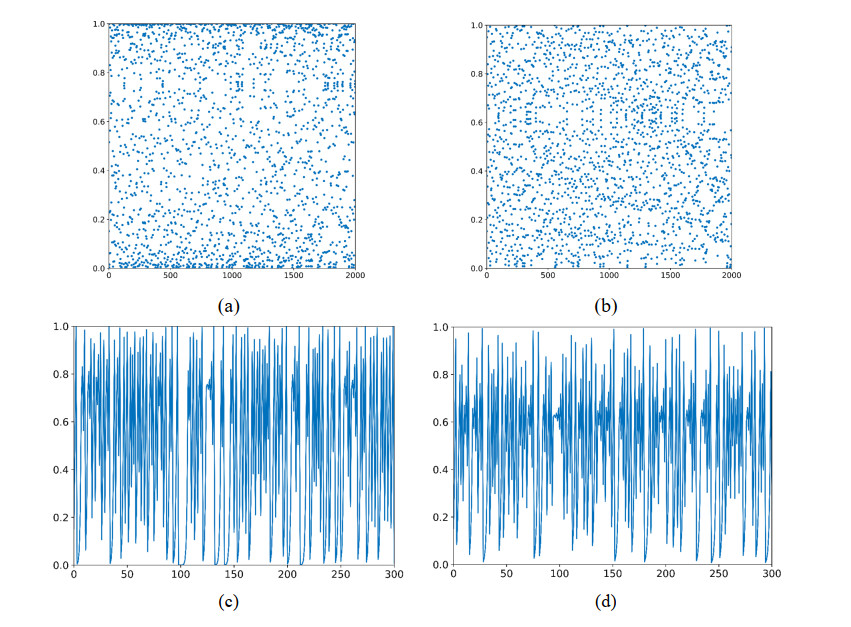
Statistical methodologies have broader applications in almost every sector of life including education, hydrology, reliability, management, and healthcare sciences. Among these sectors, statistical modeling and predicting data in the healthcare sector is very crucial. In this paper, we introduce a new method, namely, a new extended exponential family to update the distributional flexibility of the existing models. Based on this approach, a new version of the Weibull model, namely, a new extended exponential Weibull model is introduced. The applicability of the new extended exponential Weibull model is shown by considering two data sets taken from the health sciences. The first data set represents the mortality rate of the patients infected by the coronavirus disease 2019 (COVID-19) in Mexico. Whereas, the second set represents the mortality rate of COVID-19 patients in Holland. Utilizing the same data sets, we carry out forecasting using three machine learning (ML) methods including support vector regression (SVR), random forest (RF), and neural network autoregression (NNAR). To assess their forecasting performances, two statistical accuracy measures, namely, root mean square error (RMSE) and mean absolute error (MAE) are considered. Based on our findings, it is observed that the RF algorithm is very effective in predicting the death rate of the COVID-19 data in Mexico. Whereas, for the second data, the SVR performs better as compared to the other methods.
Citation: Yinghui Zhou, Zubair Ahmad, Zahra Almaspoor, Faridoon Khan, Elsayed tag-Eldin, Zahoor Iqbal, Mahmoud El-Morshedy. On the implementation of a new version of the Weibull distribution and machine learning approach to model the COVID-19 data[J]. Mathematical Biosciences and Engineering, 2023, 20(1): 337-364. doi: 10.3934/mbe.2023016
[1] | Qing Wu, Chunjiang Zhang, Mengya Zhang, Fajun Yang, Liang Gao . A modified comprehensive learning particle swarm optimizer and its application in cylindricity error evaluation problem. Mathematical Biosciences and Engineering, 2019, 16(3): 1190-1209. doi: 10.3934/mbe.2019057 |
[2] | Wenqiang Zhang, Chen Li, Mitsuo Gen, Weidong Yang, Zhongwei Zhang, Guohui Zhang . Multiobjective particle swarm optimization with direction search and differential evolution for distributed flow-shop scheduling problem. Mathematical Biosciences and Engineering, 2022, 19(9): 8833-8865. doi: 10.3934/mbe.2022410 |
[3] | Shangbo Zhou, Yuxiao Han, Long Sha, Shufang Zhu . A multi-sample particle swarm optimization algorithm based on electric field force. Mathematical Biosciences and Engineering, 2021, 18(6): 7464-7489. doi: 10.3934/mbe.2021369 |
[4] | Rongmei Geng, Renxin Ji, Shuanjin Zi . Research on task allocation of UAV cluster based on particle swarm quantization algorithm. Mathematical Biosciences and Engineering, 2023, 20(1): 18-33. doi: 10.3934/mbe.2023002 |
[5] | Xin Zhou, Shangbo Zhou, Yuxiao Han, Shufang Zhu . Lévy flight-based inverse adaptive comprehensive learning particle swarm optimization. Mathematical Biosciences and Engineering, 2022, 19(5): 5241-5268. doi: 10.3934/mbe.2022246 |
[6] | Xianzi Zhang, Yanmin Liu, Jie Yang, Jun Liu, Xiaoli Shu . Handling multi-objective optimization problems with a comprehensive indicator and layered particle swarm optimizer. Mathematical Biosciences and Engineering, 2023, 20(8): 14866-14898. doi: 10.3934/mbe.2023666 |
[7] | Dashe Li, Xueying Wang, Jiajun Sun, Huanhai Yang . AI-HydSu: An advanced hybrid approach using support vector regression and particle swarm optimization for dissolved oxygen forecasting. Mathematical Biosciences and Engineering, 2021, 18(4): 3646-3666. doi: 10.3934/mbe.2021182 |
[8] | Xiangyang Ren, Shuai Chen, Kunyuan Wang, Juan Tan . Design and application of improved sparrow search algorithm based on sine cosine and firefly perturbation. Mathematical Biosciences and Engineering, 2022, 19(11): 11422-11452. doi: 10.3934/mbe.2022533 |
[9] | Jinyun Jiang, Jianchen Cai, Qile Zhang, Kun Lan, Xiaoliang Jiang, Jun Wu . Group theoretic particle swarm optimization for gray-level medical image enhancement. Mathematical Biosciences and Engineering, 2023, 20(6): 10479-10494. doi: 10.3934/mbe.2023462 |
[10] | Li-zhen Du, Shanfu Ke, Zhen Wang, Jing Tao, Lianqing Yu, Hongjun Li . Research on multi-load AGV path planning of weaving workshop based on time priority. Mathematical Biosciences and Engineering, 2019, 16(4): 2277-2292. doi: 10.3934/mbe.2019113 |
Statistical methodologies have broader applications in almost every sector of life including education, hydrology, reliability, management, and healthcare sciences. Among these sectors, statistical modeling and predicting data in the healthcare sector is very crucial. In this paper, we introduce a new method, namely, a new extended exponential family to update the distributional flexibility of the existing models. Based on this approach, a new version of the Weibull model, namely, a new extended exponential Weibull model is introduced. The applicability of the new extended exponential Weibull model is shown by considering two data sets taken from the health sciences. The first data set represents the mortality rate of the patients infected by the coronavirus disease 2019 (COVID-19) in Mexico. Whereas, the second set represents the mortality rate of COVID-19 patients in Holland. Utilizing the same data sets, we carry out forecasting using three machine learning (ML) methods including support vector regression (SVR), random forest (RF), and neural network autoregression (NNAR). To assess their forecasting performances, two statistical accuracy measures, namely, root mean square error (RMSE) and mean absolute error (MAE) are considered. Based on our findings, it is observed that the RF algorithm is very effective in predicting the death rate of the COVID-19 data in Mexico. Whereas, for the second data, the SVR performs better as compared to the other methods.
In the past few decades, many meta-heuristic algorithms have been proposed to handle complex optimization problems, which are usually difficult to solve with traditional optimization methods relying on gradients. Meta-heuristic algorithms are problem-solving methods that mimic natural or abstract processes to efficiently explore solution spaces and find near-optimal solutions for complex optimization problems. Kennedy and Eberhart [1] developed particle swarm optimization algorithm (PSO), a population-based optimization algorithm inspired by social behavior and collective intelligence, where particles iteratively update their positions based on their own experience and the information from the best-performing particles in the swarm. Holland [2] proposed genetic algorithm (GA) that imitates the process of natural selection and genetic evolution to iteratively search for optimal solutions to complex problems. Wang et al. [3] proposed a monarch butterfly optimization algorithm (MBO) that imitates the migration behavior of monarch butterflies, where the search process is guided by a combination of exploration and exploitation strategies to find optimal solutions. Li et al. [4] presented a slime mold algorithm (SMA) that simulates the foraging behavior of slime mold to efficiently explore and exploit search spaces by forming networks of interconnected pathways. Wang [5] presented a moth search algorithm (MSA) that mimics the behavior of moths to navigate toward the optimal solution by adjusting their flight direction based on attraction and repulsion forces. Yang et al. [6] proposed a hunger games search (HGS) algorithm, where individuals compete for resources and survival. Ahmadianfar et al. [7] presented Runge-Kutta optimizer (RUN) that utilizes the logic of slope variations computed by the Runge-Kutta method to perform active exploration and exploitation for global optimization with an enhanced solution quality mechanism to avoid local optima and improve convergence speed. Tu et al. [8] proposed colony predation algorithm (CPA) that mimics the collective hunting behavior of predators to explore and exploit the solution space for optimization efficiently. Ahmadianfar et al. [9] presented INFO which utilizes the weighted mean concept, enhanced updating rules, vector combining and local search to optimize various problems. Heidari et al. [10] proposed Harris hawks optimization (HHO), which is inspired by the hunting behavior of Harris hawks, utilizing different strategies such as exploration, exploitation and cooperative hunting to solve optimization problems. Su et al. [11] proposed a RIME optimization algorithm, a nature-inspired metaheuristic algorithm based on the freezing process of water droplets in rime formation, utilizing dynamic freezing and melting mechanisms for efficient optimization. Compared with conventional algorithms, these meta-heuristic algorithms can handle non-differentiable, multimodal, hybrid and high-dimensional problems without requiring explicit gradient information.
After they are proposed, many researchers successfully solve complex optimization problems by using them in intelligent systems. Li et al. [12] introduced ACO-S, a bionic optimization algorithm-based dimension reduction method specifically designed for high-dimensional datasets like microarray data, demonstrating its ability to generate compact and informative gene subsets with high classification accuracy compared to existing bionic optimization algorithms. Thawkar et al. [13] presented a hybrid feature selection method, combining the butterfly optimization algorithm (BOA) and the ant lion optimizer (ALO) to effectively predict the benign or malignant status of breast tissue using mammogram images. Chakraborty et al. [14] developed a computational tool using a modified whale optimization method (WOA) to quickly and accurately determine the severity of COVID-19 illness through chest X-ray image analysis. Gao et al. [15] introduced a multi-objective optimization model and an improved genetic algorithm for cooperative mission assignment of heterogeneous UAVs, considering the balance between mission gains and UAV losses. Yu et al. [16] presented a multi-objective optimization problem and an improved genetic algorithm for cooperative mission planning of heterogeneous UAVs in cross-regional joint operations, considering makespan minimization, value expectation maximization, flexible base return and ammunition inventory constraints. Li et al. [17] investigated the scheduling problem of maintenance and repair tasks for carrier-based aircraft fleets in hangar bays, proposing a model and an improved teaching-learning-based optimization algorithm to enhance maintenance efficiency and reduce manual scheduling burden.
Among the algorithms mentioned above, PSO is popular because of its fast convergence, few parameters and easy programming implementation. However, the performance of the standard PSO depends mainly on its parameter settings [18] and different parameter settings may lead to different convergence outcomes. In addition, the update strategy of particles during the search process is mainly aimed at the global best, which leads the population to lose diversity in the population and increases the risk of being trapped in local optima [19,20,21]. To address these drawbacks and improve the performance of PSO, many PSO variants have been developed. Generally, research on PSO variants can be classified into three parts: parameter modification, population topology structure modification and novel learning strategy.
1) Parameter modification. PSO has some parameters such as inertia weight and acceleration coefficients. They have been modified in many ways. Shi and Eberhart [22] introduced the concept of inertia weight in PSO to improve velocity control and search effectiveness. Afterward, various adjustment strategies were advocated, including defining it as a time-varying function called global PSO (GPSO) [23]. Liu et al. [24] proposed a PSO algorithm with chaos inertia weight to enlarge the search range and increase population diversity. Chen et al. [25] proposed a hybrid PSO with sine cosine acceleration coefficients where the performance of PSO is improved by introducing sine and cosine acceleration coefficients. By using these strategies, the performance of PSO can be improved to some extent.
2) Population topology structure modification. In the standard PSO, the population size is fixed. A larger population size may increase computation costs, resulting in slow convergence, while a smaller size may converge in local optima. Some researchers have improved the performance of PSO by modifying the population topology structure. Kennedy and Mendes [26] proposed the local version of PSO (LPSO) with ring topology, in which different topologies based on neighborhood connections are used to maintain the diversity of the population. Liang and Suganthan [27] presented a dynamic multi-swarm PSO (DMS-PSO), in which the population comprises many small subgroups and a dynamic strategy is used to maintain population diversity.
3) Novel learning strategy. A well-designed learning strategy can enhance the efficiency and effectiveness of the PSO algorithm. Liang et al. [28] proposed the CLPSO, in which each particle updates its velocity by incorporating historical best information from different particles. This approach enables particles to acquire valuable information and improves their search behavior. Wang et al. [29] proposed a PSO-HLM algorithm that balances exploration and exploitation by utilizing a hybrid learning model and a multi-pools fusion strategy, improving convergence speed and avoiding local optima. Zhou et al. [30] proposed a LFIACL-PSO algorithm that enhances PSO by incorporating Lévy flight-based inverse learning, comprehensive learning strategy, ring-type topology and adaptive update of acceleration coefficients.
In this paper, an improved PSO combined with double-chaos search (DCS-PSO) is proposed. Chaotic dynamics is a branch of nonlinear dynamical systems. Chaos motion exhibits ergodicity, randomness and regularity properties and can traverse all states without repetition. For optimization problems, these properties of chaos can be used as a mechanism to avoid getting trapped in local optima during the search process. In DCS-PSO, we utilize two distinct chaotic systems to explore the search space globally and narrow it down, enabling PSO to focus on the vicinity of the optimal solution and alleviate its premature convergence caused by getting trapped in local optima. Additionally, we incorporate a chaotic motion search in parallel with the PSO search. If the solution obtained through the chaotic search outperforms the PSO's global best, it will be replaced with the solution from the chaotic motion to enhance population diversity. This strategy prevents all particles in the PSO from relying solely on the global optimal particle for learning, thereby facilitating escape from local optima.
The main contributions of this paper are described as follows.
1) Introducing a novel approach, DCS-PSO, which combines the strengths of PSO and chaotic dynamics.
2) Addressing the issue of premature convergence by narrowing down the search space using double-chaos search and enhancing population diversity through the logistic map, resulting in improved convergence accuracy and speed.
3) Conducting extensive experimental evaluations to demonstrate the effectiveness and superiority of DCS-PSO compared to standard PSO and other optimization algorithms commonly used in the field.
The rest of this paper is organized as follows. Section 2 introduces the related work. Section 3 describes the proposed DCS-PSO. Section 4 is the experimental studies. Conclusion and future work are provided in Section 5.
PSO is an optimization algorithm based on swarm intelligence inspired by collective behavior in ecosystems such as bird foraging. In PSO, the problem is treated as an optimization problem of finding the optimal solution in a D-dimensional search space, where each particle i in the population represents a possible solution and has two vectors: a position vector Xi=[xi,1,xi,2,⋅⋅⋅,xi,D] and a velocity vector Vi=[vi,1,vi,2,⋅⋅⋅,vi,D]. In searching for the optimal solution, particles move and interact with each other in the search space. The particle i adjusts its position based on the optimal position vector pbesti=[pbesti,1,pbesti,2,⋅⋅⋅pbesti,D] it has reached in the past and the globally optimal position vector gbest=[gbest1,gbest2,⋅⋅⋅,gbestD] discovered by the entire swarm. PSO attempts to find a balance between local search and global search in this way. The update rules for the velocity and position of particle i in the d th dimension are as follows:
v(n+1)i,d=v(n)i,d+c1×r1×(pbesti,d−xi,d)+c2×r2×(gbestd−xi,d), | (2.1) |
x(n+1)i,d=x(n)i,d+v(n+1)i,d | (2.2) |
where c1 and c2 denote the acceleration coefficients, usually set to 2, r1 and r2 are two uniformly distributed values in the range [0, 1]. The parameters Vmin and Vmax can be used to determine an appropriate range of velocity values for each particle to prevent it from wandering too far outside the search space.
The global minimum optimization problem (2.3) for a D-dimensional continuous object can be described as:
min f(X), X=[x1,⋅⋅⋅,xD], s.t. xd∈[ad,bd], d=1,⋅⋅⋅,D | (2.3) |
The standard PSO can be described in the following steps:
Step 1: Initialize N particles. For each particle i in the population:
Step 1.1: Initialize Xi randomly in the range [a,b].
Step 1.2: Initialize Vi randomly in the range [Vmin,Vmax].
Step 1.3: Evaluate the fitness value fi.
Step 1.4: Set pbesti as the particle's current position and gbest as the particle with the best fitness value in the population.
Step 2: Loop until the stop condition is satisfied:
Step 2.1: Update the velocity Vi and position Xi for each particle i according to Eqs (2.1) and (2.2).
Step 2.2: Evaluate the fitness value fi of each particle i.
Step 2.3: Update pbesti if fi<fpbesti,∀i⩽N for each particle i.
Step 2.4: Update the global optimal position gbest fgbest⩽fi, ∀i⩽N.
Chaos is one of the most exciting properties exhibited by the brain [31]. It is the phenomenon of complex, unpredictable and random-like behavior arising from simple deterministic nonlinear systems rather than a state of disorder [32]. Researchers have recently developed many optimization algorithms based on chaos theory to address complex optimization problems [33,34,35].
COA was first proposed by Li and Jiang [32,35] in 1997. Its core idea is to introduce the chaotic state into optimization variables via the carrier wave method, map the traversal range of chaos motion to the value range of optimization variables and then search for the global optimal solution using chaotic dynamics instead of random search. The chaotic dynamics equation chosen in COA is the Logistic map [36], which is defined as follows:
x(n+1)=μx(n)(1−x(n)), 0 ⩽x(0)⩽1 | (2.4) |
where μ is the control parameter, n=0,1,2,.... Although the above equation is definite, it exhibits chaotic dynamics when μ=4 and x(0)∉{0,0.25,0.5,0.75,1}, the property that a minute difference in the initial value of the chaotic variable would lead to significant differences in output. This is the sensitive dependence on initial conditions, the basic characteristic of chaos. This property enables the trajectory of chaotic variables to traverse the entire search space. The process of COA can be defined through the following equation.
cx(k+1)d=4cx(k)d(1−cx(k)d), d=1,2,⋅⋅⋅,D, | (2.5) |
where cxd is the d th chaotic variable and k represents the number of iterations. Obviously, cx(k)d is distributed in the range (0, 1) when the initial cx(0)d∈(0,1) and cx(0)d∉{0.25,0.5,0.75}.
For the optimization problem (2.3), the procedures of COA are as follows.
Step 1: Set k=0, and initialize D chaotic variables cx(0)d in the range (0, 1) with very small differences. The current optimal function value f∗ is initialized to a large value, with X∗=[x∗1,x∗2,⋅⋅⋅x∗D] representing the corresponding optimal variables.
Step 2: Map the D chaotic variables cxd to the D optimization variables xd of the optimization problem (2.3) through the carrier wave method of Eq (2.6).
x(k)d=cx(k)d(bd−ad)+ad | (2.6) |
Step 3: Iterative search using chaotic variables.
If f(X(k))⩽f∗, then f∗=f(X(k)), X∗=X(k). Else if f(X(k))>f∗, then give up X(k).
Step 4: Let k=k+1 and continue the chaotic traversal through Eq (2.5).
Step 5: Repeat steps 2 to 4. If the best function value f∗ remains unchanged after several iterations, the second carrier wave is applied according to Eq (2.7).
x(k+1)d=x∗d+α⋅cx(k+1)d | (2.7) |
where x∗d is the current optimal solution, α is an adjusting constant which can be less than 1 and α⋅cx(k+1)d generates i chaotic states with small ergodic ranges around x∗d.
Step 6: Continue the iterative search using the chaotic variables after the second carrier wave until the stop criterion is satisfied.
As a simple and efficient optimization algorithm, COA has garnered the attention of many researchers for further improvement. Its primary disadvantage is that the sensitive dependence of chaotic motion on the initial values will cause the algorithm's instability and a large number of traversal searches are required before the search space is reduced. Moreover, the lack of an explicit search stop condition results in the need for parameter tuning according to specific problems, limiting the algorithm's generality. Therefore, many variants of COA aimed at these problems have emerged.
Xiu et al. [37] proposed a double-chaos optimization algorithm (DCOA) that uses two different chaotic systems to explore the search space independently and in parallel and narrows down the search space when the distance between the optimal positions of the two systems satisfies specific criteria. Therefore, DCOA overcomes some of the deficiencies of COA. Additionally, DCOA provides a condition for narrowing the search space, which improves the algorithm's generality, avoids multiple blind searches and reduces its running cost. Liang and Gu [38] developed a chaos optimization algorithm based on parallel computing (PCOA), which performs parallel searches using several different sets of chaotic variables, effectively reducing the sensitive dependence of chaotic motion on initial values.
Based on in-depth research of the chaos algorithms and the PSO algorithm, we combined the advantages of the two types of algorithms and proposed DCS-PSO. The details are as follows:
Although a single chaos search mechanism is easy to implement and can avoid being trapped in local optima, it often requires a large number of iterations to narrow down the search space to ensure that the optimal solution is within the narrowed area.
Additionally, the stop condition of the search needs to be adjusted based on the specific problem, limiting the algorithm's generality. In contrast, the DCOA determines the requirement for narrowing the space based on the distance between the optimal positions found by each chaotic system, which improves the algorithm's generality. However, there is a risk that the algorithm may continuously narrow down the search space, making it difficult to converge accurately to a point and potentially excluding the global optimal solution from the search space. Nonetheless, experimental verification (see Section 4.1) suggests that the double-chaos search mechanism can narrow the search space to the neighborhood of the global optimum after only one sufficient search.
In the double-chaos search mechanism, we select two chaotic systems: The logistic map and the tent map. The orbit points of the logistic map are distributed near the edges, which to some extent can explain why chaotic motion has the advantage of escaping from local optima [39]. However, several breakpoints {0,0.25,0.5,0.75,1} in the logistic map make it difficult to traverse specific chaos states during the search process. The orbit points of the tent map are distributed more evenly. Its equation is defined in Eq (3.1). Figure 1(a) and (b) show the scatter plots of the two chaos maps for 2000 iterations, respectively and (c) and (d) show their chaotic dynamics.
x(n+1)={x(n)/β, 0<x(n)⩽β(1−x(n))/(1−β), β<x(n)⩽1 | (3.1) |
where β=0.4.
The double-chaos search mechanism is illustrated in Figure 2. In the search space A, two chaotic systems cx and cy are used to perform independent parallel searches. When the distance between the optimal solutions cx∗ and cy∗ found by cx and cy is small enough, namely,
‖ | (3.2) |
where {X^ * } and {Y^ * } represent the corresponding optimization variables, \gamma \in (0, 0.25] and is set to 0.15 in this study, the search space is narrowed down from A to B according to Eqs (3.3) and (3.4).
{a_d} = \max \left( {{a_d}, \left( {\min \left( {x_d^ * , y_d^ * } \right) - \xi \cdot \gamma \cdot {{\left\| {{X^ * } - {Y^ * }} \right\|}_2}} \right)} \right) | (3.3) |
{b_d} = \min \left( {{b_d}, \left( {\max \left( {x_d^ * , y_d^ * } \right) + \xi \cdot \gamma \cdot {{\left\| {{X^ * } - {Y^ * }} \right\|}_2}} \right)} \right) | (3.4) |
where \xi \in \left[{1, 2} \right] and it is set to 1.5 in this study.
Based on the chaos search mechanism, we propose a two-stage iteration strategy named DCS-PSO. The algorithm employs the double-chaos search mechanism for global exploration and narrowing the search space and then uses the improved PSO for further fine search in the narrowed search space.
For the D-dimensional optimization problem (2.3), the detailed steps of the first stage of DCS-PSO are listed in Algorithm 1:
Algorithm 1 Space reduction based on double-chaos search mechanism. |
1. Initialize two chaotic systems \boldsymbol c{\boldsymbol x^{\left(0 \right)}} and \boldsymbol c{\boldsymbol y^{\left(0 \right)}} in the range (0, 1); |
2. Initialize the optimal function values of the two chaotic systems f_x^ * and f_{\text{y}}^ * with large values; |
3. Initialize the corresponding optimization variables {X^ * } and {Y^ * } ; |
4. set k = 0 ; |
5. while True do: |
6. Map \boldsymbol c{\boldsymbol x^{\left(k \right)}} and \boldsymbol c{\boldsymbol y^{\left(k \right)}} to optimization variables {X^{\left(k \right)}} and {Y^{\left(k \right)}} ; |
7. Update f_x^* = f\left({{X^{\left(k \right)}}} \right) and {X^ * } = {X^{\left(k \right)}} , if f\left({{X^{\left(k \right)}}} \right) < f_x^ * ; |
8. Update f_y^* = f\left({{Y^{\left(k \right)}}} \right) and {Y^ * } = {Y^{\left(k \right)}} , if f\left({{Y^{\left(k \right)}}} \right) < f_y^ * ; |
9. if k > h then |
10. if {\left\| {{X^ * } - {Y^ * }} \right\|_2} < \gamma {\left\| {\boldsymbol a -\boldsymbol b} \right\|_2} then |
11. Narrow down the search space \left[{\boldsymbol a,\boldsymbol b} \right] according to Eqs (3.3) and (3.4); |
12. break |
13. end if |
14. end if |
15. Update \boldsymbol c{\boldsymbol x^{\left(k \right)}} and \boldsymbol c{\boldsymbol y^{\left(k \right)}} according to Eqs (2.5) and (3.1), respectively; |
16. k + + ; |
17. end while |
18. Output the narrowed search space \boldsymbol a = \left[{{a_1}, {a_2}, \cdot \cdot \cdot {a_d}} \right],\boldsymbol b = \left[{{b_1}, {b_2}, \cdot \cdot \cdot, {b_d}} \right] |
19. Output \boldsymbol{cbest }, one of {X^ * } and {Y^ * } that has the smallest fitness value |
The flowchart of the first stage of DCS-PSO is shown in Figure 3(a).
In conventional PSO, all particles learn from the global optimal particle in the population to update their positions and velocities. This mechanism is advantageous in achieving fast convergence, but it also leads to the need for more diversity and often causes premature convergence due to falling into local optimum [40]. The conventional PSO may require longer to find the optimal solution when dealing with multimodal optimization problems with a large search space.
Although the risk of being trapped in local optima can be reduced by narrowing the search space, PSO may still fall into a local optimum in the reduced search space. To enhance the population's diversity, in the narrowed search space, we perform a chaos search on the best solution found by the two chaotic systems using the logistic map. The best solution found by the logistic map and the population guides the algorithm to converge toward the global optimum. The velocity of particle i on dimension d is updated according to the following rules.
\boldsymbol{best} = \left\{ \begin{array}{l} \boldsymbol{cbest}, ~~if{\text{ }}~~f\left( \boldsymbol{cbest} \right) \leqslant f\left( \boldsymbol{gbest} \right) \hfill \\ \boldsymbol{gbest}, {\text{ }}else \hfill \\ \end{array} \right. | (3.5) |
\boldsymbol v_{i, d}^{\left( {n + 1} \right)} = w \times v_{i, d}^{\left( n \right)} + {c_1} \times {r_1} \times \left( {pbes{t_{i, d}} - {x_{i, d}}} \right) + {c_2} \times {r_2} \times \left( {bes{t_d} - {x_{i, d}}} \right) | (3.6) |
where best denotes the best solution between chaos search and population search, f\left({cbest} \right) is the optimal fitness value found by chaos search and cbest is the corresponding solution.
The detailed steps of the second stage of DCS-PSO are listed in Algorithm 2:
Algorithm 2 Improved PSO combined with chaos |
1. Input the narrowed search space \left[{\boldsymbol a,\boldsymbol b} \right] and \boldsymbol{cbest} |
2. Transform cbest to chaotic variables cx ; |
3. Initialize N particles' position and velocity within the new search space; |
4. Evaluate the fitness value of every particle; |
5. Update \boldsymbol{pbes{t_i}} and \boldsymbol{ gbest} ; |
6. for i = 1:{\text{ Max_Iter}} do |
7. Implement chaos search globally and update cbest via logistic map; |
8. if f\left(\boldsymbol{cbest} \right) \leqslant f\left(\boldsymbol{gbest} \right) then |
9. \boldsymbol{best} = \boldsymbol{cbest} ; |
10. else \boldsymbol{best} = \boldsymbol{gbest} ; |
11. end if |
12. Update particles' velocity and position according to Eqs (3.6) and (2.2), respectively; |
13. Evaluate the fitness value of every particle; |
14. Update \boldsymbol{pbes{t_i}} and \boldsymbol{gbest }; |
15. end for |
The flowchart of the second stage of DCS-PSO is shown in Figure 3(b).
It is worth noting that many researchers have attempted to introduce the chaos search mechanism into intelligent optimization algorithms [39,41]. The most common strategy is to use chaotic local search (CLS) after each iteration to conduct a fine search near the current optimum. Although this approach improves the convergence accuracy of the algorithm, it needs to address the problem of the population falling into local optima. Therefore, in addition to CLS, other strategies are often required to maintain the diversity of the population. However, some of these strategies may sacrifice the performance of convergence speed.
In this chapter, simulation experiments are conducted to validate the performance of DCS-PSO. We first conduct experiments using six 2-dimensional benchmark functions to validate the effectiveness of the DCS-PSO search space narrowing mechanism. After that, the performance of the DCS-PSO algorithm is compared with five typical PSO variants placed on ten benchmark functions in terms of solution accuracy, statistical significance test and convergence speed. Finally, to verify the performance of DCS-PSO in complex and multimodal conditions, an authoritative test suite CEC2017 is selected in this paper.
All simulation experiments were conducted on the same PC. The configuration information of the PC is shown in Table 1.
PC configuration | Information |
CPU | Inter(R) Core(TM) i7-8700 |
Frequency | 3.2GHz CPU |
RAM | 16.0GB |
Operating system | Windows 10(64Bit) |
Language | Python 3.9.13 |
To validate the effectiveness of the search space narrowing mechanism in DCS-PSO, we conducted experiments using six 2-dimensional benchmark functions [38,42]. DCS-PSO was compared with other chaos-based algorithms, namely COA, DCOA and PCOA. The narrowed search space can be visually observed through the contour map of the 2-dimensional function. The six benchmark functions are listed below.
(1) Jong function:
{f_J} = 100 \cdot {\left( {x_1^2 - {x_2}} \right)^2} + {\left( {1 - {x_1}} \right)^2}, {\text{ }} - 2.048 \leqslant {x_1}, {x_2} \leqslant 2.048. | (4.1) |
The function has a global minimum of 0 at (1, 1).
(2) Camel function:
{f_C} = \left( {4 - 2.1 \cdot x_1^2 + \frac{{x_1^4}}{3}} \right) \cdot x_1^2 + {x_1} \cdot {x_2} + \left( { - 4 + 4 \cdot x_2^2} \right) \cdot x_2^2, {\text{ }} - 2 \leqslant {x_1}, {x_2} \leqslant 2. | (4.2) |
The function has a global minimum of −1.031628 at two points (−0.0898, 0.7126) and (0.0898, −0.7126).
(3) GP-Goldstein-Price:
\begin{array}{l} {f_G}\left( x \right) = \left[ {1 + {{\left( {{x_1} + {x_2} + 1} \right)}^2}\left( {19 - 14{x_1} + 3x_1^2 - 14{x_2} + 6{x_1}{x_2} + 3x_2^2} \right)} \right] \hfill \\ {\text{ }} \times \left[ {30 + {{\left( {2{x_1} - 3{x_2}} \right)}^2}\left( {18 - 32{x_1} + 12x_1^2 + 48{x_2} - 36{x_1}{x_2} + 27x_2^2} \right)} \right], {\text{ }} - 2 \leqslant {x_1}, {x_2} \leqslant 2. \hfill \\ \end{array} | (4.3) |
The function has a global minimum of 3 at (0, −1) and there are four local minima in the minimization region.
(4) BR-Branin:
{f_B}\left( x \right) = {\left( {{x_2} - \frac{{5.1}}{{4{\pi ^2}}}x_1^2 + \frac{5}{\pi }{x_1} - 6} \right)^2} + 10\left( {1 - \frac{1}{{8\pi }}} \right)\cos {x_1} + 10, {\text{ }} - 5 \leqslant {x_1} \leqslant 10, {\text{ }}0 \leqslant {x_2} \leqslant 15. | (4.4) |
The global minimum of this function is approximately equal to 0.398 at three points (−3.142, 12.275), (3.142, 2.275) and (9.425, 2.425).
(5) RA-Rastrigin:
{f_R}\left( x \right) = x_1^2 + x_2^2 - \cos 18{x_1} - \cos 18{x_2}, {\text{ }} - 1 \leqslant {x_1}, {x_2} \leqslant 1 | (4.5) |
The function has a global minimum of −2 at (0, 0) and its lattice structure has about 50 local minima.
(6) SH-Shuber:
{f_s}\left( x \right) = \left\{ {\sum\limits_{i = 1}^5 {i\cos \left( {\left( {i + 1} \right){x_1} + i} \right)} } \right\}\left\{ {\sum\limits_{i = 1}^5 {i\cos \left( {\left( {i + 1} \right){x_2} + i} \right)} } \right\}, {\text{ }} - 10 \leqslant {x_1}, {x_2} \leqslant 10. | (4.6) |
The function has 760 local minima, 18 of which are global with a value of −186.7309.
The RA-Rastrigin function and the SH-Shuber function are relatively complex and have a large number of local minima, which can effectively test the algorithm's ability to escape from the local optima. Their 3D stereograms are shown in Figure 4.
To ensure the fairness of the experimental results, each algorithm will run on each benchmark function independently 50 times and the mean execution time, the mean optimal values and the standard deviations will be provided. The specific parameter settings of all algorithms are listed in Table 2.
Algorithm | Parameters |
COA | \max \_iter1 = {10^5}, {\text{ }}\max \_iter2 = 1.5 \times {10^5}, {\text{ }}\alpha = 0.3 |
DCOA | h = 3000, {\text{ }}\gamma = 0.25, {\text{ }}\xi {\text{ = 1}}{\text{.5}} |
PCOA | n\_group = 3, {\text{ }}\max \_iter1 = {10^5}, {\text{ }}\max \_iter2 = 1.5 \times {10^5}, {\text{ }}\alpha = 0.3 |
DCS-PSO | N = 20, {\text{ }}w = 0.4, {\text{ }}c1 = c2 = 2, {\text{ }}{V_{\max }} = 0.2 \times Range, {\text{ }}\max \_iter = 1000 |
Table 3 shows the execution time (E.T.), the mean optimal values (Mean) and standard deviations (S.D.) of COA, DCOA, PCOA and DCS-PSO. The best results of each algorithm on each test function are indicated in bold and the second-best results are marked with an underline.
Function | Criteria | COA | DCOA | PCOA | DCS-PSO |
{f_J} | E.T. | 30.8898 | 0.5753 | 17.2234 | 0.2793 |
Mean | 0.0000 | 0.0005 | 0.0000 | 0.0000 | |
S.D. | 0.0000 | 0.0014 | 0.0000 | 0.0000 | |
{f_C} | E.T. | 7.6564 | 0.6478 | 10.3990 | 0.3610 |
Mean | −1.0316 | −1.0312 | −1.0316 | −1.0316 | |
S.D. | 0.0000 | 0.0012 | 0.0000 | 0.0000 | |
{f_G} | E.T. | 14.1867 | 1.0656 | 14.0814 | 0.6097 |
Mean | 3.0022 | 3.0100 | 3.0024 | 3.0000 | |
S.D. | 0.0022 | 0.0126 | 0.0022 | 0.0000 | |
{f_B} | E.T. | 1.5663 | 0.8653 | 5.7455 | 0.4513 |
Mean | 0.3983 | 0.4003 | 0.3980 | 0.3979 | |
S.D. | 0.0003 | 0.0039 | 0.0001 | 0.0000 | |
{f_R} | E.T. | 4.4150 | 0.6652 | 5.8342 | 0.4366 |
Mean | −1.9993 | −1.9652 | −1.9992 | −2.0000 | |
S.D. | 0.0006 | 0.0558 | 0.0008 | 0.0000 | |
{f_S} | E.T. | 5.8609 | 2.1743 | 13.8397 | 2.0615 |
Mean | −186.6428 | -186.4517 | −186.6861 | −186.7309 | |
S.D. | 0.0711 | 0.4203 | 0.0680 | 0.0000 |
Based on the mean values in Table 3, the proposed DCS-PSO outperforms the other three chaos-based methods. Compared with COA, DCOA and PCOA, DCS-PSO achieves superior results on five functions ( {f_J} , {f_C} , {f_G} , {f_R} and {f_S} ). COA performs well in solving simple optimization problems and acquires the theoretically optimal solutions on both functions {f_J} and {f_C} . However, it performs poorly on function {f_S} , which has multiple local minima, possibly due to the challenge of attaining certain chaos states in a large search space. The convergence accuracy of DCOA is relatively poor. As mentioned earlier, DCOA may exclude the optimal solution while continuously narrowing the search space. However, compared with COA and PCOA, DCOA shows a shorter convergence time, which reflects its efficiency in narrowing the search space. The overall performance of PCOA is comparable to COA and it is the only algorithm that converges to the global optimum on function {f_B} . DCS-PSO has a faster convergence speed due to the combination of double-chaos search and PSO. The S.D. values in Table 3 show that DCS-PSO has the smallest S.D. values among the six benchmark functions, indicating that DCS-PSO is more stable and reliable.
Figure 5(a) and (b) show the contour plots of functions {f_R} and {f_S} projected onto the XOY plane, respectively. The red rectangle in each plot represents the narrowed search space, demonstrating the ability of DCS-PSO to reduce the search space of complex optimization problems. These plots visually display that DCS-PSO can accurately converge to the vicinity of the global optimum, validating the effectiveness of the proposed method.
In this subsection, five representative PSO variants are selected for comparison. The first one is the PSO with inertia weight (PSO-w) [22], which can better control the search process and is characteristic of fast convergence when dealing with unimodal problems. The second one is the global version of PSO (GPSO) [23], whose inertia weight is decreased linearly from 0.9 to 0.4, improving the population's global search ability. The third one is the local version of PSO (LPSO) [26] with ring topology, in which different topologies based on neighborhood connections are used to maintain the diversity of the population. The fourth one is the Comprehensive Learning PSO (CLPSO) [28], in which each particle updates its velocity by learning the historical best information of different particles. The fifth is the dynamic multi-swarm PSO (DMS-PSO) [27], in which the population comprises many small subgroups and a dynamic strategy is used to maintain population diversity. CLPSO and DMS-PSO are designed to improve the performance of PSO on multimodal problems.
To investigate the performance of DCS-PSO on different optimization problems, ten benchmark functions are tested. Based on the properties of these functions, they are divided into two groups. The first group consists of 5 unimodal benchmark functions with only one global optimum value, mainly used to test the convergence accuracy and speed. The second group consists of 5 multimodal benchmark functions used to test the algorithm's global search ability and its ability to escape from local optima. These benchmark functions are widely used in the literature [25,43,44]. The details of these benchmarks are shown in Table 4.
Name | Functions | Range | Optimum |
Sphere | {f_1}\left(\boldsymbol x \right) = \sum\limits_{i = 1}^D {x_i^2} | [−100, 100] | 0 |
Schwefel's 2.22 | {f_2}\left(\boldsymbol x \right) = \sum\limits_{i = 1}^D {|{x_i}|} + \prod\limits_{i = 1}^D {|{x_i}|} | [−10, 10] | 0 |
Schwefel's 1.2 | {f_3}\left(\boldsymbol x \right) = \sum\limits_{i = 1}^D {{{\left({\sum\limits_{j = 1}^i {{x_j}} } \right)}^2}} | [−100, 100] | 0 |
Rosenbrock | {f_4}\left(\boldsymbol x \right) = \sum\limits_{i = 1}^{D - 1} {\left[{100{{\left({{x_{i + 1}} - x_i^2} \right)}^2} + {{\left({{x_i} - 1} \right)}^2}} \right]} | [−10, 10] | 0 |
Noise | {f_5}\left(\boldsymbol x \right) = \sum\limits_{i = 1}^D {ix_i^4} + random[0, 1) | [−1.28, 1.28] | 0 |
Rastrigin | {f_6}\left(\boldsymbol x \right) = \sum\limits_{i = 1}^D {\left({x_i^2 - 10\cos \left({2\pi {x_i}} \right) + 10} \right)} | [−5.12, 5.12] | 0 |
Ackley | {f_7}\left(\boldsymbol x \right) = - 20\exp \left({ - 0.2\sqrt {\frac{1}{D}\sum\limits_{i = 1}^D {x_i^2} } } \right) - \exp \left({\frac{1}{D}\sum\limits_{i = 1}^D {\cos \left({2\pi {x_i}} \right)} } \right) + 20 + e | [−32, 32] | 0 |
Griewank | {f_8}\left(\boldsymbol x \right) = \frac{1}{{4000}}\sum\limits_{i = 1}^D {x_i^2} - \prod\limits_{i = 1}^D {\cos \left({\frac{{{x_i}}}{{\sqrt i }}} \right)} + 1 | [−600, 600] | 0 |
Penalized 1 | \begin{array}{l} {f_9}\left(\boldsymbol x \right) = \frac{\pi }{D}\left\{ {10{{\sin }^2}\left({\pi {y_1}} \right) + \sum\limits_{i = 1}^{D - 1} {{{\left({{y_i} - 1} \right)}^2}} \left[{1 + 10{{\sin }^2}\left({\pi {y_{i + 1}}} \right)} \right] + {{\left({{y_D} - 1} \right)}^2}} \right\} \\ + \sum\limits_{i = 1}^D {u\left({{x_i}, 10, 100, 4} \right)} \\ \end{array} | [−50, 50] | 0 |
Penalized 2 | \begin{array}{l} {f_{10}}\left(\boldsymbol x \right) = \frac{1}{{10}}\left\{ {{{\sin }^2}\left({3\pi {x_1}} \right) + \sum\limits_{i = 1}^D {{{\left({{x_i} - 1} \right)}^2}} \left[{1 + {{\sin }^2}\left({3\pi {x_i} + 1} \right)} \right] + {{\left({{x_D} - 1} \right)}^2}\left[{1 + {{\sin }^2}\left({2\pi {x_D}} \right)} \right]} \right\} \\ + \sum\limits_{i = 1}^D {u\left({{x_i}, 5, 100, 4} \right)} \\ \end{array} | [−50, 50] | 0 |
In the first group, the global optimal position of function {f_4} is {\left[1 \right]^D} .The global optimal position of the remaining four functions is {\left[0 \right]^D} and the theoretical optimal values of the five functions are 0. {f_1} is the sphere function and is easy to solve. {f_2} and {f_3} are the Schwefel functions. {f_4} is the Rosenbrock function, it becomes a simple multimodal problem when its dimension is more than three and has a narrow gap between the perceived local optima and the global optimum. {f_5} is the noise function, which is challenging to find the global optimum because there is a perturbation factor.
In the second group, the global optimal positions of all functions are {\left[0 \right]^D} and the global optimal values are 0. Rastrigin's function ( {f_6} ) has a large number of local optima. {f_7} has a narrow global basin and a large number of local optima. {f_8} is the Griewank's function. {f_9} and {f_{10}} are the penalized functions.
In {f_9} , the {y_i} is updated by {y_i} = 1 + \frac{1}{4}\left({{x_i} + 1} \right) .
In {f_9} and {f_{10}} , u\left({{x_i}, a, k, m} \right) = \left\{ \begin{array}{l} k{\left({{x_i} - a} \right)^m}, {\text{ }}{x_i} > a \hfill \\ {\text{ }}0, {\text{ }} - a \leqslant {x_i} \leqslant a \hfill \\ k{\left({ - {x_i} - a} \right)^m}, {\text{ }}{x_i} < - a \hfill \\ \end{array} \right. .
In the experiments, a population size of 40 and a maximum number of iterations of 2000 were used for all algorithms. The parameters for the five PSO variants were kept consistent with their respective literature. The benchmark functions were tested with dimensionalities of 10, 30 and 50 and each benchmark function was independently run 50 times in different dimensions to ensure the fairness of the experimental results. The error between the best optimum found by the algorithm and the actual global optimum was recorded and the mean error and standard deviation were displayed. The specific parameter settings for all algorithms can be found in Table 5.
Algorithm | Parameters |
PSO-w | w:0.4, {\text{ }}{c_1} = {c_2} = 2.0, {\text{ }}{V_{\max }} = 0.2 \times Range |
GPSO | w:0.9 \sim 0.4, {\text{ }}{c_1} = {c_2} = 2.0, {\text{ }}{V_{\max }} = 0.2 \times Range |
LPSO | \chi = 0.7298, {\text{ }}{c_1} = {c_2} = 1.49445, {\text{ }}{V_{\max }} = 0.2 \times Range |
CLPSO | w:0.9\sim 0.4, {\text{ }}c = 1.49445, {\text{ }}{V_{\max }} = 0.2 \times Range, {\text{ }}m = 7 |
DMS-PSO | \chi = 0.7298, {\text{ }}{c_1} = {c_2} = 1.49445, {\text{ }}{V_{\max }} = 0.2 \times Range, {\text{ }}M = 4, {\text{ }}R = 10 |
DCS-PSO | w:0.4, {c_1} = {c_2} = 2.0, {V_{\max }} = 0.2 \times Range, {\text{ }}h = 3000, \gamma = 0.15, \xi = 1.5 |
Furthermore, the nonparametric Wilcoxon rank-sum tests were executed for each benchmark function to determine whether the results obtained by DCS-PSO are significantly different from the best results achieved by the other algorithms
Tables 6, 7 and 8 display the means (Mean) and standard deviations (S.D.) of six algorithms on 10 test functions with dimensions of 10, 30 and 50, respectively. The k value presented in the second column of each table is the outcome of the nonparametric Wilcoxon rank-sum tests on our proposed algorithm and the second-best algorithms (indicated with underlining in tables). A k value of 1 indicates a significant difference in the performances of the two algorithms with 95% certainty, while a k value of 0 suggests no significant difference. Moreover, Table 7 ranks the algorithms according to their mean solution accuracy. The best results for each benchmark function are denoted in bold and the second-best results are underlined.
Function | k | Criteria | PSO-w | GPSO | LPSO | CLPSO | DMS-PSO | DCS-PSO |
{f_1} | 1 | Mean | 1.84 × 10−144 | 1.47 × 10−73 | 2.78 × 10−169 | 2.18 × 10−21 | 6.30 × 10−62 | 2.35 × 10−151 |
S.D. | 9.13 × 10−144 | 1.04 × 10−72 | 0.00 | 5.44 × 10−21 | 4.25 × 10−61 | 7.75 × 10−151 | ||
{f_2} | 1 | Mean | 1.89 × 10−81 | 6.24 × 10−38 | 7.59 × 10−09 | 4.30 × 10−14 | 3.45 × 10−20 | 9.65 × 10−84 |
S.D. | 1.26 × 10−80 | 2.99 × 10−37 | 3.80 × 10−08 | 1.39 × 10−13 | 5.86 × 10−20 | 4.81 × 10−83 | ||
{f_3} | 1 | Mean | 1.22 × 10−44 | 9.93 × 10−17 | 6.11 × 10−13 | 2.18 × 10−20 | 6.13 × 10−10 | 1.21 × 10−48 |
S.D. | 7.42 × 10−44 | 3.36 × 10−16 | 4.32 × 10−12 | 3.25 × 10−20 | 3.33 × 10−09 | 2.38 × 10−48 | ||
{f_4} | 1 | Mean | 2.79 | 2.80 | 4.00 | 2.49 | 1.60 | 9.11 |
S.D. | 1.39 | 1.16 | 2.56 | 4.95 × 10−01 | 9.79 × 10−01 | 1.89 | ||
{f_5} | 1 | Mean | 7.23 × 10−04 | 1.02 × 10−03 | 3.25 × 10−03 | 1.49 × 10−03 | 8.80 × 10−04 | 2.15 × 10−04 |
S.D. | 3.90 × 10−04 | 5.46 × 10−04 | 2.43 × 10−03 | 7.09 × 10−04 | 4.49 × 10−04 | 1.58 × 10−04 | ||
{f_6} | 0 | Mean | 5.99 | 2.13 | 9.35 | 0.00 | 2.67 | 0.00 |
S.D. | 2.74 | 1.15 | 3.53 | 0.00 | 1.36 | 0.00 | ||
{f_7} | 0 | Mean | 4.14 × 10−15 | 4.07 × 10−15 | 4.67 × 10−15 | 3.16 × 10−14 | 4.00 × 10−15 | 3.91 × 10−15 |
S.D. | 7.03 × 10−16 | 5.02 × 10−16 | 1.62 × 10−15 | 5.29 × 10−14 | 0.00 | 5.62 × 10−16 | ||
{f_8} | 1 | Mean | 7.44 × 10−02 | 6.88 × 10−02 | 1.06 × 10−01 | 1.64 × 10−03 | 7.12 × 10−02 | 6.65 × 10−02 |
S.D. | 3.41 × 10−02 | 3.13 × 10−02 | 5.81 × 10−02 | 7.61 × 10−04 | 3.55 × 10−02 | 3.75 × 10−02 | ||
{f_9} | 1 | Mean | 1.24 × 10−02 | 4.71 × 10−32 | 6.22 × 10−03 | 2.99 × 10−30 | 1.38 × 10−28 | 1.78 × 10−01 |
S.D. | 6.16 × 10−02 | 1.11 × 10−47 | 4.40 × 10−02 | 3.07 × 10−30 | 2.65 × 10−28 | 6.11 × 10−02 | ||
{f_{10}} | 1 | Mean | 3.56 × 10−04 | 9.33 × 10−20 | 9.80 × 10−04 | 3.16 × 10−27 | 2.57 × 10−13 | 1.35 × 10−32 |
S.D. | 2.52 × 10−03 | 1.90 × 10−19 | 6.86 × 10−03 | 6.79 × 10−27 | 4.33 × 10−13 | 8.29 × 10−48 |
Function | k | Criteria | PSO-w | GPSO | LPSO | CLPSO | DMS-PSO | DCS-PSO |
{f_1} | 1 | Mean | 1.28 × 10−39 | 3.42 × 10−14 | 3.82 | 3.83 × 10−13 | 6.76 × 10−28 | 1.05 × 10−40 |
S.D. | 2.48 × 10−39 | 1.20 × 10−13 | 2.18× 10+01 | 9.48 × 10−13 | 5.49 × 10−28 | 2.01 × 10−40 | ||
Rank | 2 | 4 | 6 | 5 | 3 | 1 | ||
{f_2} | 1 | Mean | 1.67 × 10−09 | 1.27 × 10−08 | 1.83 | 4.45 × 10−08 | 3.74 × 10−13 | 2.49 × 10−23 |
S.D. | 1.15 × 10−08 | 2.46 × 10−08 | 1.83 | 2.84 × 10−08 | 2.33 × 10−13 | 5.70 × 10−23 | ||
Rank | 3 | 4 | 6 | 5 | 2 | 1 | ||
{f_3} | 1 | Mean | 6.50 × 10−01 | 9.58 × 10+01 | 4.79× 10+02 | 4.37 × 10−04 | 1.16 × 10+01 | 5.90 |
S.D. | 5.22 × 10−01 | 5.10 × 10+01 | 3.08× 10+02 | 3.04 × 10−04 | 5.95 | 4.45 | ||
Rank | 2 | 5 | 6 | 1 | 4 | 3 | ||
{f_4} | 1 | Mean | 2.98× 10+01 | 4.17 × 10+01 | 9.42× 10+01 | 1.81 × 10 +01 | 2.26 × 10+01 | 2.21 × 10+01 |
S.D. | 2.16× 10+01 | 2.87 × 10+01 | 5.46× 10+01 | 3.80 | 2.29 | 6.32 × 10−01 | ||
Rank | 4 | 5 | 6 | 1 | 3 | 2 | ||
{f_5} | 1 | Mean | 8.74 × 10−03 | 1.89 × 10−02 | 8.00 × 10−02 | 3.83 × 10−02 | 9.99 × 10−03 | 5.12 × 10−03 |
S.D. | 3.28 × 10−03 | 6.58 × 10−03 | 3.51 × 10−02 | 1.47 × 10−02 | 3.98 × 10−03 | 1.69 × 10−03 | ||
Rank | 2 | 4 | 6 | 5 | 3 | 1 | ||
{f_6} | 1 | Mean | 3.88 × 10+01 | 3.34 × 10+01 | 5.12 × 10+01 | 4.40 × 10−11 | 2.73 × 10+01 | 1.31 × 10+02 |
S.D. | 8.06 | 8.19 | 1.62 × 10+01 | 6.58 × 10−11 | 8.88 × 10+00 | 1.01 × 10+01 | ||
Rank | 4 | 3 | 5 | 1 | 2 | 6 | ||
{f_7} | 1 | Mean | 3.81 × 10−01 | 1.14 × 10−06 | 4.32 | 3.15 × 10−03 | 4.37 × 10−14 | 1.20 × 10−14 |
S.D. | 6.47 × 10−01 | 1.04 × 10−06 | 1.16 | 2.18 × 10−02 | 4.86 × 10−14 | 3.90 × 10−15 | ||
Rank | 5 | 3 | 6 | 4 | 2 | 1 | ||
{f_8} | 0 | Mean | 1.46 × 10−02 | 1.38 × 10−02 | 4.14 × 10−01 | 1.41 × 10−12 | 4.44 × 10−03 | 1.15 × 10−02 |
S.D. | 1.70 × 10−02 | 1.55 × 10−02 | 3.12 × 10−01 | 2.52 × 10−12 | 4.99 × 10−03 | 1.40 × 10−02 | ||
Rank | 5 | 4 | 6 | 1 | 2 | 3 | ||
{f_9} | 0 | Mean | 1.39 × 10−01 | 1.04 × 10−02 | 3.87 | 2.32 × 10−14 | 1.08 × 10−26 | 2.96 × 10−02 |
S.D. | 2.62 × 10−01 | 3.14 × 10−02 | 2.66 | 5.72 × 10−14 | 2.24 × 10−26 | 4.74 × 10−02 | ||
Rank | 4 | 3 | 6 | 2 | 1 | 4 | ||
{f_{10}} | 1 | Mean | 2.28 × 10−01 | 1.10 × 10−02 | 2.16× 10+01 | 1.03 × 10−11 | 5.31 × 10−03 | 6.22 × 10−32 |
S.D. | 6.63 × 10−01 | 4.43 × 10−02 | 1.12× 10+01 | 1.45 × 10−11 | 4.13 × 10−04 | 7.45 × 10−32 | ||
Rank | 5 | 4 | 6 | 2 | 3 | 1 | ||
Ave. rank | 3.6 | 3.9 | 5.9 | 2.7 | 2.5 | 2.3 | ||
Final rank | 4 | 5 | 6 | 3 | 2 | 1 |
Function | k | Criteria | PSO-w | GPSO | LPSO | CLPSO | DMS-PSO | DCS-PSO |
{f_1} | 1 | Mean | 5.20 × 10−17 | 3.68 × 10−04 | 1.30 × 10+03 | 5.70 × 10−05 | 1.26 × 10−06 | 3.93 × 10−18 |
S.D. | 1.91 × 10−16 | 4.22 × 10−04 | 4.91 × 10+02 | 6.50 × 10−05 | 3.14 × 10−06 | 1.05 × 10−17 | ||
{f_2} | 0 | Mean | 9.06 × 10−06 | 2.01 × 10−01 | 2.26 × 10+01 | 2.00 × 10−01 | 1.48 × 10−04 | 5.87 × 10−07 |
S.D. | 5.83 × 10−05 | 1.41 | 4.26 | 1.41 | 6.99 × 10−04 | 2.82 × 10−06 | ||
{f_3} | 1 | Mean | 4.52 × 10+02 | 4.38 × 10+03 | 7.71 × 10+03 | 1.55 | 1.90 × 10+03 | 1.33 × 10+03 |
S.D. | 1.68 × 10+02 | 1.88× 10+03 | 2.11 × 10+03 | 9.59 × 10−01 | 2.30 × 10+03 | 5.06× 10+02 | ||
{f_4} | 1 | Mean | 7.59 × 10+01 | 1.34× 10+02 | 3.19 × 10+03 | 1.69 × 10+02 | 1.46 × 10+02 | 4.52 × 10−02 |
S.D. | 4.33 × 10+01 | 1.52× 10+02 | 1.65 × 10+03 | 2.75 × 10+02 | 1.41 × 10+02 | 2.98 × 10−02 | ||
{f_5} | 1 | Mean | 3.75 × 10−02 | 7.50 × 10−02 | 9.04 × 10−01 | 1.72 × 10−01 | 4.52 × 10−02 | 1.85 × 10−02 |
S.D. | 1.12 × 10−02 | 2.09 × 10−02 | 3.67 × 10−01 | 4.36 × 10−02 | 1.67 × 10−02 | 6.22 × 10−03 | ||
{f_6} | 1 | Mean | 7.48 × 10+01 | 8.78× 10+01 | 1.16 × 10+02 | 1.32 × 10−03 | 7.67 × 10+01 | 4.79 × 10+01 |
S.D. | 1.62 × 10+01 | 1.83× 10+01 | 2.04 × 10+01 | 6.87 × 10−03 | 1.60 × 10+01 | 3.18 | ||
{f_7} | 0 | Mean | 1.38 | 1.40 × 10−01 | 9.00 | 1.22 | 1.14 | 9.05 × 10−01 |
S.D. | 8.89 × 10−01 | 3.52 × 10−01 | 1.08 | 5.83 × 10−01 | 7.77 × 10−01 | 8.47 × 10−01 | ||
{f_8} | 0 | Mean | 1.36 × 10−02 | 8.18 × 10−03 | 1.22 × 10+01 | 3.18 × 10−04 | 3.79 × 10−02 | 1.43 × 10−02 |
S.D. | 2.61 × 10−02 | 1.02 × 10−02 | 4.71 | 1.41 × 10−03 | 7.54 × 10−02 | 1.76 × 10−02 | ||
{f_9} | 0 | Mean | 1.60 × 10−01 | 1.04 × 10−01 | 2.08 × 10+01 | 8.95 × 10−02 | 7.84 × 10−08 | 2.99 × 10−02 |
S.D. | 2.65 × 10−01 | 1.96 × 10−01 | 8.57 | 1.44 × 10−01 | 4.22 × 10−07 | 4.91 × 10−02 | ||
{f_{10}} | 1 | Mean | 4.07 | 9.60 × 10−01 | 4.06 × 10+02 | 1.35 | 8.78 | 3.53 × 10−01 |
S.D. | 3.36 | 1.12 | 3.10 × 10+02 | 1.87 | 6.03 | 3.69 × 10−01 |
1) Results for the 10-D problems: For {f_1} , the performance of DCS-PSO is second only to LPSO, which maintains population diversity based on different neighborhood topologies, so its performance varies greatly on problems with different topologies. For {f_2} and {f_3} , our DCS-PSO very clearly outperforms all other algorithms, in solution accuracy. DCS-PSO achieves comparable result to other algorithms on {f_4} with CLPSO and DMS-PSO exceeding it. {f_5} is a noise function that is difficult to solve due to the perturbation factor. However, DCS-PSO still gets the best result on this problem.
For multimodal problems, comparing DCS-PSO with CLPSO and DMS-PSO, which are algorithms proposed to improve the performance of PSO on multimodal problems, can demonstrate the competitiveness of DCS-PSO. DCS-PSO and CLPSO perform equally well with zero error on Rastrigin's function ( {f_6} ), which has many local optima. For {f_7} , DCS-PSO achieves the best result, but according to the statistical test result, its performance is comparable to that of DMS-PSO. For {f_8} , PSO-w, GPSO, DMS-PSO and DCS-PSO perform similarly, but CLPSO performs relatively better. For two penalized functions {f_9} and {f_{10}} , although the performance of DCS-PSO on {f_9} is not as good as that of GPSO, CLPSO and DMS-PSO, DCS-PSO achieves the lowest error on {f_{10}} .
Overall, DCS-PSO obtains the best results in solving three unimodal functions ( {f_2} , {f_3} and {f_5} ) and three multimodal functions ( {f_6} , {f_7} and {f_{10}} ). Additionally, Table 6 shows that the S.D. values of DCS-PSO on most test functions are much smaller than those of other algorithms, indicating that DCS-PSO is more stable during the convergence process.
2) Results for the 30-D and 50-D problems: The experiments conducted on 10-D problems are repeated for 30-D and 50-D problems and the results are presented in Tables 7 and 8. All algorithms exhibited similar characteristics as on the 10-D problems. However, high dimensionality leads to increased complexity, resulting in a decline in the performance of optimization algorithms on most problems. As shown in Tables 7 and 8, for {f_1} , {f_2} and {f_5} with 30 and 50 dimensions, DCS-PSO consistently outperforms all other algorithms, particularly on {f_2} . Notably, for the Rosenbrock function ( {f_4} ), DCS-PSO performs similarly to other algorithms in 10 dimensionalities. However, its performance does not deteriorate as the dimension increases, illustrating that high dimensionality does not significantly decrease the performance of DCS-PSO on this problem.
For {f_6} with 30 and 50 dimensions, CLPSO always performs best among all algorithms. DCS-PSO is still best on {f_7} with 30 dimensionalities, while GPSO performs better on this problem with 50 dimensions. CLPSO and DMS-PSO retain their superiority on {f_8} and {f_9} with 30 and 50 dimensionalities, respectively. However, the nonparametric Wilcoxon rank-sum test results indicate that DCS-PSO is not statistically significantly poorer than CLPSO and DMS-PSO. DCS-PSO remains the best-performing algorithm on {f_{10}} , which suggests that its performance is not affected by the increased dimensionality of this function.
3) Comparison of convergence speed: Figure 6 shows the convergence characteristics in terms of the error between the best fitness value found and the actual best function value of the median run of each algorithm for each test function with 30 dimensions. Since the convergence characteristics for the 10-D and 50-D cases are similar to those in the 30-D case, they are not presented.
Combining the numerical results and convergence plots, it can be concluded that all algorithms perform well in solving {f_1} and {f_2} with three different dimensionalities. However, DCS-PSO is faster than other algorithms. PSO exhibits similar convergence characteristics as DCS-PSO on {f_2} . The convergence characteristics of each algorithm on {f_1} and {f_2} are shown in Figure 6(a) and (b). Figure 6(c) indicates that CLPSO has better convergence speed and accuracy than other algorithms on {f_3} . Although DCS-PSO has slightly lower convergence accuracy than CLPSO on {f_4} , it has the fastest convergence speed as illustrated in Figure 6(d). All algorithms perform similarly on the noise problem ( {f_5} ). Figure 6(e) displays that these algorithms show a slow decrease in error, but DCS-PSO has the fastest convergence speed and the lowest error.
The advantages of CLPSO and DMS-PSO are revealed through some multimodal problems. As shown in Figure 6(f), although the convergence speed is slow, CLPSO has the best search accuracy on {f_6} . In contrast, other algorithms cannot continue to search effectively after reaching a certain accuracy. From the convergence characteristics in Figure 6(g), the performances of DCS-PSO and DMS-PSO are also excellent on {f_7} , but DCS-PSO has the fastest convergence speed. Although DCS-PSO is not as good as CLPSO and DMS-PSO in terms of average errors on {f_8} and {f_9} , there is no statistically significant difference according to the Wilcoxon tests, as verified in Figure 6(h, i). It can be seen from Figure 6(j) that DCS-PSO has the best search efficiency on {f_{10}} . Considering the numerical results and the convergence speeds, DCS-PSO performs best among all tested algorithms.
4) Discussion: The nonparametric Wilcoxon rank-sum tests indicate that DCS-PSO is significantly better than or equivalent to the other algorithms on most problems with three different dimensionality cases. When the problems are 10-dimensional, DCS-PSO is significantly better than other algorithms on {f_2} , {f_3} , {f_5} and {f_{10}} , and is equivalent to CLPSO and DMS-PSO on {f_6} and {f_7} , respectively. For {f_8} and {f_9} , with 30 and 50 dimensionalities, DCS-PSO performs equivalently to CLPSO and DMS-PSO, respectively. All statistical results demonstrate that DCS-PSO performs very well overall.
In the above experiments, DCS-PSO shows better convergence speed and accuracy on most unimodal problems, indicating that it is more effective in solving such problems. Simultaneously,
the performance of DCS-PSO on most multimodal problems is comparable to that of CLPSO and DMS-PSO, demonstrating its ideal global search performance and ability to escape local optima.
This section further uses a widely recognized CEC2017 test suite to confirm the performance of DCS-PSO with PSO variants. In CEC2017, there are 29 functions (F2 has been excluded because it shows unstable behavior, especially for higher dimensions) which can be classified into four categories: unimodal functions ( {f_1} , {f_3} ), multimodal functions ( {f_4} – {f_{10}} ), hybrid functions ( {f_{11}} – {f_{20}} ) and composition functions ( {f_{21}} – {f_{30}} ). Due to space constraints, we only select D = 30 for analysis and discussion. The best value for each function is shown in bold.
Table 9 shows that CLPSO and DMS-PSO obtain the best result in unimodal functions, followed by DCS-PSO. In multimodal functions, DCS-PSO achieves the best performance on {f_4} , {f_5} , {f_8} and {f_9} , 4 of 7 multimodal functions in terms of mean value, followed by GPSO, CLPSO, and DMS-PSO, which all obtain the best solutions on one function.
Function | Criteria | PSO-w | GPSO | LPSO | CLPSO | DMS-PSO | DCS-PSO |
F1 | Mean | 5.73 × 10+08 | 9.15 × 10+09 | 5.24 × 10+09 | 1.01 × 10+02 | 3.26 × 10+03 | 6.44 × 10+03 |
S.D. | 2.36 × 10+09 | 1.29 × 10+10 | 9.65 × 10+09 | 5.12 × 10−01 | 4.53 × 10+03 | 1.93 × 10+03 | |
F3 | Mean | 2.52 × 10+03 | 5.01 × 10+03 | 9.58 × 10+03 | 4.90 × 10+02 | 2.16 × 10+02 | 5.45 × 10+02 |
S.D. | 1.23 × 10+03 | 2.38 × 10+03 | 5.11 × 10+03 | 1.39 × 10+01 | 3.43 × 10+02 | 3.90 × 10−13 | |
F4 | Mean | 1.03 × 10+02 | 1.73 × 10+02 | 2.15 × 10+02 | 5.53 × 10+02 | 7.39 × 10+01 | 1.22 |
S.D. | 3.19 × 10+01 | 1.02 × 10+02 | 1.92 × 10+02 | 7.54 | 2.39 × 10+01 | 1.75 | |
F5 | Mean | 1.43 × 10+02 | 7.61 × 10+01 | 1.42 × 10+02 | 6.00 × 10+02 | 5.88 × 10+01 | 2.09 × 10+01 |
S.D. | 3.11 × 10+01 | 2.08 × 10+01 | 3.78 × 10+01 | 0.00 | 1.30 × 10+01 | 7.01 | |
F6 | Mean | 4.01 × 10+01 | 1.43 × 10+01 | 5.14 × 10+01 | 7.87 × 10+02 | 9.86 × 10−04 | 3.75 |
S.D. | 1.49 × 10+01 | 5.47 | 1.39 × 10+01 | 6.43 | 2.47 × 10−03 | 3.17 | |
F7 | Mean | 1.29 × 10+02 | 1.12 × 10+01 | 1.91 × 10+02 | 8.49 × 10+02 | 9.76 × 10+01 | 1.28 × 10+02 |
S.D. | 3.46 × 10+01 | 1.03 × 10+01 | 4.86 × 10+01 | 1.03 × 10+01 | 1.99 × 10+01 | 9.23 | |
F8 | Mean | 1.20 × 10+02 | 6.83 × 10+01 | 1.24 × 10+02 | 9.09 × 10+02 | 5.94 × 10+01 | 1.62 × 10+01 |
S.D. | 3.18 × 10+01 | 1.66 × 10+01 | 2.95 × 10+01 | 3.05 | 1.68 × 10+01 | 5.52 | |
F9 | Mean | 1.38 × 10+03 | 1.53 × 10+02 | 2.26 × 10+03 | 3.27 × 10+03 | 2.33 × 10+01 | 6.09 × 10−01 |
S.D. | 1.18 × 10+03 | 1.64 × 10+02 | 9.36 × 10+02 | 2.89 × 10+02 | 5.63 × 10+01 | 1.83 | |
F10 | Mean | 3.61 × 10+03 | 3.19 × 10+03 | 3.91 × 10+03 | 1.18 × 10+03 | 2.97 × 10+03 | 1.99 × 10+03 |
S.D. | 5.67 × 10+02 | 6.28 × 10+02 | 7.81 × 10+02 | 4.51 | 5.89 × 10+02 | 2.87 × 10+02 | |
F11 | Mean | 1.30 × 10+02 | 1.71 × 10+02 | 1.76 × 10+02 | 5.97 × 10+05 | 4.74 × 10+01 | 1.12 × 10+02 |
S.D. | 4.18 × 10+01 | 5.41 × 10+01 | 5.11 × 10+01 | 3.96 × 10+05 | 3.12 × 10+01 | 2.44 × 10+01 | |
F12 | Mean | 4.56 × 10+07 | 6.40 × 10+07 | 1.76 × 10+08 | 2.62 × 10+03 | 8.25 × 10+04 | 2.79 × 10+03 |
S.D. | 2.83 × 10+08 | 1.12 × 10+08 | 5.00 × 10+08 | 1.55 × 10+03 | 2.16 × 10+04 | 2.07 × 10+03 | |
F13 | Mean | 1.77 × 10+06 | 5.01 × 10+07 | 1.88 × 10+07 | 1.75 × 10+04 | 1.55 × 10+04 | 1.27 × 10+04 |
S.D. | 8.67 × 10+06 | 1.71 × 10+08 | 1.02 × 10+08 | 9.65 × 10+03 | 1.65 × 10+04 | 3.86 × 10+03 | |
F14 | Mean | 1.80 × 10+04 | 3.70 × 10+04 | 1.10 × 10+04 | 1.61 × 10+03 | 1.32 × 10+04 | 1.02 × 10+02 |
S.D. | 2.36 × 10+04 | 4.40 × 10+04 | 1.30 × 10+04 | 2.70 × 10+01 | 1.23 × 10+04 | 2.45 × 10+01 | |
F15 | Mean | 3.99 × 10+03 | 1.97 × 10+04 | 1.07 × 10+04 | 2.16 × 10+03 | 6.90 × 10+03 | 1.09 × 10+02 |
S.D. | 3.96 × 10+03 | 3.05 × 10+04 | 1.25 × 10+04 | 1.32 × 10+02 | 9.16 × 10+03 | 1.14 × 10+02 | |
F16 | Mean | 1.04 × 10+03 | 7.61 × 10+02 | 1.13 × 10+03 | 1.84 × 10+03 | 7.95 × 10+02 | 1.79 × 10+03 |
S.D. | 2.73 × 10+02 | 2.47 × 10+02 | 2.95 × 10+02 | 4.18 × 10+01 | 2.10 × 10+02 | 1.25 × 10+02 | |
F17 | Mean | 4.00 × 10+04 | 1.57 × 10+04 | 1.21 × 10+04 | 2.16 × 10+05 | 1.89 × 10+02 | 4.62 × 10+03 |
S.D. | 6.54 × 10+02 | 1.54 × 10+03 | 2.56 × 10+03 | 1.61 × 10+05 | 1.14 × 10+02 | 6.54 × 10+02 | |
F18 | Mean | 1.41 × 10+05 | 4.45 × 10+05 | 1.12 × 10+05 | 1.95 × 10+03 | 1.27 × 10+05 | 5.56 × 10+02 |
S.D. | 1.04 × 10+05 | 3.62 × 10+05 | 1.15 × 10+05 | 1.88 × 10+01 | 1.06 × 10+05 | 8.59 × 10+02 | |
F19 | Mean | 6.20 × 10+03 | 4.47 × 10+05 | 6.10 × 10+03 | 6.30 × 10+03 | 9.39 × 10+03 | 7.78 × 10+03 |
S.D. | 6.09 × 10+03 | 2.71 × 10+06 | 6.37 × 10+03 | 2.64 × 10+01 | 1.28 × 10+04 | 1.08 × 10+03 | |
F20 | Mean | 1.70 × 10+04 | 1.45 × 10+04 | 1.92 × 10+04 | 2.36 × 10+03 | 2.73 × 10+02 | 6.00 × 10+03 |
S.D. | 3.26 × 10+03 | 3.44 × 10+02 | 2.75 × 10+03 | 5.04 | 1.41 × 10+02 | 5.32 × 10+02 | |
F21 | Mean | 3.39 × 10+02 | 2.80 × 10+02 | 3.34 × 10+02 | 2.65 × 10+03 | 2.62 × 10+02 | 1.03 × 10+02 |
S.D. | 5.45 × 10+01 | 4.25 × 10+01 | 4.90 × 10+01 | 5.40 × 10+02 | 2.11 × 10+01 | 8.18 × 10−01 | |
F22 | Mean | 4.15 × 10+02 | 6.72 × 10+02 | 1.00 × 10+03 | 2.72 × 10+03 | 2.38 × 10+02 | 1.09 × 10+02 |
S.D. | 9.94 × 10+02 | 7.48 × 10+02 | 1.50 × 10+03 | 1.15 × 10+01 | 5.92 × 10+02 | 1.44 | |
F23 | Mean | 6.42 × 10+02 | 5.82 × 10+02 | 6.73 × 10+02 | 2.92 × 10+03 | 4.30 × 10+02 | 3.25 × 10+02 |
S.D. | 1.01 × 10+02 | 9.36 × 10+01 | 1.10 × 10+02 | 1.21 × 10+01 | 2.10 × 10+01 | 7.91 | |
F24 | Mean | 6.61 × 10+02 | 6.34 × 10+02 | 7.14 × 10+02 | 2.89 × 10+03 | 4.94 × 10+02 | 3.53 × 10+02 |
S.D. | 7.19 × 10+01 | 8.33 × 10+01 | 8.07 × 10+01 | 2.23 × 10−01 | 2.60 × 10+01 | 1.09 × 10+01 | |
F25 | Mean | 3.95 × 10+02 | 4.13 × 10+02 | 4.47 × 10+02 | 3.47 × 10+03 | 3.97 × 10+02 | 4.26 × 10+02 |
S.D. | 1.50 × 10+01 | 3.64 × 10+01 | 3.05 × 10+01 | 7.89 × 10+02 | 1.53 | 2.34 × 10+01 | |
F26 | Mean | 1.24 × 10+03 | 1.51 × 10+03 | 2.45 × 10+03 | 3.21 × 10+03 | 1.49 × 10+03 | 4.02 × 10+02 |
S.D. | 1.29 × 10+03 | 7.71 × 10+02 | 1.36 × 10+03 | 6.70 | 5.18 × 10+02 | 5.94 × 10+01 | |
F27 | Mean | 5.88 × 10+02 | 5.80 × 10+02 | 6.21 × 10+02 | 3.21 × 10+03 | 5.38 × 10+02 | 4.03 × 10+02 |
S.D. | 5.87 × 10+01 | 5.54 × 10+01 | 5.36 × 10+01 | 5.65 | 1.25 × 10+01 | 4.45 | |
F28 | Mean | 4.34 × 10+02 | 5.05 × 10+02 | 5.49 × 10+02 | 3.45 × 10+03 | 3.40 × 10+02 | 3.88 × 10+02 |
S.D. | 4.76 × 10+01 | 7.23 × 10+01 | 1.46 × 10+02 | 4.85 | 5.79 × 10+01 | 8.36 | |
F29 | Mean | 6.25 × 10+02 | 8.71 × 10+02 | 6.24 × 10+02 | 8.91 × 10+02 | 6.03 × 10+02 | 6.38 × 10+02 |
S.D. | 4.87 × 10+01 | 6.31 × 10+01 | 2.17 × 10+02 | 7.46 × 10+01 | 1.55 × 10+02 | 7.88 × 10+01 | |
F30 | Mean | 4.52 × 10+04 | 4.50 × 10+05 | 3.78 × 10+05 | 1.12 × 10+03 | 5.81 × 10+03 | 3.14 × 10+05 |
S.D. | 1.10 × 10+05 | 1.41 × 10+06 | 4.93 × 10+05 | 2.22 × 10+03 | 2.34 × 10+03 | 5.14 × 10+05 |
For the 10 hybrid functions, DCS-PSO reaches the best solutions on {f_{13}} , {f_{14}} , {f_{15}} and {f_{18}} , 4 of 10 hybrid functions. DMS-PSO also performs remarkably since it reaches the best solutions on 3 out of 10 functions, followed by GPSO, LPSO and CLPSO, which all obtain the best solutions on one function. In the ten composition functions, DMS-PSO attains remarkable results on {f_{21}} , {f_{22}} , {f_{23}} , {f_{24}} , {f_{26}} and {f_{27}} , 6 out of 10 composition functions. The comparison results indicate that DCS-PSO works well in complex and multimodal situations.
Friedman test is used in this section to compare the performance of DCS-PSO and other PSO variants. The results of the Friedman test are shown in Table 10. It can be seen from Table 10 that DCS-PSO achieves a remarkable result on the CEC2017, followed by DMS-PSO, PSO-w, GPSO, CLPSO and LPSO. The overall performance in CEC2017 reveals that DCS-PSO has a distinct advantage over other compared algorithms.
Average rank | Algorithm | Ranking |
1 | DCS-PSO | 2.03 |
2 | DMS-PSO | 2.17 |
3 | PSO-w | 3.69 |
4 | GPSO | 4.07 |
5 | CLPSO | 4.45 |
6 | LPSO | 4.59 |
In this paper, we propose an improved particle swarm optimization combined with double-chaos search mechanism, namely DCS-PSO. The standard PSO is simple and efficient, but it is difficult to maintain population diversity when dealing with complex problems with a large search space, leading to premature convergence and getting trapped in local optima. The chaos search mechanism has the advantages of global traversal and avoiding falling into local optima.
In the first stage of DCS-PSO, we adopt the double-chaos search mechanism to narrow the search space to the vicinity of the optimal solution, effectively reducing the risk of PSO getting trapped in local optima. In the second stage, to enhance the population diversity, the logistic map is utilized to perform a global search in the narrowed search space and the best solution obtained from both chaos search and population search will guide the population to converge. Experimental studies show that the algorithm can effectively narrow the search space and has better convergence accuracy and speed for most functions.
Of course, according to the no free lunch theorem, DCS-PSO cannot optimally solve every kind of global optimization problem. Although we use the chaos search mechanism to improve the convergence speed and ability to escape the local optima of DCS-PSO, it also brings about a problem worth further investigation: how to ensure that the optimal solution must exist in the narrowed search space. In the future, we will continue conducting in-depth research on chaos theory, optimize our DCS-PSO algorithm and try to apply DCS-PSO to solving these real-world problems.
The authors declare they have not used Artificial Intelligence (AI) tools in the creation of this article.
This research was supported by the National Natural Science Foundation of China (Grant No 82260849); the National Natural Science Foundation of China (Grant No 61562045); and Jiangxi University of Chinese Medicine Science and Technology Innovation Team Development Program (Grant No CXTD22015).
[1] |
B. T. Ngo, P. Marik, P. Kory, L. Shapiro, R. Thomadsen, J. Iglesias, et al., The time to offer treatments for COVID-19, Expert Opin. Invest. Drugs, 30 (2021), 505–518. https://doi.org/10.1080/13543784.2021.1901883 doi: 10.1080/13543784.2021.1901883
![]() |
[2] |
B. Pfefferbaum, C. S. North, Mental health and the COVID-19 pandemic, N. Engl. J. Med., 383 (2020), 510–512. https://doi.org/10.1056/NEJMp2008017 doi: 10.1056/NEJMp2008017
![]() |
[3] |
E. J. Kim, L. Marrast, J. Conigliaro, COVID-19: magnifying the effect of health disparities, J. Gen. Intern. Med., 35 (2020), 2441–2442. https://doi.org/10.1007/s11606-020-05881-4 doi: 10.1007/s11606-020-05881-4
![]() |
[4] |
J. Campion, A. Javed, N. Sartorius, M. Marmot, Addressing the public mental health challenge of COVID-19, Lancet Psychiatry, 7 (2020), 657–659. https://doi.org/10.1016/S2215-0366(20)30240-6 doi: 10.1016/S2215-0366(20)30240-6
![]() |
[5] |
A. T. Gloster, D. Lamnisos, J. Lubenko, G. Presti, V. Squatrito, M. Constantinou, et al., Impact of COVID-19 pandemic on mental health: an international study, PloS One, 15 (2020), e0244809. https://doi.org/10.1371/journal.pone.0244809 doi: 10.1371/journal.pone.0244809
![]() |
[6] |
D. Talevi, V. Socci, M. Carai, G. Carnaghi, S. Faleri, E. Trebbi, et al., Mental health outcomes of the COVID-19 pandemic, Riv. Psichiatr., 55 (2020), 137–144. https://doi.org/10.1708/3382.33569 doi: 10.1708/3382.33569
![]() |
[7] |
E. A. Wastnedge, R. M. Reynolds, S. R. Van Boeckel, S. J. Stock, F. C. Denison, J. A. Maybin, et al., Pregnancy and COVID-19, Physiol. Rev., 101 (2021), 303–318. https://doi.org/10.1152/physrev.00024.2020 doi: 10.1152/physrev.00024.2020
![]() |
[8] |
W. Bo, Z. Ahmad, A. R. Alanzi, A. I. Al-Omari, E. H. Hafez, S. F. Abdelwahab, The current COVID-19 pandemic in China: an overview and corona data analysis, Alexandria Eng. J., 61 (2021), 1369–1381. https://doi.org/10.1016/j.aej.2021.06.025 doi: 10.1016/j.aej.2021.06.025
![]() |
[9] |
V. H. Moreau, Forecast predictions for the COVID-19 pandemic in Brazil by statistical modeling using the Weibull distribution for daily new cases and deaths, Braz. J. Microbiol., 51 (2020), 1109–1115. https://doi.org/10.1007/s42770-020-00331-z doi: 10.1007/s42770-020-00331-z
![]() |
[10] |
S. Tuli, S. Tuli, R. Tuli, S. S. Gill, Predicting the growth and trend of COVID-19 pandemic using machine learning and cloud computing, Internet Things, 11 (2020), 100222. https://doi.org/10.1016/j.iot.2020.100222 doi: 10.1016/j.iot.2020.100222
![]() |
[11] |
S. M. Rahman, J. Kim, B. Laratte, Disruption in Circularity? Impact analysis of COVID-19 on ship recycling using Weibull tonnage estimation and scenario analysis method, Resour. Conserv. Recycl., 164 (2021), 105139. https://doi.org/10.1016/j.resconrec.2020.105139 doi: 10.1016/j.resconrec.2020.105139
![]() |
[12] |
E. M. Almetwally, R. Alharbi, D. Alnagar, E. H. Hafez, A new inverted topp-leone distribution: applications to the COVID-19 mortality rate in two different countries, Axioms, 10 (2021), 25. https://doi.org/10.3390/axioms10010025 doi: 10.3390/axioms10010025
![]() |
[13] |
M. Alizadeh, G. M. Cordeiro, A. D. Nascimento, M. D. C. S. Lima, E. M. Ortega, Odd-Burr generalized family of distributions with some applications, J. Stat. Comput. Simul., 87 (2017), 367–389. https://doi.org/10.1080/00949655.2016.1209200 doi: 10.1080/00949655.2016.1209200
![]() |
[14] |
F. Chipepa, B. Oluyede, B. Makubate, A new generalized family of odd Lindley-G distributions with application, Int. J. Stat. Probab., 8 (2019), 1–22. https://doi.org/10.5539/ijsp.v8n6p1 doi: 10.5539/ijsp.v8n6p1
![]() |
[15] |
L. Handique, S. Chakraborty, T. A. de Andrade, The exponentiated generalized Marshall–Olkin family of distribution: its properties and applications, Ann. Data Sci., 6 (2019), 391–411. https://doi.org/10.1007/s40745-018-0166-z doi: 10.1007/s40745-018-0166-z
![]() |
[16] |
M. H. Tahir, M. A. Hussain, G. M. Cordeiro, M. El-Morshedy, M. S. Eliwa, A new Kumaraswamy generalized family of distributions with properties, applications, and bivariate extension, Mathematics, 8 (2020), 1989. https://doi.org/10.3390/math8111989 doi: 10.3390/math8111989
![]() |
[17] |
S. M. Zaidi, M. M. A. Sobhi, M. El-Morshedy, A. Z. Afify, A new generalized family of distributions: properties and applications, AIMS Math., 6 (2021), 456–476. https://doi.org/10.3934/math.2021028 doi: 10.3934/math.2021028
![]() |
[18] |
F. H. Riad, E. Hussam, A. M. Gemeay, R. A. Aldallal, A. Z. Afify, Classical and Bayesian inference of the weighted-exponential distribution with an application to insurance data, Math. Biosci. Eng., 19 (2022), 6551–6581. https://doi.org/10.3934/mbe.2022309 doi: 10.3934/mbe.2022309
![]() |
[19] |
M. E. Bakr, A. A. Al-Babtain, Z. Mahmood, R. A. Aldallal, S. K. Khosa, M. M. Abd El-Raouf, et al., Statistical modelling for a new family of generalized distributions with real data applications, Math. Biosci. Eng., 19 (2022), 8705–8740. https://doi.org/10.3934/mbe.2022404 doi: 10.3934/mbe.2022404
![]() |
[20] |
A. Xu, S. Zhou, Y. Tang, A unified model for system reliability evaluation under dynamic operating conditions, IEEE Trans. Reliab., 70 (2019), 65–72. https://doi.org/10.1109/TR.2019.2948173 doi: 10.1109/TR.2019.2948173
![]() |
[21] |
C. Luo, L. Shen, A. Xu, Modelling and estimation of system reliability under dynamic operating environments and lifetime ordering constraints, Reliab. Eng. Syst. Saf., 218 (2022), 108136. https://doi.org/10.1016/j.ress.2021.108136 doi: 10.1016/j.ress.2021.108136
![]() |
[22] |
A. Alzaatreh, C. Lee, F. Famoye, A new method for generating families of continuous distributions, Metron, 71 (2013), 63–79. https://doi.org/10.1007/s40300-013-0007-y doi: 10.1007/s40300-013-0007-y
![]() |
[23] |
H. M. Almongy, E. M. Almetwally, H. M. Aljohani, A. S. Alghamdi, E. H. Hafez, A new extended rayleigh distribution with applications of COVID-19 data, Results Phys., 23 (2021), 104012. https://doi.org/10.1016/j.rinp.2021.104012 doi: 10.1016/j.rinp.2021.104012
![]() |
[24] |
M. Qi, G. P. Zhang, An investigation of model selection criteria for neural network time series forecasting, Eur. J. Oper. Res., 132 (2001), 666–680. https://doi.org/10.1016/S0377-2217(00)00171-5 doi: 10.1016/S0377-2217(00)00171-5
![]() |
[25] |
C. Cortes, V. Vapnik, Support-vector networks, Mach. Learn., 20 (1995), 273–297. https://doi.org/10.1007/BF00994018 doi: 10.1007/BF00994018
![]() |
[26] |
M. H. D. M. Ribeiro, R. G. da Silva, V. C. Mariani, L. dos Santos Coelho, Short-term forecasting COVID-19 cumulative confirmed cases: perspectives for Brazil, Chaos, Solitons Fractals, 135 (2020), 109853. https://doi.org/10.1016/j.chaos.2020.109853 doi: 10.1016/j.chaos.2020.109853
![]() |
[27] |
N. Bibi, I. Shah, A. Alsubie, S. Ali, S. A. Lone, Electricity spot prices forecasting based on ensemble learning, IEEE Access, 9 (2021), 150984–150992. https://doi.org/10.1109/ACCESS.2021.3126545 doi: 10.1109/ACCESS.2021.3126545
![]() |
[28] |
C. J. Lu, T. S. Lee, C. C. Chiu, Financial time series forecasting using independent component analysis and support vector regression, Decis. Support Syst., 47 (2009), 115–125. https://doi.org/10.1016/j.dss.2009.02.001 doi: 10.1016/j.dss.2009.02.001
![]() |
[29] |
L. Breiman, Random forests, Mach. Learn., 45 (2001), 5–32. https://doi.org/10.1023/A:1010933404324 doi: 10.1023/A:1010933404324
![]() |
[30] | T. G. Dietterich, Ensemble methods in machine learning, in International Workshop on Multiple Classifier Systems, Springer, Berlin, Heidelberg, 1857 (2000), 1–15. https://doi.org/10.1007/3-540-45014-9_1 |
[31] |
Z. Peng, F. U. Khan, F. Khan, P. A. Shaikh, Y. H. Dai, I. Ullah, et al., An application of hybrid models for weekly stock market index prediction: empirical evidence from SAARC countries, Complexity, 2021 (2021). https://doi.org/10.1155/2021/5663302 doi: 10.1155/2021/5663302
![]() |
![]() |
![]() |
1. | Santuan Qin, Huadie Zeng, Wei Sun, Jin Wu, Junhua Yang, Multi-Strategy Improved Particle Swarm Optimization Algorithm and Gazelle Optimization Algorithm and Application, 2024, 13, 2079-9292, 1580, 10.3390/electronics13081580 | |
2. | Guangyun Cui, Zhen Qi, Huaqing Zhao, Ranhang Zhao, Haofang Wang, Jiaxing Zhao, Application of F-HGAPSO Algorithm in Reservoir Flood Control Optimal Operation, 2024, 0920-4741, 10.1007/s11269-024-04045-x |
PC configuration | Information |
CPU | Inter(R) Core(TM) i7-8700 |
Frequency | 3.2GHz CPU |
RAM | 16.0GB |
Operating system | Windows 10(64Bit) |
Language | Python 3.9.13 |
Algorithm | Parameters |
COA | \max \_iter1 = {10^5}, {\text{ }}\max \_iter2 = 1.5 \times {10^5}, {\text{ }}\alpha = 0.3 |
DCOA | h = 3000, {\text{ }}\gamma = 0.25, {\text{ }}\xi {\text{ = 1}}{\text{.5}} |
PCOA | n\_group = 3, {\text{ }}\max \_iter1 = {10^5}, {\text{ }}\max \_iter2 = 1.5 \times {10^5}, {\text{ }}\alpha = 0.3 |
DCS-PSO | N = 20, {\text{ }}w = 0.4, {\text{ }}c1 = c2 = 2, {\text{ }}{V_{\max }} = 0.2 \times Range, {\text{ }}\max \_iter = 1000 |
Function | Criteria | COA | DCOA | PCOA | DCS-PSO |
{f_J} | E.T. | 30.8898 | 0.5753 | 17.2234 | 0.2793 |
Mean | 0.0000 | 0.0005 | 0.0000 | 0.0000 | |
S.D. | 0.0000 | 0.0014 | 0.0000 | 0.0000 | |
{f_C} | E.T. | 7.6564 | 0.6478 | 10.3990 | 0.3610 |
Mean | −1.0316 | −1.0312 | −1.0316 | −1.0316 | |
S.D. | 0.0000 | 0.0012 | 0.0000 | 0.0000 | |
{f_G} | E.T. | 14.1867 | 1.0656 | 14.0814 | 0.6097 |
Mean | 3.0022 | 3.0100 | 3.0024 | 3.0000 | |
S.D. | 0.0022 | 0.0126 | 0.0022 | 0.0000 | |
{f_B} | E.T. | 1.5663 | 0.8653 | 5.7455 | 0.4513 |
Mean | 0.3983 | 0.4003 | 0.3980 | 0.3979 | |
S.D. | 0.0003 | 0.0039 | 0.0001 | 0.0000 | |
{f_R} | E.T. | 4.4150 | 0.6652 | 5.8342 | 0.4366 |
Mean | −1.9993 | −1.9652 | −1.9992 | −2.0000 | |
S.D. | 0.0006 | 0.0558 | 0.0008 | 0.0000 | |
{f_S} | E.T. | 5.8609 | 2.1743 | 13.8397 | 2.0615 |
Mean | −186.6428 | -186.4517 | −186.6861 | −186.7309 | |
S.D. | 0.0711 | 0.4203 | 0.0680 | 0.0000 |
Name | Functions | Range | Optimum |
Sphere | {f_1}\left(\boldsymbol x \right) = \sum\limits_{i = 1}^D {x_i^2} | [−100, 100] | 0 |
Schwefel's 2.22 | {f_2}\left(\boldsymbol x \right) = \sum\limits_{i = 1}^D {|{x_i}|} + \prod\limits_{i = 1}^D {|{x_i}|} | [−10, 10] | 0 |
Schwefel's 1.2 | {f_3}\left(\boldsymbol x \right) = \sum\limits_{i = 1}^D {{{\left({\sum\limits_{j = 1}^i {{x_j}} } \right)}^2}} | [−100, 100] | 0 |
Rosenbrock | {f_4}\left(\boldsymbol x \right) = \sum\limits_{i = 1}^{D - 1} {\left[{100{{\left({{x_{i + 1}} - x_i^2} \right)}^2} + {{\left({{x_i} - 1} \right)}^2}} \right]} | [−10, 10] | 0 |
Noise | {f_5}\left(\boldsymbol x \right) = \sum\limits_{i = 1}^D {ix_i^4} + random[0, 1) | [−1.28, 1.28] | 0 |
Rastrigin | {f_6}\left(\boldsymbol x \right) = \sum\limits_{i = 1}^D {\left({x_i^2 - 10\cos \left({2\pi {x_i}} \right) + 10} \right)} | [−5.12, 5.12] | 0 |
Ackley | {f_7}\left(\boldsymbol x \right) = - 20\exp \left({ - 0.2\sqrt {\frac{1}{D}\sum\limits_{i = 1}^D {x_i^2} } } \right) - \exp \left({\frac{1}{D}\sum\limits_{i = 1}^D {\cos \left({2\pi {x_i}} \right)} } \right) + 20 + e | [−32, 32] | 0 |
Griewank | {f_8}\left(\boldsymbol x \right) = \frac{1}{{4000}}\sum\limits_{i = 1}^D {x_i^2} - \prod\limits_{i = 1}^D {\cos \left({\frac{{{x_i}}}{{\sqrt i }}} \right)} + 1 | [−600, 600] | 0 |
Penalized 1 | \begin{array}{l} {f_9}\left(\boldsymbol x \right) = \frac{\pi }{D}\left\{ {10{{\sin }^2}\left({\pi {y_1}} \right) + \sum\limits_{i = 1}^{D - 1} {{{\left({{y_i} - 1} \right)}^2}} \left[{1 + 10{{\sin }^2}\left({\pi {y_{i + 1}}} \right)} \right] + {{\left({{y_D} - 1} \right)}^2}} \right\} \\ + \sum\limits_{i = 1}^D {u\left({{x_i}, 10, 100, 4} \right)} \\ \end{array} | [−50, 50] | 0 |
Penalized 2 | \begin{array}{l} {f_{10}}\left(\boldsymbol x \right) = \frac{1}{{10}}\left\{ {{{\sin }^2}\left({3\pi {x_1}} \right) + \sum\limits_{i = 1}^D {{{\left({{x_i} - 1} \right)}^2}} \left[{1 + {{\sin }^2}\left({3\pi {x_i} + 1} \right)} \right] + {{\left({{x_D} - 1} \right)}^2}\left[{1 + {{\sin }^2}\left({2\pi {x_D}} \right)} \right]} \right\} \\ + \sum\limits_{i = 1}^D {u\left({{x_i}, 5, 100, 4} \right)} \\ \end{array} | [−50, 50] | 0 |
Algorithm | Parameters |
PSO-w | w:0.4, {\text{ }}{c_1} = {c_2} = 2.0, {\text{ }}{V_{\max }} = 0.2 \times Range |
GPSO | w:0.9 \sim 0.4, {\text{ }}{c_1} = {c_2} = 2.0, {\text{ }}{V_{\max }} = 0.2 \times Range |
LPSO | \chi = 0.7298, {\text{ }}{c_1} = {c_2} = 1.49445, {\text{ }}{V_{\max }} = 0.2 \times Range |
CLPSO | w:0.9\sim 0.4, {\text{ }}c = 1.49445, {\text{ }}{V_{\max }} = 0.2 \times Range, {\text{ }}m = 7 |
DMS-PSO | \chi = 0.7298, {\text{ }}{c_1} = {c_2} = 1.49445, {\text{ }}{V_{\max }} = 0.2 \times Range, {\text{ }}M = 4, {\text{ }}R = 10 |
DCS-PSO | w:0.4, {c_1} = {c_2} = 2.0, {V_{\max }} = 0.2 \times Range, {\text{ }}h = 3000, \gamma = 0.15, \xi = 1.5 |
Function | k | Criteria | PSO-w | GPSO | LPSO | CLPSO | DMS-PSO | DCS-PSO |
{f_1} | 1 | Mean | 1.84 × 10−144 | 1.47 × 10−73 | 2.78 × 10−169 | 2.18 × 10−21 | 6.30 × 10−62 | 2.35 × 10−151 |
S.D. | 9.13 × 10−144 | 1.04 × 10−72 | 0.00 | 5.44 × 10−21 | 4.25 × 10−61 | 7.75 × 10−151 | ||
{f_2} | 1 | Mean | 1.89 × 10−81 | 6.24 × 10−38 | 7.59 × 10−09 | 4.30 × 10−14 | 3.45 × 10−20 | 9.65 × 10−84 |
S.D. | 1.26 × 10−80 | 2.99 × 10−37 | 3.80 × 10−08 | 1.39 × 10−13 | 5.86 × 10−20 | 4.81 × 10−83 | ||
{f_3} | 1 | Mean | 1.22 × 10−44 | 9.93 × 10−17 | 6.11 × 10−13 | 2.18 × 10−20 | 6.13 × 10−10 | 1.21 × 10−48 |
S.D. | 7.42 × 10−44 | 3.36 × 10−16 | 4.32 × 10−12 | 3.25 × 10−20 | 3.33 × 10−09 | 2.38 × 10−48 | ||
{f_4} | 1 | Mean | 2.79 | 2.80 | 4.00 | 2.49 | 1.60 | 9.11 |
S.D. | 1.39 | 1.16 | 2.56 | 4.95 × 10−01 | 9.79 × 10−01 | 1.89 | ||
{f_5} | 1 | Mean | 7.23 × 10−04 | 1.02 × 10−03 | 3.25 × 10−03 | 1.49 × 10−03 | 8.80 × 10−04 | 2.15 × 10−04 |
S.D. | 3.90 × 10−04 | 5.46 × 10−04 | 2.43 × 10−03 | 7.09 × 10−04 | 4.49 × 10−04 | 1.58 × 10−04 | ||
{f_6} | 0 | Mean | 5.99 | 2.13 | 9.35 | 0.00 | 2.67 | 0.00 |
S.D. | 2.74 | 1.15 | 3.53 | 0.00 | 1.36 | 0.00 | ||
{f_7} | 0 | Mean | 4.14 × 10−15 | 4.07 × 10−15 | 4.67 × 10−15 | 3.16 × 10−14 | 4.00 × 10−15 | 3.91 × 10−15 |
S.D. | 7.03 × 10−16 | 5.02 × 10−16 | 1.62 × 10−15 | 5.29 × 10−14 | 0.00 | 5.62 × 10−16 | ||
{f_8} | 1 | Mean | 7.44 × 10−02 | 6.88 × 10−02 | 1.06 × 10−01 | 1.64 × 10−03 | 7.12 × 10−02 | 6.65 × 10−02 |
S.D. | 3.41 × 10−02 | 3.13 × 10−02 | 5.81 × 10−02 | 7.61 × 10−04 | 3.55 × 10−02 | 3.75 × 10−02 | ||
{f_9} | 1 | Mean | 1.24 × 10−02 | 4.71 × 10−32 | 6.22 × 10−03 | 2.99 × 10−30 | 1.38 × 10−28 | 1.78 × 10−01 |
S.D. | 6.16 × 10−02 | 1.11 × 10−47 | 4.40 × 10−02 | 3.07 × 10−30 | 2.65 × 10−28 | 6.11 × 10−02 | ||
{f_{10}} | 1 | Mean | 3.56 × 10−04 | 9.33 × 10−20 | 9.80 × 10−04 | 3.16 × 10−27 | 2.57 × 10−13 | 1.35 × 10−32 |
S.D. | 2.52 × 10−03 | 1.90 × 10−19 | 6.86 × 10−03 | 6.79 × 10−27 | 4.33 × 10−13 | 8.29 × 10−48 |
Function | k | Criteria | PSO-w | GPSO | LPSO | CLPSO | DMS-PSO | DCS-PSO |
{f_1} | 1 | Mean | 1.28 × 10−39 | 3.42 × 10−14 | 3.82 | 3.83 × 10−13 | 6.76 × 10−28 | 1.05 × 10−40 |
S.D. | 2.48 × 10−39 | 1.20 × 10−13 | 2.18× 10+01 | 9.48 × 10−13 | 5.49 × 10−28 | 2.01 × 10−40 | ||
Rank | 2 | 4 | 6 | 5 | 3 | 1 | ||
{f_2} | 1 | Mean | 1.67 × 10−09 | 1.27 × 10−08 | 1.83 | 4.45 × 10−08 | 3.74 × 10−13 | 2.49 × 10−23 |
S.D. | 1.15 × 10−08 | 2.46 × 10−08 | 1.83 | 2.84 × 10−08 | 2.33 × 10−13 | 5.70 × 10−23 | ||
Rank | 3 | 4 | 6 | 5 | 2 | 1 | ||
{f_3} | 1 | Mean | 6.50 × 10−01 | 9.58 × 10+01 | 4.79× 10+02 | 4.37 × 10−04 | 1.16 × 10+01 | 5.90 |
S.D. | 5.22 × 10−01 | 5.10 × 10+01 | 3.08× 10+02 | 3.04 × 10−04 | 5.95 | 4.45 | ||
Rank | 2 | 5 | 6 | 1 | 4 | 3 | ||
{f_4} | 1 | Mean | 2.98× 10+01 | 4.17 × 10+01 | 9.42× 10+01 | 1.81 × 10 +01 | 2.26 × 10+01 | 2.21 × 10+01 |
S.D. | 2.16× 10+01 | 2.87 × 10+01 | 5.46× 10+01 | 3.80 | 2.29 | 6.32 × 10−01 | ||
Rank | 4 | 5 | 6 | 1 | 3 | 2 | ||
{f_5} | 1 | Mean | 8.74 × 10−03 | 1.89 × 10−02 | 8.00 × 10−02 | 3.83 × 10−02 | 9.99 × 10−03 | 5.12 × 10−03 |
S.D. | 3.28 × 10−03 | 6.58 × 10−03 | 3.51 × 10−02 | 1.47 × 10−02 | 3.98 × 10−03 | 1.69 × 10−03 | ||
Rank | 2 | 4 | 6 | 5 | 3 | 1 | ||
{f_6} | 1 | Mean | 3.88 × 10+01 | 3.34 × 10+01 | 5.12 × 10+01 | 4.40 × 10−11 | 2.73 × 10+01 | 1.31 × 10+02 |
S.D. | 8.06 | 8.19 | 1.62 × 10+01 | 6.58 × 10−11 | 8.88 × 10+00 | 1.01 × 10+01 | ||
Rank | 4 | 3 | 5 | 1 | 2 | 6 | ||
{f_7} | 1 | Mean | 3.81 × 10−01 | 1.14 × 10−06 | 4.32 | 3.15 × 10−03 | 4.37 × 10−14 | 1.20 × 10−14 |
S.D. | 6.47 × 10−01 | 1.04 × 10−06 | 1.16 | 2.18 × 10−02 | 4.86 × 10−14 | 3.90 × 10−15 | ||
Rank | 5 | 3 | 6 | 4 | 2 | 1 | ||
{f_8} | 0 | Mean | 1.46 × 10−02 | 1.38 × 10−02 | 4.14 × 10−01 | 1.41 × 10−12 | 4.44 × 10−03 | 1.15 × 10−02 |
S.D. | 1.70 × 10−02 | 1.55 × 10−02 | 3.12 × 10−01 | 2.52 × 10−12 | 4.99 × 10−03 | 1.40 × 10−02 | ||
Rank | 5 | 4 | 6 | 1 | 2 | 3 | ||
{f_9} | 0 | Mean | 1.39 × 10−01 | 1.04 × 10−02 | 3.87 | 2.32 × 10−14 | 1.08 × 10−26 | 2.96 × 10−02 |
S.D. | 2.62 × 10−01 | 3.14 × 10−02 | 2.66 | 5.72 × 10−14 | 2.24 × 10−26 | 4.74 × 10−02 | ||
Rank | 4 | 3 | 6 | 2 | 1 | 4 | ||
{f_{10}} | 1 | Mean | 2.28 × 10−01 | 1.10 × 10−02 | 2.16× 10+01 | 1.03 × 10−11 | 5.31 × 10−03 | 6.22 × 10−32 |
S.D. | 6.63 × 10−01 | 4.43 × 10−02 | 1.12× 10+01 | 1.45 × 10−11 | 4.13 × 10−04 | 7.45 × 10−32 | ||
Rank | 5 | 4 | 6 | 2 | 3 | 1 | ||
Ave. rank | 3.6 | 3.9 | 5.9 | 2.7 | 2.5 | 2.3 | ||
Final rank | 4 | 5 | 6 | 3 | 2 | 1 |
Function | k | Criteria | PSO-w | GPSO | LPSO | CLPSO | DMS-PSO | DCS-PSO |
{f_1} | 1 | Mean | 5.20 × 10−17 | 3.68 × 10−04 | 1.30 × 10+03 | 5.70 × 10−05 | 1.26 × 10−06 | 3.93 × 10−18 |
S.D. | 1.91 × 10−16 | 4.22 × 10−04 | 4.91 × 10+02 | 6.50 × 10−05 | 3.14 × 10−06 | 1.05 × 10−17 | ||
{f_2} | 0 | Mean | 9.06 × 10−06 | 2.01 × 10−01 | 2.26 × 10+01 | 2.00 × 10−01 | 1.48 × 10−04 | 5.87 × 10−07 |
S.D. | 5.83 × 10−05 | 1.41 | 4.26 | 1.41 | 6.99 × 10−04 | 2.82 × 10−06 | ||
{f_3} | 1 | Mean | 4.52 × 10+02 | 4.38 × 10+03 | 7.71 × 10+03 | 1.55 | 1.90 × 10+03 | 1.33 × 10+03 |
S.D. | 1.68 × 10+02 | 1.88× 10+03 | 2.11 × 10+03 | 9.59 × 10−01 | 2.30 × 10+03 | 5.06× 10+02 | ||
{f_4} | 1 | Mean | 7.59 × 10+01 | 1.34× 10+02 | 3.19 × 10+03 | 1.69 × 10+02 | 1.46 × 10+02 | 4.52 × 10−02 |
S.D. | 4.33 × 10+01 | 1.52× 10+02 | 1.65 × 10+03 | 2.75 × 10+02 | 1.41 × 10+02 | 2.98 × 10−02 | ||
{f_5} | 1 | Mean | 3.75 × 10−02 | 7.50 × 10−02 | 9.04 × 10−01 | 1.72 × 10−01 | 4.52 × 10−02 | 1.85 × 10−02 |
S.D. | 1.12 × 10−02 | 2.09 × 10−02 | 3.67 × 10−01 | 4.36 × 10−02 | 1.67 × 10−02 | 6.22 × 10−03 | ||
{f_6} | 1 | Mean | 7.48 × 10+01 | 8.78× 10+01 | 1.16 × 10+02 | 1.32 × 10−03 | 7.67 × 10+01 | 4.79 × 10+01 |
S.D. | 1.62 × 10+01 | 1.83× 10+01 | 2.04 × 10+01 | 6.87 × 10−03 | 1.60 × 10+01 | 3.18 | ||
{f_7} | 0 | Mean | 1.38 | 1.40 × 10−01 | 9.00 | 1.22 | 1.14 | 9.05 × 10−01 |
S.D. | 8.89 × 10−01 | 3.52 × 10−01 | 1.08 | 5.83 × 10−01 | 7.77 × 10−01 | 8.47 × 10−01 | ||
{f_8} | 0 | Mean | 1.36 × 10−02 | 8.18 × 10−03 | 1.22 × 10+01 | 3.18 × 10−04 | 3.79 × 10−02 | 1.43 × 10−02 |
S.D. | 2.61 × 10−02 | 1.02 × 10−02 | 4.71 | 1.41 × 10−03 | 7.54 × 10−02 | 1.76 × 10−02 | ||
{f_9} | 0 | Mean | 1.60 × 10−01 | 1.04 × 10−01 | 2.08 × 10+01 | 8.95 × 10−02 | 7.84 × 10−08 | 2.99 × 10−02 |
S.D. | 2.65 × 10−01 | 1.96 × 10−01 | 8.57 | 1.44 × 10−01 | 4.22 × 10−07 | 4.91 × 10−02 | ||
{f_{10}} | 1 | Mean | 4.07 | 9.60 × 10−01 | 4.06 × 10+02 | 1.35 | 8.78 | 3.53 × 10−01 |
S.D. | 3.36 | 1.12 | 3.10 × 10+02 | 1.87 | 6.03 | 3.69 × 10−01 |
Function | Criteria | PSO-w | GPSO | LPSO | CLPSO | DMS-PSO | DCS-PSO |
F1 | Mean | 5.73 × 10+08 | 9.15 × 10+09 | 5.24 × 10+09 | 1.01 × 10+02 | 3.26 × 10+03 | 6.44 × 10+03 |
S.D. | 2.36 × 10+09 | 1.29 × 10+10 | 9.65 × 10+09 | 5.12 × 10−01 | 4.53 × 10+03 | 1.93 × 10+03 | |
F3 | Mean | 2.52 × 10+03 | 5.01 × 10+03 | 9.58 × 10+03 | 4.90 × 10+02 | 2.16 × 10+02 | 5.45 × 10+02 |
S.D. | 1.23 × 10+03 | 2.38 × 10+03 | 5.11 × 10+03 | 1.39 × 10+01 | 3.43 × 10+02 | 3.90 × 10−13 | |
F4 | Mean | 1.03 × 10+02 | 1.73 × 10+02 | 2.15 × 10+02 | 5.53 × 10+02 | 7.39 × 10+01 | 1.22 |
S.D. | 3.19 × 10+01 | 1.02 × 10+02 | 1.92 × 10+02 | 7.54 | 2.39 × 10+01 | 1.75 | |
F5 | Mean | 1.43 × 10+02 | 7.61 × 10+01 | 1.42 × 10+02 | 6.00 × 10+02 | 5.88 × 10+01 | 2.09 × 10+01 |
S.D. | 3.11 × 10+01 | 2.08 × 10+01 | 3.78 × 10+01 | 0.00 | 1.30 × 10+01 | 7.01 | |
F6 | Mean | 4.01 × 10+01 | 1.43 × 10+01 | 5.14 × 10+01 | 7.87 × 10+02 | 9.86 × 10−04 | 3.75 |
S.D. | 1.49 × 10+01 | 5.47 | 1.39 × 10+01 | 6.43 | 2.47 × 10−03 | 3.17 | |
F7 | Mean | 1.29 × 10+02 | 1.12 × 10+01 | 1.91 × 10+02 | 8.49 × 10+02 | 9.76 × 10+01 | 1.28 × 10+02 |
S.D. | 3.46 × 10+01 | 1.03 × 10+01 | 4.86 × 10+01 | 1.03 × 10+01 | 1.99 × 10+01 | 9.23 | |
F8 | Mean | 1.20 × 10+02 | 6.83 × 10+01 | 1.24 × 10+02 | 9.09 × 10+02 | 5.94 × 10+01 | 1.62 × 10+01 |
S.D. | 3.18 × 10+01 | 1.66 × 10+01 | 2.95 × 10+01 | 3.05 | 1.68 × 10+01 | 5.52 | |
F9 | Mean | 1.38 × 10+03 | 1.53 × 10+02 | 2.26 × 10+03 | 3.27 × 10+03 | 2.33 × 10+01 | 6.09 × 10−01 |
S.D. | 1.18 × 10+03 | 1.64 × 10+02 | 9.36 × 10+02 | 2.89 × 10+02 | 5.63 × 10+01 | 1.83 | |
F10 | Mean | 3.61 × 10+03 | 3.19 × 10+03 | 3.91 × 10+03 | 1.18 × 10+03 | 2.97 × 10+03 | 1.99 × 10+03 |
S.D. | 5.67 × 10+02 | 6.28 × 10+02 | 7.81 × 10+02 | 4.51 | 5.89 × 10+02 | 2.87 × 10+02 | |
F11 | Mean | 1.30 × 10+02 | 1.71 × 10+02 | 1.76 × 10+02 | 5.97 × 10+05 | 4.74 × 10+01 | 1.12 × 10+02 |
S.D. | 4.18 × 10+01 | 5.41 × 10+01 | 5.11 × 10+01 | 3.96 × 10+05 | 3.12 × 10+01 | 2.44 × 10+01 | |
F12 | Mean | 4.56 × 10+07 | 6.40 × 10+07 | 1.76 × 10+08 | 2.62 × 10+03 | 8.25 × 10+04 | 2.79 × 10+03 |
S.D. | 2.83 × 10+08 | 1.12 × 10+08 | 5.00 × 10+08 | 1.55 × 10+03 | 2.16 × 10+04 | 2.07 × 10+03 | |
F13 | Mean | 1.77 × 10+06 | 5.01 × 10+07 | 1.88 × 10+07 | 1.75 × 10+04 | 1.55 × 10+04 | 1.27 × 10+04 |
S.D. | 8.67 × 10+06 | 1.71 × 10+08 | 1.02 × 10+08 | 9.65 × 10+03 | 1.65 × 10+04 | 3.86 × 10+03 | |
F14 | Mean | 1.80 × 10+04 | 3.70 × 10+04 | 1.10 × 10+04 | 1.61 × 10+03 | 1.32 × 10+04 | 1.02 × 10+02 |
S.D. | 2.36 × 10+04 | 4.40 × 10+04 | 1.30 × 10+04 | 2.70 × 10+01 | 1.23 × 10+04 | 2.45 × 10+01 | |
F15 | Mean | 3.99 × 10+03 | 1.97 × 10+04 | 1.07 × 10+04 | 2.16 × 10+03 | 6.90 × 10+03 | 1.09 × 10+02 |
S.D. | 3.96 × 10+03 | 3.05 × 10+04 | 1.25 × 10+04 | 1.32 × 10+02 | 9.16 × 10+03 | 1.14 × 10+02 | |
F16 | Mean | 1.04 × 10+03 | 7.61 × 10+02 | 1.13 × 10+03 | 1.84 × 10+03 | 7.95 × 10+02 | 1.79 × 10+03 |
S.D. | 2.73 × 10+02 | 2.47 × 10+02 | 2.95 × 10+02 | 4.18 × 10+01 | 2.10 × 10+02 | 1.25 × 10+02 | |
F17 | Mean | 4.00 × 10+04 | 1.57 × 10+04 | 1.21 × 10+04 | 2.16 × 10+05 | 1.89 × 10+02 | 4.62 × 10+03 |
S.D. | 6.54 × 10+02 | 1.54 × 10+03 | 2.56 × 10+03 | 1.61 × 10+05 | 1.14 × 10+02 | 6.54 × 10+02 | |
F18 | Mean | 1.41 × 10+05 | 4.45 × 10+05 | 1.12 × 10+05 | 1.95 × 10+03 | 1.27 × 10+05 | 5.56 × 10+02 |
S.D. | 1.04 × 10+05 | 3.62 × 10+05 | 1.15 × 10+05 | 1.88 × 10+01 | 1.06 × 10+05 | 8.59 × 10+02 | |
F19 | Mean | 6.20 × 10+03 | 4.47 × 10+05 | 6.10 × 10+03 | 6.30 × 10+03 | 9.39 × 10+03 | 7.78 × 10+03 |
S.D. | 6.09 × 10+03 | 2.71 × 10+06 | 6.37 × 10+03 | 2.64 × 10+01 | 1.28 × 10+04 | 1.08 × 10+03 | |
F20 | Mean | 1.70 × 10+04 | 1.45 × 10+04 | 1.92 × 10+04 | 2.36 × 10+03 | 2.73 × 10+02 | 6.00 × 10+03 |
S.D. | 3.26 × 10+03 | 3.44 × 10+02 | 2.75 × 10+03 | 5.04 | 1.41 × 10+02 | 5.32 × 10+02 | |
F21 | Mean | 3.39 × 10+02 | 2.80 × 10+02 | 3.34 × 10+02 | 2.65 × 10+03 | 2.62 × 10+02 | 1.03 × 10+02 |
S.D. | 5.45 × 10+01 | 4.25 × 10+01 | 4.90 × 10+01 | 5.40 × 10+02 | 2.11 × 10+01 | 8.18 × 10−01 | |
F22 | Mean | 4.15 × 10+02 | 6.72 × 10+02 | 1.00 × 10+03 | 2.72 × 10+03 | 2.38 × 10+02 | 1.09 × 10+02 |
S.D. | 9.94 × 10+02 | 7.48 × 10+02 | 1.50 × 10+03 | 1.15 × 10+01 | 5.92 × 10+02 | 1.44 | |
F23 | Mean | 6.42 × 10+02 | 5.82 × 10+02 | 6.73 × 10+02 | 2.92 × 10+03 | 4.30 × 10+02 | 3.25 × 10+02 |
S.D. | 1.01 × 10+02 | 9.36 × 10+01 | 1.10 × 10+02 | 1.21 × 10+01 | 2.10 × 10+01 | 7.91 | |
F24 | Mean | 6.61 × 10+02 | 6.34 × 10+02 | 7.14 × 10+02 | 2.89 × 10+03 | 4.94 × 10+02 | 3.53 × 10+02 |
S.D. | 7.19 × 10+01 | 8.33 × 10+01 | 8.07 × 10+01 | 2.23 × 10−01 | 2.60 × 10+01 | 1.09 × 10+01 | |
F25 | Mean | 3.95 × 10+02 | 4.13 × 10+02 | 4.47 × 10+02 | 3.47 × 10+03 | 3.97 × 10+02 | 4.26 × 10+02 |
S.D. | 1.50 × 10+01 | 3.64 × 10+01 | 3.05 × 10+01 | 7.89 × 10+02 | 1.53 | 2.34 × 10+01 | |
F26 | Mean | 1.24 × 10+03 | 1.51 × 10+03 | 2.45 × 10+03 | 3.21 × 10+03 | 1.49 × 10+03 | 4.02 × 10+02 |
S.D. | 1.29 × 10+03 | 7.71 × 10+02 | 1.36 × 10+03 | 6.70 | 5.18 × 10+02 | 5.94 × 10+01 | |
F27 | Mean | 5.88 × 10+02 | 5.80 × 10+02 | 6.21 × 10+02 | 3.21 × 10+03 | 5.38 × 10+02 | 4.03 × 10+02 |
S.D. | 5.87 × 10+01 | 5.54 × 10+01 | 5.36 × 10+01 | 5.65 | 1.25 × 10+01 | 4.45 | |
F28 | Mean | 4.34 × 10+02 | 5.05 × 10+02 | 5.49 × 10+02 | 3.45 × 10+03 | 3.40 × 10+02 | 3.88 × 10+02 |
S.D. | 4.76 × 10+01 | 7.23 × 10+01 | 1.46 × 10+02 | 4.85 | 5.79 × 10+01 | 8.36 | |
F29 | Mean | 6.25 × 10+02 | 8.71 × 10+02 | 6.24 × 10+02 | 8.91 × 10+02 | 6.03 × 10+02 | 6.38 × 10+02 |
S.D. | 4.87 × 10+01 | 6.31 × 10+01 | 2.17 × 10+02 | 7.46 × 10+01 | 1.55 × 10+02 | 7.88 × 10+01 | |
F30 | Mean | 4.52 × 10+04 | 4.50 × 10+05 | 3.78 × 10+05 | 1.12 × 10+03 | 5.81 × 10+03 | 3.14 × 10+05 |
S.D. | 1.10 × 10+05 | 1.41 × 10+06 | 4.93 × 10+05 | 2.22 × 10+03 | 2.34 × 10+03 | 5.14 × 10+05 |
Average rank | Algorithm | Ranking |
1 | DCS-PSO | 2.03 |
2 | DMS-PSO | 2.17 |
3 | PSO-w | 3.69 |
4 | GPSO | 4.07 |
5 | CLPSO | 4.45 |
6 | LPSO | 4.59 |
PC configuration | Information |
CPU | Inter(R) Core(TM) i7-8700 |
Frequency | 3.2GHz CPU |
RAM | 16.0GB |
Operating system | Windows 10(64Bit) |
Language | Python 3.9.13 |
Algorithm | Parameters |
COA | \max \_iter1 = {10^5}, {\text{ }}\max \_iter2 = 1.5 \times {10^5}, {\text{ }}\alpha = 0.3 |
DCOA | h = 3000, {\text{ }}\gamma = 0.25, {\text{ }}\xi {\text{ = 1}}{\text{.5}} |
PCOA | n\_group = 3, {\text{ }}\max \_iter1 = {10^5}, {\text{ }}\max \_iter2 = 1.5 \times {10^5}, {\text{ }}\alpha = 0.3 |
DCS-PSO | N = 20, {\text{ }}w = 0.4, {\text{ }}c1 = c2 = 2, {\text{ }}{V_{\max }} = 0.2 \times Range, {\text{ }}\max \_iter = 1000 |
Function | Criteria | COA | DCOA | PCOA | DCS-PSO |
{f_J} | E.T. | 30.8898 | 0.5753 | 17.2234 | 0.2793 |
Mean | 0.0000 | 0.0005 | 0.0000 | 0.0000 | |
S.D. | 0.0000 | 0.0014 | 0.0000 | 0.0000 | |
{f_C} | E.T. | 7.6564 | 0.6478 | 10.3990 | 0.3610 |
Mean | −1.0316 | −1.0312 | −1.0316 | −1.0316 | |
S.D. | 0.0000 | 0.0012 | 0.0000 | 0.0000 | |
{f_G} | E.T. | 14.1867 | 1.0656 | 14.0814 | 0.6097 |
Mean | 3.0022 | 3.0100 | 3.0024 | 3.0000 | |
S.D. | 0.0022 | 0.0126 | 0.0022 | 0.0000 | |
{f_B} | E.T. | 1.5663 | 0.8653 | 5.7455 | 0.4513 |
Mean | 0.3983 | 0.4003 | 0.3980 | 0.3979 | |
S.D. | 0.0003 | 0.0039 | 0.0001 | 0.0000 | |
{f_R} | E.T. | 4.4150 | 0.6652 | 5.8342 | 0.4366 |
Mean | −1.9993 | −1.9652 | −1.9992 | −2.0000 | |
S.D. | 0.0006 | 0.0558 | 0.0008 | 0.0000 | |
{f_S} | E.T. | 5.8609 | 2.1743 | 13.8397 | 2.0615 |
Mean | −186.6428 | -186.4517 | −186.6861 | −186.7309 | |
S.D. | 0.0711 | 0.4203 | 0.0680 | 0.0000 |
Name | Functions | Range | Optimum |
Sphere | {f_1}\left(\boldsymbol x \right) = \sum\limits_{i = 1}^D {x_i^2} | [−100, 100] | 0 |
Schwefel's 2.22 | {f_2}\left(\boldsymbol x \right) = \sum\limits_{i = 1}^D {|{x_i}|} + \prod\limits_{i = 1}^D {|{x_i}|} | [−10, 10] | 0 |
Schwefel's 1.2 | {f_3}\left(\boldsymbol x \right) = \sum\limits_{i = 1}^D {{{\left({\sum\limits_{j = 1}^i {{x_j}} } \right)}^2}} | [−100, 100] | 0 |
Rosenbrock | {f_4}\left(\boldsymbol x \right) = \sum\limits_{i = 1}^{D - 1} {\left[{100{{\left({{x_{i + 1}} - x_i^2} \right)}^2} + {{\left({{x_i} - 1} \right)}^2}} \right]} | [−10, 10] | 0 |
Noise | {f_5}\left(\boldsymbol x \right) = \sum\limits_{i = 1}^D {ix_i^4} + random[0, 1) | [−1.28, 1.28] | 0 |
Rastrigin | {f_6}\left(\boldsymbol x \right) = \sum\limits_{i = 1}^D {\left({x_i^2 - 10\cos \left({2\pi {x_i}} \right) + 10} \right)} | [−5.12, 5.12] | 0 |
Ackley | {f_7}\left(\boldsymbol x \right) = - 20\exp \left({ - 0.2\sqrt {\frac{1}{D}\sum\limits_{i = 1}^D {x_i^2} } } \right) - \exp \left({\frac{1}{D}\sum\limits_{i = 1}^D {\cos \left({2\pi {x_i}} \right)} } \right) + 20 + e | [−32, 32] | 0 |
Griewank | {f_8}\left(\boldsymbol x \right) = \frac{1}{{4000}}\sum\limits_{i = 1}^D {x_i^2} - \prod\limits_{i = 1}^D {\cos \left({\frac{{{x_i}}}{{\sqrt i }}} \right)} + 1 | [−600, 600] | 0 |
Penalized 1 | \begin{array}{l} {f_9}\left(\boldsymbol x \right) = \frac{\pi }{D}\left\{ {10{{\sin }^2}\left({\pi {y_1}} \right) + \sum\limits_{i = 1}^{D - 1} {{{\left({{y_i} - 1} \right)}^2}} \left[{1 + 10{{\sin }^2}\left({\pi {y_{i + 1}}} \right)} \right] + {{\left({{y_D} - 1} \right)}^2}} \right\} \\ + \sum\limits_{i = 1}^D {u\left({{x_i}, 10, 100, 4} \right)} \\ \end{array} | [−50, 50] | 0 |
Penalized 2 | \begin{array}{l} {f_{10}}\left(\boldsymbol x \right) = \frac{1}{{10}}\left\{ {{{\sin }^2}\left({3\pi {x_1}} \right) + \sum\limits_{i = 1}^D {{{\left({{x_i} - 1} \right)}^2}} \left[{1 + {{\sin }^2}\left({3\pi {x_i} + 1} \right)} \right] + {{\left({{x_D} - 1} \right)}^2}\left[{1 + {{\sin }^2}\left({2\pi {x_D}} \right)} \right]} \right\} \\ + \sum\limits_{i = 1}^D {u\left({{x_i}, 5, 100, 4} \right)} \\ \end{array} | [−50, 50] | 0 |
Algorithm | Parameters |
PSO-w | w:0.4, {\text{ }}{c_1} = {c_2} = 2.0, {\text{ }}{V_{\max }} = 0.2 \times Range |
GPSO | w:0.9 \sim 0.4, {\text{ }}{c_1} = {c_2} = 2.0, {\text{ }}{V_{\max }} = 0.2 \times Range |
LPSO | \chi = 0.7298, {\text{ }}{c_1} = {c_2} = 1.49445, {\text{ }}{V_{\max }} = 0.2 \times Range |
CLPSO | w:0.9\sim 0.4, {\text{ }}c = 1.49445, {\text{ }}{V_{\max }} = 0.2 \times Range, {\text{ }}m = 7 |
DMS-PSO | \chi = 0.7298, {\text{ }}{c_1} = {c_2} = 1.49445, {\text{ }}{V_{\max }} = 0.2 \times Range, {\text{ }}M = 4, {\text{ }}R = 10 |
DCS-PSO | w:0.4, {c_1} = {c_2} = 2.0, {V_{\max }} = 0.2 \times Range, {\text{ }}h = 3000, \gamma = 0.15, \xi = 1.5 |
Function | k | Criteria | PSO-w | GPSO | LPSO | CLPSO | DMS-PSO | DCS-PSO |
{f_1} | 1 | Mean | 1.84 × 10−144 | 1.47 × 10−73 | 2.78 × 10−169 | 2.18 × 10−21 | 6.30 × 10−62 | 2.35 × 10−151 |
S.D. | 9.13 × 10−144 | 1.04 × 10−72 | 0.00 | 5.44 × 10−21 | 4.25 × 10−61 | 7.75 × 10−151 | ||
{f_2} | 1 | Mean | 1.89 × 10−81 | 6.24 × 10−38 | 7.59 × 10−09 | 4.30 × 10−14 | 3.45 × 10−20 | 9.65 × 10−84 |
S.D. | 1.26 × 10−80 | 2.99 × 10−37 | 3.80 × 10−08 | 1.39 × 10−13 | 5.86 × 10−20 | 4.81 × 10−83 | ||
{f_3} | 1 | Mean | 1.22 × 10−44 | 9.93 × 10−17 | 6.11 × 10−13 | 2.18 × 10−20 | 6.13 × 10−10 | 1.21 × 10−48 |
S.D. | 7.42 × 10−44 | 3.36 × 10−16 | 4.32 × 10−12 | 3.25 × 10−20 | 3.33 × 10−09 | 2.38 × 10−48 | ||
{f_4} | 1 | Mean | 2.79 | 2.80 | 4.00 | 2.49 | 1.60 | 9.11 |
S.D. | 1.39 | 1.16 | 2.56 | 4.95 × 10−01 | 9.79 × 10−01 | 1.89 | ||
{f_5} | 1 | Mean | 7.23 × 10−04 | 1.02 × 10−03 | 3.25 × 10−03 | 1.49 × 10−03 | 8.80 × 10−04 | 2.15 × 10−04 |
S.D. | 3.90 × 10−04 | 5.46 × 10−04 | 2.43 × 10−03 | 7.09 × 10−04 | 4.49 × 10−04 | 1.58 × 10−04 | ||
{f_6} | 0 | Mean | 5.99 | 2.13 | 9.35 | 0.00 | 2.67 | 0.00 |
S.D. | 2.74 | 1.15 | 3.53 | 0.00 | 1.36 | 0.00 | ||
{f_7} | 0 | Mean | 4.14 × 10−15 | 4.07 × 10−15 | 4.67 × 10−15 | 3.16 × 10−14 | 4.00 × 10−15 | 3.91 × 10−15 |
S.D. | 7.03 × 10−16 | 5.02 × 10−16 | 1.62 × 10−15 | 5.29 × 10−14 | 0.00 | 5.62 × 10−16 | ||
{f_8} | 1 | Mean | 7.44 × 10−02 | 6.88 × 10−02 | 1.06 × 10−01 | 1.64 × 10−03 | 7.12 × 10−02 | 6.65 × 10−02 |
S.D. | 3.41 × 10−02 | 3.13 × 10−02 | 5.81 × 10−02 | 7.61 × 10−04 | 3.55 × 10−02 | 3.75 × 10−02 | ||
{f_9} | 1 | Mean | 1.24 × 10−02 | 4.71 × 10−32 | 6.22 × 10−03 | 2.99 × 10−30 | 1.38 × 10−28 | 1.78 × 10−01 |
S.D. | 6.16 × 10−02 | 1.11 × 10−47 | 4.40 × 10−02 | 3.07 × 10−30 | 2.65 × 10−28 | 6.11 × 10−02 | ||
{f_{10}} | 1 | Mean | 3.56 × 10−04 | 9.33 × 10−20 | 9.80 × 10−04 | 3.16 × 10−27 | 2.57 × 10−13 | 1.35 × 10−32 |
S.D. | 2.52 × 10−03 | 1.90 × 10−19 | 6.86 × 10−03 | 6.79 × 10−27 | 4.33 × 10−13 | 8.29 × 10−48 |
Function | k | Criteria | PSO-w | GPSO | LPSO | CLPSO | DMS-PSO | DCS-PSO |
{f_1} | 1 | Mean | 1.28 × 10−39 | 3.42 × 10−14 | 3.82 | 3.83 × 10−13 | 6.76 × 10−28 | 1.05 × 10−40 |
S.D. | 2.48 × 10−39 | 1.20 × 10−13 | 2.18× 10+01 | 9.48 × 10−13 | 5.49 × 10−28 | 2.01 × 10−40 | ||
Rank | 2 | 4 | 6 | 5 | 3 | 1 | ||
{f_2} | 1 | Mean | 1.67 × 10−09 | 1.27 × 10−08 | 1.83 | 4.45 × 10−08 | 3.74 × 10−13 | 2.49 × 10−23 |
S.D. | 1.15 × 10−08 | 2.46 × 10−08 | 1.83 | 2.84 × 10−08 | 2.33 × 10−13 | 5.70 × 10−23 | ||
Rank | 3 | 4 | 6 | 5 | 2 | 1 | ||
{f_3} | 1 | Mean | 6.50 × 10−01 | 9.58 × 10+01 | 4.79× 10+02 | 4.37 × 10−04 | 1.16 × 10+01 | 5.90 |
S.D. | 5.22 × 10−01 | 5.10 × 10+01 | 3.08× 10+02 | 3.04 × 10−04 | 5.95 | 4.45 | ||
Rank | 2 | 5 | 6 | 1 | 4 | 3 | ||
{f_4} | 1 | Mean | 2.98× 10+01 | 4.17 × 10+01 | 9.42× 10+01 | 1.81 × 10 +01 | 2.26 × 10+01 | 2.21 × 10+01 |
S.D. | 2.16× 10+01 | 2.87 × 10+01 | 5.46× 10+01 | 3.80 | 2.29 | 6.32 × 10−01 | ||
Rank | 4 | 5 | 6 | 1 | 3 | 2 | ||
{f_5} | 1 | Mean | 8.74 × 10−03 | 1.89 × 10−02 | 8.00 × 10−02 | 3.83 × 10−02 | 9.99 × 10−03 | 5.12 × 10−03 |
S.D. | 3.28 × 10−03 | 6.58 × 10−03 | 3.51 × 10−02 | 1.47 × 10−02 | 3.98 × 10−03 | 1.69 × 10−03 | ||
Rank | 2 | 4 | 6 | 5 | 3 | 1 | ||
{f_6} | 1 | Mean | 3.88 × 10+01 | 3.34 × 10+01 | 5.12 × 10+01 | 4.40 × 10−11 | 2.73 × 10+01 | 1.31 × 10+02 |
S.D. | 8.06 | 8.19 | 1.62 × 10+01 | 6.58 × 10−11 | 8.88 × 10+00 | 1.01 × 10+01 | ||
Rank | 4 | 3 | 5 | 1 | 2 | 6 | ||
{f_7} | 1 | Mean | 3.81 × 10−01 | 1.14 × 10−06 | 4.32 | 3.15 × 10−03 | 4.37 × 10−14 | 1.20 × 10−14 |
S.D. | 6.47 × 10−01 | 1.04 × 10−06 | 1.16 | 2.18 × 10−02 | 4.86 × 10−14 | 3.90 × 10−15 | ||
Rank | 5 | 3 | 6 | 4 | 2 | 1 | ||
{f_8} | 0 | Mean | 1.46 × 10−02 | 1.38 × 10−02 | 4.14 × 10−01 | 1.41 × 10−12 | 4.44 × 10−03 | 1.15 × 10−02 |
S.D. | 1.70 × 10−02 | 1.55 × 10−02 | 3.12 × 10−01 | 2.52 × 10−12 | 4.99 × 10−03 | 1.40 × 10−02 | ||
Rank | 5 | 4 | 6 | 1 | 2 | 3 | ||
{f_9} | 0 | Mean | 1.39 × 10−01 | 1.04 × 10−02 | 3.87 | 2.32 × 10−14 | 1.08 × 10−26 | 2.96 × 10−02 |
S.D. | 2.62 × 10−01 | 3.14 × 10−02 | 2.66 | 5.72 × 10−14 | 2.24 × 10−26 | 4.74 × 10−02 | ||
Rank | 4 | 3 | 6 | 2 | 1 | 4 | ||
{f_{10}} | 1 | Mean | 2.28 × 10−01 | 1.10 × 10−02 | 2.16× 10+01 | 1.03 × 10−11 | 5.31 × 10−03 | 6.22 × 10−32 |
S.D. | 6.63 × 10−01 | 4.43 × 10−02 | 1.12× 10+01 | 1.45 × 10−11 | 4.13 × 10−04 | 7.45 × 10−32 | ||
Rank | 5 | 4 | 6 | 2 | 3 | 1 | ||
Ave. rank | 3.6 | 3.9 | 5.9 | 2.7 | 2.5 | 2.3 | ||
Final rank | 4 | 5 | 6 | 3 | 2 | 1 |
Function | k | Criteria | PSO-w | GPSO | LPSO | CLPSO | DMS-PSO | DCS-PSO |
{f_1} | 1 | Mean | 5.20 × 10−17 | 3.68 × 10−04 | 1.30 × 10+03 | 5.70 × 10−05 | 1.26 × 10−06 | 3.93 × 10−18 |
S.D. | 1.91 × 10−16 | 4.22 × 10−04 | 4.91 × 10+02 | 6.50 × 10−05 | 3.14 × 10−06 | 1.05 × 10−17 | ||
{f_2} | 0 | Mean | 9.06 × 10−06 | 2.01 × 10−01 | 2.26 × 10+01 | 2.00 × 10−01 | 1.48 × 10−04 | 5.87 × 10−07 |
S.D. | 5.83 × 10−05 | 1.41 | 4.26 | 1.41 | 6.99 × 10−04 | 2.82 × 10−06 | ||
{f_3} | 1 | Mean | 4.52 × 10+02 | 4.38 × 10+03 | 7.71 × 10+03 | 1.55 | 1.90 × 10+03 | 1.33 × 10+03 |
S.D. | 1.68 × 10+02 | 1.88× 10+03 | 2.11 × 10+03 | 9.59 × 10−01 | 2.30 × 10+03 | 5.06× 10+02 | ||
{f_4} | 1 | Mean | 7.59 × 10+01 | 1.34× 10+02 | 3.19 × 10+03 | 1.69 × 10+02 | 1.46 × 10+02 | 4.52 × 10−02 |
S.D. | 4.33 × 10+01 | 1.52× 10+02 | 1.65 × 10+03 | 2.75 × 10+02 | 1.41 × 10+02 | 2.98 × 10−02 | ||
{f_5} | 1 | Mean | 3.75 × 10−02 | 7.50 × 10−02 | 9.04 × 10−01 | 1.72 × 10−01 | 4.52 × 10−02 | 1.85 × 10−02 |
S.D. | 1.12 × 10−02 | 2.09 × 10−02 | 3.67 × 10−01 | 4.36 × 10−02 | 1.67 × 10−02 | 6.22 × 10−03 | ||
{f_6} | 1 | Mean | 7.48 × 10+01 | 8.78× 10+01 | 1.16 × 10+02 | 1.32 × 10−03 | 7.67 × 10+01 | 4.79 × 10+01 |
S.D. | 1.62 × 10+01 | 1.83× 10+01 | 2.04 × 10+01 | 6.87 × 10−03 | 1.60 × 10+01 | 3.18 | ||
{f_7} | 0 | Mean | 1.38 | 1.40 × 10−01 | 9.00 | 1.22 | 1.14 | 9.05 × 10−01 |
S.D. | 8.89 × 10−01 | 3.52 × 10−01 | 1.08 | 5.83 × 10−01 | 7.77 × 10−01 | 8.47 × 10−01 | ||
{f_8} | 0 | Mean | 1.36 × 10−02 | 8.18 × 10−03 | 1.22 × 10+01 | 3.18 × 10−04 | 3.79 × 10−02 | 1.43 × 10−02 |
S.D. | 2.61 × 10−02 | 1.02 × 10−02 | 4.71 | 1.41 × 10−03 | 7.54 × 10−02 | 1.76 × 10−02 | ||
{f_9} | 0 | Mean | 1.60 × 10−01 | 1.04 × 10−01 | 2.08 × 10+01 | 8.95 × 10−02 | 7.84 × 10−08 | 2.99 × 10−02 |
S.D. | 2.65 × 10−01 | 1.96 × 10−01 | 8.57 | 1.44 × 10−01 | 4.22 × 10−07 | 4.91 × 10−02 | ||
{f_{10}} | 1 | Mean | 4.07 | 9.60 × 10−01 | 4.06 × 10+02 | 1.35 | 8.78 | 3.53 × 10−01 |
S.D. | 3.36 | 1.12 | 3.10 × 10+02 | 1.87 | 6.03 | 3.69 × 10−01 |
Function | Criteria | PSO-w | GPSO | LPSO | CLPSO | DMS-PSO | DCS-PSO |
F1 | Mean | 5.73 × 10+08 | 9.15 × 10+09 | 5.24 × 10+09 | 1.01 × 10+02 | 3.26 × 10+03 | 6.44 × 10+03 |
S.D. | 2.36 × 10+09 | 1.29 × 10+10 | 9.65 × 10+09 | 5.12 × 10−01 | 4.53 × 10+03 | 1.93 × 10+03 | |
F3 | Mean | 2.52 × 10+03 | 5.01 × 10+03 | 9.58 × 10+03 | 4.90 × 10+02 | 2.16 × 10+02 | 5.45 × 10+02 |
S.D. | 1.23 × 10+03 | 2.38 × 10+03 | 5.11 × 10+03 | 1.39 × 10+01 | 3.43 × 10+02 | 3.90 × 10−13 | |
F4 | Mean | 1.03 × 10+02 | 1.73 × 10+02 | 2.15 × 10+02 | 5.53 × 10+02 | 7.39 × 10+01 | 1.22 |
S.D. | 3.19 × 10+01 | 1.02 × 10+02 | 1.92 × 10+02 | 7.54 | 2.39 × 10+01 | 1.75 | |
F5 | Mean | 1.43 × 10+02 | 7.61 × 10+01 | 1.42 × 10+02 | 6.00 × 10+02 | 5.88 × 10+01 | 2.09 × 10+01 |
S.D. | 3.11 × 10+01 | 2.08 × 10+01 | 3.78 × 10+01 | 0.00 | 1.30 × 10+01 | 7.01 | |
F6 | Mean | 4.01 × 10+01 | 1.43 × 10+01 | 5.14 × 10+01 | 7.87 × 10+02 | 9.86 × 10−04 | 3.75 |
S.D. | 1.49 × 10+01 | 5.47 | 1.39 × 10+01 | 6.43 | 2.47 × 10−03 | 3.17 | |
F7 | Mean | 1.29 × 10+02 | 1.12 × 10+01 | 1.91 × 10+02 | 8.49 × 10+02 | 9.76 × 10+01 | 1.28 × 10+02 |
S.D. | 3.46 × 10+01 | 1.03 × 10+01 | 4.86 × 10+01 | 1.03 × 10+01 | 1.99 × 10+01 | 9.23 | |
F8 | Mean | 1.20 × 10+02 | 6.83 × 10+01 | 1.24 × 10+02 | 9.09 × 10+02 | 5.94 × 10+01 | 1.62 × 10+01 |
S.D. | 3.18 × 10+01 | 1.66 × 10+01 | 2.95 × 10+01 | 3.05 | 1.68 × 10+01 | 5.52 | |
F9 | Mean | 1.38 × 10+03 | 1.53 × 10+02 | 2.26 × 10+03 | 3.27 × 10+03 | 2.33 × 10+01 | 6.09 × 10−01 |
S.D. | 1.18 × 10+03 | 1.64 × 10+02 | 9.36 × 10+02 | 2.89 × 10+02 | 5.63 × 10+01 | 1.83 | |
F10 | Mean | 3.61 × 10+03 | 3.19 × 10+03 | 3.91 × 10+03 | 1.18 × 10+03 | 2.97 × 10+03 | 1.99 × 10+03 |
S.D. | 5.67 × 10+02 | 6.28 × 10+02 | 7.81 × 10+02 | 4.51 | 5.89 × 10+02 | 2.87 × 10+02 | |
F11 | Mean | 1.30 × 10+02 | 1.71 × 10+02 | 1.76 × 10+02 | 5.97 × 10+05 | 4.74 × 10+01 | 1.12 × 10+02 |
S.D. | 4.18 × 10+01 | 5.41 × 10+01 | 5.11 × 10+01 | 3.96 × 10+05 | 3.12 × 10+01 | 2.44 × 10+01 | |
F12 | Mean | 4.56 × 10+07 | 6.40 × 10+07 | 1.76 × 10+08 | 2.62 × 10+03 | 8.25 × 10+04 | 2.79 × 10+03 |
S.D. | 2.83 × 10+08 | 1.12 × 10+08 | 5.00 × 10+08 | 1.55 × 10+03 | 2.16 × 10+04 | 2.07 × 10+03 | |
F13 | Mean | 1.77 × 10+06 | 5.01 × 10+07 | 1.88 × 10+07 | 1.75 × 10+04 | 1.55 × 10+04 | 1.27 × 10+04 |
S.D. | 8.67 × 10+06 | 1.71 × 10+08 | 1.02 × 10+08 | 9.65 × 10+03 | 1.65 × 10+04 | 3.86 × 10+03 | |
F14 | Mean | 1.80 × 10+04 | 3.70 × 10+04 | 1.10 × 10+04 | 1.61 × 10+03 | 1.32 × 10+04 | 1.02 × 10+02 |
S.D. | 2.36 × 10+04 | 4.40 × 10+04 | 1.30 × 10+04 | 2.70 × 10+01 | 1.23 × 10+04 | 2.45 × 10+01 | |
F15 | Mean | 3.99 × 10+03 | 1.97 × 10+04 | 1.07 × 10+04 | 2.16 × 10+03 | 6.90 × 10+03 | 1.09 × 10+02 |
S.D. | 3.96 × 10+03 | 3.05 × 10+04 | 1.25 × 10+04 | 1.32 × 10+02 | 9.16 × 10+03 | 1.14 × 10+02 | |
F16 | Mean | 1.04 × 10+03 | 7.61 × 10+02 | 1.13 × 10+03 | 1.84 × 10+03 | 7.95 × 10+02 | 1.79 × 10+03 |
S.D. | 2.73 × 10+02 | 2.47 × 10+02 | 2.95 × 10+02 | 4.18 × 10+01 | 2.10 × 10+02 | 1.25 × 10+02 | |
F17 | Mean | 4.00 × 10+04 | 1.57 × 10+04 | 1.21 × 10+04 | 2.16 × 10+05 | 1.89 × 10+02 | 4.62 × 10+03 |
S.D. | 6.54 × 10+02 | 1.54 × 10+03 | 2.56 × 10+03 | 1.61 × 10+05 | 1.14 × 10+02 | 6.54 × 10+02 | |
F18 | Mean | 1.41 × 10+05 | 4.45 × 10+05 | 1.12 × 10+05 | 1.95 × 10+03 | 1.27 × 10+05 | 5.56 × 10+02 |
S.D. | 1.04 × 10+05 | 3.62 × 10+05 | 1.15 × 10+05 | 1.88 × 10+01 | 1.06 × 10+05 | 8.59 × 10+02 | |
F19 | Mean | 6.20 × 10+03 | 4.47 × 10+05 | 6.10 × 10+03 | 6.30 × 10+03 | 9.39 × 10+03 | 7.78 × 10+03 |
S.D. | 6.09 × 10+03 | 2.71 × 10+06 | 6.37 × 10+03 | 2.64 × 10+01 | 1.28 × 10+04 | 1.08 × 10+03 | |
F20 | Mean | 1.70 × 10+04 | 1.45 × 10+04 | 1.92 × 10+04 | 2.36 × 10+03 | 2.73 × 10+02 | 6.00 × 10+03 |
S.D. | 3.26 × 10+03 | 3.44 × 10+02 | 2.75 × 10+03 | 5.04 | 1.41 × 10+02 | 5.32 × 10+02 | |
F21 | Mean | 3.39 × 10+02 | 2.80 × 10+02 | 3.34 × 10+02 | 2.65 × 10+03 | 2.62 × 10+02 | 1.03 × 10+02 |
S.D. | 5.45 × 10+01 | 4.25 × 10+01 | 4.90 × 10+01 | 5.40 × 10+02 | 2.11 × 10+01 | 8.18 × 10−01 | |
F22 | Mean | 4.15 × 10+02 | 6.72 × 10+02 | 1.00 × 10+03 | 2.72 × 10+03 | 2.38 × 10+02 | 1.09 × 10+02 |
S.D. | 9.94 × 10+02 | 7.48 × 10+02 | 1.50 × 10+03 | 1.15 × 10+01 | 5.92 × 10+02 | 1.44 | |
F23 | Mean | 6.42 × 10+02 | 5.82 × 10+02 | 6.73 × 10+02 | 2.92 × 10+03 | 4.30 × 10+02 | 3.25 × 10+02 |
S.D. | 1.01 × 10+02 | 9.36 × 10+01 | 1.10 × 10+02 | 1.21 × 10+01 | 2.10 × 10+01 | 7.91 | |
F24 | Mean | 6.61 × 10+02 | 6.34 × 10+02 | 7.14 × 10+02 | 2.89 × 10+03 | 4.94 × 10+02 | 3.53 × 10+02 |
S.D. | 7.19 × 10+01 | 8.33 × 10+01 | 8.07 × 10+01 | 2.23 × 10−01 | 2.60 × 10+01 | 1.09 × 10+01 | |
F25 | Mean | 3.95 × 10+02 | 4.13 × 10+02 | 4.47 × 10+02 | 3.47 × 10+03 | 3.97 × 10+02 | 4.26 × 10+02 |
S.D. | 1.50 × 10+01 | 3.64 × 10+01 | 3.05 × 10+01 | 7.89 × 10+02 | 1.53 | 2.34 × 10+01 | |
F26 | Mean | 1.24 × 10+03 | 1.51 × 10+03 | 2.45 × 10+03 | 3.21 × 10+03 | 1.49 × 10+03 | 4.02 × 10+02 |
S.D. | 1.29 × 10+03 | 7.71 × 10+02 | 1.36 × 10+03 | 6.70 | 5.18 × 10+02 | 5.94 × 10+01 | |
F27 | Mean | 5.88 × 10+02 | 5.80 × 10+02 | 6.21 × 10+02 | 3.21 × 10+03 | 5.38 × 10+02 | 4.03 × 10+02 |
S.D. | 5.87 × 10+01 | 5.54 × 10+01 | 5.36 × 10+01 | 5.65 | 1.25 × 10+01 | 4.45 | |
F28 | Mean | 4.34 × 10+02 | 5.05 × 10+02 | 5.49 × 10+02 | 3.45 × 10+03 | 3.40 × 10+02 | 3.88 × 10+02 |
S.D. | 4.76 × 10+01 | 7.23 × 10+01 | 1.46 × 10+02 | 4.85 | 5.79 × 10+01 | 8.36 | |
F29 | Mean | 6.25 × 10+02 | 8.71 × 10+02 | 6.24 × 10+02 | 8.91 × 10+02 | 6.03 × 10+02 | 6.38 × 10+02 |
S.D. | 4.87 × 10+01 | 6.31 × 10+01 | 2.17 × 10+02 | 7.46 × 10+01 | 1.55 × 10+02 | 7.88 × 10+01 | |
F30 | Mean | 4.52 × 10+04 | 4.50 × 10+05 | 3.78 × 10+05 | 1.12 × 10+03 | 5.81 × 10+03 | 3.14 × 10+05 |
S.D. | 1.10 × 10+05 | 1.41 × 10+06 | 4.93 × 10+05 | 2.22 × 10+03 | 2.34 × 10+03 | 5.14 × 10+05 |
Average rank | Algorithm | Ranking |
1 | DCS-PSO | 2.03 |
2 | DMS-PSO | 2.17 |
3 | PSO-w | 3.69 |
4 | GPSO | 4.07 |
5 | CLPSO | 4.45 |
6 | LPSO | 4.59 |