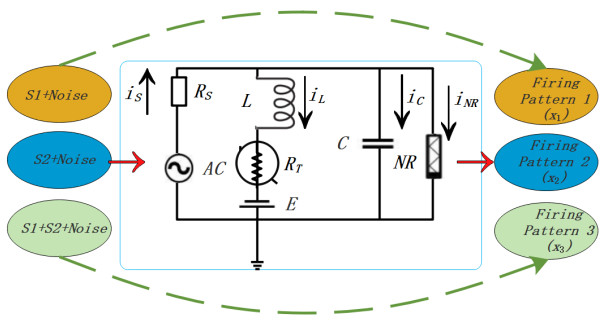
Patients with congenital analgesia who lack the ability to sense temperature generally face low survival rates, highlighting a critical need to understand the underlying mechanisms of heat sensation. While previous research has focused on modeling neural responses to stimuli, the specific mechanisms by which heat-sensitive neurons respond to external temperature changes remain unclear. This gap in knowledge is particularly relevant, as identifying how these neurons react to diverse stimuli can provide insight into sensory deficits linked to congenital analgesia. In this study, we developed a model of heat-sensitive neurons based on the FitzHugh-Nagumo (FHN) neural circuit to investigate neuronal response patterns to external heat stimuli. Two distinct stimulus patterns, each combined with Gaussian white noise, were applied to the model to induce varied firing modes. By calculating the Hamilton energy for each firing mode, we quantified the impact of each external stimulus on neuronal activity. A correlation function was further defined to explore how different stimuli influence the selection of firing modes. Simulation results demonstrate that heat-sensitive neurons show a preferential response to stimuli that induce spike discharge over stimuli that induce r-clonic patterns, as seen in changes to the periodic attractor contours. When exposed to Chua's circuit stimulus, chaotic emission patterns reveal significant shifts in attractor contour, indicating a strong response to spike, r-clonic, and periodic stimuli. These findings suggest that external stimuli capable of inducing spike-and-wave or r-clonic patterns are sensitively detected by thermosensitive neurons, leading to heightened Hamilton energy release and increased regularity in neural activity. This study enhances our understanding of thermosensitive neuronal dynamics under complex stimuli, shedding light on potential response mechanisms relevant to sensory dysfunction in congenital analgesia and advancing the broader field of neural response modeling.
Citation: Yunhai Wang, Guodong Huang, Rui Zhu, Shu Zhou, Yuan Chai. Response mechanism of heat-sensitive neurons under combined noise stimulation[J]. Electronic Research Archive, 2024, 32(11): 6405-6423. doi: 10.3934/era.2024298
[1] | Hao Yang, Peihan Wang, Fang Han, Qingyun Wang . An interpretable mechanism for grating-induced cross-inhibition and gamma oscillation based on a visual cortical neuronal network model. Electronic Research Archive, 2024, 32(4): 2936-2954. doi: 10.3934/era.2024134 |
[2] | Feibiao Zhan, Jian Song . Complex rhythm and synchronization of half-center oscillators under electromagnetic induction. Electronic Research Archive, 2024, 32(7): 4454-4471. doi: 10.3934/era.2024201 |
[3] | Feibiao Zhan, Jian Song, Shenquan Liu . The influence of synaptic strength and noise on the robustness of central pattern generator. Electronic Research Archive, 2024, 32(1): 686-706. doi: 10.3934/era.2024033 |
[4] | Zilu Cao, Lin Du, Honghui Zhang, Lianghui Qu, Luyao Yan, Zichen Deng . Firing activities and magnetic stimulation effects in a Cortico-basal ganglia-thalamus neural network. Electronic Research Archive, 2022, 30(6): 2054-2074. doi: 10.3934/era.2022104 |
[5] | Li Li, Zhiguo Zhao . Inhibitory autapse with time delay induces mixed-mode oscillations related to unstable dynamical behaviors near subcritical Hopf bifurcation. Electronic Research Archive, 2022, 30(5): 1898-1917. doi: 10.3934/era.2022096 |
[6] | Qixiang Wen, Shenquan Liu, Bo Lu . Firing patterns and bifurcation analysis of neurons under electromagnetic induction. Electronic Research Archive, 2021, 29(5): 3205-3226. doi: 10.3934/era.2021034 |
[7] | Xiaofang Jiang, Hui Zhou, Feifei Wang, Bingxin Zheng, Bo Lu . Bifurcation analysis on the reduced dopamine neuronal model. Electronic Research Archive, 2024, 32(7): 4237-4254. doi: 10.3934/era.2024191 |
[8] | Hui Zhou, Bo Lu, Huaguang Gu, Xianjun Wang, Yifan Liu . Complex nonlinear dynamics of bursting of thalamic neurons related to Parkinson's disease. Electronic Research Archive, 2024, 32(1): 109-133. doi: 10.3934/era.2024006 |
[9] | Honghui Zhang, Yuzhi Zhao, Zhuan Shen, Fangyue Chen, Zilu Cao, Wenxuan Shan . Control analysis of optogenetics and deep brain stimulation targeting basal ganglia for Parkinson's disease. Electronic Research Archive, 2022, 30(6): 2263-2282. doi: 10.3934/era.2022115 |
[10] | Xiaojing Zhu, Yufan Liu, Suyuan Huang, Ranran Li, Yuan Chai . Modulation of epileptiform discharges by heterogeneous interneurons and external stimulation strategies in the thalamocortical model. Electronic Research Archive, 2025, 33(4): 2391-2411. doi: 10.3934/era.2025106 |
Patients with congenital analgesia who lack the ability to sense temperature generally face low survival rates, highlighting a critical need to understand the underlying mechanisms of heat sensation. While previous research has focused on modeling neural responses to stimuli, the specific mechanisms by which heat-sensitive neurons respond to external temperature changes remain unclear. This gap in knowledge is particularly relevant, as identifying how these neurons react to diverse stimuli can provide insight into sensory deficits linked to congenital analgesia. In this study, we developed a model of heat-sensitive neurons based on the FitzHugh-Nagumo (FHN) neural circuit to investigate neuronal response patterns to external heat stimuli. Two distinct stimulus patterns, each combined with Gaussian white noise, were applied to the model to induce varied firing modes. By calculating the Hamilton energy for each firing mode, we quantified the impact of each external stimulus on neuronal activity. A correlation function was further defined to explore how different stimuli influence the selection of firing modes. Simulation results demonstrate that heat-sensitive neurons show a preferential response to stimuli that induce spike discharge over stimuli that induce r-clonic patterns, as seen in changes to the periodic attractor contours. When exposed to Chua's circuit stimulus, chaotic emission patterns reveal significant shifts in attractor contour, indicating a strong response to spike, r-clonic, and periodic stimuli. These findings suggest that external stimuli capable of inducing spike-and-wave or r-clonic patterns are sensitively detected by thermosensitive neurons, leading to heightened Hamilton energy release and increased regularity in neural activity. This study enhances our understanding of thermosensitive neuronal dynamics under complex stimuli, shedding light on potential response mechanisms relevant to sensory dysfunction in congenital analgesia and advancing the broader field of neural response modeling.
The human brain is the most advanced and complex organ known in the world. Highly advanced and organized, the human brain is undoubtedly an excellent computer and signal processor composed of neurons with different functions. Different regions correspond to different specific functions, and neurons primarily contribute to these functions [1,2,3,4]. For example, auditory neurons are responsible for transmitting sound signals to the brain for processing and interpretation, and the auditory nerve is very sensitive to the frequency, intensity, and direction of sound, allowing us to perceive the source and distance of sound [5,6,7]. Olfactory neurons are responsible for transmitting odor information and can distinguish different odors by comparing and analyzing different olfactory signals [8,9,10]. Visual neurons can distinguish different shapes and sizes of objects under certain circumstances [11,12,13]. Skin and temperature-sensitive neurons can effectively sense changes in temperature [14,15,16]. These functional neurons not only operate independently but also interact with one another to form complex neural networks. This interconnectedness allows for enhanced processing capabilities and the emergence of intricate behaviors, which are essential for understanding the dynamics of neural circuits. The study of neural networks, constructed from these functional neurons, reveals how individual neuron properties contribute to the overall dynamics of the system. Understanding the interactions among these neurons is critical for elucidating the mechanisms underlying their collective behavior. Yamakou et al. [17] designed scale-free FHN neural networks through mixed coupled slow recovery variables and studied the stochastic resonance phenomenon of the networks. Murza et al. [18] analyzed the dynamic oscillator of an electrically coupled modified FHN network. Yu et al. [19] analyzed the effects of bounded noise and delay on subthreshold signal transmission in FHN neural networks. Jiang et al. [20] reported the chimera state of non-local attractively repulsive coupling in FHN neural networks. In bipartite neural networks of the FHN model, in which nodes interact with one another only when they are in separate layers, Wu et al. [21] examined chimera states. The bipartite networks discovered rich chimera behaviors and multistability. In the study by Yang et al. [22], the basic FHN neural circuit was enhanced by simultaneously integrating a thermistor and a phototube into distinct branches of the circuit. This modification renders the neuron sensitive to both light and temperature. The references mentioned above can provide some direction for this study of the FHN neuron model. In order to understand in detail the propagation and interaction of electrical signals within and between neurons, it is necessary to simplify the model setup of individual neurons.
In this paper, a highly simplified dynamic model with appropriate parameters can be used to describe the main characteristics of neural firing behavior by applying appropriate external stimuli and adding a Gaussian white noise. To simulate the FHN neuron model, we have taken into account a basic circuit that is powered by an external voltage source or a photocell that has been activated. For a biological neuron, its internal field energy is represented by changes in action potential and membrane potential. The main biophysical properties of biological neurons can be reproduced by functional biophysical neural circuits, and their internal energy can be described by equivalent Hamilton energy function [23,24,25]. The Hamilton energy balance based on Helmholtz's theorem by Leutcho in [26] explains the dependence of the initial conditions on the macrostability mechanism and shows how the isosurface with constant Hamilton energy is strongly dependent on the state variable. In the presence of noise interference [27,28], most neurons can be tamed in the form of external periodic currents to form regular discharge patterns and high neural activity regularity [29]. In the absence of periodic stimulation, a certain intensity of noise can also effectively induce nonlinear resonance [30,31,32]. In addition, similar coherent resonances and regular spatial patterns can be formed when noise is applied to a network of neurons [33,34]. However, little research has been designed to investigate the effect of AWGN white Gaussian noise added to the original stimuli on different firing patterns of heat-sensitive neurons. A neural circuit using a thermistor and phototube adjusts neuron excitability in response to temperature and light, effectively simulating various firing patterns. Through bifurcation analysis, this model offers insights into neuron dynamics and potential sensor applications [35,36].
When a single stimulus from a single channel acts on a neuron, most neurons can be activated, showing different firing patterns. When neurons are stimulated by multiple channels at the same time, their mode selection becomes complex and variable. For example, when two different external stimuli are applied to an isolated neuron, which external stimulus will predominantly determine the firing pattern? The isolated neuron will respond preferentially to which external stimulus? Discovering and studying this mechanism is interesting. Taking it a step further, it is even more meaningful to discover the underlying biophysical mechanisms of information encoding and processing in these biophysical neurons. For example, by studying the response kinetics and excitability of Moris-Lecar neurons to two kinds of temperature-sensitive ion channels, calcium ion channel and leakage current, the co-dimensional bifurcation and frequency response curves under different temperatures show that the excitability of neurons varies from grade Ⅱ to grade Ⅰ with the increase of temperature under temperature-sensitive conditions [37].
The first motivation for this research was to build a heat-sensitive neuron model based on the simple FHN neural circuit. Our model is designed to simulate the behavior of heat-sensitive neurons by incorporating both chaotic stimuli and noise, allowing for the analysis of adaptive neuronal responses. The advantage of this model lies in its ability to reproduce complex firing behaviors in response to multiple stimuli, particularly the ability to switch between different firing patterns based on external inputs. The self-adaptive property is reflected in the neuron's ability to filter and select specific stimuli frequencies, a process akin to synaptic plasticity and wave filtering. We will elaborate further on this mechanism, supported by recent studies such as Ma et al. and Xu et al., which discuss that any activation of biophysical function and self-adaption of biological neurons may be dependent on energy flow, and synapse connection is controlled to reach energy balance between neurons [38,39]. The second motivation for this research was to investigate the effect of AWGN white Gaussian noise added to the original stimuli on different firing patterns of heat-sensitive neurons. Gammaitoni et al. supported this claim by explaining that it manifests in nonlinear systems, where generally weak input signals can be amplified and optimized with the assistance of noise [40]. In this paper, we attempted to explore the potential response mechanism of heat-sensitive neurons when two different external stimuli, both supplemented with Gaussian white noise, stimulate the FHN neural circuit. From a physical perspective, any type of external stimulus to a nonlinear circuit is simply the injection of a certain amount of energy, some of which is absorbed and propagated to more electronic components. The interference of multi-channel synchronous input will cause some conflicts in the nonlinear response, and the nonlinear system will show different emission modes under the guidance of energy flow. By calculating the field energy in the neural circuit and equivalent Hamilton energy in functional neurons [41], it is confirmed that neurons tend to respond to any external stimulus only when stimulated, showing higher Hamilton energy and certain regularity. That is to say, neurons are more responsive to external stimuli with higher energy, and neural activity can be regularly induced by particular stimuli, so as to effectively capture the energy injected by the outside world. However, neurons and neural circuits must meet particular energy absorption standards, which can greatly enhance nonlinear resonance, and obtain higher Hamilton energy through appropriate discharge modes; also, they must remain silent to some external stimuli of lower energy and maintain robustness to some incentives that destroy regularity. In order to clearly explain the principle of this phenomenon, we employed two distinct external stimulus types, which added Gaussian white noise to identify the priority of pattern selection in neural activity, and calculated the Hamilton energy under different discharge modes.
The key contributions of this research are two-fold: first, the final emission mode is mainly controlled by a stimulus that activates a higher Hamilton energy within the neuron. Second, the addition of periodic excitation modes helps to excite nonlinear resonances, and new periodic excitation modes can be generated even at lower Hamilton energies. The structure of this article is as follows: a detailed description of the model and stimulus is given in Section 2, the process of numerical simulation is introduced in Section 3, and conclusions are drawn in Section 4.
By adding thermistors to different branches of a simple FHN neural circuit, a temperature-thermistor neural circuit is constructed, with its schematic diagram shown in Figure 1. The modified neural circuit consists of a nonlinear resistor NR, an induction coil L, a thermistor RT, an external constant voltage source E, a capacitor C, a photocell, and a linear resistor RS. When signal S1 (W1) and white Gaussian noise stimulate neurons, firing mode 1 (x1) is triggered; firing mode 2 (x2) is triggered when the neurons are stimulated with signal S2 (W2) and white Gaussian noise; and firing mode 3 (x3) is generated by stimulating the piezoelectric neurons with signal S1 and signal S2 (W1 + W2) and Gaussian white noise.
In accordance with Kirchhoff's current law and Kirchhoff's voltage law, the circuit equation of the neural circuit drawn in Figure 1 is composed of
{CdVdt=iS−iL−iNRLdiLdt=V+E−RTiL, | (1) |
where V represents the voltage of the capacitor C and is the current through the inductor L. The current through the nonlinear resistance iNR can be estimated as
iNR=−1ρ(V−13V3V20), | (2) |
when the linear resistor RS in series with the photocell is selected with high resistance, the phototube behaves as a voltage source, and its current can be estimated by
iS=Vp−VRS, | (3) |
where Vp represents the output voltages of the photocell; the resistance of a negative temperature coefficient thermistor can be determined by
RT=R∞eBT,B=qK. | (4) |
In the above equation, q is the activation energy, T is the temperature, K is the Boltzmann constant, and R∞ is the maximum resistance at T→∞. The physical parameters and variables are updated to the following dimensionless form
{x=VV0,y=ρiLV0,τ=tρC,a=EV0,b=RTρ=b(T′),c=ρ2CL,ξ=ρRS,uS=ξVPRS. | (5) |
The dynamics of thermal neurons powered by photocurrent are calculated by changing the circuit equation to an analogous form
{dxdτ=x(1−ξ)−13x2−y+uSdydτ=c[x+a−b(T′)y], | (6) |
where the variables x and y represent the film potential and recovery variables, respectively, at slow currents. The firing pattern of biophysical neurons can be altered by parameters a, b(T′), c, ξ and external physical stimuli that we select in the form of cycles
uS=Bcos(wτ);, | (7) |
In the above formula, B and w represent amplitude and angular frequency, respectively. The dimensionless Hamilton energy HZ can take the place of the field energy present in an electrical component
{HZ=12CV2+12Li2L=CV20(12x2+12cy2)H=ZCV20=12x2+12cy2. | (8) |
As shown in (8), this neuron's Hamilton energy is determined by the intrinsic parameter c and two variables (x, y). Therefore, any change in the firing patterns and parameters of neurons can have a noticeable effect on the Hamilton energy of isolated neurons. In this paper, we will discuss switching external stimuli to detect mode shifts in electrical activity and the dependence of different modes on Hamilton energy.
Additionally, the chaotic sequence of Chua's circuit (Figure 3) is used as a chaotic sound wave, and the dynamics of Chua's system can be represented by (9), with x', y', and z' standing for the variables representing the voltage of the two capacitors and the induced current on the induction coil. α, β, γ are normalized parameters that describe the capacitance, inductance, and resistance in a circuit.
{˙x′=α(y′−x′)−αf(x′),˙y′=x′−y′+z′,˙z′=−βy′−γz′, | (9) |
f(x′)=m1x′+0.5(m0−m1)(|x′+1|−|x′−1|). | (10) |
Where m0 and m1 are normalized parameters for the conductance in the curve i-v for Chua's diode. The nonlinear function f (x') describes the dimensionless current across the nonlinear resistor (diode) of Chua's circuit. By setting appropriate parameters, Chua's system can present chaotic state and periodic state, and then the first variable x' will be used as a realistic signal source for checking the sensitivity of the neural circuit.
Additional Gaussian white noise is described by formula (11):
y=awgn(x,snr,sigpower). | (11) |
The AWGN white Gaussian noise is added to the vector signal x. The scalar SNR specifies the ratio of signal to noise in dB at each sampling point. If x is complex, AWGN will add complex noise. This syntax assumes that the energy of x is 0 dBW. The initial value of signal power is fixed at 10 dBW and the SNR is 20 dBW [42].
A statistical correlation function, where the symbol < * > denotes the average calculation of the variable over time over a specific transient duration, is constructed in order to identify the influence of various external stimuli on neural mode selection and firing mode. Additionally, the correlation function in (11) is normalized to calculate the pattern selection's dependence on external stimuli [43].
{r1′(x1,x3)=|⟨x1x3⟩−⟨x1⟩⟨x3⟩σ(x1)σ(x3)|;r2′(x2,x3)=|⟨x2x3⟩−⟨x2⟩⟨x3⟩σ(x2)σ(x3)|;σ2(x)=⟨x2⟩−⟨x⟩2; | (12) |
When r1(x1, x3) is greater than r2(x2, x3), it shows that the firing mode will be more similar to the r1 sampling time series, and the firing mode is mainly determined by the external stimulus W1 and noise. Otherwise, when the two external stimuli (W1+W2) and noise are applied simultaneously, the external W2 will completely control the firing mode and the firing mode r2.
{r1(x1,x3)=r1′(x1,x3)r1′(x1,x3)+r2′(x2,x3);r2(x2,x3)=r2′(x2,x3)r1′(x1,x3)+r2′(x2,x3); | (13) |
Calculations are made in the MATLAB environment in our simulation. The classical fourth-order Runge–Kutta method is used to solve, and the integration step is chosen to be 1 ms, the initial values for the two variables are (0.2, 0.1), and the transient period for the calculation is around 4000 time units. The excitability can be managed by external forcing current and, thereby, the firing mode in membrane potential is regulated fully. A discrete mode transition from a quiescent state, simple oscillation, peaking, r-clonic, to a chaotic state can be induced by carefully controlling the amplitude and frequency of the external stimuli. Furthermore, it is possible to choose the right parameters to activate the chaotic attractor in Chua's circuit; the outcomes are displayed in Figure 3 [44,45].
First, with the same amplitude (B1 = B2 = 0.48), we assigned two external stimuli while applying Gaussian white noise of the same intensity. In the S0 stage, the neuronal membrane potential reached a static state within 0–500 time units without external stimulation. In stage S1, the spiking discharge state was triggered by W1 and Gaussian white noise in 500–1500 time units. In THE S2 stage, the W1 and Gaussian white noise stimuli are removed, and the W2 and Gaussian white noise stimuli are used to generate r-clonic discharge patterns in 1500–2500 time units [46]. In stage S3, W1 and W2 combined with white Gaussian noise stimulus simultaneously trigger a hybrid emission mode close to both in 2500–3500 time units. Then, the W1, W2, and Gaussian white noise are removed, the static state is maintained within 3500 ~ 4000 time units, and the correlation function is computed. Figure 4 plots the emission mode change process and the evolution of Hamilton energy at each stage when the external stimulus switches over time. As depicted in Figure 4, when periodic stimulation of W1 and W2 is combined with Gaussian white noise and applied simultaneously to the neurons, the periodic discharge state is suppressed. The resulting discharge mode tends to be a mixed mode of W1 and W2, with a tendency to align more closely to the peak discharge mode. This periodic behavior can be confirmed through the formation of attractors and analysis of the power spectrum. Periodic firing coexists with spiking, and the Hamilton energy further increases compared with that in the S1 stage.
The value of the correlation function r1(x1, x3) is 1.3162, and the value of r2(x2, x3) is –0.3162. The correlation function r1(x1, x3) is greater than r2(x2, x3), indicating that external stimulus W1 and white Gaussian noise play a significant part in regulating the discharge pattern, and the excitation from W2 and white Gaussian noise can be suppressed in neurons. Due to this, neurons tend to respond quickly to external stimuli W1 and Gaussian white noise. It is worthwhile to mention the situation in which two external stimuli can induce a chaotic state and a periodic spiking state, whose outcomes are displayed in Figure 5. The Hamilton energy of neurons varies greatly when they change from the spiking state to the chaotic state, with much higher Hamilton energy in the spiking state. When W1, W2, and Gaussian white noise are applied simultaneously, the neurons show a combination of chaotic state and spiking firing mode and a higher Hamilton energy. This is somewhat different from the result in Figure 4, since the final discharge mode is a combination of chaotic state and spiking discharge mode. The occurrence of periodic spiking discharge mode creates a certain periodic oscillation profile in the final discharge mode; the correlation function r1(x1, x3) is greater than r2(x2, x3), and the outcomes show that the peak discharge mode has a significant impact on the chaotic mode with low Hamilton energy, which leads to the sensitivity of neurons to both kinds of external stimuli. A large number of studies have demonstrated that periodic attractors with multiple orbits are formed under two different types of external stimuli. When the final state of electrical activity is not represented by a different discharge, the electrical response of the neuron is sensitive to both kinds of external stimulus; otherwise, the neuron tends to respond to the external stimulus only when it is effectively stimulated to take on a higher Hamilton energy.
As shown in Figure 6, the simple oscillation pattern of neuronal activity shows a higher Hamilton energy than the chaotic state of neurons driven by a single cycle of stimulation. When the two external stimuli and Gaussian white noise activate neurons at the same time, the final firing mode shows an oscillation state close to the blasting mode, and the Hamilton energy increases greatly. In fact, the introduction of simple oscillatory discharge will enhance the nonlinear oscillation of the pulse train and then make the chaotic mode close to the blasting mode. The value of the correlation function r1(x1, x3) is -0.2291 and the value of r2(x2, x3) is 1.2291. The correlation function r1(x1, x3) is less than r2(x2, x3), indicating that the chaotic state has a great influence on the simple oscillatory discharge mode, resulting in neurons being sensitive to both kinds of external stimuli. It is worth noting that the intensity of the Gaussian white noise remains constant regardless of the difference in the amplitude and frequency of the two external stimuli, which means that the average Hamilton of the various periods and transient periods may differ depending on the two inputs [47].
As depicted in Figure 7, we considered two distinct external stimuli, one that effectively triggers the spike discharge pattern and the other that effectively induces the r-clonic pattern. By altering the external stimulus, the neural activity changed from the spiking mode to the r-clonic mode, and the Hamilton energy increased. The value of the correlation function r1(x1, x3) is 1.3793, and the value of r2(x2, x3) is -0.3793. The correlation function r1(x1, x3) is greater than r2(x2, x3), indicating that neurons are perceptive of external stimuli and can induce the spiking mode rather than the r-clonic mode. Thus, when two periodic stimuli fire neurons by absorbing more energy, the r-clonic mode can be suppressed by the r-clonic mode. Therefore, the peak discharge mode is more effective in controlling the emission mode. This process can be compared with Figure 4. With the change of amplitude, the level of Hamilton energy of the two shows opposite results, indicating that the change of amplitude can affect the level of Hamilton energy to a certain extent and neurons are more inclined to respond to external stimuli with higher amplitude and present higher Hamilton energy when other conditions remain unchanged.
As shown in Figure 8, under the two kinds of periodic stimuli, the S1 stage presents the spiking discharge stage, and the S2 stage presents the blasting mode. Moreover, under the strong amplitude (B2 > B1), the burst sequence can be suppressed by the spiking discharge mode to a certain extent. Because W2 has a higher amplitude and more energy injected into neurons than W1, the Hamilton energy corresponding to W2 is lower than that corresponding to W1. Therefore, the periodic spiking discharge state is accompanied by a higher Hamilton energy. Moreover, the value of the correlation function r1(x1, x3) is 1.5891, and the value of r2(x2, x3) is -0.5891. The correlation function r1(x1, x3) is greater than r2(x2, x3), confirming that neurons are more sensitive to W1.
In a similar way, competition between the r-clonic mode and the chaotic state is considered in the presence of external stimuli and Gaussian white noise, and the outcomes are shown in Figure 9. In the event that both external stimuli are activated, the neurons exhibit r-clonic mode in the S1 stage of electrical activity, which is effectively modulated due to the higher amplitude (B2 > B1) of neurons with chaotic firing mode. The r-clonic pattern of neurons shows a higher Hamilton energy than the chaotic state. The balance between two separate discharge patterns brought on by two different stimuli determines the final discharge pattern. The value of the correlation function r1(x1, x3) is 0.8130, and the value of r2(x2, x3) is 0.1870. The correlation function r1(x1, x3) is greater than r2(x2, x3), which indicates that in the final developed mode, the r-clonic discharge mode contributes more than the chaotic discharge mode due to the higher Hamilton energy provided by the r-clonic mode, and the neurons tend to respond preferentially to the r-clonic mode. The results of Figure 9 and Figure 10 show that neurons are more inclined to respond to stimuli that exhibit continuous regularity rather than chaotic or explosive states, and exhibit higher Hamilton energies, thus forming different periodic firing patterns.
As shown in Figure 10, the study of mode selection is also interesting when external stimuli trigger chaotic and periodic spike discharge patterns. When the external stimulus is forced to change from periodic spiking mode to chaotic mode, the Hamilton energy increases greatly. In addition, when spiking and chaotic stimuli are applied at the same time, the final firing pattern of neurons begins to oscillate irregularly and is accompanied by chaotic patterns. The value of the correlation function r1(x1, x3) is 0.9312, and the value of r2(x2, x3) is 0.0688. The correlation function r1(x1, x3) is greater than r2(x2, x3), indicating that neurons are more inclined to react to the burst mode induced by the spiking discharge mode stimulus than the chaotic discharge mode induced by the chaotic stimulus. A large number of numerical findings confirm the emergence and growth of chaotic attractors. The combination of chaotic activity and peak discharge activity causes Hamilton energy to fall dramatically, as can also be seen from the S3 stage. The Hamilton energy of the S2 stage is much larger than that of the S1 stage, indicating that the energy change caused by Chua's circuit stimulation is larger.
As shown in Figure 11, when periodic and chaotic stimuli are used to trigger both the r-clonic pattern and chaotic neural activity, the resulting discharge pattern is dominated by an oscillatory state accompanying the chaotic pattern. The value of the correlation function r1(x1, x3) is 1.2850, and the value of r2(x2, x3) is -0.2850. The correlation function r1(x1, x3) is greater than r2(x2, x3), indicating that r-clonic mode mostly regulated mode choice, so neurons are more sensitive to periodic stimulation than chaotic stimulation. Numerous numerical studies have also confirmed the emergence of dense orbital chaotic attractors in the S3 stage. The attractors of the S3 stage of development show chaotic and dense orbits.
In conclusion, an external drive can regulate the firing pattern of a single heat-sensitive neuron. A proper firing pattern can be created for any generic and functional neuron because its excitability and intrinsic energy pumping are also regulated. In other words, a neuron can only fire in a single pattern in response to a single channel of input, and when two distinct external stimuli are supplied at the same time, the final firing pattern becomes competitive. In the case of multi-channel stimulation, the competition between the two isolated discharge states as well as the injection and absorption of energy all affect the final discharge pattern of electrical activity. While inducing lower Hamilton energies, the periodic stimuli can significantly increase the occurrence of nonlinear resonance. When the periodic discharge mode of peak discharge and r-clonic discharge is induced, the participation of the periodic discharge mode helps to increase the sensitivity to the external signal. The chaotic excitation is increased, and the chaotic attractor's contour is altered when the chaotic discharge mode is activated. When the spiking emission mode interacts with the chaotic mode, the final discharge mode is more inclined to oscillate irregularly and is accompanied by the chaotic mode, because the spiking mode releases higher Hamilton energy than the chaotic mode, and the developed attractors tend to exhibit periodic orbits. In summary, neurons are more likely to react to stimuli that have higher amplitudes and energies, as well as those that cause more resonance and regularity in neuronal activity.
The main goal of the present study was to discuss which external stimulus can be rapidly received in neurons driven by two separate stimuli and white Gaussian noise, and then to analyze the potential response mechanism of the electrical activity of heat-sensitive neurons. Three main contributions can be drawn from the research:
(1) When neurons are excited, the firing pattern is related to the level of Hamilton energy. For example, the Hamilton energy of the peak mode is higher than that of the r-clonic mode and the chaotic mode. Physically speaking, any external stimulus has the ability to inject energy, some of which is absorbed by neurons. We have defined a correlation function to estimate the primary effect and contribution of each external stimulus and its corresponding pattern. Appropriate excitation in the peak mode can suppress r-clonic as well as chaotic mode, the final emission mode usually remains close to the mixed emission mode profile, and periodic attractors can be developed.
(2) The participation of periodic emission modes tends to cause significant emission mode changes and certain regularity, while other emission modes are suppressed due to possible nonlinear resonances. This means that external stimuli that produce periodic discharge patterns are more easily sensed and responded to.
(3) The main excited states and attractors show periodic and chaotic characteristics when peak mode interacts with r-clonic and chaotic modes. When a chaotic stimulus is applied, the chaotic discharge patterns remain active, and the profile of attractors can be changed due to the interaction with pulses, spike patterns, or even periodic discharge states that can provide a certain amount of energy to the neuron. However, variations in the chaotic attractor profile mean that interactions between chaotic modes, spikes, r-clonic, and periodic states can be efficiently detected, as chaotic states typically exhibit lower Hamilton energies than other emission modes. However, the Hamilton energy of Chua's circuit is often higher than that of the peak mode and r-clonic mode. These findings imply that energy flow controls competition between various discharge patterns, which ultimately results in regularity of neuronal activity, and that energy input from external stimuli is essential. This implies that controlling energy input may be another effective method for regulating neural activity.
The authors declare that they have not used Artificial Intelligence (AI) tools in the creation of this article.
This work was supported by the National Natural Science Foundation of China (Grant Nos. 12172210 and 11502139). The authors would like to thank the anonymous referees for their efforts and valuable comments.
The authors declare that there is no conflict of interest regarding the publication of this paper.
[1] |
S. A. H. Batouli, Seven Ambiguities in explaining the human memory system in the principles of neural science book, Basic Clin. Neurosci., 14 (2023), 543-548. https://doi.org/10.32598/bcn.2023.1774.4 doi: 10.32598/bcn.2023.1774.4
![]() |
[2] |
S. Masoli, M. F. Rizza, M. Sgritta, W. V. Geit, F. Schürmann, E. D'Angelo, Single neuron optimization as a basis for accurate biophysical modeling: the case of cerebellar granule cells, Front. Cell. Neurosci., 11 (2017), 71. https://doi.org/10.3389/fncel.2017.00071 doi: 10.3389/fncel.2017.00071
![]() |
[3] |
L. N. Groschner, J. G. Malis, B. Zuidinga, A. Borst, A biophysical account of multiplication by a single neuron, Nature, 603 (2022), 119–123. https://doi.org/10.1038/s41586-022-04428-3 doi: 10.1038/s41586-022-04428-3
![]() |
[4] |
E. Iavarone, J. Yi, Y. Shi, B. Zandt, C. O'Reilly, W. V. Geit, et al., Experimentally-constrained biophysical models of tonic and burst firing modes in thalamocortical neurons, PLOS Comput. Biol., 15 (2019), e1006753. https://doi.org/10.1371/journal.pcbi.1006753 doi: 10.1371/journal.pcbi.1006753
![]() |
[5] |
A. R. Cody, B. M. Johnstone, Single auditory neuron response during acute acoustic trauma, Hear. Res., 3 (1980), 3–16. https://doi.org/10.1016/0378-5955(80)90004-0 doi: 10.1016/0378-5955(80)90004-0
![]() |
[6] |
S. Karak, J. S. Jacobs, M. Kittelmann, C. Spalthoff, R. Katana, E. Sivan-Loukianova, et al., Diverse roles of axonemal dyneins in Drosophila auditory neuron function and mechanical amplification in hearing, Sci. Rep., 5 (2015), 17085. https://doi.org/10.1038/srep17085 doi: 10.1038/srep17085
![]() |
[7] |
A. Mizrahi, A. Shalev, I. Nelken, Single neuron and population coding of natural sounds in auditory cortex, Curr. Opin. Neurobiol., 24 (2014), 103–110. https://doi.org/10.1016/j.conb.2013.09.007 doi: 10.1016/j.conb.2013.09.007
![]() |
[8] |
S. Serizawa, K. Miyamichi, H. Sakano, One neuron–one receptor rule in the mouse olfactory system, Trends Genet., 20 (2004), 648–653. https://doi.org/10.1016/j.tig.2004.09.006 doi: 10.1016/j.tig.2004.09.006
![]() |
[9] |
G. M. Shepherd, Discrimination of molecular signals by the olfactory receptor neuron, Neuron, 13 (1994), 771–790. https://doi.org/10.1016/0896-6273(94)90245-3 doi: 10.1016/0896-6273(94)90245-3
![]() |
[10] |
A. Menini, Calcium signalling and regulation in olfactory neurons, Curr. Opin. Neurobiol., 9 (1999), 419–426. https://doi.org/10.1016/S0959-4388(99)80063-4 doi: 10.1016/S0959-4388(99)80063-4
![]() |
[11] |
F. Gabbiani, H. G. Krapp, C. Koch, G. Laurent, Multiplicative computation in a visual neuron sensitive to looming, Nature, 420 (2002), 320–324. https://doi.org/10.1038/nature01190 doi: 10.1038/nature01190
![]() |
[12] |
S. D. Wiederman, D. C. O'Carroll, Selective attention in an insect visual neuron, Curr. Biol., 23 (2013), 156–161. https://doi.org/10.1016/j.cub.2012.11.048 doi: 10.1016/j.cub.2012.11.048
![]() |
[13] |
D. A. Butts, Data-driven approaches to understanding visual neuron activity, Ann. Rev. Vis. Sci., 5 (2019), 451–477. https://doi.org/10.1146/annurev-vision-091718-014731 doi: 10.1146/annurev-vision-091718-014731
![]() |
[14] |
C. Tan, E. K. Cooke, D. E. Leib, Y. Lin, G. E. Daly, C. A. Zimmerman, et al., Warm-sensitive neurons that control body temperature, Cell, 167 (2016), 47–59. https://doi.org/10.1016/j.cell.2016.08.028 doi: 10.1016/j.cell.2016.08.028
![]() |
[15] | U. Homberg, S. Würden, Movement-sensitive, polarization-sensitive, and light-sensitive neurons of the medulla and accessory medulla of the locust, J. Comp. Neurol., 386 (1997), 329–346. |
[16] |
E. M. Callaway, R. Yuste, Stimulating neurons with light, Curr. Opin. Neurobiol., 12 (2002), 587–592. https://doi.org/10.1016/S0959-4388(02)00364-1 doi: 10.1016/S0959-4388(02)00364-1
![]() |
[17] |
M. E. Yamakou, T. D. Tran, L. H. Duc, J. Jost, The stochastic Fitzhugh–Nagumo neuron model in the excitable regime embeds a leaky integrate-and-fire model, J. Math. Biol., 79 (2019), 509–532. https://doi.org/10.1007/s00285-019-01366-z doi: 10.1007/s00285-019-01366-z
![]() |
[18] |
A. C. Murza, Oscillation patterns in tori of modified FHN neurons, Appl. Math. Modell., 35 (2011), 1096–1106. https://doi.org/10.1016/j.apm.2010.07.055 doi: 10.1016/j.apm.2010.07.055
![]() |
[19] |
D. Yu, G. Wang, Q. Ding, T. Li, Y. Jia, Effects of bounded noise and time delay on signal transmission in excitable neural networks, Chaos Solitons Fractals, 157 (2022), 111929. https://doi.org/10.1016/j.chaos.2022.111929 doi: 10.1016/j.chaos.2022.111929
![]() |
[20] |
Y. Jiang, J. Wu, H. Yang, F. Xu, M. Wang, S. Huang, et al., Chimera states mediated by nonlocally attractive-repulsive coupling in FitzHugh–Nagumo neural networks, Chin. J. Phys., 66 (2020), 172–179. https://doi.org/10.1016/j.cjph.2020.03.019 doi: 10.1016/j.cjph.2020.03.019
![]() |
[21] |
Z. Wu, H. Cheng, Y. Feng, H. Li, Q. Dai, J. Yang, Chimera states in bipartite networks of FitzHugh–Nagumo oscillators, Front. Phys., 13 (2018), 130503. https://doi.org/10.1007/s11467-017-0737-z doi: 10.1007/s11467-017-0737-z
![]() |
[22] |
F. Yang, Y. Wang, J. Ma, Creation of heterogeneity or defects in a memristive neural network under energy flow, Commun. Nonlinear Sci. Numer. Simul., 119 (2023), 107127. https://doi.org/10.1016/j.cnsns.2023.107127 doi: 10.1016/j.cnsns.2023.107127
![]() |
[23] |
Y. Wang, C. Wang, G. Ren, J. Tang, W. Jin, Energy dependence on modes of electric activities of neuron driven by multi-channel signals, Nonlinear Dyn., 89 (2017), 1967–1987. https://doi.org/10.1007/s11071-017-3564-4 doi: 10.1007/s11071-017-3564-4
![]() |
[24] |
K. Usha, P. A. Subha, Collective dynamics and energy aspects of star-coupled Hindmarsh–Rose neuron model with electrical, chemical and field couplings, Nonlinear Dyn., 96(2019), 2115–2124. https://doi.org/10.1007/s11071-019-04909-7 doi: 10.1007/s11071-019-04909-7
![]() |
[25] |
Y. Yang, J. Ma, Y. Xu, Y. Jia, Energy dependence on discharge mode of Izhikevich neuron driven by external stimulus under electromagnetic induction, Cogn. Neurodyn., 15 (2021), 265–277. https://doi.org/10.1007/s11571-020-09596-4 doi: 10.1007/s11571-020-09596-4
![]() |
[26] |
B. Ramakrishnan, G. D. Leutcho, K. Rajagopal, S. Jafari, P. L. Ndukum, G. D. Leutcho, Approximate symmetry memristive mega-stable oscillator with attractor growing and its Hamilton energy balance, Eur. Phys. J. Plus, 137 (2022), 596. https://doi.org/10.1140/epjp/s13360-022-02779-4 doi: 10.1140/epjp/s13360-022-02779-4
![]() |
[27] |
M. Ge, G. Wang, Y. Jia, Influence of the Gaussian colored noise and electromagnetic radiation on the propagation of subthreshold signals in feedforward neural networks, Sci. China Technol. Sci., 64 (2021), 847–857. https://doi.org/10.1007/s11431-020-1696-8 doi: 10.1007/s11431-020-1696-8
![]() |
[28] |
Y. Chen, L. Yu, Y. Chen, Reliability of weak signals detection in neurons with noise, Sci. China Technol. Sci, 59 (2016), 411–417. https://doi.org/10.1007/s11431-015-6000-3 doi: 10.1007/s11431-015-6000-3
![]() |
[29] |
P. Fatemeh, R. Karthikeyan, A. Karthikeyan, A. Alsaedi, T. Hayat, V. Pham, Complex dynamics of a neuron model with discontinuous magnetic induction and exposed to external radiation, Cogn. Neurodyn., 12 (2018), 607–614. https://doi.org/10.1007/s11571-018-9497-x doi: 10.1007/s11571-018-9497-x
![]() |
[30] |
B. Cao, R. Wang, H. Gu, Y. Li, Coherence resonance for neuronal bursting with spike undershoot, Cogn. Neurodyn., 15 (2021), 77–90. https://doi.org/10.1007/s11571-020-09595-5 doi: 10.1007/s11571-020-09595-5
![]() |
[31] |
Y. Kang, R. Liu, X. Mao, Aperiodic stochastic resonance in neural information processing with Gaussian colored noise, Cogn. Neurodyn., 15 (2021), 517–532. https://doi.org/10.1007/s11571-020-09632-3 doi: 10.1007/s11571-020-09632-3
![]() |
[32] |
J. Zhao, Y. Qin, Y. Che, H. Ran, J. Li, Effects of network topologies on stochastic resonance in feedforward neural network, Cogn. Neurodyn., 14 (2020), 399–409. https://doi.org/10.1007/s11571-020-09576-8 doi: 10.1007/s11571-020-09576-8
![]() |
[33] |
Y. Jia, H. Gu, Transition from double coherence resonances to single coherence resonance in a neuronal network with phase noise, Chaos, 25 (2015), 123124. https://doi.org/10.1063/1.4938733 doi: 10.1063/1.4938733
![]() |
[34] |
X. Yang, Y. Yu, Z. Sun, Autapse-induced multiple stochastic resonances in a modular neuronal network, Chaos, 27 (2017), 083117. https://doi.org/10.1063/1.4999100 doi: 10.1063/1.4999100
![]() |
[35] |
Y. Xu, M. Liu, Z. Zhu, J. Ma, Dynamics and coherence resonance in a thermosensitive neuron driven by photocurrent, Chin. Phys. B, 29 (2020), 098704. https://doi.org/10.1088/1674-1056/ab9dee doi: 10.1088/1674-1056/ab9dee
![]() |
[36] |
Y. Yu, F. Han, Q. Wang, Dynamic modeling of neuromodulation techniques: Towards elaboration and individual specificity, Europhysics Letters, 145 (2024), 32001. https://doi.org/10.1209/0295-5075/ad239b doi: 10.1209/0295-5075/ad239b
![]() |
[37] |
Y. B. Jia, X. L. Yang, J. Kurths, Diversity and time delays induce resonance in a modular neuronal network, Chaos, 24 (2014), 043140. https://doi.org/10.1063/1.4904101 doi: 10.1063/1.4904101
![]() |
[38] |
Y. Xu, J. Ma, Control of firing activities in thermosensitive neuron by activating excitatory autapse, Chin. Phys. B, 30 (2021), 100501. https://doi.org/10.1088/1674-1056/abeeef doi: 10.1088/1674-1056/abeeef
![]() |
[39] |
J. Ma, Biophysical neurons, energy, and synapse controllability: A review, J. Zhejiang Univ. Sci. A, 24 (2023), 109–129. https://doi.org/10.1631/jzus.A2200469 doi: 10.1631/jzus.A2200469
![]() |
[40] |
L. Gammaitoni, P. Hänggi, P. Jung, F. Marchesoni, Stochastic resonance, Rev. Modern Phys., 70 (1998), 223–287. https://doi.org/10.1103/RevModPhys.70.223 doi: 10.1103/RevModPhys.70.223
![]() |
[41] |
M. Xing, X. Song, Z. Yang, Y. Chen, Bifurcations and excitability in the temperature-sensitive Morris–Lecar neuron, Nonlinear Dyn., 100 (2020), 2687–2698. https://doi.org/10.1007/s11071-020-05667-7 doi: 10.1007/s11071-020-05667-7
![]() |
[42] |
G. D. Leutcho, L. Woodward, F. Blanchard, Nonlinear dynamics of a single-gap terahertz split-ring resonator under electromagnetic radiation, Chaos, 33 (2023), 103131. https://doi.org/10.1063/5.0157489 doi: 10.1063/5.0157489
![]() |
[43] |
A. Hariri, M. Babaie-Zadeh, Compressive detection of sparse signals in additive white Gaussian noise without signal reconstruction, Signal Process., 131 (2017), 376–385. https://doi.org/10.1016/j.sigpro.2016.08.020 doi: 10.1016/j.sigpro.2016.08.020
![]() |
[44] |
Y. Guo, Z. Yao, Y. Xu, J. Ma, Control the stability in chaotic circuit coupled by memristor in different branch circuits, AEU Int. J. Electron. Commun., 145 (2022), 154074. https://doi.org/10.1016/j.aeue.2021.154074 doi: 10.1016/j.aeue.2021.154074
![]() |
[45] |
Y. Xie, P. Zhou, Z. Yao, J. Ma, Response mechanism in a functional neuron under multiple stimuli, Phys. A, 607 (2022), 128175. https://doi.org/10.1016/j.physa.2022.128175 doi: 10.1016/j.physa.2022.128175
![]() |
[46] |
N. Kuznetsov, T. Mokaev, V. Ponomarenko, E. Seleznev, N. Stankevich, L. Chua, Hidden attractors in Chua circuit: Mathematical theory meets physical experiments, Nonlinear Dyn., 111 (2023), 5859–5887. https://doi.org/10.1007/s11071-022-08078-y doi: 10.1007/s11071-022-08078-y
![]() |
[47] |
L. Yan, H. Zhang, Z. Sun, S. Liu, Y. Liu, P. Xiao, Optimization of stimulation waveforms for regulating spike-wave discharges in a thalamocortical model, Chaos Solitons Fractals, 158 (2022), 112025. https://doi.org/10.1016/j.chaos.2022.112025 doi: 10.1016/j.chaos.2022.112025
![]() |