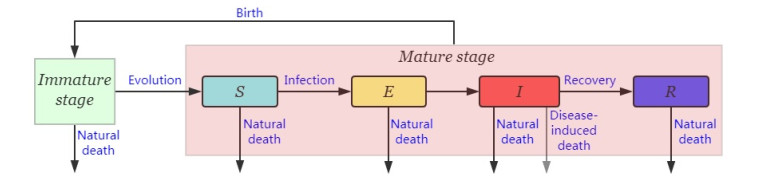
Citation: Li-zhen Du, Shanfu Ke, Zhen Wang, Jing Tao, Lianqing Yu, Hongjun Li. Research on multi-load AGV path planning of weaving workshop based on time priority[J]. Mathematical Biosciences and Engineering, 2019, 16(4): 2277-2292. doi: 10.3934/mbe.2019113
[1] | Jinliang Wang, Hongying Shu . Global analysis on a class of multi-group SEIR model with latency and relapse. Mathematical Biosciences and Engineering, 2016, 13(1): 209-225. doi: 10.3934/mbe.2016.13.209 |
[2] | Islam A. Moneim, David Greenhalgh . Use Of A Periodic Vaccination Strategy To Control The Spread Of Epidemics With Seasonally Varying Contact Rate. Mathematical Biosciences and Engineering, 2005, 2(3): 591-611. doi: 10.3934/mbe.2005.2.591 |
[3] | Hai-Feng Huo, Fan Wu, Hong Xiang . On threshold dynamics for periodic and time-delayed impulsive systems and application to a periodic disease model. Mathematical Biosciences and Engineering, 2022, 19(1): 836-854. doi: 10.3934/mbe.2022038 |
[4] | Gergely Röst, Jianhong Wu . SEIR epidemiological model with varying infectivity and infinite delay. Mathematical Biosciences and Engineering, 2008, 5(2): 389-402. doi: 10.3934/mbe.2008.5.389 |
[5] | Rundong Zhao, Qiming Liu, Huazong Zhang . Dynamical behaviors of a vector-borne diseases model with two time delays on bipartite networks. Mathematical Biosciences and Engineering, 2021, 18(4): 3073-3091. doi: 10.3934/mbe.2021154 |
[6] | Zhenguo Bai . Threshold dynamics of a periodic SIR model with delay in an infected compartment. Mathematical Biosciences and Engineering, 2015, 12(3): 555-564. doi: 10.3934/mbe.2015.12.555 |
[7] | Yan Zhang, Sanyang Liu, Zhenguo Bai . Global dynamics of a di usive single species model with periodic delay. Mathematical Biosciences and Engineering, 2019, 16(4): 2293-2304. doi: 10.3934/mbe.2019114 |
[8] | Jie He, Zhenguo Bai . Global Hopf bifurcation of a cholera model with media coverage. Mathematical Biosciences and Engineering, 2023, 20(10): 18468-18490. doi: 10.3934/mbe.2023820 |
[9] | Hai-Feng Huo, Qian Yang, Hong Xiang . Dynamics of an edge-based SEIR model for sexually transmitted diseases. Mathematical Biosciences and Engineering, 2020, 17(1): 669-699. doi: 10.3934/mbe.2020035 |
[10] | Lin Zhao, Zhi-Cheng Wang, Liang Zhang . Threshold dynamics of a time periodic and two–group epidemic model with distributed delay. Mathematical Biosciences and Engineering, 2017, 14(5&6): 1535-1563. doi: 10.3934/mbe.2017080 |
Throughout history, epidemics have been a serious threat to human survival and development, and human has been committed to find effective ways to prevent and control epidemics. Mathematical modeling provides a useful way to understand the dynamics of transmission of an infectious disease, and in the process, it provides effective guides and strategies for the control of diseases. One of the earliest mathematical models in epidemiology was introduced by Kermack and McKendrick [1]. The Kermack-McKendrick model is a compartmental model based on relatively simple assumptions on the rates of flow between different classes of members of the population [2], and it plays an important role in characterizing the transmission dynamics of disease within a short outbreak period. Following the work of Kermack and McKendrick, a large number of researchers pay attention to the study of epidemic models (see, e.g., [2,3,4,5]).
In the earlier epidemic models, the attributes of individuals such as contact patterns, models of transmission and geographic distributions are assumed to be homogeneous. In population biology, however, the life cycle of many populations go through different stages and the habits are different at different stages (see, e.g., [5,6]). During the last decades, quite a few stage-structured models have been used to characterize the population dynamics, see, e.g., [2,5,7]. In epidemiology, empirical evidence indicates that developmental stages of population have a profound impact on the transmission dynamics of some diseases, and the individuals in some stages don't participate in the infection cycle and don't have the reproductive capacity. For example, TB is highly age-dependent (see [8]), and the population who is likely to be infected is the adult individuals since newborns are vaccinated in many countries, but the immunity has decreased with increasing age. Moreover, the biological cycle of some species goes through some separate and distinct stages, and the biological and epidemiological properties of different stages are quite different. For example, the biological cycle of some salmon species goes through three stages: egg, juvenile and adult fish, and juveniles mature in the fresh water stream [9], so that the individuals in egg and juvenile stages do not participate in the infection cycle of some diseases for mature individuals. It is therefore necessary to incorporate the stage structure into the epidemic models. When the stage structure is introduced, there will be a time-delay term in the model, see, e.g., [5].
In epidemiology, another time-delay factor which should be considered is the latency. Empirical observation shows that many diseases have incubation period which differs from disease to disease, and the habit at latent stage is different at infectious stage (see, e.g., [4]). Therefore, it is significant that brings incubation period into epidemic models. During the past decades, a lot of models with latency have been studied and used to characterize the disease transmission. An SEIRS epidemic model with latent and immune periods was formulated and analyzed by Cooke and van den Driessche [10]. Li and Zou [11,12] generalized SIR model to patchy environments with incubation period. In [11], they found that the disease exists multiple outbreaks before it goes to extinction, which is in sharp contrast to the dynamics of classic Kermack-McKendrick SIR model.
Note that the aforementioned models are all given by autonomous systems of differential equations. Autonomous systems of differential equations provide an appropriate characterization for the spread of epidemics. However, certain diseases admit seasonal fluctuation and it is now well known that seasonal changes are ubiquitous and can exert strong influence on the spread of infectious diseases. Recently, the interaction of time delay and seasonality in epidemic models has attracted much attention. Zhao [13] established the theory of basic reproduction ratio for periodic and time-delayed compartmental population models, and considered a periodic SEIR model with an incubation period. More and more attentions pay to the study of periodic epidemic models with latency since then, see e.g., [14,15,16,17].
In reality, due to the stochastic effects and the complexity of external environments, fluctuations in nature may not exactly periodic, and hence, the parameters in an epidemic model are not necessary to be periodic. Even if they are periodic, they are also not always share a common period. Though one can obtain the exactly periodic parameters in controlled laboratory experiments, as noted in [18,19], environmental changes in nature are hardly periodic. In an almost periodic epidemic model, the time almost periodic dependence reflects the influence of certain "seasonal" fluctuations which are roughly but not exactly periodic. Therefore, almost periodic epidemic models are significant in the study of dynamics of infectious diseases. Up to now, there have been a few works concerning the global dynamics for almost periodic epidemic models with stage structure and latency, in which the maturation and latency periods are assumed to be constants (see, e.g., [20,21]). However, for the time-dependent case, the global dynamics of epidemic models dose not have an adequate characterization.
It is worth noting that for some populations, the maturation period and latency of some diseases also depend on environment factors. For example, fish egg development time to hatching is temperature dependent, see, e.g., [22,23], and the lengths of the latent periods of some diseases for fish are also temperature dependent, such as furunculosis [24,25]. Based on this observation, population and epidemiology models concerning time-dependent delay were developed in quite a few works, see, e.g., [26,27,28]. In particular, Li and Zhao [29] derived and studied an SEIRS epidemic model with a time-dependent latent period in a periodic environment. To our best knowledge, however, there is no work at present of considering the interaction of time-dependent maturation and incubation periods in the directly transmitted diseases models. The purpose of this paper is to study the global dynamics of an SEIR models with maturation and incubation delays in an almost periodic environment, in which the time delays are time-dependent.
In this paper, we employ an almost periodic time-delayed system framework to evaluate the effects of seasonal fluctuations, stage structure and latent period on the spread of infectious disease. The remaining parts are organized as follows. In section 2, we formulate an almost periodic SEIR model with time-dependent time delays, and give the underlying assumptions. In section 3, we introduce the basic reproduction ratios ˆR0 and R0 for population and disease, respectively, and establish threshold-type results on the global dynamics in terms of basic reproduction ratios. In section 4, we present some numerical simulations to interpret the obtained theoretical results, and reveal the effect of parameters on R0.
Let (X,d) be a metric space. A function f∈C(R,X) is said to be almost periodic if for any ϵ>0, the set
T(f,ϵ):={s∈R:d(f(t+s)−f(t))<ϵ,∀t∈R} |
is a relatively dense subset of R, that is, there exists a positive number h>0 such that [c,c+h]∩T(f,ϵ)≠∅, ∀c∈R. Let AP(R,X):={f∈C(R,X): f is an almost periodic function}. Then AP(R,X) is a Banach space equipped with the supremum norm ‖⋅‖. A function f∈C(D×R,X) is said to be uniformly almost periodic in t if f(x,⋅) is almost periodic for each x∈D, and for any compact set E⊂D, f is uniformly continuous on E×R ([30,31]).
We consider a disease for some population with immature and mature stages, where the immature individuals don't have reproductive capacity, and use G(t) and N(t) to denote the total numbers of immature and mature individuals. Let α(t) represent the maturation delay. Mathematically, we assume that α(t) is continuously differential in R. We use b(t,N) to denote the per-capita birth rate of the population, and let d1(t) and μ(t) be the natural death rates of immature and mature individuals, respectively. In the absence of disease, it then follows from [32] that the change of N(t) is governed by equation
dN(t)dt=(1−α′(t))Λ(t−α(t),N(t−α(t)))e−∫tt−α(t)d1(r)dr−μ(t)N(t), | (2.1) |
where Λ(t,N)=b(t,N)N. In order to determine the sign of 1−α′(t), we introduce the development rate of immature stage at time t, denoted by k(t)≥ε>0 (see, e.g., [28]). It then follows that the following relationship holds,
∫tt−α(t)k(s)ds=1. | (2.2) |
Differentiating equation (2.2) with respect to time t, we get
1−α′(t)=k(t)k(t−α(t)). |
It then follows that there exists a ρ>0 such that ρ<1−α′(t)<1ρ.
We assume that only mature individuals participate in the infection cycle, and let S(t), E(t), I(t) and R(t) represent the total numbers of the susceptible, exposed, infective and recovered populations at time t, respectively. Moreover, we assume that there is no vertical infection. Base on these assumptions, the schematic diagram of SEIR model is given in Figure 1. We adopt the mass action infection mechanism that the lost of susceptible individuals by infection is at a rate proportional to the number of infectious individuals. Then we get the following system
dS(t)dt=(1−α′(t))Λ(t−α(t),N(t−α(t))))e−∫tt−α(t)d1(r)dr−β(t)S(t)I(t)−μ(t)S(t),dE(t)dt=β(t)S(t)I(t)−μ(t)E(t)−C(t),dI(t)dt=C(t)−(μ(t)+d(t)+γ(t))I(t),dR(t)dt=γ(t)I(t)−μ(t)R(t). | (2.3) |
Here, β(t) is the infection rate, d(t) represents the disease-induced death rate, γ(t) is the recovery rate, and C(t) denotes the number of newly occurred infectious population per unit time at time t. Let τ(t) represent the time-dependent latent period. Mathematically, we also assume that τ(t) is continuously differential in R. In consideration of biological meanings, the following compatibility condition should be imposed:
E(0)=∫0−τ(0)e−∫0sμ(r)drβ(s)S(s)I(s)ds. |
Moreover, we have
E(t)=∫tt−τ(t)e−∫tsμ(r)drβ(s)S(s)I(s)ds. |
It is then necessary to determine C(t). By the results in [29,section 2], it follows that
C(t)=(1−τ′(t))e−∫tt−τ(t)μ(r)drβ(t−τ(t))S(t−τ(t))I(t−τ(t)). |
Thus, the SEIR model is governed by the following system,
dS(t)dt=(1−α′(t))Λ(t−α(t),N(t−α(t)))e−∫tt−α(t)d1(r)dr−β(t)S(t)I(t)−μ(t)S(t),dE(t)dt=β(t)S(t)I(t)−(1−τ′(t))e−∫tt−τ(t)μ(r)drβ(t−τ(t))S(t−τ(t))I(t−τ(t))−μ(t)E(t),dI(t)dt=(1−τ′(t))e−∫tt−τ(t)μ(r)drβ(t−τ(t))S(t−τ(t))I(t−τ(t))−(μ(t)+d(t)+γ(t))I(t),dR(t)dt=γ(t)I(t)−μ(t)R(t). | (2.4) |
By an argument similar to that of α(t), we get that there exists an η>0 such that η<1−τ′(t)<1η. Considering the effect of environmental factors on disease transmission, we assume that b(t,N) is uniformly almost periodic and the time-dependent coefficients of system (2.4) are all almost periodic in t. By [32,Lemma 2.2], it follows that e−∫tt−α(t)d1(r)dr and e−∫tt−τ(t)μ(r)dr are almost periodic.
For the sake of convenience, we let
p(t):=(1−α′(t))e−∫tt−α(t)d1(r)dr and q(t):=(1−τ′(t))e−∫tt−τ(t)μ(r)dr. |
Note that we can drop the E and R equations in model (2.4), and the system (2.4) can be rewritten as
dN(t)dt=p(t)Λ(t−α(t),N(t−α(t)))−μ(t)N(t)−d(t)I(t),dS(t)dt=p(t)Λ(t−α(t),N(t−α(t))))−β(t)S(t)I(t)−μ(t)S(t),dI(t)dt=q(t)β(t−τ(t))S(t−τ(t))I(t−τ(t))−(μ(t)+d(t)+γ(t))I(t). | (2.5) |
In order to investigate the dynamics of system (2.5), we make the following assumptions (see, e.g., [5,32]).
(A1) β(t), μ(t), d(t) and γ(t) are nonnegative and continuous functions, and inf.
(A2) b(t, N) is continuous, and satisfies \inf\limits_{t\in\mathbb{R}}b(t, 0) > 0 and \frac{\partial b(t, N)}{\partial N} < 0 , \forall N\geq0 , t\in \mathbb{R} .
(A3) There exists a constant K^{*} > 0 such that \mu(t) > p(t)b(t-\alpha(t), K) holds for all t\in \mathbb{R} when K\geq K^{*} .
Let \alpha^{*} = \sup\limits_{t\in \mathbb{R}}\alpha(t) , \tau^{*} = \sup\limits_{t\in \mathbb{R}}\tau(t) , and L = \max\{\alpha^{*}, \tau^{*}\} . We define C_{L}: = C([-L, 0], \mathbb{R}^{3}) . The norm of C_{L} is defined by \|\varphi\| = \max\limits_{\theta\in[-L, 0]} \|\varphi(\theta)\|_{\mathbb{R}^{3}} . Define positive cone C^{+}_{L}: = C([-L, 0], \mathbb{R}_{+}^{3}) . It is clear that the interior of C^{+}_{L} , \text{Int}(C^{+}_{L}) = C([-L, 0], \text{Int}(\mathbb{R}_{+}^{3})) , is nonempty. Then the ordering on C_{L} generated by C^{+}_{L} is defined as follows,
\begin{equation*} \begin{aligned} \varphi\leq\psi&\Leftrightarrow \varphi_i(\theta) \leq\psi_i(\theta), \quad &\forall \theta\in[-L^{*}, 0], \ 1\leq i\leq3, \\ \varphi \lt \psi&\Leftrightarrow \varphi_i(\theta)\leq\psi_i(\theta), \ \varphi\neq\psi, \quad &\forall\theta\in[-L^{*}, 0], \ 1\leq i\leq3, \\ \varphi\ll\psi&\Leftrightarrow \varphi_i(\theta) \lt \psi_i(\theta), \quad &\forall \theta\in[-L^{*}, 0], \ 1\leq i\leq3. \end{aligned} \end{equation*} |
For a continuous function f\in C([-L, T), \mathbb{R}^{3}) ( T > 0 ), define f_{t}\in C_{L} by f_{t}(\theta) = f(t+\theta) , \forall\theta\in [-L, 0] , t\in[0, T) .
Lemma 2.1. Let (A1)-(A3) hold. Then system (2.5) admits a unique nonnegative and bounded solution on [0, \infty) with the initial datum \phi\in C^{+}_{L} .
Proof. For any \phi = (\phi_{1}, \phi_{2}, \phi_{3})\in C^{+}_{L} , we define G(t, \phi) = (G_{1}(t, \phi), G_{2}(t, \phi), G_{3}(t, \phi)) as follows,
\begin{equation*} G(t, \phi) = \left( \begin{aligned} &p(t)\Lambda(t-\alpha(t), \phi_{1}(-\alpha(t)))-\mu(t)\phi_{1}(0)-d(t)\phi_{3}(0)\\ &p(t)\Lambda(t-\alpha(t), \phi_{1}(-\alpha(t)))) -\beta(t)\phi_{2}(0)\phi_{3}(0)-\mu(t)\phi_{2}(0)\\ &q(t)\beta(t-\tau(t))\phi_{2}(-\tau(t)) \phi_{3}(-\tau(t))-(\mu(t)+d(t)+\gamma(t))\phi_{3}(0) \end{aligned} \right). \end{equation*} |
Note that G(t, \phi) is continuous and Lipschitzian with respect to \phi in each compact subset of C^{+}_{L} . It then follows from [33,Theorems 2.2.1 and 2.2.3] that system (2.5) admits a unique solution w(t, \phi) on its maximal interval of existence with initial condition w_{0} = \phi\in C^{+}_{L} . Moreover, G_{i}(t, \phi)\geq0 whenever \phi = (\phi_{1}, \phi_{2}, \phi_{3})\geq0 and \phi_{i}(0) = 0 , \forall 1\leq i\leq3 . It then follows from [34,Theorem 5.2.1] that the solution w(t, \phi) of system (2.5) with w_{0} = \phi\in C^{+}_{L} is nonnegative for all t\geq 0 in its maximal interval of existence.
Define
Y = \left\{\varphi\in C([-L, 0], \mathbb{R}_{+}^{4}):\varphi_{2}(0) = \int_{-\tau}^{0}e^{-\int_{s}^{0}\mu(r)dr} \beta(s)\varphi_{1}(s)\varphi_{3}(s)ds\right\}. |
By an argument similar to that in [13], we obtain that for any \varphi\in Y , system (2.4) admits a unique nonnegative solution u(t, \varphi) = (S(t), E(t), I(t), R(t)) satisfying initial condition u_{0} = \varphi . Recall that N(t) = S(t)+E(t)+I(t)+R(t) , and it satisfies
\begin{equation} \frac{dN(t)}{dt}\leq p(t)\Lambda(t-\alpha(t), N(t-\alpha(t)))-\mu(t)N(t). \end{equation} | (2.6) |
Assumptions (A3) together with comparison principle indicate that C([-L, 0], [0, K^{*}]) is positively invariant for equation (2.6), and hence, C([-L, 0], [0, K^{*}]^3) is positively invariant for system (2.5). Thus, the solutions of system (2.5) with initial data in C^{+}_{L} exist globally on [0, \infty) , and are also ultimately bounded.
In this section, we establish threshold-type results on the global dynamics of system (2.5) or (2.4) in terms of basic reproduction ratios.
We first establish the basic reproduction ratio \hat{R}_{0} for population equation (2.1) in the absence of disease. Let C_{\alpha^{*}}: = C([-\alpha^{*}, 0], \mathbb{R}) and C_{\alpha^{*}}^{+}: = C([-\alpha^{*}, 0], \mathbb{R}_{+}) . Note that equation (2.1) has a trivial zero solution. Linearizing equation (2.1) at the zero solution, we obtain the following linearized equation,
\begin{equation} \frac{d N(t)}{d t} = r(t)N(t-\alpha(t))-\mu(t)N(t), \end{equation} | (3.1) |
where r(t): = p(t)b(t-\alpha(t), 0) . By the general theory of linear functional differential equations in [33,Section 8.1], it follows that for any \varphi\in C_{\alpha^{*}} , system (3.1) has a unique solution N(t, s, \varphi) ( t\geq s ) with initial condition N_{s} = \varphi .
Let F_{1}(t)\varphi: = r(t)\varphi(-\alpha(t)) , \forall\varphi\in C_{\alpha^{*}} , and V_{1}(t): = \mu(t) . Then linear equation (3.1) can be written as
\begin{equation*} \frac{d N(t)}{d t} = F_{1}(t)N_{t}-V_{1}(t)N(t), \end{equation*} |
where N_{t}(\theta) = N(t+\theta) , \forall \theta\in[-\alpha^{*}, 0] . Refer to [32], we define the next generation operator \hat{L} as
\begin{equation*} \ [\hat{L}\phi](t) = \int^{\infty}_{0}e^{-\int^{t}_{t-s}V_{1}(\sigma)d\sigma} F_{1}(t-s)\phi(t-s+\cdot)ds. \end{equation*} |
Motivated by [20,13], the basic reproduction ratio \hat{R}_{0} is defined as \hat{R}_{0}: = r(\hat{L}) , the spectral radius of \hat{L} . Let N(t, \phi) denote the solution of equation (2.1) with initial condition N_{0} = \phi\in C_{\alpha^{*}}^{+} , it then follows from [32,Theorem 3.5] that the following threshold-type result holds.
Lemma 3.1. Let (A1)-(A3) hold, then the following statements are valid:
\rm(i) If \hat{R}_{0} < 1 , then \lim\limits_{t\rightarrow\infty}N(t, \phi) = 0 , \forall \phi\in C_{\alpha^{*}}^{+} ;
\rm(ii) If \hat{R}_{0} > 1 , then there exists an \epsilon > 0 such that \liminf\limits_{t\rightarrow\infty}N(t, \phi)\geq\epsilon holds for all \phi\in C_{\alpha^{*}}^{+} with \phi(0) > 0 .
Lemma 3.1 shows that if \hat{R}_{0} < 1 , then the population will die out whether the disease breaks out or not. In the following, we investigate the threshold dynamics for system (2.5) in the case where \hat{R}_{0} > 1 . We first define the basic reproduction ratio R_{0} for system (2.5). In order to ensure the existence of the disease-free almost periodic solution, we further make the following assumption.
(A4) \frac{\partial \Lambda(t, N)}{\partial N} > 0 for all N\geq0 and t\geq0 .
It then follows from [32,Theorem 3.7] that equation (2.1) admits a unique positive almost periodic solution N^{*}(t) , and \lim\limits_{t\rightarrow\infty}|N(t, \varphi)-N^{*}(t)| = 0 for all \varphi \in C^{+}_{\alpha^{*}} with \varphi(0) > 0 , and hence, system (2.5) admits a unique nontrivial positive disease-free almost periodic solution E^{*}(t) = (N^{*}(t), N^{*}(t), 0) . Assumption (A4) implies that the birth rate function is monotone. For a non-monotone case \Lambda(t, N) = a(t)e^{-b(t)N}N , the condition that (2.1) exists a globally stable almost periodic solution can be found in [32].
Linearizing system (2.5) at E^{*}(t) , we then have the equation for infectious class as follows,
\begin{equation} \frac{dI(t)}{dt} = k(t)I(t-\tau(t))-h(t)I(t), \end{equation} | (3.2) |
where
k(t) = q(t)\beta(t-\tau(t))N^{*}(t-\tau(t)) , \quad h(t) = (\mu(t)+d(t)+\gamma(t)). |
Let C_{\tau^{*}}: = C([-\tau^{*}, 0], \mathbb{R}) and C_{\tau^{*}}^{+}: = C([-\tau^{*}, 0], \mathbb{R}_{+}) . By the general theory of linear functional differential equations in [33,Section 8.1], it follows that for any \varphi\in C_{\tau^{*}} , system (3.2) admits a unique solution I(t, s, \varphi) ( t\geq s ) with I_{s} = \varphi . We define the evolution family U(t, s) on C_{\tau^{*}} of equation (3.2) as
\begin{equation*} U(t, s)\varphi = I_{t}(s, \varphi), \ \forall \varphi\in C_{\tau^{*}}, \ t\geq s, \ s\in\mathbb{R}, \end{equation*} |
where I_{t}(s, \varphi)(\theta) = I(t+\theta, s, \varphi) , \forall\theta\in[-\tau^{*}, 0] . Let \omega(U) be the exponential growth bound of the evolution family U(t, s) , that is,
\begin{equation*} \omega(U) = \inf \{\omega\in\mathbb{R}:\exists K_{0}\geq1\ \text{such that}\ \|U(t+s, s)\|\leq K_{0} e^{\omega t}, \ \forall s\in\mathbb{R}, \ t\geq0\}. \end{equation*} |
Lemma 3.2. [32,Theorem 3.2] The following statements are valid:
\rm(i) There exists an almost periodic function a(t) such that e^{\int^{t}_{0}a(s)ds} is a solution of (3.2).
\rm(ii) \omega(U) = \lim\limits_{t\rightarrow\infty} \frac{1}{t}\int^{t}_{0}a(s)ds .
\rm(iii) Let u(t, \phi) be the unique solution of (3.2) with initial condition u_{0} = \phi . Then for any \phi \in {\rm{Int}}(C_{\tau^{*}}^{+}) , there holds \omega(U) = \lim\limits_{t\rightarrow\infty} \frac{\ln u(t, \phi)}{t} .
Let \hat{U}(t, s) denote the evolution family on \mathcal{C}_{L}: = C([-L, 0], \mathbb{R}) of equation (3.2) and \omega(\hat{U}) be its exponential growth bound. We have the following observation.
Proposition 3.3. \omega(\hat{U}) = \omega(U) .
Proof. Note that L\geq\tau^{*} , if L = \tau^{*} , then the conclusion is natural, and we just need to prove the case that L > \tau^{*} . By the definition of \omega(\hat{U}) , for any \delta > 0 , there exists K_{\delta} > 1 such that
\|\hat{U}(t+s, s)\phi\|_{\mathcal{C}_{L}}\leq K_{\delta}e^{(\omega(\hat{U})+\delta)t} \|\phi\|_{\mathcal{C}_{L}}, \quad \forall t\geq0, \ s \in\mathbb{R}, \ \phi\in \mathcal{C}_{L}. |
For any \varphi\in C_{\tau^{*}} , we can find a \phi\in \mathcal{C}_{L} such that \|\varphi\|_{C_{\tau^{*}}} = \|\phi\|_{\mathcal{C}_{L}} and \varphi(\theta) = \phi(\theta) , \forall\theta\in[-\tau^{*}, 0] . Thus, we have
\|U(t+s, s)\varphi\|_{C_{\tau^{*}}}\leq \|\hat{U}(t+s, s)\phi\|_{\mathcal{C}_{L}}\leq K_{\delta}e^{(\omega(\hat{U})+\delta)t} \|\phi\|_{\mathcal{C}_{L}} = K_{\delta}e^{(\omega(\hat{U})+\delta)t} \|\varphi\|_{C_{\tau^{*}}} , \quad \forall t\geq0, \ s \in\mathbb{R}. |
By the definition of \omega(U) , we get \omega(U)\leq \omega(\hat{U})+\delta .
On the other hand, the definition of \omega(U) shows that for any \delta > 0 , there exists L_{\delta} > 1 such that
I(t+s, s, \varphi)\leq \|U(t+s, s)\varphi\|_{C_{\tau^{*}}}\leq L_{\delta}e^{(\omega(U)+\delta)t} \|\varphi\|_{C_{\tau^{*}}}, \quad \forall t\geq0, \ s \in\mathbb{R}, \ \varphi\in C_{\tau^{*}}. |
For any \phi\in \mathcal{C}_{L} , there exists \varphi\in C_{\tau^{*}} such that
I(t+s, s, \phi) = I(t+s, s, \varphi)\leq L_{\delta}e^{(\omega(U)+\delta)t} \|\varphi\|_{C_{\tau^{*}}}\leq L_{\delta}e^{(\omega(U)+\delta)t} \|\phi\|_{\mathcal{C}_{L}}, |
and hence,
\|\hat{U}(t+s, s)\phi\|_{\mathcal{C}_{L}} = \|I_{t+s}(s, \phi)\|_{\mathcal{C}_{L}} \leq L_{\delta}He^{(\omega(U)+\delta)t} \|\phi\|_{\mathcal{C}_{L}}, |
where H = \max\limits_{0\leq \theta\leq L} \{L_{\delta}e^{{(\omega(U)+\delta)\theta}}\} . Thus, \|\hat{U}(t+s, s)\| \leq L_{\delta}He^{(\omega(U)+\delta)t} . By the definition of \omega(\hat{U}) , we get \omega(\hat{U})\leq \omega(U)+\delta .
In above proof, letting \delta\rightarrow 0^{+} , we obtain that \omega(U)\leq \omega(\hat{U}) and \omega(\hat{U})\leq \omega(U) , and hence, \omega(\tilde{U}) = \omega(U) .
Let V_{2}(t) = h(t) and F_{2} be a map defined as follows,
F_{2}(t)\phi = k(t)\phi(-\tau(t)), \ \forall\phi\in C_{\tau^{*}}. |
It then follows that equation (3.2) can be written as
\begin{equation*} \frac{dI(t)}{dt} = F_{2}(t)I_{t}-V_{2}(t)I(t). \end{equation*} |
Let \Psi_{-V_{2}}(t, s) , t\geq s , be the evolution family of linear equation
\begin{equation*} \frac{dI(t)}{dt} = -V_{2}(t)I(t). \end{equation*} |
A simple computation shows that
\Psi_{-V_{2}}(t, s) = e^{-\int_{s}^{t}h(r)dr}, \quad \forall t\geq s, \ s\in\mathbb{R}. |
Let AP(\mathbb{R}, \mathbb{R}) be the order Banach space of all continuous almost periodic functions from \mathbb{R} to \mathbb{R} equipped with the supremum norm and the positive cone AP(\mathbb{R}, \mathbb{R}_{+}) . Following [20], let \phi\in AP(\mathbb{R}, \mathbb{R}_{+}) denote the initial distribution of infectious individuals. For any given s\geq0 , F_{2}(t-s)\phi_{t-s} , t\geq s , represents the distribution of newly infectious individuals at time t-s , which is produced by the infectious individuals at time t-s-\tau(t) and still in the infectious compartment at time t-s . Then \Psi_{-V_{2}}(t, t-s)F_{2}(t-s)\phi_{t-s} denotes the distribution of those infectious individuals who were newly reproduced at time t-s-\tau(t) and remain in the infectious compartment at time t . Thus, \int_{0}^{\infty}\Psi_{-V_{2}}(t, t-s)F_{2}(t-s)\phi_{t-s}ds represents the distribution of accumulative new infectious individuals at time t produced by all those infectious individuals introduced at all previous time to t and still in the infectious compartment at time t . Define the next generation operator L as
\begin{equation*} \begin{aligned} \ [L\phi](t) = \int^{\infty}_{0}e^{-\int^{t}_{t-s}V_{2}(\sigma)d\sigma} F_{2}(t-s)\phi(t-s+\cdot)ds, \quad \forall \phi\in AP(\mathbb{R}, \mathbb{R}), \ t\in\mathbb{R}. \end{aligned} \end{equation*} |
Motivated by [13,20,29], the basic reproduction ratio R_{0} is defined as R_{0}: = r(L) , the spectral radius of L .
Consider the following linear almost periodic equation with a parameter \rho > 0 :
\begin{equation} \left\{ \begin{aligned} &\frac{d I(t)}{dt} = \frac{1}{\rho}F_{2}(t)I_{t}-V_{2}(t)I(t), \quad t\geq s, \\ &I_{s} = \phi\in C_{\tau^{*}}^{+}. \end{aligned} \right. \end{equation} | (3.3) |
Let U(t, s, \rho) be the evolution family of equation (3.3) and \omega(U(\rho)) be its exponential growth bound. Then we have the following result, which comes from [20,Theorems 3.8 and 3.10].
Lemma 3.4. R_{0}-1 has the same sign as \omega(U) . Furthermore, if R_{0} > 0 , then \rho = R_{0} is the unique solution of \omega(U(\rho)) = 0 .
We now establish a threshold-type result on the global dynamics of system (2.5) in terms of R_{0} . Let X_{0} = \{\psi = (\psi_{1}, \psi_{2}, \psi_{3})\in C^{+}_{L}:\psi_{3}(0) > 0\} . We first show the global extinction.
Theorem 3.5. Let (A1)-(A4) hold. In the case where \hat{R}_{0} > 1 , if R_{0} < 1 , then the disease-free almost periodic solution (N^{*}(t), N^{*}(t), 0) of system (2.5) is globally attractive in X_{0} .
Proof. Let (N(t, \phi), S(t, \phi), I(t, \phi)) be the solution of system (2.5) with initial datum \phi\in X_{0} . The global stability of N^{*}(t) for (2.1) indicates that for any \delta > 0 , there exists t_{0} > 0 such that
\begin{equation*} N(t, \phi) \lt N^{*}(t)+\delta, \quad\forall t\geq t_{0}. \end{equation*} |
Let \hat{U}_{\delta}(t, s) denote the evolution family on C([-L, 0], \mathbb{R}_{+}) associated with the following perturbed linear almost periodic equation:
\begin{equation} \frac{dI(t)}{dt} = q(t)\beta(t-\tau(t))(N^{*}(t-\tau(t))+\delta) I(t-\tau(t))-h(t)I(t). \end{equation} | (3.4) |
Since R_{0} < 1 , Lemma 3.4 together with Proposition 3.3 yield \omega(U) = \omega(\hat{U}) . Thus, we can restrict \delta small enough such that \omega(\hat{U}_{\delta}) < 0 . Again by Lemma 3.2, there exists an almost periodic function a^{*}_{\delta}\in AP(\mathbb{R}, \mathbb{R}) such that y(t) = e^{\int_{0}^{t}a^{*}_{\delta}(\mu)d\mu} is a solution of (3.4), and \omega(\hat{U}_{\delta}) = \lim\limits_{t\rightarrow\infty} \frac{\int^{t}_{0}a^{*}_{\delta}(\mu)d\mu}{t} < 0 . By the third equation of system (2.5), we have that I(t, \phi) satisfies
\begin{equation*} \frac{dI(t)}{dt}\leq q(t)\beta(t-\tau(t)) (N^{*}(t-\tau(t))+\delta) I(t-\tau(t))-h(t)I(t) \end{equation*} |
for all t\geq t_{0} . Choose a positive constant M such that I(t, \phi)\leq My(t) , \forall t\in[t_{0}-L, t_{0}] . By the comparison theorem for delay differential equations ([34,Theorem 5.1.1]), we obtain
I(t, \phi)\leq My(t) = Me^{\int_{0}^{t}a^{*}_{\delta}(r)dr}, \quad \forall t\geq t_{0}. |
Note that
\begin{equation*} \lim\limits_{t\rightarrow \infty} e^{\int_{0}^{t}a^{*}_{\delta}(r)dr} = \lim\limits_{t\rightarrow \infty} \left(e^{\frac{1}{t}\int_{0}^{t}a^{*}_{\delta}(r)dr}\right)^{t} = 0. \end{equation*} |
Hence, we deduce that I(t, \phi)\rightarrow0 as t\rightarrow\infty . Using the chain transitive arguments similar to those in the proof of [21,Theorem 4.2], we further obtain \lim\limits_{t\rightarrow\infty}(N(t)-N^{*}(t)) = 0 and \lim\limits_{t\rightarrow\infty}(S(t)-S^{*}(t)) = 0 .
Theorem 3.6. Let (A1)-(A4) hold. In the case where \hat{R}_{0} > 1 , if R_{0} > 1 , then there exists \epsilon > 0 such that the solution (N(t, \phi), S(t, \phi), I(t, \phi)) of system (2.5) with initial datum \phi\in X_{0} satisfies
\liminf\limits_{t\rightarrow \infty} (N(t, \phi), S(t, \phi), I(t, \phi))\geq (\epsilon, \epsilon, \epsilon). |
Proof. We use the skew-product semiflows approach to prove the desired uniform persistence. Let
\Gamma(t) = (p(t), \Lambda(t), \alpha(t), \mu(t), \beta(t), q(t), \tau(t), d(t), \gamma(t)) |
and H(\Gamma) be the closure of \{\Gamma_{s}:s\in\mathbb{R}\} under the compact open topology, where \Gamma_{s} is defined by \Gamma_{s}(t) = \Gamma(t+s) , \forall t\in\mathbb{R} . It then follows from [31,Theorem 1.6] that H(\Gamma) is compact. Define \zeta_{t}(\Theta) = \Theta_{t} , for all \Theta\in H(\Gamma) and t\in\mathbb{R} . Then \zeta_{t}:H(\Gamma)\rightarrow H(\Gamma) is a compact, almost periodic minimal and distal flow (see [35,Section VI.C]). Consider a family of almost periodic systems,
\begin{equation} \begin{array}{ll} \frac{dN(t)}{dt} = \bar{p}(t)\bar{\Lambda}(t-\bar{\alpha}(t), N(t-\bar{\alpha}(t)))-\bar{\mu}(t)N(t)-\bar{d}(t)I(t), \\ \frac{dS(t)}{dt} = \bar{p}(t)\bar{\Lambda}(t-\bar{\alpha}(t), N(t-\bar{\alpha}(t)))) -\bar{\beta}(t)S(t)I(t)-\bar{\mu}(t)S(t), \\ \frac{dI(t)}{dt} = \bar{q}(t)\bar{\beta}(t-\bar{\tau}(t))S(t-\bar{\tau}(t)) I(t-\bar{\tau}(t))-(\bar{\mu}(t)+\bar{d}(t)+\bar{\gamma}(t))I(t), \end{array} \end{equation} | (3.5) |
where (\bar{p}, \bar{\Lambda}, \bar{\alpha}, \bar{\mu}, \bar{\beta}, \bar{q}, \bar{\tau}, \bar{d}, \bar{\gamma}) = \Theta\in H(\Gamma) . Let
\begin{equation*} \begin{array}{lll} \partial X_{0}: = X\setminus X_{0}, \ Z: = X\times H(\Gamma), \ Z_{0}: = X_{0}\times H(\Gamma), \ \partial Z_{0}: = Z\setminus Z_{0}. \end{array} \end{equation*} |
Then Z_{0} and \partial Z_{0} are relatively open and closed in Z , respectively. For any given (\phi, \Theta)\in Z , let x(t, \phi, \Theta) = (N(t, \Theta), S(t, \Theta), I(t, \Theta)) be the unique solution of system (3.5) with initial condition x_{0}(\phi, \Theta) = \phi . It is easy to see that for any given \Theta\in H(\Gamma) , both X and X_{0} are positively invariant for solutions of (3.5). Let x_{t}(\phi, \Theta)(\theta): = x(t+\theta, \phi, \Theta) , \forall \theta\in[-L, 0] , we define a skew-product semiflow
\begin{equation*} \begin{aligned} \Pi:\mathbb{R}^{+}\times Z &\rightarrow Z, \\ (t, \phi, \Theta)&\mapsto (x_{t}(\phi, \Theta), \zeta_{t}(\Theta)). \end{aligned} \end{equation*} |
We use the notation \Pi_{t}(\phi, \Theta) = \Pi(t, \phi, \Theta) . Obviously, \Pi_{t} Z_{0}\subseteq Z_{0} , \forall t\geq 0 . Recall that the solutions of (2.5) are ultimately bounded in X , and hence, so does (3.5), which implies that \Pi_{t} is point dissipative on Z . By [36,Theorem 3.4.8], it follows that \Pi_{t}:Z\rightarrow Z admits a global compact attractor \hat{\mathcal{V}} .
Define
\begin{equation*} M_{\partial}: = \{(\phi, \Theta)\in \partial Z_{0}: \Pi_{t}(\phi, \Theta)\in \partial Z_{0}, \forall t\geq0 \}. \end{equation*} |
We now show that
\begin{equation} M_{\partial} = \{(N, S, 0, \Theta)\in Z:N\geq0, S\geq0, \Theta\in H(\Gamma)\}. \end{equation} | (3.6) |
For any (\hat{\phi}, \hat{\Theta})\in \{(N, S, 0, \Theta)\in Z:N\geq0, S\geq0, \Theta\in H(\Gamma)\} , the solution
x(t, \hat{\phi}, \hat{\Theta}) = (N(t, \hat{\Theta}), S(t, \hat{\Theta}), I(t, \hat{\Theta})) |
satisfies I(t, \hat{\Theta}) = 0 for all t\geq 0 and \hat{\Theta}\in H(\Gamma) , and hence, (\hat{\phi}, \hat{\Theta})\in M_{\partial} . This indicates that \{(N, S, 0, \Theta)\in Z:N\geq0, S\geq0, \Theta\in H(\Gamma)\}\subset M_{\partial} . For any given (\tilde{\phi}, \tilde{\Theta})\in M_{\partial} , x_{t}(\tilde{\phi}, \tilde{\Theta})\in \partial X_0 holds for all t\geq 0 . We further show that I(t, \tilde{\Theta}) = 0 for all t\geq0 . Assume, for the sake of contradiction, that there exists t_{1}\geq0 such that I(t_{1}, \tilde{\Theta}) > 0 . It then follows from the second equation of (3.5) that I(t, \tilde{\Theta}) > 0 for all t\geq t_{1} , which contradicts the fact that (N(t, \tilde{\Theta}), S(t, \tilde{\Theta}), I(t, \tilde{\Theta}))\in \partial X_0 . Since I(t, \tilde{\Theta}) = 0 for all t\geq0 , we have
(\tilde{\phi}, \tilde{\Theta})\in \{(N, S, 0, \Theta)\in Z:N\geq0, S\geq0, \Theta\in H(\Gamma)\}, |
and hence, M_{\partial}\subset \{(N, S, 0, \Theta)\in Z:N\geq0, S\geq0, \Theta\in H(\Gamma)\} . This proves (3.6).
Note that for each \Theta\in H(\Gamma) , in the case where \hat{R}_{0} > 1 , the following equation,
\begin{equation} \frac{dN(t)}{dt} = \bar{p}(t)\bar{\Lambda}(t-\bar{\alpha}(t), N(t-\bar{\alpha}(t)))-\bar{\mu}(t)N(t), \end{equation} | (3.7) |
admits a positive almost periodic solution N^{*}(t, \Theta) , which is uniformly asymptotically stable. Let \mathcal{M} = \{(N^{*}_{0}(\Theta), N^{*}_{0}(\Theta), 0, \Theta):\Theta\in H(\Omega)\} , where N^{*}_{0}(\Theta)(\theta) = N^{*}(\theta, \Theta) , \forall\theta\in[-L, 0] . By the uniqueness and continuity of solutions, it follows that N^{*}_{t}(\Theta) = N^{*}_{0}(\zeta_{t}(\Theta)) . Hence, \mathcal{M} is a compact and invariant set for \Pi_{t}:Z\rightarrow Z .
For any given (\phi, \Theta)\in M_{\partial} , let \omega(\phi, \Theta) be the omega limit set of (\phi, \Theta) for \Pi_{t} . Let (\check{\phi}, \check{\Theta})\in\omega (\phi, \Theta) be given. Then there exists a sequence t_{n}\rightarrow\infty such that \lim\limits_{n\rightarrow \infty}\Pi_{t_{n}}(\phi, \Theta) = (\check{\phi}, \check{\Theta}) . Note that \Pi_{t_{n}}(\phi, \Theta) = (x_{t_{n}}(\phi, \Theta), \zeta_{t_{n}}(\Theta)) and \lim\limits_{n\rightarrow \infty}\|x_{t_{n}}(\phi, \Theta)- (N^{*}_{t_{n}}(\Theta), N^{*}_{t_{n}}(\Theta), 0)\| = 0 . Since N^{*}_{0}(\Theta) is continuous in \Theta , and H(\Gamma) is compact, it follows that N^{*}_{0}(\Theta) is uniformly continuous in \Theta\in H(\Gamma) . This indicates that N^{*}_{t_{n}}(\Theta) = N^{*}_{0}(\zeta_{t_{n}}(\Theta)) \rightarrow N^{*}_{0}(\check{\Theta}) as n\rightarrow\infty . Hence, (\check{\phi}, \check{\Theta}) = (N^{*}_{0}(\check{\Theta}), N^{*}_{0}(\check{\Theta}), 0, \check{\Theta})\in \mathcal{M} . Thus, \omega(\phi, \Theta)\subseteq \mathcal{M} . It then follows that \mathcal{M} is a compact and isolated invariant set for \Pi_{t} in \partial Z_{0} , \cup_{(\phi, \Sigma)\in M_{\partial}}\omega(\phi, \Theta)\subseteq \mathcal{M} , and no subset of \mathcal{M} forms a cycle for \Pi_{t} in \partial Z_{0} .
Since R_{0} > 1 , Lemma 3.4 shows that \omega(U) > 0 . We let \omega(\bar{U}) be the exponential growth bound of the evolution family of system (3.2) with (k, \tau, h) replaced by (\bar{k}, \bar{\tau}, \bar{h}) on C([-L, 0], \mathbb{R}) , where
\bar{k}(t) = \bar{q}(t)\bar{\beta}(t-\bar{\tau}(t)) N^{*}(t-\bar{\tau}(t), \Theta), \quad \bar{h}(t) = (\bar{\mu}(t)+\bar{d}(t)+\bar{\gamma}(t)). |
It then follows from Proposition 3.3 that \omega(\bar{U}) = \omega(\hat{U}) = \omega(U) > 0 . We use \omega(\hat{U}_{\vartheta}) to denote the exponential growth bound associated with the following linear equation on C([-L, 0], \mathbb{R}) ,
\begin{equation*} \frac{dI(t)}{dt} = \bar{k}_{\rho}(t)I(t-\bar{\tau}(t))-\bar{h}(t)I(t), \end{equation*} |
where
\begin{equation*} \bar{k}_{\rho}(t) = \bar{q}(t)\bar{\beta}(t-\bar{\tau}(t)) (N^{*}(t-\bar{\tau}(t), \Theta)-\rho) \ \text{and} \ \rho \lt \inf\limits_{t\in\mathbb{R}} N^{*}(t). \end{equation*} |
Hence, we can choose a sufficiently small constant \rho > 0 such that \omega(\hat{U}_{\rho}) > 0 .
Next, we claim that
\begin{equation*} \limsup\limits_{t\rightarrow \infty}d(\Pi_{t}(\phi, \Theta), \mathcal{M})\geq\rho, \quad\forall(\phi, \Theta)\in Z_{0}. \end{equation*} |
Assume, by contradiction, that for some (\bar{\phi}, \bar{\Theta})\in Z_{0} , \bar{\Theta} = (\bar{p}^{1}, \bar{\Lambda}^{1}, \bar{\alpha}^{1}, \bar{\mu}^{1}, \bar{\beta}^{1}, \bar{q}^{1}, \bar{\tau}^{1}, \bar{d}^{1}, \bar{\gamma}^{1}) , there holds
\begin{equation*} \limsup\limits_{t\rightarrow \infty}d(\Pi_{t}(\bar{\phi}, \bar{\Theta}), \mathcal{M}) \lt \rho. \end{equation*} |
It then follows that there exists t_{2} > 0 such that S(t, \bar{\phi}, \bar{\Theta}) \geq N^{*}(t, \bar{\Theta})-\rho , \forall t\geq t_{2} . Thus, I(t, \bar{\phi}, \bar{\Theta}) satisfies
\begin{equation} \frac{dI(t)}{dt}\geq\bar{k}^{1}_{\rho}(t)I(t-\bar{\tau}^{1}(t)) -\bar{h}^{1}(t)I(t), \quad \forall t\geq t_{2}, \end{equation} | (3.8) |
where
\begin{equation*} \bar{k}^{1}(t) = \bar{q}^{1}(t)\bar{\beta}^{1}(t-\bar{\tau}^{1}(t)) (N^{*}(t-\bar{\tau}^{1}(t), \bar{\Theta})-\rho), \quad \bar{h}^{1}(t) = (\bar{\mu}^{1}(t)+\bar{d}^{1}(t)+\bar{\gamma}^{1}(t)). \end{equation*} |
By virtue of Lemma 3.2, there exists an almost periodic function a^{*}(t, \bar{\Theta}) such that \hat{I}(t, \bar{\Theta}) = e^{\int_{0}^{t}a^{*}(r, \bar{\Theta})dr} is a solution of
\begin{equation} \frac{dI(t)}{dt} = \bar{k}^{1}_{\rho}(t)I(t-\bar{\tau}^{1}(t)) -\bar{h}^{1}(t)I(t), \end{equation} | (3.9) |
and
\begin{equation*} \omega(\tilde{U}_{\rho}) = \lim\limits_{t\rightarrow \infty}\frac{1}{t}\int_{0}^{t}a^{*}(r, \bar{\Theta})dr \gt 0, \end{equation*} |
where \tilde{U}_{\rho}(t, s) (t\geq s) denotes the evolution family of equation (3.9) on C([-L, 0], \mathbb{R}) . Since (\bar{\phi}, \bar{\Theta})\in Z_{0} indicates that there exists a t_{3} > t_{2}+L such that I(t_{3}+\theta, \bar{\phi}, \bar{\Theta}) \in \rm{Int}(\mathbb{R}_{+}) , \forall \theta\in[-L, 0] , we can take \xi > 0 small enough such that I(t_{3}+\theta, \bar{\phi}, \bar{\Theta})\geq\xi\hat{I} (t_{3}+\theta, \bar{\Theta}) , \forall \theta\in[-L, 0] . By the comparison principle, as applied to system (3.8), it then follows that
\begin{equation*} I(t, \bar{\phi}, \bar{\Theta})\geq \xi\hat{I}(t, \bar{\Theta}) = \xi e^{\int_{0}^{t}a^{*}(r, \bar{\Theta}) dr}, \quad \forall t\geq t_{3}. \end{equation*} |
Moreover, since
\begin{equation*} \lim\limits_{t\rightarrow \infty} e^{\int_{0}^{t}a^{*}(r, \bar{\Theta})dr} = \lim\limits_{t\rightarrow \infty} \left(e^{\frac{1}{t}\int_{0}^{t}a^{*} (r, \bar{\Theta})dr}\right)^{t} = \infty, \end{equation*} |
we get \lim\limits_{t\rightarrow \infty}I(t, \bar{\phi}, \bar{\Theta}) = \infty , a contradiction.
Recall that \mathcal{M} is an isolated invariant set for \Pi_{t} in \partial Z_{0} , the claim above shows that \mathcal{M} is also an isolated invariant set for \Pi_{t} in Z . The above claim also indicates that W^{s}(\mathcal{M})\cap Z_{0} = \emptyset , where the set
\begin{equation*} W^{s}( \mathcal{M}): = \{(\phi, \Theta)\in Z:\omega(\phi, \Theta)\neq\emptyset, \omega(\phi, \Theta)\subset \mathcal{M}\} \end{equation*} |
represents the stable set of \mathcal{M} for \Pi_{t} . By the continuous-time version of [37,Theorem 1.3.1 and Remark 1.3.1], the skew-product semiflow \Pi_{t}:Z\rightarrow Z is uniformly persistent with respect to Z_{0} . That is, there exists \varepsilon > 0 such that for any (\phi, \Theta)\in Z_{0} , \liminf\limits_{t\rightarrow \infty}d(\Pi_{t}(\phi, \Theta), \partial Z_{0})\geq\varepsilon . Since \Pi_{t} is compact for any t > L , it follows that \Pi_{t} is asymptotically smooth. By [38,Theorem 3.7 and Remark 3.10], we get that \Pi_{t}:Z_{0}\rightarrow Z_{0} admits a global attractor \mathcal{\hat{V}}_{0} .
It remains to prove the practical uniform persistence. Since \mathcal{\hat{V}}_{0}\in Z_{0} and \Pi_{t}\mathcal{\hat{V}}_{0} = \mathcal{\hat{V}}_{0} , it follows that \phi_{2}(0) > 0 for all (\phi_{1}, \phi_{2}, \phi_{3}, \Theta)\in \mathcal{\hat{V}}_{0} . From the invariance of \mathcal{\hat{V}}_{0} , we get that \phi_{i}(0) > 0 for i = 1, 3 . Obviously, \lim\limits_{t\rightarrow\infty} d(\Pi_{t}(\phi, \Theta), \mathcal{\hat{V}}_{0}) = 0 for all (\phi, \Theta)\in Z_{0} . Define a continuous function g:Z\rightarrow[0, \infty) by
g(\phi, \Theta) = \min\limits_{i = 1, 2, 3}\{\phi_{i}(0)\}, \quad \forall (\phi, \Theta) = (\phi_{1}, \phi_{2}, \phi_{3}, \Theta)\in Z. |
It is easy to see that g(\phi, \Theta) > 0 for all (\phi, \Theta)\in \mathcal{V}_{0} . The compactness of \mathcal{V}_{0} implies that \inf\limits_{(\phi, \Theta)\in \mathcal{V}_{0}} g(\phi, \Theta) = \min\limits_{(\phi, \Theta)\in \mathcal{V}_{0}} g(\phi, \Theta) > 0 . Consequently, we conclude that there exists an \epsilon > 0 such that \liminf\limits_{t\rightarrow\infty} (N(t, \Theta), S(t, \Theta), I(t, \Theta)) \geq(\epsilon, \epsilon, \epsilon) for any (\phi, \Theta)\in Z_{0} .
In this section, we carry out some numerical simulations to illustrate the theoretical results obtained in previous sections, and numerically analyze the influence of the almost periodic time delays on the disease transmission. The numerical computation of \hat{R}_{0} and R_{0} is based on Lemmas 3.2 and 3.4, see the numerical simulations in [32] for detail.
For the sake of simplicity, we first suppose that all the parameters except \tau(t) are independent of time t . In our numerical simulations, we take b(t, N) = \frac{c}{z+N} Day ^{-1} , where c, z > 0 , which is a classical birth rate function. Other examples of birth rate functions \Lambda(t, N) in the biological literature can be found in, e.g., [5]. In this case, the population change in the absence of disease is governed by
\begin{equation} \frac{d N(t)}{d t} = \frac{c}{z+N(t-\alpha)}e^{-d_{1}\alpha}N(t-\alpha)-\mu(t)N(t). \end{equation} | (4.1) |
Corresponding to equation (3.1), the linearized equation of (2.1) at trivial solution is
\begin{equation*} \label{linapapdelaysnn} \frac{d N(t)}{d t} = \frac{c}{z}e^{-d_{1}\alpha}N(t-\alpha)-\mu N(t). \end{equation*} |
For \phi\in AP(\mathbb{R}, \mathbb{R}_{+}) , the next generation operator \hat{L} for population model in the absence of disease is defined as
\begin{equation*} \ [\hat{L}\phi](t) = \int^{\infty}_{0}\frac{c}{z}e^{-\mu s} e^{-d_{1}(s+\alpha)}\phi(t-s-\alpha)ds. \end{equation*} |
We take z = 20 , \alpha = 365 Day, d_{1} = \frac{1}{365\times20} Day ^{-1} and \mu = \frac{1}{365\times5} Day ^{-1} . Thanks to Lemma 3.4, it easily follows that \hat{R}_{0} is in scale with c , that is, \frac{\hat{R}^{1}_{0}}{c_{1}} = \frac{\hat{R}^{2}_{0}}{c_{2}} , where c_{i} > 0 ( i = 1, 2 ), \hat{R}^{1}_{0} and \hat{R}^{2}_{0} are the basic reproduction ratios corresponding to c = c_{1} and c = c_{2} , respectively. The graph of \hat{R}_{0} versus c is presented in Figure 2. We choose c = 0.02 , in this case we have \hat{R}_{0} = 1.736 > 1 , and Figure 3 shows that the population is uniformly persistent in the absence of disease. A direct computation, furthermore, shows that the equilibrium solution of equation (4.1) is \frac{ce^{^{-d_{1}\alpha}}}{\mu}-z , and hence, the disease-free equilibrium is (\frac{ce^{^{-d_{1}\alpha}}}{\mu}-z, \frac{ce^{^{-d_{1}\alpha}}}{\mu}-z, 0) . Thus, the linearized equation of infectious class equation at disease-free equilibrium reads
\begin{equation} \frac{dI(t)}{dt} = (1-\tau^{\prime}(t))e^{-\mu\tau(t)}\beta\cdot (\frac{ce^{^{-d_{1}\alpha}}}{\mu}-z)I(t-\tau(t))-(\mu+d+\gamma)I(t). \end{equation} | (4.2) |
Let \beta = 0.002 Day ^{-1} , d = 0.001 Day ^{-1} , \gamma = 0.01 Day ^{-1} and \tau(t) = 30+12\cos(\frac{2\pi t}{365})+\sin(\frac{\sqrt{2}t}{365}) Day. By numerical computation, we obtain R_{0} = 2.509 > 1 . The numerical simulations further show that the disease is uniformly persistent, see Figure 4. Similar to the relationship between \hat{R}_{0} and c , it is easy to see that R_{0} is in scale with \beta . We choose \beta = 0.0007 Day ^{-1} , it follows that R_{0} = 0.878 < 1 , and the numerical simulations indicate that the disease will vanish, see Figure 5. Clearly, the numerical simulations are consistent with the threshold results obtained in section 3.
By Lemma 3.4, we can easily obtain the effect of parameters \mu , \beta , c , d_{1} , \alpha , z , d and \gamma on R_{0} , see Table 1. If \tau is a positive constant, moreover, then the numerical simulations in [20] shows that R_{0} is decreasing with respect to \tau . We now are interested in the sensitivity of R_{0} on the fluctuation of latent period. Let \tau(t) = 30+a\cdot(12\cos(\frac{2\pi t}{365})+\sin(\frac{\sqrt{2}t}{365})) Day, a\in[0, 4] . It is clear that if a = 0 , then latent period is a constant delay, and the amplitude of latent period is increasing with respect to a . Let other parameters remain unchanged, the relationship between R_{0} and a is presented in Figure 6. It indicates that increasing the amplitude of latent period has a positive effect on disease transmission, but the effect is negligible. Note that \mu = \frac{1}{365\times5} Day ^{-1} , it causes that the amplitude of e^{-\mu\tau(t)} is very small. Choose \mu = 0.02 Day ^{-1} , c = 2 , and other parameters remain unchanged. By numerical simulations, in this case, we obtain that the effect of a on R_{0} should not be ignored, see Figure 7.
Parameter | the effect on R_{0} |
\mu | - |
\beta | + |
c | + |
d_{1} | - |
\alpha | - |
z | - |
d | - |
\gamma | - |
In order to consider the effect of the fluctuation of maturation delay \alpha(t) on R_{0} , we let d_{1} = 0.02 Day ^{-1} , c = 0.05 , \tau(t) = \tau = 30 Day, \alpha(t) = 30+b\cdot(12\cos(\frac{2\pi t}{365})+\sin(\frac{\sqrt{2}t}{365})) Day, b\in[0, 4] , and other parameters remain unchanged. Figure 8 shows the relationship between R_{0} and b . It indicates that the amplitude of maturation period has a little effect on the disease transmission, and R_{0} is increasing with respect to the amplitude of maturation period.
In our numerical simulations, we numerically analyze the effects of the fluctuations of maturation and incubation periods on disease transmission. It is shown that both the amplitudes of maturation and latent periods have a little effect on the disease transmission, and R_{0} is increasing with respect to them. But if the death rates \mu and d_{1} are very small, then the effect can be negligible. Furthermore, by numerical computations, we obtain that the mean values of e^{\mu\tau(t)} and e^{d_{1}\alpha(t)} are increasing with respect to a and b , respectively, which may cause that R_{0} is increasing with respect to a and b .
It is well-known in epidemiology that seasonal changes have profound effects on disease transmission. With the combination of environmental factors, stage structure and incubation period of disease, we formulate and study an almost periodic epidemic model with time-dependent delays. The almost periodicity reflects the influence of certain seasonal variations which are approximatively but not exactly periodic, and allows one to consider general seasonal fluctuations. For this mathematical model, we first introduce the basic reproduction ratio \hat{R}_{0} for population, it can be regarded as an index of reproductive ability of population. By the theory developed in [32], we present the threshold dynamics for population in the absence of disease. Furthermore, in the case where \hat{R}_{0} > 1 , we introduce the basic reproduction ratio R_{0} for disease, it provides an index of transmission intensity. With the recent theory developed in [20], we can characterize the basic reproduction ratio R_{0} by the exponential growth bound associated with a linear functional differential equation (3.2). By the skew-product semiflow, comparison arguments and persistence theory, we show that the sign of R_{0}-1 completely determines the extinction and persistence of the disease. More precisely, the disease will be eliminated if R_{0} < 1 , while the disease persists in the population if R_{0} > 1 .
In the final section, we carry out some numerical simulations to illustrate the theoretical results obtained in previous sections, and numerically analyze the influence of the parameters of model on the disease transmission. Note that, in particular, the maturation delay and incubation period are time-dependent, we are interested in the dependence between R_{0} and the amplitudes of maturation and latent periods. Numerical simulations indicate that both the amplitudes of maturation and latent periods have a little effect on the disease transmission, and R_{0} is increasing with respect to them. But if the death rates \mu and d_{1} are very small, then the effect can be neglected.
The authors are very grateful to the editors and the referees for careful reading and valuable comments which led to important improvements of the original manuscript. This work is supported by NNSF of China (12071193).
All authors declare no conflicts of interest in this paper.
[1] | H. Fazlollahtabar and M. Saidi-Mehrabad, Models for AGVs' scheduling and routing, Autonomous Guided Vehicles, 20 (2015), 1–15. |
[2] | G. M. Li, X. Y. Li, L. Gao, et al., Tasks assigning and sequencing of multiple AGVs based on an improved harmony search algorithm, J. Amb. Inter. Hum. Comp., 2018. Available from: DOI, 10.1007/s12652-018-1137-0. |
[3] | D. H. Lee, Resource-based task allocation for multi-robot systems, Robot Auton. Syst., 103 (2018), 151–161. |
[4] | T. J. Chen, Y. Sun, W. Dai, et al., On the shortest and conflict-free path planning of multi-AGV system based on dijkstra algorithm and the dynamic time-window method, Adv. Mater. Res., 645 (2013), 267–271. |
[5] | A. Kaplan, N. Kingry, P. Uhing, et al., Time-optimal path planning with power schedules for a solar-powered ground robot, IEEE T. Autom. Sci. Eng., 14 (2017), 1235–1244. |
[6] | N. Smolic-Rocak, S. Bogdan, Z. Kovacic, et al., Time windows based dynamic routing in multi-AGV systems, IEEE T. Autom. Sci. Eng., 7 (2010), 151–155. |
[7] | K. G. Huo, Y. Q. Zhang, Z. H. Hu, et al., Research on scheduling problem of multi-load AGV at automated container terminal, J. Dalian Univ. Techno., 6 (2016), 24–251. |
[8] | G. M. Li, B. Zeng, W. Liao, et al., A new AGV scheduling algorithm based on harmony search for material transfer in a real-world manufacturing system, Adv. Mech. Eng., 10 (2018), 1–13. |
[9] | M. Mousavi, H. J. Yap, S. N. Musa, et al., Multi-objective AGV scheduling in an FMS using a hybrid of genetic algorithm and particle swarm optimization, PLoS One, 12 (2017), 1–24. |
[10] | F. Hamed and M. Nezam, An optimal path in a bi-criteria AGV-based flexible job shop manufacturing system having uncertain parameters, Int. J. Ind. Syst. Eng., 13 (2010), 27–55. |
[11] | H. Fazlollahtabara and M. Saidi-Mehrabad, Optima path in an intelligent AGV based manufacturing system, Transp. Lett., 7 (2015), 219–228. |
[12] | V. K. Chawla, A. K. Chanda, S. Angra, et al., Multi-load AGVs scheduling by application of modified memetic particle swarm optimization algorithm, J. Braz. Socy. Mech. Sci., 2018. Available from: 10.1007/s40430-018-1357-4. |
[13] | S. Bakshi, Z. Y. Yan, D. M. Chen, et al., A fast algorithm on minimum-time scheduling of an autonomous ground vehicle using a traveling salesman framework, J. Dyn. Syst. Meas. Control, 2018. Available from: DOI, 10.1115/1.4040665. |
[14] | H. Fazlollahtabar and S. Hassanli, Hybrid cost and time path planning for multiple autonomous guided vehicles, Appl. Intell., 48 (2018), 482–498. |
[15] | M. Grunow, H. Gunther, M. Lehmann, et al., Dispatching multi-load AGVs in highly automatedseaport container terminals, OR Spectrum., 26 (2014), 211–235. |
[16] | T. J. Park, J. W. Ahn, C. S. Han, et al., A path generation algorithm of an automatic guided vehicle using sensor scanning method, J. Mech. Sci. Technol., 16 (2002), 137–146. |
[17] | H. Y. Wang, Q. Huang, C. T. Li, et al., Graph theory algorithm and MATLAB simulation, BeiJing (2009), Beihang University Press. |
[18] | A. Ali and M Tawhid, A hybrid particle swarm optimization and genetic algorithm with population partitioning for large scale optimization problems, Ain. Shams. Eng. J., 8 (2017), 191–206. |
[19] | X. Y. Li and L. Gao, An effective hybrid genetic algorithm and tabu search for flexible job shop scheduling problem, Int. J. Prod. Econ., 174 (2016), 93–110. |
[20] | P. B. C. Miranda and R. B. C. Prudêncio, Generation of particle swarm optimization algorithms: An experimental study using grammar guided genetic, Appl. Soft. Comput., 60 (2017), 281–296. |
[21] | S. Hamed and K. Govindan, A hybrid particle swarm optimization and genetic algorithm for closed loop supply chain network design in large scale networks, Appl. Math. Model., 39 (2015), 3990–4012. |
[22] | K. Deb and N. Padhye, Enhancing performance of particle swarm optimization through an algorithmic link with genetic algorithms, Comput. Optim. Appl., 57 (2014), 761–794. |
[23] | X. Y. Li, L. Gao, Q. K. Pan, et al., An effective hybrid genetic algorithm and variable neighborhood search for integrated process planning and scheduling in a packaging machine workshop, IEEE T. Syst. Man. Cy. S., 2018. |
[24] | A. M. Bertram, Q. Zhang, S. C. Kong, et al., A novel particle swarm and genetic algorithm hybrid method for diesel engine performance optimization, Int. J. Engine. Res., 17 (2016), 732–747. |
[25] | X. Y. Li, C. Lu, L. Gao, et al., An effective multi-objective algorithm for energy efficient scheduling in a real-life welding shop, IEEE T. Ind. Inform., 14 (2018), 5400–5409. |
[26] | Y. N. Yan, L. Z. Liu, B. Y. Luo, et al., Arrangement of garment production line by particle swarm algorithm, J. Text. Res., 39 (2018), 120–124. |
1. | Manoj Kumar, Syed Abbas, Abdessamad Tridane, A novel method for basic reproduction ratio of a diffusive size-structured population model with delay, 2022, 109, 0924-090X, 3189, 10.1007/s11071-022-07558-5 | |
2. | Yun Liu, Xijuan Liu, Francisco Balibrea, Bifurcations and Structures of the Parameter Space of a Discrete-Time SIS Epidemic Model, 2022, 2022, 2314-4785, 1, 10.1155/2022/2233452 |
Parameter | the effect on R_{0} |
\mu | - |
\beta | + |
c | + |
d_{1} | - |
\alpha | - |
z | - |
d | - |
\gamma | - |