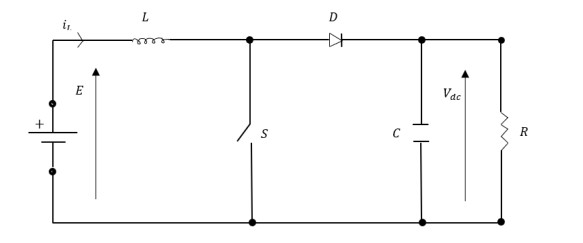
To synthesize the proper control signal while guaranteeing the necessary performance indices (speed, resilience, accuracy, etc.), mathematical models were frequently used to represent physical systems. These descriptions were utilized for control, monitoring, and detection in these kinds of systems. Quality and performance of the process may suffer if the model is inaccurate or incomplete. As a result, conformable systems (CS) may be used to make these mathematical models more near to the real world. However, non-power-electronics experts who need to model and simulate complex systems may find the task of modeling static converters to be rather challenging. Researchers have just recently outlined the properties of the general conformable systems (GCS). This innovative approach built upon the principle of the classical integer order systems, employing the same mathematical foundations for its derivation. With the introduction of this novel description of systems, a fresh array of differential equations emerged, specifically tailored for the realm of direct current to direct current (DC-DC) static converters. GCS has been proved to be more flexible and profitable than the traditional integer-order one for representing DC-DC static converters. This advancement paved the way for more effective control techniques based on the Lyapunov method, with practical applications in photovoltaic (PV) systems and beyond.
Citation: Omar kahouli, Mourad Elloumi, Omar Naifar, Abdellatif Ben Makhlouf, Yassine Bouteraa, Sarra Elgharbi. Enhanced general conformable controller based on Lyapunov technique for DC-DC static converters: Application to a solar system[J]. AIMS Mathematics, 2024, 9(5): 10698-10716. doi: 10.3934/math.2024522
[1] | Yinghui Dong, Chengjin Tang, Chunrong Hua . Optimal investment of DC pension plan under a joint VaR-ES constraint. AIMS Mathematics, 2024, 9(1): 2084-2104. doi: 10.3934/math.2024104 |
[2] | Fidel Meléndez-Vázquez, Guillermo Fernández-Anaya, Aldo Jonathan Muñóz-Vázquez, Eduardo Gamaliel Hernández-Martínez . Generalized conformable operators: Application to the design of nonlinear observers. AIMS Mathematics, 2021, 6(11): 12952-12975. doi: 10.3934/math.2021749 |
[3] | Hatoon Shoaib . Double circulant complementary dual codes over $ \mathbb{F}_4 $. AIMS Mathematics, 2023, 8(9): 21636-21643. doi: 10.3934/math.20231103 |
[4] | Mustafa Inc, Hadi Rezazadeh, Javad Vahidi, Mostafa Eslami, Mehmet Ali Akinlar, Muhammad Nasir Ali, Yu-Ming Chu . New solitary wave solutions for the conformable Klein-Gordon equation with quantic nonlinearity. AIMS Mathematics, 2020, 5(6): 6972-6984. doi: 10.3934/math.2020447 |
[5] | Narongrit Kaewbanjak, Watcharin Chartbupapan, Kamsing Nonlaopon, Kanit Mukdasai . The Lyapunov-Razumikhin theorem for the conformable fractional system with delay. AIMS Mathematics, 2022, 7(3): 4795-4802. doi: 10.3934/math.2022267 |
[6] | Xiaoyi Tang, Wei Liu, Wanyin Wu, Yijun Hu . An optimal investment strategy for DC pension plans with costs and the return of premium clauses under the CEV model. AIMS Mathematics, 2024, 9(8): 21731-21754. doi: 10.3934/math.20241057 |
[7] | B. El-Sobky, M. F. Zidan . A trust-region based an active-set interior-point algorithm for fuzzy continuous Static Games. AIMS Mathematics, 2023, 8(6): 13706-13724. doi: 10.3934/math.2023696 |
[8] | Mesut GUVEN . Leveraging deep learning and image conversion of executable files for effective malware detection: A static malware analysis approach. AIMS Mathematics, 2024, 9(6): 15223-15245. doi: 10.3934/math.2024739 |
[9] | Tareq Eriqat, Rania Saadeh, Ahmad El-Ajou, Ahmad Qazza, Moa'ath N. Oqielat, Ahmad Ghazal . A new analytical algorithm for uncertain fractional differential equations in the fuzzy conformable sense. AIMS Mathematics, 2024, 9(4): 9641-9681. doi: 10.3934/math.2024472 |
[10] | Danhua He, Baizeng Bao, Liguang Xu . Robust stability and boundedness of uncertain conformable fractional-order delay systems under input saturation. AIMS Mathematics, 2023, 8(9): 21123-21137. doi: 10.3934/math.20231076 |
To synthesize the proper control signal while guaranteeing the necessary performance indices (speed, resilience, accuracy, etc.), mathematical models were frequently used to represent physical systems. These descriptions were utilized for control, monitoring, and detection in these kinds of systems. Quality and performance of the process may suffer if the model is inaccurate or incomplete. As a result, conformable systems (CS) may be used to make these mathematical models more near to the real world. However, non-power-electronics experts who need to model and simulate complex systems may find the task of modeling static converters to be rather challenging. Researchers have just recently outlined the properties of the general conformable systems (GCS). This innovative approach built upon the principle of the classical integer order systems, employing the same mathematical foundations for its derivation. With the introduction of this novel description of systems, a fresh array of differential equations emerged, specifically tailored for the realm of direct current to direct current (DC-DC) static converters. GCS has been proved to be more flexible and profitable than the traditional integer-order one for representing DC-DC static converters. This advancement paved the way for more effective control techniques based on the Lyapunov method, with practical applications in photovoltaic (PV) systems and beyond.
In recent years, clean energy has become increasingly popular. Given its boundless potential and benign impact on the environment, green energy is a legitimate and effective means of stimulating the economy. Improving the efficiency of "green" energy production requires research into the entire conversion chain, from power generation extraction to structural conversion, to electrical conversion, to battery storage, to transformation, to grid integration. In this case, DC-DC converters are a crucial part of the conversion process. They are often used in photovoltaics (PVs), wind turbines, and fuel cells, among other power generation and storage devices [1,2,3,4].
For instance, they are used in grid-connected fixed-speed wind turbines [4], fault detection methods for boost converters [5], and power regulation of wind generators [1]. These inverters are frequently used to match the input voltage of a system to the required output voltage [5].
Conversely, it's worth noting that ordinal computations aren't enough for exploring a wide variety of systems whose behavior is better described by fractional order. Particularly, the simulation of battery systems [6] and thermal performance systems [7] have found fruitful use of the fractional-order calculus. Stability analysis employing fractional-order systems has also seen a dramatic uptick in popularity over the past decade [8,9,10]. In fact, while the Lyapunov technique is a powerful method for investigating the robustness of dynamical systems, much recent research has concentrated on the asymptotic stability of such systems and has paid far less attention to their boundedness. Considering this, the topic of the boundedness of nonlinear nabla fractional-order systems has been addressed in work [8]. Using the nabla Laplace transform, two stability conditions in the form of the Lyapunov theorem are constructed. The theoretical conclusions are then used in two numerical examples to show how practical they are. In addition, a fractional-order Lyapunov-based robust controller with a fuzzy neural network compensator has been studied in work [9]. First, a procedure for building a finite-time fractional-order nonsingular fast terminal sliding mode control is described. Second, a fuzzy neural network technique is created to evaluate model uncertainty and external disturbances. Different ideas associated with the fractional differential equation are starting to emerge in research. Numerous re-cent articles in the field of dynamic systems make use of multiple fractional derivative (FD) concepts, most notably the Caputo derivative [11] and/or the Caputo-Katugampola derivative [12].
Recent advancements in applied physics and engineering have led to significant progress in understanding complex phenomena. Abo-Dahab, Abouelregal, and Marin explored thermoelastic behavior in functionally graded materials, focusing on the effects of non-Gaussian laser beams on thin, slim strips [13]. Othman, Fekry, and Marin investigated plane wave propagation in a generalized magneto-thermo-viscoelastic medium with voids, revealing insights into material dynamics under mechanical and thermal loads [14]. Meanwhile, Sene and Srivastava's study delved into the generalized Mittag-Leffler input stability, contributing to the theoretical framework for dynamic systems described by fractional calculus [15]. These studies represent significant strides in understanding complex physical phenomena with wide-ranging applications in science and engineering.
The "conformable derivative, " as it is named in Khalil et al. [12], is a unique and elegant that has been the subject of extensive research used on the general conformable differential equation (GCDE). In the context of studying nonlinear systems, it's important to remember that the conformable derivative can be used to address some technical problems. With a few exceptions, all fractional derivatives, with the exception of the conformable one, have shortcomings. One such example is the product and chain rule of two functions. The monotonicity of a function f cannot be inferred from the sign of its fractional derivative either in the Riemann-Liouville or Caputo approaches [9]. The following references [16,17,18,19] are used as examples of when one may use the conformable derivative. As a matter of fact, a method of estimating for a non-integer order derivative was proposed in [19]. There is discussion of both a situation when everything is working, as it should and one where something goes wrong. This study demonstrates the convergence of estimate errors by using a version of Barbalat's lemma that fails when using the widely known Caputo derivative. In [17], a robust H∞ observer is introduced. In addition, the integrated power system is studied in [18]. Also, the authors of [20,21,22] characterize the resistor (R), inductor (L), and capacitor (C) components that form the RLC, RC, and LC circuits using the conformable derivative (CD). Furthermore, the general conformable derivative (GCS) and its features have recently been characterized by academics.
These systems are extensions of the conformable systems and are calculated the same. Authors in [23,24,25] use this novel approach to develop a new family of differential equations applicable to electrical circuits like RLC, RC, and LC. It has been demonstrated that the GCSs of electrical circuits is more flexible and advantageous than the more popular conformable representation.
Mathematical models are used to represent electrical circuits. This talk is being given in response to this inquiry. In mathematical terms, what best represents reality? We note that GCS are superior to classical integer-order systems, as previously demonstrated in the literature. We conclude that the GCS is a viable method for characterizing DC-DC converters and present this approach in our research. We have shown that this option is more flexible, and our modeling problem can now be solved with just a few equations.
Considering the foregoing, it should come as no surprise that simulations of various kinds involving renewable energy systems require great precision and accuracy in DC-DC converter models. It is a well-accepted fact that mathematical models can serve as adequate descriptions of physical systems. Such mathematical models are employed in the management of these systems for the purposes of control, monitoring, and sensing. Inaccuracies in the models used to create the system can have a negative effect on its overall effectiveness. This means that GCS can be used to bring mathematical models closer to the real world. Therefore, the purpose of this work is to investigate and suggest a simple modeling approach based on general conformable (GC) representation. To the best of our knowledge, no GCS-based power converters have been described in the literature. It has been demonstrated that GCS is a more flexible and lucrative representation of DC-DC converters than the traditional integer-order representation.
The essence of our efforts can be encapsulated in the following highlights:
● The utilization of the GC representation for modeling DC-DC converters represents a groundbreaking approach that has hitherto remained unexplored in the existing body of literature.
● Employing the GC representation for DC-DC converter modeling offers superior suitability and widens the spectrum of available model choices. This matter has profound implications for the controlled system, exerting a direct influence on both performance and the precision of the sought-after outcomes.
● The paper presents a novel control approach based on the Lyapunov technique for DC-DC static converters using the GC representation.
● The paper demonstrates the practical application of the proposed control technique to PV systems. By incorporating the GCS-based control approach, the performance of PV systems can be enhanced, leading to improved energy conversion efficiency and better utilization of renewable energy sources.
The subsequent sections of the paper are structured as follows:
(1) Section 2 introduces the fundamental principles of GC calculus.
(2) Following that, in the third section, we provide a mathematical formulation and simulation of the GC model for the boost converter.
(3) Section 4 delves into the GC mathematical model and simulation of the buck-boost converter.
(4) In Section 5, we explore the GC mathematical model of the non-inverting buck-boost converter.
(5) Section 7 outlines the model-based reference control design.
(6) Section 8 contains the results and discussion, showcasing its application to a DC-DC converter connected to a PV panel.
(7) Finally, Section 9 offers a conclusion and a succinct summary of the work.
Some basis and preliminaries are given at the outset of this section [26,27].
Definition 2.1. Suppose α∈(0,1]. Let ϕ be a function defined on [0b), then the GC derivative of ϕ is given by:
Tα,ψϕ(t)=limε⟶0ϕ(t+εψ(t,α))−ϕ(t)ε,∀t>0, |
with ψ(t,α) as a continuous positive function verifying:
ψ(t,1)=1, |
ψ(.,α1)≠ψ(.,α2),whereα1≠α2andα1,α2∈(0,1]. |
Remark 2.1. If Tα,ψϕ(t), ∀t∈(0,c) exists, and for a certain c>0, limt⟶0+Tα,ψϕ(t) exists, then:
Tα,ψϕ(0):=limt⟶0+Tα,ψϕ(t). |
Remark 2.2. The integer order derivative (α=1) and the CD ψ(t)=t1−α are generalized by the general conformable derivative [28].
Remark 2.3. In order to learn more about the characteristics of the GC derivative, we assume that ψ(t,α)>0 ∀t>0 and 1ψ(.,α) is locally integrable.
In this section, we consider a boost converter, which can be represented by the following electrical circuit (Figure 1):
By applying the Kirchhoff's rules to the previous electrical circuit, as shown in Figure 1, and based on the operating mode and the condition of the switch S, we can express the considered dynamical system by the following mathematical model that describes its dynamic behavior [3]:
LdiL(t)dt=E−Vdc(t)(1−u), | (1a) |
CdVdc(t)dt=iL(1−u)−VdcR, | (1b) |
where iL is the current in the coil L, E is the input voltage, Vdc is the output voltage and u is the control. The system parameters are coil inductance L presented in H, the capacitance of the capacitor C in F and the resistance of the load R in Ω.
The voltage across the capacitor and the current flowing through the coil serve as the state variables. The control signal u specifies the status of the switch S, which is open for 0 and closed for 1, and is part of the discrete domain of 0 and 1. It can be replaced by its average value over a chopping period which represents the duty cycle γ=TonTs where Ton is the conduction time and Ts is the chopping period.
The purpose of this section is to show how to transform an ordinary differential equation to a GCDE. This will be accomplished through the application of the chopper's dynamic ordinary equations (1). Rosales et al. [29] developed differential equations using a comprehensive technique, as they had previously done [30,31]. It entails the following presentation of an item in an appropriately sized format [29]. Now, inspired by the method used in [24], the transformation to the GC derivative is:
ddt⟶1ψ(τ,α)dαdt, | (2) |
where α∈(0,1] is the derivative order and τ is a component that describes the system's temporal elements; its measurement is the second (s). In our case, τ in (1a) does indeed have a sequence as L and it has a sequence as RC in (1b). Thus, we might extract it and formulate the following expression:
ddt→1ψ(L,α)dαdt, | (3a) |
ddt→1ψ(RC,α)dαdt. | (3b) |
Given the connection (3) and employing the general conformable derivative, we have:
dαf(t)dt=ψ(t,α)ddtf(t), | (4) |
Inspired by [32], we get:
ddt⟶1ψ(L,α)dαdt=ψ(t,α)ψ(L,α)ddt, | (5a) |
ddt⟶1ψ(RC,α)dαdt=ψ(t,α)ψ(RC,α)ddt. | (5b) |
The formula (5) can be described as a time conformable transform. We have (6) by substituting (5) into (1).
diL(t)dt=Eψ(L,α)Lψ(t,α)−ψ(L,α)Lψ(t,α)(1−u)Vdc(t), | (6a) |
dVdc(t)dt=ψ(RC,α)Cψ(t,α)iL(1−u)−ψ(RC,α)RCψ(t,α)Vdc. | (6b) |
For the boost converter, Eqs (6a) and (6b) present the GCDE.
Remark 3.1. If one takes α=1, we get the classical model of the DC-DC converter described by Eq (1).
Remark 3.2. If one takes ψ(t,α)=t1−α, we get the mathematical model of the DC-DC converter described by the classical conformable derivative.
For the simulation, one chooses the following parameters: R=3Ω, C=47nF, L=0.01H, E=10V, u=0.5, and f=60Hz. One chooses ψ(t,α)=t1−α. In that case, one gets the curves of the classical conformable derivative. We obtain the following responses for various values of α. When α equals to 1, the GCD acts identically to the conventional case, as illustrated in Figure 2. When α drops, it is observed that the conformable derivative reaches the steady state more rapidly. As can be seen, the amplitude at startup is also dropping.
For various expressions of ψ, the generated curves results of the GCDE are plotted. One chooses, for example, ψ(t,α)=t1−α((1−α)g2(t)+1), in which one supposes two expressions of g(t);thefistoneisg(t)=g1(t)=√30sin(t) and the second one is g(t)=g2(t)=√10e−t. Figure 3 illustrates the following responses for the derivative order α=0.8.
The presentation of this sort of converter operation using mathematical equations must consider the state of the interrupter S in Figure 4. When the switch is closed, then Ton=γTs. As a result, the energy stored in the inductance increases. However, in the opposite case, Toff=(1−γ)Ts and the energy accumulated in the inductance transfers to the capacitance and the load.
We have the following equations [3]:
LdiL(t)dt=uE−Vdc(t)(1−u), | (7a) |
CdVdc(t)dt=−iL(1−u)−VdcR. | (7b) |
The approach used to get the dynamic equations in a continuous conduction regime is identical to that used in the boost converter scenario. When S is equal to 1, the diode is blocked, and the following equations apply:
LdiL(t)dt=uE, | (8a) |
CdVdc(t)dt=−VdcR. | (8b) |
When the switch is blocked, S is equal to 0 and the diode is on. The equations are:
LdiL(t)dt=Vdc, | (9a) |
CdVdc(t)dt=−iL−VdcR. | (9b) |
Following the preceding arguments, the GCDE (7), (8), and (9) are represented by Eqs (10)–(12), respectively:
diL(t)dt=ψ(L,α)Lψ(t,α)u−ψ(L,α)Lψ(t,α)Vdc(t)(1−u), | (10a) |
dVdc(t)dt=−ψ(RC,α)Cψ(t,α)iL(1−u)−ψ(RC,α)RCψ(t,α)Vdc. | (10b) |
diL(t)dt=ψ(L,α)Lψ(t,α)uE, | (11a) |
dVdc(t)dt=−ψ(RC,α)RCψ(t,α)Vdc. | (11b) |
diL(t)dt=ψ(L,α)Lψ(t,α)Vdc, | (12a) |
dVdc(t)dt=−ψ(RC,α)Cψ(t,α)iL−ψ(RC,α)RCψ(t,α)Vdc. | (12b) |
For the simulation, one chose the same parameters as previous. We get the following results for different values of α.
When α = 1, the GCD behaves identically to the normal case, as illustrated in Figure 5. When α decreases, the conformable derivative approaches the steady state more rapidly. We can also see that the amplitude in the start is decreasing.
For various expressions of ψ, the generated curves results of the GCDE are plotted. One chooses ψ(t,α)=t1−α((1−α)g2(t)+1). One supposes two equations of g(t),g(t)=g1(t)=√30sin(t) and g(t)=g2(t)=√10e−t. Figure 6 illustrates the following responses for the derivative order α=0.8.
If the output voltage cannot be reversed, a cascade configuration of the buck converter and the boost converter with a complementary switch can be employed, as illustrated in Figure 7. The model for this converter can be produced quickly by following the same procedure as for the buck-boost converter, but just by changing the ratio of the input and output voltages.
Based on [3], we have:
LdiL(t)dt=uE−Vdc(t)(1−u), | (13a) |
CdVdc(t)dt=−iL(1−u)−VdcR. | (13b) |
According to the previous reasoning, the GCDE of Eq (13) is provided by Eq (14), which is as follows:
diL(t)dt=ψ(L,α)Lψ(t,α)uE−ψ(L,α)Lψ(t,α)(1−u)Vdc(t), | (14a) |
dVdc(t)dt=−ψ(RC,α)Cψ(t,α)(1−u)iL−ψ(RC,α)RCψ(t,α)Vdc. | (14b) |
For the simulation, one uses the identical settings as before. For various values of α, Figure 8 shows the evolution of Vdc.
When α=1, the GCD behaves exactly as in the usual case, as shown in Figure 6. It is shown that as α goes down, the CD approaches the steady state quicker. We can see that the amplitude at the start is likewise decreasing.
For various expressions of ψ, the generated curves results of the GCDE are plotted. One chooses ψ(t,α)=t1−α((1−α)g2(t)+1). One supposes two expressions of g(t),g(t)=g1(t)=√30sin(t) and g(t)=g2(t)=√10e−t. Figure 9 illustrates the following responses for the derivative order α=0.8.
One supposes x=[iLVdc]Tv0=Vdc is the output voltage and vin=E is the input voltage. The state space form during the "ON" mode can be written as follows:
Tα,ψ(iLVdc)=(000−1RC)(iLVdc)+(1L0)vin,v0=(01)(iLVdc). | (15) |
In the "OFF" mode of the boost converter circuit, the energy stored in the inductor is transferred to the output RC circuit through the diode. By applying Kirchhoff's Voltage Law (KVL) and Kirchhoff's Current Law (KCL) to the circuit shown in Figure 1 when the switch is off, we can obtain the following set of state equations.
Tα,ψ(iLVdc)=(0−1L1C−1RC)(iLVdc)+(1L0)vin,v0=(01)(iLVdc)=0. | (16) |
The utilization of the state-space averaging technique enables the derivation of a converter model that effectively characterizes the behavior of the circuit across a full switching period. Rather than relying on distinct state-space descriptions for individual modes, a consolidated state-space description is attained to effectively approximate the circuit's behavior throughout the entire temporal span denoted as T. Using Eqs (15) and (16), This averaged modified model is obtained through the application of the state-space averaging technique, yielding the following representation:
A=A1d+A2(1−h),B=B1d+B2(1−h), | (17) |
where h is the Duty cycle and A1=(000−1RC), A2=(0−1L1C−1RC),B1=B2=(1L0).
Using Eq (17), we have:
Tα,ψ(iLVdc)=(0−(1−h)L(1−h)C−1RC)(iLVdc)+(1L0)vin,v0=(01)(iLVdc). | (18) |
By substituting the appropriate values and simplifying Eq (18), it is possible to derive the steady-state model of the boost converter: Tα,ψ(iLVdc)=0 and h=H where H is the steady-state duty cycle. In this case, Eq (18) becomes:
(00)=(0−(1−H)L(1−H)C−1RC)(iLVdc)+(1L0)vin,v0=(01)(iLVdc). | (19) |
Equation (19) can be used to express the steady-state relationship between the output voltage v0 and the input voltage E.
v0E=11−H. | (20) |
To attain the transfer function of the boost converter, we embark on a process of linearizing the model defined in Eq (19) with respect to a designated operational state. This state, we hypothesize, hinges on the steady values of inductor current (IL), capacitor voltage (VDC), duty cycle (H), and input voltage (Vin). By accounting for slight deviations from this operational point, we can express the variables related to the average model in the following manner:
iL=IL+~iL, |
Vdc=VDC+~Vdc, |
vin=Vin+~vin, |
h=H+˜h. |
Within this equation, we represent the minor deviations in inductor current, capacitor voltage, and input voltage as ~iL, ~Vdc, and ~vin. Accordingly, we can reframe Eq (18) as follows:
Tα,ψ(IL+~iLVDC+~Vdc)=(0−(1−H)L(1−H)C−1RC)(ILVDC)+(0−(1−H)L(1−H)C−1RC)(~iL~Vdc)+(0˜hL−˜hC0)(ILVDC)+(1L0)(Vin+~vin). | (21) |
It is important to highlight that the steady-state component of Eq (21) can be expressed as:
(0−(1−H)L(1−H)C−1RC)(ILVDC)+(1L0)Vin=0and(0˜hL−˜hC0)(ILVDC)=(VDCL−ILC)˜h. |
Therefore, Eq (21) is simplified to:
Tα,ψ(~iL~Vdc)=(0−(1−H)L(1−H)C−1RC)(~iL~Vdc)+(1LVDCL0−ILC)(~vin˜h). | (22) |
In the end, we can directly express the disturbance in the output voltage as follows:
~v0=(01)(~iL~Vdc). | (23) |
The state-space depiction of Eqs (22) and (23) is presented as follows:
Tα,ψx=Ax+Bu,y=Cx, | (24) |
where =(~iL~Vdc), A=(0−(1−H)L(1−H)C−1RC), B=(1LVDCL0−ILC) and C=(01).
Consider the system:
Tα,ψx(t)=F(t,x), | (25) |
where F:R+×Rn→Rn is a continuous function.
Definition 7.1. [33] System (23) is said to be uniformly practically exponentially stable if ∃σ>0, λ>0, ρ>0 such that:
||x(t)||≤σ||x0||Eψα(−λ,t,0)+ρ,∀t≥0. |
Remark 7.1. When ρ=0, system (23) is said to be exponentially stable.
In this paper, we focus on the following linear plant, applicable ∀t≥t0.
Tα,ψx(t)=Ax(t)+Bu(t),y(t)=Cx(t). | (26) |
Here, x(t) represents the state of the system in n-dimensional real space, while u(t) denotes the control input under design in m-dimensional real space, and y(t) signifies the output of the system in q-dimensional real space.
The model to be adhered to is delineated and applies for all instances where t≥t0 as follows:
Tα,ψxm(t)=Amxm(t),ym(t)=Cmxm(t). | (27) |
In Eqs (26) and (27), we have xm(t) in n1-dimensional real space, representing the model state, and ym(t) in q1-dimensional real space, signifying the model output. The matrices A,B, and C are established in n-dimensional real space, whereas Am is in n1×n1-dimensional real space and Cm is in q1×n1-dimensional real space, all being familiar components.
Within this section, our aim is to devise a model reference control strategy that relies on an observer and empowers the plant (Eq (25)) to faithfully follow the model (Eq (26)) with a diminishing tracking error that ultimately reaches zero. To accomplish this, we introduce the ensuing assumptions.
Assumption 7.1. Matrix Am exhibits stability. The duo (A,B) possesses controllability. The pair (A,C) showcases observability.
Assumption 7.2. There is a matrix F in n×n1-dimensional real space and another matrix G in m×n1-dimensional real space such that the following condition is satisfied:
[ABC0][FG]=[FAmCm]. | (28) |
To estimate the unmeasured states of the plant, including those that are not directly measurable, we employ a Luenberger-like observer as follows:
Tα,ψxz(t)=Axz(t)+Bu(t)+LC(xp(t)−xz(t)). | (29) |
Here, xz(t) belongs to n-dimensional real space, representing the estimated state vector, and L in Rn×q stands for a gain matrix to be appropriately determined at a later stage. Let's define the error between the model states and the plant states as follows:
e(t)=Fxm(t)−xp(t). | (30) |
The dynamics of the derivative of the observer, based on Eqs (26) and (27), can be described by:
Tα,ψe(t)=Ae(t)−Bu(t)+(FAm−AF)xm(t). | (31) |
Denote the error in state estimation as:
Tα,ψe(t)=Ae(t)−Bu(t)+(FAm−AF)xm(t). | (32) |
Allow the error in estimating the state to be defined as follows:
ez(t)=xz(t)−xp(t). | (33) |
Subsequently, the derivative can be expressed as follows:
Tα,ψez(t)=(A−LC)ez(t). | (34) |
Establish the subsequent linear control law as follows:
u(t)=γBTPxz(t)−(γBTPF−G)xm(t). | (35) |
In this context, we introduce a design scalar, γ, and a design symmetric positive definite matrix, P, in Rn×n. According to the following theorem, it is proclaimed that the proposed control law (Eq (35)) is adept at achieving exponential stability of tracking errors, ensuring that the plant output accurately traces the model output.
Theorem 7.1. Contemplating the linear plant (Eq (26)) and the linear model (Eq (27)) within the framework of Assumptions 7.1 and 7.2, the observer-based control law (Eq (35)) assures the exponential stability of the error origin, denoted as (ep,ez)=(0,0), which consequently leads to y(t) converging to ym(t) as t approaches infinity. This guarantee holds true when there are symmetric positive definite matrices P and Q, along with a positive scalar ε, meeting the following conditions:
[PA+ATP+2γPBBTP+εI−γPBBTP−γPBBTPQX+XTQ+εI]<0, | (36) |
where X is the matrix such that X=A−LC, with L representing the observer gain matrix to be determined prior to solving Eq (35). Additionally, I stands for the identity matrix, and γ is a design scalar.
Proof. When employing control law (34), the rate of change of the error, e(t), is diminished to:
Tα,ψe(t)=Ae(t)+γBBTP(e(t)−ez(t)). | (37) |
Let's introduce the Lyapunov function candidate as V=epTPep+ezTQez. At any time t greater than or equal to t0, the evolution of the Lyapunov function's derivative is determined by:
Tα,ψV(t)=2eTPTα,ψe+2ezTQTα,ψez |
=eTPCDαt0,te+Tα,ψeTPe+ezTQTα,ψez+Tα,ψezTQez |
≤eTP[Ae+γBBTP(e−ez)]+[Ae+γBBTP(e−ez)]TPe |
+ezTQ(A−LC)ez+ezT(A−LC)TQez, |
Tα,ψV(t)≤eT[PA+ATP+2γPBBTP]e+ezT(QX+XTQ)ez−2γeTPBBTPez, |
≤[eez]T[PA+ATP+2γPBBTP−γPBBTP−γPBBTPQX+XTQ][eez] |
≤[eez]TΩ[epez]−ε||(eez)||2, |
where
Ω=[PA+ATP+2γPBBTP+εI−γPBBTP−γPBBTPQX+XTQ+εI]. |
Drawing from Eq (10), we deduce that Tα,ψV(t) exhibits the property of being less than or equal to -ε multiplied by the norm of the vector formed by the elements of e and ez, squared (≤−ε||(eez)||2). Consequently, it is established that the error origin, represented as (ep,ez)=(0,0), is exponentially stable. Moving forward, based on Eq (28), we can affirm that x(t)−Fxm(t) converges to zero as t approaches infinity. Furthermore, Cx(t)−CFxm(t) follows the same trajectory and tends to zero as t heads toward infinity. Notably, it's worth mentioning that CF equals Cm, as indicated by the relationship in Eq (28). In conclusion, we ascertain that y(t) converges to ym(t) as time extends to infinity. With this, we have successfully completed the proof.
Remark 7.2. When α takes on a value of 1, corresponding to classical integer-order systems, the theorem we've established retains its validity. In this scenario, the derived result reverts to the classical notion of exponential stability. It's important to highlight that when dealing with the Caputo fractional order derivative, similar findings have been established in previous work.
In this subsection, we considered a renewable energy system in order to evaluate the developed control strategy based on the Lyapunov technique, which corresponds to a DC-DC converter connected to a PV panel. The purpose of this simulation study is to assess the effectiveness and the efficiency of the proposed control strategy in achieving desired performance objectives and to appraise its robustness under different operating conditions. In this discussion section, we will discuss the simulation setup, compare the simulation results for two cases, and provide insights into the advantages and limitations.
The following figure (Figure 10) represents the block diagram of the considered renewable energy process in a closed-loop.
Some simulation results are introduced hereafter. The following figure (Figure 11) illustrates the evolution curves of the outputs voltage signals for two scenarios: The first case corresponds to the ordinary case where the order derivative is α = 1 or the second one is relative to α = 0, 5.
It can be remarked that the evolution curves of the output voltage for the two scenarios converge to the reference signal within a fraction of seconds. We add that the output voltage signal of the second case, as compared to the first one, is a faster response with a smaller overshoot, and this is due to the decrease of the order derivative of the controller based on the Lyapunov technique.
In conclusion, the simulation findings of the controller, which is applied to a DC-DC converter connected to a PV panel, provides valuable insights into the performance and robustness of the control strategy under various operating conditions. The comparative analysis enables the selection of the most suitable controller based on specific control objectives, performance metrics, and operating conditions. It helps in refining the appropriate controller, optimizing its parameters, and assessing its suitability for real-world implementation.
Modeling and simulation of complex systems can be challenging for non-power electronics specialists who need to model and simulate static converters. Models of DC-DC converters must be precise and accurate for use in simulations of renewable energy systems. This paper provides a straightforward and accurate method for modeling a boost type converter and a Buck/Boost type converter using GCD. In fact, researchers have recently described GCD and its properties. Compared to fractional derivative definitions, this derivative generalizes the conformable derivative definition and uses the same derivation formulas. Using this unique derivative, we obtain a new class of differential equations for DC-DC static converters. The use of GCD to represent DC-DC static converters has been shown to be more flexible and profitable than the conventional conformable derivative. This progress opens up opportunities for the development of more efficient control methods using the Lyapunov technique, with practical implementations not only in PV systems but also in various other domains. As a perspective, we will compare the developed theoretical results with experimental ones in order to determine the adequate value of the derivative order that should be closer to physical reality.
The authors declare they have not used Artificial Intelligence (AI) tools in the creation of this article.
This research has been funded by Deputy for Research & Innovation, Ministry of Education through Initiative of Institutional Funding at University of Ha'il-Saudi Arabia through project number IFP-22 121.
All the authors declare that there are no potential conflicts of interest and approval of the submission.
[1] | K. Amei, Y. Takayasu, T. Ohji, M. Sakui, A maximum power control of wind generator system using a permanent magnet synchronous generator and a boost chopper circuit, In: Proceedings of the power conversion conference-Osaka 2002, 3 (2002), 1447–1452. https://doi.org/10.1109/PCC.2002.9981866. |
[2] | K. Ohyama, S. Arinaga, Y. Yamashita, Modelling and simulation of variable speed wind generator system using boost converter of permanent magnet synchronous generator, In: 2007 European conference on power electronics and applications, 2007, 1–9. https://doi.org/10.1109/EPE.2007.4417541 |
[3] | M. A. Zdiri, B. Bouzidi, O. Kahouli, H. H. Abdallah, Fault detection method for boost converters in solar PV systems, In: 2019 19th International conference on sciences and techniques of automatic control and computer engineering (STA), 2019,237–242. https://doi.org/10.1109/STA.2019.8717239 |
[4] |
B. Dhouib, Z. Alaas, O. Kahouli, H. H. Abdallah, Determination of optimal location of FACTS device to improve integration rate of wind energy in presence of MBPSS regulator, IET Renew. Power Gen., 14 (2020), 3526–3540. https://doi.org/10.1049/iet-rpg.2020.0679 doi: 10.1049/iet-rpg.2020.0679
![]() |
[5] | J. P. Ferrieux, F. Forest, Alimentations à découpage convertisseurs à resonance: Principes composants, modélisation, 3 Eds., Dunod, 2002. |
[6] |
V. Martynyuk, M. Ortigueira, Fractional model of an electrochemical capacitor, Signal Process., 107 (2015), 355–360. https://doi.org/10.1016/j.sigpro.2014.02.021 doi: 10.1016/j.sigpro.2014.02.021
![]() |
[7] |
A. Atangana, D. Baleanu, New fractional derivatives with nonlocal and non-singular kernel: Theory and application to heat transfer model, J. Thermal Sci., 20 (2016), 763–769. http://dx.doi.org/10.2298/TSCI160111018A doi: 10.2298/TSCI160111018A
![]() |
[8] |
Y. Wei, Lyapunov stability theory for nonlinear Nabla fractional order systems, IEEE Trans. Circuits Syst. II, 68 (2021), 3246–3250. http://doi.org/10.1109/TCSII.2021.3063914 doi: 10.1109/TCSII.2021.3063914
![]() |
[9] |
A. Razzaghian, A fuzzy neural network-based fractional-order Lyapunov-based robust control strategy for exoskeleton robots: Application in upper-limb rehabilitation, Math. Comput. Simul., 193 (2022), 567–583. https://doi.org/10.1016/j.matcom.2021.10.022 doi: 10.1016/j.matcom.2021.10.022
![]() |
[10] |
R. Peng, C. Jiang, R. Guo, Stabilization of a class of fractional order systems with both uncertainty and disturbance, IEEE Access, 9 (2021), 42697–42706. https://doi.org/10.1109/ACCESS.2021.3060093 doi: 10.1109/ACCESS.2021.3060093
![]() |
[11] |
F. Du, J. G. Lu, New results on finite-time stability of fractional-order Cohen-Grossberg neural networks with time delays, Asian J. Control, 24 (2022), 2328–2337. https://doi.org/10.1002/asjc.2641 doi: 10.1002/asjc.2641
![]() |
[12] |
R. Khalil, M. Al Horani, A. Yousef, M. Sababheh, A new definition of fractional derivative, J. Comput. Appl. Math., 264 (2014), 65–70. https://doi.org/10.1016/j.cam.2014.01.002 doi: 10.1016/j.cam.2014.01.002
![]() |
[13] |
S. M. Abo-Dahab, A. E. Abouelregal, M. Marin, Generalized thermoelastic functionally graded on a thin slim strip non-gaussian laser beam, Symmetry, 12 (2020), 1094. https://doi.org/10.3390/sym12071094 doi: 10.3390/sym12071094
![]() |
[14] | M. I. A. Othman, M. Fekry, M. Marin, Plane waves in generalized magneto-thermo-viscoelastic medium with voids under the effect of initial stress and laser pulse heating, Struct. Eng. Mech., 73 (2020), 621–629. |
[15] |
N. Sene, G. Srivastava, Generalized Mittag-Leffler input stability of the fractional differential equations, Symmetry, 11 (2019), 608. https://doi.org/10.3390/sym11050608 doi: 10.3390/sym11050608
![]() |
[16] |
O. Naifar, A. Jmal, A. B. Makhlouf, Non-fragile H∞ observer for Lipschitz conformable fractional-order systems, Asian J. Control, 24 (2021), 2202–2212. https://doi.org/10.1002/asjc.2626 doi: 10.1002/asjc.2626
![]() |
[17] |
M. Abu-Shady, M. K. A. Kaabar, A generalized definition of the fractional derivative with applications, Math. Probl. Eng., 2021 (2021), 9444803. https://doi.org/10.1155/2021/9444803 doi: 10.1155/2021/9444803
![]() |
[18] |
A. Jmal, M. Elloumi, O. Naifar, A. B. Makhlouf, M. A. Hammami, State estimation for nonlinear conformable fractional-order systems: A healthy operating case and a faulty operating case, Asian J. Control, 22 (2020), 1870–1879. https://doi.org/10.1002/asjc.2122 doi: 10.1002/asjc.2122
![]() |
[19] | O. Naifar, A. B. Makhlouf, Fractional order systems-control theory and applications, Springer Cham, 2022. https://doi.org/10.1007/978-3-030-71446-8 |
[20] |
F. Gomez, J. Rosales, M. Guia, RLC electrical circuit of non-integer order, Cent. Eur. J. Phys., 11 (2013), 1361–1365. https://doi.org/10.2478/s11534-013-0265-6 doi: 10.2478/s11534-013-0265-6
![]() |
[21] |
O. Kahouli, M. Elloumi, O. Naifar, H. Alsaif, B. Kahouli, Y. Bouteraa, Electrical circuits described by general fractional conformable derivative, Front. Energy Res., 10 (2022). https://doi.org/10.3389/fenrg.2022.851070 doi: 10.3389/fenrg.2022.851070
![]() |
[22] |
T. T. Hartley, R. J. Veillette, J. L. Adams, C. F. Lorenzo, Energy storage and loss in fractional-order circuit elements, IET Circ. Device. Syst., 9 (2015), 227–235. https://doi.org/10.1049/iet-cds.2014.0132 doi: 10.1049/iet-cds.2014.0132
![]() |
[23] | V. Lazarov, D. Roye, Z. Zarkov, D. Spirov, Analysis of DC converters for wind generators, In: XVIth international symposium on electrical apparatus and technologies, 2 (2009), 157–164. https://dx.doi.org/10.2298/fuee0902235l |
[24] | J. J. Rosales, J. F. Gomez, M. Guía, V. I. Tkach, Fractional electromagnetic waves (LFNM), In: 2011 11th International conference on laser and fiber-optical networks modeling (LFNM), Kharkov, 2011, 1–3. https://doi.org/10.1109/LFNM.2011.6144969 |
[25] |
R. B. Salah, O. Kahouli, H. Hadjabdallah, A nonlinear Takagi-Sugeno fuzzy logic control for single machine power system, Int. J. Adv. Manuf. Technol., 90 (2017), 575–590. https://doi.org/10.1007/s00170-016-9351-4 doi: 10.1007/s00170-016-9351-4
![]() |
[26] |
D. Zhao, M. Luo, General conformable fractional derivative and its physical interpretation, Calcolo, 54 (2015), 903–917. https://doi.org/10.1007/s10092-017-0213-8 doi: 10.1007/s10092-017-0213-8
![]() |
[27] |
S. Li, S. Zhang, R. Liu, The existence of solution of diffusion equation with the general conformable derivative, J. Funct. Spaces, 2020 (2020), 3965269. https://doi.org/10.1155/2020/3965269 doi: 10.1155/2020/3965269
![]() |
[28] | S. G. Samko, A. A. Kilbas, O. I. Marichev, Fractional integrals and derivatives: Theory and applications, New York: Gordon and Breach Publishers, 1993. |
[29] | I. Podlubny, Fractional differential equations, Elsevier, 198 (1999), 1–340. |
[30] | R. Hermann, Fractional calculus, World Scientific Publishing Company, 2011. |
[31] |
Z. Lu, Y. Zhu, Q. Xu, Asymptotic stability of fractional neutral stochastic systems with variable delays, Eur. J. Control, 57 (2021), 119–124. https://doi.org/10.1016/j.ejcon.2020.05.005 doi: 10.1016/j.ejcon.2020.05.005
![]() |
[32] |
L. Martínez, J. J. Rosales, C. A. Carreño, J. M. Lozano, Electrical circuits described by fractional conformable derivative, Int. J. Circuit Theory Appl., 46 (2018), 1091–1100. https://doi.org/10.1002/cta.2475 doi: 10.1002/cta.2475
![]() |
[33] |
H. Gassara, O. Naifar, A. B. Makhlouf, L. Mchiri, Global practical conformable stabilization by output feedback for a class of nonlinear fractional-order systems, Math. Probl. Eng., 2022 (2022), 4920540. https://doi.org/10.1155/2022/4920540 doi: 10.1155/2022/4920540
![]() |
1. | Prabir Ranjan Kasari, Subhadeep Bhattacharjee, An Enhanced Cascaded Five-Level Inverter Based on Switched Capacitors and Single dc Source, 2024, 2193-567X, 10.1007/s13369-024-09544-y | |
2. | O. A. Ángeles‐Ramírez, S. González‐Figueroa, G. Fernández‐Anaya, E. G. Martínez‐Hernández, J. González‐Sierra, M. Ramírez‐Neria, Decentralized formation with collision avoidance of multi‐agent conformable fractional nonlinear robot systems, 2025, 1561-8625, 10.1002/asjc.3580 | |
3. | Malek Laajimi, Hamdi Gassara, Mohamed Rhaima, Lassaad Mchiri, Abdellatif Ben Makhlouf, H$$_\infty $$ Control for General Conformable Polynomial Fuzzy Models, 2025, 1562-2479, 10.1007/s40815-025-01983-5 |