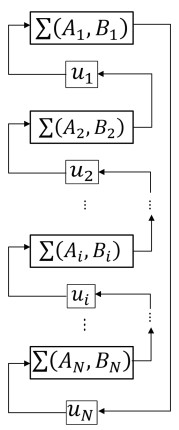
This paper investigates the cooperative state feedback control problem for delta operator-based large-scale systems with independent subsystems. First, the state feedback controller is introduced to interconnect the adjacent subsystems into a closed-loop system. Second, the Lyapunov function in delta domain is constructed, and the linear matrix inequality method is used to design the cooperative state feedback stability controller for the whole large-scale interconnected system. Third, a performance index is introduced for the design of the optimal cooperative state feedback controller. Finally, stability of the closed-loop system is proved on the basis of stability theory, and simulation examples are given for showing the effectiveness of the design method.
Citation: Yanmei Xue, Jinke Han, Ziqiang Tu, Xiangyong Chen. Stability analysis and design of cooperative control for linear delta operator system[J]. AIMS Mathematics, 2023, 8(6): 12671-12693. doi: 10.3934/math.2023637
[1] | Teekam Singh, Ramu Dubey, Vishnu Narayan Mishra . Spatial dynamics of predator-prey system with hunting cooperation in predators and type I functional response. AIMS Mathematics, 2020, 5(1): 673-684. doi: 10.3934/math.2020045 |
[2] | Zhaohui Chen, Jie Tan, Yong He, Zhong Cao . Decentralized observer-based event-triggered control for an interconnected fractional-order system with stochastic Cyber-attacks. AIMS Mathematics, 2024, 9(1): 1861-1876. doi: 10.3934/math.2024091 |
[3] | Nasser. A. Saeed, Amal Ashour, Lei Hou, Jan Awrejcewicz, Faisal Z. Duraihem . Time-delayed control of a nonlinear self-excited structure driven by simultaneous primary and 1:1 internal resonance: analytical and numerical investigation. AIMS Mathematics, 2024, 9(10): 27627-27663. doi: 10.3934/math.20241342 |
[4] | Gaoran Wang, Weiwei Sun, Shuqing Wang . Stabilization of wind farm integrated transmission system with input delay. AIMS Mathematics, 2021, 6(9): 9177-9193. doi: 10.3934/math.2021533 |
[5] | Ridha Mdimagh, Fadhel Jday . Spectral stability analysis of the Dirichlet-to-Neumann map for fractional diffusion equations with a reaction coefficient. AIMS Mathematics, 2024, 9(3): 5394-5406. doi: 10.3934/math.2024260 |
[6] | Xuelian Jin . Exponential stability analysis and control design for nonlinear system with time-varying delay. AIMS Mathematics, 2021, 6(1): 102-113. doi: 10.3934/math.2021008 |
[7] | Nazmul Sk, Bapin Mondal, Abhijit Sarkar, Shyam Sundar Santra, Dumitru Baleanu, Mohamed Altanji . Chaos emergence and dissipation in a three-species food web model with intraguild predation and cooperative hunting. AIMS Mathematics, 2024, 9(1): 1023-1045. doi: 10.3934/math.2024051 |
[8] | Fang Zhu, Pengtong Li . Adaptive fuzzy consensus tracking control of multi-agent systems with predefined time. AIMS Mathematics, 2025, 10(3): 5307-5331. doi: 10.3934/math.2025245 |
[9] | Weili Kong, Yuanfu Shao . Bifurcations of a Leslie-Gower predator-prey model with fear, strong Allee effect and hunting cooperation. AIMS Mathematics, 2024, 9(11): 31607-31635. doi: 10.3934/math.20241520 |
[10] | Haowen Gong, Huijun Xiang, Yifei Wang, Huaijin Gao, Xinzhu Meng . Strategy evolution of a novel cooperative game model induced by reward feedback and a time delay. AIMS Mathematics, 2024, 9(11): 33161-33184. doi: 10.3934/math.20241583 |
This paper investigates the cooperative state feedback control problem for delta operator-based large-scale systems with independent subsystems. First, the state feedback controller is introduced to interconnect the adjacent subsystems into a closed-loop system. Second, the Lyapunov function in delta domain is constructed, and the linear matrix inequality method is used to design the cooperative state feedback stability controller for the whole large-scale interconnected system. Third, a performance index is introduced for the design of the optimal cooperative state feedback controller. Finally, stability of the closed-loop system is proved on the basis of stability theory, and simulation examples are given for showing the effectiveness of the design method.
There exist complex large-scale interconnected systems in many control applications, such as networked control systems, power systems, economic systems and so on, they are usually connected by many subsystems and their model structures are complex and robust. Since the 21st century, the problem of large-scale interconnected system has attracted more and more attention from scholars [1,2,3,4,5,6,7,8,9,10,11,12], including adaptive identification [1], state estimation [2], asynchronous control [3], fault detection [4], longitudinal and laternal control [5], decentralized control [6,7,8,9,10,11,12], adaptive tracking control [13,14,15,16], and so on. Among them, how to improve the stability of large-scale complex systems has become an increasingly important and concerned topic in the research of dynamical systems.
In traditional stability analysis and control synthesis of large-scale interconnected systems, it needs very conservative requirement that each subsystems are stable. And some examples are given to verify that the subsystems are unstable to make the large-scale system reach a stable equilibrium state via interconnection control actions [17,18]. In [17], the appropriate interconnection and cooperative feedback controllers are used to make the unstable subsystems into a stable large-scale system. In [18], the algorithms for the interconnected stability and cooperative stabilization of two unstable subsystems are given by using the technique of the bilinear matrix inequality. Up to now, research on interconnected and cooperative control has made many achievements [19,20,21,22,23,24,25]. A perturbed cooperative control method is proposed for interconnected systems exchanging data via the communication network in [21]. In [22,23], the design of event-triggered cooperative stability controller of multi-agent systems with interconnected dynamics are presented. In [24], a novel decentralized adaptive control algorithm considering discontinuity caused by state-triggering is shown for uncertain interconnected systems. And a class of cooperative control approach for the constrained interconnected nonlinear systems is proposed in [25].
In recent years, the advantages of the delta operator system in fast sampling make it widely used in industrial automation, power network, computer communication and other fields [26,27,28,29,30,31]. And some good sliding mode control (SMC) methods by utilizing its robust property have been proposed for delta operator system [32,33,34]. In [32], two kinds of dynamical SMC design methods are explored, and sufficient conditions for the asymptotic stability of delta operator systems are obtained. In [33], a SMC law for the adaptive control of Markovian jump systems under delta operator framework is proposed. A novel quantized SMC method in delta operator system is developed for dealing with the quantized state feedback problem in [34].
However, there are few researches on the cooperative control problem of delta operator system. In [35], for a delta operator based large-scale system composed of linear subsystems, a stable cooperative state feedback controller is given by constructing the Lyapunov function in the delta domain and using the linear matrix inequality (LMI) technique. However, the design method here can only be used in the case of two indpendent subsystems, which limits the application of the method in actual engineering. Motivated by above mentioned results, this paper mainly investigates the cooperative control design for the large-scale interconnected systems with N independent subsystems in delta operator framework.
The main contribution of this paper is summarized twofold. Sufficient conditions for the asymptotic stability of large-scale interconnected system via cooperative state feedback are derived in the delta operator framework. And the optimal cooperative control with performance index problem is further investigated. Both theoretical analysis and examples show that the designed methods have better performance.
The following description is given for N independent subsystems under the delta operator framework:
δ(xi(tk))=Aixi(tk)+Biui(tk),i=1,2,⋯,N | (2.1) |
where xi(tk)∈Rni, ui(tk)∈Rmi represent the state variables and the control input variables of the subsystem i. The constant value matrices Ai ∈Rni×ni and Bi∈Rni×mi are known to represent the system matrix and control input matrix of the subsystem i, respectively.
Definition 1. (Yang et al. [26]) Let tk=kT, delta operator δ(⋅) is defined as follows:
δ(v(tk))={dv(t)dt,T=0v(tk+T)−v(tk)T,T≠0 |
where v(tk) is the variable, T represents a high-speed sampling period.
Lemma 1. (Yang et al. [26], Zheng et al. [36]) For any function x(t) and y(t),
δ(x(t)y(t))=δ(x(t))y(t)+x(t)δ(y(t))+Tδ(x(t))δ(y(t)). |
The N independent subsystems (2.1) are interconnected, and the state feedback controllers are designed as shown in Eq (2.2):
{ui(tk)=Kixi+1(tk), i=1,2,⋯,N−1uN(tk)=KNx1(tk) | (2.2) |
where Ki∈Rmi×ni+1(i=1,2,⋯,N−1) and KN∈RmN×n1 are the state feedback gain matrices with appropriate dimensions.
The schematic of the interconnected system with cooperative state feedback controller (2.2) is shown in Figure 1.
One can see from the schematic of the system that there are information feedback from one subsystem to another, and thus it forms a whole large-scale interconnected system. In the existing literature, most of them consider that two subsystems cooperate as one interconnected system, such as [17,18,19,20]. Here, the cooperative control of large-scale system with N subsystems is considered.
By the combination of the subsystems (2.1) and the state feedback controllers (2.2), one can get the large-scale interconnected closed-loop system as follows:
δ(x(tk))=Ax(tk) | (2.3) |
where
A=[A1B1K1⋯00A2⋯0⋮⋮⋱⋮BNKN0⋯AN], | (2.4) |
and
x(tk)=[xT1(tk),xT2(tk),⋯,xTN(tk)]T. |
Definition 2. (Duan et al. [17]) The system (2.1) is said to be cooperatively stable if there exist cooperative controllers (2.2) such that the closed-loop system (2.3) is asymptotically stable. To optimize the performance of the designed controller, a performance index J is introduced:
J=T∞∑k=0[xT(tk)Qx(tk)+uT1(tk)C1u1(tk)+uT2(tk)C2u2(tk)+⋯+uTN(tk)CNuN(tk)]=T∞∑k=0xT(tk)(Q+KTCK)x(tk) | (2.5) |
where Q∈R(n1+n2+⋯+nN)×(n1+n2+⋯+nN) represents the positive definite matrix to be designed, Ci (i=1,2,⋯,N) represents a positive definite known constant matrix, and C=blockdiag[CN,C1,⋯,CN−1], K=blockdiag[KN,K1,⋯,KN−1].
Remark 1. Performance index (2.5) is commonly used in control system analysis and design. For example, for discrete-time systems with state and input quantizations, the robust guaranteed performance control design is investigated in [37]. In [38], robust stability analysis of guaranteed performance control of impulsive switched systems is well studied. In addition, cooperative control of interconnected systems with performance requirements is presented in [17,18,20,35].
Definition 3. (Duan et al. [17]) The systems (2.1) are said to be cooperatively stable and satisfy the performance J if there exist cooperative controllers (2.2) such that the closed-loop system (2.3) is asymptotically stable and satisfy the performance index (2.5).
It can be seen from the above statement that the design of cooperative feedback control stability method is to solve the asymptotic stability problem of interconnected systems (2.3). The design of cooperative feedback optimal control method is to solve the asymptotic stability of driving the state to zero problem of interconnected systems (2.3) that satisfy the performance index (2.5).
Theorem 1. For the interconnected closed-loop system (2.3), if there exist symmetric positive definite matrices Xii, Wii, Xij, Wij,i≠j, and general matrices Yij, i,j=1,2,⋯,N satisfy the following conditions:
X=[X11…X1N⋮⋱⋮∗…XNN]>0 |
W=[W11…W1N⋮⋱⋮∗…WNN]>0 |
Σ=[Σ11Σ12Σ13∗Σ220∗∗Σ33]<0 | (3.1) |
where
Σ11=[−2W11…−2W1N⋮⋱⋮∗…−2WNN] |
Σ12=[A1X11+B1Y21⋯A1X1N+B1Y2N⋮⋱⋮ANXT1N+BNY11⋯ANXNN+BNY1N] |
Σ13=[TW11…TW1N⋮⋱⋮TWN1…TWNN] |
Σ22=Σ12+ΣT12 |
Σ33=[−TX11…−TX1N⋮⋱⋮∗…−TXNN] |
Then the linear system (2.1) is said to be cooperatively stable, and the gains of the cooperative controllers can be obtained by
{Ki=Y(i+1)(i+1)X−1(i+1)(i+1), i=1,2,...,N−1KN=Y11X−111 | (3.2) |
Proof. Taking
V(x(tk))=xT(tk)Px(tk) | (3.3) |
According to Lemma 1 and the system (2.3), one can see that
δV(x(tk))=xT(tk)ATPx(tk)+xT(tk)PAx(tk)+TδT(x(tk))Pδ(x(tk)) | (3.4) |
Noting system Eq (2.3), we can get that A(x(tk))−δ(x(tk))=0. And for any positive definite matrix ˆW>0, we have
0=δT(x(tk))ˆW(A(x(tk))−δ(x(tk))) | (3.5) |
Substituting (3.5) into (3.4), we can get
δV(x(tk))=ξT(tk)Σ1ξ(tk) | (3.6) |
where Σ1=[TP−2ˆWˆWA∗ATP+PA] and ξ(tk)=[δT(x(tk))xT(tk)]T. Then the inequality δV(x(tk))<0 holds if and only if
Σ1<0 | (3.7) |
By multiplying positive definite matrix blockdiag[ˆW−1P−1] on both sides of (3.7), we get
[ˆW−1TPˆW−1−2ˆW−1AP−1∗P−1AT+AP−1]<0 | (3.8) |
Applying Schur Complement Lemma [39], one can get that
[−2ˆW−1AP−1TˆW−1∗P−1AT+AP−10∗∗−TP−1]<0 |
Let P−1=X and W−1=ˆW, we have
Σ=[−2WAXTW∗He(AX)0∗∗−TX]<0 | (3.9) |
where the notation He(X) represents the sum of X and its transpose. Combining (3.2) and (3.9), one can see that
Σ=[Σ11Σ12Σ13∗Σ220∗∗Σ33]<0 |
So Σ1<0.
It follows from Definition 1 that
δV(x(tk))=V(x(tk+T))−V(x(tk))T=1T[xT(tk+T)Px(tk+T)−xT(tk)Px(tk)]<0 |
Then
xT(tk+T)Px(tk+T)−xT(tk)Px(tk)<0 | (3.10) |
if x(tk)≠0. Since P is positive definite, then x(tk)→0 when k→∞.
Therefore, the closed-loop system (2.3) is asymptotically stable and the system (2.1) is cooperatively stable if the LMI Σ<0 holds. This completes Theorem 1.
Theorem 2. For the large-scale interconnected closed-loop system (2.3), if there exist symmetric positive definite matrices Xii, Wii, Zii, and matrices Xij(i≠j), Wij, Zij(i≠j), i,j=1,2,⋯,N such that
X=[X11…X1N⋮⋱⋮∗…XNN]>0 |
W=[W11…W1N⋮⋱⋮∗…WNN]>0 |
Z=[Z11…Z1N⋮⋱⋮∗…ZNN]>0 |
Π=[Π11Π12Π1300∗Π220Π24Π25∗∗Π3300∗∗∗Π440∗∗∗∗Π55]<0 | (3.11) |
where
Π11=[−2W11…−2W1N⋮⋱⋮∗…−2WNN], |
Π13=[TW11…TW1N⋮⋱⋮TWT1N…TWNN], |
Π12=[A1X11+B1Y21⋯A1X1N+B1Y2N⋮⋱⋮ANXT1N+BNY11⋯ANXNN+BNY1N], |
Π22=Π12+ΠT12, |
Π33=[−TX11…−TX1N⋮⋱⋮∗…−TXNN], |
Π24=[XT11…XT1N⋮⋱⋮XTN1…XTNN], |
Π25=[YT11⋯YTN1⋮⋱⋮YT1N⋯YTNN], |
Π44=[−Z11…−Z1N⋮⋱⋮∗…−ZNN], |
Π55=[−C−1N⋯0⋮⋱⋮∗⋯−C−1N−1]. |
Then the linear system (2.1) is said to be cooperatively stable, and the cooperative state feedback controllers are taken as
{ui(tk)=Y(i+1)(i+1)X−1(i+1)(i+1)xi+1(tk),i=1,⋯,N−1uN(tk)=Y11X−111x1(tk) | (3.12) |
and the performance index (2.5) satisfies
J≤xT(t0)Px(t0) | (3.13) |
Proof. We also take
V(x(tk))=xT(tk)Px(tk) |
And suppose that the matrix inequality
δV(x(tk))+xT(tk)(Q+KTCK)x(tk)<0 | (3.14) |
holds.
Substituting (3.14) into (3.5), one can obtain that
δV(x(tk))+xT(tk)(Q+KTCK)x(tk)=xT(tk)(He(ATP)+Q+KTCK)x(tk)+δT(x(tk))(TP−2ˆW)δ(x(tk))+δT(x(tk))ˆWAx(tk)+xT(tk)ATˆWδ(x(tk))=ξT(tk)Π1ξ(tk)<0 | (3.15) |
where He(ATP)=ATP+PA, ξ(tk)=[δT(x(tk))xT(tk)]T and Π1=[TP−2ˆWˆWA∗ATP+PA+Q+KTCK].
Then the inequality (3.15) holds if and only if
Π1<0 | (3.16) |
Multiplying the positive definite matrix blockdiag[ˆW−1P−1] on both sides of (3.16), we get
[ˆW−1TPˆW−1−2ˆW−1AP−1∗Ω]<0 | (3.17) |
where Ω=He(AP−1)+P−1QP−1+P−1KTCKP−1.
Applying Schur Complement Lemma, and let ˆW−1=W, P−1=X, we have
Π=[−2WAXTW00∗He(AX)0XTYT∗∗−TX00∗∗∗−Q−10∗∗∗∗−C−1]<0 | (3.18) |
It follows from (3.2) and (3.9) that
Π=[Π11Π12Π1300∗Π220Π24Π25∗∗Π3300∗∗∗Π440∗∗∗∗Π55]<0 | (3.19) |
Then the inequality Π<0 holds if and only if the inequality Π1<0 holds.
According to Definition 1 and formula (3.14), one can obtain that
T∞∑k=0xT(tk)(Q+KTCK)x(tk)≤V(x(t0)) |
Further
J≤xT(t0)Px(t0) |
This completes Theorem 2.
The above two theorems design methods of the cooperative state feedback control of large-scale interconnected system (2.1), where Theorem 1 gives the feasible solution of the cooperative controller design, and Theorem 2 presents the cooperative controller design with performance index (2.5). The upper bound condition of the optimization performance index J for large-scale interconnected close-loop system (2.1) is given in Theorem 3.
Theorem 3. For delta operator based linear system (2.1), if the following LMI-based optimization problems
minγ>0 |
s.t. (i)X=[X11…X1N⋮⋱⋮∗…XNN]>0 |
(ii)W=[W11…W1N⋮⋱⋮∗…WNN]>0 |
(iii)Z=[Z11…Z1N⋮⋱⋮∗…ZNN]>0 |
(iv)Π=[Π11Π12Π1300∗Π220Π24Π25∗∗Π3300∗∗∗Π440∗∗∗∗Π55]<0 |
(v)[−γxT1(0)⋯xTN(0)∗−X11⋯−X1N⋮⋮⋱⋮∗∗⋯−XNN]<0 |
can be solvable, then the controller defined as (3.12) is the cooperative state feedback guaranteed cost controller with the performance J≤xT(t0)Px(t0)<γ.
Proof. Let J≤xT(t0)Px(t0)<γ. The matrix −X<0 can be known from condition (i). Using Schur complement Lemma 2 for the condition (v), we can get
−γ−xT(t0)(−X)−1x(t0)<0 |
Since P−1=X, we have
−γ+xT(t0)Px(t0)<0 |
Then
J≤xT(t0)Px(t0)<γ |
This completes Theorem 3.
To illustrate the effectiveness and superiority of the design method in this paper, the comparions and discussions with the existing method and open loop system are presented in this section.
Example 1. Three independent subsystems described by delta operator framework are considered. Choosing the sampling period T=0.05, the initial state value x1(0)=x2(0)=x3(0)=[0.5−0.5]T. Other parameters are presented as follows:
A1=[−0.00550.0050.0025−0.005],A2=[−0.050−0.05−0.05],A3=[−0.0050.0150−0.005], |
B1=[0.010.050.010.07],B2=[0.010.050.030.01],B3=[0.010.070.070.01]. |
Applying the existing cooperative control design method in [35], one can find that both the feasible solution algorithm in Theorem 1 and the optimal solution algorithm in Theorem 2 there have no solution.
Applying the proposed cooperative control design method in this paper, one can get a set of feasible solutions K1, K2, K3 according to Theorem 1.
K1=[16.3636−2.1197−2.15911.5437], |
K2=[3.2301−0.50710.21662.2275], |
K3=[1.00640.49120.27411.2461]. |
Further, one can get a set of optimal solutions K1, K2, K3 via the design method in Theorem 3.
K1=[−0.0678−1.32253.50193.3100], |
K2=[0.8520−0.47580.07340.8885], |
K3=[0.5000−0.09060.09060.5000]. |
At the same time, the optimized performance index J=5.0068 is obtained.
The simulation results are presented in Figures 2-10 (Performed on Matlab R2021b under LENOVO Yoga Pro14c, intel i7, Windows 10).
The open loop state response curves of the three subsystems are shown in Figures 2-4. It can be easily observed that none of the three subsystems is stable with good convergence performance.
Figures 5-10 show the state response and control input simulation results of the three subsystems under the proposed cooperative control algorithms. It can be seen from the state response curves 5-7 that both the proposed feasible solution and optimal solution methods can guarantee the interconnected closed-loop systems are stable. Among them, all the three subsystems can converge to the desired origin in 0.2 seconds using the proposed feasible solution method. While applying the proposed optimal solution method, subsystem 1 can converge to zero in 0.1 seconds and subsystem 2 and subsystem 3 can converge to zero in less than 0.2 seconds, respectively. Furthermore, combined with the control input response curves 8-10, it can be seen under the proposed optimal solution algorithm that the large-scale interconnected systems can achieve faster convergence speed with lower control input cost, i.e., lower control input amplitude.
Example 2. Another three independent subsystems described by delta operator framework are considered here. Choosing the sampling period T=0.001, the initial state values x1(0)=[0.2−0.1]T, x2(0)=[0.10.5]T, x3(0)=[−0.50.5]T. Other parameters are presented as follows:
A1=[−0.01650.0150.0075−0.015],A2=[−0.10−0.1−0.1],A3=[−0.0050.0150−0.005], |
B1=[0.010.030.010.05],B2=[0.020.050.030.06],B3=[0.020.070.040.01]. |
One can also find that both the feasible solution algorithm and the optimal solution algorithm in [35] have no solution. Now applying the proposed cooperative control design method in this paper, one can get a set of feasible solutions K1, K2, K3 according to Theorem 1.
K1=[20.1585−3.6572−3.22233.2373], |
K2=[24.4035−7.2248−9.72455.4192], |
K3=[1.65660.13750.11381.6238]. |
Further, one can get a set of optimal solutions K1, K2, K3 via the design method in Theorem 3.
K1=[2.58330.26540.45781.6416], |
K2=[1.77180.5237−0.56311.3065], |
K3=[0.6799−0.24180.20280.5021]. |
At the same time, the optimized performance index J=5 is obtained.
The simulation results are presented in Figures 11-16. Figures 11-16 show the state response and control input simulation results of the three subsystems under the proposed cooperative control algorithms. Similar to that in example 1, it also shows the effectiveness and advantage of the proposed method in this paper.
This paper discusses the cooperative control problem with N independent subsystems via delta operator, and gives the asymptotic stability control method and the optimal control method based on performance index of closed-loop system. Compared with the existing algorithms, this method has broader restrictions on the positive definite matrix in the design of collaborative feedback controller, and extends the system to general N subsystems, so that it can better describe the dynamic system in more actual engineerings. Simulation examples verify the feasibility and superiority of the two control methods designed in this paper. Generally, large-scale interconnected systems will suffer from unpredictable cyber attacks and uncertainties during actual operation process. However, the design of this paper does not consider its impact to system stability and performance. In the future, the design problem of security cooperative control for interconnected uncertain systems subject to cyber attacks and the practical applications of the designed methods will become the focuses of our research.
This research was funded by the National Natural Science Foundation of China (Grant No. 61973169), the Jiangsu Natural Science Foundation (Grant No. BK20201392).
The authors declared that they have no conflicts of interest to this work.
[1] |
J. Chen, Y. W. Mao, M. Gan, F. Ding, Adaptive regularised kernel-based identification method for large-scale systems with unknown order, Automatica, 143 (2022), 110437. https://doi.org/10.1016/j.automatica.2022.110437 doi: 10.1016/j.automatica.2022.110437
![]() |
[2] |
M. A. Chitsazan, M. S. Fadali, A. M. Trzynadlowski, State estimation for large-scale power systems and facts devices based on spanning tree maximum exponential absolute value, IEEE T. Power Syst., 35 (2020), 238–248. https://doi.org/10.1109/TPWRS.2019.2934705 doi: 10.1109/TPWRS.2019.2934705
![]() |
[3] |
J. J. Li, X. Tian, G. L. Wei, Asynchronous partially mode-dependent control for switched larger-scale nonlinear systems with bounded sojourn time, Appl. Math. Comput., 418 (2022), 126809. https://doi.org/10.1016/j.amc.2021.126809 doi: 10.1016/j.amc.2021.126809
![]() |
[4] |
Z. L. Ma, X. J. Li, Data-driven fault detection for large-scale network systems: a mixed optimization approach, Appl. Math. Comput., 426 (2022), 127134. https://doi.org/10.1016/j.amc.2022.127134 doi: 10.1016/j.amc.2022.127134
![]() |
[5] |
C. Latrach, A. Chaibet, M. Boukhnifer, S. Glaser, Integrated longitudinal and lateral networked control system design for vehicle platooning, Sensors, 18 (2018), 3085. https://doi.org/10.3390/s18093085 doi: 10.3390/s18093085
![]() |
[6] |
Y. M. Shao, X. L. Jia, X. X. Ju, X. C. Shi, Global decentralized control for uncertain large-scale feedforward nonlinear time-delay systems via output feedback, IMA J. Math. Control Inform., 39 (2022), 155–170. https://doi.org/10.1093/imamci/dnab035 doi: 10.1093/imamci/dnab035
![]() |
[7] |
Y. Zhu, E. Fridman, Observer-based decentralized predictor control for large-scale interconnected systems with large delays, IEEE T. Automat. Contr., 66 (2021), 2897–2904. https://doi.org/10.1109/TAC.2020.3011396 doi: 10.1109/TAC.2020.3011396
![]() |
[8] |
Y. Zhu, E. Fridman, Predictor methods for decentralized control of large-scale systems with input delays, Automatica, 116 (2020), 108903. https://doi.org/10.1016/j.automatica.2020.108903 doi: 10.1016/j.automatica.2020.108903
![]() |
[9] |
C. Latrach, M. Kchaou, H. Guéguen, H∞ observer-based decentralised fuzzy control design for nonlinear interconnected systems: an application to vehicle dynamics, Int. J. Syst. Sci., 48 (2017), 1485–1495. https://doi.org/10.1080/00207721.2016.1266527 doi: 10.1080/00207721.2016.1266527
![]() |
[10] |
Y. Yang, X. H. Li, X. P. Liu, Decentralized finite-time connective tracking control with prescribed settling time for p-normal form stochastic large-scale systems, Appl. Math. Comput., 412 (2022), 126581. https://doi.org/10.1016/j.amc.2021.126581 doi: 10.1016/j.amc.2021.126581
![]() |
[11] |
T. Wang, Y. P. Li, W. M. Xiang, Design of interval observer for continuous linear large-scale systems with disturbance attenuation, J. Franklin I., 359 (2022), 3910–3929. https://doi.org/10.1016/j.jfranklin.2022.03.014 doi: 10.1016/j.jfranklin.2022.03.014
![]() |
[12] |
T. Yu, J. L. Xiong, Distributed L2-gain control of large-scale systems: a space construction approach, ISA T., 116 (2021), 58–70. https://doi.org/10.1016/j.isatra.2021.01.025 doi: 10.1016/j.isatra.2021.01.025
![]() |
[13] |
H. Y. Yue, Z. Wei, Q. J. Chen, X. Y. Zhang, Dynamic surface control for a class of nonlinearly parameterized systems with input time delay using neural network, J. Franklin I., 357 (2020), 1961–1986. https://doi.org/10.1016/j.jfranklin.2019.10.034 doi: 10.1016/j.jfranklin.2019.10.034
![]() |
[14] |
H. Y. Yue, W. Yang, S. B. Li, S. Y. Jiang, Fuzzy adaptive tracking control for a class of nonlinearly parameterized systems with unknown control directions, Iran. J. Fuzzy Syst., 16 (2019), 97–112. https://doi.org/10.22111/IJFS.2019.4554 doi: 10.22111/IJFS.2019.4554
![]() |
[15] |
H. Y. Yue, J. R. Shi, L. Y. Du, X. J. Li, Adaptive fuzzy tracking control for a class of perturbed nonlinearly parameterized systems using minimal learning parameters algorithm, Iran. J. Fuzzy Syst., 15 (2018), 99–116. https://doi.org/10.22111/ijfs.2018.3952 doi: 10.22111/ijfs.2018.3952
![]() |
[16] |
H. Y. Yue, C. M. Gong, Adaptive tracking control for a class of stochastic nonlinearly parameterized systems with time-varying input delay using fuzzy logic systems, J. Low Freq. Noise, 41 (2022), 1192–1213. https://doi.org/10.1177/14613484211045761 doi: 10.1177/14613484211045761
![]() |
[17] |
Z. S. Duan, J. Z. Wang, L. Huang, Special decentralized control problems in discrete-time interconnected systems composed of two subsystems, Syst. Control Lett., 56 (2007), 206–214. https://doi.org/10.1016/j.sysconle.2006.09.002 doi: 10.1016/j.sysconle.2006.09.002
![]() |
[18] |
X. H. Nian, L. Cao, BMI approach to the interconnected stability and cooperative control of linear systems, Acta Mathematica Scientia, 34 (2008), 438–444. https://doi.org/10.3724/SP.J.1004.2008.00438 doi: 10.3724/SP.J.1004.2008.00438
![]() |
[19] |
Z. S. Duan, L. Huang, J. Z. Wang, L. Wang, Harmonic control between two systems, Acta Automatica Sinica, 2003 (2003), 14–29. https://doi.org/10.16383/j.aas.2003.01.003 doi: 10.16383/j.aas.2003.01.003
![]() |
[20] |
H. Zhao, D. Y. Chen, J. Hu, The interconnected stability and cooperative control for a class of uncertain time-delay systems, Electric Machines and Control, 14 (2010), 89–97. https://doi.org/10.15938/j.emc.2010.06.014 doi: 10.15938/j.emc.2010.06.014
![]() |
[21] |
T. Tran, Q. P. Ha, Perturbed cooperative-state feedback strategy for model predictive networked control of interconnected systems, ISA T., 72 (2018), 110–121. https://doi.org/10.1016/j.isatra.2017.09.017 doi: 10.1016/j.isatra.2017.09.017
![]() |
[22] |
V. Rezaei, M. Stefanovic, Event-triggered cooperative stabilization of multiagent systems with partially unknown interconnected dynamics, Automatica, 130 (2021), 109657. https://doi.org/10.1016/j.automatica.2021.109657 doi: 10.1016/j.automatica.2021.109657
![]() |
[23] |
V. Rezaei, M. Stefanovic, Event-triggered robust cooperative stabilization in nonlinearly interconnected multiagent systems, Eur. J. Control, 48 (2019), 9–20. https://doi.org/10.1016/j.ejcon.2019.01.004 doi: 10.1016/j.ejcon.2019.01.004
![]() |
[24] |
Z. R. Zhang, C. Y. Wen, K. Zhao, Y. D. Song, Decentralized adaptive control of uncertain interconnected systems with triggering state signals, Automatica, 141 (2022), 110283. https://doi.org/10.1016/j.automatica.2022.110283 doi: 10.1016/j.automatica.2022.110283
![]() |
[25] |
A. Mirzaei, A. Ramezani, Cooperative optimization-based distributed model predictive control for constrained nonlinear large-scale systems with stability and feasibility guarantees, ISA T., 116 (2021), 81–96. https://doi.org/10.1016/j.isatra.2021.01.022 doi: 10.1016/j.isatra.2021.01.022
![]() |
[26] | H. J. Yang, Y. Q. Xia, P. Shi, L. Zhao, Analysis and synthesis of delta operator systems, Heidelberg: Springer, 2012. https://doi.org/10.1007/978-3-642-28774-9 |
[27] |
H. Hu, Y. Li, J. L. Liu, E. G. Tian, X. P. Xie, Fault estimation for delta operator switched systems with mode-dependent average dwell-time, J. Franklin I., 358 (2021), 5971–5984. https://doi.org/10.1016/j.jfranklin.2021.04.047 doi: 10.1016/j.jfranklin.2021.04.047
![]() |
[28] |
D. H. Zheng, H. B. Zhang, A. D. Zhang, G. Wang, Consensus of multi-agent systems with faults and mismatches under switched topologies using a delta operator method, Neurocomputing, 315 (2018), 198–209. https://doi.org/10.1016/j.neucom.2018.07.017 doi: 10.1016/j.neucom.2018.07.017
![]() |
[29] |
K. Kumari, B. Bandyopadhyay, K. S. Kim, H. Shim, Output feedback based event-triggered sliding mode control for delta operator systems, Automatica, 103 (2019), 1–10. https://doi.org/10.1016/j.automatica.2019.01.015 doi: 10.1016/j.automatica.2019.01.015
![]() |
[30] |
Y. K. Cui, J. Shen, G. Z. Cao, Estimation and synthesis of reachable set for delta operator systems, Nonlinear Anal. Hybri., 32 (2019), 267–275. https://doi.org/10.1016/j.nahs.2019.01.001 doi: 10.1016/j.nahs.2019.01.001
![]() |
[31] |
X. C. Pu, L. Ren, Y. Liu, R. Pu, Couple-group consensus for heterogeneous MASs under switched topologies in cooperative-competitive systems: a hybrid pinning and delta operator skills, Neurocomputing, 441 (2021), 335–349. https://doi.org/10.1016/j.neucom.2020.11.013 doi: 10.1016/j.neucom.2020.11.013
![]() |
[32] |
W. Q. Ji, M. Ma, J. B. Qiu, A new fuzzy sliding mode controller design for delta operator time-delay nonlinear systems, Int. J. Syst. Sci., 50 (2019), 1580–1594. https://doi.org/10.1080/00207721.2019.1617368 doi: 10.1080/00207721.2019.1617368
![]() |
[33] |
D. Y. Zhao, Y. Liu, M. Liu, J. Y. Yu, Adaptive fault-tolerant sliding mode control for Markovian jump systems via delta operator method, IMA J. Math. Control I., 36 (2019), 659–679. https://doi.org/10.1093/imamci/dny002 doi: 10.1093/imamci/dny002
![]() |
[34] |
B. C. Zheng, X. H. Yu, Y. M. Xue, Quantized sliding mode control in delta operator framework, Int. J. Robust Nonlin., 28 (2018), 519–535. https://doi.org/10.1002/rnc.3882 doi: 10.1002/rnc.3882
![]() |
[35] | X. You, H. B. Li, H. J. Yang, Z. X. Liu, Cooperative control for a class of large-scale linear system via delta operator approach, 2013 10th IEEE International Conference on Control and Automation (ICCA), Hangzhou, China, 2013, 1945–1949. https://doi.org/10.1109/ICCA.2013.6564955 |
[36] |
B. C. Zheng, Y. W. Wu, H. Li, Z. P. Chen, Adaptive sliding mode attitude control of quadrotor UAVs based on the delta operator framework, Symmetry, 14 (2022), 498. https://doi.org/10.3390/sym14030498 doi: 10.3390/sym14030498
![]() |
[37] |
Q. X. Zheng, H. L. Chen, S. Y. Xu, Robust guaranteed cost control for uncertain discrete-time systems with state and input quantizations, Inform. Sciences, 546 (2021), 288–305. https://doi.org/10.1016/j.ins.2021.02.057 doi: 10.1016/j.ins.2021.02.057
![]() |
[38] |
H. L. Xu, K. L. Teo, X. Z. Liu, Robust stability analysis of guaranteed cost control for impulsive switched systems, IEEE T. Syst. Man Cy-S., 38 (2008), 1419–1422. https://doi.org/10.1109/TSMCB.2008.925747 doi: 10.1109/TSMCB.2008.925747
![]() |
[39] | L. Yu, Robust control-linear matrix inequality processing method, Beijing: Tsinghua University Press, 2002. |
1. | Mingming Xu, Quanxin Zhu, Hongying Xiao, An Improved Non-Monotonic Adaptive Trust Region Algorithm for Unconstrained Optimization, 2024, 12, 2227-7390, 3398, 10.3390/math12213398 | |
2. | Zhao Li, Chen Peng, Dynamics and Embedded Solitons of Stochastic Quadratic and Cubic Nonlinear Susceptibilities with Multiplicative White Noise in the Itô Sense, 2023, 11, 2227-7390, 3185, 10.3390/math11143185 | |
3. | Chunsheng Wang, Xiangdong Liu, Feng Jiao, Hong Mai, Han Chen, Runpeng Lin, Generalized Halanay Inequalities and Relative Application to Time-Delay Dynamical Systems, 2023, 11, 2227-7390, 1940, 10.3390/math11081940 | |
4. | Arindam Mondal, Sujay Dolai, Prasanta Sarkar, A unified approach for digital realization of fractional order operator in delta domain, 2023, 36, 0353-3670, 379, 10.2298/FUEE2303379M | |
5. | Yan Yan, Multiplicity of positive periodic solutions for a discrete impulsive blood cell production model, 2023, 8, 2473-6988, 26515, 10.3934/math.20231354 | |
6. | Mustafa R. S. Kulenović, Mehmed Nurkanović, Zehra Nurkanović, Susan Trolle, Stability of Certain Non-Autonomous Cooperative Systems of Difference Equations with the Application to Evolutionary Dynamics, 2024, 13, 2075-1680, 730, 10.3390/axioms13110730 | |
7. | Chao Wang, Yinfang Song, Fengjiao Zhang, Yuxiao Zhao, Exponential Stability of a Class of Neutral Inertial Neural Networks with Multi-Proportional Delays and Leakage Delays, 2023, 11, 2227-7390, 2596, 10.3390/math11122596 | |
8. | A. M. Sayed Ahmed, Hamdy M. Ahmed, Nesreen Sirelkhtam Elmki Abdalla, Assmaa Abd-Elmonem, E. M. Mohamed, Approximate controllability of Sobolev-type Atangana-Baleanu fractional differential inclusions with noise effect and Poisson jumps, 2023, 8, 2473-6988, 25288, 10.3934/math.20231290 | |
9. | Mohammad F.M. Naser, Mohammad Abdel Aal, Ghaleb Gumah, Stability analysis of Abel's equation of the first kind, 2023, 8, 2473-6988, 30574, 10.3934/math.20231563 | |
10. | Marya Zainab, Salah Boulaaras, Adnan Aslam, Sidra Shafiq, Takasar Hussain, Muhammad Ozair, Study of fractional order rabies transmission model via Atangana–Baleanu derivative, 2024, 14, 2045-2322, 10.1038/s41598-024-77282-0 | |
11. | Adel M. Al-Mahdi, Long-time behavior for a nonlinear Timoshenko system: Thermal damping versus weak damping of variable-exponents type, 2023, 8, 2473-6988, 29577, 10.3934/math.20231515 | |
12. | Narges Rezaei Kookhdan, Hamid Reza Koofigar, 2023, Dissipativity-Based Stability Analysis of Power Networks with Uncertain Interconnections, 979-8-3503-6601-3, 1, 10.1109/ICCIA61416.2023.10506355 | |
13. | Pitchaimani M, Brasanna Devi M, Quanxin Zhu, Stochastic probes in delay viral infection model with general incidence rate and control strategies, 2023, 360, 00160032, 8506, 10.1016/j.jfranklin.2023.06.005 | |
14. | Shifan Wen, Wanpeng Zhang, Wenxiang Fang, Robustness of linear time‐invariant systems with feedback control protocols under random disturbances, 2024, 1561-8625, 10.1002/asjc.3513 | |
15. | Wei Ouyang, Kui Mei, Quantitative Stability of Optimization Problems with Stochastic Constraints, 2023, 11, 2227-7390, 3885, 10.3390/math11183885 | |
16. | Qing Geng, Jinling Li, Li Li, Dynamic event-triggered control for CPSs under QoS-based variable sampling approach, 2024, 151, 00190578, 12, 10.1016/j.isatra.2024.05.030 | |
17. | Xiangqing Zhao, Wanmei Hou, Optimal Control of SLBRS with Recovery Rates, 2023, 12, 2227-7390, 132, 10.3390/math12010132 | |
18. | Zhengqi Ma, Shoucheng Yuan, Kexin Meng, Shuli Mei, Mean-Square Stability of Uncertain Delayed Stochastic Systems Driven by G-Brownian Motion, 2023, 11, 2227-7390, 2405, 10.3390/math11102405 | |
19. | Wei Ouyang, Kui Mei, A General Iterative Procedure for Solving Nonsmooth Constrained Generalized Equations, 2023, 11, 2227-7390, 4577, 10.3390/math11224577 | |
20. | Tzu-Chien Yin, Nawab Hussain, Hind Alamri, Asim Asiri, Maha Mohammed Saeed, A nonlinear split problem regarding variational inequalities and equilibrium problems, 2024, 2024, 1029-242X, 10.1186/s13660-024-03196-0 | |
21. | Mariyah Aslam, Adnan Malik, Impact of Tolman–Kuchowicz solution on dark energy compact stars in f(R) theory, 2025, 472, 00034916, 169854, 10.1016/j.aop.2024.169854 | |
22. | Xinyu Pan, Research on discrete differential solution methods for derivatives of chaotic systems, 2024, 9, 2473-6988, 33995, 10.3934/math.20241621 | |
23. | Changjin Xu, Muhammad Farman, Aamir Shehzad, Kottakkaran Sooppy Nisar, Modeling and Ulam–Hyers stability analysis of oleic acid epoxidation by using a fractional‐order kinetic model, 2025, 48, 0170-4214, 3726, 10.1002/mma.10510 |