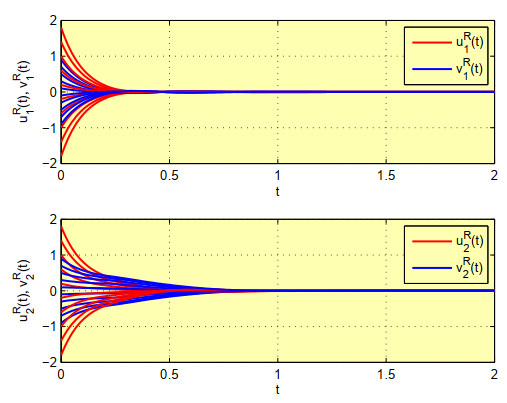
This paper investigates the global asymptotic stability problem for a class of quaternion-valued Takagi-Sugeno fuzzy BAM neural networks with time-varying delays. By applying Takagi-Sugeno fuzzy models, we first consider a general form of quaternion-valued Takagi-Sugeno fuzzy BAM neural networks with time-varying delays. Then, we apply the Cauchy-Schwarz algorithm and homeomorphism principle to obtain sufficient conditions for the existence and uniqueness of the equilibrium point. By utilizing suitable Lyapunov-Krasovskii functionals and newly developed quaternion-valued Wirtinger-based integral inequality, some sufficient criteria are obtained to guarantee the global asymptotic stability of the considered networks. Further, the results of this paper are presented in the form of quaternion-valued linear matrix inequalities, which can be solved using the MATLAB YALMIP toolbox. Two numerical examples are presented with their simulations to demonstrate the validity of the theoretical analysis.
Citation: R. Sriraman, P. Vignesh, V. C. Amritha, G. Rachakit, Prasanalakshmi Balaji. Direct quaternion method-based stability criteria for quaternion-valued Takagi-Sugeno fuzzy BAM delayed neural networks using quaternion-valued Wirtinger-based integral inequality[J]. AIMS Mathematics, 2023, 8(5): 10486-10512. doi: 10.3934/math.2023532
[1] | R. Sriraman, R. Samidurai, V. C. Amritha, G. Rachakit, Prasanalakshmi Balaji . System decomposition-based stability criteria for Takagi-Sugeno fuzzy uncertain stochastic delayed neural networks in quaternion field. AIMS Mathematics, 2023, 8(5): 11589-11616. doi: 10.3934/math.2023587 |
[2] | Abdulaziz M. Alanazi, R. Sriraman, R. Gurusamy, S. Athithan, P. Vignesh, Zaid Bassfar, Adel R. Alharbi, Amer Aljaedi . System decomposition method-based global stability criteria for T-S fuzzy Clifford-valued delayed neural networks with impulses and leakage term. AIMS Mathematics, 2023, 8(7): 15166-15188. doi: 10.3934/math.2023774 |
[3] | Ailing Li, Mengting Lv, Yifang Yan . Asymptotic stability for quaternion-valued BAM neural networks via a contradictory method and two Lyapunov functionals. AIMS Mathematics, 2022, 7(5): 8206-8223. doi: 10.3934/math.2022457 |
[4] | Li Zhu, Er-yong Cong, Xian Zhang . Global exponential stability conditions for quaternion-valued neural networks with leakage, transmission and distribution delays. AIMS Mathematics, 2023, 8(8): 19018-19038. doi: 10.3934/math.2023970 |
[5] | Rupak Datta, Ramasamy Saravanakumar, Rajeeb Dey, Baby Bhattacharya . Further results on stability analysis of Takagi–Sugeno fuzzy time-delay systems via improved Lyapunov–Krasovskii functional. AIMS Mathematics, 2022, 7(9): 16464-16481. doi: 10.3934/math.2022901 |
[6] | Nina Huo, Bing Li, Yongkun Li . Global exponential stability and existence of almost periodic solutions in distribution for Clifford-valued stochastic high-order Hopfield neural networks with time-varying delays. AIMS Mathematics, 2022, 7(3): 3653-3679. doi: 10.3934/math.2022202 |
[7] | Jin Gao, Lihua Dai . Anti-periodic synchronization of quaternion-valued high-order Hopfield neural networks with delays. AIMS Mathematics, 2022, 7(8): 14051-14075. doi: 10.3934/math.2022775 |
[8] | YeongJae Kim, YongGwon Lee, SeungHoon Lee, Palanisamy Selvaraj, Ramalingam Sakthivel, OhMin Kwon . Design and experimentation of sampled-data controller in T-S fuzzy systems with input saturation through the use of linear switching methods. AIMS Mathematics, 2024, 9(1): 2389-2410. doi: 10.3934/math.2024118 |
[9] | Xiaofang Meng, Yongkun Li . Pseudo almost periodic solutions for quaternion-valued high-order Hopfield neural networks with time-varying delays and leakage delays on time scales. AIMS Mathematics, 2021, 6(9): 10070-10091. doi: 10.3934/math.2021585 |
[10] | Wenxiang Fang, Tao Xie . Robustness analysis of stability of Takagi-Sugeno type fuzzy neural network. AIMS Mathematics, 2023, 8(12): 31118-31140. doi: 10.3934/math.20231593 |
This paper investigates the global asymptotic stability problem for a class of quaternion-valued Takagi-Sugeno fuzzy BAM neural networks with time-varying delays. By applying Takagi-Sugeno fuzzy models, we first consider a general form of quaternion-valued Takagi-Sugeno fuzzy BAM neural networks with time-varying delays. Then, we apply the Cauchy-Schwarz algorithm and homeomorphism principle to obtain sufficient conditions for the existence and uniqueness of the equilibrium point. By utilizing suitable Lyapunov-Krasovskii functionals and newly developed quaternion-valued Wirtinger-based integral inequality, some sufficient criteria are obtained to guarantee the global asymptotic stability of the considered networks. Further, the results of this paper are presented in the form of quaternion-valued linear matrix inequalities, which can be solved using the MATLAB YALMIP toolbox. Two numerical examples are presented with their simulations to demonstrate the validity of the theoretical analysis.
Over the past few years, neural networks (NNs) and their generalizations have attracted considerable attention for their ability to address the problems involving associative memory, secure communications, signal and image processing, optimization, and others [1,2,3,4]. In 1987, Kosko created a significant type of two-layer hetero-associative memory network named bidirectional associative memory (BAM) NNs [5]. Then, BAM NNs have attracted significant attention due to their wide range of applications, such as data mining, pattern recognition, automatic control, and others [6,7,8]. In recent years, several theoretical studies regarding various dynamics of BAM NNs based on Lyapunov-Krasovskii functionals (LKFs) and linear matrix inequalities (LMIs) have been published [9,10,11,12,13,14,15,16,17].
There is no doubt that real-, complex-valued NNs have been successfully applied to a variety of engineering applications [18,19,20,21,22,23]. However, these two NNs have some limitations when it comes to symmetry detection and high-dimensional data problems [24]. In order to address these issues, some scholars have developed quaternion-valued NNs by incorporating quaternions into standard NNs. Moreover, quaternion-valued NNs have shown superior performance than real-, complex-valued NNs because of their general representation and high-efficiency [25,26,27]. Therefore, the research on quaternion-valued NNs has become a popular topic in modern science [28,29,30,31]. Recently, several researchers have investigated various dynamics of quaternion-valued NNs using LKFs and LMI methods [15,16,29,30,31]. Particularly, by utilizing homeomorphism principle and the Lyapunov stability method, the global μ-stability analysis for quaternion-valued NNs with unbounded distributed delays has been investigated in [29], besides the quaternion-valued NNs are decomposed into two complex-valued NNs. By applying the direct quaternion method and LMI algorithm, new stability conditions for neutral-type quaternion-valued NNs with discrete delays were established in [30]. Some exponential stability criteria of quaternion-valued discrete-time NNs with leakage and discrete delays were presented in [31]. There are similar results to be found in [15,16].
In [32], Takagi-Sugeno (T-S) proposed the fuzzy systems, which have been effectively utilized for modeling and analyzing complex nonlinear systems [32,33]. The T-S fuzzy system has the advantage of being able to approximate a nonlinear system with a set of linear models. It should be noted that, unlike typical NN structures, T-S fuzzy NNs have fuzzy operations, and they are able to preserve the direct correlation between the cells. Due to their good approximation properties, T-S fuzzy NNs have proved to be an important research topic. Many papers have proposed the idea of incorporating fuzzy logic into the NNs in order to enhance their performance [34,35,36,37]. For example, by considering impulsive effects and time delays, the authors of [36] have determined the exponential convergence for T-S fuzzy complex-valued NNs.
On the other hand, time delays inherently occur in NN implementations, and they can cause undesirable system behaviors. Therefore, it is essential to study how delays affect the system's dynamics. Recently, various time delays have been extensively studied in a variety of dynamic models, especially in control theory [38,39,40]. Furthermore, there are two types of stability criteria that are associated with delayed NNs: delay-dependent stability criteria and delay-independent stability criteria. Recently, delay-dependent stability criteria have received significant attention compared to delay-independent criteria because of their practical importance [41,42]. On the other hand, the main purpose of delay-dependent stability criteria is to obtain less conservatism of the obtained results. Generally, there are two factors that contribute to less conservative stability conditions, namely the augmented LKFs and the tighter bound integral inequalities. Recently, a number of studies have developed various integral inequalities in order to handle integral terms in the real domain [44,45,46]. However, only Jensen's inequality has been utilized since the beginning to deal with integral terms in the quaternion domain. To fill such gaps, a new quaternion-valued integral inequality has been developed in this paper, which includes the famous Wirtinger-based integral inequality (WBII) [44] and the reciprocal convex combination (RCC) lemma [43].
Following the above discussions, in this paper, we aim to investigate the global asymptotic stability of quaternion-valued T-S fuzzy BAM NNs by applying the direct quaternion method. There are several results discussed in the literature regarding various stability problems of quaternion-valued BAM NNs, however, quaternion-valued T-S fuzzy BAM NNs have not been fully explored and are not receiving much attention, which motivates us to investigate this topic. This paper has the following main merits: 1) To represent more realistic dynamical behaviors of quaternion-valued NNs, we present a general form of the quaternion-valued T-S fuzzy BAM NNs with time delays for the first time in this paper. 2) The direct quaternion method is employed to examine the global asymptotic stability of quaternion-valued T-S fuzzy BAM NNs for the first time in this paper. 3) By considering suitable LKFs that contain double integral terms and by employing newly developed quaternion-valued WBII, enhanced stability conditions for the concerned NN model are derived in the form of quaternion-valued LMIs, which could be verified directly by MATLAB YALMIP toolbox. 4) The proofs for the quaternion-valued WBII and quaternion-valued RCC lemma are presented for the first time in this paper.
The paper is structured as follows: Section 2 provides the problem model, definitions of global asymptotic stability, assumptions about activation functions and time-varying delays, and some useful lemmas. The results of this study are stated in Section 3; Theorem (3.1) presents sufficient criteria for the existence and uniqueness of the equilibrium point; Theorem (3.2) provides sufficient criteria for the global asymptotic stability of the considered network models. In Corollary (3.4), (3.6), the results of stability criteria are discussed in a special case. Section 4 discusses two numerical case studies that demonstrate the feasibility of the derived results. Section 5 shows the conclusion of this paper.
Let the set of all quaternion, complex, and real numbers are denoted by H,C and R, respectively. The symbols Hn,Cn and Rn stand for n-dimensional quaternion, complex and real vectors, respectively. The quaternion, complex and real matrices of size n×n are represented by the symbols Hn×n,Cn×n and Rn×n, respectively. Let the matrix P<0 (P>0) means P is negative (positive) definite matrix. The block diagonal matrix is shown in diag{⋅}. The conjugate transposition and matrix transposition, respectively, are denoted by the superscripts ∗ and T. The symmetric term in a matrix is showed by ⋆.
The quaternion was first invented by Hamilton in 1843. The skew field of a quaternion is denoted by
z=zR+izI+jzJ+kzK∈H, |
where zR,zI,zJ,zK∈R, z is the quaternion-valued input and i,j,k are the quaternion basis which subjects to Hamilton's multiplication rules as follows:
k2=j2=i2=−1 jk=−kj=i, ki=−ik=j, ij=−ji=k. |
The conjugate of the quaternion as follows:
ˉz=zR−izI−jzJ−kzK∈H. |
The modulus of the quaternion as follows:
|z|=√zˉz=√(zR)2+(zI)2+(zJ)2+(zK)2. |
Let x=xR+ixI+jxJ+kxK∈H and y=yR+iyI+jyJ+kyK∈H. The addition and multiplication of two quaternions can be accomplished as follows:
x+y= (xR+yR)+i(xI+yI)+j(xJ+yJ)+k(xK+yK),xy= (xRyR−xIyI−xJyJ−xKyK)+i(xRyI+xIyR+xJyK−xKyJ)+j(xRyJ+xJyR−xIyK+xKyI)+k(xRyK+xKyI+xIyJ−xJyI). |
In this paper, we consider the quaternion-valued BAM NNs with time-varying delays as follows:
{˙pr(t)= −d1rpr(t)+m∑s=1a1rsf1s(qs(t−ℓ(t)))+J1r, r=1,2,...,n,pr(t)= φ1r(t), t∈[−ℓ,0],˙qs(t)= −d2sqs(t)+n∑r=1a2srf2r(pr(t−ℓ(t)))+J2s, s=1,2,...,m,qs(t)= φ2s(t), t∈[−ℓ,0], | (2.1) |
or equivalently
{˙p(t)= −D1p(t)+A1f1(q(t−ℓ(t)))+J1,p(t)= φ1(t), t∈[−ℓ,0],˙q(t)= −D2q(t)+A2f2(p(t−ℓ(t)))+J2,q(t)= φ2(t), t∈[−ℓ,0], | (2.2) |
where p(t)=[p1(t),....,pn(t)]T∈Hn, q(t)=[q1(t),...,qm(t)]T∈Hm are the state vectors; f1(q(⋅))=[f11(q1(⋅)),...,f1m(qm(⋅))]T∈Hm, f2(p(⋅))=[f21(p1(⋅)),...,f2n(pn(⋅))]T∈Hn are the vector valued activation functions; J1=[J11,...,J1n]T∈Hn, J2=[J21,...,J2m]T∈Hm are the input vectors. D1=diag{d11,...,d1n}∈Rn×n, D2=diag{d21,...,d2m}∈Rm×m are the self-feedback connection weight matrices with each d1r>0, d2s>0, r=1,...,n, s=1,...,m. A1∈Hn×m,A2∈Hm×n are the delayed interconnection weight matrices. φ1∈C([−ℓ,0],Hn) and φ2∈C([−ℓ,0],Hm) are the initial conditions.
This paper assumes that the activation functions f1(q(t−ℓ(t))), f2(p(t−ℓ(t))) and transmission delays ℓ(t) satisfy the following conditions:
Assumption 1: The activation functions f1s and f2r are Lipschitz continuous; that is, there exist positive constants lf1s>0,lf2r>0, such that for all r=1,2,...,n, s=1,2,...,m
|f1s(x)−f1s(y)|≤lf1s|x−y|, ∀x,y∈H,|f2r(x)−f2r(y)|≤lf2r|x−y|, ∀x,y∈H. |
Furthermore, we define Lf1=diag{lf11,lf12,...,lf1m}, Lf2=diag{lf21,lf22,...,lf2n}.
Assumption 2: The delay ℓ(t):R→R is differentiable and bounded, which satisfy the conditions 0≤ℓ(t)≤ℓ, ˙ℓ(t)≤μ<1, where ℓ and μ are real numbers.
In order to derive our main results, we present some definition and lemmas.
Definition 2.1. For the NN model (2.2) with any initial conditions φ1∈C([−ℓ,0],Hn) and φ2∈C([−ℓ,0],Hm), the trivial solution is called globally asymptotically stable (GAS) if limt→+∞{‖p(t,φ1)‖2+‖q(t,φ2)‖2}=0, where p(t,φ1) and q(t,φ2) are the solutions of NN (2.2) at time t under the initial conditions φ1 and φ2, respectively.
Lemma 2.2. [47] For any vectors p,q∈Hn and a scalar ϵ>0, then the following inequality holds: p∗q+q∗p≤ϵp∗p+ϵ−1q∗q.
Lemma 2.3. [47] Let H(p,q):Hn+m→Hn+m is a continuous map that fulfills the following criteria:
(i) H(p,q) is injective on Hn+m,
(ii) ‖H(p,q)‖→∞ as ‖(p,q)‖→∞, then H is homeomorphism of Hn+m onto itself.
Lemma 2.4. [48] Let α,β∈H; X,Y∈Hn×n and Hermitian matrix 0<P∈Hn×n, then
(1) |α+β|≤|α|+|β| and |αβ|=|βα|=|α||β|,
(2) (¯X)T=¯(XT),
(3) (XY)∗=Y∗X∗,
(4) (XY)−1=Y−1X−1, if X and Y are invertible,
(5) (A∗)−1=(A−1)∗, if A is invertible,
(6) Any quaternion α can be formulated uniquely as α=γ1+γ2j, where γ1,γ2∈C,
(7) jγ=¯γj or jγj∗=¯γ, for all γ∈C,
(8) There exist an invertible matrix Z∈Hn×n, i.e. P=Z∗Z.
Lemma 2.5. [49] Let X,Y∈Hn×n; X1,X2,Y1,Y2∈Cn×n and X=X1+X2j, Y=Y1+Y2j. Then
(1) X∗=X∗1−XT2j,
(2) XY=(X1Y1−X2¯Y2)+(X1Y2+X2¯Y1)j.
Lemma 2.6. [50] A Hermitian matrix W=WR+iWI+jWJ+kWK∈Hn×n, then W<0 is equivalent to
[WR−WJ−WIWKWJWRWKWIWI−WKWR−WJ−WK−WIWJWR]<0. |
where WR=Re(W), WI=Im(W), WJ=Im(W) and WK=Im(W).
Remark 2.7. As indicated in Lemma (3.3), the n×n Hermitian matrix equals the 4n×4n real matrix. With the aid of this lemma, it is possible to transform the quaternion-valued LMIs into the real-valued LMIs and vice versa.
Lemma 2.8. (Quaternion-valued WBII) For every differentiable function w:[a,b]→Hn and Hermitian matrix 0<W=WR+iWI+jWJ+kWK∈Hn×n, the following criteria holds:
∫baw∗(s)Ww(s)ds≥1b−a[ζ1ζ2]∗[W003W][ζ1ζ2], |
where
ζ1= ∫baw(s)ds,ζ2= ∫baw(s)ds−2b−a∫ba∫buw(s)dsdu. |
Proof: Let w(s)=wR(s)+iwI(s)+jwJ(s)+kwK(s)∈H, ζ1=ζR1+iζI1+jζJ1+kζK1∈H, ζ2=ζR2+iζI2+jζJ2+kζK2∈H, W=WR+iWI+jWJ+kWK∈Hn×n, where W∗=W⇔(WR)T=WR, −(WI)T=WI, −(WJ)T=WJ, (WK)T=−WK. Using Lemma (2.6) and WBII [44], we get
∫baw∗(s)Ww(s)ds=∫ba[wR(s)wI(s)wJ(s)wK(s)]T[WR−WJ−WIWKWJWRWKWIWI−WKWR−WJ−WK−WIWJWR][wR(s)wI(s)wJ(s)wK(s)]ds,≥1b−a[ζR1ζI1ζJ1ζK1ζR2ζI2ζJ2ζK2]T[WR−WJ−WIWK0000WJWRWKWI0000WI−WKWR−WJ0000−WK−WIWJWR000000003WR−3WJ−3WI3WK00003WJ3WR3WK3WI00003WI−3WK3WR−3WJ0000−3WK−3WI3WJ3WR][ζR1ζI1ζJ1ζK1ζR2ζI2ζJ2ζK2],=1b−a[ζ1ζ2]∗[W003W][ζ1ζ2]. |
Lemma 2.9. (Quaternion-valued RCC lemma) For any vectors ζ1,ζ2∈Hn, Hermitian matrix 0<E∈Hn×n, any matrix F∈Hn×n and any α∈(0,1), such that [EFF∗E]≥0, the following criteria holds
1αζ∗1Eζ1+11−αζ∗2Eζ2≥[ζ1ζ2]∗[EFF∗E][ζ1ζ2]. |
Proof: Let ζ1=ζR1+iζI1+jζJ1+kζK1∈H; ζ2=ζR2+iζI2+jζJ2+kζK2∈H; E=ER+iEI+jEJ+kEK∈Hn×n, where E∗=E⇔(ER)T=ER, −(EI)T=EI, −(EJ)T=EJ, (EK)T=−EK; F=FR+iFI+jFJ+kFK∈Hn×n. Using Lemma (2.6) and RCC lemma [43], we get
1αζ∗1Eζ1+ 11−αζ∗2Eζ2=1α[ζR1ζI1ζJ1ζK1]T[ER−EJ−EIEKEJEREKEIEI−EKER−EJ−EK−EIEJER][ζR1ζI1ζJ1ζK1]+11−α[ζR2ζI2ζJ2ζK2]T[ER−EJ−EIEKEJEREKEIEI−EKER−EJ−EK−EIEJER][ζR2ζI2ζJ2ζK2],≥ [ζR1ζI1ζJ1ζK1ζR2ζI2ζJ2ζK2]T[ER−EJ−EIEKFR−FJ−FIFKEJEREKEIFJFRFKFIEI−EKER−EJFI−FKFR−FJ−EK−EIEJER−FK−FIFJFR(FR)T(FJ)T(FI)T−(FK)TER−EJ−EIEK−(FJ)T(FR)T−(FK)T−(FI)TEJEREKEI−(FI)T(FK)T(FR)T(FJ)TEI−EKER−EJ(FK)T(FI)T−(FJ)T(FR)T−EK−EIEJER][ζR1ζI1ζJ1ζK1ζR2ζI2ζJ2ζK2],= [ζ1ζ2]∗[EFF∗E][ζ1ζ2]. |
This section presents new sufficient criteria to ensure the global asymptotic stability of the considered NN model based on the LKFs and LMI method, as well as quaternion-valued WBII.
In this subsection, we discuss sufficient criteria that guarantee the existence and uniqueness of the equilibrium point for NNs (2.2).
Theorem 3.1. Under Assumptions 1 and 2, the NN model (2.2) has a unique equilibrium point if there exist positive definite Hermitian matrices 0<P1, 0<P2, and diagonal matrix 0<G1, 0<G2, such that the following LMIs hold:
[−P1D1−D1P1+L∗f2G2Lf2P1A1⋆−G1]<0, | (3.1) |
[−P2D2−D2P2+L∗f1G1Lf1P2A2⋆−G2]<0, | (3.2) |
Proof: Define the function H(p,q):Hn+m→Hn+m by
H(p,q)=−[D100D2][pq]+[A100A2][f1(q)f2(p)]+[J1J2]. | (3.3) |
We start by demonstrating that H(p,q) is injective. Assume by contradiction that there exist [pq], [p′q′]∈Hn+m, [pq]≠[p′q′], such that H(p,q)=H(p′,q′), or equivalently
H(p,q)−H(p′,q′)= −[D100D2][p−p′q−q′]+[A100A2][f1(q)−f1(q′)f2(p)−f2(p′)]=0. | (3.4) |
Pre multiplication on both sides of (3.4) with [p−p′q−q′]∗[P100P2], we get
[p−p′q−q′]∗[P100P2]×(−[D100D2][p−p′q−q′]+[A100A2][f1(q)−f1(q′)f2(p)−f2(p′)])=0, | (3.5) |
that is
−(p−p′)∗P1D1(p−p′)−(q−q′)∗P2D2(q−q′)+(p−p′)∗P1A1(f1(q)−f1(q′))+(q−q′)∗P2A2(f2(p)−f2(p′))=0. | (3.6) |
Applying the complex conjugate, we get
−(p−p′)∗D1P1(p−p′)−(q−q′)∗D2P2(q−q′)+(f1(q)−f1(q′))∗A∗1P1(p−p′)+(f2(p)−f2(p′))∗A∗2P2(q−q′)=0. | (3.7) |
By combining (3.6) and (3.7), one has
−(p−p′)∗(P1D1+D1P1)(p−p′)−(q−q′)∗(P2D2+D2P2)(q−q′)+2(p−p′)∗P1A1(f1(q)−f1(q′))+2(q−q′)∗P2A2(f2(p)−f2(p′))=0. | (3.8) |
From Lemma (2.2), there exist diagonal matrices 0<G1, 0<G2, yields
2(p−p′)∗P1A1(f1(q)−f1(q′))≤ (p−p′)∗P1A1G−11A∗1P1(p−p′)+(f1(q)−f1(q′))∗G1(f1(q)−f1(q′)), | (3.9) |
2(q−q′)∗P2A2(f2(p)−f2(p′))≤ (q−q′)∗P2A2G−12A∗2P2(q−q′)+(f2(p)−f2(p′))∗G2(f2(p)−f2(p′)). | (3.10) |
Substituting (3.9) and (3.10) in (3.8), we get
−(p−p′)∗(P1D1+D1P1)(p−p′)−(q−q′)∗(P2D2+D2P2)(q−q′)+(p−p′)∗P1A1G−11A∗1P1(p−p′)+(f1(q)−f1(q′))∗G1(f1(q)−f1(q′))+(q−q′)∗P2A2G−12A∗2P2(q−q′)+(f2(p)−f2(p′))∗G2(f2(p)−f2(p′))≤0. | (3.11) |
Based on Assumption 1, we get
(f1(q)−f1(q′))∗G1(f1(q)−f1(q′))≤(q−q′)∗L∗f1G1Lf1(q−q′), | (3.12) |
(f2(p)−f2(p′))∗G2(f2(p)−f2(p′))≤(p−p′)∗L∗f2G2Lf2(p−p′). | (3.13) |
Incorporating (3.11)–(3.13), we get
(p−p′)∗(−P1D1−D1P1)(p−p′)+(q−q′)∗(−P2D2−D2P2)(q−q′)+(p−p′)∗P1A1G−11A∗1P1(p−p′)+(q−q′)∗L∗f1G1Lf1(q−q′)+(q−q′)∗P2A2G−12A∗2P2(q−q′)+(p−p′)∗L∗f2G2Lf2(p−p′)≤0,(p−p′)∗(−P1D1−D1P1+P1A1G−11A∗1P1+L∗f2G2Lf2)(p−p′)+(q−q′)∗(−P2D2−D2P2+P2A2G−12A∗2P2+L∗f1G1Lf1)(q−q′)≤0, | (3.14) |
which implies that
−P1D1−D1P1+P1A1G−11A∗1P1+L∗f2G2Lf2<0, | (3.15) |
−P2D2−D2P2+P2A2G−12A∗2P2+L∗f1G1Lf1<0. | (3.16) |
Using Schur's complement can be directly inferred from the criteria which can be immediately deduced from (3.1) and (3.2). This contradicts that H(p,q)−H(p′,q′)<0 with initial conditions. Hence the function H(p,q) is injective.
Now, we shall show that ‖H(p,q)‖→∞ as ‖(p,q)‖→∞. We infer that from (3.15), (3.16) and small constant, ϵ>0 exist, such that
−P1D1−D1P1+P1A1G−11A∗1P1+L∗f2G2Lf2<−ϵIn,−P2D2−D2P2+P2A2G−12A∗2P2+L∗f1G1Lf1<−ϵIm. |
Taking (p′,q′)=(0,0), and using (3.14) and the above relations, we have
[pq]∗[P100P2](H(p,q)−H(0,0))≤ p∗(−P1D1−D1P1+P1A1G−11A∗1P1+L∗f2G2Lf2)p+q∗(−P2D2−D2P2P2A2G−12A∗2P2+L∗f1G1Lf1)q≤−ϵ(‖p‖2+‖q‖2). | (3.17) |
Using the Cauchy-Schwarz inequality, then (3.17) becomes:
ϵ(‖p‖2+‖q‖2)≤2‖(p,q)‖ ‖P1‖ ‖P2‖ (‖H(p,q)‖+‖H(0,0)‖), | (3.18) |
which gives result that ‖H(p,q)‖→∞ as ‖(p,q)‖→∞. Hence, the map H(p,q) satisfies all conditions in Lemma (2.3) and is homeomorphism of Hn+m onto itself. Then, there exist (p∗,q∗) such that H(p∗,q∗)=0, that is, NN (2.2) has a unique equilibrium point (p∗,q∗).
Let u(t)=p(t)−p∗, v(t)=q(t)−q∗, we can get
{˙u(t)= −D1u(t)+A1g1(v(t−ℓ(t))),u(t)= ϕ1(t), t∈[−ℓ,0],˙v(t)= −D2v(t)+A2g2(u(t−ℓ(t))),v(t)= ϕ2(t), t∈[−ℓ,0], | (3.19) |
where g1(v(t−ℓ(t)))=f1(q(t−ℓ(t))+q∗+J1)−f1(q∗+J1) and g2(u(t−ℓ(t)))=f2(p(t−ℓ(t))+p∗+J2)−f2(p∗+J2), ϕ1=φ1−p∗, ϕ2=φ2−q∗, ϕ1∈C([−ℓ,0],Hn) and ϕ2∈C([−ℓ,0],Hm) are continuous functions.
Assumption 3: The activation functions g1s and g2r are Lipschitz continuous; that is, there exist positive constants lg1s>0,lg2r>0, such that for all r=1,2,...,n, s=1,2,...,m
|g1s(x)−g1s(y)|≤lg1s|x−y|, ∀x,y∈H,|g2r(x)−g2r(y)|≤lg2r|x−y|, ∀x,y∈H. |
Furthermore, we define Lg1=diag{lg11,lg12,...,lg1m}, Lg2=diag{lg21,lg22,...,lg2n}.
Based on the works [32,33,36], the T-S fuzzy delayed quaternion-valued NNs can be described as bellow.
Plant Rule h:
If {ϑ1(t) is ηh1}, {ϑ2(t) is ηh2},...,{ϑg(t) is ηhg}.
Then
{˙u(t)= −Dh1u(t)+Ah1g1(v(t−ℓ(t))),u(t)= ϕ1(t), t∈[−ℓ,0],˙v(t)= −Dh2v(t)+Ah2g2(u(t−ℓ(t))),v(t)= ϕ2(t), t∈[−ℓ,0], | (3.20) |
where the premise variables are ϑc(t), c=1,...,g, the fuzzy sets are ηhc, h=1,...,m and m is the total number of If-Then rules.
Hence, the T-S fuzzy quaternion-valued NN can be achieved by inferring from the fuzzy NN model (3.20), as follows
{˙u(t)= m∑h=1wh(ϑ(t)){−Dh1u(t)+Ah1g1(v(t−ℓ(t)))}m∑h=1wh(ϑ(t)),u(t)= ϕ1(t), t∈[−ℓ,0],˙v(t)= m∑h=1wh(ϑ(t)){−Dh2v(t)+Ah2g2(u(t−ℓ(t)))}m∑h=1wh(ϑ(t)),v(t)= ϕ2(t), t∈[−ℓ,0], | (3.21) |
or equivalently
{˙u(t)= m∑h=1ψh(ϑ(t)){−Dh1u(t)+Ah1g1(v(t−ℓ(t)))},u(t)= ϕ1(t), t∈[−ℓ,0],˙v(t)= m∑h=1ψh(ϑ(t)){−Dh2v(t)+Ah2g2(u(t−ℓ(t)))},v(t)= ϕ2(t), t∈[−ℓ,0], | (3.22) |
where ϑ(t)=(ϑ1(t),...,ϑg(t))T, ψh(ϑ(t))=wh(ϑ(t))m∑h=1wh(ϑ(t)) and wh(ϑ(t))=g∏c=1ηhc(ϑ(t)). The term ηhc(ϑ(t)) is the grade membership of ϑc(t) in ηhc. It is stated that wh(ϑ(t))≥0, h=1,...,m and m∑h=1wh(ϑ(t))>0 for all t≥0. By fuzzy set theory, we have ψh(ϑ(t))≥0, h=1,...,m and m∑h=1ψh(ϑ(t))=1 for all t≥0.
Theorem 3.2. Let the activation function and time delay satisfy Assumptions 1–3. Suppose that there exist Hermitian matrices 0<P1, 0<P2, 0<Q1, 0<Q2, 0<R1, 0<R2, 0<S1, 0<S2, and diagonal matrix 0<G1, 0<G2, such that the following conditions holds for all h=1,2,...,m
Ωh=(Ωhi,j)12×12<0, | (3.23) |
where Ωh1,1=−P1Dh1−Dh1P1+Q1+R1+ℓ2S1, Ωh1,10=P1Ah1, Ωh2,2=−(1−μ)R1+L∗g2G1Lg2, Ωh3,3=−Q1, Ωh4,4=−G1, Ωh4,7=A∗2hP2, Ωh5,5=−4S1, Ωh5,6=6S1, Ωh6,6=−12S1, Ωh7,7=−P2Dh2−Dh2P2+Q2+R2+ℓ2S2, Ωh8,8=−(1−μ)R2+L∗g1G2Lg1, Ωh9,9=−Q2, Ωh10,10=−G2, Ωh11,11=−4S2, Ωh11,12=6S2, Ωh12,12=−12S2, then the equilibrium point of NN model (3.22) is GAS.
Proof: Consider the LKF (3.24) for NNs (3.22) described by
V(t,u(t),v(t),h)= [u(t)v(t)]∗[P100P2][u(t)v(t)]+∫tt−ℓ[u(s)v(s)]∗[Q100Q2][u(s)v(s)]ds+∫tt−ℓ(t)[u(s)v(s)]∗[R100R2][u(s)v(s)]ds+ℓ∫0−ℓ∫tt+r[u(s)v(s)]∗[S100S2][u(s)v(s)]dsdr. | (3.24) |
The time-derivative of V(t,u(t),v(t),h) can be obtained as
˙V(t,u(t),v(t),h)= 2[u(t)v(t)]∗[P100P2][˙u(t)˙v(t)]+[u(t)v(t)]∗[Q100Q2][u(t)v(t)]−[u(t−ℓ)v(t−ℓ)]∗[Q100Q2][u(t−ℓ)v(t−ℓ)]+[u(t)v(t)]∗[R100R2][u(t)v(t)]−(1−˙ℓ(t))[u(t−ℓ(t))v(t−ℓ(t))]∗[R100R2][u(t−ℓ(t))v(t−ℓ(t))]+ℓ2[u(t)v(t)]∗[S100S2][u(t)v(t)]−ℓ∫tt−ℓ[u(s)v(s)]∗[S100S2][u(s)v(s)]ds,≤ 2[u(t)v(t)]∗[P100P2][m∑h=1ψh(ϑ(t)){−Dh1u(t)+Ah1g1(v(t−ℓ(t)))}m∑h=1ψh(ϑ(t)){−Dh2v(t)+Ah2g2(u(t−ℓ(t)))}]+[u(t)v(t)]∗[Q100Q2][u(t)v(t)]−[u(t−ℓ)v(t−ℓ)]∗[Q100Q2][u(t−ℓ)v(t−ℓ)]+[u(t)v(t)]∗[R100R2][u(t)v(t)]−(1−μ)[u(t−ℓ(t))v(t−ℓ(t))]∗[R100R2]×[u(t−ℓ(t))v(t−ℓ(t))]+ℓ2[u(t)v(t)]∗[S100S2][u(t)v(t)]−ℓ∫tt−ℓ[u(s)v(s)]∗[S100S2][u(s)v(s)]ds,= m∑h=1ψh(ϑ(t)){u∗(t)(−P1Dh1−Dh1P1+Q1+R1+ℓ2S1)u(t)+u∗(t)(P1Ah1)g1(v(t−ℓ(t)))+v∗(t)(−P2Dh2−Dh2P2+Q2+R2+ℓ2S2)v(t)+v∗(t)(P2Ah2)g2(u(t−ℓ(t))))−u∗(t−ℓ)(Q1)×u(t−ℓ)−v∗(t−ℓ)(Q2)v(t−ℓ)−u∗(t−ℓ(t))((1−μ)R1)×u(t−ℓ(t))−v∗(t−ℓ(t))((1−μ)R2)v(t−ℓ(t))−ℓ∫tt−ℓu∗(s)S1u(s)ds−ℓ∫tt−ℓv∗(s)S2v(s)ds}. | (3.25) |
To obtain tighter bounds for the integral terms −ℓ∫tt−ℓu∗(s)S1u(s)ds, −ℓ∫tt−ℓv∗(s)S2v(s)ds in (3.25), the proposed Lemma (2.8) were applied as follow:
−ℓ∫tt−ℓu∗(s)S1u(s)ds≤−[∫tt−ℓu(s)ds∫tt−ℓu(s)ds−2ℓ∫0−ℓ∫tt+ru(s)dsdr]∗×[S1003S1][∫tt−ℓu(s)ds∫tt−ℓu(s)ds−2ℓ∫0−ℓ∫tt+ru(s)dsdr]= −[∫tt−ℓu(s)ds]∗S1[∫tt−ℓu(s)ds]−[∫tt−ℓu(s)ds]∗3S1×[∫tt−ℓu(s)ds]+[∫tt−ℓu(s)ds]∗6S1[1ℓ∫0−ℓ∫tt+ru(s)dsdr]−[1ℓ∫0−ℓ∫tt+ru(s)dsdr]∗12S1[1ℓ∫0−ℓ∫tt+ru(s)dsdr], | (3.26) |
−ℓ∫tt−ℓv∗(s)S2v(s)ds≤−[∫tt−ℓv(s)ds∫tt−ℓv(s)ds−2ℓ∫0−ℓ∫tt+rv(s)dsdr]∗×[S2003S2][∫tt−ℓv(s)ds∫tt−ℓv(s)ds−2ℓ∫0−ℓ∫tt+rv(s)dsdr]= −[∫tt−ℓv(s)ds]∗S2[∫tt−ℓv(s)ds]−[∫tt−ℓv(s)ds]∗3S2×[∫tt−ℓv(s)ds]+[∫tt−ℓv(s)ds]∗6S2[1ℓ∫0−ℓ∫tt+rv(s)dsdr]−[1ℓ∫0−ℓ∫tt+rv(s)dsdr]∗12S2[1ℓ∫0−ℓ∫tt+rv(s)dsdr]. | (3.27) |
Combining (3.25)–(3.27), we get
˙V(t,u(t),v(t),h)≤m∑h=1ψh(ϑ(t)){u∗(t)(−P1Dh1−Dh1P1+Q1+R1+ℓ2S1)u(t)+u∗(t)×(P1Ah1)g1(v(t−ℓ(t)))+v∗(t)(−P2Dh2−Dh2P2+Q2+R2+ℓ2S2)v(t)+v∗(t)(P2Ah2)×g2(u(t−ℓ(t)))−u∗(t−ℓ)(Q1)u(t−ℓ)−v∗(t−ℓ)(Q2)v(t−ℓ)−u∗(t−ℓ(t))×((1−μ)R1)u(t−ℓ(t))−v∗(t−ℓ(t))((1−μ)R2)v(t−ℓ(t))−[∫tt−ℓu(s)ds]∗(4S1)[∫tt−ℓu(s)ds]+[∫tt−ℓu(s)ds]∗(6S1)[1ℓ∫0−ℓ∫tt+ru(s)dsdr]−[1ℓ∫0−ℓ∫tt+ru(s)dsdr]∗(12S1)[1ℓ∫0−ℓ∫tt+ru(s)dsdr]−[∫tt−ℓv(s)ds]∗(4S2)[∫tt−ℓv(s)ds]+[∫tt−ℓv(s)ds]∗(6S2)[1ℓ∫0−ℓ∫tt+rv(s)dsdr]−[1ℓ∫0−ℓ∫tt+rv(s)dsdr]∗(12S2)[1ℓ∫0−ℓ∫tt+rv(s)dsdr]}. | (3.28) |
From Assumption 3, there exist two diagonal matrices 0<G1, 0<G2 such that
0≤u∗(t−ℓ(t))L∗g2G1Lg2u(t−ℓ(t))−g∗2(u(t−ℓ(t)))G1g2(u(t−ℓ(t))), | (3.29) |
0≤v∗(t−ℓ(t))L∗g1G2Lg1v(t−ℓ(t))−g∗1(v(t−ℓ(t)))G2g1(v(t−ℓ(t))). | (3.30) |
By combining (3.28)–(3.30), we obtain
˙V(t,u(t),v(t),h)≤ m∑h=1ψh(ϑ(t)){ξ∗(t)Ωhξ(t)}. | (3.31) |
where
ξ(t)=[u∗(t) u∗(t−ℓ(t)) u∗(t−ℓ) g∗2(u(t−ℓ(t))) ∫tt−ℓu∗(s)ds 1ℓ∫0−ℓ∫tt+ru∗(s)dsdrv∗(t) v∗(t−ℓ(t)) v∗(t−ℓ) g∗1(v(t−ℓ(t))) ∫tt−ℓv∗(s)ds 1ℓ∫0−ℓ∫tt+rv∗(s)dsdr]∗. |
It is obvious that for Ωh<0,h=1,2,...,m, it shows that the NN (3.22) is GAS according to the Lyapunov stability theory. This completes the proof.
The following shows how our results can be specialized to different cases.
Remark 3.3. When ℓ(t)=ℓ, the NN model (3.22) becomes:
{˙u(t)= m∑h=1ψh(ϑ(t)){−Dh1u(t)+Ah1g1(v(t−ℓ))},u(t)= ϕ1(t), t∈[−ℓ,0],˙v(t)= m∑h=1ψh(ϑ(t)){−Dh2v(t)+Ah2g2(u(t−ℓ))},v(t)= ϕ2(t), t∈[−ℓ,0], | (3.32) |
Corollary 3.4. Let the activation function satisfy Assumptions 3 and time delay ℓ>0. Suppose that there exist Hermitian matrices 0<P1, 0<P2, 0<Q1, 0<Q2, 0<S1, 0<S2, and diagonal matrix 0<G1, 0<G2, such that the following conditions holds for all h=1,2,...,m
ˉΩh=(ˉΩhi,j)10×10<0, | (3.33) |
where ˉΩh1,1=−P1Dh1−Dh1P1+Q1+ℓ2S1, ˉΩh1,8=P1Ah1, ˉΩh2,2=−Q1+L∗g2G1Lg2, ˉΩh3,3=−G1, ˉΩh3,6=A∗2hP2, ˉΩh4,4=−4S1, ˉΩh4,5=6S1, ˉΩh5,5=−12S1, ˉΩh6,6=−P2Dh2−Dh2P2+Q2+ℓ2S2, ˉΩh7,7=−Q2+L∗g1G2Lg1, ˉΩh8,8=−G2, ˉΩh9,9=−4S2, ˉΩh9,10=6S2, ˉΩh10,10=−12S2, then the equilibrium point of NN model (3.32) is GAS.
Proof: Replacing R1=R2=0 in LKF (3.24). The remaining proof is similar to that in Theorem (3.2), and so it is omitted.
Remark 3.5. When m=1 it is a special form of NN model (3.22). For simplicity, we deleted the superscript "1".
{˙u(t)= −D1u(t)+A1g1(v(t−ℓ(t))),u(t)= ϕ1(t), t∈[−ℓ,0],˙v(t)= −D2v(t)+A2g2(u(t−ℓ(t))),v(t)= ϕ2(t), t∈[−ℓ,0], | (3.34) |
Corollary 3.6. Let the activation function and time delay satisfy Assumptions 1-3. Suppose that there exist Hermitian matrices 0<P1, 0<P2, 0<Q1, 0<Q2, 0<R1, 0<R2, 0<S1, 0<S2, and diagonal matrix 0<G1, 0<G2, such that the following conditions holds
˜Ω=(˜Ωi,j)12×12<0, | (3.35) |
where ˜Ω1,1=−P1D1−D1P1+Q1+R1+ℓ2S1, ˜Ω1,10=P1A1, ˜Ω2,2=−(1−μ)R1+L∗g2G1Lg2, ˜Ω3,3=−Q1, ˜Ω4,4=−G1, ˜Ω4,7=A∗2P2, ˜Ω5,5=−4S1, ˜Ω5,6=6S1, ˜Ω6,6=−12S1, ˜Ω7,7=−P2D2−D2P2+Q2+R2+ℓ2S2, ˜Ω8,8=−(1−μ)R2+L∗g1G2Lg1, ˜Ω9,9=−Q2, ˜Ω10,10=−G2, ˜Ω11,11=−4S2, ˜Ω11,12=6S2, ˜Ω12,12=−12S2, then the equilibrium point of NN model (3.34) is GAS.
Proof: Consider the same LKF (3.24). The remaining proof is similar to that in Theorem (3.2), and so it is omitted.
Remark 3.7. Recently, several methods have been proposed to investigate the stability of delayed quaternion-valued NNs [28]-[31]. As an example, (ⅰ) The real-valued decomposition method [28]; (ⅱ) The complex-valued decomposition method [29]; (ⅲ) The direct quaternion method [30]. In general, real-valued and complex-valued decomposition methods have two problems; i.e. they increase the size of the systems, which makes mathematical challenges. In addition, due to the non-commutative nature of quaternion multiplication, some data regarding quaternions may be loss in decomposition. As a result, rather than employing the real or complex decomposition methods, this paper explored the GAS criteria for quaternion-valued T-S fuzzy BAM NNs directly.
Remark 3.8. The authors of [41] used WBII to investigate the Mittag-Leffler synchronization criteria of complex-valued memristive NNs by real-valued decomposition method. The authors of [42] used complex-valued WBII to analyze the global μ-stability criteria of complex-valued BAM NNs by direct method. In comparison to [41,42], we extended the WBII into the quaternion domain and its proof has been presented for the first time. Furthermore, the GAS criteria for quaternion-valued T-S fuzzy BAM NNs are established by using new quaternion-valued WBII and direct quaternion method.
This section includes two numerical evaluations to emphasize the applicability of this analysis.
Example 1: Determine the two neuron quaternion-valued BAM NNs as given below.
{˙pr(t)= −d1rpr(t)+2∑s=1a1rsf1s(qs(t−ℓ(t)))+J1r, r=1,2,pr(t)= φ1r(t), t∈[−ℓ,0],˙qs(t)= −d2sqs(t)+2∑r=1a2srf2r(pr(t−ℓ(t)))+J2s, s=1,2,qs(t)= φ2s(t), t∈[−ℓ,0], | (4.1) |
where d11=d12=3, d21=d22=2, a111=1.2+i−1.5j−0.8k, a112=1+1.2i+1.3j−1.5k, a121=1.4−2.7i−2j−1.3k, a122=0.5+0.8i−1.4j−1.7k, a211=1.2+i−1.5j−0.8k, a212=1+1.2i+1.3j−1.5k, a221=1.4−2.7i−2j−1.3k, a222=0.5+0.8i−1.4j−1.7k, J11=0.2+0.1i−0.2j−0.1k, J12=0.1+0.2i−0.1j−0.1k, J21=0.2+0.2i−0.1j−0.2k, J22=0.3+0.1i−0.1j−0.2k.
The activation functions are regarded as f1s(qs(⋅))=0.5 tanh(qs(⋅))+0.5 tanh(qs(⋅))i+0.5 tanh(qs(⋅))j+0.5 tanh(qs(⋅))k, s=1,2, f2r(pr(⋅))=0.5 tanh(pr(⋅))+0.5 tanh(pr(⋅))i+0.5 tanh(pr(⋅))j+0.5 tanh(pr(⋅))k, r=1,2. Then Assumption 1 holds with lf11=lf12=lf21=lf22=0.25. The delay ℓ(t) is regarded as ℓ(t)=0.4+0.3 sin(t) implying that the maximum permissible upper bound is ℓ=0.7. It is observable that 0≤˙ℓ(t)≤μ=0≤0.3 cos(t)≤0.3. By employing MATLAB YALMIP toolbox, the LMI conditions (3.1) and (3.2) in Theorem (3.1) are verified, and the feasibility are
P1= [0.6718−0.0034−0.0270i+0.0052j−0.0277k−0.0034+0.0270i−0.0052+0.0277k0.6384],P2= [0.49720.0066+0.0196i+0.0040j+0.0226k0.0066−0.0196i−0.0040j−0.0226k0.5029],G1= [8.6913008.6976], G2= [8.7196008.6904]. |
Based on example 1, we conclude that all the conditions associated with Theorem (3.1) are fulfilled; this means that the NN model (2.2) has a unique equilibrium point.
Example 2: Determine the two neuron quaternion-valued T-S fuzzy BAM NNs with h=1,2 as given below:
{˙ur(t)= 2∑h=1ψh(ϑ(t)){−d1hrur(t)+2∑s=1a1hrsg1s(vs(t−ℓ(t)))},ur(t)= ϕ1r(t), t∈[−ℓ,0], r,s=1,2,˙vs(t)= 2∑h=1ψh(ϑ(t)){−d2hsvs(t)+2∑r=1a2hsrg2r(ur(t−ℓ(t)))},vs(t)= ϕ2s(t), t∈[−ℓ,0], r,s=1,2. | (4.2) |
Plant Rule 1: IF {ϑ1(t) is η11}, THEN
{˙ur(t)= −d11rur(t)+2∑s=1a11rsg1s(vs(t−ℓ(t))),ur(t)= ϕ1r(t), t∈[−ℓ,0], r,s=1,2,˙vs(t)= −d21svs(t)+2∑r=1a21srg2r(ur(t−ℓ(t))),vs(t)= ϕ2s(t), t∈[−ℓ,0], r,s=1,2. |
Plant Rule 2: IF {ϑ2(t) is η22}, THEN
{˙ur(t)= −d12rur(t)+2∑s=1a12rsg1s(vs(t−ℓ(t))),ur(t)= ϕ1r(t), t∈[−ℓ,0], r,s=1,2,˙vs(t)= −d22svs(t)+2∑r=1a22srg2r(ur(t−ℓ(t))),vs(t)= ϕ2s(t), t∈[−ℓ,0], r,s=1,2, |
where d111=d112=7, d211=d212=9, d121=d122=8, d221=d222=9, a1111=1.2+i−1.5j−0.8k, a1112=1+1.2i+1.3j−1.5k, a1121=1.4−2.7i−2j−1.3k, a1122=0.5+0.8i−1.4j−1.7k, a2111=1.1−1.4i−1.3j−1.2k, a2112=2.1+1.3i−0.9j−1.1k, a2121=1.3+1.2i−1.2j+1.1k, a2122=−1.5+i+1.2j+1.4k, a1211=1.2+i−1.5j−0.8k, a1212=1+1.2i+1.3j−1.5k, a1221=1.4−2.7i−2j−1.3k, a1222=0.5+0.8i−1.4j−1.7k, a2211=1.1−1.4i−1.3j−1.2k, a2212=2.1+1.3i−0.9j−1.1k, a2221=1.3+1.2i−1.2j+1.1k, a2222=−1.5+i+1.2j+1.4k.
The activation functions are chosen as g1s(vs(⋅))=0.5 tanh(vs(⋅))+0.5 tanh(vs(⋅))i+0.5 tanh(vs(⋅))j+0.5 tanh(vs(⋅))k, s=1,2, g2r(ur(⋅))=0.5 tanh(ur(⋅))+0.5 tanh(ur(⋅))i+0.5 tanh(ur(⋅))j+0.5 tanh(ur(⋅))k, s,r=1,2. Then Assumption 3 hold with lg1s=lg2r=0.25, s,r=1,2. The delay ℓ(t) is regarded as ℓ(t)=0.4+0.3 sin(t), implying that the maximum permissible upper bound is ℓ=0.7. It is observable that 0≤˙ℓ(t)≤μ=0≤0.3 cos(t)≤0.3. Furthermore, the membership functions are considered as ψ1(ϑ(t))=11+e−2t, ψ2(ϑ(t))=1−11+e−2t. The LMI condition (3.23) in Theorem (3.2) are verified by applying MATLAB YALMIP toolbox, and the feasibility are
P1= [0.6965−0.0028−0.0287i−0.0118j−0.0094k−0.0028+0.0287i+0.0118j+0.0094k0.6708],P2= [0.5718−0.0104+0.0184i−0.0024j+0.0245k−0.0104−0.0184i+0.0024j−0.0245k0.5231],Q1= [3.2612−0.0105−0.1072i−0.0439j−0.0351k−0.0105+0.1072i+0.0439j+0.0351k3.1652],Q2= [3.2225−0.0466+0.0824i−0.0108j+0.1098k−0.0466−0.0824i+0.0108j−0.1098k3.0044],R1= [4.0153−0.0160−0.1624i−0.0666j−0.0531k−0.0160+0.1624i+0.0666j+0.0531k3.8695],R2= [3.9566−0.0706+0.1248i−0.0164j+0.1664k−0.0706−0.1248i+0.0164j−0.1664k3.6254],S1= [0.2526−0.0000−0.0002i−0.7085j−0.5655k−0.0000+0.0002i+0.7085j+0.5655k0.2525],S2= [0.2526−0.0001+0.0001i−0.0174j+0.1772k−0.0001−0.0001i+0.0174j−0.1772k0.2522],G1= [3.5058003.4995], G2= [3.5033003.4888]. |
Under randomly selected 10 initial values of ϕ11(t), ϕ12(t), ϕ21(t) and ϕ22(t), the time responses of states of the NNs (4.2) uR1(t), uI1(t), uJ1(t), uK1(t), uR2(t), uI2(t), uJ2(t), uK2(t), vR1(t), vI1(t), vJ1(t), vK1(t), vR2(t), vI2(t), vJ2(t), vK2(t) are illustrated in Figures (1)– (8). According to example 2, we can see that the equilibrium point of the NN model (3.22) is GAS since all the conditions in Theorem (3.2) have been fulfilled, which is in accordance with Theorem (3.2).
This paper investigated the GAS problem for a class of quaternion-valued T-S fuzzy BAM NNs with time-varying delays. By applying T-S fuzzy models, we first considered a general form of quaternion-valued T-S fuzzy BAM NNs with time-varying delays. Then, we applied the Cauchy-Schwarz algorithm and homeomorphism principle to obtain sufficient conditions for the existence and uniqueness of the equilibrium point. By utilizing suitable LKFs and newly developed quaternion-valued WBII, some sufficient criteria are obtained to guarantee the GAS of the considered networks. Further, the results of this paper are presented in terms of quaternion-valued LMIs, which can be solved using the MATLAB YALMIP toolbox. Two numerical examples are presented with their simulations to demonstrate the validity of the theoretical analysis.
The proposed results of this paper can be used to analyze various dynamics of quaternion-valued T-S fuzzy BAM NNs such as finite-time stability, dissipativity, state estimation, synchronization and so on. Thus, we will soon examine the finite-time stability of the following quaternion-valued fractional-order T-S fuzzy BAM NNs with impulses.
{C0Dαtpr(t)=m∑h=1ψh(ϑ(t)){−d1hrpr(t)+m∑s=1a1hrsf1s(qs(t))+m∑s=1b1hrsf1s(qs(t−ℓ(t)))+J1r},t≠tk, r=1,2...,n,△pr(tk)=αk(pr(tk)), t=tk, k=1,2,...,pr(t)=φ1r(t), t∈[−ℓ,0],C0Dαtqs(t)=m∑h=1ψh(ϑ(t)){−d2hsqs(t)+n∑r=1a2hsrf2r(pr(t))+n∑r=1b2hsrf2r(pr(t−ℓ(t)))+J2s},t≠tk, s=1,2,...,m,△qs(tk)=βk(qs(tk)), t=tk, k=1,2,...,qs(t)=φ2s(t), t∈[−ℓ,0]. |
The authors would like to express her gratitude to King Khalid University, Saudi Arabia for providing administrative and technical support. Also, the authors would like to thank the referee for their comments and suggestions on the manuscript.
Funding Statement: This research is financially supported by the Deanship of Scientific Research at King Khalid University under research grant number (RGP.2/202/43).
The authors declare no conflict of interest.
[1] |
J. J. Hopfield, Neural networks and physical systems with emergent collective computational abilities, Proc. Natl. Acad. Sci. U.S.A, 79 (1982), 2554–2558. https://doi.org/10.1073/pnas.79.8.2554 doi: 10.1073/pnas.79.8.2554
![]() |
[2] |
M. A. Cohen, S. Grossberg, Absolute stability of global pattern formation and parallel memory storage by competitive neural networks, IEEE Trans. Syst. Man Cybern., 13 (1983), 815–826. https://doi.org/10.1109/TSMC.1983.6313075 doi: 10.1109/TSMC.1983.6313075
![]() |
[3] |
L. Chua, L. Yang, Cellular neural networks: Applications, IEEE Trans. Circuits Syst., 35 (1988), 1273-1290. https://doi.org/10.1109/31.7601 doi: 10.1109/31.7601
![]() |
[4] |
Q. Song, J. Cao, Stability analysis of impulsive Cohen-Grossberg neural network with unbounded discrete time-varying delays, Int. J. Neural Syst., 17 (2007), 407–417. https://doi.org/10.1142/S012906570700124X doi: 10.1142/S012906570700124X
![]() |
[5] |
B. Kosko, Bidirectional associative memories, IEEE Trans. Syst. Man Cybern., 18 (1988), 49–60. https://doi.org/10.1109/21.87054 doi: 10.1109/21.87054
![]() |
[6] |
K. Gopalsamy, X. Z. He, Delay-independent stability in bi-directional associative memory networks, IEEE Trans. Neural Netw., 5 (1994), 998–1002. https://doi.org/10.1109/72.329700 doi: 10.1109/72.329700
![]() |
[7] |
Q. Song, J. Cao, Global exponential stability and existence of periodic solutions in BAM networks with delays and reaction-diffusion terms, Chaos Soliton. Fract., 23 (2005), 421–430. https://doi.org/10.1016/j.chaos.2004.04.011 doi: 10.1016/j.chaos.2004.04.011
![]() |
[8] |
J. H. Park, C. H. Park, O. M. Kwon, S. M. Lee, A new stability criterion for bidirectional associative memory neural networks of neutral-type, Appl. Math. Comput., 199 (2008), 716–722. https://doi.org/10.1016/j.amc.2007.10.032 doi: 10.1016/j.amc.2007.10.032
![]() |
[9] |
J. H. Park, O. M. Kwon, Delay-dependent stabilility criterion for bidirectional associative memory neural networks with interval time-varying delays, Modern Phys. Lett. B, 23 (2009), 35–46. https://doi.org/10.1142/S0217984909017807 doi: 10.1142/S0217984909017807
![]() |
[10] |
Q. Zhu, C. Huang, X. Yang, Exponential stability for stochastic jumping BAM neural networks with time-varying and distributed delays, Nonlinear Anal. Hybrid Syst., 5 (2011), 52–57. https://doi.org/10.1016/j.nahs.2010.08.005 doi: 10.1016/j.nahs.2010.08.005
![]() |
[11] |
J. Li, W. Zhou, Z. Yang, State estimation and input-to-state stability of impulsive stochastic BAM neural networks with mixed delays, Neurocomputing, 227 (2017), 37–45. https://doi.org/10.1016/j.neucom.2016.08.101 doi: 10.1016/j.neucom.2016.08.101
![]() |
[12] |
Y. Zhao, J. Kurths, L. Duan, Input-to-state stability analysis for memristive BAM neural networks with variable time delays, Phys. Lett. A, 383 (2019), 1143–1150. https://doi.org/10.1016/j.physleta.2019.01.015 doi: 10.1016/j.physleta.2019.01.015
![]() |
[13] |
Z. Wang, L. Huang, Global stability analysis for delayed complex-valued BAM neural networks, Neurocomputing, 173 (2016), 2083–2089. https://doi.org/10.1016/j.neucom.2015.09.086 doi: 10.1016/j.neucom.2015.09.086
![]() |
[14] |
R. Guo, Z. Zhang, X. Liu, C. Lin, Existence, uniqueness, and exponential stability analysis for complex-valued memristor-based BAM neural networks with time delays, Appl. Math. Comput., 311 (2017), 100–117. https://doi.org/10.1016/j.amc.2017.05.021 doi: 10.1016/j.amc.2017.05.021
![]() |
[15] |
J. Liu, J. Jian, Global dissipativity of a class of quaternion-valued BAM neural networks with time delay, Neurocomputing, 349 (2019), 123–132. https://doi.org/10.1016/j.neucom.2019.03.026 doi: 10.1016/j.neucom.2019.03.026
![]() |
[16] |
R. Zhao, B. Wang, J. Jian, Lagrange stability of BAM quaternion-valued inertial neural networks via auxiliary function-based integral inequalities, Neural Process. Lett., 54 (2022), 1351–1369. https://doi.org/10.1007/s11063-021-10685-6 doi: 10.1007/s11063-021-10685-6
![]() |
[17] |
Z. Zhang, Z. Quan, Global exponential stability via inequality technique for inertial BAM neural networks with time delays, Neurocomputing, 151 (2015), 1316–1326. https://doi.org/10.1016/j.neucom.2014.10.072 doi: 10.1016/j.neucom.2014.10.072
![]() |
[18] |
J. Zhang, S. Zhu, N. Lu, S. Wen, Multistability of state-dependent switching neural networks with discontinuous nonmonotonic piecewise linear activation functions, Neurocomputing, 437 (2021), 300–311. https://doi.org/10.1016/j.neucom.2021.01.046 doi: 10.1016/j.neucom.2021.01.046
![]() |
[19] |
S. Zhu, Y. Shen, Robustness analysis of global exponential stability of neural networks with Markovian switching in the presence of time-varying delays or noises, Neural Comput. Appl., 23 (2013), 1563–1571. https://doi.org/10.1007/s00521-012-1105-0 doi: 10.1007/s00521-012-1105-0
![]() |
[20] |
R. Sriraman, R. Samidurai, Global asymptotic stability analysis for neutral-type complex-valued neural networks with random time-varying delays, Int. J. Syst. Sci., 50 (2019), 1742–1756. https://doi.org/10.1080/00207721.2019.1623340 doi: 10.1080/00207721.2019.1623340
![]() |
[21] |
R. Vijay Aravind, P. Balasubramaniam, Stochastic stability of fractional-order Markovian jumping complex-valued neural networks with time-varying delays, Neurocomputing, 439 (2021), 122–133. https://doi.org/10.1016/j.neucom.2021.01.053 doi: 10.1016/j.neucom.2021.01.053
![]() |
[22] |
R. Sriraman, Y. Cao, R. Samidurai, Global asymptotic stability of stochastic complex-valued neural networks with probabilistic time-varying delays, Math. Comput. Simul., 171 (2020), 103–118. https://doi.org/10.1016/j.matcom.2019.04.001 doi: 10.1016/j.matcom.2019.04.001
![]() |
[23] |
Z. Zhang, T. Zheng, Global asymptotic stability of periodic solutions for delayed complex-valued Cohen-Grossberg neural networks by combining coincidence degree theory with LMI method, Neurocomputing, 289 (2018), 220–230. https://doi.org/10.1016/j.neucom.2018.02.033 doi: 10.1016/j.neucom.2018.02.033
![]() |
[24] |
Y. Liu, Y. Zheng, J. Lu, J. Cao, L. Rutkowski, Constrained quaternion-variable convex optimization: A quaternion-valued recurrent neural network approach, IEEE Trans. Neural Netw. Learn. Syst., 31 (2020), 1022–1035. https://doi.org/10.1109/TNNLS.2019.2916597 doi: 10.1109/TNNLS.2019.2916597
![]() |
[25] |
A. Sudbery, Quaternionic analysis, Math. Proc. Camb. Philos. Soc., 85 (1979), 199–225. https://doi.org/10.1017/S0305004100055638 doi: 10.1017/S0305004100055638
![]() |
[26] |
T. Isokawa, T. Kusakabe, N. Matsui, F. Peper, Quaternion neural network and its application, Knowl.-Based Intell. Eng. Syst., 2774 (2003), 318–324. https://doi.org/10.1007/978-3-540-45226-3-44 doi: 10.1007/978-3-540-45226-3-44
![]() |
[27] |
T. Parcollet, M. Morchid, G. Linares, A survey of quaternion neural networks, Artif. Intell. Rev., 53 (2020), 2957–2982. https://doi.org/10.1007/s10462-019-09752-1 doi: 10.1007/s10462-019-09752-1
![]() |
[28] |
R. Sriraman, G. Rajchakit, C. P. Lim, P. Chanthorn, R. Samidurai, Discrete-time stochastic quaternion-valued neural networks with time delays: An asymptotic stability analysis, Symmetry, 12 (2020), 936. https://doi.org/10.3390/sym12060936 doi: 10.3390/sym12060936
![]() |
[29] |
X. You, Q. Song, J. Liang, Y. Liu, F. E. Alsaadi, Global μ-stability of quaternion-valued neural networks with mixed time-varying delays, Neurocomputing, 290 (2018), 12–25. https://doi.org/10.1016/j.neucom.2018.02.030 doi: 10.1016/j.neucom.2018.02.030
![]() |
[30] |
Q. Song, L. Long, Z. Zhao, Y. Liu, F. E. Alsaadi, Stability criteria of quaternion-valued neutral-type delayed neural networks, Neurocomputing, 412 (2020), 287–294. https://doi.org/10.1016/j.neucom.2020.06.086 doi: 10.1016/j.neucom.2020.06.086
![]() |
[31] |
X. You, S. Dian, R. Guo, S. Li, Exponential stability analysis for discrete-time quaternion-valued neural networks with leakage delay and discrete time-varying delays, Neurocomputing, 430 (2021), 71–81. https://doi.org/10.1016/j.neucom.2020.12.021 doi: 10.1016/j.neucom.2020.12.021
![]() |
[32] |
T. Takagi, M. Sugeno, Fuzzy identification of systems and its applications to modeling and control, IEEE T. Syst. Man CY-S., 15 (1985), 116–132. https://doi.org/10.1109/TSMC.1985.6313399 doi: 10.1109/TSMC.1985.6313399
![]() |
[33] |
L. Wang, H. K. Lam, New stability criterion for continuous-time Takagi-Sugeno fuzzy systems with time-varying delay, IEEE T. Cybernetics., 49 (2019), 1551–1556. https://doi.org/10.1109/TCYB.2018.2801795 doi: 10.1109/TCYB.2018.2801795
![]() |
[34] |
Y. Y. Hou, T. L. Liao, J. J. Yan, Stability analysis of Takagi-Sugeno fuzzy cellular neural networks with time-varying delays, IEEE T. Syst. Man CY-S., 37 (2007), 720–726. https://doi.org/10.1109/TSMCB.2006.889628 doi: 10.1109/TSMCB.2006.889628
![]() |
[35] |
C. K. Ahn, Delay-dependent state estimation for T-S fuzzy delayed Hopfield neural networks, Nonlinear Dyn., 61 (2010), 483–489. https://doi.org/10.1007/s11071-010-9664-z doi: 10.1007/s11071-010-9664-z
![]() |
[36] |
J. Jian, P. Wan, Global exponential convergence of fuzzy complex-valued neural networks with time-varying delays and impulsive effects, Fuzzy Sets Syst., 338 (2018), 23–39. https://doi.org/10.1016/j.fss.2017.12.001 doi: 10.1016/j.fss.2017.12.001
![]() |
[37] |
Z. Zhang, J. Cao, Finite-time synchronization for fuzzy inertial neural networks by maximum value approach, IEEE Trans. Fuzzy Syst., 30 (2022), 1436–1446. https://doi.org/10.1109/TFUZZ.2021.3059953 doi: 10.1109/TFUZZ.2021.3059953
![]() |
[38] |
S. Zhu, Y. Shen, Robustness analysis for connection weight matrices of global exponential stable time varying delayed recurrent neural networks, Neurocomputing, 113 (2013), 220–226. https://doi.org/10.1016/j.neucom.2013.01.006 doi: 10.1016/j.neucom.2013.01.006
![]() |
[39] |
G. Rajchakit, P. Chanthorn, P. Kaewmesri, R. Sriraman, C. P. Lim, Global Mittag-Leffler stability and stabilization analysis of fractional-order quaternion-valued memristive neural networks, Mathematics, 8 (2020), 422. https://doi.org/10.3390/math8030422 doi: 10.3390/math8030422
![]() |
[40] |
U. Humphries, G. Rajchakit, P. Kaewmesri, P. Chanthorn, R. Sriraman, R. Samidurai, C. P. Lim, Global stability analysis of fractional-order quaternion-valued bidirectional associative memory neural networks, Mathematics, 8 (2020), 801. https://doi.org/10.3390/math8050801 doi: 10.3390/math8050801
![]() |
[41] |
J. Wang, H. Jiang, T. Ma, C. Hu, Delay-dependent dynamical analysis of complex-valued memritive neural networks: Continuous-time and discrete-time cases, Neural Netw., 101 (2018), 33–46. https://doi.org/10.1016/j.neunet.2018.01.015 doi: 10.1016/j.neunet.2018.01.015
![]() |
[42] |
C. A. Popa, Global μ-stability of neutral-type impulsive complex-valued BAM neural networks with leakage delay and unbounded time-varying delays, Neurocomputing, 376 (2020), 73–94. https://doi.org/10.1016/j.neucom.2019.09.008 doi: 10.1016/j.neucom.2019.09.008
![]() |
[43] |
P. G. Park, J. W. Ko, C. Jeong, Reciprocally convex approach to stability of systems with time-varying delays, Automatica, 47 (2011), 235–238. https://doi.org/10.1016/j.automatica.2010.10.014 doi: 10.1016/j.automatica.2010.10.014
![]() |
[44] |
A. Seuret, F. Gouaisbaut, Wirtinger-based integral inequality: Application to time-delay systems, Automatica, 49 (2013), 2860–2866. https://doi.org/10.1016/j.automatica.2013.05.030 doi: 10.1016/j.automatica.2013.05.030
![]() |
[45] |
Z. Zhang, J. Cao, Novel finite-time synchronization criteria for inertial neural networks with time delays via integral inequality method, IEEE Trans. Neural Netw. Learn. Syst., 30 (2019), 1476–1485. https://doi.org/10.1109/TNNLS.2018.2868800 doi: 10.1109/TNNLS.2018.2868800
![]() |
[46] |
Z. Zhang, L. Ren, New sufficient conditions on global asymptotic synchronization of inertial delayed neural networks by using integrating inequality techniques, Nonlinear Dyn., 95 (2018), 905–917. https://doi.org/10.1007/s11071-018-4603-5 doi: 10.1007/s11071-018-4603-5
![]() |
[47] |
X. Chen, Z. Li, Q. Song, J. Hu, Y. Tan, Robust stability analysis of quaternion-valued neural networks with time delays and parameter uncertainties, Neural Netw., 91 (2017), 55–65. https://doi.org/10.1016/j.neunet.2017.04.006 doi: 10.1016/j.neunet.2017.04.006
![]() |
[48] |
F. Zhang, Quaternions and matrices of quaternions, Linear Algebra Appl., 251 (1997), 21–57. https://doi.org/10.1016/0024-3795(95)00543-9 doi: 10.1016/0024-3795(95)00543-9
![]() |
[49] |
X. Chen, Q. Song, State estimation for quaternion-valued neural networks with multiple time delays, IEEE T. Syst. Man Cy-S., 49 (2019), 2201–2209. https://doi.org/10.1109/TSMC.2017.2776940 doi: 10.1109/TSMC.2017.2776940
![]() |
[50] |
Z. Tu, Y. Zhao, N. Ding, Y. Feng, W. Zhang, Stability analysis of quaternion-valued neural networks with both discrete and distributed delays, Appl. Math. Comput., 343 (2019), 342–353. https://doi.org/10.1016/j.amc.2018.09.049 doi: 10.1016/j.amc.2018.09.049
![]() |
1. | R. Sriraman, R. Samidurai, V. C. Amritha, G. Rachakit, Prasanalakshmi Balaji, System decomposition-based stability criteria for Takagi-Sugeno fuzzy uncertain stochastic delayed neural networks in quaternion field, 2023, 8, 2473-6988, 11589, 10.3934/math.2023587 | |
2. | Zhiwei Zhang, Xue Liang, Jinbao Lan, Xian Zhang, Global exponential stability of quaternion bidirectional associative memory neural networks with multiple delays, 2024, 47, 0170-4214, 7165, 10.1002/mma.9962 | |
3. | R. Sriraman, Prasanalakshmi Balaji, R. Veerasivaji, System Decomposition Method-Based Exponential Stability of Clifford-Valued BAM Delayed Neural Networks, 2023, 11, 2169-3536, 77324, 10.1109/ACCESS.2023.3295420 | |
4. | Weinan Li, Maoxin Liao, Dongsheng Li, Changjin Xu, Bingbing Li, Dynamic Behavior of a Class of Six-Neuron Fractional BAM Neural Networks, 2023, 7, 2504-3110, 520, 10.3390/fractalfract7070520 | |
5. | Moufid Bouhentala, Mouna Ghanai, Kheireddine Chafaa, Interval-valued fuzzy estimation and its application to adaptive control of quadrotor, 2023, 13, 26667207, 100337, 10.1016/j.rico.2023.100337 | |
6. | Omar Kahouli, Amina Turki, Mohamed Ksantini, Mohamed Ali Hammami, Ali Aloui, On the boundedness of solutions of some fuzzy dynamical control systems, 2024, 9, 2473-6988, 5330, 10.3934/math.2024257 | |
7. | Jinbao Lan, Xian Zhang, Xin Wang, Global robust exponential stability of interval BAM neural networks with multiple time-varying delays: A direct method based on system solutions, 2024, 144, 00190578, 145, 10.1016/j.isatra.2023.11.001 | |
8. | Ningning Zhao, Yuanhua Qiao, Jun Miao, Lijuan Duan, New predefined-time stability results of impulsive systems with time-varying impulse strength and its application to synchronization of delayed BAM neural networks, 2024, 129, 10075704, 107724, 10.1016/j.cnsns.2023.107724 |