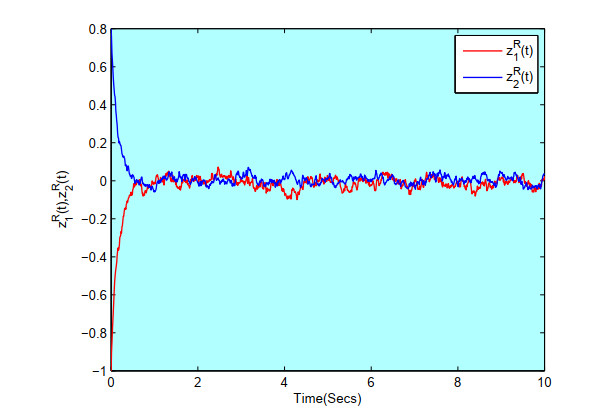
Stochastic disturbances often occur in real-world systems which can lead to undesirable system dynamics. Therefore, it is necessary to investigate stochastic disturbances in neural network modeling. As such, this paper examines the stability problem for Takagi-Sugeno fuzzy uncertain quaternion-valued stochastic neural networks. By applying Takagi-Sugeno fuzzy models and stochastic analysis, we first consider a general form of Takagi-Sugeno fuzzy uncertain quaternion-valued stochastic neural networks with time-varying delays. Then, by constructing suitable Lyapunov-Krasovskii functional, we present new delay-dependent robust and global asymptotic stability criteria for the considered networks. Furthermore, we present our results in terms of real-valued linear matrix inequalities that can be solved in MATLAB LMI toolbox. Finally, two numerical examples are presented with their simulations to demonstrate the validity of the theoretical analysis.
Citation: R. Sriraman, R. Samidurai, V. C. Amritha, G. Rachakit, Prasanalakshmi Balaji. System decomposition-based stability criteria for Takagi-Sugeno fuzzy uncertain stochastic delayed neural networks in quaternion field[J]. AIMS Mathematics, 2023, 8(5): 11589-11616. doi: 10.3934/math.2023587
[1] | R. Sriraman, P. Vignesh, V. C. Amritha, G. Rachakit, Prasanalakshmi Balaji . Direct quaternion method-based stability criteria for quaternion-valued Takagi-Sugeno fuzzy BAM delayed neural networks using quaternion-valued Wirtinger-based integral inequality. AIMS Mathematics, 2023, 8(5): 10486-10512. doi: 10.3934/math.2023532 |
[2] | Abdulaziz M. Alanazi, R. Sriraman, R. Gurusamy, S. Athithan, P. Vignesh, Zaid Bassfar, Adel R. Alharbi, Amer Aljaedi . System decomposition method-based global stability criteria for T-S fuzzy Clifford-valued delayed neural networks with impulses and leakage term. AIMS Mathematics, 2023, 8(7): 15166-15188. doi: 10.3934/math.2023774 |
[3] | Wenxiang Fang, Tao Xie . Robustness analysis of stability of Takagi-Sugeno type fuzzy neural network. AIMS Mathematics, 2023, 8(12): 31118-31140. doi: 10.3934/math.20231593 |
[4] | Rupak Datta, Ramasamy Saravanakumar, Rajeeb Dey, Baby Bhattacharya . Further results on stability analysis of Takagi–Sugeno fuzzy time-delay systems via improved Lyapunov–Krasovskii functional. AIMS Mathematics, 2022, 7(9): 16464-16481. doi: 10.3934/math.2022901 |
[5] | YeongJae Kim, YongGwon Lee, SeungHoon Lee, Palanisamy Selvaraj, Ramalingam Sakthivel, OhMin Kwon . Design and experimentation of sampled-data controller in T-S fuzzy systems with input saturation through the use of linear switching methods. AIMS Mathematics, 2024, 9(1): 2389-2410. doi: 10.3934/math.2024118 |
[6] | Zhengqi Zhang, Huaiqin Wu . Cluster synchronization in finite/fixed time for semi-Markovian switching T-S fuzzy complex dynamical networks with discontinuous dynamic nodes. AIMS Mathematics, 2022, 7(7): 11942-11971. doi: 10.3934/math.2022666 |
[7] | Tiecheng Zhang, Wei He . Global robust stability of fuzzy cellular neural networks with parameter uncertainties. AIMS Mathematics, 2024, 9(4): 8063-8078. doi: 10.3934/math.2024392 |
[8] | Li Zhu, Er-yong Cong, Xian Zhang . Global exponential stability conditions for quaternion-valued neural networks with leakage, transmission and distribution delays. AIMS Mathematics, 2023, 8(8): 19018-19038. doi: 10.3934/math.2023970 |
[9] | Ailing Li, Mengting Lv, Yifang Yan . Asymptotic stability for quaternion-valued BAM neural networks via a contradictory method and two Lyapunov functionals. AIMS Mathematics, 2022, 7(5): 8206-8223. doi: 10.3934/math.2022457 |
[10] | Chuang Liu, Jinxia Wu, Weidong Yang . Robust $ {H}_{\infty} $ output feedback finite-time control for interval type-2 fuzzy systems with actuator saturation. AIMS Mathematics, 2022, 7(3): 4614-4635. doi: 10.3934/math.2022257 |
Stochastic disturbances often occur in real-world systems which can lead to undesirable system dynamics. Therefore, it is necessary to investigate stochastic disturbances in neural network modeling. As such, this paper examines the stability problem for Takagi-Sugeno fuzzy uncertain quaternion-valued stochastic neural networks. By applying Takagi-Sugeno fuzzy models and stochastic analysis, we first consider a general form of Takagi-Sugeno fuzzy uncertain quaternion-valued stochastic neural networks with time-varying delays. Then, by constructing suitable Lyapunov-Krasovskii functional, we present new delay-dependent robust and global asymptotic stability criteria for the considered networks. Furthermore, we present our results in terms of real-valued linear matrix inequalities that can be solved in MATLAB LMI toolbox. Finally, two numerical examples are presented with their simulations to demonstrate the validity of the theoretical analysis.
Since the 1970s, different types of neural networks (NNs) have attracted substantial interest from researchers due to their potential applications in various fields including secure communications, parallel computing, artificial intelligence, signal and image processing, optimization, and others [1,2,3,4,5]. It is well known that real-world systems are subject to random factors, which influence the system dynamics. As pointed out in [6,7,8], a real nervous system is usually affected by external noise which is great uncertainty and hence may be regarded as a stochastic perturbation. Because of this, stochastic perturbations are inevitable in neural systems, and they should be considered in the modeling process [7,9,10,11,12]. Meanwhile, NNs are classified into two groups: deterministic NNs and stochastic NNs. In the study of NNs, deterministic NNs are very effective for describing and analyzing the system when there is no external disturbance [3,4,5]. Conversely, deterministic NNs fail when external disturbances occur. While stochastic NNs are very effective for describing and analyzing the system when they are subjected to external disturbances [7,8,9,10,13,14]. In recent years, stochastic NNs have drawn increasing attention from researchers and several results have been published [15,16,17,18,19,20,21].
It has been shown recently that real-valued NNs and complex-valued NNs have been successfully applied to a variety of engineering applications [1,2,11,12]. However, real-valued NNs and complex-valued NNs have some limitations when it comes to the problem of symmetry detection and high-dimensional data [22,23]. In order to address these issues, some researchers have developed quaternion-valued neural networks (QVNNs) by incorporating quaternions into conventional NNs [24,25,26,27]. Moreover, QVNNs have shown superior performance compared to complex-valued NNs and real-valued NNs because of the general representation, as well as their ability to handle multidimensional data with high efficiency. As a result of these aspects, a variety of applications have been developed including color images [28,29], signal processing [30], optimization [31], sparse representation [32], extreme learning machine [33], and so on. Recently, several research works have been published regarding various dynamics of stochastic QVNNs using the Lyapunov-Krasovskii functional (LKF) and linear matrix inequality (LMI) [34,35,36,37,38]. For example, by employing decomposition method in [34], discrete-time stochastic QVNNs with time-varying delays are discussed, and some sufficient conditions are obtained to ensure global asymptotic stability. In [35], stochastic QVNNs with event-triggered control are studied, as well as various criteria are derived for stochastic stability based on direct quaternion method. Recently, mean square exponential input-to-state stability criterion based on a real-valued decomposition was found in [38] for stochastic delayed QVNNs. There are similar results can also be found in [36,37].
Furthermore, Takagi-Sugeno (T-S) fuzzy system is a powerful and convenient tool in functional approximations for complex nonlinear systems [39,40]. The T-S fuzzy system has the advantage of being able to approximate a nonlinear system with a set of linear models. Unlike typical NN structures, T-S fuzzy NNs have fuzzy operations and they can preserve the direct connection among cells. Due to their good approximation properties, T-S fuzzy NNs have proved to be an important research topic. Many scientific papers have been proposed the idea of incorporating fuzzy logic into the NNs in order to enhance their performance [41,42,43,44,45,46]. For example, using LKFs and matrix inequality, the authors of [45] have demonstrated exponential convergence for T-S fuzzy complex-valued NNs with impulsive effects and time delays. By decomposing Clifford-valued NNs into 2mn-dimensional real-valued NNs, the authors of [46] have derived the global asymptotic stability criteria for T-S fuzzy Clifford-valued NNs with time-varying delays and impulses.
As we all know, the stability issue is the most significant problem in the field of NNs because it is a precondition for an actual system to be able to function normally, which is fundamental for solving any other issues [2,4,6]. Unfortunately, time delays are often observed when implementing NNs due to the limited switching speed of amplifiers or information processing, which may result in oscillations, divergences, and even instability in the designing systems [34,37,39]. Therefore, it is essential to study how delays affect the system's dynamics. Several theoretical studies on the stability of NNs with time delays can be found in [46,47,48]. On the other hand, parameter uncertainties also occur in real systems, as well as NNs, as a result of modeling inaccuracies and/or environmental changes, which can lead to undesirable dynamic behaviours. In this regard, it is important to ensure that the system is stable with respect to uncertainties. Recently, the robustness analysis of various uncertain systems has gained an increasing amount of attention [11,12,18,21,50].
As far as we know, no papers have been published on Takagi-Sugeno fuzzy uncertain quaternion-valued stochastic neural networks (T-S FUQVSNNs) with time-varying delays. The purpose of this study is to fill such gaps by investigating the robust and global asymptotic stability criteria for T-S FUQVSNNs. Recently, several results have been published regarding the stability of stochastic QVNNs; however, T-S FUQVSNNs have not been thoroughly explored and have not received much attention, which motivates us to investigate this topic. The main merits of this paper are:
(1) To represent more realistic dynamics of QVNNs, we present a general form of T-S FUQVSNNs with time-varying delays.
(2) We analyze the robust and global asymptotic stability criteria for T-S FUQVSNNs by employing the system decomposition method.
(3) By constructing suitable LKFs and employing integral inequalities, enhanced stability conditions for the T-S FUQVSNNs are derived in terms of real-valued LMIs, which could be verified directly by MATLAB LMI toolbox.
The paper is structured as follows: Section 2 provides the problem model, definitions of robust asymptotic stability, assumptions about activation functions and time delays, and helpful lemmas. The main results of this study are stated in Section 3; Theorem (3.1) presents the robust and global asymptotic stability criteria; Theorem (3.5) provides the global asymptotic stability criteria for the considered networks. In Corollary (3.3), (3.7), the results of stability criteria are discussed in a particular case. Section 4 discusses two numerical case studies. Section 5 shows the conclusion of this paper.
This paper uses the following notations. Let the quaternion, complex and real numbers are denoted by H,C and R, respectively. The n-dimensional quaternion, complex and real vectors are denoted by Hn,Cn and Rn, respectively. The quaternion, complex and real matrices of size n×n are represented by Hn×n,Cn×n and Rn×n, respectively. Let the matrix P<0 (P>0) means P is negative (positive) definite matrix. The block diagonal matrix is shown in diag{⋅}. PT denotes the transpose of matrix P and P∗ denotes the Hermitian transpose of matrix P. I denotes the identity matrix with appropriate dimensions. For ℓ>0, C([−ℓ,0],Hn) denotes the family of continuous functions from φ to Hn with the norm ‖φ‖=sup−ℓ≤t≤0|φ(t)|. Let (Ω,F,{F}t≥0,P) be a complete probability space with a filtration {F}t≥0 satisfying the usual conditions. The symmetric term in a matrix is showed by ✠.
The quaternion was first invented by Hamilton in 1843. The skew field of a quaternion is denoted by
z=zR+izI+jzJ+kzK∈H, |
where zR,zI,zJ,zK∈R, z is the quaternion-valued input and i,j,k are the quaternion basis which subjects to Hamilton's multiplication rules as follows:
k2=j2=i2=−1 jk=−kj=i, ki=−ik=j, ij=−ji=k. |
The following are some fundamental operation rules for quaternions and quaternion matrices [24,49].
(1) The conjugate of the quaternion as follows:
ˉz=zR−izI−jzJ−kzK∈H. |
(2) The modulus of the quaternion as follows:
|z|=√zˉz=√(zR)2+(zI)2+(zJ)2+(zK)2. |
(3) Let x=xR+ixI+jxJ+kxK∈H and y=yR+iyI+jyJ+kyK∈H. The addition and multiplication of two quaternions can be accomplished as follows:
x+y= (xR+yR)+i(xI+yI)+j(xJ+yJ)+k(xK+yK),xy= (xRyR−xIyI−xJyJ−xKyK)+i(xRyI+xIyR+xJyK−xKyJ)+j(xRyJ+xJyR−xIyK+xKyI)+k(xRyK+xKyI+xIyJ−xJyI). |
In this section, we consider the following uncertain stochastic QVNNs with time-varying delays:
dz(t)= [−(¯D+△¯D(t))z(t)+(¯A+△¯A(t))g(z(t))+(¯B+△¯B(t))g(z(t−ℓ(t)))]dt+σ(t,z(t),z(t−ℓ(t)))dω(t), | (2.1) |
where z(t)=(z1(t),z2(t),....,zn(t))T∈Hn and g(z(⋅))=(g1(z1(⋅)),g2(z2(⋅)),...,gn(zn(⋅)))T∈Hn are the state vector and neuron activation functions, respectively. ¯D=[¯di]n×n∈Rn×n, ¯A=[¯aij]n×n∈Hn×n, ¯B=[¯bij]n×n∈Hn×n are known matrices with appropriate dimensions. ω(t)=(ω1(t),ω2(t),...,ωn(t))T is n-dimensional Brownian motion defined on (Ω,F,{F}t≥0,P) with E[ω(t)]=0 and E[ω(t)2]=t. We also suppose that the stochastic disturbance σ(t,z(t),z(t−ℓ(t))):R×Hn×Hn→Hn×m is locally Lipschitz continuous and satisfies the linear growth condition, i.e., σ(t,0,0)=0, see [6,7,8,9,10] and the references therein.
This paper also makes the following assumptions about the transmission delays ℓ(t) and activation functions g(z(⋅)).
Assumption 1: ℓ(t) is bounded on R, that is, 0≤ℓ(t)≤ℓ and is differentiable function with ˙ℓ(t)≤μ<1, where ℓ,μ are constants.
Assumption 2: For any z,z′∈H, there exists a positive constant lgα such that
|gα(z)−gα(z′)|≤lgα|z−z′|, α=1,2,...,n. |
We also assume that g(0)=0.
From Assumption 2, we have
(g(z)−g(z′))∗(g(z)−g(z′))≤(z−z′)∗LTgLg(z−z′), | (2.2) |
where Lg=diag{lg1,lg2,....,lgn}.
Assumption 3: The parameter uncertainties △D(t),△¯A(t)=△¯AR(t)+i△¯AI(t)+j△¯AJ(t)+k△¯AK(t), △¯B(t)=△¯BR(t)+i△¯BI(t)+j△¯BJ(t)+k△¯BK(t) in (2.1) are assumed to satisfy: △¯D(t)=GF(t)H1, △¯AR(t)=GF(t)H2, △¯AI(t)=GF(t)H3, △¯AJ(t)=GF(t)H4, △¯AK(t)=GF(t)H5, △¯BR(t)=GF(t)H6, △¯BI(t)=GF(t)H7, △¯BJ(t)=GF(t)H8, △¯BK(t)=GF(t)H9, where G and Hα, α=1,2,...,9 are constant matrices and F(t) is the time-varying uncertain matrix satisfies FT(t)F(t)≤I.
The initial condition of the NNs (2.1) is given by
z(t)=φ(t), t∈[−ℓ,0], | (2.3) |
where φ(t) is continuously differential on t∈[−ℓ,0].
As shown in [39,40,41,42], this paper presents a class of T-S FUQVSNNs with time-varying delays based on the T-S fuzzy models as follows.
Plant Rule a: IF ϑ1(t) is ηa1 and ϑ2(t) is ηa2 and... and ϑg(t) is ηag, THEN
dz(t)= [−(¯Da+△¯Da(t))z(t)+(¯Aa+△¯Aa(t))g(z(t))+(¯Ba+△¯Ba(t))g(z(t−ℓ(t)))]dt+σa(t,z(t),z(t−ℓ(t)))dω(t), | (2.4) |
where ϑc(t) (c=1,...,g) is the premise variables vector; ηac (a=1,...,m; c=1,...,g) is the fuzzy set, and m is the number of If-Then rules.
By inferring from the fuzzy models, the final output of T-S FUQVSNNs can be obtained as follows
dz(t)= m∑a=1ψa(ϑ(t)){−(¯Da+△¯Da(t))z(t)+(¯Aa+△¯Aa(t))g(z(t))+(¯Ba+△¯Ba(t))g(z(t−ℓ(t)))dt+σa(t,z(t),z(t−ℓ(t)))dω(t)}m∑a=1ψa(ϑ(t)), | (2.5) |
or equivalently
dz(t)= m∑a=1χa(ϑ(t)){−(¯Da+△¯Da(t))z(t)+(¯Aa+△¯Aa(t))g(z(t))+(¯Ba+△¯Ba(t))g(z(t−ℓ(t)))dt+σa(t,z(t),z(t−ℓ(t)))dω(t)}, | (2.6) |
where ϑ(t)=(ϑ1(t),...,ϑg(t))T, χa(ϑ(t))=ψa(ϑ(t))m∑a=1ψa(ϑ(t)), and ψa(ϑ(t))=g∏c=1ηac(ϑ(t)). The term ηac(ϑc(t)) is the grade membership of ϑc(t) in ηac. It is stated that ψa(ϑ(t))≥0, a=1,...,m and m∑a=1ψa(ϑ(t))>0 for all t≥0. By fuzzy set theory, we have χa(ϑ(t))≥0, a=1,...,m and m∑a=1χa(ϑ(t))=1 for all t≥0.
Assumption 4: For z=zR+izI+jzJ+kzK, ˆz=ˆzR+iˆzI+jˆzJ+kˆzK, with zR,zI,zJ,zK, ˆzR,ˆzI,ˆzJ,ˆzK∈Rn, σa(t,z,ˆz) is defined as
σa(t,z,ˆz)= σRa(t,zR,zI,zJ,zK,ˆzR,ˆzI,ˆzJ,ˆzK)+iσIa(t,zR,zI,zJ,zK,ˆzR,ˆzI,ˆzJ,ˆzK)+jσJa(t,zR,zI,zJ,zK,ˆzR,ˆzI,ˆzJ,ˆzK)+kσKa(t,zR,zI,zJ,zK,ˆzR,ˆzI,ˆzJ,ˆzK), | (2.7) |
where σRa,σIa,σJa,σKa:R+×Rn×Rn×Rn×Rn×Rn×Rn×Rn×Rn→Rn×m. There exist matrices ¯Uαa≥0,¯Vαa≥0,¯Mαa≥0,¯Nαa≥0, α=1,2,...,8 such that
trace{σRa(t,zR,zI,zJ,zK,ˆzR,ˆzI,ˆzJ,ˆzK)TσRa(t,zR,zI,zJ,zK,ˆzR,ˆzI,ˆzJ,ˆzK)}≤ (zR)T¯U1a(zR)+(zI)T¯U2a(zI)+(zJ)T¯U3a(zJ)+(zK)T¯U4a(zK)+(ˆzR)T¯U5a(ˆz)R+(ˆzI)T¯U6a(ˆzI)+(ˆzJ)T¯U7a(ˆzJ)+(ˆzK)T¯U8a(ˆzK),trace{σIa(t,zR,zI,zJ,zK,ˆzR,ˆzI,ˆzJ,ˆzK)TσIa(t,zR,zI,zJ,zK,ˆzR,ˆzI,ˆzJ,ˆzK)}≤ (zR)T¯V1a(zR)+(zI)T¯V2a(zI)+(zJ)T¯V3a(zJ)+(zK)T¯V4a(zK)+(ˆzR)T¯V5a(ˆz)R+(ˆzI)T¯V6a(ˆzI)+(ˆzJ)T¯V7a(ˆzJ)+(ˆzK)T¯V8a(ˆzK),trace{σJa(t,zR,zI,zJ,zK,ˆzR,ˆzI,ˆzJ,ˆzK)TσJa(t,zR,zI,zJ,zK,ˆzR,ˆzI,ˆzJ,ˆzK)}≤ (zR)T¯M1a(zR)+(zI)T¯M2a(zI)+(zJ)T¯M3a(zJ)+(zK)T¯M4a(zK)+(ˆzR)T¯M5a(ˆz)R+(ˆzI)T¯M6a(ˆzI)+(ˆzJ)T¯M7a(ˆzJ)+(ˆzK)T¯M8a(ˆzK),trace{σKa(t,zR,zI,zJ,zK,ˆzR,ˆzI,ˆzJ,ˆzK)TσKa(t,zR,zI,zJ,zK,ˆzR,ˆzI,ˆzJ,ˆzK)}≤ (zR)T¯N1a(zR)+(zI)T¯N2a(zI)+(zJ)T¯N3a(zJ)+(zK)T¯N4a(zK)+(ˆzR)T¯N5a(ˆz)R+(ˆzI)T¯N6a(ˆzI)+(ˆzJ)T¯N7a(ˆzJ)+(ˆzK)T¯N8a(ˆzK). |
To further investigate, we define z(t)=zR(t)+izI(t)+jzJ(t)+kzK(t), ¯A=¯AR+i¯AI+j¯AJ+k¯AK, ¯B=¯BR+i¯BI+j¯BJ+k¯BK, g(z(t))=gR(zR(t),zI(t),zJ(t),zK(t))+igI(zR(t),zI(t),zJ(t),zK(t))+jgJ(zR(t),zI(t),zJ(t),zK(t))+kgK(zR(t),zI(t),zJ(t),zK(t)), g(z(t−ℓ(t)))=gR(zR(t−ℓ(t)),zI(t−ℓ1(t)),zJ(t−ℓ(t)),zK(t−ℓ(t)))+igI(zR(t−ℓ(t)),zI(t−ℓ(t)),zJ(t−ℓ(t)),zK(t−ℓ(t)))+jgJ(zR(t−ℓ(t)),zI(t−ℓ(t)),zJ(t−ℓ(t)),zK(t−ℓ(t)))+kgK(zR(t−ℓ(t)),zI(t−ℓ(t)),zJ(t−ℓ(t)),zK(t−ℓ(t))).\\ In order to simplify the resulting parts, the following notations are used:
zR=zR(t), zI=zI(t), zJ=zJ(t), zK=zK(t), zRℓ(t)=zR(t−ℓ(t)), zIℓ(t)=zI(t−ℓ(t)), zJℓ(t)=zJ(t−ℓ(t)), zKℓ(t)=zK(t−ℓ(t)).
Hence, the T-S FUQVSNNs (2.6) can be splitting into real and imaginary parts as
{dzR=m∑a=1χa(ϑ(t)){[−(¯Da+△¯Da(t))zR+(¯ARa+△¯ARa(t))gR(zR,zI,zJ,zK)−(¯AIa+△¯AIa(t))gI(zR,zI,zJ,zK)−(¯AJa+△¯AJa(t))gJ(zR,zI,zJ,zK)−(¯AKa+△¯AKa(t))gK(zR,zI,zJ,zK)+(¯BRa+△¯BRa(t))gR(zRℓ(t),zIℓ(t),zJℓ(t),zKℓ(t))−(¯BIa+△¯BIa(t))gI(zRℓ(t),zIℓ(t),zJℓ(t),zKℓ(t))−(¯BJa+△¯BJa(t))gJ(zRℓ(t),zIℓ(t),zJℓ(t),zKℓ(t))−(¯BKa+△¯BKa(t))gK(zRℓ(t),zIℓ(t),zJℓ(t),zKℓ(t))]dt+σRa(t,zR,zI,zJ,zK,zRℓ(t),zIℓ(t),zJℓ(t),zKℓ(t))dω(t)}dzI=m∑a=1χa(ϑ(t)){[−(¯Da+△¯Da(t))zI+(¯ARa+△¯ARa(t))gI(zR,zI,zJ,zK)+(¯AIa+△¯AIa(t))gR(zR,zI,zJ,zK)+(¯AJa+△¯AJa(t))gK(zR,zI,zJ,zK)−(¯AKa+△¯AKa(t))gJ(zR,zI,zJ,zK)+(¯BRa+△¯BRa(t))gI(zRℓ(t),zIℓ(t),zJℓ(t),zKℓ(t))+(¯BIa+△¯BIa(t))gR(zRℓ(t),zIℓ(t),zJℓ(t),zKℓ(t))+(¯BJa+△¯BJa(t))gK(zRℓ(t),zIℓ(t),zJℓ(t),zKℓ(t))−(¯BKa+△¯BKa(t))gJ(zRℓ(t),zIℓ(t),zJℓ(t),zKℓ(t))]dt+σIa(t,zR,zI,zJ,zK,zRℓ(t),zIℓ(t),zJℓ(t),zKℓ(t))dω(t)}dzJ=m∑a=1χa(ϑ(t)){[−(¯Da+△¯Da(t))zJ+(¯ARa+△¯ARa(t))gJ(zR,zI,zJ,zK)+(¯AJa+△¯AJa(t))gR(zR,zI,zJ,zK)−(¯AIa+△¯AIa(t))gK(zR,zI,zJ,zK)+(¯AKa+△¯AKa(t))gI(zR,zI,zJ,zK)+(¯BRa+△¯BRa(t))gJ(zRℓ(t),zIℓ(t),zJℓ(t),zKℓ(t))+(¯BJa+△¯BJa(t))gR(zRℓ(t),zIℓ(t),zJℓ(t),zKℓ(t))−(¯BIa+△¯BIa(t))gK(zRℓ(t),zIℓ(t),zJℓ(t),zKℓ(t))+(¯BKa+△¯BKa(t))gI(zRℓ(t),zIℓ(t),zJℓ(t),zKℓ(t))]dt+σJa(t,zR,zI,zJ,zK,zRℓ(t),zIℓ(t),zJℓ(t),zKℓ(t))dω(t)}dzK=m∑a=1χa(ϑ(t)){[−(¯Da+△¯Da(t))zK+(¯ARa+△¯ARa(t))gK(zR,zI,zJ,zK)+(¯AKa+△¯AKa(t))gR(zR,zI,zJ,zK)+(¯AIa+△¯AIa(t))gJ(zR,zI,zJ,zK)−(¯AJa+△¯AJa(t))gI(zR,zI,zJ,zK)+(¯BRa+△¯BRa(t))gK(zRℓ(t),zIℓ(t),zJℓ(t),zKℓ(t))+(¯BKa+△¯BKa(t))gR(zRℓ(t),zIℓ(t),zJℓ(t),zKℓ(t))+(¯BIa+△¯BIa(t))gJ(zRℓ(t),zIℓ(t),zJℓ(t),zKℓ(t))−(¯BJa+△¯BJa(t))gI(zRℓ(t),zIℓ(t),zJℓ(t),zKℓ(t))]dt+σKa(t,zR,zI,zJ,zK,zRℓ(t),zIℓ(t),zJℓ(t),zKℓ(t))dω(t)}. | (2.8) |
Based on (2.8), the following NNs can be written as:
{[dzRdzIdzJdzK]=m∑a=1χa(ϑ(t)){(−[¯Da+△¯Da(t)0000¯Da+△¯Da(t)0000¯Da+△¯Da(t)0000¯Da+△¯Da(t)][zRzIzJzK]+[¯ARa+△¯ARa(t)−¯AIa−△¯AIa(t)−¯AJa−△¯AJa(t)−¯AKa−△¯AKa(t)¯AIa+△¯AIa(t)¯ARa+△¯ARa(t)−¯AKa−△¯AKa(t)¯AJa+△¯AJa(t)¯AJa+△¯AJa(t)¯AKa+△¯AKa(t)¯ARa+△¯ARa(t)−¯AIa−△¯AIa(t)¯AKa+△¯AKa(t)−¯AJa−△¯AJa(t)¯AIa+△¯AIa(t)¯ARa+△¯ARa(t)][gR(zR,zI,zJ,zK)gI(zR,zI,zJ,zK)gJ(zR,zI,zJ,zK)gK(zR,zI,zJ,zK)]+[¯BRa+△¯BRa(t)−¯BIa−△¯BIa(t)−¯BJa−△¯BJa(t)−¯BKa−△¯BKa(t)¯BIa+△¯BIa(t)¯BRa+△¯BRa(t)−¯BKa−△¯BKa(t)¯BJa+△¯BJa(t)¯BJa+△¯BJa(t)¯BKa+△¯BKa(t)¯BRa+△¯BRa(t)−¯BIa−△¯BIa(t)¯BKa+△¯BKa(t)−¯BJa−△¯BJa(t)¯BIa+△¯BIa(t)¯BRa+△¯BRa(t)][gR(zRℓ(t),zIℓ(t),zJℓ(t),zKℓ(t))gI(zRℓ(t),zIℓ(t),zJℓ(t),zKℓ(t))gJ(zRℓ(t),zIℓ(t),zJℓ(t),zKℓ(t))gK(zRℓ(t),zIℓ(t),zJℓ(t),zKℓ(t))])dt+[σRa(t,zR,zI,zJ,zK,zRℓ(t),zIℓ(t),zJℓ(t),zKℓ(t))σIa(t,zR,zI,zJ,zK,zRℓ(t),zIℓ(t),zJℓ(t),zKℓ(t))σJa(t,zR,zI,zJ,zK,zRℓ(t),zIℓ(t),zJℓ(t),zKℓ(t))σKa(t,zR,zI,zJ,zK,zRℓ(t),zIℓ(t),zJℓ(t),zKℓ(t))]dω(t)}, | (2.9) |
which is equivalent to
{[dzRdzIdzJdzK]=m∑a=1χa(ϑ(t)){(−([¯Da0000¯Da0000¯Da0000¯Da]+[△¯Da(t)0000△¯Da(t)0000△¯Da(t)0000△¯Da(t)])[zRzIzJzK]+([¯ARa−¯AIa−¯AJa−¯AKa¯AIa¯ARa−¯AKa¯AJa¯AJa¯AKa¯ARa−¯AIa¯AKa−¯AJa¯AIa¯ARa]+[△¯ARa(t)−△¯AIa(t)−△¯AJa(t)−△¯AKa(t)△¯AIa(t)△¯ARa(t)−△¯AKa(t)△¯AJa(t)△¯AJa(t)△¯AKa(t)△¯ARa(t)−△¯AIa(t)△¯AKa(t)−△¯AJa(t)△¯AIa(t)△¯ARa(t)])[gR(zR,zI,zJ,zK)gI(zR,zI,zJ,zK)gJ(zR,zI,zJ,zK)gK(zR,zI,zJ,zK)]+([¯BRa−¯BIa−¯BJa−¯BKa¯BIa¯BRa−¯BKa¯BJa¯BJa¯BKa¯BRa−¯BIa¯BKa−¯BJa¯BIa¯BRa]+[△¯BRa(t)−△¯BIa(t)−△¯BJa(t)−△¯BKa(t)△¯BIa(t)△¯BRa(t)−△¯BKa(t)△¯BJa(t)△¯BJa(t)△¯BKa(t)△¯BRa(t)−△¯BIa(t)△¯BKa(t)−△¯BJa(t)△¯BIa(t)△¯BRa(t)])[gR(zRℓ(t),zIℓ(t),zJℓ(t),zKℓ(t))gI(zRℓ(t),zIℓ(t),zJℓ(t),zKℓ(t))gJ(zRℓ(t),zIℓ(t),zJℓ(t),zKℓ(t))gK(zRℓ(t),zIℓ(t),zJℓ(t),zKℓ(t))])dt+[σRa(t,zR,zI,zJ,zK,zRℓ(t),zIℓ(t),zJℓ(t),zKℓ(t))σIa(t,zR,zI,zJ,zK,zRℓ(t),zIℓ(t),zJℓ(t),zKℓ(t))σJa(t,zR,zI,zJ,zK,zRℓ(t),zIℓ(t),zJℓ(t),zKℓ(t))σKa(t,zR,zI,zJ,zK,zRℓ(t),zIℓ(t),zJℓ(t),zKℓ(t))]dω(t)}. | (2.10) |
Let
ˆg(π(t))= [gR(zR,zI,zJ,zK)gI(zR,zI,zJ,zK)gJ(zR,zI,zJ,zK)gK(zR,zI,zJ,zK)], ˆg(π(t−ℓ(t)))=[gR(zRℓ(t),zIℓ(t),zJℓ(t),zKℓ(t))gI(zRℓ(t),zIℓ(t),zJℓ(t),zKℓ(t))gJ(zRℓ(t),zIℓ(t),zJℓ(t),zKℓ(t))gK(zRℓ(t),zIℓ(t),zJℓ(t),zKℓ(t))], |
π(t)= [zRzIzJzK], ˆDa=[¯Da0000¯Da0000¯Da0000¯Da], ˆAa=[¯ARa−¯AIa−¯AJa−¯AKa¯AIa¯ARa−¯AKa¯AJa¯AJa¯AKa¯ARa−¯AIa¯AKa−¯AJa¯AIa¯ARa],ˆBa= [¯BRa−¯BIa−¯BJa−¯BKa¯BIa¯BRa−¯BKa¯BJa¯BJa¯BKa¯BRa−¯BIa¯BKa−¯BJa¯BIa¯BRa], ςa(t)=[σRa(t,zR,zI,zJ,zK,zRℓ(t),zIℓ(t),zJℓ(t),zKℓ(t))σIa(t,zR,zI,zJ,zK,zRℓ(t),zIℓ(t),zJℓ(t),zKℓ(t))σJa(t,zR,zI,zJ,zK,zRℓ(t),zIℓ(t),zJℓ(t),zKℓ(t))σKa(t,zR,zI,zJ,zK,zRℓ(t),zIℓ(t),zJℓ(t),zKℓ(t))],△ˆDa= [△¯Da(t)0000△¯Da(t)0000△¯Da(t)0000△¯Da(t)],△ˆAa= [△¯ARa(t)−△¯AIa(t)−△¯AJa(t)−△¯AKa(t)△¯AIa(t)△¯ARa(t)−△¯AKa(t)△¯AJa(t)△¯AJa(t)△¯AKa(t)△¯ARa(t)−△¯AIa(t)△¯AKa(t)−△¯AJa(t)△¯AIa(t)△¯ARa(t)],△ˆBa= [△¯BRa(t)−△¯BIa(t)−△¯BJa(t)−△¯BKa(t)△¯BIa(t)△¯BRa(t)−△¯BKa(t)△¯BJa(t)△¯BJa(t)△¯BKa(t)△¯BRa(t)−△¯BIa(t)△¯BKa(t)−△¯BJa(t)△¯BIa(t)△¯BRa(t)]. |
Now, the system (2.10) is equivalent form as
dπ(t)=m∑a=1χa(ϑ(t)){[−(ˆDa+△ˆDa)π(t)+(ˆAa+△ˆAa)ˆg(π(t))+(ˆBa+△ˆBa)ˆg(π(t−ℓ(t)))]dt+ςa(t)dω(t)}. | (2.11) |
From (2.11), the parameter uncertainties △ˆDa,△ˆAa,△ˆBa, which satisfy:
△ˆDa=ˆGaˆFa(t)ˆH1a, △ˆAa=ˆGaˆFa(t)ˆH2a, △ˆBa=ˆGaˆFa(t)ˆH3a, | (2.12) |
where
ˆGa= [Ga0000Ga0000Ga0000Ga], ˆFa(t)=[Fa(t)0000Fa(t)0000Fa(t)0000Fa(t)],ˆH1a= [H1a0000H1a0000H1a0000H1a], ˆH2a=[H2a−H3a−H4a−H5aH3aH2a−H5aH4aH4aH5aH2a−H3aH5a−H4aH3aH2a],ˆH3a= [H6a−H7a−H8a−H9aH7aH6a−H9aH8aH8aH9aH6a−H7aH9a−H8aH7aH6a]. |
The initial condition of the system (2.11) is given by
π(t)=ˆφ(t),t∈[−ℓ,0], | (2.13) |
where ˆφ(t)=(φR(t) φI(t) φJ(t) φK(t))T.
To simplify the sequel, the following abbreviations are used
θa(t)= −(ˆDa+△ˆDa)π(t)+(ˆAa+△ˆAa)ˆg(π(t))+(ˆBa+△ˆBa)ˆg(π(t−ℓ(t))). |
The system (2.11) read as
dπ(t)= m∑a=1χa(ϑ(t))θa(t)dt+m∑a=1χa(ϑ(t))ςa(t)dω(t). | (2.14) |
In order to derive our main results, we present some definitions, lemmas.
Definition 2.1. [50] The NN model (2.1) is said to be mean-square stable if for any ϵ>0 there exists a scalar κ(ϵ)>0 such that E{‖z(t)‖2}<ϵ, t>0, whenever sup−ℓ≤t≤0E{‖φ(t)‖2}<κ(ϵ). In addition, if limt→∞E{‖z(t)‖2}=0 for any initial condition, then the NNs (2.1) is said to be mean-square robustly asymptotically stable.
Lemma 2.2. [51] Let M∈Rn×n be a positive definite matrix, vector function z(s):[a,b]→Rn with scalars a<b, then
−(b−a)∫bazT(s)Mz(s)ds≤−[∫baz(s)ds]TM[∫baz(s)ds]. |
Lemma 2.3. [51] Let Ω=ΩT, J1 and J2 be real matrices, F(t) satisfies FT(t)F(t)≤I. Then Ω+(J1F(t)J2)+(J1F(t)J2)T<0, iff there exist a scalar ϵ>0 such that Ω+ϵJ1JT1+ϵ−1JT2J2<0.
Lemma 2.4. [51] Given constant matrices M,N and O with 0<M=MT and 0<N=NT, then [MOOTN]<0, is equivalent to one of the following conditions: (i) N<0, M−ON−1OT< 0, (ii) M<0, N−OTM−1O< 0.
Lemma 2.5. [52] Let M∈Rn×n be a positive definite matrix, two matrices Λ1,Λ2∈Rn×m, positive integers n and m, scalar ζ∈(0,1), any vector ξ∈Rm, denote the function Ξ(ζ,M) with the following form:
Ξ(ζ,M)=1ζξTΛT1MΛ1ξ+11−ζξTΛT2MΛ2ξ. |
There exists a matrix N∈Rn×n satisfying [MNNTM]>0, then
minζ∈(0,1)Ξ(ζ,M)≥[Λ1ξΛ2ξ]T[MNNTM][Λ1ξΛ2ξ]. |
This section derives sufficient criteria for the robust and global asymptotic stability criteria for T-S FUQVSNNs using the Lyapunov stability theory and LMI method.
In the following Theorem (3.1), we derive the mean square robust asymptotic stability criteria for T-S FUQVSNNs (2.11).
Theorem 3.1. Suppose Assumptions 1-4 hold. If there exist positive symmetric matrices P∈R4n×4n,Q∈R4n×4n,R∈R4n×4n,S∈R4n×4n, any matrix T∈R4n×4n and positive scalars ϵ1∈Rn,ϵ2∈Rn,ϵ3∈Rn,γ1∈Rn,γ2∈Rn,λ∈Rn, such that the following LMIs hold for all a=1,2,...,m
P≤ λI, | (3.1) |
[ˆΦaΥa✠Ωa]< 0, | (3.2) |
where ˆΦa=(ˆΦi,j,a)7×7, Υa=(Υi,j,a)7×3, Ωa=(Ωi,j,a)3×3 with ˆΦ1,1,a=−PˆDa−ˆDaPT+ϵ1ˆH1aTˆH1a+Q+R+ℓ2S+γ1ˆLg+λΠ1, ˆΦ1,4,a=PˆAa, ˆΦ1,5,a=PˆBa, ˆΦ2,2,a=−(1−μ)Q+γ2ˆLg+λΠ2, ˆΦ3,3,a=−R, ˆΦ4,4,a=−γ1+ϵ2ˆH2aTˆH2a, ˆΦ5,5,a=−γ2+ϵ3ˆH3aTˆH3a, ˆΦ6,6,a=−S, ˆΦ6,7,a=−T, ˆΦ7,7,a=−S, Υ1,1,a=PˆGa, Υ1,2,a=PˆGa, Υ1,3,a=PˆGa, Ω1,1,a=−ϵ1, Ω2,2,a=−ϵ2, Ω3,3,a=−ϵ3, then the NN model (2.11) is robustly asymptotically stable in the mean square.
Proof: Take the following LKF (3.3) for the NNs (2.11)
V(t,π(t),a)= πT(t)Pπ(t)+∫tt−ℓ(t)πT(s)Qπ(s)ds+∫tt−ℓπT(s)Rπ(s)ds+ℓ∫0−ℓ∫tt+uπT(s)Sπ(s)dsdu. | (3.3) |
Suppose L is the weak infinitesimal generator. By Ito's formula, the time derivative of V(t,π(t),a) can be calculated along the trajectories of the system (2.11) is given by
LV(t,π(t),a)= m∑a=1χa(ϑ(t)){2πT(t)Pθa(t)+trace{ςTa(t)Pςa(t)}+πT(t)Qπ(t)−(1−˙ℓ(t))πT(t−ℓ(t))Qπ(t−ℓ(t))+πT(t)Rπ(t)−πT(t−ℓ)Rπ(t−ℓ)+ℓ2πT(t)Sπ(t)−ℓ∫tt−ℓπT(u)Sπ(u)du}= m∑a=1χa(ϑ(t)){2πT(t)P[−(ˆDa+△ˆDa)π(t)+(ˆAa+△ˆAa)ˆg(π(t))+(ˆBa+△ˆBa)ˆg(π(t−ℓ(t)))]+trace{ςTa(t)Pςa(t)}+πT(t)Qπ(t)−(1−˙ℓ(t))πT(t−ℓ(t))Qπ(t−ℓ(t))+πT(t)Rπ(t)−πT(t−ℓ)Rπ(t−ℓ)+ℓ2πT(t)Sπ(t)−ℓ∫tt−ℓπT(u)Sπ(u)du}≤ m∑a=1χa(ϑ(t)){−2πT(t)(PˆDa)π(t)−2πT(t)(PˆGaˆFa(t)ˆH1a)π(t)+2πT(t)(PˆAa)ˆg(π(t))+2πT(t)(PˆGaˆFa(t)ˆH2a)ˆg(π(t))+2πT(t)(PˆBa)ˆg(π(t−ℓ(t)))+2πT(t)(PˆGaˆFa(t)ˆH3a)ˆg(π(t−ℓ(t)))+trace{ςTa(t)Pςa(t)}+πT(t)Qπ(t)−(1−μ)πT(t−ℓ(t))Q×π(t−ℓ(t))+πT(t)Rπ(t)−πT(t−ℓ)Rπ(t−ℓ)+ℓ2πT(t)Sπ(t)−ℓ∫tt−ℓπT(u)Sπ(u)du}. | (3.4) |
By using Lemma (2.3), we can get
LV(t,π(t),a)≤ m∑a=1χa(ϑ(t)){−2πT(t)(PˆDa)π(t)+ϵ−11πT(t)(PˆGaˆGTaPT)π(t)+ϵ1πT(t)(ˆH1aTˆH1a)π(t)+2πT(t)(PˆAa)ˆg(π(t))+ϵ−12πT(t)(PˆGaˆGTaPT)×π(t)+ϵ2ˆgT(π(t))(ˆH2aTˆH2a)ˆg(π(t))+2πT(t)(PˆBa)ˆg(π(t−ℓ(t)))+ϵ−13πT(t)(PˆGaˆGTaPT)π(t)+ϵ3ˆgT(π(t−ℓ(t)))(ˆH3aTˆH3a)ˆg(π(t−ℓ(t)))+trace{ςTa(t)Pςa(t)}+πT(t)Qπ(t)−(1−μ)πT(t−ℓ(t))Q×π(t−ℓ(t))+πT(t)Rπ(t)−πT(t−ℓ)Rπ(t−ℓ)+ℓ2πT(t)Sπ(t)−ℓ∫tt−ℓπT(u)Sπ(u)du}. | (3.5) |
By using Assumption 1 and Lemma (2.2), we have
−ℓ∫tt−ℓπT(u)Sπ(u)du= −ℓ∫t−ℓ(t)t−ℓπT(u)Sπ(u)du−ℓ∫tt−ℓ(t)πT(u)Sπ(u)du−ℓ∫tt−ℓπT(u)Sπ(u)du=−ℓℓ−ℓ(t)[∫t−ℓ(t)t−ℓπ(u)du]TS[∫t−ℓ(t)t−ℓπ(u)du]−ℓℓ(t)[∫tt−ℓ(t)π(u)du]TS[∫tt−ℓ(t)π(u)du]=−[∫t−ℓ(t)t−ℓπ(u)du]TS[∫t−ℓ(t)t−ℓπ(u)du]−ℓ(t)ℓ−ℓ(t)[∫t−ℓ(t)t−ℓπ(u)du]TS[∫t−ℓ(t)t−ℓπ(u)du]−[∫tt−ℓ(t)π(u)du]TS[∫tt−ℓ(t)π(u)du]−ℓ−ℓ(t)ℓ(t)[∫tt−ℓ(t)π(u)du]TS[∫tt−ℓ(t)π(u)du]. | (3.6) |
If [STTTS]≥0 by Lemma (2.5), the following inequality is true:
[√ℓ(t)ℓ−ℓ(t)[∫t−ℓ(t)t−ℓπ(u)du]√ℓ−ℓ(t)ℓ(t)[∫tt−ℓ(t)π(u)du]]T[STTTS][√ℓ(t)ℓ−ℓ(t)[∫t−ℓ(t)t−ℓπ(u)du]√ℓ−ℓ(t)ℓ(t)[∫tt−ℓ(t)π(u)du]]≥0, | (3.7) |
which implies
−ℓ(t)ℓ−ℓ(t)[∫t−ℓ(t)t−ℓπ(u)du]TS[∫t−ℓ(t)t−ℓπ(u)du]−ℓ−ℓ(t)ℓ(t)[∫tt−ℓ(t)π(u)du]TS[∫tt−ℓ(t)π(u)du]≤−[∫t−ℓ(t)t−ℓπ(u)du]TT[∫tt−ℓ(t)π(u)du]−[∫tt−ℓ(t)π(u)du]TTT[∫t−ℓ(t)t−ℓπ(u)du]. | (3.8) |
From (3.6) and (3.8), one can obtain that
−ℓ∫tt−ℓπT(u)Sπ(u)du≤−[∫t−ℓ(t)t−ℓπ(u)du]TS[∫t−ℓ(t)t−ℓπ(u)du]−[∫tt−ℓ(t)π(u)du]TS[∫tt−ℓ(t)π(u)du]−[∫t−ℓ(t)t−ℓπ(u)du]TT[∫tt−ℓ(t)π(u)du]−[∫tt−ℓ(t)π(u)du]TTT[∫t−ℓ(t)t−ℓπ(u)du]. | (3.9) |
From the Assumption 4, one has
trace(ςTa(t)ςa(t))≤ (zR)T(¯U1a+¯V1a+¯M1a+¯N1a)(zR)+(zR)Tℓ(t)(¯U5a+¯V5a+¯M5a+¯N5a)(zR)ℓ(t)+(zI)T(¯U2a+¯V2a+¯M2a+¯N2a)(zI)+(zI)Tℓ(t)(¯U6a+¯V6a+¯M6a+¯N6a)(zI)ℓ(t)+(zJ)T(¯U3a+¯V3a+¯M3a+¯N3a)(zJ)+(zJ)Tℓ(t)(¯U7a+¯V7a+¯M7a+¯N7a)(zJ)ℓ(t)+(zK)T(¯U4a+¯V4a+¯M4a+¯N4a)(zK)+(zK)Tℓ(t)(¯U8a+¯V8a+¯M8a+¯N8a)(zK)ℓ(t)≤ πT(t)Π1π(t)+πT(t−ℓ(t))Π2π(t−ℓ(t)), | (3.10) |
where
Π1=[¯U1a+¯V1a+¯M1a+¯N1a0000¯U2a+¯V2a+¯M2a+¯N2a0000¯U3a+¯V3a+¯M3a+¯N3a0000¯U4a+¯V4a+¯M4a+¯N4a],Π2=[¯U5a+¯V5a+¯M5a+¯N5a0000¯U6a+¯V6a+¯M6a+¯N6a0000¯U7a+¯V7a+¯M7a+¯N7a0000¯U8a+¯V8a+¯M8a+¯N8a]. |
From (3.10) and (3.1), one can obtain
trace(ςTa(t)Pςa(t))≤ πT(t)(λΠ1)π(t)+πT(t−ℓ(t))(λΠ2)π(t−ℓ(t)). | (3.11) |
Moreover, from Assumption 2 it follows that
0≤ γ1[πT(t)ˆLgπ(t)−ˆgT(π(t))ˆg(π(t))], | (3.12) |
0≤ γ2[πT(t−ℓ(t))ˆLgπ(t−ℓ(t))−ˆgT(π(t−ℓ(t)))ˆg(π(t−ℓ(t)))]. | (3.13) |
Adding from (3.5)-(3.13), we get
LV(t,π(t),a)≤ m∑a=1χa(ϑ(t)){ξT(t)Φaξ(t)}, | (3.14) |
where
ξ(t)= [πT(t) πT(t−ℓ(t)) πT(t−ℓ) ˆgT(π(t)) ˆgT(π(t−ℓ(t))) ∫t−ℓ(t)t−ℓπT(u)du ∫tt−ℓ(t)πT(u)du]T, |
and Φa=(Φi,j,a)7×7 with Φ1,1,a=−PˆDa−ˆDaPT+ϵ−11PˆGaˆGTaPT+ϵ1ˆH1aTˆH1a+ϵ−12PˆGaˆGTaPT+ϵ−13PˆGaˆGTaPT+Q+R+ℓ2S+γ1ˆLg+λΠ1, Φ1,4,a=PˆAa, Φ1,5,a=PˆBa, Φ2,2,a=−(1−μ)Q+γ2ˆLg+λΠ2, Φ3,3,a=−R, Φ4,4,a=−γ1+ϵ2ˆH2aTˆH2a, Φ5,5,a=−γ2+ϵ3ˆH3aTˆH3a, Φ6,6,a=−S, Φ6,7,a=−T, Φ7,7,a=−S.
By Schur Complement Lemma (2.4), it is obvious that Φa is equivalent to [ˆΦaΥa✠Ωa]< 0. Then taking mathematical expectation, we have
E{LV(t,π(t),a)}≤ E{ξT(t)[ˆΦaΥa✠Ωa]ξ(t)},≤−ϵE{‖π(t)‖2}. | (3.15) |
This implies that the NNs (2.11) is robustly asymptotically stable in the mean square. The proof is completed.
Remark 3.2. Suppose there has no stochastic disturbance, then NNs (2.6) turns to
dz(t)dt=m∑a=1χa(ϑ(t)){−(Da+△Da(t))z(t)+(Aa+△Aa(t))g(z(t))+(Ba+△Ba(t))g(z(t−ℓ(t)))}. | (3.16) |
At the same time, system (2.11) turns to
dπ(t)dt=m∑a=1χa(ϑ(t)){−(ˆDa+△ˆDa)π(t)+(ˆAa+△ˆAa)ˆg(π(t))+(ˆBa+△ˆBa)ˆg(π(t−ℓ(t)))}. | (3.17) |
By setting the stochastic disturbance ςa(t)dω(t)=0 in Theorem (3.1), Corollary (3.3) can be obtained.
Corollary 3.3. Suppose Assumptions 1-3 hold. If there exist positive symmetric matrices P∈R4n×4n,Q∈R4n×4n,R∈R4n×4n,S∈R4n×4n, any matrix T∈R4n×4n and positive scalars ϵ1∈Rn,ϵ2∈Rn,ϵ3∈Rn,γ1∈Rn,γ2∈Rn, such that the following LMI hold for all a=1,2,...,m
[˜ΦaΥa✠Ωa]<0, | (3.18) |
where ˜Φa=(˜Φi,j,a)7×7, Υa=(Υi,j,a)7×3, Ωa=(Ωi,j,a)3×3 with ˜Φ1,1,a=−PˆDa−ˆDaPT+ϵ1ˆH1aTˆH1a+Q+R+ℓ2S+γ1ˆLg, ˜Φ1,4,a=PˆAa, ˜Φ1,5,a=PˆBa, ˜Φ2,2,a=−(1−μ)Q+γ2ˆLg, ˜Φ3,3,a=−R, ˜Φ4,4,a=−γ1+ϵ2ˆH2aTˆH2a, ˜Φ5,5,a=−γ2+ϵ3ˆH3aTˆH3a, ˜Φ6,6,a=−S, ˜Φ6,7,a=−T, ˜Φ7,7,a=−S, Υ1,1,a=PˆGa, Υ1,2,a=PˆGa, Υ1,3,a=PˆGa, Ω1,1,a=−ϵ1, Ω2,2,a=−ϵ2, Ω3,3,a=−ϵ3, then the NN model (3.17) is robustly asymptotically stable.
Remark 3.4. In Theorem (3.1) and Corollary (3.3), sufficient conditions are obtained to ensure that the NN (2.11) model is robust asymptotic stability by decomposes QVNNs into real-valued NNs, but the result we achieve is actually about QVNNs themselves.
If there are no uncertainties, then NNs (2.6) becomes
dz(t)=m∑a=1χa(ϑ(t)){[−Daz(t)+Aag(z(t))+Bag(z(t−ℓ(t)))]dt+σa(t,z(t),z(t−ℓ(t)))dω(t)}. | (3.19) |
At the same time, the NNs (2.11) turns to
dπ(t)= m∑a=1χa(ϑ(t)){[−ˆDaπ(t)+ˆAaˆg(π(t))+ˆBaˆg(π(t−ℓ(t)))]dt+ςa(t)dω(t)}. | (3.20) |
By setting △ˆDa=△ˆAa=△ˆBa=0 in Theorem (3.1), Theorem (3.5) can be obtained.
Theorem 3.5. Suppose Assumptions 1, 2, 4 hold. If there exist positive symmetric matrices P∈R4n×4n,Q∈R4n×4n,R∈R4n×4n,S∈R4n×4n, any matrix T∈R4n×4n and positive scalars γ1∈Rn,γ2∈Rn,λ∈Rn, such that the following LMIs hold for all a=1,2,...,m
P≤ λI, | (3.21) |
˘Φa< 0, | (3.22) |
where ˘Φa=(˘Φi,j,a)7×7, with ˘Φ1,1,a=−PˆDa−ˆDaPT+Q+R+ℓ2S+γ1ˆLg+λΠ1, ˘Φ1,4,a=PˆAa, ˘Φ1,5,a=PˆBa, ˘Φ2,2,a=−(1−μ)Q+γ2ˆLg+λΠ2, ˘Φ3,3,a=−R, ˘Φ4,4,a=−γ1, ˘Φ5,5,a=−γ2, ˘Φ6,6,a=−S, ˘Φ6,7,a=−T, ˘Φ7,7,a=−S, then the NN model (3.20) is globally asymptotically stable in the mean square.
Remark 3.6. When stochastic disturbance is not appear, then the NNs (3.19) turns to
dz(t)dt= m∑a=1χa(ϑ(t)){−Daz(t)+Aag(z(t))+Bag(z(t−ℓ(t)))}. | (3.23) |
At the same time, the NNs (3.20) turns to
dπ(t)dt= m∑a=1χa(ϑ(t)){−ˆDaπ(t)+ˆAaˆg(π(t))+ˆBaˆg(π(t−ℓ(t)))}. | (3.24) |
By setting △ˆDa=△ˆAa=△ˆBa=0 and ςa(t)dω(t)=0 in Theorem (3.5), Corollary (3.7) can be obtained.
Corollary 3.7. Suppose Assumptions 1, 2 hold. If there exist positive symmetric matrices P∈R4n×4n,Q∈R4n×4n,R∈R4n×4n,S∈R4n×4n, any matrix T∈R4n×4n and positive scalars γ1∈Rn,γ2∈Rn, such that the following LMIs hold for all a=1,2,...,m
ˇΦa<0, | (3.25) |
where ˘Φa=(˘Φi,j,a)7×7 with ˇΦ1,1,a=−PˆDa−ˆDaPT+Q+R+ℓ2S+γ1ˆLg, ˇΦ1,4,a=PˆAa, ˇΦ1,5,a=PˆBa, ˇΦ2,2,a=−(1−μ)Q+γ2ˆLg, ˇΦ3,3,a=−R, ˇΦ4,4,a=−γ1, ˇΦ5,5,a=−γ2, ˇΦ6,6,a=−S, ˇΦ6,7,a=−T, ˇΦ7,7,a=−S, then the NN model (3.19) is globally asymptotically stable.
Remark 3.8. As is well known, QVNNs are the extensions of real-valued and complex-valued NNs with quaternion-valued states, inputs, and connection weights. The activation functions in the real domain are generally assumed to be smooth and bounded, whereas these assumptions do not apply to the quaternion domain. Therefore, choosing the activation function is very important when dealing with quaternions. Because of this, quaternion-valued activation functions are generally examined in three different ways: (i) The real-valued decomposition method, (ii) The complex-valued decomposition method, (iii) The direct quaternion method. Based on these three approaches, several works have been published on the dynamics of QVNNs [28,30,34,35,36,37,38].
Remark 3.9. By using suitable LKF, T-S fuzzy system model and the theory of stochastic analysis, we obtain a novel set of sufficient conditions for T-S FUQVSNNs (2.6) to ascertain the robust and global asymptotic stability. As far as we are aware, no results have been published on the robust and global asymptotic stable for T-S FUQVSNNs with time-varying delays (2.6).
Remark 3.10. As we all know, QVNNs are aimed for investigating new capabilities and improved accuracy to address issues that cannot be resolved using complex-valued and real-valued NN models. For instance, the global stability of complex-valued NN [18,19,45], and real-valued NNs [12,13,14] can be summarized as a particular case of the results of this work.
Remark 3.11. It is obvious that real-valued LMI can be solved straightforwardly with MATLAB LMI toolbox; however, solving quaternion-valued LMI is more challenging. Therefore, we establish the robust and global asymptotic stability criteria for T-S FUQVSNNs by decomposing n-dimensional quaternion-valued NNs into 4n-dimensional real-valued NNs. Based on that, sufficient criteria in this paper are derived in terms of real-valued LMIs.
In this section, two numerical examples illustrate the effectiveness of the theoretical results presented in the previous section.
Example 1: Consider the plant rules with a=1,2, the T-S FUQVSNNs is described as follows
dz(t)=2∑a=1χa(ϑ(t)){[−(¯Da+△¯Da(t))z(t)+(¯Aa+△¯Aa(t))g(z(t))+(¯Ba+△¯Ba(t))g(z(t−ℓ(t)))]dt+σa(t,z(t),z(t−ℓ(t)))dω(t)}. | (4.1) |
Plant Rule 1: IF ϑ1(t) is η11, THEN
dz(t)= [−(¯D1+△¯D1(t))z(t)+(¯A1+△¯A1(t))g(z(t))+(¯B1+△¯B1(t))g(z(t−ℓ(t)))]dt+σ1(t,z(t),z(t−ℓ(t)))dω(t). |
Plant Rule 2: IF ϑ1(t) is η21, THEN
dz(t)= [−(¯D2+△¯D2(t))z(t)+(¯A2+△¯A2(t))g(z(t))+(¯B2+△¯B2(t))g(z(t−ℓ(t)))]dt+σ2(t,z(t),z(t−ℓ(t)))dω(t), |
where η11 is z1(t)≤1, η21 is z1(t)>1, and
¯D1= [9009], ¯D2= [100010],¯A1= [0.5−0.5i+0.3j−0.4k0.5+0.5i−0.4j+0.3k0.4+0.5i−0.4j−0.5k0.5−0.5i+0.4j+0.4k],¯A2= [0.4−0.5i+0.3j−0.4k0.4+0.3i−0.4j+0.3k0.2+0.4i−0.4j−0.4k0.3−0.3i+0.2j+0.2k],¯B1= [0.6−0.5i+0.5j−0.4k0.6+0.5i−0.6j+0.3k0.6+0.5i−0.6j−0.5k0.4−0.5i+0.6j+0.4k],¯B2= [0.5−0.4i+0.3j−0.4k0.2+0.3i−0.5j+0.3k0.6+0.5i−0.4j−0.3k0.3−0.5i+0.2j+0.4k],G1= G2=[0.1000.1], H11=[0.1000.1], H12=[0.3000.3],H21= [0.2000.2], H22=[0.1000.1], H31=[0.2000.2], H32=[0.3000.3],H41= [0.1000.1], H42=[0.2000.2], H51=[0.3000.3], H52=[0.2000.2],H61= [0.2000.2], H62=[0.3000.3], H71=[0.3000.3], H72=[0.1000.1],H81= [0.2000.2], H82=[0.2000.2], H91=[0.2000.2], H92=[0.3000.3],F1(t)= F2(t)=[0.1 sin(t)000.1 sin(t)]. |
The premise variable ϑ(t) is chosen as a state-dependent term, that is, ϑ(t)=z1(t). Using the same procedure as in [40], the membership functions can be obtained from the property of χ1(z1(t))+χ2(z1(t))=1, where χ1(z1(t))=11+e−z1(t), χ2(z1(t))=1−11+e−z1(t).
Assumption 4 is further assumed to be satisfied by
¯U11= ¯U12=[0.001000.001], ¯V11=¯V12=[0.005000.001], ¯M11=¯M12=[0.002000.001],¯N11= ¯N12=[0.001000.002], ¯U21=¯U22=[0.005000.002], ¯V21=¯V22=[0.001000.003],¯M21= ¯M22=[0.005000.005], ¯N21=¯N22=[0.005000.001], ¯U31=¯U32=[0.002000.002],¯V31= ¯V32=[0.003000.003], ¯M31=¯M32=[0.002000.003], ¯N31=¯N32=[0.005000.004],¯U41= ¯U42=[0.004000.004], ¯V41=¯V42=[0.004000.001], ¯M41=¯M42=[0.004000.002],¯N41= ¯N42=[0.004000.003], ¯U51=¯U52=[0.003000.005], ¯V51=¯V52=[0.003000.004],¯M51= ¯M52=[0.003000.003], ¯N51=¯N52=[0.003000.002], ¯U61=¯U62=[0.003000.001],¯V61= ¯V62=[0.002000.005], ¯M61=¯M62=[0.002000.004], ¯N61=¯N62=[0.002000.003],¯U71= ¯U72=[0.002000.002], ¯V71=¯V72=[0.002000.001], ¯M71=¯M72=[0.001000.005],¯N71= ¯N72=[0.001000.004], ¯U81=¯U82=[0.001000.003], ¯V81=¯V82=[0.001000.002],¯M81= ¯M82=[0.001000.001], ¯N81=¯N82=[0.003000.002]. |
Using simple calculations, we can find out ¯AR1, ¯AR2, ¯AI1, ¯AI2, ¯AJ1, ¯AJ2, ¯AK1, ¯AK2, ¯BR1, ¯BR2, ¯BI1, ¯BI2, ¯BJ1, ¯BJ2, ¯BK1, ¯BK2, ˆD1, ˆD2, ˆA1, ˆA2, ˆB1, ˆB2, ˆG1, ˆG2, ˆF1(t), ˆF2(t), ˆH11, ˆH12, ˆH21, ˆH22, ˆH31 and ˆH32.
Moreover, the activation functions gα(zα(t)), gα(zα(t−ℓ(t))) in (4.1) can be chosen as gα(zα(t))=0.5 tanh(zα(t))+0.5 tanh(zα(t))i+0.5 tanh(zα(t))j+0.5 tanh(zα(t))k, gα(zα(t−ℓ(t)))=0.5 tanh(zα(t−ℓ(t)))+0.5 tanh(zα(t−ℓ(t)))i+0.5 tanh(zα(t−ℓ(t)))j+0.5 tanh(zα(t−ℓ(t)))k for all α=1,2. Obviously, the activation functions gα(zα(t)) and gα(zα(t−ℓ(t))) are satisfies Assumption 2 with ˆLg=diag{0.25,0.25,0.25,0.25,0.25,0.25,0.25,0.25}. The delay ℓ(t) is defined as ℓ(t)=0.1+0.2 sin(t), which implies that the maximum permissible upper bound is ℓ=0.3. It is observable that 0≤˙ℓ(t)≤μ=0≤0.2 cos(t)≤0.2. By applying MATLAB LIM toolbox, the LMI conditions of Theorem (3.1) are verified. Under initial values of φ1(t)=−1+0.9i−1.5j+0.8k, φ2(t)=0.8−0.8i+j−1.2k, the time responses of states zR1(t), zI1(t), zJ1(t), zK1(t), zR2(t), zI2(t), zJ2(t), zK2(t) are illustrated in Figures (1)–(4).
This example confirms all of the conditions associated with Theorem (3.1), then the equilibrium point of NNs (2.11) is robustly asymptotically stable in the mean square.
Example 2: Determine the T-S fuzzy stochastic QVNNs with a=1,2 as given below:
dz(t)=2∑a=1χa(ϑ(t)){[−¯Daz(t)+¯Aag(z(t))+¯Bag(z(t−ℓ(t)))]dt+σa(t,z(t),z(t−ℓ(t)))dω(t)}. | (4.2) |
Plant Rule 1: IF ϑ1(t) is η11, THEN
dz(t)= [−¯D1z(t)+¯A1g(z(t))+¯B1g(z(t−ℓ(t)))]dt+σ1(t,z(t),z(t−ℓ(t)))dω(t)}. |
Plant Rule 2: IF ϑ1(t) is η21, THEN
dz(t)= [−¯D2z(t)+¯A2g(z(t))+¯B2g(z(t−ℓ(t)))]dt+σ2(t,z(t),z(t−ℓ(t)))dω(t), |
where η11 is z1(t), η21 is z1(t), and let z1(t)∈[−s,s], where 0<s=3, and
¯D1= [8008], ¯D2= [9009],¯A1= [0.6−0.6i+0.4j−0.5k0.6+0.6i−0.5j+0.4k0.5+0.6i−0.5j−0.6k0.6−0.6i+0.5j+0.5k],¯A2= [0.5−0.6i+0.4j−0.5k0.5+0.4i−0.5j+0.4k0.3+0.5i−0.5j−0.5k0.4−0.4i+0.3j+0.3k],¯B1= [0.7−0.6i+0.5j−0.5k0.7+0.6i−0.5j+0.4k0.6+0.6i−0.5j−0.5k0.7−0.6i+0.6j+0.4k],¯B2= [0.4−0.4i+0.6j−0.5k0.4+0.3i−0.6j+0.2k0.5+0.3i−0.4j−0.2k0.6−0.4i+0.4j+0.2k]. |
The premise variable ϑ(t) is chosen as a state-dependent term, that is, ϑ(t)=z1(t). Using the same procedure as in [40], the membership functions can be obtained from the property of χ1(z1(t))+χ2(z1(t))=1, where χ1(z1(t))=11+e−3z1(t), χ2(z1(t))=1−11+e−3z1(t).
Assumption 4 is further assumed to be satisfied by
¯U11= ¯U12=[0.001000.001], ¯V11=¯V12=[0.005000.001], ¯M11=¯M12=[0.002000.001],¯N11= ¯N12=[0.001000.002], ¯U21=¯U22=[0.005000.002], ¯V21=¯V22=[0.001000.003],¯M21= ¯M22=[0.005000.005], ¯N21=¯N22=[0.005000.001], ¯U31=¯U32=[0.002000.002],¯V31= ¯V32=[0.003000.003], ¯M31=¯M32=[0.002000.003], ¯N31=¯N32=[0.005000.004],¯U41= ¯U42=[0.004000.004], ¯V41=¯V42=[0.004000.001], ¯M41=¯M42=[0.004000.002],¯N41= ¯N42=[0.004000.003], ¯U51=¯U52=[0.003000.005], ¯V51=¯V52=[0.003000.004],¯M51= ¯M52=[0.003000.003], ¯N51=¯N52=[0.003000.002], ¯U61=¯U62=[0.003000.001],¯V61= ¯V62=[0.002000.005], ¯M61=¯M62=[0.002000.004], ¯N61=¯N62=[0.002000.003],¯U71= ¯U72=[0.002000.002], ¯V71=¯V72=[0.002000.001], ¯M71=¯M72=[0.001000.005],¯N71= ¯N72=[0.001000.004], ¯U81=¯U82=[0.001000.003], ¯V81=¯V82=[0.001000.002],¯M81= ¯M82=[0.001000.001], ¯N81=¯N82=[0.003000.002]. |
The following can be obtained by simple calculations ¯AR1, ¯AR2, ¯AI1, ¯AI2, ¯AJ1, ¯AJ2, ¯AK1, ¯AK2, ¯BR1, ¯BR2, ¯BI1, ¯BI2, ¯BJ1, ¯BJ2, ¯BK1, ¯BK2, ˆD1, ˆD2, ˆA1, ˆA2, ˆB1 and ˆB2. Further, the activation functions gα(zα(t)), gα(zα(t−ℓ(t))) in (4.2) can be selected as gα(zα(t))=0.5 tanh(zα(t))+0.5 tanh(zα(t))i+0.5 tanh(zα(t))j+0.5 tanh(zα(t))k, gα(zα(t−ℓ(t)))=0.5 tanh(zα(t−ℓ(t)))+0.5 tanh(zα(t−ℓ(t)))i+0.5 tanh(zα(t−ℓ(t)))j+0.5 tanh(zα(t−ℓ(t)))k for all α=1,2. Clearly, the activation functions gα(zα(t)), gα(zα(t−ℓ(t))) are satisfies Assumption 2 with ˆLg=diag{0.25,0.25,0.25,0.25,0.25,0.25,0.25,0.25}.
The delay ℓ(t) is regarded as ℓ(t)=0.1+0.2 sin(t), implying that the maximum permissible upper bound is ℓ=0.3. It is observable that 0≤˙ℓ(t)≤μ=0≤0.2 cos(t)≤0.2.
The LMI conditions of Theorem (3.5) are verified by applying MATLAB LIM toolbox. Under randomly selected 15 initial values, the time responses of states zR1(t), zI1(t), zJ1(t), zK1(t), zR2(t), zI2(t), zJ2(t), zK2(t) are illustrated in Figures (5)–(7).
This example confirms all of the conditions associated with Theorem (3.5), then the equilibrium point of NNs (3.19) is globally asymptotically stable in the mean square.
This paper investigated the robust and global asymptotic stability problem for a class of T-S FUQVSNNs with discrete time-varying delays using the system decomposition method. By applying T-S fuzzy models and stochastic analysis, we first considered a general form of T-S FUQVSNNs with time delays. Then, we presented some delay-dependent stability conditions for the considered NNs using LKFs to ensure the robust and global asymptotic stability. Furthermore, we established our results in terms of real-valued LMIs that can be solved in MATLAB LMI toolbox. Finally, two numerical examples are presented with their simulations to demonstrate the validity of the theoretical analysis. By using the results of this paper, we can analyze various dynamic behaviours of T-S FUQVSNNs including finite-time stability, passivity, state estimation, synchronization, and others. There are certain advancements worth investigating further in this proposed area of research. Soon, we will attempt to investigate the stability of delayed impulsive T-S FUQVSNNs in the finite-time case.
The authors would like to express her gratitude to King Khalid University, Saudi Arabia for providing administrative and technical support. Also, the authors would like to thank the referee for their comments and suggestions on the manuscript.
Funding Statement: This research is financially supported by the Deanship of Scientific Research at King Khalid University under research grant number (RGP.2/202/43).
The authors declare no conflict of interest.
[1] |
J. J. Hopfield, Neural networks and physical systems with emergent collective computational abilities, Proc. Natl. Acad. Sci. U.S.A, 79 (1982), 2554–2558. https://doi.org/10.1073/pnas.79.8.2554 doi: 10.1073/pnas.79.8.2554
![]() |
[2] |
M. A. Cohen, S. Grossberg, Absolute stability of global pattern formation and parallel memory storage by competitive neural networks, IEEE Trans. Syst. Man Cybern., 13 (1983), 815–826. https://doi.org/10.1109/TSMC.1983.6313075 doi: 10.1109/TSMC.1983.6313075
![]() |
[3] |
L. Chua, L. Yang, Cellular neural networks: Applications, IEEE Trans. Circuits Syst., 35 (1988), 1273–1290. https://doi.org/10.1109/31.7601 doi: 10.1109/31.7601
![]() |
[4] |
H. Huang, J. Cao, On global asymptotic stability of recurrent neural networks with time-varying delays, Appl. Math. Comput., 142 (2003), 143–154. https://doi.org/10.1016/S0096-3003(02)00289-8 doi: 10.1016/S0096-3003(02)00289-8
![]() |
[5] |
B. Kosko, Bidirectional associative memories, IEEE Trans. Syst. Man Cybern., 18 (1988), 49–60. https://doi.org/10.1109/21.87054 doi: 10.1109/21.87054
![]() |
[6] |
S. Blythe, X. R. Mao, X. X. Liao, Stability of stochastic delay neural networks, J. Franklin Inst., 338 (2001), 481–495. https://doi.org/10.1016/S0016-0032(01)00016-3 doi: 10.1016/S0016-0032(01)00016-3
![]() |
[7] |
R. Yang, Z. Zhang, P. Shi, Exponential stability on stochastic neural networks with discrete interval and distributed delays, IEEE Trans. Neural Netw., 21 (2010), 169–175. https://doi.org/10.1109/TNN.2009.2036610 doi: 10.1109/TNN.2009.2036610
![]() |
[8] | X. Mao, Stochastic Differential Equations and Applications, Horwood Publishing, (1997). |
[9] | L. Arnold, Stochastic Differential Equations: Theory and Applications, Wiley, New York, (1972). |
[10] | A. Friedman, Stochastic Differential Equations and Applications, Academic Press, New York, (1976). |
[11] |
D. Yang, X. Li, Robust stability analysis of stochastic switched neural networks with parameter uncertainties via state-dependent switching law, Neurocomputing, 452 (2021), 813–819. https://doi.org/10.1016/j.neucom.2019.11.120 doi: 10.1016/j.neucom.2019.11.120
![]() |
[12] |
G. Liu, S. X. Yang, Y. Chai, W. Feng, W. Fu, Robust stability criteria for uncertain stochastic neural networks of neutral-type with interval time-varying delays, Neural Comput. Appl., 22 (2013), 349–359. https://doi.org/10.1007/s00521-011-0696-1 doi: 10.1007/s00521-011-0696-1
![]() |
[13] | Z. Meng, Z. Xiang, Stability analysis of stochastic memristor-based recurrent neural networks with mixed time-varying delays, Neural Comput. Appl., 28 (2017), 1787–1799. https://doi.org/10.1007/s00521-015-2146-y |
[14] |
W. Xie, Q. Zhu, F. Jiang, Exponential stability of stochastic neural networks with leakage delays and expectations in the coefficients, Neurocomputing, 173 (2016), 1268–1275. https://doi.org/10.1016/j.neucom.2015.08.086 doi: 10.1016/j.neucom.2015.08.086
![]() |
[15] |
K. Zhong, S. Zhu, Q. Yang, Further results for global exponential stability of stochastic memristor-based neural networks with time-varying delays, Int. J. Syst. Sci., 47 (2016), 3573–3580. https://doi.org/10.1080/00207721.2015.1095955 doi: 10.1080/00207721.2015.1095955
![]() |
[16] |
G. Sun, Y. Zhang, Exponential stability of impulsive discrete-time stochastic BAM neural networks with time-varying delay, Neurocomputing, 131 (2014), 323–330. https://doi.org/10.1016/j.neucom.2013.10.010 doi: 10.1016/j.neucom.2013.10.010
![]() |
[17] |
Q. Song, Z. Zhao, Y. Liu, F. E. Alsaadi, Mean-square input-to-state stability for stochastic complex-valued neural networks with neutral delay, Neurocomputing, 470 (2022), 269–277. https://doi.org/10.1016/j.neucom.2021.10.117 doi: 10.1016/j.neucom.2021.10.117
![]() |
[18] |
Y. Cao, R. Sriraman, N. Shyamsundarraj, R. Samidurai, Robust stability of uncertain stochastic complex-valued neural networks with additive time-varying delays, Math. Comput. Simul., 171 (2020), 207–220. https://doi.org/10.1016/j.matcom.2019.05.011 doi: 10.1016/j.matcom.2019.05.011
![]() |
[19] |
R. Sriraman, Y. Cao, R. Samidurai, Global asymptotic stability of stochastic complex-valued neural networks with probabilistic time-varying delays, Math. Comput. Simul., 171 (2020), 103–118. https://doi.org/10.1016/j.matcom.2019.04.001 doi: 10.1016/j.matcom.2019.04.001
![]() |
[20] |
D. Liu, S. Zhu, W. Chang, Mean square exponential input-to-state stability of stochastic memristive complex-valued neural networks with time varying delay, Int. J. Syst. Sci., 48 (2017), 1966–1977. https://doi.org/10.1080/00207721.2017.1300706 doi: 10.1080/00207721.2017.1300706
![]() |
[21] |
W. Gong, J. Liang, X. Kan, L. Wang, A. M. Dobaie, Robust state estimation for stochastic complex-valued neural networks with sampled-data, Neural Comput. Appl., 31 (2019), 523–542. https://doi.org/10.1007/s00521-017-3030-8 doi: 10.1007/s00521-017-3030-8
![]() |
[22] |
D. L. Lee, Relaxation of the stability condition of the complex-valued neural networks, IEEE Trans. Neural Netw., 12 (2001), 1260–1262. https://doi.org/10.1109/72.950156 doi: 10.1109/72.950156
![]() |
[23] |
B. Zhou, Q. Song, Boundedness and complete stability of complex-valued neural networks with time delay, IEEE Trans. Neural Netw. Learn. Syst., 24 (2013), 1227–1238. https://doi.org/10.1109/TNNLS.2013.2247626 doi: 10.1109/TNNLS.2013.2247626
![]() |
[24] | A. Sudbery, Quaternionic analysis, Math. Proc. Camb. Philos. Soc., 85 (1979), 199–225. https://doi.org/10.1017/S0305004100055638 |
[25] |
T. Isokawa, T. Kusakabe, N. Matsui, F. Peper, Quaternion neural network and its application, Knowl. Based Intell. Eng. Syst., 2774 (2003), 318–324. https://doi.org/10.1007/978-3-540-45226-3-44 doi: 10.1007/978-3-540-45226-3-44
![]() |
[26] |
Y. Liu, Y. Zheng, J. Lu, J. Cao, L. Rutkowski, Constrained quaternion-variable convex optimization: A quaternion-valued recurrent neural network approach, IEEE Trans. Neural Netw. Learn. Syst., 31 (2020), 1022–1035. https://doi.org/10.1109/TNNLS.2019.2916597 doi: 10.1109/TNNLS.2019.2916597
![]() |
[27] |
A. B. Greenblatt, S. S. Agaian, Introducing quaternion multi-valued neural networks with numerical examples, Inf. Sci., 423 (2018), 326–342. https://doi.org/10.1016/j.ins.2017.09.057 doi: 10.1016/j.ins.2017.09.057
![]() |
[28] |
Q. Song, X. Chen, Multistability analysis of quaternion-valued neural networks with time delays, IEEE Trans. Neural Netw. Learn. Syst., 29 (2018), 5430–5440. https://doi.org/10.1109/TNNLS.2018.2801297 doi: 10.1109/TNNLS.2018.2801297
![]() |
[29] |
J. Wang, T. Li, X. Luo, Y. Q. Shi, S. K. Jha, Identifying computer generated images based on quaternion central moments in color quaternion wavelet domain, IEEE Trans. Circuits Syst. Video Technol., 29 (2018), 2775–2785. https://doi.org/10.1109/TCSVT.2018.2867786 doi: 10.1109/TCSVT.2018.2867786
![]() |
[30] |
Y. Liu, D. Zhang, J. Lou, J. Lu, J. Cao, Stability analysis of quaternion-valued neural networks: Decomposition and direct approaches, IEEE Trans. Neural Netw. Learn. Syst., 29 (2018), 4201–4211. https://doi.org/10.1109/TNNLS.2017.2755697 doi: 10.1109/TNNLS.2017.2755697
![]() |
[31] |
Z. Xia. Y. Liu, J. Lu, J. Cao, L. Rutkowski, Penalty method for constrained distributed quaternion-variable optimization, IEEE Trans. Cybern., 51 (2021), 5631–5636. https://doi.org/10.1109/TCYB.2020.3031687 doi: 10.1109/TCYB.2020.3031687
![]() |
[32] |
Y. Wang, K. I. Kou, C. Zou, Y. Y. Tang, Robust sparse representation in quaternion space, IEEE Trans. Image Process., 30 (2021), 3637–3649. https://doi.org/10.1109/TIP.2021.3064193 doi: 10.1109/TIP.2021.3064193
![]() |
[33] |
H. Chen, T. Wang, J. Cao, P. P. Vidal, Y. Yang, Dynamic quaternion extreme learning machine, IEEE Trans. Circuits Syst. II: Exp. Briefs, 68 (2021), 3012–3016. https://doi.org/10.1109/TCSII.2021.3067014 doi: 10.1109/TCSII.2021.3067014
![]() |
[34] |
R. Sriraman, G. Rajchakit, C. P. Lim, P. Chanthorn, R. Samidurai, Discrete-time stochastic quaternion-valued neural networks with time delays: An asymptotic stability analysis, Symmetry, 12 (2020), 936. https://doi.org/10.3390/sym12060936 doi: 10.3390/sym12060936
![]() |
[35] |
J. Shu, B. Wu, L. Xiong, Stochastic stability criteria and event-triggered control of delayed Markovian jump quaternion-valued neural networks, Appl. Math. Comput., 420 (2022), 126904. https://doi.org/10.1016/j.amc.2021.126904 doi: 10.1016/j.amc.2021.126904
![]() |
[36] |
Q. Song, R. Zeng, Z. Zhao, Y. Liu, F. E. Alsaadi, Mean-square stability of stochastic quaternion-valued neural networks with variable coefficients and neutral delays, Neurocomputing, 471 (2022), 130–138. https://doi.org/10.1016/j.neucom.2021.11.033 doi: 10.1016/j.neucom.2021.11.033
![]() |
[37] |
C. Li, J. Cao, A. Kashkynbayev, Synchronization in quaternion-valued neural networks with delay and stochastic impulses, Neural Process. Lett., 54 (2022), 691–708. https://doi.org/10.1007/s11063-021-10653-0 doi: 10.1007/s11063-021-10653-0
![]() |
[38] |
U. Humphries, G. Rajchakit, P. Kaewmesri, P. Chanthorn, R. Sriraman, R. Samidurai, C. P. Lim, Stochastic memristive quaternion-valued neural networks with time delays: An analysis on mean square exponential input-to-state stability, Mathematics, 8 (2020), 815. https://doi.org/10.3390/math8050815 doi: 10.3390/math8050815
![]() |
[39] |
T. Takagi, M. Sugeno, Fuzzy identification of systems and its applications to modeling and control, IEEE Trans. Syst. Man Cybern., 15 (1985), 116–132. https://doi.org/10.1109/TSMC.1985.6313399 doi: 10.1109/TSMC.1985.6313399
![]() |
[40] | K. Tanaka, H. O. Wang, Fuzzy Control Systems Design and Analysis: A Linear Matrix Inequality Approach, Wiley, New York, (2001). |
[41] |
C. K. Ahn, Delay-dependent state estimation for T-S fuzzy delayed Hopfield neural networks, Nonlinear Dyn., 61 (2010), 483–489. https://doi.org/10.1007/s11071-010-9664-z doi: 10.1007/s11071-010-9664-z
![]() |
[42] |
R. Li, J. Cao, Passivity and dissipativity of fractional-order quaternion-valued fuzzy memristive neural networks: Nonlinear scalarization approach, IEEE Trans. Cybern., 52 (2022), 2821–2832. https://doi.org/10.1109/TCYB.2020.3025439 doi: 10.1109/TCYB.2020.3025439
![]() |
[43] |
B. Liu, P. Shi, Delay-range-dependent stability for fuzzy BAM neural networks with time-varying delays, Phys. Lett. A, 373 (2009), 1830–1838. https://doi.org/10.1016/j.physleta.2009.03.044 doi: 10.1016/j.physleta.2009.03.044
![]() |
[44] |
R. Sriraman, P. Vignesh, V. C. Amritha, G. Rachakit, P. Balaji, Direct quaternion method-based stability criteria for quaternion-valued Takagi-Sugeno fuzzy BAM delayed neural networks using quaternion-valued Wirtinger-based integral inequality, AIMS Math., 8 (2023), 10486–10512. https://doi.org/10.3934/math.2023532 doi: 10.3934/math.2023532
![]() |
[45] |
J. Jian, P. Wan, Global exponential convergence of fuzzy complex-valued neural networks with time-varying delays and impulsive effects, Fuzzy Sets Syst., 338 (2018), 23–39. https://doi.org/10.1016/j.fss.2017.12.001 doi: 10.1016/j.fss.2017.12.001
![]() |
[46] |
R. Sriraman, N. Asha, Global stability of Clifford-valued Takagi-Sugeno fuzzy neural networks with time-varying delays and impulses, Kybernetika, 58 (2022), 498–521. https://doi.org/10.14736/kyb-2022-4-0498 doi: 10.14736/kyb-2022-4-0498
![]() |
[47] | Y. Cao, S. Ramajayam, R. Sriraman, R. Samidurai, Leakage delay on stabilization of finite-time complex-valued BAM neural network: Decomposition approach, Neurocomputing, 463 (2021), 505–513. https://doi.org/10.1016/j.neucom.2021.08.056 |
[48] | R. Samidurai, R. Sriraman, S. Zhu, Leakage delay-dependent stability analysis for complex-valued neural networks with discrete and distributed time-varying delays, Neurocomputing, 338 (2021), 262–273. https://doi.org/10.1016/j.neucom.2019.02.027 |
[49] |
F. Zhang, Quaternions and matrices of quaternions, Linear Algebra Appl., 251 (1997), 21–57. https://doi.org/10.1016/0024-3795(95)00543-9 doi: 10.1016/0024-3795(95)00543-9
![]() |
[50] |
C. Pradeep, A. Chandrasekar, R. Murugesu, R. Rakkiyappan, Robust stability analysis of stochastic neural networks with Markovian jumping parameters and probabilistic time-varying delays, Complexity, 21 (2016), 59–72. https://doi.org/10.1002/cplx.21630 doi: 10.1002/cplx.21630
![]() |
[51] |
X. Chen, Z. Li, Q. Song, J. Hu, Y. Tan, Robust stability analysis of quaternion-valued neural networks with time delays and parameter uncertainties, Neural Netw., 91 (2017), 55–65. https://doi.org/10.1016/j.neunet.2017.04.006 doi: 10.1016/j.neunet.2017.04.006
![]() |
[52] |
P. G. Park, J. W. Ko, C. Jeong, Reciprocally convex approach to stability of systems with time-varying delays, Automatica, 47 (2011), 235–238. https://doi.org/10.1016/j.automatica.2010.10.014 doi: 10.1016/j.automatica.2010.10.014
![]() |
1. | Omar Kahouli, Amina Turki, Mohamed Ksantini, Mohamed Ali Hammami, Ali Aloui, On the boundedness of solutions of some fuzzy dynamical control systems, 2024, 9, 2473-6988, 5330, 10.3934/math.2024257 | |
2. | Dehao Ruan, Yao Lu, Generalized exponential stability of neutral stochastic quaternion-valued neural networks with variable coefficients and infinite delay, 2024, 191, 01676911, 105869, 10.1016/j.sysconle.2024.105869 | |
3. | Runtian Zeng, Qiankun Song, Shuning Sun, Quaternion version of the Itô’s formula, 2024, 222, 03784754, 242, 10.1016/j.matcom.2023.08.040 |