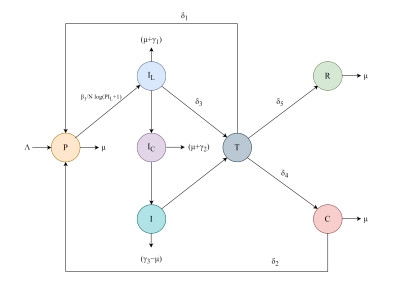
Citation: Anil Chavada, Nimisha Pathak, Sagar R. Khirsariya. A fractional mathematical model for assessing cancer risk due to smoking habits[J]. Mathematical Modelling and Control, 2024, 4(3): 246-259. doi: 10.3934/mmc.2024020
[1] | Anil Chavada, Nimisha Pathak . Transmission dynamics of breast cancer through Caputo Fabrizio fractional derivative operator with real data. Mathematical Modelling and Control, 2024, 4(1): 119-132. doi: 10.3934/mmc.2024011 |
[2] | Ihtisham Ul Haq, Nigar Ali, Hijaz Ahmad . Analysis of a chaotic system using fractal-fractional derivatives with exponential decay type kernels. Mathematical Modelling and Control, 2022, 2(4): 185-199. doi: 10.3934/mmc.2022019 |
[3] | Ankur Jyoti Kashyap, Arnab Jyoti Bordoloi, Fanitsha Mohan, Anuradha Devi . Dynamical analysis of an anthrax disease model in animals with nonlinear transmission rate. Mathematical Modelling and Control, 2023, 3(4): 370-386. doi: 10.3934/mmc.2023030 |
[4] | Ihtisham Ul Haq, Nigar Ali, Shabir Ahmad . A fractional mathematical model for COVID-19 outbreak transmission dynamics with the impact of isolation and social distancing. Mathematical Modelling and Control, 2022, 2(4): 228-242. doi: 10.3934/mmc.2022022 |
[5] | Mohamed COULIBALY, Modeste N'Zi . A stochastic model with jumps for smoking incorporating media coverage. Mathematical Modelling and Control, 2022, 2(3): 122-130. doi: 10.3934/mmc.2022013 |
[6] | Anusmita Das, Kaushik Dehingia, Nabajit Ray, Hemanta Kumar Sarmah . Stability analysis of a targeted chemotherapy-cancer model. Mathematical Modelling and Control, 2023, 3(2): 116-126. doi: 10.3934/mmc.2023011 |
[7] | Abduljawad Anwar, Shayma Adil Murad . On the Ulam stability and existence of Lp-solutions for fractional differential and integro-differential equations with Caputo-Hadamard derivative. Mathematical Modelling and Control, 2024, 4(4): 439-458. doi: 10.3934/mmc.2024035 |
[8] | Mrutyunjaya Sahoo, Dhabaleswar Mohapatra, S. Chakraverty . Wave solution for time fractional geophysical KdV equation in uncertain environment. Mathematical Modelling and Control, 2025, 5(1): 61-72. doi: 10.3934/mmc.2025005 |
[9] | Erick Manuel Delgado Moya, Diego Samuel Rodrigues . Fractional order modeling for injectable and oral HIV pre-exposure prophylaxis. Mathematical Modelling and Control, 2023, 3(2): 139-151. doi: 10.3934/mmc.2023013 |
[10] | Muhammad Nawaz Khan, Imtiaz Ahmad, Mehnaz Shakeel, Rashid Jan . Fractional calculus analysis: investigating Drinfeld-Sokolov-Wilson system and Harry Dym equations via meshless procedures. Mathematical Modelling and Control, 2024, 4(1): 86-100. doi: 10.3934/mmc.2024008 |
The smoking disease, also known as chronic obstructive pulmonary disease (COPD), is a group of progressive lung diseases that cause breathing difficulties [1]. It is primarily caused by long-term exposure to cigarette smoke, although exposure to second-hand smoke, air pollution, and certain occupational fumes can also contribute to its development. Common symptoms of COPD include chest tightness, wheezing, shortness of breath, and persistent cough. Over time, the disease progressively worsens, resulting in a reduced ability to carry out physical activities and everyday tasks. Individuals with COPD may also experience frequent respiratory infections, fatigue, unintended weight loss, and swelling in the ankles, feet, or legs [2].
This smoking-related disease mainly affects the lungs' alveoli, or air sacs, and airways. The toxic compounds in tobacco smoke affect the respiratory system and cause damage to the airways, leading to a narrowing of the air passages and mucus production [3]. As a result, the lungs become less efficient at transferring oxygen into the bloodstream, making breathing more difficult. In advanced stages, COPD can also affect the heart, leading to complications such as heart failure [4]. While there is currently no cure for COPD, there are treatments that can help to control the symptoms and delay the illness's progression. In order to effectively manage COPD, changes in lifestyle like giving up smoking, avoiding triggers in the environment, and engaging in regular exercise are essential. Medications like bronchodilators, inhaled corticosteroids, and oxygen therapy are commonly prescribed to improve breathing and reduce inflammation [5].
Smoking is a major cause of various types of cancer, including lung, oral, throat, bladder, kidney, pancreas, and stomach cancer, among others [6]. It is estimated that smoking is responsible for nearly one-third of all cancer deaths in the United States. When tobacco is smoked, thousands of harmful chemicals are released into the body. These chemicals can cause damage to the DNA in cells, leading to the formation of cancerous cells. Smoking also weakens the immune system, making it harder for the body to fight off cancer cells [7].
Lung cancer is the most common and deadliest form of cancer associated with smoking. The risk of developing lung cancer is directly related to the duration and intensity of smoking. The longer a person smokes and the more cigarettes they consume, the higher their risk of developing lung cancer. Oral and throat cancers are also strongly linked to smoking [8]. The toxic chemicals from tobacco can cause mutations in the cells of the mouth and throat, leading to the formation of cancerous growths. Smokers are also at a higher risk of developing bladder, kidney, pancreas, and stomach cancer, as these organs come into contact with the harmful chemicals through the bloodstream or urine [9].
Quitting smoking significantly decreases the risk of developing cancer. Although it can be challenging, quitting smoking at any age can provide immediate and long-term health benefits. Additionally, early detection through cancer screening programs and regular medical check-ups is crucial for detecting and treating cancer at an early stage, improving the chances of successful treatment and survival [10,11].
Mathematical modeling is highly significant in various fields and has numerous practical applications. Mathematical models provide a means to simulate and analyze real-world phenomena, allowing scientists to conduct virtual experiments and test hypotheses. This enables researchers to explore complex systems and generate insights that may be difficult or impossible through direct experimentation [12,13]. Therefore, mathematical modeling is a great tool as it provides a framework for understanding complex systems, predicting future outcomes, solving problems, making informed decisions, optimizing designs, evaluating policies, conducting scientific research, and fostering education [14,15].
Mathematical models can be used to determine the probability of developing different types of cancer due to smoking. Researchers can incorporate various factors such as smoking duration, intensity, and exposure to second-hand smoke to estimate individual and population-level cancer risks. We can simulate the natural history and progression of smoking-related cancers with the help of modeling [16,17]. Researchers can create models that simulate the effects of implementing intervention strategies like tobacco taxes, smoking cessation programs, or smoke-free regulations, allowing policy-makers to make evidence-based decisions [18].
Models can assist in optimizing treatment strategies for smoking-related cancers. By simulating treatment outcomes and comparing different treatment approaches, researchers can identify the most effective combinations of surgery, chemotherapy, radiation therapy and targeted therapies [19]. This can improve patient outcomes and resource allocation. Mathematical modeling can also be used to evaluate the economic implications of different smoking-related cancer interventions. By considering factors such as healthcare costs, productivity losses, and quality-adjusted life years gained, policymakers can assess the value of various interventions and allocate resources accordingly (see [20]).
Fractional differential equations (FDEs) are a type of differential equation that involve fractional order derivatives. They provide a more flexible and accurate mathematical tool for modeling complex physical phenomena with memory effects, such as viscoelastic materials, anomalous diffusion, and biological systems [21]. To implement FDEs in modeling, one can use semi-analytical or numerical methods that approximate the fractional-order derivatives, as exact closed-form solutions are often unavailable. Several popular numerical methods for FDEs include the Riemann-Liouville, Caputo, Atangana-Baleanu fractional derivative in Caputo sense (ABC), or any other fractional-order operator. Once the system of FDEs is solved, we can analyze and interpret the obtained solution in the context of the physical problem being modeled [22,23]. This may involve calculating key quantities of interest or comparing the results with experimental data.
In the last few decades, many studies have been noted as significant in fractional-order modeling, which include optimal systems, series solutions and conservation laws for a time fractional cancer tumor model [24,25], the Mittag-Leffler kernel approach for time-fractional advection-reaction-diffusion equations [26,27], codimension two Lie invariant solutions of the modified Khokhlov-Zabolotskaya-Kuznetsov equation [28,29], mathematical studies of the solution of Burgers' equations [30,31], numerical studies of the neural network-based fractional mathematical model of immunotherapy and chemotherapy for breast cancer [32], mathematical modeling of integer and fractional order and its simulations for Q-fever [33], an examination of the glucose-insulin alliance scheme in the fractional-order diabetes model [34,35], evaluation of a fractional vector-host disease system mathematically using the Caputo-Fabrizio operator [36], the Atangana-Baleanu-Caputo derivative-based numerical and geometric interpretation of a fractional-order model of the cancer-immune mode [37], the solution of fractional sawada-kotera-ito equation employing the derivatives of Caputo and Atangana-Baleanu [38], and the new semi-analytical approach to solve the fractional-order Sharma-Tasso-Olver equation with the derivatives of Caputo and Atangana-Baleanu [39]. In addition, we have Hopf bifurcation control of a fractional-order delayed turbidostat model, delayed chemostat model, and three-triangle multi-delayed neural networks [40,41,42], the COVID-19 stochastic model, the plankton–oxygen dynamical model owning delay, and a control technique in a predator-prey system [43,44,45], hybrid controller design of a 2D Lotka-Volterra commensal symbiosis system, fractional order uncertain BAM neural networks, and as well as a Hopf bifurcation of a type of BAM neural network model concerning three nonidentical delays [46,47,48].
The present work is organized as follows: Section 2 includes the fractional smoking-related cancer model, its diagram, and detailing of the parameters. Section 3 covers the well-defined definitions and theorems which we have used in this work. Qualitative and stability analysis are discussed in Sections 4 and 5, respectively. Numerical computation and its behavior in the form of figures are displayed in Section 6. Valedictory results and graphical interpretation are discussed in Section 7.
The present mathematical model of smoking-related cancer is classified into seven specific compartments: smoking individuals as the susceptible class P(t), lately infected individuals as IL(t), chronically infected individuals without treatment as IC(t), infected individuals with and without treatment as T(t) and I(t), smoking-related cancer individuals as C(t), and recovered individuals as R(t). We contemplated some parameters in the formation of the model. The disease transmission coefficient from the susceptible P(t) to the lately infected class IL(t) is β1, and from the lately infected IL(t) to the chronically infected class IC(t) is β2. The total population size is denoted as Δ. The infected individuals without treatment I(t) to with treatment T(t) transmission rate is defined as δ1, and smoker individuals P(t) to cancer patient C(t) transmit rate is δ2. For TB-infected patients that transmit from the lately infected IL(t) to infected with treatment class T(t) is defined as δ3, those infected with treatment T(t) that transmit to the smoking-related cancer class C(t) as δ4, and those that transmit from the infected with treatment T(t) to recovered class R(t) as δ5. The death rate due to smoking is marked as μ. Notations α1,α2, and α3 are assigned for infection growth from IL(t) to IC(t) class, from IC(t) to I(t) class, and from I(t) to T(t) class, respectively. The disease death rate in the IL(t),IC(t), and I(t) compartments are γ1,γ2, and γ3, respectively.
By linking the above-mentioned parameters, the mathematical model as a system of differential equations is represented as:
dP(t)dt=Λ−β1N(t)log(P(t)IL(t)+1)+δ1T(t)+δ2C(t)−μP(t),dIL(t)dt=β1N(t)log(P(t)IL(t)+1)−(α1+δ3+γ1+μ)IL(t)−β2IL(t)IC(t),dIC(t)dt=β2IL(t)IC(t)−α2IC(t)−(γ2+μ)IC(t)+α1IL(t),dI(t)dt=α2IC(t)−(α3+γ3−μ)I(t),dT(t)dt=δ3IL(t)−δ4T(t)−(δ1+μ)T(t)+α3I(t)−δ5T(t),dC(t)dt=δ4T(t)−δ2C(t)−μC(t),dR(t)dt=δ5T(t)−μR(t), | (2.1) |
with the initial conditions
P(0)=P0,IL(0)=IL0,IC(0)=IC0,I(0)=I0,T(0)=T0,C(0)=C0,R(0)=R0. |
Here, the total population by adding all of the compartments is defined as
N(t)=P(t)+IL(t)+IC(t)+I(t)+T(t)+C(t)+R(t). |
The proposed smoking-related cancer model, by implementing the ABC fractional derivative [49], is as follows:
ABC0DαtP(t)=Λ−β1N(t)log(P(t)IL(t)+1)+δ1T(t)+δ2C(t)−μP(t),ABC0DαtIL(t)=β1N(t)log(P(t)IL(t)+1)−β2IL(t)IC(t)−(α1+δ3+γ1+μ)IL(t),ABC0DαtIC(t)=β2IL(t)IC(t)−α2IC(t)−(γ2+μ)IC(t)+α1IL(t),ABC0DαtI(t)=α2IC(t)−(α3+γ3−μ)I(t),ABC0DαtT(t)=δ3IL(t)−δ4T(t)−(δ1+μ)T(t)+α3I(t)−δ5T(t),ABC0DαtC(t)=δ4T(t)−δ2C(t)−μC(t),ABC0DαtR(t)=δ5T(t)−μR(t). | (2.2) |
Definition 3.1. [49] Let G∈H1(a,b),a>b, then the ABC fractional derivative is given as:
ABC0DαtG(t)=N(α)1−α∫taEα[−α(t−δ)α1−α]G′(δ)dδ, | (3.1) |
where Eα(⋅) is the Mittag-Leffler function of order one and can be expressed as
Eα(z)=∞∑k=0zkΓ(kα+1). |
Definition 3.2. [49] The ABC fractional integral of order α is defined as:
ABC0IαtG(t)=1−αN(α)G(t)+αN(α)Γ(α)∫t0G(δ)(t−δ)α−1dδ, | (3.2) |
where Γ(⋅) denotes the Gamma function and it can be defined as
Γ(n)=∫∞0e−xxn−1dx. |
Lemma 3.1. [50] The solution of any fractional differential equation
ABC0Dαtψ(t)=f(t),f(t)∈C([0,T)),0<α<1, | (3.3) |
with ψ(0)=ψ0, is given by
ψ(t)=ψ0+(1−α)N(α)f(t)+αΓ(α)N(α)∫t0(t−u)α−1f(u)du. | (3.4) |
The existence and uniqueness of the considered model (2.2) will be covered in this section. The considered model (2.2) can be rewritten as follows:
ABC0DαtP(t)=g1(t,P,IL,IC,I,T,C,R),ABC0DαtIL(t)=g2(t,P,IL,IC,I,T,C,R),ABC0DαtIC(t)=g3(t,P,IL,IC,I,T,C,R),ABC0DαtI(t)=g4(t,P,IL,IC,I,T,C,R),ABC0DαtT(t)=g5(t,P,IL,IC,I,T,C,R),ABC0DαtC(t)=g6(t,P,IL,IC,I,T,C,R),ABC0DαtR(t)=g7(t,P,IL,IC,I,T,C,R), | (4.1) |
where
g1(t,P,IL,IC,I,T,C,R)=Λ−β1Nlog(PIL+1)+δ1T+δ2C−μP,g2(t,P,IL,IC,I,T,C,R)=β1Nlog(PIL+1)−β2ILIC−(α1+δ3+γ1+μ)IL,g3(t,P,IL,IC,I,T,C,R)=β2ILIC−α2IC−(γ2+μ)IC+α1IL,g4(t,P,IL,IC,I,T,C,R)=α2IC−(α3+γ3−μ)I,g5(t,P,IL,IC,I,T,C,R)=δ3IL−δ4T−(δ1+μ)T+α3I−δ5T,g6(t,P,IL,IC,I,T,C,R)=δ4T−δ2C−μC,g7(t,P,IL,IC,I,T,C,R)=δ5T−μR. | (4.2) |
For the purpose of simplicity, we refer to the suggested model (4.1) as follows:
ABC0DαtG(t)=Δ(t,G(t)),G(0)=G0≥0, | (4.3) |
where,
G(t)=(P(t),IL(t),IC(t),I(t),T(t),C(t),R(t))T,G0=(P0,IL0,IC0,I0,T0,C0,R0)T,Δ(t,G(t))=(g1,g2,g3,g4,g5,g6,g7)T. |
Applying fractional ABC integral (3.2) on (4.3), we get:
G(t)=G0+(1−α)N(α)Δ(t,G(t))+αN(α)Γ(α)∫t0(t−δ)α−1Δ(δ,G(δ))dδ. | (4.4) |
Utilizing χ=[0,T] as
Ω=C(χ,R7) |
under the norm specified as follows, let us define the Banach space as:
‖G‖=supt∈χ{G(t):G∈Ω}. |
Assume that the function Δ(t,G(t)) satisfies the following two conditions for each G∈Ω and t∈[0,T].
● ∃ constants μΔ and νΔ such that:
|Δ(t,G(t))|≤μΔ|G|+νΔ. |
● ∃ constant λΔ>0 such that:
|Δ(t,G1(t))−Δ(t,G2(t))|≤λΔ|G1−G2|. |
Now we define the operators Λ1 and Λ2 such that:
Λ1G=G0+(1−α)N(α)Δ(t,G(t)),Λ2G=αN(α)Γ(α)∫t0(t−δ)α−1Δ(δ,G(δ))dδ, |
where
Λ1+Λ2=Ω. |
Theorem 4.1. Suppose that the above-mentioned Lipschitz and growth conditions hold. If the following are true, then there is at least one solution for (4.4):
(I) (1−α)N(α)λΔ<1.
(II) Ψ1=[(1−α)N(α)+TαN(α)Γ(α)]νΔ<1.
(III) Ψ2=[(1−α)N(α)+TαN(α)Γ(α)]μΔ<1.
Proof. Assume a closed convex set
Bτ={G∈Ω:‖G‖≤τ}. |
In order to establish that Λ1G1+Λ2G2∈Bτ for any G1, G2 ∈Bτ. Using the growth condition, we get:
‖Λ1G1+Λ2G2‖≤supt∈χ{|G0|+(1−α)N(α)|Δ(t,G(t))|+αN(α)Γ(α)∫t0(t−δ)α−1|Δ(δ,G(δ))|dδ}≤{|G0|+(1−α)N(α)(μΔ‖G‖+νΔ)+αN(α)Γ(α)∫t0(t−δ)α−1(μΔ‖G‖+νΔ)dδ}=|G0|+{(1−α)N(α)+TαN(α)Γ(α)}νΔ+{(1−α)N(α)+TαN(α)Γ(α)}μΔτ=Ψ1+Ψ2τ≤τ. |
This shows that
Λ1G1+Λ2G2∈Bτ. |
Next, we will show that Λ1 is a contraction. For any G1, G2 ∈Bτ, and using the Lipschitz condition, we get
‖Λ1G1+Λ2G1‖=supt∈[0,T](1−α)N(α)|Δ(t,G1(t))−Δ(t,G2(t))|≤(1−α)N(α)λΔsupt∈[0,T]|G1−G2|≤(1−α)N(α)λΔ‖G1−G2‖, |
where
(1−α)N(α)λΔ<1. |
Thus, Λ1 is a contraction.
Let us now demonstrate the relative compactness of Λ2. Considering G∈Bτ for this
‖Λ2G‖≤supt∈[0,T]αN(α)Γ(α)∫t0(t−δ)α−1|Δ(δ,G(δ))|dδ≤αN(α)Γ(α)∫t0(t−δ)α−1supt∈[0,T][μΔ|G|+νΔ]dδ≤αN(α)Γ(α)∫t0(t−δ)α−1[μΔ‖G‖+νΔ]dδ≤TαN(α)Γ(α)[μΔτ+νΔ]. |
Therefore, on Bτ, Λ2 is uniformly bounded. Lastly, we demonstrate the equicontinuous nature of Λ2. Let t1, t2 ∈[0,T] such that t1<t2. Let G∈Bτ. Following that:
‖Λ2G(t1)−Λ2G(t2)‖≤αN(α)Γ(α)∫t2t1(t2−δ)α−1|Δ(δ,G(δ))|dδ+αN(α)Γ(α)∫t20[(t1−δ)α−1−(t2−δ)α−1]|Δ(δ,G(δ))|dδ≤2[μΔτ+νΔ]N(α)Γ(α)[(t2−t1)α]. |
Thus, ‖Λ2G(t1)−Λ2G(t2)‖→0 as t2→t1. The operator Λ2 is equicontinuous because it is relatively compact, according to the Arzela-Ascoli theorem. Hence, there is at least one solution for Eq (4.4). The considered model has at least one solution since the proposed model is equivalent to Eq (4.4).
Theorem 4.2. Assuming that the Lipschitz condition is satisfied, then the unique solution of Eq (4.4) can be found if
{(1−α)N(α)+TαN(α)Γ(α)}λΔ<1. |
Proof. Considering t∈[0,T] and for any G, G∗ ∈Ω, we have
‖ΛG(t)−ΛG∗(t)‖≤maxt∈[0,T](1−α)N(α)|Δ(t,G(t))−Δ(t,G∗(t))|+maxt∈[0,T]αN(α)Γ(α)∫t0(t−δ)α−1|Δ(t,G(t))−Δ(t,G∗(t))|dδ≤{(1−α)N(α)+TαN(α)Γ(α)}λΔ‖G−G∗‖. |
The operator Λ is a contraction as per the hypothesis
{(1−α)N(α)+TαN(α)Γ(α)}λΔ<1. |
The solution to Eq (4.4) is unique according to the Banach contraction theorem. As a result, the solution for the suggested model (2.2) is unique.
We discuss the equilibrium points of presented model (2.1). Here, we consider the two stage of the population.
(1) Disease-free equilibrium point E0
At this stage, there is no contamination due to the smoking habit in the class. Hence, we set every infection class to zero. Therefore, the disease-free equilibrium point E0 can be defined as:
E0=(Λμ,0,0,0,0,0,0). |
(2) Endemic equilibrium point Eend
To obtain the value of endemic stage Eend, we will equate the system of Eq (2.1) with zero. By solving the system of linear equations, we achieve the endemic equilibrium point Eend as:
Eend=(Pend,IendL,IendC,Iend,Tend,Cend,Rend), |
where
Pend=2β1(1−PIL)μN(1+PIL)+R(δ1δ2+δ1μ+δ2)δ5(δ2+μ),IendL=2β1(PIC(μ+α2+γ2)−α1−β2IC)N(PIC(μ+α2+γ2)+α2+β2IC)(μ+α1+γ1+δ3+β2IC),IendC=1β2(2β1(PIC(μ+α2+γ2)−α1−β2IC)NIL(PIC(μ+α2+γ2)+α2+β2IC)−(μ+α1+γ1+δ3)),Iend=α1α2IL+α2β2ILIC(μ+α2+γ2)(−μ+α3+γ3),Tend=IL(α1α2α3+α2α3β2IC+δ3)(2δ4+δ5−μ)(μ+α2+γ2)(−μ+α3+γ3),Cend=IL(α1α2α3+α2α3β2IC+δ3)(μ+δ2)(2δ4+δ5−μ)(μ+α2+γ2)(−μ+α3+γ3),Rend=IL(δ3δ5+α1α2α3δ5+α2α3β2δ5IC)μ(2δ4+δ5−μ)(μ+α2+γ2)(−μ+α3+γ3). |
To calculate R0, we employ the next generation matrix method. Let us consider the following sub-system by involving the infective compartments as below:
dIL(t)dt=β1N(t)log(P(t)IL(t)+1)−β2IL(t)IC(t)−(α1+δ3+γ1+μ)IL(t),dIC(t)dt=β2IL(t)IC(t)−α2IC(t)−(γ2+μ)IC(t)+α1IL(t),dI(t)dt=α2IC(t)−(α3+γ3−μ)I(t),dC(t)dt=δ4T(t)−δ2C(t)−μC(t). | (5.1) |
Now we find the eigenvalues for the matrix FV−1 to evaluate R0, where matrix F and V for the system (5.1) can be defined as:
F=[−β2IC+β1PN(1+PIL)−β2IL00β2ICβ2IL0000000000] |
and
V=[μ+α1+γ1+δ3000−α1μ+α2+γ2000−α2−μ+α3+γ30000μ+δ2]. |
Next, we find the inverse of matrix V−1 and with simple matrix multiplication, we get
FV−1=[−ILβ2+β1PN(1+PIL)−β2IL(μ+α1+γ1+δ3)−β2ILα100β2IC+β2IL(μ+α1+γ1+δ3)−β2ILα10000000000]. | (5.2) |
Further, the dominant eigenvalues can be found by
|FV−1−λI|=0. |
The basic reproduction number R0 can be defined as
R0=m1+m2+m32α1c1N, | (5.3) |
where
m1=α1β1c1,m2=Nβ2(α1c1IC+α1IL+c1IL) |
and
m3=√α21β21c21+2α1c1N(α1c1IC+β1β2IL(α1−c1)+N2(α1c1IC+β22IL(α1+c1)2)). |
Theorem 5.1. The disease-free stage of smoking-related cancer model (2.1) is locally asymptotically stable if the basic reproduction number R0<0.
Proof. Available in [51].
Theorem 5.2. The endemic stage of smoking-rated cancer model (2.1) is locally asymptotically stable if the endemic Lyaponove function L<0 and the basic reproduction number R0>0.
Proof. Available in [51].
Using the concept of nonlinear functional analysis, we address the Ulam-Hyres (UH) stability of the suggested fractional model (2.2) in this section.
Definition 5.1. If the following property holds true and ∃ ω>0, then the proposed system (2.2) is UH stable. For any ¯G∈Ω and ϵ>0, If
|ABC0Dαt¯G(t)−Δ(t,¯G(t))|≤ϵ, | (5.4) |
then ∃ G∈Ω satisfies the system (2.2) with the initial condition
G(0)=¯G(0)=¯G0, |
such that
‖¯G−G‖≤ωϵ. |
Where
¯G(t)=(¯P(t),¯IL(t),¯IC(t),¯I(t),¯T(t),¯C(t),¯R(t),)T,G0=(¯P0(t),¯IL0(t),¯IC0(t),¯I0(t),¯T0(t),¯C0(t),¯R0(t),)T,Δ(t,¯G(t))=(¯g1,¯g2,¯g3,¯g4,¯g5,¯g6,¯g7)T,ϵ=max(ϵj)T,j=1,2,3,4,5,ω=max(ωj)T,j=1,2,3,4,5. |
Remark 5.1. Consider a small perturbation ρ∈C[0,T] such that ρ(0)=0 along with the following property:
|ρ(t)|≤¯ϵ, |
for t∈[0,T] and ¯ϵ>0.
Lemma 5.3. The solution ¯Gρ(t) of the perturbed system
ABC0Dαt¯G(t)=Δ(t,¯G(t))+ρ(t),¯G(0)=¯G0, | (5.5) |
satisfies the relation
|¯Gρ(t)−¯G(t)|≤[(1−α)Γ(α)+TαN(α)Γ(α)]¯ϵ, |
where
ρ(t)=(ρ1(t),ρ2(t),ρ3(t),ρ4(t),ρ5(t))T. |
Proof. Applying fractional integral (3.2) on (5.5), we get
¯Gρ(t)=¯G0+(1−α)N(α)Δ(t,¯G(t))+αN(α)Γ(α)∫t0(t−δ)α−1Δ(δ,¯G(δ))dδ+(1−α)N(α)ρ(t)+αN(α)Γ(α)∫t0(t−δ)α−1ρ(δ)dδ, |
and also,
¯G(t)=¯G0+(1−α)N(α)Δ(t,¯G(t))+αN(α)Γ(α)∫t0(t−δ)α−1Δ(δ,¯G(δ))dδ. | (5.6) |
Using Remark 5.1,
|¯Gρ(t)−¯G(t)|≤(1−α)N(α)|ρ(t)|+αN(α)Γ(α)∫t0(t−δ)α−1|ρ(δ)|dδ,≤[(1−α)Γ(α)+TαN(α)Γ(α)]¯ϵ. |
This completes the proof.
Theorem 5.4. The proposed fractional system (2.2) is UM stable if
‖¯G(t)−G(t)‖≤ω¯ϵ. |
Proof. Since G is a unique solution of the system (5.5) and ¯G is the solution of (5.4), then
|¯G(t)−G(t)|≤|¯Gρ(t)−¯G(t)|+|¯Gρ(t)−G(t)|≤2[(1−α)Γ(α)+TαN(α)Γ(α)]¯ϵ+1−αN(α)|Δ(t,¯G(t))−Δ(t,G(t))|+αN(α)Γ(α)∫t0(t−δ)α−1|Δ(δ,¯G(δ))−Δ(δ,G(δ))|dδ≤2[(1−α)Γ(α)+TαN(α)Γ(α)]¯ϵ+[(1−α)Γ(α)+TαN(α)Γ(α)]λΔ|¯G−G|, |
which implies that
|¯G(t)−G(t)|≤2ξ¯ϵ1−η, |
where
ξ=2[(1−α)Γ(α)+TαN(α)Γ(α)] |
and
η=[(1−α)Γ(α)+TαN(α)Γ(α)]λΔ. |
For
ω=2ξ1−η, |
then
|¯G(t)−G(t)|≤ω¯ϵ. |
Hence, the considered fractional system (2.2) is UM stable.
In this work, we obtain the solution of the fractional-order smoking-related cancer model (2.2) using the Adams-Bashforth-Moulton method [52]. We take some specific values of the necessary parameters
Λ=0.002,β1=0.00012,β2=0.002,δ1=0.0032,δ2=0.00233,δ3=0.00462,δ4=0.00023,δ5=0.000123,μ=0.05,α1=0.001,α2=0.0045,α3=0.000123,γ1=0.007,γ2=0.00023,γ3=0.00034 |
with the initial values
P0=40, IL0=20, IC0=15, I0=10, T0=10, C0=5, R0=0. |
Figures 1–7 represent the behavior of each compartment when we utilized the ABC with some fractional orders between 0.9 and 1. We plot the 3D behavior of the transmission rate from the IL(t) class to the IC(t) class when β2 and α1 are between 0 and 0.004 in Figures 8 and 9, respectively. When infective parameter δ4 is between 0.0001 and 0.0004, the rate of transmission from the I(t) and C(t) class is demonstrated as Figure 10. When another infective parameter δ5 is between 0 and 0.0004, the rate of transmission from I(t) to R(t) class is obtained as in Figure 11. Figure 12 is the plot of the transmission rate from infected to recovered individuals when infective parameter δ5 change.
We demonstrated a fractional-order smoking-related cancer mathematical model with seven compartments under the duration of 60 days. We utilized the ABC fractional operator as it has non-local and non-singular properties. Further, we acquired a well-known Adams-Bashforth-Moulton method which provided a fast convergence to the solution. The existence and uniqueness of the system was proved with the help of Banach's fix point theorem. By calculating the equilibrium points and basic reproduction number, we validated the local stability and, with the use of Ulam-Hyres stability, we admitted the global stability of the suggested model. From Figures 1–8, we see that the behavior of the solution of each class having a stable region. Figures 9 and 10 show that when transmission coefficients β2 and α1 change, the lately infected individuals transmit to chronically infected individuals. Figure 11 displays the transmission rate from infected individuals to cancer due to smoking individuals when infective parameter δ4 differs. By incorporating data on smoking-related cancer and its treatment effectiveness, researchers can gain insights into how different factors affect the cancer progression and design strategies for prevention, diagnosis, and treatment. It can help to evaluate the impact of different tobacco control policies on cancer incidence and mortality.
The use of the smoking-related cancer model in research can support the UN's sustainable development goals in several ways. Researchers can contribute valuable insights into the causes and mechanisms of cancer development, which can ultimately lead to improved prevention, early detection, and treatment strategies. This can have a significant impact on good health and well-being, by reducing the burden of cancer worldwide and improving overall public health. Additionally, research on the smoking-related cancer model can also contribute to quality education, by providing valuable training and educational opportunities for researchers and healthcare professionals. By sharing knowledge and expertise gained from studying the smoking cancer model, researchers can help build capacity in cancer research and care, ultimately improving the quality of education and healthcare delivery in this field.
The authors declare they have not used Artificial Intelligence (AI) tools in the creation of this article.
The authors have no conflicts of interest to declare.
[1] |
J. M. Sethi, C. L. Rochester, Smoking and chronic obstructive pulmonary disease, Clin. Chest Med., 21 (2000), 67–86. https://doi.org/10.1016/S0272-5231(05)70008-3 doi: 10.1016/S0272-5231(05)70008-3
![]() |
[2] |
R. M. Senior, N. R. Anthonisen, Chronic obstructive pulmonary disease (COPD), Amer. J. Resp. Crit. Care Med., 157 (1998), 139–147. https://doi.org/10.1164/ajrccm.157.4.nhlbi-12 doi: 10.1164/ajrccm.157.4.nhlbi-12
![]() |
[3] |
D. Twardella, M. Loew, D. Rothenbacher, C. Stegmaier, H. Ziegler, H. Brenner, The diagnosis of a smoking-related disease is a prominent trigger for smoking cessation in a retrospective cohort study, J. Clin. Epidemiol., 59 (2006), 82–89. https://doi.org/10.1016/j.jclinepi.2005.05.003 doi: 10.1016/j.jclinepi.2005.05.003
![]() |
[4] |
T. Alnima, R. Meijer, H. Spronk, M. Warlé, H. Cate, Diabetes-versus smoking-related thrombo-inflammation in peripheral artery disease, Cardiovasc. Diabetol., 22 (2023), 257. https://doi.org/10.1186/s12933-023-01990-6 doi: 10.1186/s12933-023-01990-6
![]() |
[5] |
G. Kaur, R. Begum, S. Thota, S. Batra, A systematic review of smoking-related epigenetic alterations, Arch. Toxicol., 93 (2019), 2715–2740. https://doi.org/10.1007/s00204-019-02562-y doi: 10.1007/s00204-019-02562-y
![]() |
[6] |
Z. J. Phua, R. J. MacInnis, H. Jayasekara, Cigarette smoking and risk of second primary cancer: a systematic review and meta-analysis, Cancer Epidemiol., 78 (2022), 102160. https://doi.org/10.1016/j.canep.2022.102160 doi: 10.1016/j.canep.2022.102160
![]() |
[7] |
M. F. Weber, P. E. Sarich, P. Vaneckova, S. Wade, S. Egger, P. Ngo, et al., Cancer incidence and cancer death in relation to tobacco smoking in a population-based australian cohort study, Int. J. Cancer, 149 (2021), 1076–1088. https://doi.org/10.1002/ijc.33685 doi: 10.1002/ijc.33685
![]() |
[8] |
L. M. Reynales-Shigematsu, J. Barnoya, T. Cavalcante, T. C. Aburto, I. Romieu, M. C. Stern, et al., Latin america and the caribbean code against cancer 1st edition: tobacco and nicotine-related products, secondhand smoke, and alcohol and cancer, Cancer Epidemiol., 86 (2023), 102413. https://doi.org/10.1016/j.canep.2023.102413 doi: 10.1016/j.canep.2023.102413
![]() |
[9] |
N. Nwizu, J. Wactawski-Wende, R. J. Genco, Periodontal disease and cancer: epidemiologic studies and possible mechanisms, Periodontol. 2000, 83 (2020), 213–233. https://doi.org/10.1111/prd.12329 doi: 10.1111/prd.12329
![]() |
[10] |
Z. Z. Tu, Q. Lu, Y. B. Zhang, Z. Shu, Y. W. Lai, M. N. Ma, et al., Associations of combined healthy lifestyle factors with risks of diabetes, cardiovascular disease, cancer, and mortality among adults with prediabetes: Four prospective cohort studies in china, the united kingdom, and the united states, Engineering, 22 (2023), 141–148. https://doi.org/10.1016/j.eng.2022.04.010 doi: 10.1016/j.eng.2022.04.010
![]() |
[11] |
A. Plym, Y. Zhang, K. H. Stopsack, B. Delcoigne, F. Wiklund, C. Haiman, et al., A healthy lifestyle in men at increased genetic risk for prostate cancer, Eur. Urol., 83 (2023), 343–351. https://doi.org/10.1016/j.eururo.2022.05.008 doi: 10.1016/j.eururo.2022.05.008
![]() |
[12] |
S. S. Tuly, M. Mahiuddin, A. Karim, Mathematical modeling of nutritional, color, texture, and microbial activity changes in fruit and vegetables during drying: a critical review, Crit. Rev. Food Sci. Nutr., 63 (2023), 1877–1900. https://doi.org/10.1080/10408398.2021.1969533 doi: 10.1080/10408398.2021.1969533
![]() |
[13] |
A. Abidemi, Optimal cost-effective control of drug abuse by students: insight from mathematical modeling, Model. Earth Syst. Environ., 9 (2023), 811–829. https://doi.org/10.1007/s40808-022-01534-z doi: 10.1007/s40808-022-01534-z
![]() |
[14] |
O. J. Peter, H. S. Panigoro, M. A. Ibrahim, O. M. Otunuga, T. A. Ayoola, A. O. Oladapo, Analysis and dynamics of measles with control strategies: a mathematical modeling approach, Int. J. Dyn. Control, 11 (2023), 2538–2552. https://doi.org/10.1007/s40435-022-01105-1 doi: 10.1007/s40435-022-01105-1
![]() |
[15] |
N. Bellomo, N. Outada, J. Soler, Y. Tao, M. Winkler, Chemotaxis and cross-diffusion models in complex environments: models and analytic problems toward a multiscale vision, Math. Mod. Methods Appl. Sci., 32 (2022), 713–792. https://doi.org/10.1142/S0218202522500166 doi: 10.1142/S0218202522500166
![]() |
[16] |
I. Sofia, M. Ghosh, Mathematical modeling of smoking habits in the society, Stoch. Anal. Appl., 41 (2023), 918–937. https://doi.org/10.1080/07362994.2022.2093223 doi: 10.1080/07362994.2022.2093223
![]() |
[17] |
I. R. Sofia, S. R. Bandekar, M. Ghosh, Mathematical modeling of smoking dynamics in society with impact of media information and awareness, Results Control Optim., 11 (2023), 100233. https://doi.org/10.1016/j.rico.2023.100233 doi: 10.1016/j.rico.2023.100233
![]() |
[18] |
T. Hussain, A. U. Awan, K. A. Abro, M. Ozair, M. Manzoor, J. F. Gómez-Aguilar, et al., A passive verses active exposure of mathematical smoking model: a role for optimal and dynamical control, Nonlinear Eng., 11 (2022), 507–521. https://doi.org/10.1515/nleng-2022-0214 doi: 10.1515/nleng-2022-0214
![]() |
[19] | S. Blauth, F. Hübner, C. Leithäuser, N. Siedow, T. J. Vogl, Mathematical modeling and simulation of laser-induced thermotherapy for the treatment of liver tumors, In: R. Pinnau, N. R. Gauger, A. Klar, Modeling, simulation and optimization in the health-and energy-sector, Springer, 2022. https://doi.org/10.1007/978-3-030-99983-4_1 |
[20] |
S. A. Jose, R. Raja, Q. Zhu, J. Alzabut, M. Niezabitowski, V. E. Balas, Impact of strong determination and awareness on substance addictions: a mathematical modeling approach, Math. Methods Appl. Sci., 45 (2022), 4140–4160. https://doi.org/10.1002/mma.7859 doi: 10.1002/mma.7859
![]() |
[21] | E. Shishkina, S. Sitnik, Transmutations, singular and fractional differential equations with applications to mathematical physics, Academic Press, 2020. |
[22] |
J. P. Chauhan, S. R. Khirsariya, A semi-analytic method to solve nonlinear differential equations with arbitrary order, Results Control Optim., 12 (2023), 100267. https://doi.org/10.1016/j.rico.2023.100267 doi: 10.1016/j.rico.2023.100267
![]() |
[23] |
H. Sun, A. Chang, Y. Zhang, W. Chen, A review on variable-order fractional differential equations: mathematical foundations, physical models, numerical methods and applications, Fract. Calc. Appl. Anal., 22 (2019), 27–59. https://doi.org/10.1515/fca-2019-0003 doi: 10.1515/fca-2019-0003
![]() |
[24] |
S. G. Simon, B. Bira, D. Zeidan, Optimal systems, series solutions and conservation laws for a time fractional cancer tumor model, Chaos Solitons Fract., 169 (2023), 113311. https://doi.org/10.1016/j.chaos.2023.113311 doi: 10.1016/j.chaos.2023.113311
![]() |
[25] |
M. Izadi, D. Zeidan, A convergent hybrid numerical scheme for a class of nonlinear diffusion equations, Comput. Appl. Math., 41 (2022), 318. https://doi.org/10.1007/s40314-022-02033-8 doi: 10.1007/s40314-022-02033-8
![]() |
[26] |
S. Kumar, D. Zeidan, An efficient Mittag-Leffler kernel approach for time-fractional advection-reaction-diffusion equation, Appl. Numer. Math., 170 (2021), 190–207. https://doi.org/10.1016/j.apnum.2021.07.025 doi: 10.1016/j.apnum.2021.07.025
![]() |
[27] |
D. Zeidan, C. K. Chau, T. T. Lu, On the characteristic Adomian decomposition method for the Riemann problem, Math. Methods Appl. Sci., 44 (2021), 8097–8112. https://doi.org/10.1002/mma.5798 doi: 10.1002/mma.5798
![]() |
[28] |
B. Bira, H. Mandal, D. Zeidan, Exact solution of the time fractional variant Boussinesq-Burgers equations, Appl. Math., 66 (2021), 437–449. https://doi.org/10.21136/AM.2021.0269-19 doi: 10.21136/AM.2021.0269-19
![]() |
[29] |
P. Satapathy, T. R. Sekhar, D. Zeidan, Codimension two Lie invariant solutions of the modified Khokhlov-Zabolotskaya-Kuznetsov equation, Math. Methods Appl. Sci., 44 (2021), 4938–4951. https://doi.org/10.1002/mma.7078 doi: 10.1002/mma.7078
![]() |
[30] |
F. Sultana, D. Singh, R. K. Pandey, D. Zeidan, Numerical schemes for a class of tempered fractional integro-differential equations, Appl. Numer. Math., 157 (2020), 110–134. https://doi.org/10.1016/j.apnum.2020.05.026 doi: 10.1016/j.apnum.2020.05.026
![]() |
[31] |
D. Zeidan, C. K. Chau, T. T. Lu, W. Q. Zheng, Mathematical studies of the solution of Burgers' equations by Adomian decomposition method, Math. Methods Appl. Sci., 43 (2020), 2171–2188. https://doi.org/10.1002/mma.5982 doi: 10.1002/mma.5982
![]() |
[32] |
Z. Sabir, M. Munawar, M. A. Abdelkawy, M. A. Z. Raja, C. Ünlü, M. B. Jeelani, et al., Numerical investigations of the fractional-order mathematical model underlying immune-chemotherapeutic treatment for breast cancer using the neural networks, Fractal Fract., 6 (2022), 184. https://doi.org/10.3390/fractalfract6040184 doi: 10.3390/fractalfract6040184
![]() |
[33] |
J. K. K. Asamoah, E. Okyere, E. Yankson, A. A. Opoku, A. Adom-Konadu, E. Acheampong, et al., Non-fractional and fractional mathematical analysis and simulations for Q fever, Chaos Solitons Fract., 156 (2022), 111821. https://doi.org/10.1016/j.chaos.2022.111821 doi: 10.1016/j.chaos.2022.111821
![]() |
[34] |
S. R. Khirsariya, S. B. Rao, G. S. Hathiwala, Investigation of fractional diabetes model involving glucose-insulin alliance scheme, Int. J. Dyn. Control, 12 (2024), 1–14. https://doi.org/10.1007/s40435-023-01293-4 doi: 10.1007/s40435-023-01293-4
![]() |
[35] |
S. R. Khirsariya, J. P. Chauhan, G. S. Hathiwala, Study of fractional diabetes model with and without complication class, Results Control Optim., 12 (2023), 100283. https://doi.org/10.1016/j.rico.2023.100283 doi: 10.1016/j.rico.2023.100283
![]() |
[36] |
Y. M. Chu, M. F. Khan, S. Ullah, S. A. A. Shah, M. Farooq, M. bin Mamat, Mathematical assessment of a fractional-order vector-host disease model with the Caputo-Fabrizio derivative, Math. Methods Appl. Sci., 46 (2023), 232–247. https://doi.org/10.1002/mma.8507 doi: 10.1002/mma.8507
![]() |
[37] |
B. Maayah, O. A. Arqub, S. Alnabulsi, H. Alsulami, Numerical solutions and geometric attractors of a fractional model of the cancer-immune based on the Atangana-Baleanu-Caputo derivative and the reproducing kernel scheme, Chinese J. Phys., 80 (2022), 463–483. https://doi.org/10.1016/j.cjph.2022.10.002 doi: 10.1016/j.cjph.2022.10.002
![]() |
[38] |
S. R. Khirsariya, S. B. Rao, Solution of fractional Sawada-Kotera-Ito equation using caputo and Atangana-Baleanu derivatives, Math. Methods Appl. Sci., 46 (2023), 16072–16091. https://doi.org/10.1002/mma.9438 doi: 10.1002/mma.9438
![]() |
[39] |
J. P. Chauhan, S. R. Khirsariya, G. S. Hathiwala, M. B. Hathiwala, New analytical technique to solve fractional-order sharma-tasso-olver differential equation using caputo and Atangana-Baleanu derivative operators, J. Appl. Anal., 30 (2024), 1–16. https://doi.org/10.1515/jaa-2023-0043 doi: 10.1515/jaa-2023-0043
![]() |
[40] |
C. Xu, W. Ou, Y. Pang, Q. Cui, M. U. Rahman, M. Farman, et al., Hopf bifurcation control of a fractional-order delayed turbidostat model via a novel extended hybrid controller, Match Commun. Math. Comput. Chem., 91 (2024), 367–413. https://doi.org/10.46793/match.91-2.367X doi: 10.46793/match.91-2.367X
![]() |
[41] |
C. Xu, Z. Liu, P. Li, J. Yan, L. Yao, Bifurcation mechanism for fractional-order three-triangle multi-delayed neural networks, Neural Process. Lett., 55 (2023), 6125–6151. https://doi.org/10.1007/s11063-022-11130-y doi: 10.1007/s11063-022-11130-y
![]() |
[42] |
C. Xu, Q. Cui, Z. Liu, Y. Pan, W. Ou, X. Cui, et al., Extended hybrid controller design of bifurcation in a delayed chemostat model, Match Commun. Math. Comput. Chem., 90 (2023), 609–648. https://doi.org/10.46793/match.90-3.609X doi: 10.46793/match.90-3.609X
![]() |
[43] |
C. Xu, Y. Pang, Z. Liu, J. Shen, M. Liao, P. Li, Insights into COVID-19 stochastic modelling with effects of various transmission rates: simulations with real statistical data from UK, Australia, Spain, and India, Phys. Scr., 99 (2024), 025218. https://doi.org/10.1088/1402-4896/ad186c doi: 10.1088/1402-4896/ad186c
![]() |
[44] | C. Xu, Y. Zhao, J. Lin, Y. Pang, Z. Liu, J. Shen, et al., Mathematical exploration on control of bifurcation for a plankton-oxygen dynamical model owning delay, J. Math. Chem., 2023. https://doi.org/10.1007/s10910-023-01543-y |
[45] |
W. Ou, C. Xu, Q. Cui, Y. Pang, Z. Liu, J. Shen, et al., Hopf bifurcation exploration and control technique in a predator-prey system incorporating delay, AIMS Math., 9 (2024), 1622–1651. https://doi.org/10.3934/math.2024080 doi: 10.3934/math.2024080
![]() |
[46] |
Q. Cui, C. Xu, W. Ou, Y. Pang, Z. Liu, P. Li, et al., Bifurcation behavior and hybrid controller design of a 2D Lotka-Volterra commensal symbiosis system accompanying delay, Mathematics, 11 (2023), 4808. https://doi.org/10.3390/math11234808 doi: 10.3390/math11234808
![]() |
[47] |
M. Chinnamuniyandi, S. Chandran, C. Xu, Fractional order uncertain BAM neural networks with mixed time delays: an existence and quasi-uniform stability analysis, J. Intell. Fuzzy Syst., 46 (2024), 4291–4313. https://doi.org/10.3233/JIFS-234744 doi: 10.3233/JIFS-234744
![]() |
[48] |
P. Li, R. Gao, C. Xu, J. Shen, S. Ahmad, Y. Li, Exploring the impact of delay on Hopf bifurcation of a type of BAM neural network models concerning three nonidentical delays, Neural Process. Lett., 55 (2023), 11595–11635. https://doi.org/10.1007/s11063-023-11392-0 doi: 10.1007/s11063-023-11392-0
![]() |
[49] | A. Atangana, D. Baleanu, New fractional derivatives with nonlocal and non-singular kernel: theory and application to heat transfer model, arXiv, 2016. https://doi.org/10.48550/arXiv.1602.03408 |
[50] |
A. Din, Y. Li, Lévy noise impact on a stochastic hepatitis b epidemic model under real statistical data and its fractal-fractional Atangana-Baleanu order model, Phys. Scr., 96 (2021), 124008. https://doi.org/10.1088/1402-4896/ac1c1a doi: 10.1088/1402-4896/ac1c1a
![]() |
[51] |
I. Shah, Eiman, H. Alrabaiah, B. Ozdemir, A. ur R. Irshad, Using advanced analysis together with fractional order derivative to investigate a smoking tobacco cancer model, Results Phys., 51 (2023), 106700. https://doi.org/10.1016/j.rinp.2023.106700 doi: 10.1016/j.rinp.2023.106700
![]() |
[52] |
K. Diethelm, An efficient parallel algorithm for the numerical solution of fractional differential equations, Fract. Calc. Appl. Anal., 14 (2011), 475–490. https://doi.org/10.2478/s13540-011-0029-1 doi: 10.2478/s13540-011-0029-1
![]() |
1. | Abdul Hamid Ganie, N. S. Alharthi, Adnan Khan, Abdulkafi Mohammed Saeed, Mohd Asif Shah, Saurav Mallik, The series solutions of fractional foam drainage and fractional modified regularized long wave problems, 2024, 2024, 1029-242X, 10.1186/s13660-024-03227-w | |
2. | Mashael M. AlBaidani, Fahad Aljuaydi, Shahad Abdullah F. Alsubaie, Abdul Hamid Ganie, Adnan Khan, Computational and Numerical Analysis of the Caputo-Type Fractional Nonlinear Dynamical Systems via Novel Transform, 2024, 8, 2504-3110, 708, 10.3390/fractalfract8120708 | |
3. | Weiguang Zhou, Guangxin Wang, Jixiao Lu, Hongxin Ruan, Jun Wang, Rui Zhang, On a data model associated with antitrust behaviors, 2024, 4, 2767-8946, 459, 10.3934/mmc.2024036 | |
4. | Aziz Khan, Thabet Abdeljawad, Mahmoud Abdel-Aty, D.K. Almutairi, Digital analysis of discrete fractional order cancer model by artificial intelligence, 2025, 118, 11100168, 115, 10.1016/j.aej.2025.01.036 | |
5. | Akanksha Singh, Anil Chavada, Nimisha Pathak, Fractional Calculus Approach to Pancreatic Cancer Therapy: Modeling Tumor and Immune Interactions with siRNA Treatment, 2025, 11, 2349-5103, 10.1007/s40819-025-01873-2 | |
6. | Sanjay Bhatter, Sangeeta Kumawat, Sunil Dutt Purohit, D. L. Suthar, Mathematical modeling of tuberculosis using Caputo fractional derivative: a comparative analysis with real data, 2025, 15, 2045-2322, 10.1038/s41598-025-97502-5 |