1]T, ys=[ys1,ys2,...,ysn]T, λmax(L+A) denotes (L+A) the maximum eigenvalue. Thus, the tracking error of the MASs converges to the following set:
|ˉzk,n|≤min{√2λmax(L+A)(Ωμ6)12}. | (3.56) |
(3.28), as well as t∈[tk,F,tk,F+1), yields
d|Ek,b|dt=sgn(Ek,b)˙Ek,b≤|˙ϖk,b|. | (3.57) |
Expressed by (3.38), ˙ϖk,b remains continuously bounded with the existence of a constant ˉϖk,b>0 ensuring, |˙ϖk,b|<ˉϖk,b. Furthermore, it can be found that limtk,m→tk,m+1Ek,b(t)=η|ˉuk,b(t)|+η1. According to the Lagrange mean value theorem, it can be obtained that:
tk,F+1−tk,F≥η|ˉuk,b(t)|+η1ˉϖk,b. | (3.58) |
Since \(t_{k, F+1} - t_{k, F} \geq t^\diamond\), \(t^\diamond\) should be guaranteed to satisfy \(t^\diamond \geq \frac{\eta |\bar u_{k, b} (t)|+ \eta_1}{\bar \varpi _{k, b}}\). Obviously, the considered system can effectively avoid the Zeno behavior.
Remark 3. Zeno's phenomenon involves an infinite number of events occurring in a finite period of time, resulting in a system requiring an infinite update. This may aggravate the computational weight of the system, making real-time control unattainable, further leading to communication overload, and possibly even causing system crashes or performance degradation. Therefore, designing event-triggered control systems that exclude the Zeno phenomenon ensures that the system maintains computational feasibility, communication efficiency, and utility in long-term operation while maintaining the desired performance and reliability.
Remark 4. Further analysis reveals that |zk,1|≤max{(2Vk,n(0))12,(2Ωμ6)12}. It is evident that the set can be adjusted by selecting the parameters to be designed, such as βk,q, βk,n, ξk,q, gk,b, and so on. Additionally, from Eqs (3.50)–(3.54), it can be observed that when ρk,b remains constant, the residual set varies with the changes in ck,b. Similarly, when ck,b remains constant, Ω increases with the rising of ρk,b, resulting in an expansion of the residual set of tracking errors.
This section aims to validate the control method's efficacy by conducting a comprehensive analysis of numerical and practical examples.
Consider a MAS with actuator failures comprising a virtual leader and three follower agents. The communication topology is illustrated in Figure 2, and the model for the follower agents are detailed as
{˙xk,1=xk,2+fk,1(Xk,1)˙xk,2=2∑b=1lk,buk,b+fk,2(Xk,2)yk=xk,1 | (4.1) |
where k=1,2,3 and selecting the nonlinear functions as fk,1=0.1(1+sin2(xk,1))xk,1 and fk,2=2.5xk,2+xk,1x2k,2; uk,b=ρk,bˉuk,b+ck,b, lk,b=1(b=1,2) unknown constants and uk,1 and uk,2 are represent system input signals. xk,1(0)=xk,2(0)=0.1 are initial states and the initial values of the state observer estimated are ˆxk,1(0)=ˆxk,2(0)=0.2. The desired tracking signal is ys=sin(2t).
The basis function for RBFNNs is designed as follows:
Wk,i(Xk,i)=−exp((Xk,i−pi)T(Xk,i−pi)κ2i) | (4.2) |
where i=1,2,…,16; the Gaussian function centered at κi has a distribution interval in [−1,1].
Other correlated parameters to be designed as η=0.2, λ=0.5, η1=0.2, γ=η1/(1−η)+0.01, σk,1=σk,2=rk,1=rk,2=ξk,1=ξk,2=0.1, hk,1=120, hk,2=602, βk,1=37, βk,2=18, gk,b=0.3, Γk,b=[1,0;0,1], ˆKk,b(0)=[0,0,0]T, and ˆζk,1(0)=ˆζk,2(0)=0.
Consider the cases of two actuator failures, which are shown as follows. Case 1: Actuator 1 of each agent keeps operating normally, and Actuator 2 of each agent loses 30% of performance after t=8s. Case 2: Each agent's Actuator 1 fails 20% of the faults throughout the run and each agent's Actuator 2 fails completely.
The simulation results from Figure 3 to Figure 8 indicate that all signals are bounded. From Figures 3(a) and 4(a), it can be observed that when facing actuator failures, every agent's output can effectively track the output signal of the virtual leader, enabling MASs to achieve signal synchronization. Figures 4 and 7 show the values of observer state and real state for two cases, with small error values between them. In Figures 5 and 8, it is evident that whether the actuator partially fails or a complete failure occurs after 8 seconds of normal operation, the updated control signal will be delivered to the system upon satisfying the event-triggered condition. This serves as compensation for the actuator failures, demonstrating the better effectiveness of the proposed control method on the system. By observing Figure 3(c), it significantly exceeds the simulation time step of 0.01s, indicating the absence of the Zeno phenomenon. The values in Table 1 elucidate that the two cases conserve a maximum of 72.60% and 77.60% of communication resources, respectively. This illustrates that, in contrast to traditional TTM, ETM offers significant conservation of communication resources.
Example | Cases | Agent 1 | Agent 2 | Agent 3 |
Example 1 | Case 1 | 72.53% | 68.33% | 63.20% |
Case 2 | 77.60% | 69.20% | 69.26% | |
Example 2 | Case 1 | 62.20% | 61.66% | 63.73% |
Case 2 | 61.66% | 62.40% | 65.60% |
The designed control method is compared with the method in the literature [24] to compare its tracking performance in the uncertain nonlinear MASs with actuator failures. From Figures 3 and 4, it can be observed that the designed state observer is effective in estimating the system state and the tracking error of each agent converges quickly to the range of the ±0.05 error band within 0.2s. The tracking performance is better than that of the literature [24], and there is no need to assume in advance that the system state is measurable at the time. Therefore, the designed control method is more general.
To assess the proposed control approach in the actual system effectiveness, reference [35] for the multi-single-link robotic arm system (k=1,2,3)
{˙xk,1=xk,2˙xk,2=∑2b=1lk,buk,b−1J(Bxk,2+mglsin(xk,1))yk=xk,1 | (4.3) |
where fk,2=−1J(Bxk,2+mglsin(xk,1)) denotes the unknown smooth nonlinear functions; uk,b=ρk,bˉuk,b+ck,b, lk,b=1(b=1,2) are unknown constants; and uk,1 and uk,2 represent system input signals. xk,1(0)=xk,2(0)=0.1 are initial states, representing joint angle and angular velocity, respectively and the initial values of the state observer's estimated state are ˆxk,1(0)=ˆxk,2(0)=0.2. The robotic arm system parameters are specified as follows: J=0.8, B=1 and mgl=10. The desired tracking signal is ys=sin(2t).
The communication topology graph and the selection of the basis functions for the cases of actuator failures are the same as for the numerical example. The other relevant parameters to be designed are as follows: η=0.1, λ=3, η1=0.5, γ=η1/(1−η)+0.001, σk,1=σk,2=rk,1=rk,2=0.1, ξk,1=ξk,2=0.5, hk,1=112, hk,2=562, βk,1=44, βk,2=16, gk,b=0.01, Γk,b=[1,0;0,1], ˆKk,b(0)=[0,0,0]T and ˆζk,1(0)=ˆζk,2(0)=0.
Figure 9 to Figure 14 and Table 1 show the simulation results of the multi-single-link robotic arm system (4.3), which is analyzed similarly to the numerical simulation. By utilizing the proposed control method, the multi-single-link robotic arm system achieves the desired tracking performance and signal bound while synchronizing the signals in the case of actuator failures subjected to the two cases respectively. Furthermore, the nonexistence of the Zeno phenomenon is guaranteed.
This research is targeted at a class of uncertain nonlinear MASs with actuator failures, whose control objective is to design an adaptive NNs event-triggered consensus control method with state observers to estimate unmeasurable states of the MASs, in order to realize the dynamic compensation of actuator failures while reducing the communication resources among the agents and avoiding Zeno phenomenon, and to make the all follower synchronization. Finally, the simulation results show the effectiveness of the control method. In future work, we will utilize the proposed method in combination with a fixed-time disturbance observer, speeding up the convergence of the system as well as directly measuring the disturbance signals, which is a valuable research work.
Kairui Chen: Conceptualization, methodology, investigation, writing - review and editing, validation, project administration; Yongping Du: methodology, Methodology, software, formal analysis, writing-review and editing, methodology; Shuyan Xia: Supervision, project administration, writing - review and editing, validation. All authors have read and approved the final version of the manuscript for publication.
The authors declare they have not used Artificial Intelligence (AI) tools in the creation of this article.
This work was supported in part by the National Natural Science Foundation of China (62103115, 52075108), in part by the Scientific and Technological Research Program of Chongqing Municipal Education Commission (KJZD-K202301203), in part by the Scientific and Technological Research Program of Wanzhou District(wzstc-20230309), in part by the Multi-dimensional Data Sensing and Intelligent Information Processing Key Laboratory Open Foundation (DWSJ2306), and in part by the Science and Technology Research Program of Guangzhou (2024404T9895, SL2023A04J00530).
The authors declare that they have no known competing financial interests or personal relationships that could have appeared to influence the work reported in this paper.
[1] |
M. Shahriari-kahkeshi, N. Meskin, Adaptive cooperative control of nonlinear multi-agent systems with uncertain time-varying control directions and dead-zone nonlinearity, Neurocomputing, 464 (2021), 151–163. https://doi.org/10.1016/j.neucom.2021.08.065 doi: 10.1016/j.neucom.2021.08.065
![]() |
[2] |
X. D. Li, Y. Z. Lv, G. H. Wen, X. H. Yu, Tracking consensus of multi-agent systems with varying number of agents under actuator attacks, IEEE Trans. Circuits Syst. II, 70 (2023), 4514–4518. https://doi.org/10.1109/TCSII.2023.3289847 doi: 10.1109/TCSII.2023.3289847
![]() |
[3] |
M. Z. Xia, Z. C. Liu, T. P. Zhang, Distributed adaptive cooperative control via command filters for multi-agent systems including input unmodeled dynamics and sensor faults, Appl. Math. Comput., 457 (2023), 128194. https://doi.org/10.1016/j.amc.2023.128194 doi: 10.1016/j.amc.2023.128194
![]() |
[4] |
G. L. Xiao, J. R. Wang, D. Shen, Adaptive fixed-time consensus for stochastic multi-agent systems with uncertain actuator faults, ISA Trans., 137 (2023), 369–378. https://doi.org/10.1016/j.isatra.2023.01.003 doi: 10.1016/j.isatra.2023.01.003
![]() |
[5] |
J. X. Chen, J. M. Li, S. Y. Liu, A. L. Zhao, Adaptive neural consensus of nonlinearly parameterized multi-agent systems with periodic disturbances, ISA Trans., 126 (2022), 160–170. https://doi.org/10.1016/j.isatra.2021.07.024 doi: 10.1016/j.isatra.2021.07.024
![]() |
[6] |
G. Y. Bao, L. F. Ma, X. J. Yi, Recent advances on cooperative control of heterogeneous multi-agent systems subject to constraints: a survey, Syst. Sci. Control Eng., 10 (2022), 539–551. https://doi.org/10.1080/21642583.2022.2074169 doi: 10.1080/21642583.2022.2074169
![]() |
[7] | J. Sun, J. X. Zhang, L. Liu, Y. M. Wu, Q. H. Shan, Output consensus control of multi-agent systems with switching networks and incomplete leader measurement, IEEE Trans. Autom. Sci. Eng., 2022, 1–10. https://doi.org/10.1109/TASE.2023.3328897 |
[8] |
W. L. Cheng, K. Zhang, B. Jiang, S. X. Ding, Fixed-fime fault-tolerant formation control for heterogeneous multi-agent systems with parameter uncertainties and disturbances, IEEE Trans. Circuits Syst. I, 68 (2021), 2121–2133. https://doi.org/10.1109/TCSI.2021.3061386 doi: 10.1109/TCSI.2021.3061386
![]() |
[9] |
Z. B. Lin, Z. Liu, C. Y. Su, Y. N. Wang, C. L. P. Chen, Y. Zhang, Adaptive fuzzy prescribed performance output-feedback cooperative control for uncertain nonlinear multiagent systems, IEEE Trans. Fuzzy Syst., 31 (2023), 4459–4470. https://doi.org/10.1109/TFUZZ.2023.3285649 doi: 10.1109/TFUZZ.2023.3285649
![]() |
[10] |
S. C. Tong, M. Xiao, Y. X. Li, Observer-based adaptive fuzzy tracking control for strict-feedback nonlinear systems with unknown control gain function, IEEE Trans. Cybern., 50 (2020), 3903–3913. https://doi.org/10.1109/TCYB.2020.2977175 doi: 10.1109/TCYB.2020.2977175
![]() |
[11] |
D. Cui, C. K. Ahn, Z. R. Xiang, Fault-tolerant fuzzy observer-based fixed-time tracking control for nonlinear switched systems, IEEE Trans. Fuzzy Syst., 31 (2023), 4410–4420. https://doi.org/10.1109/TFUZZ.2023.3284917 doi: 10.1109/TFUZZ.2023.3284917
![]() |
[12] |
G. D. Zong, Y. D. Wang, H. R. Karimi, K. B. Shi, Observer-based adaptive neural tracking control for a class of nonlinear systems with prescribed performance and input dead-zone constraints, Neural Networks, 147 (2022), 126–135. https://doi.org/10.1016/j.neunet.2021.12.019 doi: 10.1016/j.neunet.2021.12.019
![]() |
[13] |
X. Y. Chen, F. Zhao, Y. Liu, H. W. Liu, T. W. Huang, J. L. Qiu, Reduced-order observer-based preassigned finite-time control of nonlinear systems and its applications, IEEE Trans. Syst., Man, Cybern.: Syst., 53 (2023), 4205–4215. https://doi.org/10.1109/TSMC.2023.3241365 doi: 10.1109/TSMC.2023.3241365
![]() |
[14] |
Y. X. Lian, J. W. Xia, J. H. Park, W. Sun, H. Shen, Disturbance observer-based adaptive neural network output feedback control for uncertain nonlinear systems, IEEE Trans. Neural Networks Learn. Syst., 34 (2023), 7260–7270. https://doi.org/10.1109/TNNLS.2021.3140106 doi: 10.1109/TNNLS.2021.3140106
![]() |
[15] |
H. Q. Wang, J. W. Ma, X. D. Zhao, B. Niu, M. Chen, W. Wang, Adaptive fuzzy fixed-time control for high-order nonlinear systems with sensor and actuator faults, IEEE Trans. Fuzzy Syst., 31 (2023), 2658–2668. https://doi.org/10.1109/TFUZZ.2023.3235395 doi: 10.1109/TFUZZ.2023.3235395
![]() |
[16] |
C. Sun, Y. Lin, Adaptive output feedback compensation for a class of nonlinear systems with actuator and sensor failures, IEEE Trans. Syst., Man, Cybern.: Syst., 52 (2022), 4762–4771. https://doi.org/10.1109/TSMC.2021.3103908 doi: 10.1109/TSMC.2021.3103908
![]() |
[17] |
Z. Y. Ma, H. B. Kang, H. J. Ma, Adaptive output-Feedback asymptotic tracking control for a class of nonlinear systems with actuator failure, J. Franklin Inst., 359 (2022), 1881–1898. https://doi.org/10.1016/j.jfranklin.2022.01.037 doi: 10.1016/j.jfranklin.2022.01.037
![]() |
[18] |
Y. Sun, P. Shi, C. C. Lim, Adaptive consensus control for output-constrained nonlinear multi-agent systems with actuator faults, J. Franklin Inst., 359 (2022), 4216–4232. https://doi.org/10.1016/j.jfranklin.2022.03.025 doi: 10.1016/j.jfranklin.2022.03.025
![]() |
[19] |
Y. T. Cao, B. Q. Li, S. P. Wen, T. W. Huang, Consensus tracking of stochastic multi-agent system with actuator faults and switching topologies, Inf. Sci., 607 (2022), 921–930. https://doi.org/10.1016/j.ins.2022.06.009 doi: 10.1016/j.ins.2022.06.009
![]() |
[20] |
J. S. Zhao, Y. Lin, Adaptive actuator failure compensation control: a new scheme based on fault detection and isolation, IEEE/ASME Trans. Mechatron., 28 (2023), 2236–2247. https://doi.org/10.1109/TMECH.2022.3232574 doi: 10.1109/TMECH.2022.3232574
![]() |
[21] |
Y. Liu, L. Li, Adaptive leader-follower consensus control of multiple flexible manipulators with actuator failures and parameter uncertainties, IEEE/CAA J. Autom. Sin., 10 (2023), 1020–1031. https://doi.org/10.1109/JAS.2023.123093 doi: 10.1109/JAS.2023.123093
![]() |
[22] |
Y. Yang, X. Fan, W. N. Gao, W. B. Yue, A. Liu, S. C. Geng, et al., Event-triggered output feedback control for a class of nonlinear systems via disturbance observer and adaptive dynamic programming, IEEE Trans. Fuzzy Syst., 31 (2023), 3148–3160. https://doi.org/10.1109/TFUZZ.2023.3245294 doi: 10.1109/TFUZZ.2023.3245294
![]() |
[23] |
S. Al Issa, I. Kar, Event-triggered adaptive control of uncertain non-linear systems under input delay and limited resources, Int. J. Dynam. Control, 9 (2021), 1703–1710. https://doi.org/10.1007/s40435-021-00767-7 doi: 10.1007/s40435-021-00767-7
![]() |
[24] |
Y. Wang, Y. H. Yang, L. B. Wu, Adaptive fault-tolerant consensus control of multi-agent systems with event-triggered input, Inf. Sci., 650 (2023), 119594. https://doi.org/10.1016/j.ins.2023.119594 doi: 10.1016/j.ins.2023.119594
![]() |
[25] |
J. H. Wang, J. R. Liu, Y. H. Li, C. L. P. Chen, Z. Liu, F. Y. Li, Prescribed time fuzzy adaptive consensus control for multiagent systems with dead-zone input and sensor faults, IEEE Trans. Autom. Sci. Eng., 21 (2023), 4016–4027. https://doi.org/10.1109/TASE.2023.3291716 doi: 10.1109/TASE.2023.3291716
![]() |
[26] |
L. Cao, Q. Zhou, G. W. Dong, H. Y. Li, Observer-based adaptive event-triggered control for nonstrict-feedback nonlinear systems with output constraint and actuator failures, IEEE Trans. Syst., Man, Cybern.: Syst., 51 (2021), 1380–1391. https://doi.org/10.1109/TSMC.2019.2895858 doi: 10.1109/TSMC.2019.2895858
![]() |
[27] |
Y. X. Li, Z. Hou, W. W. Che, Z. G. Wu, Event-based design of finite-time adaptive control of uncertain nonlinear systems, IEEE Trans. Neural Networks Learn. Syst., 33 (2022), 3804–3813. https://doi.org/10.1109/TNNLS.2021.3054579 doi: 10.1109/TNNLS.2021.3054579
![]() |
[28] |
J. J. Chen, P. Jiang, B. S. Chen, Z. G. Zeng, Adaptive neural event-triggered consensus control for unknown nonlinear second-order delayed multi-agent systems, Neurocomputing, 598 (2024), 128067. https://doi.org/10.1016/j.neucom.2024.128067 doi: 10.1016/j.neucom.2024.128067
![]() |
[29] |
T. T. Chen, B. Niu, J. M. Zhang, D. Wang, Z. H. Wang, Time-/event-triggered adaptive neural asymptotic tracking control of nonlinear interconnected systems with unmodeled dynamics and prescribed performance, IEEE Trans. Neural Networks Learn. Syst., 34 (2023), 6557–6567. https://doi.org/10.1109/TNNLS.2021.3129228 doi: 10.1109/TNNLS.2021.3129228
![]() |
[30] |
J. H. Wang, C. Wang, Z. Liu, C. L. P. Chen, C. L. Zhang, Practical fixed-time adaptive erbfnns event-triggered control for uncertain nonlinear systems with dead-zone constraint, IEEE Trans. Syst., Man, Cybern.: Syst., 54 (2023), 342–351. https://doi.org/10.1109/TSMC.2022.3211658 doi: 10.1109/TSMC.2022.3211658
![]() |
[31] |
J. H. Wang, Y. C. Yan, Z. Liu, C. L. P. Chen, C. L. Zhang, K. R. Chen, Finite-time consensus control for multi-agent systems with full-state constraints and actuator failures, Neural Networks, 157 (2023), 350–363. https://doi.org/10.1016/j.neunet.2022.10.028 doi: 10.1016/j.neunet.2022.10.028
![]() |
[32] |
D. J. Yao, C. X. Dou, N. Zhao, T. J. Zhang, Practical fixed-time adaptive consensus control for a class of multi-agent systems with full state constraints and input delay, Neurocomputing, 446 (2021), 156–164. https://doi.org/10.1016/j.neucom.2021.03.032 doi: 10.1016/j.neucom.2021.03.032
![]() |
[33] |
J. H. Wang, Q. J. Gong, K. F. Huang, Z. Liu, C. L. P. Chen, J. Liu, Event-triggered prescribed settling time consensus compensation control for a class of uncertain nonlinear systems with actuator failures, IEEE Trans. Neural Networks Learn. Syst., 34 (2023), 5590–5600. https://doi.org/10.1109/TNNLS.2021.3129816 doi: 10.1109/TNNLS.2021.3129816
![]() |
[34] |
Y. Zhao, H. Yu, X. H. Xia, Event-triggered adaptive control of multi-agent systems with saturated input and partial state constraints, J. Franklin Inst., 359 (2022), 3333–3365. https://doi.org/10.1016/j.jfranklin.2022.04.004 doi: 10.1016/j.jfranklin.2022.04.004
![]() |
[35] |
W. Wang, D. Wang, Z. H. Peng, T. S. Li, Prescribed performance consensus of uncertain nonlinear strict-feedback systems with unknown control directions, IEEE Trans. Syst., Man, Cybern.: Syst., 46 (2016), 1279–1286. https://doi.org/10.1109/TSMC.2015.2486751 doi: 10.1109/TSMC.2015.2486751
![]() |
Example | Cases | Agent 1 | Agent 2 | Agent 3 |
Example 1 | Case 1 | 72.53% | 68.33% | 63.20% |
Case 2 | 77.60% | 69.20% | 69.26% | |
Example 2 | Case 1 | 62.20% | 61.66% | 63.73% |
Case 2 | 61.66% | 62.40% | 65.60% |