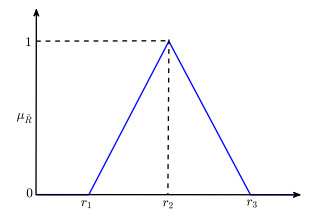
This work aims to develop an approximate analytical solution for the geophysical Korteweg-de Vries (GeoKdV) equation with time-fractional derivatives defined in the Caputo sense. This equation is relevant to shallow water wave (SWW) propagation, which may have uses in mathematical physics and engineering sciences. In real-world scenarios, factors such as environmental or climate changes or the dynamics of air and water waves introduce uncertainty or ambiguity into parameters like the Coriolis effect and initial or boundary conditions. Unlike previous studies that solely focused on either integer-order or fractional-order models, this research introduces fractional dynamics with fuzzy uncertainty. To deal with such uncertainty, this work aims to find the approximate fuzzy solution to the said physical problem by applying a double parametric approach with the help of an effective method called the fractional reduced differential transform method (FRDTM). This approach has been shown to be highly effective, thereby efficiently addressing both fractional calculus and fuzzy initial conditions. Furthermore, to validate the obtained solution, we conduct a comparison between the special case of the current fuzzy solution, thereby considering the fractional order of the index with the existing precise (crisp) solutions of the integer order governing equation. The solutions are presented in both fuzzy and precise formats, and graphical representations are provided to enhance the understanding of their physical significance across various parameter values.
Citation: Mrutyunjaya Sahoo, Dhabaleswar Mohapatra, S. Chakraverty. Wave solution for time fractional geophysical KdV equation in uncertain environment[J]. Mathematical Modelling and Control, 2025, 5(1): 61-72. doi: 10.3934/mmc.2025005
[1] | Abduljawad Anwar, Shayma Adil Murad . On the Ulam stability and existence of $ L^p $-solutions for fractional differential and integro-differential equations with Caputo-Hadamard derivative. Mathematical Modelling and Control, 2024, 4(4): 439-458. doi: 10.3934/mmc.2024035 |
[2] | Lusong Ding, Weiwei Sun . Neuro-adaptive finite-time control of fractional-order nonlinear systems with multiple objective constraints. Mathematical Modelling and Control, 2023, 3(4): 355-369. doi: 10.3934/mmc.2023029 |
[3] | Iman Malmir . Novel closed-loop controllers for fractional nonlinear quadratic systems. Mathematical Modelling and Control, 2023, 3(4): 345-354. doi: 10.3934/mmc.2023028 |
[4] | Zhenguo Luo, Liping Luo . New criteria for oscillation of damped fractional partial differential equations. Mathematical Modelling and Control, 2022, 2(4): 219-227. doi: 10.3934/mmc.2022021 |
[5] | Muhammad Nawaz Khan, Imtiaz Ahmad, Mehnaz Shakeel, Rashid Jan . Fractional calculus analysis: investigating Drinfeld-Sokolov-Wilson system and Harry Dym equations via meshless procedures. Mathematical Modelling and Control, 2024, 4(1): 86-100. doi: 10.3934/mmc.2024008 |
[6] | Kexin Ouyang, Xinmin Qu, Huiqin Lu . Sign-changing and signed solutions for fractional Laplacian equations with critical or supercritical nonlinearity. Mathematical Modelling and Control, 2025, 5(1): 1-14. doi: 10.3934/mmc.2025001 |
[7] | Anil Chavada, Nimisha Pathak, Sagar R. Khirsariya . A fractional mathematical model for assessing cancer risk due to smoking habits. Mathematical Modelling and Control, 2024, 4(3): 246-259. doi: 10.3934/mmc.2024020 |
[8] | Ihtisham Ul Haq, Nigar Ali, Hijaz Ahmad . Analysis of a chaotic system using fractal-fractional derivatives with exponential decay type kernels. Mathematical Modelling and Control, 2022, 2(4): 185-199. doi: 10.3934/mmc.2022019 |
[9] | Oluwatayo Michael Ogunmiloro . A fractional order mathematical model of teenage pregnancy problems and rehabilitation in Nigeria. Mathematical Modelling and Control, 2022, 2(4): 139-152. doi: 10.3934/mmc.2022015 |
[10] | M. Sathish Kumar, M. Deepa, J Kavitha, V. Sadhasivam . Existence theory of fractional order three-dimensional differential system at resonance. Mathematical Modelling and Control, 2023, 3(2): 127-138. doi: 10.3934/mmc.2023012 |
This work aims to develop an approximate analytical solution for the geophysical Korteweg-de Vries (GeoKdV) equation with time-fractional derivatives defined in the Caputo sense. This equation is relevant to shallow water wave (SWW) propagation, which may have uses in mathematical physics and engineering sciences. In real-world scenarios, factors such as environmental or climate changes or the dynamics of air and water waves introduce uncertainty or ambiguity into parameters like the Coriolis effect and initial or boundary conditions. Unlike previous studies that solely focused on either integer-order or fractional-order models, this research introduces fractional dynamics with fuzzy uncertainty. To deal with such uncertainty, this work aims to find the approximate fuzzy solution to the said physical problem by applying a double parametric approach with the help of an effective method called the fractional reduced differential transform method (FRDTM). This approach has been shown to be highly effective, thereby efficiently addressing both fractional calculus and fuzzy initial conditions. Furthermore, to validate the obtained solution, we conduct a comparison between the special case of the current fuzzy solution, thereby considering the fractional order of the index with the existing precise (crisp) solutions of the integer order governing equation. The solutions are presented in both fuzzy and precise formats, and graphical representations are provided to enhance the understanding of their physical significance across various parameter values.
Nonlinear evolution equations (NLEEs) have gained a significant importance in engineering and mathematical sciences, thereby influencing diverse fields such as nuclear physics, plasma physics, kinematics, chemistry, biology, bioengineering, mechanics, electromagnetism, nonlinear optics, mathematical biosciences, and so on. These equations are essential to describe complex scientific phenomena, enabling the modeling and analysis of dynamical systems with differential equations. In particular, mathematicians have made substantial progress in understanding the dynamics of nonlinear partial differential equations (NPDEs), particularly the soliton aspect, thus contributing valuable methods and insights. The exploration of physical behaviors within this framework not only enhances the understanding, but also opens doors to innovative applications, potentially leading to practical breakthroughs in various scientific domains.
A specific class of NLEEs includes the shallow water equations, a set of hyperbolic partial differential equations (PDEs), which have been widely used to model various real-world phenomena governed by PDEs of an arbitrary order. One prominent example of such an equation is the Korteweg-de Vries (KdV) equation, which can be expressed as follows:
ητ+ηηξ+ηξξξ=0. | (1.1) |
This equation was introduced by Korteweg and de Vries in [1], which describes the evolution of small amplitude, long waves in shallow water, where factors such as depth and bottom topography influence the wave speed, thus leading to changes in wave profiles. The KdV equation, precisely solvable with suitable initial data, has captivated widespread interest among researchers for its significance in understanding phenomena like wave breaking and dispersion.
In contrast to the KdV equation, Geyer et al. [2] introduced a nonlinear equation to analyze single-layer oceanic fluids in equatorial regions by incorporating the effects of the rotatory motion of Earth on the fluid, commonly referred to as the Coriolis effect. This formulation, termed the geophysical KdV (GeoKdV) equation, contributes to a more comprehensive understanding of oceanic phenomena, and can be written as follows:
ητ−ωηξ+32ηηξ+16ηξξξ=0. | (1.2) |
Here, η is a function of (ξ,τ) and represents the free surface elevation, and ω is the Coriolis parameter.
In recent years, several authors have explored various aspects of the GeoKdV equation. For instance, Turgut et al. [3] investigated the Coriolis effect on oceanic flows in the equatorial region using Lie symmetries and implemented the finite element method for numerical solutions. Rizvi et al. [4] employed the Hirota bilinear method to obtain lump-kink and lump-periodic solutions. The solitons and complexions of the GeoKdV equation were derived using Kudryashov and Hirota methods, and the impact of the Coriolis parameter on nonlinear waves was studied by Hosseini et al. [5]. Additionally, Alharbi and Almatraf [6] investigated the GeoKdV equation by utilizing an improved exp-expansion method, and obtained various solitary solutions. Additionally, they applied shooting and adaptive moving methods to compute numerical solutions and used Fourier analysis to evaluate the accuracy and stability of these numerical schemes. In recent years, Sayed et al. [7] explored the modified and generalized forms of the GeoKdV equation. They utilized the extended Tanh method and the generalized Kudryashov method with the Riccati equation to derive sine and cosine hyperbolic solutions.
To address the limitations of integer order operators, which are localized and incapable of predicting complex behaviors, various mathematicians and scientists have introduced non-integer order operators. In recent years, scientific research has increasingly focused on exploring more complex changes through fractional calculus due to its non-local properties and memory-retaining characteristics. This shift has led to significant growth in its applications across various disciplines, including mathematics, physics, engineering, biotechnology, electrochemistry, and more; see [8,9,10,11,12,13,14,15,16]. However, despite considerable progress, obtaining precise or numerical solutions for models that involve fractional calculus remains a significant challenge. Addressing these complexities requires innovative approaches, as many existing methodologies in the literature employ nonlinear fractional differential equations (FDEs) to capture the behavior of physical phenomena within dynamic systems. In this regard, Karunakar and Chakraverty [17] utilized the homotopy perturbation method (HPM) to solve time-fractional third- and fifth-order KdV equations. Shah et al. [18] utilized the Mohand decomposition method with Caputo derivatives to tackle fractional and integer-order KdV equations, demonstrating that fractional-order solutions gradually converge to their integer-order counterparts. Similarly, Jena and Chakraverty [19] efficiently obtained solitary wave solutions for generalized Hirota-Satsuma coupled KdV and MKdV equations. Al-Sawalha et al. [20] made further advancements by applying a natural decomposition method alongside Atangana-Baleanu and Caputo-Fabrizio fractional derivatives to obtain approximate solutions for the Kersten-Krasil'shchik coupled KdV and modified KdV systems. Cao et al. [21] explored variational principles for two types of nonlinear GeoKdV equations with fractal derivatives. In recent times, Shehzada et al. [22] delved into a modified form of the fractional GeoKdV equation with a power-law kernel. These studies collectively highlight the growing importance of fractional calculus to solve various types of KdV equations, thus demonstrating its potential to provide deeper insights into dynamic systems.
On the contrary, the information about the parameters involved in shallow water wave equations (SWWEs) is often incomplete. To address this, fuzzy parameters can be incorporated into the SWWEs. Zadeh [23] introduced the fuzzy set theory in 1965, offers a mathematical framework to handle uncertainty and imprecision. Unlike the classical set theory, where an element either fully belongs or does not belong to a set, fuzzy sets allow for partial membership, with elements belonging to a set to varying degrees, typically represented by values between 0 and 1. This approach is especially useful in cases where boundaries between the categories are not clearly defined, such as when dealing with linguistic variables or ambiguous data. In our study, we apply the fuzzy set theory to address the uncertainty present either in system parameters or initial conditions.
By representing uncertain variables as fuzzy numbers, we capture a range of possible values rather than relying on a single deterministic value. This approach increases the robustness of our model and provides a more comprehensive understanding of the system behavior, particularly in situations where precise information is unavailable. In this regard, several authors have also applied the fuzzy set theory to water wave equations, thus demonstrating its effectiveness in capturing uncertainties in such complex systems. As such, the behavior of two-dimensional SWWEs with fuzzy parameters was investigated by Karunakar and Chakraverty [24] using the HPM, treating water depth as uncertain and expressed in terms of fuzzy parameters. Sahoo and Chakraverty [25] introduced the Sawi transform-based HPM as a hybrid approach to approximate solutions to one-dimensional SWWEs in tsunami predictions. Additionally, they applied HPM to solve coupled SWWEs, considering the wave height as fuzzy [26]. Using this approach, the impact of the coastal slope and the sea depth on tsunami wave characteristics, particularly wave velocity and run-up height, was analyzed in uncertain environments. Extending the study of fuzzy uncertainty in these fields, they further derived an approximate solution for the GeoKdV equation by using the Adomian decomposition method within the fuzzy environment [27].
To the best of our knowledge, the proposed model has not been explored within the domain of fractional calculus and fuzzy environment. Drawing inspiration from valuable contributions in fractional calculus and fuzzy uncertainty, we aim to investigate the uncertain GeoKdV equation in the fractional order of Caputo's sense by using the fractional reduced differential transform method (FRDTM). Consequently, we consider the GeoKdV Eq (1.2) in the fuzzy fractional form as:
˜ηατ−ω˜ηξ+32˜η˜ηξ+16˜ηξξξ=0. | (1.3) |
This study is motivated by the desire to understand the intricate behaviors exhibited by the nonlinear GeoKdV equation in the fuzzy environment and to investigate the effect of the fractional order on its dynamics. Unlike traditional methods, the FRDTM simplifies the complex dynamics of the GeoKdV equation under uncertain and fractional-order conditions, offering an improved accuracy and an ease of implementation. This study not only demonstrates the effectiveness of the FRDTM through multiple case analyses, but also establishes strong agreements between simulated results and provided data, highlighting the robustness of the method. These results confirm that the FRDTM technique is a reliable method for addressing fractional-order nonlinear problems.
The subsequent part of this article is structured as follows: Section 2 covers the fundamental concepts of fuzzy set theory and fractional calculus; in Section 3, the methodology is discussed, with a detailed analysis of the FRDTM; Section 4 aims to provide a comprehensive understanding of application of the FRDTM to solve the time fractional fuzzified GeoKdV equation; in Section 5, results and discussion are presented, where the research outcomes are thoroughly analyzed and interpreted; and finally, Section 6 offers a conclusive summary of the findings and insights for future research directions.
In our subsequent discussions, we will look at some fundamental concepts related to fuzzy and fractional calculus.
Definition 2.1. [23,28,29] A fuzzy number denoted as ˜S, is a type of fuzzy set defined on the real line R. adhering to the conditions of convexity and normality. It is characterized by a piecewise continuous membership function μ˜S(u) that satisfies the following:
{μ˜S(u):R→[0,1],∀u∈R}. |
Definition 2.2. [23,28,29] A triangular fuzzy number (TFN) ˜R can be represented as a triplet
˜R=(r1,r2,r3), r1≤r2≤r3. |
It falls within the classification of triangular-shaped fuzzy numbers, as shown in Figure 1. The membership function μ˜R(u) of a TFN ˜R may be stated as follows:
μ˜R(u)={0,u<r1,u−r1r2−r1,r1≤u≤r2,r3−ur3−r2,r2≤u≤r3,0,u>r3. | (2.1) |
Definition 2.3. [23,28,29] Consider the following TFN
˜R=(r1,r2,r3). |
Utilizing the p-cut approach, it may be transformed into a fuzzy interval representation as follows:
˜Rp=[˜Rl,˜Ru]=[r1+p(r2−r1),r3−p(r3−r2)], | (2.2) |
where p∈[0,1].
Definition 2.4. [23,28,29] By applying the (p,r)-cut technique, the above Eq (2.2) may be expressed in a double parametric form (DPF) as follows:
˜R(p,r)=r[˜Ru−˜Rl]+˜Rl, p,r∈[0,1]. | (2.3) |
Definition 2.5. [23,28,29] For a function ˜f(t;p,r), with fuzzy values, the fuzzy fractional Riemann-Liouville operator of order α (i.e. Jtα) is defined as follows:
Jtα˜f(t;p,r)=1Γ(α)∫t0(t−x)α−1˜f(x;p,r)dx, | (2.4) |
where t>0, α>0.
Definition 2.6. [23,28,29] For a fuzzy valued function ˜f(t;p,r) based on its DPF, the fuzzy fractional differential operator Dtα in the Riemann-Liouville sense is interpreted as follows:
Dtα˜f(t;p,r)={1Γ(m−α)dmdtm∫t0˜f(x)(t−x)α−m+1dx,m−1<α≤m,m∈N,dmdtm˜f(t;p,r), α=m,m∈N. | (2.5) |
In Caputo's sense, the fuzzy fractional differential operator Dtα can be interpreted as follows:
Dtα˜f(t;p,r)={1Γ(m−α)∫t0˜f(m)(x;p,r)(t−x)α−m+1dx,m−1<α≤m,m∈N,dmdtm˜f(t;p,r), α=m,m∈N. | (2.6) |
To enhance the understanding of the FRDTM, we will start by looking over the local fractional Taylor's theorem. Moreover, this theorem has been extended to incorporate fractional derivatives, thus establishing a thorough foundation for applying the FRDTM.
Assuming that
ψ(k+1)α∈Cα(s,v) |
for k=0,1,…,n and 0<α≤1, we may represent the relationship in the following manner:
ψ(ξ)=∞∑k=0ψ(kα)(ξ0)(ξ−ξ0)kαΓ(1+kα), | (3.1) |
where
s<ξ0<ξ<v,∀ξ∈(s,v) |
and
ψ(k+1)α(ξ)=DξαDξα…(k+1 times )ψ(ξ). |
Definition 3.1. [30,31] For an analytic function ψ(ξ,τ), the fractional reduced differential transform denoted as ψk(ξ) can be expressed as follows:
ψk(ξ)=1Γ(1+kα)(∂αkψ(ξ,τ)∂ταk)τ=τ0, | (3.2) |
where k=0,1,…n.
Definition 3.2. [30,31] The fractional inverse differential transform of ψk(ξ) can be expressed as follows:
ψ(ξ,τ)=∞∑r=0ψk(ξ)(τ−τ0)αk. | (3.3) |
The following results can be drawn from Definitions 3.2 and 3.3:
ψ(ξ,τ)=∞∑r=01Γ(1+kα)(∂αkψ(ξ,τ)∂ταk)τ=τ0(τ−τ0)αk. | (3.4) |
For a specific time τ0=0, we have
ψ(ξ,τ)=∞∑r=0ψk(ξ)ταk=∞∑r=01Γ(1+kα)(∂αkψ(ξ,τ)∂ταk)τ=0ταk. | (3.5) |
Let f(ξ,τ), g(ξ,τ), and h(ξ,τ) be three analytical functions such that
f(ξ,τ)=RD−1[fk(ξ)],g(ξ,τ)=RD−1[gk(ξ)],h(ξ,τ)=RD−1[hk(ξ)]. | (3.6) |
Here, RD−1 is the inverse reduced differential transform operator. In this instance, we might have the following:
(1) If
f(ξ,τ)=g(ξ,τ)±h(ξ,τ), |
then
fk(ξ)=gk(ξ)±hk(ξ). |
(2) If
f(ξ,τ)=ag(ξ,τ), |
then
fk(ξ)=agk(ξ), |
where a is a constant.
(3) If
f(ξ,τ)=g(ξ,τ)h(ξ,τ), |
then
fk(ξ)=j∑i=0gi(ξ)hj−i(ξ). |
(4) If
f(ξ,τ)=∂n∂ξnh(ξ,τ), |
then
fk(ξ)=∂n∂ξnhk(ξ). |
(5) If
f(ξ,τ)=∂nα∂τnαh(ξ,τ), |
then
fk(ξ)=Γ(1+(k+n)α)Γ(1+kα)hk+n(ξ). |
Here, we have discussed the step-by-step explanation of the FRDTM in order to solve a non-linear fractional order PDE.
Consider the following fractional PDE in the operator form:
Dτmαg(ξ,τ)+Lg(ξ,τ)+Ng(ξ,τ)=z(ξ,τ), | (3.7) |
where m−1<mα≤m,m∈N, and
● L represents the linear operator;
● N represents the nonlinear operator;
● z(ξ,τ) is the inhomogenous source term.
Let us consider the initial conditions (ICs) associated with the above equation, i.e., Eq (3.7) is as follows:
g(i)(ξ,0)=li(ξ),i=0,1,…,m−1. | (3.8) |
Step 1. Implementation of properties of FRDTM
By employing the properties discussed in Section 3.4, Eq (3.7) simplifies to the following:
Γ(1+(k+n)α)Γ(1+kα)gk+m(ξ)=zk(ξ)−Rgk(ξ)−Ngk(ξ), | (3.9) |
where
k=0,1,2,…, |
gk(ξ) and zk(ξ) are the differential transformed form of g(ξ,τ) and z(ξ,τ), respectively.
Now, applying the FRDTM to the given initial conditions in (3.8), we obtain
gk(ξ)=lk(ξ), for k=0,1,2,…,m−1. | (3.10) |
Step 2. Calculation of gk(ξ)
Utilizing Eqs (3.9) and (3.10), the value of gk(ξ) for k=0,1,2,… can be iteratively obtained.
Step 3. Perform the inverse transformation
After determining the values of gk(ξ), let us perform the inverse differential transformation to obtain the approximate solution for g(ξ,τ). The inverse transformation allows us to reconstruct the solution from the transformed terms.
The approximate solution for the n term can be expressed as follows:
gn(ξ,τ)=n∑k=0gk(ξ)ταk. | (3.11) |
Step 4. Final solution
The required solution of Eq (3.7) is given by
g(ξ,τ)=limn→∞gn(ξ,τ). | (3.12) |
By incorporating fuzziness, we may express the time-fractional GeoKdV equation (Eq (1.3)) in an operator form as follows:
Dtα˜η−ω˜ηξ+32˜η˜ηξ+16˜ηξξξ=˜0, 0<α≤1, | (4.1) |
with ICs
˜η(ξ,0)=˜η0(ξ). | (4.2) |
Applying the FRDTM to Eqs (4.1) and (4.2), we arrive at the following recurrence relation:
{Γ(1+kα+α)Γ(1+kα)˜ηk+1(ξ)=ω∂∂ξ˜ηk(ξ)−16∂3∂ξ3˜ηk(ξ)−32k∑i=0˜ηi∂∂ξ˜ηk−i(ξ),˜η(ξ,0)=˜η0. | (4.3) |
Now, using the DPF of Eq (4.3), we obtain the following:
{Γ(1+kα+α)Γ(1+kα)˜ηk+1(ξ;p,r)=ω∂∂ξ˜ηk(ξ;p,r)−16∂3∂ξ3˜ηk(ξ;p,r)−32k∑i=0˜ηi∂∂ξ˜ηk−i(ξ;p,r),˜η(ξ,0;p,r)=˜η0(ξ;p,r). | (4.4) |
Solving the above for k=0,1,2,…, we obtain the following:
˜η1(ξ;p,r)=1Γ(1+α)[ω∂∂ξ˜η0(ξ;p,r)−16∂3∂ξ3˜η0(ξ;p,r)−32˜η0(ξ;p,r)∂∂ξ˜η0(ξ;p,r)],˜η2(ξ;p,r)=Γ(1+α)Γ(1+2α)[ω∂∂ξ˜η1(ξ;p,r)−16∂3∂ξ3˜η1(ξ;p,r)−32{˜η0(ξ;p,r)∂∂ξ˜η1(ξ;p,r)+˜η1(ξ;p,r)∂∂ξ˜η0(ξ;p,r)}],˜η3(ξ;p,r)=Γ(1+2α)Γ(1+3α)[ω∂∂ξ˜η2(ξ;p,r)−16∂3∂ξ3˜η2(ξ;p,r)−32{˜η0(ξ;p,r)∂∂ξ˜η2(ξ;p,r)+˜η1(ξ;p,r)∂∂ξ˜η1(ξ;p,r)+˜η2(ξ;p,r)∂∂ξ˜η0(ξ;p,r)}],⋮ |
Continuing with the process, the remaining components, including ˜η4(ξ;p,r),˜η5(ξ;p,r),˜η6(ξ;p,r) and so on, may be evaluated. By combining these results, we derive the series solution of Eq (4.1) as follows:
˜η(ξ,τ;p,r)=[˜η0(ξ,τ;p,r)+˜η1(ξ,τ;p,r)+˜η2(ξ,τ;p,r)+…]τnα,or˜η(ξ,τ;p,r)=∞∑n=0˜ηn(ξ,τ;p,r)τnα. | (4.5) |
In this work, we obtained approximate solutions for ˜η using up to a four-term solution. This was done by considering the initial condition in the context of a TFN. Calculations up to the determination of ˜η4(ξ,τ;p,r) have been taken due to the complexity associated with presenting more terms. For an improved accuracy, one can calculate an increased number of terms as needed. It is important to note that the obtained solutions can be influenced by adjusting the parameters p and r along with the fractional order α. To demonstrate convergence, we produced plots and compared with existing solutions we have assumed the fractional order index with α=1. Moreover, some graphs are generated with different values of α. Additionally, exploring different values of the parameters linked to the governing equation enables the effective computation of the desired solutions.
To execute our approach, we considered the following initial condition [5] in the context of a TFN:
˜η(ξ,0)=˜v{−13b2+13c+4b21+daξ−(c6−ω)τ−13b2(11+daξ−(c6−ω)τ)2}, | (5.1) |
where
c=√(b4+108C),b=ln(a), |
with a,d, and C being arbitrary constants, whereas
˜v=(0.43,13,1.63) |
is a TFN.
Additionally, the DPF can be applied to the provided initial condition to simplify the calculations. This leads to the following representation:
˜η(ξ,0;p,r)=˜v(p,r){−13b2+13c+4b21+daξ−(c6−ω)τ−13b2(11+daξ−(c6−ω)τ)2} | (5.2) |
where p,r∈[0,1].
By applying the discussed FRDTM approach, the governing equation (Eq (4.1)) with the provided IC in Eq (5.2) can be solved. The solution is expressed in terms of ˜η(ξ,τ;p,r), thereby considering up to four terms. To obtain the solution and analyze its physical behavior, the following sets of data are considered:
Set 1. ω=1,a=2,d=1,C=0.15.Set 2. ω=1.2,a=2,d=0.5,C=0.15.Set 3. ω=1,1.5, and a=2,d=0.5,C=0.15. |
Now, when α=1, the series solution
∞∑n=0˜ηn(ξ,τ;p,r)τnα |
efficiently converges to ˜η(ξ,τ;p,r).
From a graphical point of view, Figure 2 represents the convergence of the obtained fuzzy solutions which are in a triangular-shaped form. It can be observed that as the number of terms increases, the fuzzy approximate solutions demonstrate clear convergence. Some fuzzy solutions are obtained by taking different ξ with a fixed time followed by the set of data 1, and this can be depicted in Figure 3. To derive the lower and upper bound solutions from the obtained results, it is essential to substitute, r=0 and 1, respectively, using p=0. Furthermore, setting p=1 allows determination of the central/crisp value of the fuzzy solution. By performing these operations, we plotted a 2D figure (Figure 4) to illustrate the lower, middle, and upper solutions of the obtained results. Similarly, Figure 5 represents a 3D plot of the obtained fuzzy solution for τ=0.5 and 1.
The validation phase involves the verification of the accuracy and the reliability of the obtained fuzzy solution by comparing it with established results. A detailed description is given below.
The fuzzy solution is inherently represented as a range of possible values due to the uncertainty modeled by the fuzzy set theory. To facilitate a meaningful comparison, we extract the central values from the fuzzy solution. These central values correspond to specific cases where the uncertainty is minimized, representing a crisp solution derived from the fuzzy framework. This extraction is crucial as it allows us to compare these specific results with existing classical solutions. In this regard, the central values obtained from the fuzzy solution are then compared to an existing solution using dataset 2. The detailed comparison is presented in Table 1, which allows for a direct numerical comparison at different time levels. The visual representation of this comparison for different time levels τ=0.25,0.5,0.75, and 1 can be observed in Figure 6, which illustrates a good convergence with [5].
τ=0.5 | |||
ξ | Third term | Fourth term | Exact [5] |
-5 | 0.356134 | 0.356137 | 0.356137 |
-4 | 0.364948 | 0.364954 | 0.364954 |
-3 | 0.387575 | 0.387588 | 0.387589 |
-2 | 0.44102 | 0.441032 | 0.441031 |
-1 | 0.542995 | 0.542953 | 0.542949 |
0 | 0.656964 | 0.656866 | 0.656868 |
1 | 0.663263 | 0.663319 | 0.663324 |
2 | 0.553581 | 0.55366 | 0.553656 |
3 | 0.447823 | 0.447823 | 0.447821 |
4 | 0.390691 | 0.390676 | 0.390676 |
5 | 0.366198 | 0.366189 | 0.36619 |
τ=1 | |||
-5 | 0.356986 | 0.357007 | 0.357009 |
-4 | 0.367185 | 0.367236 | 0.36724 |
-3 | 0.393177 | 0.393281 | 0.393285 |
-2 | 0.453388 | 0.453481 | 0.453468 |
-1 | 0.562592 | 0.562257 | 0.5622 |
0 | 0.668483 | 0.667698 | 0.667739 |
1 | 0.650593 | 0.651041 | 0.651131 |
2 | 0.534035 | 0.534664 | 0.534608 |
3 | 0.435965 | 0.435967 | 0.435942 |
4 | 0.385426 | 0.385307 | 0.385309 |
5 | 0.364114 | 0.364044 | 0.364047 |
Furthermore, to enhance understanding, Figure 7 presents the absolute error plot using τ=0.75, ω=1.5, and other parameters from dataset 2. The central values of the obtained fuzzy solutions are used to calculate the absolute errors at each term's solution. As seen in the zoomed section of this figure, increasing the number of terms reduces the error. A similar pattern is observed in Table 2, where τ=0.5 and ω=0.5 are used for different ξ values. For the purpose of this discussion, the fractional order α is taken to be 1. The comparison helps to assess how closely the fuzzy-based results align with traditional methods, thereby testing the accuracy and reliability of the fuzzy solution.
No. | ξ values | |||||
ξ = 0 | ξ = 2 | ξ = 4 | ξ = 6 | ξ = 8 | ξ= 10 | |
1 | 0.5251×10−4 | 0.2224×10−2 | 0.1118×10−2 | 0.3349×10−3 | 0.8766×10−4 | 0.2217×10−4 |
2 | 0.5251×10−4 | 0.0313×10−4 | 0.1545×10−4 | 0.0572×10−4 | 0.0157×10−4 | 0.0040×10−4 |
3 | 0.0114×10−6 | 0.0427×10−6 | 0.0836×10−6 | 0.0605×10−6 | 0.0184×10−6 | 0.0048×10−6 |
4 | 0.2341×10−6 | 0.4636×10−6 | 0.1164×10−6 | 0.0094×10−6 | 0.0027×10−7 | 0.0082×10−8 |
In addition, to investigate the behavior of the solution, a 3D plot is presented in Figure 8 along with 2D and 3D filled contour plots. These visualizations provide a clearer understanding of the solution's evolution over time and space, thus illustrating the dynamic nature of the model. Another key part of the validation phase involves analyzing how external factors, such as the Coriolis parameter, influence the solution. The plot showcased in Figure 9 demonstrates the effect of increasing the Coriolis parameter on the dynamic behavior of η. By following the parameter regimes outlined in dataset 3, the observation indicates a decrease in the time required for the free surface elevation to approach zero as the Coriolis parameter increases [5].
Moreover, in the context of the fractional-order GeoKdV equation, Figure 10 displays a plot of the physical behavior and relationship between the exact solution of the integer-order GeoKdV equation [5] and the crisp case of the obtained fuzzy solution for different values of α. It can be observed that as the fractional order increases towards 1, the approximated special case fuzzy solution converges towards the integer-order exact solution, thus highlighting the consistency between the two approaches. Additionally, Figure 11 presents a 3D representation of the obtained solution for various values of the fractional order derivative α under the crisp scenario.
This article explored the application of the FRDTM to solve a time-fractional GeoKdV equation under fuzzy initial conditions. Specifically, triangular fuzzy initial conditions are considered, and the study observed that the approximate fuzzy series solution exhibited strong convergence as the number of terms in the series increased, demonstrating the method's robustness and reliability. Under crisp conditions, for the fractional order α=1, the results produced by the FRDTM closely align with existing precise solutions [5], further affirming the accuracy of this approach. This consistency emphasizes the FRDTM's effectiveness in handling fuzzy and crisp cases. The graphical representations provide a visual insight into the solution's behavior, thus showing the impact of different parameter values. A significant advantage observed is that FRDTM avoids the complications of linearization or complex transformations, thus making the method computationally efficient.
Building on the findings of this study, future research could extend the FRDTM to other nonlinear fractional differential equations, particularly those that model complex physical phenomena in higher-dimensional systems. Additionally, incorporating more generalized forms of fuzzy uncertainty, such as trapezoidal or Gaussian fuzzy numbers, could offer a broader perspective on handling imprecision in real-world applications. Furthermore, the implementation of higher dimensional fuzzy numbers, viz., interval type-2 fuzzy numbers and generalised type-2 fuzzy numbers, can be one interesting topic to be considered in the study of various shallow water wave equations.
The authors declare they have not used Artificial Intelligence (AI) tools in the creation of this article.
The first author would like to express his gratitude to the University Grants Commission (UGC), New Delhi, for providing fellowship for conducting research work.
The authors declare that they have no conflicts of interest.
[1] |
D. J. Korteweg, G. de Vries, On the change of form of long waves advancing in a rectangular canal, and on a new type of long stationary waves, Lond. Edinb. Dublin Philos. Mag. J. Sci., 39 (1895), 422–443. https://doi.org/10.1080/14786435.2010.547337 doi: 10.1080/14786435.2010.547337
![]() |
[2] |
A. Geyer, R. Quirchmayr, Shallow water equations for equatorial tsunami waves, Philos. Trans. R. Soc. A, 376 (2018), 20170100. https://doi.org/10.1098/rsta.2017.0100 doi: 10.1098/rsta.2017.0100
![]() |
[3] |
T. Ak, A. Saha, S. Dhawan, A. H. Kara, Investigation of Coriolis effect on oceanic flows and its bifurcation via geophysical Korteweg-de Vries equation, Numer. Methods Partial Differ. Equations, 36 (2020), 1234–1253. https://doi.org/10.1002/num.22469 doi: 10.1002/num.22469
![]() |
[4] |
S. T. R. Rizvi, A. R. Seadawy, F. Ashraf, M. Younis, H. Iqbal, D. Baleanu, Lump and interaction solutions of a geophysical Korteweg-de Vries equation, Results Phys., 19 (2020), 103661. https://doi.org/10.1016/j.rinp.2020.103661 doi: 10.1016/j.rinp.2020.103661
![]() |
[5] |
K. Hosseini, A. Akbulut, D. Baleanu, S. Salahshour, M. Mirzazadeh, L. Akinyemi, The geophysical KdV equation: its solitons, complexiton, and conservation laws, GEM, 13 (2022), 12. https://doi.org/10.1007/s13137-022-00203-8 doi: 10.1007/s13137-022-00203-8
![]() |
[6] |
A. R. Alharbi, M. B. Almatrafi, Exact solitary wave and numerical solutions for geophysical KdV equation, J. King Saud Univ. Sci., 34 (2022), 102087. https://doi.org/10.1016/j.jksus.2022.102087 doi: 10.1016/j.jksus.2022.102087
![]() |
[7] |
S. Saifullah, N. Fatima, S. A. M. Abdelmohsen, M. M. Alanazi, S. Ahmad, D. Baleanu, Analysis of a conformable generalized geophysical KdV equation with Coriolis effect, Alex. Eng. J., 73 (2023), 651–663. https://doi.org/10.1016/j.aej.2023.04.058 doi: 10.1016/j.aej.2023.04.058
![]() |
[8] | I. Podlubny, Fractional differential equations, Academic Press, 1999. |
[9] | D. Baleanu, K. Diethelm, E. Scalas, J. J. Trujillo, Fractional calculus: models and numerical methods, World Scientific Publishing Company, 2016. https://doi.org/10.1142/10044 |
[10] | S. Chakraverty, R. M. Jena, S. K. Jena, Time-fractional order biological systems with uncertain parameters, Synthesis Lectures on Mathematics and Statistics 12 (2020), 1–160. https://doi.org/10.2200/s00976ed1v01y201912mas03 |
[11] |
Y. Qin, A. Khan, I. Ali, M. A. Qurashi, H. Khan, R. Shah, et al., An efficient analytical approach for the solution of certain fractional-order dynamical systems, Energies, 13 (2020), 2725. https://doi.org/10.3390/en13112725 doi: 10.3390/en13112725
![]() |
[12] |
A. A. Alderremy, R. Shah, N. Iqbal, S. Aly, K. Nonlaopon, Fractional series solution construction for nonlinear fractional reaction-diffusion brusselator model utilizing laplace residual power series, Symmetry, 14, (2022), 1944. https://doi.org/10.3390/sym14091944 doi: 10.3390/sym14091944
![]() |
[13] |
S. Alshammari, M. M. Al-Sawalha, R. Shah, Approximate analytical methods for a fractional-order nonlinear system of Jaulent-Miodek equation with energy-dependent Schrödinger potential, Fractal Fract., 7 (2023), 140. https://doi.org/10.3390/fractalfract7020140 doi: 10.3390/fractalfract7020140
![]() |
[14] |
D. Mohapatra, S. Chakraverty, Legendre wavelets based approach for the solution of type-2 fuzzy uncertain smoking model of fractional order, Eng. Comput., 40 (2023), 868–920. https://doi.org/10.1108/EC-08-2022-0540 doi: 10.1108/EC-08-2022-0540
![]() |
[15] |
D. Mohapatra, S. Chakraverty, M. Alshammari, Time fractional heat equation of n+1-dimension in type-1 and type-2 fuzzy environment, Int. J. Fuzzy Syst., 26 (2023), 1–16. https://doi.org/10.1007/s40815-023-01569-z doi: 10.1007/s40815-023-01569-z
![]() |
[16] | M. Sahoo, S. Chakraverty, Solitary wave solution for time-fractional SMCH equation in fuzzy environment, In: S. Chakraverty, R. M. Jena, Computation and modeling for fractional order systems, Elsvier, 2024,227–239. https://doi.org/10.1016/B978-0-44-315404-1.00019-9 |
[17] |
P. Karunakar, S. Chakraverty, Solutions of time-fractional third- and fifth-order Korteweg-de-Vries equations using homotopy perturbation transform method, Eng. Comput., 36 (2019), 2309–2326. https://doi.org/10.1108/EC-01-2019-0012 doi: 10.1108/EC-01-2019-0012
![]() |
[18] |
R. Shah, U. Farooq, H. Khan, D. Baleanu, P. Kumam, M. Arif, Fractional view analysis of third order Kortewege-de Vries equations, using a new analytical technique, Front. Phys., 7 (2020), 00244. https://doi.org/10.3389/fphy.2019.00244 doi: 10.3389/fphy.2019.00244
![]() |
[19] |
R. M. Jena, S. Chakraverty, D. Baleanu, Solitary wave solution for a generalized Hirota-Satsuma coupled KdV and MKdV equations: a semi-analytical approach, Alex. Eng. J., 59 (2020), 2877–2889. https://doi.org/10.1016/j.aej.2020.01.002 doi: 10.1016/j.aej.2020.01.002
![]() |
[20] |
M. M. Al-Sawalha, R. Shah, A. Khan, O. Y. Ababneh, T. Botmart, Fractional view analysis of Kersten-Krasil'shchik coupled KdV-mKdV systems with non-singular kernel derivatives, AIMS Math., 7 (2022), 18334–18359. https://doi.org/10.3934/math.20221010 doi: 10.3934/math.20221010
![]() |
[21] |
X. Cao, B. Liu, M. Liu, K. Peng, W. Tian, Variational principles for two kinds of non-linear geophysical KdV equation with fractal derivatives, Therm. Sci., 26 (2022), 2505–2515. https://doi.org/10.2298/TSCI2203505C doi: 10.2298/TSCI2203505C
![]() |
[22] |
K. Shehzada, A. Ullah, S. Saifullah, A. Akgül, Fractional generalized perturbed KdV equation with a power Law kernel: a computational study, Results Control Optim., 12 (2023), 100298. https://doi.org/10.1016/j.rico.2023.100298 doi: 10.1016/j.rico.2023.100298
![]() |
[23] |
L. A. Zadeh, Fuzzy sets, Inf. Control, 8 (1965), 338–353. https://doi.org/10.1016/S0019-9958(65)90241-X doi: 10.1016/S0019-9958(65)90241-X
![]() |
[24] |
P. Karunakar, S. Chakraverty, Comparison of solutions of linear and non-linear shallow water wave equations using homotopy perturbation method, Int. J. Numer. Methods Heat Fluid Flow, 27 (2017), 2015–2029. https://doi.org/10.1108/hff-09-2016-0329 doi: 10.1108/hff-09-2016-0329
![]() |
[25] |
M. Sahoo, S. Chakraverty, Sawi fransform based homotopy perturbation method for solving shallow water wave equations in fuzzy environment, Mathematics, 10 (2022), 2900. https://doi.org/10.3390/math10162900 doi: 10.3390/math10162900
![]() |
[26] |
M. Sahoo, S. Chakraverty, Dynamics of tsunami wave propagation in uncertain environment, Comput. Appl. Math., 43 (2024), 266. https://doi.org/10.1007/s40314-024-02776-6 doi: 10.1007/s40314-024-02776-6
![]() |
[27] |
M. Sahoo, S. Chakraverty, Influence of uncertain coriolis parameter on wave solution of Korteweg-de Vries equation, Int. J Geomath., 15 (2024), 10. https://doi.org/10.1007/s13137-024-00252-1 doi: 10.1007/s13137-024-00252-1
![]() |
[28] |
D. Behera, S. Chakraverty, New approach to solve fully fuzzy system of linear equations using single and double parametric form of fuzzy numbers, Sadhana, 40 (2015), 35–49. https://doi.org/10.1007/s12046-014-0295-9 doi: 10.1007/s12046-014-0295-9
![]() |
[29] | S. Chakraverty, D. M. Sahoo, N. R. Mahato, Concepts of soft computing, Springer, 2019, 53–69. https://doi.org/10.1007/978-981-13-7430-2 |
[30] |
R. M. Jena, S. Chakraverty, D. Baleanu, On new solutions of time-fractional wave equations arising in shallow water wave propagation, Mathematics, 7 (2019), 722. https://doi.org/10.3390/math7080722 doi: 10.3390/math7080722
![]() |
[31] |
Y. Keskin, G. Oturanç, Reduced differential transform method for generalized KdV equations, Math. Comput. Appl., 15 (2010), 382–393. https://doi.org/10.3390/mca15030382 doi: 10.3390/mca15030382
![]() |
τ=0.5 | |||
ξ | Third term | Fourth term | Exact [5] |
-5 | 0.356134 | 0.356137 | 0.356137 |
-4 | 0.364948 | 0.364954 | 0.364954 |
-3 | 0.387575 | 0.387588 | 0.387589 |
-2 | 0.44102 | 0.441032 | 0.441031 |
-1 | 0.542995 | 0.542953 | 0.542949 |
0 | 0.656964 | 0.656866 | 0.656868 |
1 | 0.663263 | 0.663319 | 0.663324 |
2 | 0.553581 | 0.55366 | 0.553656 |
3 | 0.447823 | 0.447823 | 0.447821 |
4 | 0.390691 | 0.390676 | 0.390676 |
5 | 0.366198 | 0.366189 | 0.36619 |
τ=1 | |||
-5 | 0.356986 | 0.357007 | 0.357009 |
-4 | 0.367185 | 0.367236 | 0.36724 |
-3 | 0.393177 | 0.393281 | 0.393285 |
-2 | 0.453388 | 0.453481 | 0.453468 |
-1 | 0.562592 | 0.562257 | 0.5622 |
0 | 0.668483 | 0.667698 | 0.667739 |
1 | 0.650593 | 0.651041 | 0.651131 |
2 | 0.534035 | 0.534664 | 0.534608 |
3 | 0.435965 | 0.435967 | 0.435942 |
4 | 0.385426 | 0.385307 | 0.385309 |
5 | 0.364114 | 0.364044 | 0.364047 |
No. | ξ values | |||||
ξ = 0 | ξ = 2 | ξ = 4 | ξ = 6 | ξ = 8 | ξ= 10 | |
1 | 0.5251×10−4 | 0.2224×10−2 | 0.1118×10−2 | 0.3349×10−3 | 0.8766×10−4 | 0.2217×10−4 |
2 | 0.5251×10−4 | 0.0313×10−4 | 0.1545×10−4 | 0.0572×10−4 | 0.0157×10−4 | 0.0040×10−4 |
3 | 0.0114×10−6 | 0.0427×10−6 | 0.0836×10−6 | 0.0605×10−6 | 0.0184×10−6 | 0.0048×10−6 |
4 | 0.2341×10−6 | 0.4636×10−6 | 0.1164×10−6 | 0.0094×10−6 | 0.0027×10−7 | 0.0082×10−8 |
τ=0.5 | |||
ξ | Third term | Fourth term | Exact [5] |
-5 | 0.356134 | 0.356137 | 0.356137 |
-4 | 0.364948 | 0.364954 | 0.364954 |
-3 | 0.387575 | 0.387588 | 0.387589 |
-2 | 0.44102 | 0.441032 | 0.441031 |
-1 | 0.542995 | 0.542953 | 0.542949 |
0 | 0.656964 | 0.656866 | 0.656868 |
1 | 0.663263 | 0.663319 | 0.663324 |
2 | 0.553581 | 0.55366 | 0.553656 |
3 | 0.447823 | 0.447823 | 0.447821 |
4 | 0.390691 | 0.390676 | 0.390676 |
5 | 0.366198 | 0.366189 | 0.36619 |
τ=1 | |||
-5 | 0.356986 | 0.357007 | 0.357009 |
-4 | 0.367185 | 0.367236 | 0.36724 |
-3 | 0.393177 | 0.393281 | 0.393285 |
-2 | 0.453388 | 0.453481 | 0.453468 |
-1 | 0.562592 | 0.562257 | 0.5622 |
0 | 0.668483 | 0.667698 | 0.667739 |
1 | 0.650593 | 0.651041 | 0.651131 |
2 | 0.534035 | 0.534664 | 0.534608 |
3 | 0.435965 | 0.435967 | 0.435942 |
4 | 0.385426 | 0.385307 | 0.385309 |
5 | 0.364114 | 0.364044 | 0.364047 |
No. | ξ values | |||||
ξ = 0 | ξ = 2 | ξ = 4 | ξ = 6 | ξ = 8 | ξ= 10 | |
1 | 0.5251×10−4 | 0.2224×10−2 | 0.1118×10−2 | 0.3349×10−3 | 0.8766×10−4 | 0.2217×10−4 |
2 | 0.5251×10−4 | 0.0313×10−4 | 0.1545×10−4 | 0.0572×10−4 | 0.0157×10−4 | 0.0040×10−4 |
3 | 0.0114×10−6 | 0.0427×10−6 | 0.0836×10−6 | 0.0605×10−6 | 0.0184×10−6 | 0.0048×10−6 |
4 | 0.2341×10−6 | 0.4636×10−6 | 0.1164×10−6 | 0.0094×10−6 | 0.0027×10−7 | 0.0082×10−8 |