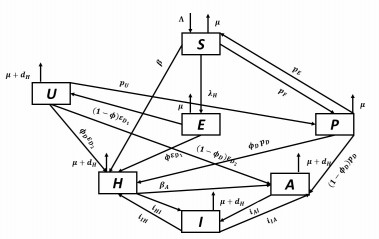
The search for therapies and prevention methods for HIV infection is essential for controlling the virus in a population. In this paper, we introduce a fractional order mathematical model to study the impact of the oral to the injectable Pre-Exposured Prophylaxis modality, which is completely new in terms of public health. For that, we consider current antiretroviral therapies, undiagnosed cases, and the impact of PrEP on the case diagnosis. To investigate the model, besides the use of fractional order derivatives, we also consider illustrative cases by means of demographic data from Brazil and parameter values from the literature. We compare the influence on incidence, prevalence, diagnosis, and mortality of oral PrEP with the injectable PrEP, which is the new current trend on the subject. As a result, an increasing in incidence, prevalence and also mortality are revealed by augmented fractional order of derivatives for both PrEP modalities, but PrEP reached better results in its oral modality. Despite the need for further studies, this contribution is intended as a first preliminary step to contribute to decision-making by health authorities.
Citation: Erick Manuel Delgado Moya, Diego Samuel Rodrigues. Fractional order modeling for injectable and oral HIV pre-exposure prophylaxis[J]. Mathematical Modelling and Control, 2023, 3(2): 139-151. doi: 10.3934/mmc.2023013
[1] | Anil Chavada, Nimisha Pathak, Sagar R. Khirsariya . A fractional mathematical model for assessing cancer risk due to smoking habits. Mathematical Modelling and Control, 2024, 4(3): 246-259. doi: 10.3934/mmc.2024020 |
[2] | Iman Malmir . Novel closed-loop controllers for fractional nonlinear quadratic systems. Mathematical Modelling and Control, 2023, 3(4): 345-354. doi: 10.3934/mmc.2023028 |
[3] | Biresh Kumar Dakua, Bibhuti Bhusan Pati . A frequency domain-based loop shaping procedure for the parameter estimation of the fractional-order tilt integral derivative controller. Mathematical Modelling and Control, 2024, 4(4): 374-389. doi: 10.3934/mmc.2024030 |
[4] | Mrutyunjaya Sahoo, Dhabaleswar Mohapatra, S. Chakraverty . Wave solution for time fractional geophysical KdV equation in uncertain environment. Mathematical Modelling and Control, 2025, 5(1): 61-72. doi: 10.3934/mmc.2025005 |
[5] | Archit Chaturvedi . Polymer physics-based mathematical models for the correlation of DNA and mRNA in a eukaryotic cell. Mathematical Modelling and Control, 2022, 2(3): 131-138. doi: 10.3934/mmc.2022014 |
[6] | Anil Chavada, Nimisha Pathak . Transmission dynamics of breast cancer through Caputo Fabrizio fractional derivative operator with real data. Mathematical Modelling and Control, 2024, 4(1): 119-132. doi: 10.3934/mmc.2024011 |
[7] | Ankur Jyoti Kashyap, Arnab Jyoti Bordoloi, Fanitsha Mohan, Anuradha Devi . Dynamical analysis of an anthrax disease model in animals with nonlinear transmission rate. Mathematical Modelling and Control, 2023, 3(4): 370-386. doi: 10.3934/mmc.2023030 |
[8] | Ihtisham Ul Haq, Nigar Ali, Hijaz Ahmad . Analysis of a chaotic system using fractal-fractional derivatives with exponential decay type kernels. Mathematical Modelling and Control, 2022, 2(4): 185-199. doi: 10.3934/mmc.2022019 |
[9] | Erick M. D. Moya, Diego Samuel Rodrigues . A mathematical model for the study of latent tuberculosis under 3HP and 1HP regimens. Mathematical Modelling and Control, 2024, 4(4): 400-416. doi: 10.3934/mmc.2024032 |
[10] | S. Y. Tchoumi, Y. Kouakep-Tchaptchie, D. J. Fotsa-Mbogne, J. C. Kamgang, J. M. Tchuenche . Optimal control of a malaria model with long-lasting insecticide-treated nets. Mathematical Modelling and Control, 2021, 1(4): 188-207. doi: 10.3934/mmc.2021018 |
The search for therapies and prevention methods for HIV infection is essential for controlling the virus in a population. In this paper, we introduce a fractional order mathematical model to study the impact of the oral to the injectable Pre-Exposured Prophylaxis modality, which is completely new in terms of public health. For that, we consider current antiretroviral therapies, undiagnosed cases, and the impact of PrEP on the case diagnosis. To investigate the model, besides the use of fractional order derivatives, we also consider illustrative cases by means of demographic data from Brazil and parameter values from the literature. We compare the influence on incidence, prevalence, diagnosis, and mortality of oral PrEP with the injectable PrEP, which is the new current trend on the subject. As a result, an increasing in incidence, prevalence and also mortality are revealed by augmented fractional order of derivatives for both PrEP modalities, but PrEP reached better results in its oral modality. Despite the need for further studies, this contribution is intended as a first preliminary step to contribute to decision-making by health authorities.
HIV (human immunodeficiency virus) is a virus that attacks the immune system. Over time, HIV weakens the immune system and makes it lose its ability to fight certain diseases. HIV is the cause of AIDS (Acquired Immune Deficiency Syndrome) which is an advanced stage of the disease where the immune system is compromised [1].
HIV/AIDS data shows that in 2021, 38.4 million [33.9 million-43.8 million] people in the world are living with HIV, 1.5 million [1.1 million-2 million] people have become infected with HIV, 650,000 [510,000 - 860,000] people have died from AIDS-related illnesses, and 28.7 million people are accessing antiretroviral therapy [2]. In Brazil, every hour, at least five people were infected with HIV in 2021. The World of Health Organization (WHO) estimates that throughout 2021 Brazil had 50,000 new cases, which brought the country to the mark of 960,000 people living with HIV.
Oral PrEP uses antiretroviral drugs in pill form to prevent the spread of HIV/AIDS. Currently, there are two approved forms of oral PrEP in use: a combination of tenofovir and emtricitabine or TDF/FTC (brand name Truvada) and a combination of tenofovir, alafenamide, and emtricitabine or F/TAF (brand name Descovy) [3]. Both are for daily use. PrEP reduces the risk of getting HIV from sex by about 99% and the risk of getting HIV from injection drug use by at least 74%.
According to data provided by WHO, about 940,000 people in 83 countries worldwide received oral PrEP at least once in 2020. This is a 49% increase over the approximately 630,000 PrEP users reported in 76 countries in 2019 and more than 2.5 times the number of PrEP users in 2018 (370,000). The majority of PrEP users in 2020 were reported in the Africa region (52%) and the Americas (30%; 26% in the United States). Ninety-seven percent of global PrEP users are in 30 countries with more than 2,000 PrEP users each [4].
In September 2021, there were 270 health centers in all Brazilian states providing PrEP to approximately 23,000 people. Another 3,000 or more received PrEP through research studies, such as the PrEP Implementation Project (ImPrEP). Recent Brazilian studies estimate HIV prevalence among men who have sex with men as high as 23.0%, but it is impossible to know how many who do not have HIV would benefit from PrEP [5].
Long-acting injectable PrEP with the drug cabotegravir was approved in the United States for the prevention of sexual transmission of HIV in December 2021 [6]. WHO has recently issued new guidelines on long-acting injectable PrEP and advises countries to consider it as another effective HIV prevention option (including oral PrEP, condoms, and post-exposure prophylaxis) for people at risk for HIV [7]. Injectable PrEP is administered once every two months, which strongly contributes to adherence to treatment and avoids some of the problems that occur with oral PrEP, which is used daily.
Mathematical models studying the influence of PrEP have increased since its implementation [8,9,10,11]. For example, Delgado et al. [8] presented a mathematical model for the study of the influence of PrEP and PEP in the presence of non-diagnosed and undetected cases. Kim et al. [9] build a mathematical model of HIV infection among MSM in South Korea and simulate the effects of early antiretroviral therapy (ART), early diagnosis, PrEP and combined interventions on the incidence and prevalence of HIV/AIDS infection. Nsuami and Witbooi [10] presented a stochastic model for the HIV/AIDS epidemic with the use of PrEP. Silva and Torres [11] proposed an epidemiological model for HIV/AIDS transmission that includes PrEP and calibrated the model with cumulative cases of HIV infection and AIDS recorded in Cape Verde from 1987 to 2014 and demonstrated that PrEP significantly reduces HIV transmission.
The main contribution of our work is the presentation and study of a mathematical model that takes into account the effect of PrEP in a population and how it contributes to the diagnosis of cases. In more practical terms, with the model we studied the impact on the Brazilian population of oral PrEP and the new modality of injectable PrEP, comparing their impact on HIV incidence, prevalence, mortality, and case diagnosis. To make a wider exploration, fractional order derivatives are used to obtain different behaviors aiming to contribute to health authorities in decision making. Another reason for using a fractional order derivatives is the effect of memory of such operators, which can be relevant in epidemiology [12,13,14].
This paper is organized as follows: in Section 2, a theoretical background of derivatives of fractional order is presented for completeness. In Section 3, we present the model using the Caputo fractional derivative, studying its dynamics in the light of mathematical epidemiology. Section 4 is devoted to illustrative cases using computational simulations, and Section 5 presents the final remarks and conclusions of the paper.
The following definitions are used to formulate and study the fractional order mathematical model.
We assume that α∈R+∗, b>0, f∈ACn[a,b], and n=[α]. We define the left-sided and right-sided fractional integral Riemann-Louville for f:R+⟶R, α>0 are:
IaIαtf(t):=1Γ(α)∫taf(s)ds(t−s)1−α,(Left) | (2.1) |
ItIαbf(t):=1Γ(α)∫btf(s)ds(s−t)1−α.(Right) | (2.2) |
Remark 2.1. Let's define IIαtf(t)=I0Iαtf(t).
The left-sided and right-sided Riemann–Liouville fractional derivatives are defined as [15,16]:
DaDαtf(t)=dndtn(1Γ(n−α)∫ta(t−s)n−α−1f(s)ds),(Left) | (2.3) |
DtDαbf(t)=dndtn((−1)nΓ(n−α)∫bt(s−t)n−α−1f(s)ds).(Right) | (2.4) |
Remark 2.2. Let's denote Dαtf(t)=D0Dαtf(t).
The left-sided and right-sided fractional derivatives proposed by Caputo are given by [15,16]:
DcaDαtf(t)=1Γ(n−α)∫ta(t−s)n−1−αfn(s)ds,(Left) | (2.5) |
DctDαbf(t)=(−1)nΓ(n−α)∫bt(s−t)n−1−αfn(s)ds.(Right) | (2.6) |
Remark 2.3. Let's define DcDαtf(t)=Dc0Dαtf(t).
The compartments of the model are: S the susceptible to HIV, E the exposed to the virus through sexual contact, U the cases who have the virus but are not yet diagnosed, P are the individuals who use PrEP, H the diagnosed HIV cases, A the diagnosed AIDS cases and I the cases who achieved with the use of antiretroviral therapy an undetectable viral load.
The parameter Λ is recruitment rate, as the parameter β is the cases that are transmitted by other non-sexual routes. In our model, we assume that contagion is not only sexual and is related to parameter β, which influences that our recruitment rate not only contains sexually active people but also the population in general. The parameter μ represents cases of death from natural causes and dH represents deaths associated with HIV/AIDS. The transmission rate of the HIV is defined as:
λαH=ναN(H+A+U), | (3.1) |
where ν is the contact effective rate and N is the total population. The parameter pE is the PrEP coverage in the susceptible population, pU is the cases with undiagnosed HIV/AIDS who seek to enter PrEP use without knowing their serological status, and pF is the failure rate of PrEP use, which is related to the effectiveness of the type of PrEP. The individuals not diagnosed with the virus who want to start the use of PrEP require an initial assessment of their health status, including their HIV serology and this will contribute to the diagnosis of new cases and directly affects the pD parameter which is the diagnosis of cases due to the intent to use PrEP. The parameter ϕ is the diagnosis rate of cases in a first risk contact, and ϕD is the rate of diagnosis of HIV cases. The parameters ϵD1, ϵD2 and pD are associated with the diagnosis of new HIV cases. The rate βA represents the cases of HIV that progress to AIDS and we assume that those from AIDS to HIV first achieve undetectability of the virus. The parameters iHI and iAI represent the cases that with the use of antiretroviral therapy achieve undetectability of the virus in the organism, HIV and AIDS, respectively, and iIH and iIA represent the cases that lose undetectability and reach HIV and AIDS, respectively. According to [19], instead of time−1, the fractional derivative operator DcDαt has units of time−α in such a way that on the right-hand side of the system, all parameters will have power α. Using fractional derivatives in the Caputo sense, HIV transmission with the use of antiretroviral therapy and incorporation of PrEP use is defined by the following system:
DcDαtS=Λα+pαFP−(μα+λαH+βα+pαE)S,DcDαtE=λαHS−(μα+ϵαD1)E,DcDαtU=(1−ϕα)ϵαD1E−(pαU+μα+ϵαD2+dαH)U,DcDαtP=pαES+pαUU−(μα+pαF+pαD)P,DcDαtH=ϕαϵαD1E+ϕαD(ϵαD2U+pαDP)+βαS+iαIHI−(μα+dαH+βαA+iαHI)H,DcDαtA=(1−ϕαD)(ϵαD2U+pαDP)+βαAH+iαIAI−(μα+dαH+iαAI)A,DcDαtI=iαHIH+iαAIA−(μα+dαH+iαIH+iαIA)I, | (3.2) |
with the initial condition
S(0)=S0>0,E(0)=E0>0,U(0)=U0>0,P(0)=P0>0,H(0)=H0>0,A(0)=A0>0,I(0)=I0>0 and with α∈(0,1].
Initially, we define relevant elements in epidemiology and those in our study in particular.
Prevalence is a measure of disease that allows us to determine a person's likelihood of having a disease. Therefore, the number of prevalent cases is the total number of cases of disease existing in a population [17,18].
Incidence is a measure of disease that determines the probability that a person will be diagnosed with a disease during a given time. Incidence is therefore the number of newly diagnosed cases of a disease. The incidence rate is the number of new cases of a disease divided by the number of people at risk for the disease [17,18]. Prevalence differs from incidence in that prevalence includes all cases, both new and preexisting, in the population at the specified time, whereas incidence is limited to new cases only.
The definition of mortality refers to death due to a certain epidemic or disease. The mortality rate is the number of deaths due to a disease divided by the total population [17,18].
In our model, prevalence coincides with the number of HIV and AIDS cases in the respective compartments, because by the HIV and AIDS compartments are defined in terms of the number of active cases. Regarding incidence, we decided to study the incidence of HIV, because PrEP directly affects the number of new cases of HIV, and in turn also the number of AIDS cases. This way, HIV incidence and mortality are incorporated in the model as the following:
DcDαtIm=ϕαϵαD1E+ϕαD(ϵαD2U+pαDP)+βαS, | (3.3) |
DcDαtM=dαH(U+H+A+I), | (3.4) |
where Im is the cumulative HIV numbers per year and M is the cumulative deaths per year. Then, incidence and mortality are defined as:
Im(n)=Im(n)−Im(n−1), | (3.5) |
M(n)=M(n)−M(n−1), | (3.6) |
where n is the current year and n−1 in previous year.
Basic Properties of Model
Now, let us prove the existence and positivity of the solution of Model (3.2), and let's find the biologically feasible region.
Existence and Non-Negativity of Solutions
Let's denote the feasible region by
Ω={x=(S,E,U,P,H,A,I):S,E,U,P,H,A,I≥0}. |
The following lemma and corollary will be used in the proof of Theorem (3.2) and it can be found in [19].
Lemma 3.1. (Generalized mean value theorem) Suppose that f∈C[a,b] and DcDαtf∈C[a,b], for α∈(0,1]. Then, ∀t∈(a,b], with a≤ϵ≤t,
f(t)=f(a)+1Γ(α)(DcDαtf)(ϵ)(t−a)α, |
where Γ(⋅) is the Gamma function.
Corollary 3.1. Consider that f∈C[a,b] and DcDαtf∈C[a,b], for α∈(0,1]. Then if
● DcDαtf(t)≥0, ∀t∈(a,b), then f(t) is non-decreasing for each t∈[a,b],
● DcDαtf(t)≤0, ∀t∈(a,b), then f(t) is non-increasing for each t∈[a,b].
Theorem 3.2. There is a unique solution x(t)=(S,E,U,P,H,A,I)T of Model (3.2) for t≥0 and the solution will remain in Ω.
Proof. By Theorem 3.1 and Remark 3.2 of [20], the solution in (0,∞) of the initial value problem (3.2) exists and is unique. Now, we will prove the positivity of the solution of Model (3.2). To do this, we need to prove that for every hyperplane bounding the nonnegative orthant, the vector field points to Ω. From Model (3.2):
DcDαtS|S=0=Λα+pαFP>0,DcDαtE|E=0=λαHS≥0,DcDαtU|U=0=(1−ϕα)ϵαD1E≥0,DcDαtP|P=0=pαES+pαUU≥0,DcDαtH|H=0=ϕαϵαD1E+ϕαD(ϵαD2U+pαDP)+βαS+iαIHI≥0,DcDαtA|A=0=(1−ϕαD)(ϵαD2U+pαDP)+βαAH+iαIAI≥0,DcDαtI|I=0=iαHIH+iαAIA≥0. |
As a result of the Corollary 3.1 the solution will remain in Ω.
Biologically Feasible Region
Now, let's prove that Ωα is the biologically feasible region for Model (3.2).
Lemma 3.3. The closed set Ωα={(S,E,U,P,H,A,I)∈R7+:N(t)≤Λαμα} is positively invariant concerning the Model (3.2).
Proof. The fractional derivative in the Caputo sense of the total population is
DcDαtN(t)=DcDαtS(t)+DcDαtE(t)+DcDαtU(t)+DcDαtP(t)+DcDαtH(t)+DcDαtA(t)+DcDαtI(t)=Λα−μαN(t)−dαH(U(t)+A(t)+H(t)+I(t)), |
and then
DcDαtN(t)+μαN(t)≤Λα. | (3.7) |
To continue the proof, we use the following definitions:
Definition 3.1. The Laplace transform of the Caputo fractional derivatives of the function ϕ(t) with order α>0 is defined as
L[DcDαtϕ(t)]=sαϕ(s)−n−1∑v=0ϕv(0)sα−v−1. | (3.8) |
Definition 3.2. The Laplace transform of the function tα1−1Eα,α1(±λtα) is defined as
L[tα1−1Eα,α1(±λtα)]=sα−α1sα∓λ | (3.9) |
where Eα,α1 is two-parameters Mittag-Leffler function α,α1>0. Further, the Mittag-Leffler function satisfies the following equation:
Eα,α1(f)=fEα,α+1(f)+1Γ(α1). | (3.10) |
Applying the Laplace transform to (3.7),
sαϕ(N)−sα−1ϕ(0)≤Λα−μαϕ(N), | (3.11) |
which further gives
ϕ(N)≤Λαs(sα+μ)+sα−1sα+μαN(0). | (3.12) |
Using the equations (3.8)-(3.10), we assumed that (S(0),E(0),U(0),P(0),H(0),A(0),I(0))∈R7+, then
N(t)≤(Λα)tαEα,α+1(−μαtα)+N(0)Eα,1(−μtα). | (3.13) |
Using the asymptotic behavior of the Mittag-Leffler function, we observe that N(t)→Λαμα as t→∞.
The Ωα region is well established and all solutions with initial values that belong to Ωα remain in Ωα for each time t>0.
Analogous proofs to the ones we present can be found at [21,22,23].
In a population composed only of susceptible individuals, the average number of infections caused by an infected individual is defined as basic reproduction number ℜ0.
If 0<ℜ0<1 the infection will die out in the long run and if ℜ0>1 the infection will be able to spread in a population [24]. The higher the ℜ0 the more difficult it is to control the epidemic. The ℜ0 can be affected by several factors, such as the duration of infectivity of the affected patients, the infectivity of the organism, and the degree of contact between the susceptible and infected populations.
The infection-free equilibrium point of Model (3.2) is ϵ0=(Λαμα,0,0,0,0,0,0). To compute the basic reproduction number, we use the next-generation matrix method presented in [24,25]. Using the notation in [24,25] on system (3.2), matrices for the new infection terms, F, and the other terms, V, are given by:
F=(0ΛαναμαNΛαναμαNΛαναμαN000000000000), |
V=(k1000−(1−ϕα)ϵαD1k200−ϕαϵαD1−ϕαDϵαD2k300−(1−ϕαD)ϵαD2−βαAk4). |
Then, the basic reproduction number is
ℜ0=ρ(FV−1)=|ΛαναϵαD1(k3k4(1−ϕα)+k2ϕα(k4+βαA)+ϵαD2(ϕα−1)(k3(ϕαD−1)−ϕαD(k4+βαA)))Nμαk1k2k3k4|, | (3.14) |
where k1=μα+ϵαD1, k2=μα+ϵαD2+dαH, k3=μα+dαH+βαA+iαHI, k4=μα+dαH+iαAI and ρ(FV−1) is the spectral radius of FV−1. The local stability of ϵ0 can be determined using the following theorem:
Theorem 3.4. (Theorem 2, [26]) Let α=pq where p,q∈Z+ and gdc(p,q)=1. Define M=q(p=Mα), then the disease-free equilibrium point ϵ0 of model (3.2) is asymptotically stable if [arg(λ)]>π2M for all roots λ of the following equation
det(diag[λpλpλpλpλpλpλp]−M1)=0, | (3.15) |
where M1 is the matrix of the linearization of model (3.2) at ϵ0.
This theorem can be demonstrated with analogous ideas in references [27,28,29]. From Theorem 2 of [25,29], we have the following lemma characterizing the instability with the ℜ0:
Lemma 3.5. The disease-free equilibrium point ϵ0 of Model (3.2) is unstable if ℜ0>1.
Now, using a methodology analogous to that applied to Model (3.2), we prove the global stability of the infection-free equilibrium point. Following [30], we can rewrite the Model (3.2) as
DcDαtS=F(S,J),DcDαtI=G(S,J),G(S,0)=0, | (3.16) |
where S∈R3+ is the vector with susceptibles, undectables and PrEP use and J∈R4+ have the compartments of HIV, AIDS, exposed and non-diagnosed of Model (3.2).
The infection-free equilibrium is now denoted by Dα0=(D0,0R4), where D0=(Λαμα,0,0).
The conditions (Hα1) and (Hα2) below must be satisfied to guarantee the global asymptotic stability of Dα0.
(Hα1):ForDcDαtS=F(S,0),D0is globally asymptotically stable, (Hα2):G(S,J)=AI−G∗(S,J),G∗(S,J)≥0,for(S,I)∈Ωα, | (3.17) |
where A is the Jacobian of G at (D0,0R4) is a M-matrix (the off-diagonal elements of A are non-negative) and Ωα is the biologically feasible region.
The following results show the global stability of the infection-free equilibrium point.
Theorem 3.6. The fixed point Dα0 is a globally asymptotically stable equilibrium (g.a.s) of model (3.2) provided that ℜ0<1 and that the conditions (Hα1) and (Hα2) are satisfied.
Proof. Let
F(S,0)=(ΛαpαE0). |
As F(S,0) is a linear equation, then D0 is globally stable. Then, (Hα1) is satisfied. Let
A=(−k1νανανα(1−ϕα)ϵαD1−k200ϕαϵαD1ϕαDϵαD2−k300(1−ϕαD)ϵαD2βαA−k4), |
I=(E,U,H,A), |
G∗(S,J)=AIT−G(S,J). |
G∗(S,I)=(G∗1(S,J)G∗2(S,J)G∗3(S,J)G∗4(S,J))=(να(H+A+U)(1−SN)000). |
Since SN≤1 then 1−SN≥0. Thus G∗(S,J)≥0 for all (S,J)∈Ωα. The Dα0 is globally asymptotically stable.
The analogous proofs can be found in the bibliographical references [21,22,23,28,31].
Model (3.2) can be written by the system of linear equations AX=B where:
A=(−(k0+λαH)00pαF000λαH−k1000000(1−ϕα)ϵαD1−k20000pαE0pαU−k020000ϕαϵαD1ϕαDϵαD2ϕαDpαD−k30iαIH00(1−ϕαD)ϵαD2(1−ϕαD)pαDβαA−k4iαIA0000iαHIiαAI−k04), |
X=(SEUPHAI),B=(−Λα000000), |
k0=μα+βα+pαE, k02=μα+pαF+pαD and k04=μα+dαH+iαIH+iαIA.
The determinant of the matrix A is (iαAIβαAiαIH+iαAIiαIAk3+iαHIiαIHk4−k04k3k4)(k1k2(k02(k0+λαH)−pαEpαF)+ϵαD1λαH(ϕα−1)pαFpαU). In this case, we can observe that the determinant of A is non-zero, because it is sufficient to assume that the rate of natural deaths (μ) is different from zero. This makes sense, we always have people who die from causes other than the consequences of HIV/AIDS. With that, one can say that the system has a solution and it is also unique. Such a solution would be the endemic point, which is in accordance that that in many places worldwide HIV/AIDS is endemic.
The demographic data for Brazil are extracted from [32] and the initial conditions are: S0=210147125,E0=10000,P0=64000,U0=50000,H0=41919,A0=37308,I0=0.4⋅A(0),Im(0)=77058 and D(0)=4566. These values were based on official incidence and mortality data from the Brazilian Ministry of Health [33,34].
For our study, we used that the probability of retention in clinical care after 3 months post initiation is 72.5% and the probability of full adherence to oral PrEP is 92.3% using more than 4 pills per week for oral PrEP [35]. For injectable PrEP, the probability of retention in clinical care after 2 months post-initiation is 84.8% [35] and we assume that the probability of full adherence to oral PrEP is 96.3%. This information is used to obtain the parameter value (pF) for failure to use oral and injectable PrEP, respectively. For the value of the parameter pE, we use the following information. In the year 2018 in brazil, 64 thousand people have initiated PrEP and about 24.8 thousand people have discontinued [5].
We started the implementation of oral PrEP in 2018 and studied two possibilities to maintain it throughout the study period and replace it with injectable PrEP in 2024. Studying the prevalence of HIV, we observe that the higher the fractional order (values studied) the higher the number of accumulated cases, see Figure 2a. The substitution of oral PrEP for injectable PrEP showed better results in reducing prevalence compared to maintaining oral PrEP.
Parameter | Value | Reference |
Λ | 4,590,490.56 | [32] |
β | 0.00000001 | Assumed |
μ | 1/75.50 | [32] |
ν | 2.5 | Assumed |
iAI | 0.52 | [37,38] |
ϕ | 0.65 | Assumed |
ϕD | 0.25 | Assumed |
ϵD1 | 0.007 | [2] |
ϵD2 | 0.005 | Assumed |
pD | 0.0005 | Assumed |
pU | 0.0005 | Assumed |
βA | 0.096 | [39] |
iHI | 2 | [37] |
iIA | 0.06 | [40,41,42,43] |
iIH | 0.253 | [40,41,42,43] |
dH | 0.072 | [44] |
In the case of accumulated deaths, the behavior is analogous to prevalence: the higher fractional order, the greater the number of accumulated deaths, and if we maintain oral PrEP, there are more deaths than if we substitute it for injectable PrEP, see Figure 2b.
For this study, we are evaluating the effect of PrEP but we must bear in mind that these are not the only implementations in the population. Examples of others are social assistance programs, social assistance to families with children in school ("Bolsa Família"), and the Family Health Strategy (FHS), which also have an impact on the reduction of new transmission cases [45,46,47].
Figure 2c shows the number of undiagnosed cases accumulated with HIV/AIDS. We observe that with injectable PrEP, we managed to reduce the number of undiagnosed cases. This is a relevant achievement because it allows us to maintain control of infected patients, incorporate them into antiretroviral therapies and achieve undetectable virus in blood and thus it is not a risk in the population.
Figure 3 shows the incidence and mortality rates. The calculation of incidence and mortality are given by [17,18]
Incidence Rate=Number of new cases∗100000Population size, | (4.1) |
Mortality Rate=Number of new deaths∗100000Population size. | (4.2) |
In the incidence of HIV, new cases reported annually inscreases for higher values of the fractional orders for both oral and injectable implementations (see Figure 3a). At the end of the period, with the substitution of oral PrEP, the incidence was reduced compared to the results when oral PrEP alone was maintained, resulting in a decreasing of 43.29 for α=1.0, 45.89 for α=0.9 and in 43.3 for α=0.6.
Regarding mortality, the behavior is similar (see Figure 3b). In this case, new deaths due to the new implementation of injectable PrEP are reduced in comparison to maintaining only oral PrEP; it is reduced by 31.56 for α=1.0, by 26.33 for α=0.9, and by 15.54 for α=0.6.
For incidence in that period, the reduction from injectable PrEP to oral PrEP is at [39.7,45.14] percent for α=1.0, [38.81,45.89] for α=0.9 and [40.30,44.07] for α=0.6. For mortality, the percent reduction was [6.11,31.56] for α=1.0, [4.93,26.33] for α=0.9 and for α=0.6 it was [1.1,19.06]. Table 2 shows the percent reduction per year and Figures 4a-4b show the percent reduction graphically for incidence and mortality between 2024-2035, respectively. We can conclude that with the implementation of injectable PrEP, the number of new cases and new deaths are reduced if we compare to if we maintain oral PrEP.
Incidence | Mortality | |||||
Year | 1.0 | 0.9 | 0.6 | 1.0 | 0.9 | 0.6 |
2024 | 43.79 | 41.30 | 41.56 | 6.64 | 4.93 | 1.10 |
2025 | 41.45 | 40.26 | 43.63 | 7.14 | 5.25 | 6.77 |
2026 | 43.39 | 43.08 | 42.96 | 12.10 | 5.67 | 6.41 |
2027 | 39.70 | 44.23 | 41.26 | 6.11 | 11.89 | 7.56 |
2028 | 45.14 | 40.66 | 40.37 | 15.66 | 16.52 | 8.72 |
2029 | 42.00 | 45.16 | 41.34 | 16.83 | 10.43 | 7.08 |
2030 | 40.51 | 39.83 | 43.20 | 20.52 | 21.57 | 13.83 |
2031 | 41.71 | 42.48 | 44.07 | 21.59 | 17.02 | 12.19 |
2032 | 41.61 | 43.42 | 42.40 | 24.98 | 21.38 | 11.99 |
2033 | 40.57 | 38.81 | 41.51 | 22.26 | 23.18 | 11.75 |
2034 | 44.12 | 42.31 | 42.40 | 27.73 | 18.63 | 19.06 |
2035 | 43.29 | 45.89 | 43.30 | 31.56 | 26.33 | 15.54 |
In summary, here we studied three fractional orders 0.6, 0.9, and 1.0 to study what happened to the implementations when varying the fractional order. Among the results obtained for the fractional orders, the model reported higher values in prevalence, non-diagnosed, cumulative deaths, incidence and mortality for higher values of the fractional order studied here.
In this paper, we presented a mathematical model for the study of PrEP implementation. The model takes into account the effect of antiretroviral therapy in the achievement of an undetectable viral load and of PrEP in the diagnosis of HIV cases. With the model, can be studied different health system scenarios using the respective initial condition and parameters and to address the impact of oral or oral/injectable PrEP. Besides demonstrating the existence of a solution for the referred system, we also find the biologically feasible region and study the infection-free equilibrium point as a function of the basic reproduction number. We performed computational simulations with data from Brazil, to study our model in a concrete scenario of oral and oral/injectable PrEP in Brazil, aiming to compare these strategies of prophylaxis.
With respect to the prevalence of HIV cases, for the initial conditions used in 2003 and implemented oral PrEP in 2018, the substitution of oral PrEP for injectable PrEP showed better results than maintaining oral PrEP. This also happened for cumulative deaths and undiagnosed cases, taking into account that the compartment of undiagnosed cases is hypothetical (since one does not know exactly how many undiagnosed cases there are in the population). For incidence and mortality the substitution of oral PrEP for injectable PrEP also resulted in a better strategy.
From this study, based on the values reported by the model, and the initial conditions and parameters used, we can conclude that injectable PrEP replacing oral PrEP in 2024 has a positive impact on the reduction of new cases and deaths on the diagnosis of patients with respect to maintaining the implementation of oral PrEP only. In future works, we aim to study the impact of injectable PrEP as an incorporation in the health systems and not a substitution, the impact of increased PrEP coverage in the population, stratification of the dynamics by sex and age and to advance in terms of parameter estimation.
All authors declare that they have no conflicts of interest.
[1] |
I. Boniphace, M. Omari, R. S. Fred, M. Ferdinand, T. Marcel, HIV/AIDS, Clinical Manifestations and their Implication for Patient Clinical Staging in Resource Limited Settings in Tanzania, The Open AIDS Journal, 5 (2011), 9–16. https://doi.org/10.2174/1874613601105010009 doi: 10.2174/1874613601105010009
![]() |
[2] | UNAIDS, Global HIV and AIDS statistics, 2022. Available from: https://www.unaids.org/en/resources/fact-sheet |
[3] | A. B. D'Angelo, D. A. Westmoreland, P. B. Carneiro, J. Johnson, C. Grov, Why Are Patients Switching from Tenofovir Disoproxil Fumarate/Emtricitabine (Truvada) to Tenofovir Alafenamide/Emtricitabine (Descovy) for Pre-Exposure Prophylaxis? AIDS Patient Care STDS, 35 (2021), 327–334. https://doi.org/10.1089/apc.2021.0033 |
[4] |
R. Schaefer, H. M. A. Schmidt, G. Ravasi, A. Mozalevskis, B. B. Rewari, F. Lule, et al., Adoption of guidelines on and use of oral pre-exposure prophylaxis: a global summary and forecasting study, The LANCET HIV, 8 (2021), e502–e510. https://doi.org/10.1016/S2352-3018(21)00127-2 doi: 10.1016/S2352-3018(21)00127-2
![]() |
[5] | P.M. Luz, A. Benzaken, T. M. de Alencar, C. Pimenta, V. G. Veloso, B. Grinsztejn, PrEP adopted by the brazilian national health system: What is the size of the demand? Medicine (Baltimore), 97 (2018), S75–S77. https://doi.org/10.1097/MD.0000000000010602 |
[6] | M. Montess, D. Tan, N. J. Lachowsky, Special to Western News, Expert insight: Long-acting injectable PrEP is a big step forward in HIV prevention. The HIV prevention drug cabotegravir is not yet available in Canada, 2021. Available from: https://news.westernu.ca/2022/12/expert-insight-long-acting-injectable-prep-is-a-big-step-forward-in-hiv-prevention/ |
[7] | WHO, WHO recommends long-acting cabotegravir for HIV prevention. New WHO guidelines advise countries to deliver long-acting cabotegravir as part of comprehensive approach to HIV prevention, 2022. Available from: https://www.who.int/news/item/28-07-2022-who-recommends-long-acting-cabotegravir-for-hiv-prevention#:~:text=WHO%20today%20released%20new%20guidelines%20for%20the%20use,for%20people%20at%20substantial%20risk%20of%20HIV%20infection. |
[8] |
E. M. D. Moya, D. S. Rodrigues, A. Pietrus, A. M. Severo, A Mathematical Model for HIV/AIDS Under Pre-Exposure and Post-Exposure Prophylaxis, BIOMATH, 11 (2022), 1–28. https://doi.org/10.55630/j.biomath.2022.08.319 doi: 10.55630/j.biomath.2022.08.319
![]() |
[9] |
S. B. Kim, M. Yoon, N. S. Ku, M. H. Kim, J. E. Song, J. Y. Ahn, et al., Mathematical modeling of HIV prevention measures including pre-exposure prophylaxis on HIV incidence in South Korea, PLoS One, 9 (2014), 537–553. https://doi.org/10.1371/journal.pone.0090080 doi: 10.1371/journal.pone.0090080
![]() |
[10] |
M. U. N. Nsuami, P. J. Witbooi, A stochastic model for HIV with the use of PrEP, J. Math. Model., 9 (2021), 537–553. https://doi.org/10.22124/JMM.2021.16870.1461 doi: 10.22124/JMM.2021.16870.1461
![]() |
[11] |
C. J. Silva, D. F. M. Torres, Modeling and optimal control of HIV/AIDS prevention through PrEP, Discrete and Continuous Dynamical Systems, 11 (2018), 119–141. https://doi.org/10.1016/j.physa.2018.10.033 doi: 10.1016/j.physa.2018.10.033
![]() |
[12] | K. Diethelm, The Analysis of Fractional Differential Equations, 1 Eds., Springer-Verlag Berlin Heidelberg, 2004. |
[13] |
L. Carvalho de Barros, M. M. Lopes, F. S. Pedro, E. Esmi, J. P. C. D. Santos, D. E. Sánchez, The memory effect on fractional calculus: an application in the spread of COVID-19, Comput. Appl. Math., 40 (2021), 72. https://doi.org/10.1007/s40314-021-01456-z doi: 10.1007/s40314-021-01456-z
![]() |
[14] |
M. Saeedian, M. Khalighi, N. Azimi-Tafreshi, G. R. Jafari, M. Ausloos, Memory effects on epidemic evolution: The susceptible-infected-recovered epidemic model, Phys. Rev. E, 95 (2017), 022409. https://doi.org/10.1103/PhysRevE.95.022409 doi: 10.1103/PhysRevE.95.022409
![]() |
[15] | R. Figueiredo Camargo, E. Capelas de Oliveira, Cálculo fracionário, 1 Eds., Brasil: Livraria da Fısica, Sao Paulo, 2015. |
[16] |
H. Kheiri, M. Jafari, Optimal control of a fractional order model for the HIV/AIDS epidemic, Int. J. Biomath., 7 (2018), 1850086. https://doi.org/10.1142/S1793524518500869 doi: 10.1142/S1793524518500869
![]() |
[17] | B. B. Gerstman, Epidemiology Kept Simple: An Introduction to Traditional and Modern Epidemiology, 2 Eds., Hoboken, NJ: Wiley-Liss, 2003. |
[18] | K. J. Rothman, Epidemiology: An Introduction, Oxford University Press, 2012. |
[19] |
K. Diethelm, A fractional calculus based model for the simulation of an outbreak of dengue fever, Nonlinear Dynamics, 71 (2013), 613–619. https://doi.org/10.1007/s11071-012-0475-2 doi: 10.1007/s11071-012-0475-2
![]() |
[20] |
W. Lin, Global existence theory and chaos control of fractional differential equations, J. Math. Anal. Appl., 332 (2007), 709–726. https://doi.org/10.1016/j.jmaa.2006.10.040 doi: 10.1016/j.jmaa.2006.10.040
![]() |
[21] |
E. D. Moya, A Pietrus, S. M. Oliva, Mathematical model with fractional order derivatives for Tuberculosis taking into account its relationship with HIV/AIDS and Diabetes, Jambura Journal of Biomathematics, 2 (2021), 80–95. https://doi.org/10.34312/jjbm.v2i2.11553 doi: 10.34312/jjbm.v2i2.11553
![]() |
[22] |
C. M. A. Pinto, A. R. M. Carvalho, Diabetes mellitus and TB co-existence: Clinical implications from a fractional order modelling, Appl. Math. Model., 68 (2019), 219–243. https://doi.org/10.1016/j.apm.2018.11.029 doi: 10.1016/j.apm.2018.11.029
![]() |
[23] |
C. M. A. Pinto, A. R. M. Carvalho, The HIV/TB coinfection severity in the presence of TB multi-drug resistant strains, Ecol. Complex., 32 (2019), 1–20. https://doi.org/10.1016/j.ecocom.2017.08.001 doi: 10.1016/j.ecocom.2017.08.001
![]() |
[24] |
O. Diekmann, J. A. P. Heesterbeek, M. G. Roberts, The construction of next-generation matrices for compartmental epidemic models, J. R. Soc. Interface, 7 (2010), 873–885. https://doi.org/10.1098/rsif.2009.0386 doi: 10.1098/rsif.2009.0386
![]() |
[25] |
P. van den. Driessche, J. Watmough, Reproduction numbers and sub-threshold endemic equilibria for compartmental models of disease transmission, Math. Biosci., 180 (2003), 29–48. https://doi.org/10.1016/S0025-5564(02)00108-6 doi: 10.1016/S0025-5564(02)00108-6
![]() |
[26] |
D. Valério, A.M. Lopes, J. A. Tenreiro Machado, Entropy analysis of a railway network's complexity, Entropy, 18 (2016), 388. https://doi.org/10.3390/e18110388 doi: 10.3390/e18110388
![]() |
[27] |
Fatmawati, M. A. Khan, E. Bonyah, Z. Hammouch, E. M. Shaiful, A mathematical model of tuberculosis (TB) transmission with children and adults groups: A fractional model, AIMS Math., 5 (2020), 2813–2842. https://doi.org/10.3934/math.2020181 doi: 10.3934/math.2020181
![]() |
[28] |
C. M. A. Pinto, A. R. M. Carvalho, A latency fractional order model for HIV dynamics, J. Comput. Appl. Math., 312 (2017), 240–256. https://doi.org/10.1016/j.cam.2016.05.019 doi: 10.1016/j.cam.2016.05.019
![]() |
[29] |
A. R. M. Carvalho, C. M. A. Pinto, D. Baleanu, HIV/HCV coinfection model: a fractional-order perspective for the effect of the HIV viral load, Adv. Differ. Equations, 2018 (2018), 1–22. https://doi.org/10.1186/s13662-017-1456-z doi: 10.1186/s13662-017-1456-z
![]() |
[30] | C. Castillo-Chavez, Z. Feng, W. Huang, On the computation of ℜ0 and its role on global stability. In: Mathematical Approaches for Emerging and Reemerging Infectious Diseases: An Introduction. Springer-Verlag, New York, 2002. |
[31] | A. R. M. Carvalho, C. M. A. Pinto, Non-integer order analysis of the impact of diabetes and resistant strains in a model for TB infection, Commun. Nonlinear Sci. Numer. Simulat., 61 (2018), 104–126. |
[32] | Projeção da população, 2022. Available from: https://www.ibge.gov.br/apps/populacao/projecao\/index.html |
[33] | Secretaria de Vigilância em saúde-Ministêria da Saúde, Boletim Epidemiológico-HIV/AIDS, 2018. Available from: https://www.saude.df.gov.br/documents/37101\/87308/Boletim-AIDS-IST-2018.pdf/d8af8a94-6af7-f3b8-b6ba-7165108b759c?t=1648581629043 |
[34] | Secretaria de Vigilância em saúde-Ministêria da Saúde, Boletim Epidemiológico-HIV/AIDS, 2019. Available from: https://www.gov.br/saude/pt-br/centrais-de\-conteudo/publicacoes/boletins/epidemiologicos/especiais\/2019/boletim-epidemiologico-especial-hiv-aids-2019/view |
[35] |
B. D. L. Marshall, W. C. Goedel, M. R. King, A. Singleton, D. P. Durham, P. A. Chan, Potential effectiveness of long-acting injectable pre-exposure prophylaxis for HIV prevention in men who have sex with men: A modelling study, Lancet HIV, 5 (2018), e498–e505. https://doi.org/10.1016/S2352-3018(18)30097-3 doi: 10.1016/S2352-3018(18)30097-3
![]() |
[36] |
A. K. P. Barbosa, P. Monteiro, D. Montenegro, J. D. A. L. Batista, U. R. Montarroyos, HIV Pre-Exposure Prophylaxis (PrEP) in a Brazilian Clinical Setting: Adherence, Adverse Events, Sexual Behavior, and Sexually Transmitted Infections, Arch. Sex. Behav., 21 (2007), 2603–2611. https://doi.org/10.1007/s10508-021-02112-7 doi: 10.1007/s10508-021-02112-7
![]() |
[37] |
J. H. Ali, T. G. Yirtaw, Time to viral load suppression and its associated factors in cohort of patients taking antiretroviral treatment in East Shewa zone, Oromiya, Ethiopia, 2018, BMC Infect. Dis., 19 (2019), 1–6. https://doi.org/10.1186/s12879-019-4702-z doi: 10.1186/s12879-019-4702-z
![]() |
[38] | CDC, Evidence of HIV Treatment and Viral Suppression in Preventing the Sexual Transmission of HIV, BMC Infect. Dis., 19 (2020), 1–6. |
[39] | HIV/AIDS, 2022. Available from: https://www.who.int/news-room/questions-and-answers/item/hiv-aids |
[40] |
X. Leng, S. Liang, Y. Ma, Y. Dong, W. Kan, D. Goan, et al., HIV virological failure and drug resistance among injecting drug users receiving first-line ART in China, BMJ Open, 4 (2014), e005886. http://dx.doi.org/10.1136/bmjopen-2014-005886 doi: 10.1136/bmjopen-2014-005886
![]() |
[41] |
K. Jobanputra, L. A. Parker, C. Azih, V. Okello, G. Maphalala, B. Kershberger, et al., Factors Associated with Virological Failure and Suppression after Enhanced Adherence Counselling, in Children, Adolescents and Adults on Antiretroviral Therapy for HIV in Swaziland, PLoS One, 10 (2015), e0116144. https://doi.org/10.1371/journal.pone.0116144 doi: 10.1371/journal.pone.0116144
![]() |
[42] |
A. S. Hassan, H. M. Nabwera, S. M. Mwaringa, C. A. Obonyo, E. J. Sanders, T. F. Rinke de Wit, et al., HIV-1 virologic failure and acquired drug resistance among first-line antiretroviral experienced adults at a rural HIV clinic in coastal Kenya: A cross-sectional study, AIDS Res. Ther., 11 (2014), 1–12. https://doi.org/10.1186/1742-6405-11-9 doi: 10.1186/1742-6405-11-9
![]() |
[43] |
M. Rupérez, C. Pou, S. Maculuve, S. Cedeño, L. Luis, J. Rodríguez, et al., Determinants of virological failure and antiretroviral drug resistance in Mozambique, J. Antimicrob. Chemoth., 70 (2015), 2639–2647. https://doi.org/10.1093/jac/dkv143 doi: 10.1093/jac/dkv143
![]() |
[44] |
J. Todd, J. R. Glynn, M. Marston, T. Lutalo, S. Biraro, W. Mwita, et al., Time from HIV seroconversion to death: a collaborative analysis of eight studies in six low and middle-income countries before highly active antiretroviral therapy, AIDS, 21 (2007), S55. https://doi.org/10.1097/01.AIDS.0000299411.75269.E8 doi: 10.1097/01.AIDS.0000299411.75269.E8
![]() |
[45] | Ministério do Desenvolvimento Social e Agrário, Bolsa Família: como funciona. Assistência Social, 2015. Available from: http://mds.gov.br/assuntos/bolsafamilia/o-que-e/como-funciona |
[46] | Ministério do Desenvolvimento Social e Combate à Fome. Secretaria Nacional de Renda de Cidadania, Programa Bolsa Família: uma década de inclusão e cidadania, 2013. Available from: http://www.ipea.gov.br/portal/images/stories/PDFs/livros\/livros/livro−bolsafamilia−10anos.pdf |
[47] |
M. L. Bastos, D. Menzies, T. Hone, K. Dehghani, A. Trajman, The impact of the Brazilian family health strategy on selected primary care sensitive conditions: a systematic review, PLoS One, 12 (2017), e0182336. https://doi.org/10.1371/journal.pone.0189557 doi: 10.1371/journal.pone.0189557
![]() |
Parameter | Value | Reference |
Λ | 4,590,490.56 | [32] |
β | 0.00000001 | Assumed |
μ | 1/75.50 | [32] |
ν | 2.5 | Assumed |
iAI | 0.52 | [37,38] |
ϕ | 0.65 | Assumed |
ϕD | 0.25 | Assumed |
ϵD1 | 0.007 | [2] |
ϵD2 | 0.005 | Assumed |
pD | 0.0005 | Assumed |
pU | 0.0005 | Assumed |
βA | 0.096 | [39] |
iHI | 2 | [37] |
iIA | 0.06 | [40,41,42,43] |
iIH | 0.253 | [40,41,42,43] |
dH | 0.072 | [44] |
Incidence | Mortality | |||||
Year | 1.0 | 0.9 | 0.6 | 1.0 | 0.9 | 0.6 |
2024 | 43.79 | 41.30 | 41.56 | 6.64 | 4.93 | 1.10 |
2025 | 41.45 | 40.26 | 43.63 | 7.14 | 5.25 | 6.77 |
2026 | 43.39 | 43.08 | 42.96 | 12.10 | 5.67 | 6.41 |
2027 | 39.70 | 44.23 | 41.26 | 6.11 | 11.89 | 7.56 |
2028 | 45.14 | 40.66 | 40.37 | 15.66 | 16.52 | 8.72 |
2029 | 42.00 | 45.16 | 41.34 | 16.83 | 10.43 | 7.08 |
2030 | 40.51 | 39.83 | 43.20 | 20.52 | 21.57 | 13.83 |
2031 | 41.71 | 42.48 | 44.07 | 21.59 | 17.02 | 12.19 |
2032 | 41.61 | 43.42 | 42.40 | 24.98 | 21.38 | 11.99 |
2033 | 40.57 | 38.81 | 41.51 | 22.26 | 23.18 | 11.75 |
2034 | 44.12 | 42.31 | 42.40 | 27.73 | 18.63 | 19.06 |
2035 | 43.29 | 45.89 | 43.30 | 31.56 | 26.33 | 15.54 |
Parameter | Value | Reference |
Λ | 4,590,490.56 | [32] |
β | 0.00000001 | Assumed |
μ | 1/75.50 | [32] |
ν | 2.5 | Assumed |
iAI | 0.52 | [37,38] |
ϕ | 0.65 | Assumed |
ϕD | 0.25 | Assumed |
ϵD1 | 0.007 | [2] |
ϵD2 | 0.005 | Assumed |
pD | 0.0005 | Assumed |
pU | 0.0005 | Assumed |
βA | 0.096 | [39] |
iHI | 2 | [37] |
iIA | 0.06 | [40,41,42,43] |
iIH | 0.253 | [40,41,42,43] |
dH | 0.072 | [44] |
Incidence | Mortality | |||||
Year | 1.0 | 0.9 | 0.6 | 1.0 | 0.9 | 0.6 |
2024 | 43.79 | 41.30 | 41.56 | 6.64 | 4.93 | 1.10 |
2025 | 41.45 | 40.26 | 43.63 | 7.14 | 5.25 | 6.77 |
2026 | 43.39 | 43.08 | 42.96 | 12.10 | 5.67 | 6.41 |
2027 | 39.70 | 44.23 | 41.26 | 6.11 | 11.89 | 7.56 |
2028 | 45.14 | 40.66 | 40.37 | 15.66 | 16.52 | 8.72 |
2029 | 42.00 | 45.16 | 41.34 | 16.83 | 10.43 | 7.08 |
2030 | 40.51 | 39.83 | 43.20 | 20.52 | 21.57 | 13.83 |
2031 | 41.71 | 42.48 | 44.07 | 21.59 | 17.02 | 12.19 |
2032 | 41.61 | 43.42 | 42.40 | 24.98 | 21.38 | 11.99 |
2033 | 40.57 | 38.81 | 41.51 | 22.26 | 23.18 | 11.75 |
2034 | 44.12 | 42.31 | 42.40 | 27.73 | 18.63 | 19.06 |
2035 | 43.29 | 45.89 | 43.30 | 31.56 | 26.33 | 15.54 |