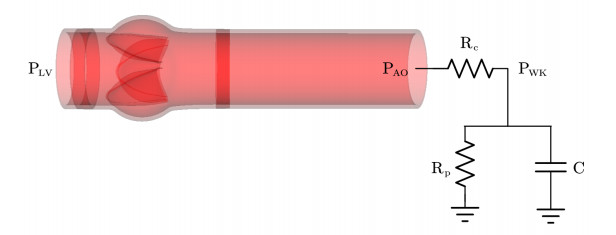
The coronary artery constitutes a vital vascular system that sustains cardiac function, with its primary role being the conveyance of indispensable nutrients to the myocardial tissue. When coronary artery disease occurs, it will affect the blood supply of the heart and induce myocardial ischemia. Therefore, it is of great significance to numerically simulate the coronary artery and evaluate its blood supply capacity. In this article, the coronary artery lumped parameter model was derived based on the relationship between circuit system parameters and cardiovascular system parameters, and the blood supply capacity of the coronary artery in healthy and stenosis states was studied. The aortic root pressure calculated by the aortic valve fluid-structure interaction (AV FSI) simulator was employed as the inlet boundary condition. To emulate the physiological phenomenon of sudden pressure drops resulting from an abrupt reduction in blood vessel radius, a head loss model was connected at the coronary artery's entrance. For each coronary artery outlet, the symmetric structured tree model was appended to simulate the terminal impedance of the missing downstream coronary arteries. The particle swarm optimization (PSO) algorithm was used to optimize the blood flow viscous resistance, blood flow inertia, and vascular compliance of the coronary artery model. In the stenosis states, the relative flow and fractional flow reserve (FFR) calculated by numerical simulation corresponded to the published literature data. It was anticipated that the proposed model can be readily adapted for clinical application, serving as a valuable reference for diagnosing and treating patients.
Citation: Li Cai, Qian Zhong, Juan Xu, Yuan Huang, Hao Gao. A lumped parameter model for evaluating coronary artery blood supply capacity[J]. Mathematical Biosciences and Engineering, 2024, 21(4): 5838-5862. doi: 10.3934/mbe.2024258
[1] | Nattawan Chuchalerm, Wannika Sawangtong, Benchawan Wiwatanapataphee, Thanongchai Siriapisith . Study of Non-Newtonian blood flow - heat transfer characteristics in the human coronary system with an external magnetic field. Mathematical Biosciences and Engineering, 2022, 19(9): 9550-9570. doi: 10.3934/mbe.2022444 |
[2] | B. Wiwatanapataphee, D. Poltem, Yong Hong Wu, Y. Lenbury . Simulation of Pulsatile Flow of Blood in Stenosed Coronary Artery Bypass with Graft. Mathematical Biosciences and Engineering, 2006, 3(2): 371-383. doi: 10.3934/mbe.2006.3.371 |
[3] | Benchawan Wiwatanapataphee, Yong Hong Wu, Thanongchai Siriapisith, Buraskorn Nuntadilok . Effect of branchings on blood flow in the system of human coronary arteries. Mathematical Biosciences and Engineering, 2012, 9(1): 199-214. doi: 10.3934/mbe.2012.9.199 |
[4] | Lorenzo Civilla, Agnese Sbrollini, Laura Burattini, Micaela Morettini . An integrated lumped-parameter model of the cardiovascular system for the simulation of acute ischemic stroke: description of instantaneous changes in hemodynamics. Mathematical Biosciences and Engineering, 2021, 18(4): 3993-4010. doi: 10.3934/mbe.2021200 |
[5] | Fan He, Minru Li, Xinyu Wang, Lu Hua, Tingting Guo . Numerical investigation of quantitative pulmonary pressure ratio in different degrees of stenosis. Mathematical Biosciences and Engineering, 2024, 21(2): 1806-1818. doi: 10.3934/mbe.2024078 |
[6] | Honghui Zhang, Jun Xia, Yinlong Yang, Qingqing Yang, Hongfang Song, Jinjie Xie, Yue Ma, Yang Hou, Aike Qiao . Branch flow distribution approach and its application in the calculation of fractional flow reserve in stenotic coronary artery. Mathematical Biosciences and Engineering, 2021, 18(5): 5978-5994. doi: 10.3934/mbe.2021299 |
[7] | Scott R. Pope, Laura M. Ellwein, Cheryl L. Zapata, Vera Novak, C. T. Kelley, Mette S. Olufsen . Estimation and identification of parameters in a lumped cerebrovascular model. Mathematical Biosciences and Engineering, 2009, 6(1): 93-115. doi: 10.3934/mbe.2009.6.93 |
[8] | Xintong Wu, Yingyi Geng, Xinhong Wang, Jucheng Zhang, Ling Xia . Continuous extraction of coronary artery centerline from cardiac CTA images using a regression-based method. Mathematical Biosciences and Engineering, 2023, 20(3): 4988-5003. doi: 10.3934/mbe.2023231 |
[9] | Ziyu Jin, Ning Li . Diagnosis of each main coronary artery stenosis based on whale optimization algorithm and stacking model. Mathematical Biosciences and Engineering, 2022, 19(5): 4568-4591. doi: 10.3934/mbe.2022211 |
[10] | Hongtao Liu, Shuqin Liu, Xiaoxu Ma, Yunpeng Zhang . A numerical model applied to the simulation of cardiovascular hemodynamics and operating condition of continuous-flow left ventricular assist device. Mathematical Biosciences and Engineering, 2020, 17(6): 7519-7543. doi: 10.3934/mbe.2020384 |
The coronary artery constitutes a vital vascular system that sustains cardiac function, with its primary role being the conveyance of indispensable nutrients to the myocardial tissue. When coronary artery disease occurs, it will affect the blood supply of the heart and induce myocardial ischemia. Therefore, it is of great significance to numerically simulate the coronary artery and evaluate its blood supply capacity. In this article, the coronary artery lumped parameter model was derived based on the relationship between circuit system parameters and cardiovascular system parameters, and the blood supply capacity of the coronary artery in healthy and stenosis states was studied. The aortic root pressure calculated by the aortic valve fluid-structure interaction (AV FSI) simulator was employed as the inlet boundary condition. To emulate the physiological phenomenon of sudden pressure drops resulting from an abrupt reduction in blood vessel radius, a head loss model was connected at the coronary artery's entrance. For each coronary artery outlet, the symmetric structured tree model was appended to simulate the terminal impedance of the missing downstream coronary arteries. The particle swarm optimization (PSO) algorithm was used to optimize the blood flow viscous resistance, blood flow inertia, and vascular compliance of the coronary artery model. In the stenosis states, the relative flow and fractional flow reserve (FFR) calculated by numerical simulation corresponded to the published literature data. It was anticipated that the proposed model can be readily adapted for clinical application, serving as a valuable reference for diagnosing and treating patients.
The report released by the American Heart Association states that cardiovascular disease maintains its status as the primary global cause of death, claiming over 17.6 million lives annually, with forecasts suggesting this figure will rise to exceed 23.6 million by 2030 [1]. The coronary artery, the vascular system dedicated to providing nutrients and energy to the heart, has been the main research focus in recent years.
The lumped parameter model, also known as the 0D model, was first developed by Otto Frank in 1898. It is the simplest mathematical model to describe the cardiovascular system and it is frequently employed to simulate the systemic characteristics of the cardiovascular system. The 0D model is known for its simple structure, easy-to-understand features, low computational cost, and efficient computing power. It has a wide range of simulations and can cover many fields and application scenarios. Compared with the coronary artery multi-scale model, its accuracy is relatively low and difficult to handle in the simulation of some complex physiological conditions, but it is still an effective modeling method in many fields [2]. Rideout et al. simulated the human pulmonary circulation system [3], accounting for the interactive effects of left atrial and right ventricular contractions on pressures in the pulmonary veins. They also developed an analog computer model to research the human cardiovascular system[4,5], with a particular emphasis on pressure and flow dynamics within veins, encompassing gravitational effects, respiration, venous collapse, and valve function. Liang et al. [6] developed a human cardiovascular system lumped parameter model featuring a detailed compartmental representation of both the main vascular circulations and the heart.
Wang et al. [7] employed a closed-loop circuit model to simulate the left coronary arterial system and studied the impacts of stenosis, heart rate, and other factors on coronary hemodynamics. Mantero et al. [8] studied the role of the coronary artery bed in the cardiovascular system. Pietrabissa et al. [9] improved the cardiovascular system closed-loop circuit model, incorporating a more intricate depiction of the left coronary artery, which was also utilized to assess the coronary artery hemodynamics of surgical reconstruction. Duanmu et al. [10] established a new closed-loop lumped parameter model for the whole coronary network (including the right coronary artery). They first proposed the head loss model, which describes the physiological phenomenon of a sudden pressure drop at the coronary artery's entrance.
Since considering the entire coronary artery becomes prohibitively expensive computationally, the model needs an outflow boundary condition to simulate the truncated tree. The three-element Windkessel model, put forth by Westerhof et al. [11], is the most classical lumped parameter model and is widely employed as the outflow boundary condition. At present, there is no strict theoretical support for the selection of parameters in the Windkessel model, which is usually obtained through experiments. Olufsen et al. [12,13,14] proposed the structured tree model that can also serve as a boundary condition, with its evaluation based on the root radius of each blood vessel.
Arterial system stenosis can cause a variety of cardiovascular diseases. Zhang et al. [15] studied carotid artery stenosis. Pagiatakis et al. [16,17] studied the influence of stenosis eccentricity and stenosis severity on coronary bifurcation lesions. The fractional flow reserve (FFR), a nondimensional index, can combine the physiological effects of the coronary artery with the severity of stenosis to evaluate the hemodynamic significance of the coronary artery disease noninvasively. FFR is referred to as the ratio of the maximum hyperemic blood flow in the coronary artery with stenosis to the maximum hyperemic blood flow that occurs in the same coronary artery under normal conditions [18,19,20]. Clinical research has demonstrated that FFR between 0.75 and 0.8 (that is, under maximum congestion conditions, when the pressure of the distal coronary artery is 75–80% of the proximal) is usually considered to be the threshold for ischemic attacks. Lesions below this threshold are considered to be the cause of ischemia [21,22].
Our purpose is to establish a coronary artery lumped parameter model that can effectively simulate its blood supply capacity. The aortic valve fluid-structure interaction (AV FSI) simulator can simulate the interaction between the heart and the arterial system and provide an in-depth understanding of the function of the cardiovascular system. The 0D model simplifies the description of the cardiovascular system and provides a series of algebraic equations to simulate changes in blood flow and pressure. In this paper, combining the AV FSI simulator and the 0D model can make full use of the advantages of both to achieve more accurate simulation and prediction. The head loss model is incorporated at the entrance of the coronary artery. The outlet connects with the symmetric structured tree model proposed by Olufsen et al. The resistance-inductance-capacitance (RLC) parameters in the 0D coronary artery model are optimized in a special way. In addition, the coronary artery stenosis model is established by changing the local RLC parameters, and the blood supply capacity of the coronary artery under different degrees of stenosis is analyzed using FFR and relative flow.
The AV FSI simulator is built using a porcine pericardial valve, as demonstrated in our previous research [23,24]. The thickness of the valve leaflet is 0.04 cm, and the outer wall connecting with it has a thickness of about 0.15 cm and an inner diameter of 1.3 cm. The length of the whole blood vessel is 13 cm. The three-element Windkessel model, frequently used to simulate the afterload [25], is coupled in the outlet. Figure 1 shows the schematic illustration of the AV FSI simulator.
Aortic valve leaflets are considered to be nonlinear, incompressible, anisotropic, and hyperelastic. Specifically, the strain energy function of aortic valve leaflets [26] is
W=C10[eC01(I1−3)−1]+k12k2[ek2(I4−1)2−1], | (2.1) |
in which k1, k2, C10, and C01 are material parameters; k1, k2 are the material constants related to the stress and the dimensionless parameter, respectively; C10, C01 are utilized to characterize the properties of the matrix material. There are two strain invariants, I1=tr(C) and I4=f0⋅(Cf0), along with the reference fiber direction (f0). C is the right Cauchy-Green deformation tensor. These parameter values are presented in Table 1.
C10(kPa) | C01 | k1(kPa) | k2 | C(ml/mmHg) | Rp(mmHg⋅s/ml) | Rc (mmHg⋅s/ml) |
1.21 | 7.99 | 24.23 | 57.62 | 1.75 | 0.79 | 0.033 |
The Windkessel model [28] is
CdPWKdt+PWKRp=QAO,PAO−PWKRc=QAO, | (2.2) |
in which C represents the vascular compliance, PWK represents the pressure stored within the Windkessel model, Rp represents the peripheral resistance, and Rc represents the characteristic resistance. These parameter values are presented in Table 1.
In the Immersion Boundary/Finite Element (IB/FE) method [29], the fluid domain (Ωf(0)) uses the Eulerian grids and finite difference discretization, while the structure domain (Ωs(0)) employs the Lagrangian grids and finite element discretization [23,30]. The influence of the structure on the fluid is represented by incorporating a force source term into the Navier-Stokes equation [31]. The Dirac delta function δ(x) is utilized to establish the coupling between the structure described in Lagrangian form and the fluid represented in Eulerian form. The IB/FE method can be described as follows [31,32]:
ρ(∂u(x,t)∂t+u(x,t)⋅∇u(x,t))=−∇p(x,t)+μΔu(x,t)+fe(x,t),∇⋅u(x,t)=0,fe(x,t) = ∫Ωs(0)Fe(X,t)δ(x−χ(X,t))dX,∂χ(X,t)∂t = ∫Ωu(x,t)δ(x−χ(X,t))dx,∫Ωs(0)Fe(X,t)⋅V(X)dX = −∫Ωs(0)Pe(X,t):∇XV(X)dX, | (2.3) |
where x=(x1,x2,x3)∈Ω represents the Euler coordinate system, and Ω represents the physiil region occupied by the aortic valve model. X=(X1,X2,X3)∈Ωs(0) represents the Lagrange coordinate system. χ(X,t)∈Ω represents the Eulerian coordinate of the position that a Lagrangian coordinate point X occupies at time t. u(x,t) represents the Eulerian velocity, and p(x,t) represents the Eulerian pressure. fe(x,t) and Fe(X,t) are the Eulerian elastic force and the Lagrangian elastic force, respectively. Pe(X,t) represents the first Piola-Kirchhoff stress tensor, which can be derived from the strain energy function. In general, the density of blood (ρ) and its dynamic viscosity (μ) are assumed to remain constant, e.g., ρ=1.0 g/cm3 and μ=4.0×10−3 kg/m⋅s. For more details of the AV FSI simulator and the IB/FE method, see [24,29,30].
The blood regarded as a continuous, incompressible Newtonian fluid, and the blood vessel has a cylindrical wall that is rigid [33,34]. According to the analogy between the hemodynamic system and the circuit system, we can establish an equivalent circuit model of a blood vessel; see Figure 2. The blood flow satisfies the Newton equation and the continuity equation for any minuscule artery length [7]. The governing equations [7,35] for flow rate and pressure of each blood vessel is
Pin−Pout=L∂Qin∂t+RQin,Qin−Qout=C∂Pout∂t, | (2.4) |
in which Pin and Pout are the average inlet and outlet pressures that vary with time. Similarly, Qin and Qout represent the average inlet and outlet flow rates changing with time, respectively. R, L, and C are the blood flow viscous resistance, blood flow inertia, and vascular compliance, respectively, analogous to the resistance, inductance, and capacitance in the circuit system.
The coronary artery emerges from the aortic sinus at the root of the aorta and is divided into the left and right branches that contain many vascular branches. Based on the CT of a patient-specific coronary [10] and literature [9], we consider a coronary artery model composed of 20 segments: 17 segments attributed to the left coronary artery (three additional septal branch (SPT) vascular segments are added) and 3 segments pertaining to the right coronary artery. The schematic diagram of the coronary artery simplified structure model is shown in Figure 3, and the 0D model of the whole coronary artery is detailed in Figure 4. Each segment of the coronary artery can be modeled with Eq (2.4). The complete system can be mathematically modeled by the ordinary differential equations (ODEs) as
∂y∂t=Ay+b. | (2.5) |
In this system, y denotes the vector consisting of unknown vessel flow rates and pressures, A represents a square matrix composed of parameter values, and b represents a column vector derived from the specified boundary conditions.
Initial conditions, inlet and outlet boundary conditions, bifurcation boundary conditions, and so on are needed to solve the hemodynamics of the coronary artery described by Eq (2.5). In this study, we give the following conditions:
(1) After several numerical simulations, it is observed that the initial conditions mainly have a significant effect on the speed of reaching the stable solution but have a small effect on the final numerical results of the steady state. According to the Wiggers diagram [36], the initial pressure and flow rate at each segment can be set to 80 mmHg and 0 ml/s.
(2) In order to obtain the physiologically realistic blood pressure and flow rate in the coronary artery, the aortic root pressure calculated by the AV FSI simulator (see Section 2.1) is selected as the inlet boundary condition.
(3) At the entrance of LMCA and RCA, the sudden decrease in blood vessel radius will lead to an abrupt decrease in blood pressure. The pressure drop can be described by the following empirical formula:
Δp=ζρv22, | (2.6) |
where ζ=2.88 is the local energy loss coefficient [37] and v is the average velocity. Therefore, the flow resistance Rh can be calculated using the following Eq [10]:
Rh=ΔpQ=ζρ2A2Q, | (2.7) |
in which A represents the cross-sectional area of the blood vessel. It can be seen that Rh is a variable flow resistance proportional to the flow rate.
(4) Each vessel's terminal is connected to the capillaries downstream, making it challenging to assess the boundary conditions at the outlet. An outflow boundary condition, the structured tree model presented by Olufsen et al. [12], has been used in literature, in which each vessel is modeled as a compliant linear segment, and the root impedance (Z) depends largely on the minimum vessel diameter at the end truncation (rmin). The structured tree has nothing to do with the actual geometry of the vessel but a topological structure based on the literature data [14]. In order to simplify the model, we choose the symmetric structured tree model and set rmin = 0.01 mm, indicating that the structured tree terminates when the vessel radius is less than 0.01 mm. The root impedance calculation formula [13] of the N-generation symmetric structured tree model is
Z=8μλπr302α3−(2α3)−N2α3−1, | (2.8) |
in which λ=50 defines the ratio of vessel length to radius [38], r0 represents the root radius of the initial vessel, and the ratio of the radius of the daughter vessel (rd) to the radius of the parent vessel (rp) is α=0.778. The terminal impedance of each coronary artery outlet is shown in Table 2.
Segment | Results of Eq (2.9) | Optimization results | Z | ||||
R | L | C | R | L | C | ||
LMCA | 0.139 | 0.034 | 0.001 | 2.0159 | 0.01 | 0.00218 | |
LAD | 0.473 | 0.069 | 0.001 | 0.3685 | 0.001 | 0.0012 | |
LAD1 | 3.190 | 0.238 | 0.001 | 2.9761 | 0.2064 | 0.00163 | 145.6 |
LAD2 | 0.922 | 0.100 | 0.001 | 0.747 | 0.0017 | 0.00107 | |
SPT1 | 3.184 | 0.235 | 0.001 | 2.689 | 0.2031 | 0.0012 | 155.6 |
SPT2 | 2.906 | 0.287 | 0.002 | 3.8576 | 0.0017 | 0.0001 | 407.5 |
SPT3 | 2.819 | 0.281 | 0.002 | 3.6832 | 0.0014 | 0.0001 | 327 |
LAD3 | 6.803 | 0.238 | 0.0002 | 3.6025 | 0.009 | 0.00018 | 155 |
LAD4 | 4.775 | 0.353 | 0.001 | 3.731 | 0.1658 | 0.00188 | 80.2 |
DIAG | 1.941 | 0.184 | 0.001 | 2.0509 | 0.094 | 0.00194 | 55.1 |
LCX | 0.336 | 0.050 | 0.001 | 0.4362 | 0.001 | 0.00104 | |
MARG1 | 1.477 | 0.140 | 0.001 | 1.5214 | 0.184 | 0.00146 | 155 |
LCX1 | 0.401 | 0.048 | 0.001 | 0.2699 | 0.0264 | 0.00075 | |
MARG2 | 2.292 | 0.161 | 0.001 | 2.014 | 0.150 | 0.001 | 168.4 |
LCX2 | 0.856 | 0.082 | 0.001 | 0.9891 | 0.0082 | 0.00049 | |
MARG3 | 2.463 | 0.186 | 0.001 | 2.7604 | 0.0794 | 0.00083 | 72 |
LCX3 | 2.807 | 0.203 | 0.001 | 3.7397 | 0.1812 | 0.00095 | 170.1 |
RCA | 1.602 | 0.257 | 0.049 | 2.5284 | 0.5 | 0.02061 | |
PLA | 1.195 | 0.138 | 0.013 | 5.0 | 0.1896 | 0.01017 | 59.81 |
PDA | 2.078 | 0.170 | 0.008 | 4.6671 | 0.001 | 0.00913 | 139.72 |
F | 1.228 | 0.345 |
(5) Blood flow in the coronary artery is distinct from that in other parts of the arterial system, as it is significantly affected by the relaxation and contraction of the heart. In order to simulate coronary blood flow veritably, it is necessary to include the cardiac pressure acting on the coronary artery vessel (i.e., ventricular pressure) [39]. At the end of the model, the VPG is connected to simulate the intramyocardial pressure, which selected left ventricular pressure according to the location of the coronary artery.
(6) The principles of flow conservation and pressure continuity are ensured at the points where blood vessels bifurcate. Specifically, the blood flow in the parent vessel is equivalent to the aggregate sum of the flows in all its daughter vessels, and the blood pressure of the parent vessel is equal to the blood pressure of each daughter vessel.
In this paper, a fourth-order Runge-Kutta method [40] is employed for the solution of the ODEs describing the hemodynamics of the coronary artery. In order to obtain stable numerical results, each simulation performs three cycles.
Generally, there are two methods to determine the RLC parameter values in the 0D coronary artery model. One is the manual estimate method, which depends on experimental measurements, empirical data, or iterative adjustment of parameters. This method takes a lot of time. The other is calculated through the lumped parameter modeling formula, but the formula is not unique, there is [7],
R=128μl/πD4,L=4ρl/πD2,C=πD3l/4Gh, | (2.9) |
in which the Young's modulus of blood vessel G=2×105 Pa [41], l is the length of the blood vessel, D represents the diameter of the blood vessel, and the thickness of the blood vessel wall h=0.08D [42]. These parameter values related to the anatomical structure of the patient's coronary artery can be obtained through a series of medical examinations, such as coronary angiography and echocardiography.
In order to obtain realistic coronary artery blood pressure and flow rate, the value range of RLC parameters in the 0D coronary artery model is preliminarily estimated by Eq (2.9), and then optimized by the intelligent optimization algorithm, common artificial intelligence [43], genetic algorithm [44], and particle swarm optimization (PSO) algorithm [45]. The PSO algorithm was first developed by Russell Eberhart and James Kennedy in 1995, inspired by the foraging mode of birds (the birds allow groups to find optimal purposes through information sharing). In the PSO algorithm, each optimization solution is simulated as a 'particle'. Each particle navigates through the search space, continually updating its position and velocity information to iteratively converge toward the optimal solution. The algorithm is simple, easy to understand, and easy to program. It has fast convergence speed and can find the approximate optimal solution to the problem in a short time. The number of parameters is small, and the adjustment of parameters is relatively simple [46]. It is suitable for solving high-dimensional optimization problems. The summary of the PSO algorithm can be seen in Appendix A.
The optimization objective function is defined as the root mean square error between the reference solution (yn) and the numerical result (y′n), expressed as:
F=√1KK∑n=1|yn−y′n|2, | (2.10) |
in which K represents the number of sample points. The optimal results are obtained when the objective function attains its minimum value. The termination condition is to reach the maximum number of iterations or obtain an acceptable satisfactory solution.
Coronary stenosis leads to diminished blood flow reaching the heart, causing myocardial ischemia, which in turn leads to various cardiovascular diseases. It is necessary to research the influence of coronary artery stenosis on local blood flow. To establish the lumped parameter model of coronary artery stenosis, we adjust the RLC parameter values of the corresponding vascular segments in our 0D coronary artery model. The reduction rate of the vascular cross-sectional area (β) is defined as the ratio between the stenosed vessel's cross-sectional area (As) and the healthy cross-sectional area (A0), namely,
β=AsA0. | (2.11) |
According to the Eq (2.9), the relationship [47] between the RLC parameter values in the stenosis state and the healthy state can be derived as follows:
Rs=R0β−2 ,Ls=L0β−1 ,Cs=C0β3/2, | (2.12) |
where Rs, Ls, and Cs represent the resistance, inductance, and capacitance in the stenosis state, respectively. R0, L0, and C0 represent the ones in the healthy state. The value (1−β) represents the vascular stenosis rate, usually expressed as a percentage. It is assumed that other parameters remain constant in the stenosis state.
FFR quantifies the percentage of normal, hyperemic myocardial blood flow retained when the supplying artery has stenosis [17], has become an important clinical indicator for judging the degree of arterial stenosis that prevents blood flow from reaching the myocardium [20], and is also the gold standard for evaluating whether coronary artery stenosis causes ischemia [48]. In clinical practice, FFR can be expressed as the ratio between the time-averaged proximal pressure Pp and the time-averaged distal pressure Pd in coronary artery stenosis (under conditions of maximal myocardial hyperemia) [20], i.e.,
FFR=PdPp. | (2.13) |
For the flow rates predicted by the 0D model, the average flow is calculated by integrating the flow rate waveform over one heartbeat. This average flow is subsequently normalized by dividing it with the average flow rate that would occur in the absence of stenosis, thus obtaining the relative flow [7]. Relative flow represents the change of flow under different stenosis degrees based on normal conditions. It can be employed to compare the blood flow under different conditions and is an effective index for gauging the coronary artery's ability to supply blood to the myocardium.
The cross-sectional area of the coronary artery is decreased by 20%, 40%, 60%, and 80% to simulate different degrees of stenosis. The pressure-related FFR and flow rate-related relative flow are analyzed to study the blood supply capacity under coronary artery stenosis.
In all numerical simulations, the heart rate is set to 75 beats/min, so one cardiac cycle is 60/75 = 0.8 s. Using the IBAMR open source software platform (http://github.com/IBAMR/IBAMR) to implement the AV FSI simulator introduced in Section 2.1, the aortic root pressure and the flow rate through the aortic valve are in line with the real physiological conditions, as shown in Figure 5. The systolic/diastolic blood pressure of the aorta is maintained within the normal physiological range (120/80 mmHg). With the aortic valve opening, blood flows into the aorta, and the flow rate increases to a peak of 572.43 ml/s. When the aortic valve closes, regurgitation occurrs and the backflow rate can reach up to 170.70 ml/s. Over the course of a single cardiac cycle, the volume of blood that flows into the aorta is 89.73 ml. The numerical results align well with the experimental data reported in articles [49] and [50].
It should be noted that there are oscillations in the pressure and flow rate curves in Figure 5, which are mainly caused by numerical effects and also involve physiological effects. The oscillations occur at about 0.4 s. This period is the stage when the aortic valve is about to close completely, and the hemodynamic behavior is particularly complex. The AV FSI simulator cannot accurately simulate this phenomenon.
In order to obtain the parameters that can better describe the properties, the RLC parameters in the coronary artery lumped parameter model are optimized as introduced in Section 2.3. For the right coronary artery model, the numerical results of Duanmu et al. [10] (the flow rate and pressure of RCA and PLA) are selected as the reference solution, and their left ventricular pressure and aortic pressure are used as the boundary conditions to complete the parameter optimization. The comparison results are shown in Figure 6. The black circular data are the results of Duanmu et al. [10], and the red triangle data are numerical results after parameter optimization. It can be seen that the numerical results of the flow rate and pressure after parameter optimization are in good agreement with the reference solution. The fitness values of pressure and flow rate waveforms are calculated separately. Specifically, the pressure-related fitness value is 0.195, while the flow rate-related fitness value is 0.239. Therefore, the optimization result of the pressure waveform seems to be more ideal than that of the flow rate waveform.
For the left coronary artery model, the numerical results of Pietrabissa et al. [9] (the flow rate of LMCA, LAD, and SPT2) are selected as the reference solution, and their left ventricular pressure and aortic pressure are used as the boundary conditions to obtain the parameter optimization values. Figure 7 presents the comparison of the flow rate numerical results with those obtained by Pietrabissa et al. [9]. It can be seen that the numerical results of the flow rate after parameter optimization are similar to the waveforms of the reference solution. After parameter optimization, the objective function values of the left and right coronary arteries calculated by Eq (2.10) are 0.526 and 0.209, respectively. This shows that optimizing the right coronary artery model is easier than optimizing the left coronary artery model. On the one hand, the complexity of the structure of the left and right coronary arteries is different; the left coronary artery has more branches, its structure is more complex, and the parameter optimization is more difficult. On the other hand, the choice of the reference solution is different. The left coronary artery lacks the pressure reference solution, and the number of reference solutions is relatively scarce. To address this issue, we employed two strategies. First, the RLC parameters with an ideal optimization effect are fixed, reducing the number of variables while dynamically adjusting them based on optimization outcomes. Second, the numerical results with an ideal optimization effect can be approximated as a reference solution, increasing the available data. Table 2 lists the optimized parameter values of the 0D coronary artery model.
We first set the aortic root pressure calculated by the AV FSI simulator as the inlet boundary condition, and the corresponding flow rate of each segment of the left coronary artery is shown in Figure 8. The blood flow dynamics in the coronary artery differ from those in other sections of the arterial system. During the systolic period (the time is 0–0.39 s), the left ventricle contracts, the intramyocardial pressure increases, the extravascular pressure of the left coronary artery increases, and the blood flow is restricted, resulting in a decrease in the flow rate, which is not synchronized with aortic flow. Then, it increases with the decrease in intramyocardial pressure until entering the diastolic period (the time is 0.39–0.8 s). The trend is well-captured in Figure 8. The relative position of the coronary artery in the heart also has an effect on the flow rate. For example, the flow rate of the epicardial coronary artery (e.g., LMCA, LAD, and LCX) in the systolic period is not as pronounced as that of the endocardial coronary artery (e.g., SPT2). Specifically, the flow rate of the endocardial coronary artery decreases rapidly at the beginning of the systolic period and maintains a low level for a period of time. The flow rate of the epicardial coronary artery decreases slowly during systole, and there is a slight increase. During diastole, the flow rate of the endocardial coronary artery and epicardial coronary artery increases rapidly.
Figure 9(a) depicts the flow rate waveforms at various spatial locations along the main trunk, starting from the entrance of the LMCA trunk (LMCA, LAD, LAD2, and LAD4). It can be observed that the magnitude of the flow rate wave decreases significantly, but the waveforms remain basically unchanged. The flow rate waveforms in the LCX trunk (LCX, LCX1, LCX2, LCX3) shown in Figure 9(b) also present the same trend. In contrast, the pressure waveforms change little at different positions in the same trunk, as illustrated in Figure 9(c).
Figure 10 shows the flow rate and pressure of each segment of the right coronary artery. In the systolic period, the flow rate in the right coronary artery does not significantly decrease compared with the left coronary artery. This is mainly due to the lower pressure environment in which the right ventricle functions, with its peak pressure being only about one-fifth of the mean aortic pressure [51]. Based on the numerical results obtained from the 0D coronary artery model, it is estimated that during a single cardiac cycle, the blood flow entering the coronary artery distributes as follows: 3.96 ml into the overall coronary artery, with 3.11 ml flowing to the left coronary artery and 0.85 ml directed toward the right coronary artery. In total, the coronary artery blood flow accounted for about 4.41% of the cardiac output.
Coronary artery stenosis is very important for the study of coronary artery blood supply capacity, which leads to a decrease in the cross-sectional area of blood vessels, hindering blood flow. In severe cases, it can cause myocardial ischemia. The coronary artery stenosis model is established by changing the RLC parameters of a specific segment; see Eqs (2.11) and (2.12). In these simulations, we observe the changes in flow rate and pressure at different degrees of stenosis (20%, 40%, 60%, and 80%) to study how the stenosis rate affects flow rate and pressure. Figure 11 illustrates the relationship between flow rate, pressure, and stenosis rate in the PDA of the right coronary artery. We find that when the stenosis rate rises, the flow rate and the pressure decrease, and when the stenosis rate is higher than 60%, the blood pressure decreases significantly.
In the left coronary artery, six typical blood vessels (LAD, LAD2, SPT1, LCX, LCX2, and MARG2) are selected for stenosis simulation according to different locations (different branches and different generations). The relationship between flow rate, pressure, and stenosis rate of the left coronary artery is similar to that of the right coronary artery. Figure 12 displays the variations in flow rate and pressure across six different segments at varying stenosis rates. The relationship between flow rate, pressure, and stenosis rate in the left coronary artery is analogous to that in the right coronary artery. Compared with flow rate, pressure decreases gradually with an increase in stenosis rate, which indicates that blood pressure is a more stable diagnostic criterion than flow rate in clinical medicine.
According to the flow rate and pressure at different stenosis rates, the relative flow and FFR can be calculated. Figure 13(a) shows the relative flow of the right coronary artery PDA when PDA is stenosed, and its FFR is shown in Figure 13(b), which is calculated by Eq (2.13). The FFR of PDA in healthy state is 0.98. With the increase in stenosis rate, the relative flow and FFR both decrease. The rate of change in relative flow and FFR increases significantly when the stenosis rate exceeds 60%, consistent with Figure 11. When the stenosis rate is higher than 72%, the FFR is lower than 0.8, reaching the threshold of myocardial ischemia.
In the left coronary artery, the relative flow and FFR of LMCA trunk (LAD, LAD2, and LAD4), LCX trunk (LCX1, LCX2, and LCX3), and MARGs at different locations (MARG1, MARG2, and MARG3) are calculated and shown in Figure 14. Similar to PDA, the relative flow and FFR of the left coronary artery decrease with increased stenosis. The FFR of each vessel segment of the LMCA trunk in the healthy state is 0.990, 0.989, and 0.967, respectively. The FFR of the LCX trunk and MARGs are also above 0.97. When the stenosis rate is between 50% and 60%, the relative flow and FFR begin to decrease significantly. In addition, for the LMCA trunk, LCX trunk, and MARGs, the decrease in relative flow and FFR becomes more and more significant with the increase in generation.
In a cardiac cycle, the movement of the aortic valve occurs mainly in the systolic period. The strong contraction of the ventricular myocardium leads to a dramatic rise in the intraventricular pressure. Once the left ventricular pressure surpasses the aortic pressure, the aortic valve opens, and the blood starts to flow into the aorta. When the left ventricular pressure reaches its peak, the aortic valve is completely opened, and blood rushes into the aorta. The maximum flow rate was 572.43 ml/s. As the left ventricular pressure decreases below that of the aorta, the aortic valve begins to close. The AV FSI simulator can well simulate the hemodynamics of the aortic valve in a cardiac cycle and is consistent with clinical measurement data and published literature results [49,50]. Therefore, the AV FSI simulator can provide the boundary condition in a healthy state for the 0D model to achieve a more realistic simulation. In the future, we plan to introduce more boundary conditions in different states to simulate the performance of the cardiovascular system in various complex situations.
Taking the results of the coronary artery published by Pietrabissa et al. [9] and Duanmu et al. [10] as the reference solution, the PSO algorithm is utilized to optimize the RLC parameters in the 0D model. Because the right coronary artery has a simple structure (including only three vessels) and gives more reference solution, its optimization outcome has superior performance compared to that of the left coronary artery. Table 2 presents the RLC parameter values for each segment of the coronary artery. These optimized parameters are then implemented in subsequent studies.
The 0D coronary artery model established in this paper can simulate the flow rate and pressure of all vessel segments in a cardiac cycle and evaluate the blood supply capacity of the coronary artery. The predictions align well with previous research findings and clinical experimental data. However, there is oscillation in the numerical result, which may be due to the influence of discretization error, mesh accuracy, boundary condition processing, and other factors. The flow rate in the coronary artery is highly correlated with the heart and arterial system. During the systolic period, the driving force for perfusing the left myocardial is the difference between the intramyocardial pressure and the aortic pressure, and the flow rate decreases with myocardial contraction [10,17,51] and then increases with the reduction of intramyocardial pressure until the beginning of diastole. However, the flow rate in the right coronary artery does not significantly decrease during the systolic period because the lower pressure environment within the right ventricle is compared to the left ventricle [9,39]. In addition, we also studied the flow rate and pressure waveforms along the different spatial positions of the trunk. Since the different cross-sectional areas of vessels along the trunk, the flow rate shows different amplitudes. Concretely, the flow rate waveforms tend to scale (normalized relative to their average) into a curve except for a small phase difference, and the pressure waveforms change little. Huo et al. [52] discovered that the attenuation of the pressure waveforms' amplitude is particularly pronounced in blood vessels with a diameter less than 10 μm. Under normal circumstances, the average coronary blood flow constitutes 4% to 5% of the total cardiac output [51], and our numerical result is 4.41%.
To investigate the impact of coronary artery stenosis on both flow rate and pressure dynamics and evaluate the blood supply capacity of the stenotic coronary artery, the stenosis model is established to simulate the flow rate and pressure of each segment of the coronary artery when the stenosis rate varied between 20% and 80%. As the stenosis rate increases, the flow rate and pressure decrease to varying degrees. In clinical medicine, pressure is a very stable diagnostic criterion because the reduction of pressure is more regular [10]. The flow rate-related relative flow and pressure-related FFR show good concurrence with the previously published experimental data [7,53]. With the increase in stenosis rate, the relative flow and FFR have the same trend, which is decreasing. Hamilos et al. [54] showed that the FFR value of the stenosis rate between 60% and 70% is between 0.68 and 0.97. Koo et al. [55] found that when the stenosis rate is 79 ± 11%, the average FFR is 0.81 ± 0.12, and only one-third of lesions exhibit an FFR value below 0.75. For intermediate blood vessel segments, the FFR in healthy status is not less than 0.97, while for the terminal vessels, the range is between 0.9 and 0.97 [16].
However, the coronary artery model still has some limitations. This model ignored the interplay between the heart, the arterial system, and the coronary artery, and it could not predict wave propagation. We assume that the RLC parameters are always constant, which is approximately true in the diastolic period, but in the systolic period, the parameters will change due to ventricular contraction [7]. For a solitary lesion, the occurrence of ischemia is affected by many factors, such as the shape and location of the lesion, the size and function of the myocardium being perfused, and the degree of collateral circulation [56,57,58]. These factors are not taken into account in the coronary artery stenosis model.
In this paper, we establish a complete coronary artery lumped parameter model based on the similarity between the circuit system and the cardiovascular system, including the left and right coronary arteries. The model uses RLC parameters to describe each vessel segment and introduces the AV FSI simulator to calculate the inlet boundary condition. It can predict the pressure and flow rate distribution throughout the coronary artery tree up to the terminal blood vessel and evaluate the blood supply capacity under health status and different degrees of stenosis. The relative flow and FFR align well with the published literature. The model can effectively simulate the common characteristics of coronary artery hemodynamics and be easily transformed into clinical practice for real-time clinical diagnosis.
The authors declare they have not used Artificial Intelligence (AI) tools in the creation of this article.
This work is supported by National Natural Science Foundation of China (Grant No. 12271440), which are gratefully acknowledged.
The authors declare there is no conflict of interest.
The PSO algorithm is programmed on the MATLAB platform without relying on any ready-made libraries or toolkits. It is constructed according to the core idea of the PSO algorithm. Its pseudocode is as follows:
Algorithm 1 PSO algorithm. |
for each particle i do |
Initialize velocity vi and position xi for particle i |
Evaluate particle i and set optimal position of individual history pBesti=xi |
end for |
Optimal position of group history gBest=min{pBesti} |
while no stop do |
for i=1 to N do |
Update the velocity and position of particle i using Eq (9.1) |
Evaluate particle i |
if Fmin(xi)<Fmin(pBesti) then |
pBesti=xi |
end if |
if Fmin(pBesti)<Fmin(gBest) then |
gBest=pBesti |
end if |
end for |
end while |
vk+1id=ωvkid+c1rand1(pBestkid−xkid)+c2rand2(gBestkd−xkid),xk+1id=xkid+vkid, | (A.1) |
where N is the particle swarm size set to 200. k is the current number of iterations and cannot exceed the maximum number of iterations of 400. d represents the particle dimension, that is, the number of independent variables, and there are 60 RLC parameters to be optimized in this paper. The inertia weight ω = 0.7, the individual learning factor (c1), and group learning factor (c2) are set to 0.5. rand1 and rand2 are two random numbers over [0, 1] intervals.
[1] |
C. W. Tsao, A. W. Aday, Z. I. Almarzooq, A. Alonso, A. Z. Beaton, M. S. Bittencourt, et al., Heart disease and stroke statistics–2022 update: A report from the american heart association, Circulation, 145 (2022), e153–e639. https://doi.org/10.1161/CIR.0000000000001052 doi: 10.1161/CIR.0000000000001052
![]() |
[2] |
L. Papamanolis, H. J. Kim, C. Jaquet, M. Sinclair, M. Schaap, I. Danad, et al., Myocardial perfusion simulation for coronary artery disease: A coupled patient-specific multiscale model, Ann. Biomed. Eng., 49 (2021), 1432–1447. https://doi.org/10.1007/s10439-020-02681-z doi: 10.1007/s10439-020-02681-z
![]() |
[3] |
V. C. Rideout, J. A. Katra, Computer simulation study of the pulmonary circulation, Simulation, 12 (1969), 239–245. https://doi.org/10.1177/003754976901200505 doi: 10.1177/003754976901200505
![]() |
[4] |
M. F. Snyder, V. C. Rideout, Computer simulation studies of the venous circulation, IEEE Trans. Biomed. Eng., BME-16 (1969), 325–334. https://doi.org/10.1109/TBME.1969.4502663 doi: 10.1109/TBME.1969.4502663
![]() |
[5] |
V. C. Rideout, Cardiovascular system simulation in biomedical engineering education, IEEE Trans. Biomed. Eng., BME-19 (1972), 101–107. https://doi.org/10.1109/TBME.1972.324103 doi: 10.1109/TBME.1972.324103
![]() |
[6] |
F. Liang, H. Liu, A closed-loop lumped parameter computational model for human cardiovascular system, JSME Int. J., Ser. C, 48 (2005), 484–493. https://doi.org/10.1299/jsmec.48.484 doi: 10.1299/jsmec.48.484
![]() |
[7] |
J. Wang, B. Tie, W. Welkowitz, J. Kostis, J. Semmlow, Incremental network analogue model of the coronary artery, Med. Biol. Eng. Comput., 27 (1989), 416–422. https://doi.org/10.1007/BF02441434 doi: 10.1007/BF02441434
![]() |
[8] |
S. Mantero, R. Pietrabissa, R. Fumero, The coronary bed and its role in the cardiovascular system: A review and an introductory single-branch model, J. Biomed. Eng., 14 (1992), 109–116. https://doi.org/10.1016/0141-5425(92)90015-d doi: 10.1016/0141-5425(92)90015-d
![]() |
[9] |
R. Pietrabissa, S. Mantero, T. Marotta, L. Menicanti, A lumped parameter model to evaluate the fluid dynamics of different coronary bypasses, Med. Eng. Phys., 18 (1996), 477–484. https://doi.org/10.1016/1350-4533(96)00002-1 doi: 10.1016/1350-4533(96)00002-1
![]() |
[10] |
Z. Duanmu, M. Yin, X. Fan, X. Yang, X. Luo, A patient-specific lumped-parameter model of coronary circulation, Sci. Rep., 8 (2018), 874. https://doi.org/10.1038/s41598-018-19164-w doi: 10.1038/s41598-018-19164-w
![]() |
[11] |
N. Westerhof, G. Elzinga, P. Sipkema, An artificial arterial system for pumping hearts, J. Appl. Physiol., 31 (1971), 776–781. https://doi.org/10.1152/jappl.1971.31.5.776 doi: 10.1152/jappl.1971.31.5.776
![]() |
[12] | A. S. Olufsen, Modeling the Arterial System with Reference to an Anesthesia Simulator, PhD. thesis, Roskilde University, 1998. |
[13] |
M. S. Olufsen, Structured tree outflow condition for blood flow in larger systemic arteries, J. Appl. Physiol. Heart Circulat. Physiol., 276 (1999), H257–H268. https://doi.org/10.1152/ajpheart.1999.276.1.H257 doi: 10.1152/ajpheart.1999.276.1.H257
![]() |
[14] |
M. S. Olufsen, C. S. Peskin, W. Y. Kim, E. M. Pedersen, A. Nadim, J. Larsen, Numerical simulation and experimental validation of blood flow in arteries with structured-tree outflow conditions, Ann. Biomed. Eng., 28 (2000), 1281–1299. https://doi.org/10.1114/1.1326031 doi: 10.1114/1.1326031
![]() |
[15] |
Y. Zhang, T. Furusawa, S. F. Sia, M. Umezu, Y. Qian, Proposition of an outflow boundary approach for carotid artery stenosis CFD simulation, Comput. Method Biomech. Biomed. Eng., 16 (2013), 488–494. https://doi.org/10.1080/10255842.2011.625358 doi: 10.1080/10255842.2011.625358
![]() |
[16] |
C. Pagiatakis, J. C. Tardif, P. L. L'Allier, R. Mongrain, A numerical investigation of the functionality of coronary bifurcation lesions with respect to lesion configuration and stenosis severity, J. Biomech., 48 (2015), 3103–3111. https://doi.org/10.1016/j.jbiomech.2015.07.018 doi: 10.1016/j.jbiomech.2015.07.018
![]() |
[17] |
C. Pagiatakis, J. C. Tardif, P. L. L'Allier, R. Mongrain, Effect of stenosis eccentricity on the functionality of coronary bifurcation lesions—A numerical study, Med. Biol. Eng. Comput., 55 (2017), 2079–2095. https://doi.org/10.1007/s11517-017-1653-7 doi: 10.1007/s11517-017-1653-7
![]() |
[18] |
A. S. Yong, A. C. Ng, D. Brieger, H. C. Lowe, M. K. Ng, L. Kritharides, Three-dimensional and two-dimensional quantitative coronary angiography, and their prediction of reduced fractional flow reserve, Eur. Heart J., 32 (2011), 345–353. https://doi.org/10.1093/eurheartj/ehq259 doi: 10.1093/eurheartj/ehq259
![]() |
[19] |
S. Sen, J. Escaned, I. S. Malik, G. W. Mikhail, R. A. Foale, R. Mila, et al., Development and validation of a new adenosine-independent index of stenosis severity from coronary wave-intensity analysis: results of the advise (adenosine vasodilator independent stenosis evaluation) study, J. Am. Coll. Cardiol., 59 (2012), 1392–1402. https://doi.org/10.1016/j.jacc.2011.11.003 doi: 10.1016/j.jacc.2011.11.003
![]() |
[20] |
Z. Yan, Z. Yao, W. Guo, D. Shang, R. Chen, J. Liu, et al., Impact of pressure wire on fractional flow reserve and hemodynamics of the coronary arteries: A computational and clinical study, IEEE Trans. Biomed. Eng., 70 (2022), 1683–1691. https://doi.org/10.1109/tbme.2022.3225188 doi: 10.1109/tbme.2022.3225188
![]() |
[21] |
B. De Bruyne, J. Bartunek, S. U. Sys, G. R. Heyndrickx, Relation between myocardial fractional flow reserve calculated from coronary pressure measurements and exercise-induced myocardial ischemia, Circulation, 92 (1995), 39–46. https://doi.org/10.1161/01.cir.92.1.39 doi: 10.1161/01.cir.92.1.39
![]() |
[22] |
N. H. Pijls, B. Van Gelder, P. Van der Voort, K. Peels, F. A. Bracke, H. J. Bonnier, et al., Fractional flow reserve: A useful index to evaluate the influence of an epicardial coronary stenosis on myocardial blood flow, Circulation, 92 (1995), 3183–3193. https://doi.org/10.1161/01.cir.92.11.3183 doi: 10.1161/01.cir.92.11.3183
![]() |
[23] |
L. Cai, R. Zhang, Y. Li, G. Zhu, X. Ma, Y. Wang, et al., The comparison of different constitutive laws and fiber architectures for the aortic valve on fluid-structure interaction simulation, Front. Physiol., 12 (2021), 725. https://doi.org/10.3389/fphys.2021.682893 doi: 10.3389/fphys.2021.682893
![]() |
[24] |
L. Cai, Y. Hao, P. Ma, G. Zhu, X. Luo, H. Gao, Fluid-structure interaction simulation of calcified aortic valve stenosis, Math. Biosci. Eng., 19 (2022), 13172–13192. https://doi.org/10.3934/mbe.2022616 doi: 10.3934/mbe.2022616
![]() |
[25] |
N. Westerhof, J. W. Lankhaar, B. E. Westerhof, The arterial windkessel, Med. Biol. Eng. Comput., 47 (2009), 131–141. https://doi.org/10.1007/s11517-008-0359-2 doi: 10.1007/s11517-008-0359-2
![]() |
[26] |
Q. Wang, W. Sun, Finite element modeling of mitral valve dynamic deformation using patient-specific multi-slices computed tomography scans, Ann. Biomed. Eng., 41 (2013), 142–153. https://doi.org/10.1007/s10439-012-0620-6 doi: 10.1007/s10439-012-0620-6
![]() |
[27] |
N. Stergiopulos, B. E. Westerhof, N. Westerhof, Total arterial inertance as the fourth element of the windkessel model, J. Appl. Physiol. Heart Circulat. Physiol., 276 (1999), H81–H88. https://doi.org/10.1152/ajpheart.1999.276.1.H81 doi: 10.1152/ajpheart.1999.276.1.H81
![]() |
[28] |
B. E. Griffith, Immersed boundary model of aortic heart valve dynamics with physiological driving and loading conditions, Int. J. Numer. Method. Biomed. Eng., 28 (2012), 317–345. https://doi.org/10.1002/cnm.1445 doi: 10.1002/cnm.1445
![]() |
[29] |
B. E. Griffith, X. Luo, Hybrid finite difference/finite element immersed boundary method, Int. J. Numer. Method. Biomed. Eng., 33 (2017), e2888. https://doi.org/10.1002/cnm.2888 doi: 10.1002/cnm.2888
![]() |
[30] |
H. Gao, X. Ma, N. Qi, C. Berry, B. E. Griffith, X. Luo, A finite strain nonlinear human mitral valve model with fluid-structure interaction, Int. J. Numer. Method. Biomed. Eng., 30 (2014), 1597–1613. https://doi.org/10.1002/cnm.2691 doi: 10.1002/cnm.2691
![]() |
[31] |
C. S. Peskin, The immersed boundary method, Acta Numer., 11 (2002), 479–517. https://doi.org/10.1016/j.jcp.2023.112148 doi: 10.1016/j.jcp.2023.112148
![]() |
[32] |
D. Boffi, L. Gastaldi, L. Heltai, C. S. Peskin, On the hyper-elastic formulation of the immersed boundary method, Comput. Methods Appl. Mech. Eng., 197 (2008), 2210–2231. https://doi.org/10.1016/j.cma.2007.09.015 doi: 10.1016/j.cma.2007.09.015
![]() |
[33] | C. Vlachopoulos, M. O'Rourke, W. W. Nichols, McDonald's Blood Flow in Arteries: Theoretical, Experimental and Clinical Principles, 6th edition, CRC Press, London, 2012. https://doi.org/10.1201/b13568 |
[34] |
Y. Liu, L. Cai, Y. Chen, P. Ma, Q. Zhong, Variable separated physics-informed neural networks based on adaptive weighted loss functions for blood flow model, Comput. Math. Appl., 153 (2024), 108–122. https://doi.org/10.1016/j.camwa.2023.11.018 doi: 10.1016/j.camwa.2023.11.018
![]() |
[35] | L. R. Waite, Biofluid Mechanics in Cardiovascular Systems, McGraw Hill Professional, 2005. https://doi.org/10.1036/0071447881 |
[36] |
J. R. Mitchell, J. J. Wang, Expanding application of the Wiggers diagram to teach cardiovascular physiology, Adv. Physiol. Educ., 38 (2014), 170–175. https://doi.org/10.1152/advan.00123.2013 doi: 10.1152/advan.00123.2013
![]() |
[37] |
V. Fester, B. Mbiya, P. Slatter, Energy losses of non-Newtonian fluids in sudden pipe contractions, Chem. Eng. J., 145 (2008), 57–63. https://doi.org/10.1016/j.cej.2008.03.003 doi: 10.1016/j.cej.2008.03.003
![]() |
[38] |
A. Iberall, Anatomy and steady flow characteristics of the arterial system with an introduction to its pulsatile characteristics, Math. Biosci., 1 (1967), 375–395. https://doi.org/10.1016/0025-5564(67)90009-0 doi: 10.1016/0025-5564(67)90009-0
![]() |
[39] |
H. J. Kim, I. Vignon-Clementel, J. Coogan, C. Figueroa, K. Jansen, C. Taylor, Patient-specific modeling of blood flow and pressure in human coronary arteries, Ann. Biomed. Eng., 38 (2010), 3195–3209. https://doi.org/10.1007/s10439-010-0083-6 doi: 10.1007/s10439-010-0083-6
![]() |
[40] | L. P. Huelsman, Basic Circuit Theory: with Digital Computations, Prentice-Hall, 1972. |
[41] |
B. S. Gow, D. Schonfeld, D. J. Patel, The dynamic elastic properties of the canine left circumflex coronary artery, J. Biomech., 7 (1974), 389–395. https://doi.org/10.1016/0021-9290(74)90001-3 doi: 10.1016/0021-9290(74)90001-3
![]() |
[42] |
W. Welkowitz, Engineering hemodynamics: Application to cardiac assist devices, J. Clin. Eng., 13 (1988), 79. https://doi.org/10.1097/00004669-198803000-00003 doi: 10.1097/00004669-198803000-00003
![]() |
[43] | P. Siena, M. Girfoglio, G. Rozza, Fast and accurate numerical simulations for the study of coronary artery bypass grafts by artificial neural networks, in Reduced Order Models for the Biomechanics of Living Organs, (2023), 167–183. |
[44] |
N. M. Marazzi, G. Guidoboni, M. Zaid, L. Sala, S. Ahmad, L. Despins, et al., Combining physiology-based modeling and evolutionary algorithms for personalized, noninvasive cardiovascular assessment based on electrocardiography and ballistocardiography, Front. Physiol., 12 (2022), 739035. https://doi.org/10.3389/fphys.2021.739035 doi: 10.3389/fphys.2021.739035
![]() |
[45] | J. Kennedy, R. Eberhart, Particle swarm optimization, in International Conference on Neural Networks, 4 (1995), 1942–1948. https://doi.org/10.1109/ICNN.1995.488968 |
[46] | Y. Shi, R. Eberhart, A modified particle swarm optimizer, in IEEE International Conference on Evolutionary Computation, (1998), 69–73. https://doi.org/10.1109/ICEC.1998.699146 |
[47] |
J. R. Womersley, Oscillatory flow in arteries: The constrained elastic tube as a model of arterial flow and pulse transmission, Phys. Med. Biol., 2 (1957), 178–187. https://doi.org/10.1088/0031-9155/2/2/305 doi: 10.1088/0031-9155/2/2/305
![]() |
[48] |
N. H. Pijls, B. De Bruyne, Coronary pressure measurement and fractional flow reserve, Heart, 80 (1998), 539–542. https://doi.org/10.1136/hrt.80.6.539 doi: 10.1136/hrt.80.6.539
![]() |
[49] |
J. H. Lee, A. D. Rygg, E. M. Kolahdouz, S. Rossi, S. M. Retta, N. Duraiswamy, et al., Fluid-structure interaction models of bioprosthetic heart valve dynamics in an experimental pulse duplicator, Ann. Biomed. Eng., 48 (2020), 1475–1490. https://doi.org/10.1007/s10439-020-02466-4 doi: 10.1007/s10439-020-02466-4
![]() |
[50] |
G. Zhu, M. B. Ismail, M. Nakao, Q. Yuan, J. H. Yeo, Numerical and in-vitro experimental assessment of the performance of a novel designed expanded-polytetrafluoroethylene stentless bi-leaflet valve for aortic valve replacement, PLoS One, 14 (2019), e0210780. https://doi.org/10.1371/journal.pone.0210780 doi: 10.1371/journal.pone.0210780
![]() |
[51] |
L. H. Opie, Heart physiology: from cell to circulation, Circulation, 110 (2004), e313. https://doi.org/10.1161/01.cir.0000143724.99618.62 doi: 10.1161/01.cir.0000143724.99618.62
![]() |
[52] |
Y. Huo, G. S. Kassab, A hybrid one-dimensional/womersley model of pulsatile blood flow in the entire coronary arterial tree, J. Appl. Physiol. Heart Circulat. Physiol., 292 (2007), H2623–H2633. https://doi.org/10.1152/ajpheart.00987.2006 doi: 10.1152/ajpheart.00987.2006
![]() |
[53] |
P. Meimoun, S. Sayah, A. Luycx-Bore, J. Boulanger, F. Elmkies, T. Benali, et al., Comparison bween non-invasive coronary flow reserve and fractional flow reserve to assess the functional significance of left anterior descending artery stenosis of intermediate severity, J. Am. Soc. Echocardiog., 24 (2011), 374–381. https://doi.org/10.1016/j.echo.2010.12.007 doi: 10.1016/j.echo.2010.12.007
![]() |
[54] |
M. Hamilos, O. Muller, T. Cuisset, A. Ntalianis, G. Chlouverakis, G. Sarno, et al., Long-term clinical outcome after fractional flow reserve-guided treatment in patients with angiographically equivocal left main coronary artery stenosis, Circulation, 120 (2009), 1505–1512. https://doi.org/10.1161/circulationaha.109.850073 doi: 10.1161/circulationaha.109.850073
![]() |
[55] |
B. K. Koo, K. W. Park, H. J. Kang, Y. S. Cho, W. Y. Chung, T. J. Youn, et al., Physiological evaluation of the provisional side-branch intervention strategy for bifurcation lesions using fractional flow reserve, Eur. Heart J., 29 (2008), 726–732. https://doi.org/10.1093/eurheartj/ehn045 doi: 10.1093/eurheartj/ehn045
![]() |
[56] |
N. Pijls, J. Van Son, R. L. Kirkeeide, B. De Bruyne, K. L. Gould, Experimental basis of determining maximum coronary, myocardial, and collateral blood flow by pressure measurements for assessing functional stenosis severity before and after percutaneous transluminal coronary angioplasty, Circulation, 87 (1993), 1354–1367. https://doi.org/10.1161/01.cir.87.4.1354 doi: 10.1161/01.cir.87.4.1354
![]() |
[57] |
N. H. Pijls, J. W. E. Sels, Functional measurement of coronary stenosis, J. Am. Coll. Cardiol., 59 (2012), 1045–1057. https://doi.org/10.1016/j.jacc.2011.09.077 doi: 10.1016/j.jacc.2011.09.077
![]() |
[58] |
M. P. Opolski, C. Kepka, S. Achenbach, J. Pregowski, M. Kruk, A. D. Staruch, et al., Advanced computed tomographic anatomical and morphometric plaque analysis for prediction of fractional flow reserve in intermediate coronary lesions, Eur. J. Radiol., 83 (2014), 135–141. https://doi.org/10.1016/j.ejrad.2013.10.005 doi: 10.1016/j.ejrad.2013.10.005
![]() |
Segment | Results of Eq (2.9) | Optimization results | Z | ||||
R | L | C | R | L | C | ||
LMCA | 0.139 | 0.034 | 0.001 | 2.0159 | 0.01 | 0.00218 | |
LAD | 0.473 | 0.069 | 0.001 | 0.3685 | 0.001 | 0.0012 | |
LAD1 | 3.190 | 0.238 | 0.001 | 2.9761 | 0.2064 | 0.00163 | 145.6 |
LAD2 | 0.922 | 0.100 | 0.001 | 0.747 | 0.0017 | 0.00107 | |
SPT1 | 3.184 | 0.235 | 0.001 | 2.689 | 0.2031 | 0.0012 | 155.6 |
SPT2 | 2.906 | 0.287 | 0.002 | 3.8576 | 0.0017 | 0.0001 | 407.5 |
SPT3 | 2.819 | 0.281 | 0.002 | 3.6832 | 0.0014 | 0.0001 | 327 |
LAD3 | 6.803 | 0.238 | 0.0002 | 3.6025 | 0.009 | 0.00018 | 155 |
LAD4 | 4.775 | 0.353 | 0.001 | 3.731 | 0.1658 | 0.00188 | 80.2 |
DIAG | 1.941 | 0.184 | 0.001 | 2.0509 | 0.094 | 0.00194 | 55.1 |
LCX | 0.336 | 0.050 | 0.001 | 0.4362 | 0.001 | 0.00104 | |
MARG1 | 1.477 | 0.140 | 0.001 | 1.5214 | 0.184 | 0.00146 | 155 |
LCX1 | 0.401 | 0.048 | 0.001 | 0.2699 | 0.0264 | 0.00075 | |
MARG2 | 2.292 | 0.161 | 0.001 | 2.014 | 0.150 | 0.001 | 168.4 |
LCX2 | 0.856 | 0.082 | 0.001 | 0.9891 | 0.0082 | 0.00049 | |
MARG3 | 2.463 | 0.186 | 0.001 | 2.7604 | 0.0794 | 0.00083 | 72 |
LCX3 | 2.807 | 0.203 | 0.001 | 3.7397 | 0.1812 | 0.00095 | 170.1 |
RCA | 1.602 | 0.257 | 0.049 | 2.5284 | 0.5 | 0.02061 | |
PLA | 1.195 | 0.138 | 0.013 | 5.0 | 0.1896 | 0.01017 | 59.81 |
PDA | 2.078 | 0.170 | 0.008 | 4.6671 | 0.001 | 0.00913 | 139.72 |
F | 1.228 | 0.345 |
C10(kPa) | C01 | k1(kPa) | k2 | C(ml/mmHg) | Rp(mmHg⋅s/ml) | Rc (mmHg⋅s/ml) |
1.21 | 7.99 | 24.23 | 57.62 | 1.75 | 0.79 | 0.033 |
Segment | Results of Eq (2.9) | Optimization results | Z | ||||
R | L | C | R | L | C | ||
LMCA | 0.139 | 0.034 | 0.001 | 2.0159 | 0.01 | 0.00218 | |
LAD | 0.473 | 0.069 | 0.001 | 0.3685 | 0.001 | 0.0012 | |
LAD1 | 3.190 | 0.238 | 0.001 | 2.9761 | 0.2064 | 0.00163 | 145.6 |
LAD2 | 0.922 | 0.100 | 0.001 | 0.747 | 0.0017 | 0.00107 | |
SPT1 | 3.184 | 0.235 | 0.001 | 2.689 | 0.2031 | 0.0012 | 155.6 |
SPT2 | 2.906 | 0.287 | 0.002 | 3.8576 | 0.0017 | 0.0001 | 407.5 |
SPT3 | 2.819 | 0.281 | 0.002 | 3.6832 | 0.0014 | 0.0001 | 327 |
LAD3 | 6.803 | 0.238 | 0.0002 | 3.6025 | 0.009 | 0.00018 | 155 |
LAD4 | 4.775 | 0.353 | 0.001 | 3.731 | 0.1658 | 0.00188 | 80.2 |
DIAG | 1.941 | 0.184 | 0.001 | 2.0509 | 0.094 | 0.00194 | 55.1 |
LCX | 0.336 | 0.050 | 0.001 | 0.4362 | 0.001 | 0.00104 | |
MARG1 | 1.477 | 0.140 | 0.001 | 1.5214 | 0.184 | 0.00146 | 155 |
LCX1 | 0.401 | 0.048 | 0.001 | 0.2699 | 0.0264 | 0.00075 | |
MARG2 | 2.292 | 0.161 | 0.001 | 2.014 | 0.150 | 0.001 | 168.4 |
LCX2 | 0.856 | 0.082 | 0.001 | 0.9891 | 0.0082 | 0.00049 | |
MARG3 | 2.463 | 0.186 | 0.001 | 2.7604 | 0.0794 | 0.00083 | 72 |
LCX3 | 2.807 | 0.203 | 0.001 | 3.7397 | 0.1812 | 0.00095 | 170.1 |
RCA | 1.602 | 0.257 | 0.049 | 2.5284 | 0.5 | 0.02061 | |
PLA | 1.195 | 0.138 | 0.013 | 5.0 | 0.1896 | 0.01017 | 59.81 |
PDA | 2.078 | 0.170 | 0.008 | 4.6671 | 0.001 | 0.00913 | 139.72 |
F | 1.228 | 0.345 |