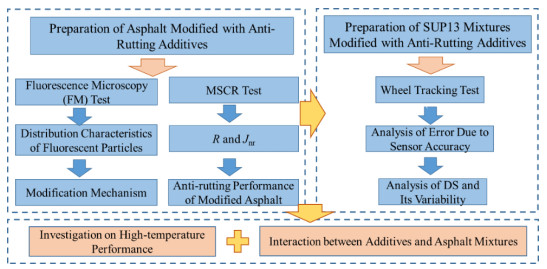
As a principal category in the promising field of medical image processing, medical image enhancement has a powerful influence on the intermedia features and final results of the computer aided diagnosis (CAD) system by increasing the capacity to transfer the image information in the optimal form. The enhanced region of interest (ROI) would contribute to the early diagnosis and the survival rate of patients. Meanwhile, the enhancement schema can be treated as the optimization approach of image grayscale values, and metaheuristics are adopted popularly as the mainstream technologies for medical image enhancement. In this study, we propose an innovative metaheuristic algorithm named group theoretic particle swarm optimization (GT-PSO) to tackle the optimization problem of image enhancement. Based on the mathematical foundation of symmetric group theory, GT-PSO comprises particle encoding, solution landscape, neighborhood movement and swarm topology. The corresponding search paradigm takes place simultaneously under the guidance of hierarchical operations and random components, and it could optimize the hybrid fitness function of multiple measurements of medical images and improve the contrast of intensity distribution. The numerical results generated from the comparative experiments show that the proposed GT-PSO has outperformed most other methods on the real-world dataset. The implication also indicates that it would balance both global and local intensity transformations during the enhancement process.
Citation: Jinyun Jiang, Jianchen Cai, Qile Zhang, Kun Lan, Xiaoliang Jiang, Jun Wu. Group theoretic particle swarm optimization for gray-level medical image enhancement[J]. Mathematical Biosciences and Engineering, 2023, 20(6): 10479-10494. doi: 10.3934/mbe.2023462
[1] | Kun Lan, Gloria Li, Yang Jie, Rui Tang, Liansheng Liu, Simon Fong . Convolutional neural network with group theory and random selection particle swarm optimizer for enhancing cancer image classification. Mathematical Biosciences and Engineering, 2021, 18(5): 5573-5591. doi: 10.3934/mbe.2021281 |
[2] | Anam Mehmood, Ishtiaq Rasool Khan, Hassan Dawood, Hussain Dawood . A non-uniform quantization scheme for visualization of CT images. Mathematical Biosciences and Engineering, 2021, 18(4): 4311-4326. doi: 10.3934/mbe.2021216 |
[3] | Binju Saju, Neethu Tressa, Rajesh Kumar Dhanaraj, Sumegh Tharewal, Jincy Chundamannil Mathew, Danilo Pelusi . Effective multi-class lungdisease classification using the hybridfeature engineering mechanism. Mathematical Biosciences and Engineering, 2023, 20(11): 20245-20273. doi: 10.3934/mbe.2023896 |
[4] | Mei-Ling Huang, Zong-Bin Huang . An ensemble-acute lymphoblastic leukemia model for acute lymphoblastic leukemia image classification. Mathematical Biosciences and Engineering, 2024, 21(2): 1959-1978. doi: 10.3934/mbe.2024087 |
[5] | Jiande Zhang, Chenrong Huang, Ying Huo, Zhan Shi, Tinghuai Ma . Multi-population cooperative evolution-based image segmentation algorithm for complex helical surface image. Mathematical Biosciences and Engineering, 2020, 17(6): 7544-7561. doi: 10.3934/mbe.2020385 |
[6] | Jun Gao, Qian Jiang, Bo Zhou, Daozheng Chen . Convolutional neural networks for computer-aided detection or diagnosis in medical image analysis: An overview. Mathematical Biosciences and Engineering, 2019, 16(6): 6536-6561. doi: 10.3934/mbe.2019326 |
[7] | Zhuang Zhang, Wenjie Luo . Hierarchical volumetric transformer with comprehensive attention for medical image segmentation. Mathematical Biosciences and Engineering, 2023, 20(2): 3177-3190. doi: 10.3934/mbe.2023149 |
[8] | Vasileios E. Papageorgiou, Georgios Petmezas, Pantelis Dogoulis, Maxime Cordy, Nicos Maglaveras . Uncertainty CNNs: A path to enhanced medical image classification performance. Mathematical Biosciences and Engineering, 2025, 22(3): 528-553. doi: 10.3934/mbe.2025020 |
[9] | Shen Jiang, Jinjiang Li, Zhen Hua . Transformer with progressive sampling for medical cellular image segmentation. Mathematical Biosciences and Engineering, 2022, 19(12): 12104-12126. doi: 10.3934/mbe.2022563 |
[10] | Janarthanan R, Eshrag A. Refaee, Selvakumar K, Mohammad Alamgir Hossain, Rajkumar Soundrapandiyan, Marimuthu Karuppiah . Biomedical image retrieval using adaptive neuro-fuzzy optimized classifier system. Mathematical Biosciences and Engineering, 2022, 19(8): 8132-8151. doi: 10.3934/mbe.2022380 |
As a principal category in the promising field of medical image processing, medical image enhancement has a powerful influence on the intermedia features and final results of the computer aided diagnosis (CAD) system by increasing the capacity to transfer the image information in the optimal form. The enhanced region of interest (ROI) would contribute to the early diagnosis and the survival rate of patients. Meanwhile, the enhancement schema can be treated as the optimization approach of image grayscale values, and metaheuristics are adopted popularly as the mainstream technologies for medical image enhancement. In this study, we propose an innovative metaheuristic algorithm named group theoretic particle swarm optimization (GT-PSO) to tackle the optimization problem of image enhancement. Based on the mathematical foundation of symmetric group theory, GT-PSO comprises particle encoding, solution landscape, neighborhood movement and swarm topology. The corresponding search paradigm takes place simultaneously under the guidance of hierarchical operations and random components, and it could optimize the hybrid fitness function of multiple measurements of medical images and improve the contrast of intensity distribution. The numerical results generated from the comparative experiments show that the proposed GT-PSO has outperformed most other methods on the real-world dataset. The implication also indicates that it would balance both global and local intensity transformations during the enhancement process.
Pavement rutting, as one of the most typical distresses in asphalt pavement, may influence driving comfort and safety [1] and even accelerate the decline of pavement serviceability [2]. The generation of pavement rutting is primarily due to the effects of densification and shearing slip of mixtures [3], which has a strong relationship with the asphalt properties and mechanical interaction of aggregates. In order to objectively treat the rutting distress, many studies developed applicable technologies or new materials to improve the performances of asphalt binder and asphalt mixtures, including employing effective additives and optimizing gradation design and combination design [4,5,6]. The anti-rutting additive was considered as an efficient material to increase the rutting resistance of asphalt pavement, which could be utilized by directly mixing with mineral aggregates [7]. The asphalt mixture is viscoelastic, which implies that a high temperature will affect its stiffness and resistance to deformation. With the application of additives, the elastic or viscous behaviors will hugely change, accordingly resulting in modification on the binder and improvement of the material's structure. However, the dispersed form of anti-rutting additives in a binder and aggregate skeleton remains unclear. Therefore, the issue of high-temperature performance is an essential focus for extensive research on asphalt mixtures with anti-rutting additives.
There are many types of anti-rutting additives, typically including PR PLAST.S produced by PR. INDUSTRIE in France, composite modifiers Duroflex and Domix developed by a company in Berlin, Germany as well as SEAM developed by Shell in the United States [8]. However, these additives are different in their application conditions and effects. Zhang et al. [9] compared the improvement effects of asphalt mixtures modified with anti-rutting additives PR, RA and Duroflex, and found that the types of anti-rutting additives have a greater impact on the high-temperature performance of asphalt mixtures compared to their dosage. Further, a novel anti-rutting asphalt mixture was developed by using matrix asphalt with a penetration of 40/60, anti-rutting additive and hard basalt aggregate, which demonstrates promising practicality of road intersection maintenance [10]. Wang et al. [11] prepared a permeative anti-rutting agent based on epoxy resin and Buton rock asphalt. Han et al. [12] investigated the effects of granulated polymer anti-rutting additives on the comprehensive performance of asphalt mixtures. Xiao et al. [13] compared three binders modified with polymer additives and found that the modified asphalt with high polymer contents showed a similar performance to that of styrene–butadiene–styrene (SBS) modified asphalt.
Based on the background above, a considerable number of performance-centered researches have been conducted to investigate the mixtures' enhancement due to anti-rutting additives. However, the interaction between the mixtures and anti-rutting additives remains unclear. Besides, the studies on the effect of anti-rutting additives on the sole binder or aggregate system have not been reported yet, as they are both contributory subcomponents of the mixture. To fill this gap, this study examined the high-temperature performance of anti-rutting additives modified asphalt and asphalt mixtures. Afterward, the modification mechanism of the additive was investigated.
To test the road performances of binders, three modified asphalts were prepared by mixing with three anti-rutting additives, respectively. Thereafter, the fluorescence microscopy (FM) test and multiple stress creep recovery (MSCR) test were performed to examine the binders' performance and compatibility. Further error analyses of the wheel tracking test were carried out to ensure the reliability of the test. Most significantly, the interaction mechanism between additives and asphalt was figured out by associating the material's performance and compatibility. The technical framework of this study is shown in Figure 1.
70# base asphalt was used to prepare modified asphalt and asphalt mixtures, and its fundamental properties were tested in the laboratory in accordance with JTG E20-2011 [14], as presented in Table 1.
Properties | Result | Technical standard | Test method |
Density (g∙cm-3) | 1.036 | - | T0603 |
Penetration (25℃, 0.1 mm) | 71 | 60~80 | T0604 |
Softening point (℃) | 47 | > 46 | T0606 |
Ductility (10℃, cm) | 76 | > 20 | T0605 |
Three industrial granular anti-rutting additives, ARA-A, ARA-B and ARA-C (ARA is an abbreviation for anti-rutting additive, and A, B and C are marks that stand for different additives), were used to prepare modified asphalt binders. Figure 2 demonstrates the appearance of three additives, which are manufactured with a new mono-component epoxy and reactive tackifying resin. The specific composition of the three additives may be somewhat different. However, from the perspective of application in asphalt pavement, they all belong to the same category, namely, polymer modifiers.
Limestone was adopted for both coarse aggregate, fine aggregate and mineral powder. Their basic properties were tested according to JTG E42-2005 [15], as shown in Tables 2–4.
Technical indices | Test results | requirements | Test methods |
Crushing value (%) | 21 | ≤ 26 | T 0316 |
Los Angeles Abrasion (%) | 16.8 | ≤ 28 | T 0317 |
Water absorption (%) | 1.5 | ≤ 2.0 | T 0304 |
Robustness (%) | 3.5 | ≤ 12 | T 0314 |
Soft stone content (%) | 1.2 | ≤ 3.0 | T 0320 |
Elongated particles content (%) | 10.5 | ≤ 15 | T 0312 |
Technical indices | Test results | Requirements | Test methods |
Mud content (%) | 2.1 | ≤ 3 | T 0333 |
Apparent relative density | 2.635 | ≥ 2.50 | T 0304 |
Sand equivalent (%) | 76.7 | ≥ 60 | T 0334 |
Robustness (%) | 4.2 | ≤ 12 | T 0340 |
Technical indices | Test result | Requirements | Test methods |
Density (g•cm-3) | 2.68 | > 2.5 | T0352 |
Hydrophilic coefficient | 0.60 | < 1 | T0353 |
Moisture content (%) | 0.18 | < 1 | T0103 |
BET specific surface area (m2/g) | 0.49 | - | - |
In order to allow the additives to fully interact with the asphalt binder, a higher temperature (180℃) was conditioned to prepare ARA modified asphalts. A high-speed shearing machine was employed to efficiently shear the additives into tiny fragments so as to uniformly blend the mix. The rotation speed was 2000 r/min for preliminary blending, followed by 5000 r/min for shearing. Afterward, it was blended by a fan mixer at the rate of 1000 r/min to achieve a uniform phase state.
The Sup-13 was selected to design the gradation of asphalt mixtures, as shown in Figure 3. For anti-rutting asphalt mixtures, higher temperatures are required for both mixing and compaction, so that the additives have sufficient interaction between the asphalt and develop a considerable interlock and reinforcement with aggregate. The content of the ARA is 1% (mass ratio of additives to mixtures) according to the manufacturer's recommendation. Three types of ARAs were incorporated into the asphalt mixture by dry process, which implies additives were directly mixed with aggregates. During the preparing process, additives and aggregates were first mixed for 90 s to sufficiently soften the ARAs. Following that, asphalt was added to mix for 90 s so that additives could fully react with the asphalt. Finally, mineral powder was introduced into the compound as the filler. As suggested in engineering examples, the mixing temperature was confined within 185 to 190℃ and the compaction temperature was controlled within 175 to 180℃ [12].
FM is a desirable approach to reveal the compatibility of asphalt and ARAs. The major form existing in the compounds includes the asphalt phase and additive phase. The distribution characteristics of additives in asphalt may cause a considerable difference in the compatibility of modified asphalts, thus generating a variation of road performances. To prepare the FM samples, the modified asphalt was first heated to a flow state (180℃), then a small amount of asphalt was dropped on a glass slide, followed by covering a cover glass. Afterward, when asphalt samples were cooled down, they were observed via the fluorescence microscope. The magnification is 100 times. The most representative FM images were captured.
To investigate the anti-rutting performance of the asphalt containing ARAs, the MSCR test was conducted under stress-recovery controlled mode at a high temperature (60℃) according to AASHTO TP 70. Total 20 cycles of 0.1 kPa shear stress were initially exerted on modified asphalt specimens, followed by 10 cycles of 3.2 kPa shear stress. Each cycle contained loading time for one second and strain recovery for nine second, respectively [16]. The recovery rate R of each cycle was utilized to characterize the ability of elastic recovery, as expressed in Eq (1). The non-recoverable creep compliance Jnr of each cycle was employed to study the resistance to permanent deformation, as given by Eq (2). Both R and Jnr are significant indicators to describe the anti-rutting performance of asphalt at high temperature.
(1) |
(2) |
where σ is the stress; ε0 is the initial strain of each circle; εr is the strain after recovery of each circle; εc is the creep strain of each circle.
Pavement rutting is generally induced by severe high temperatures and repeated wheel loads. The wheel tracking test is thereby extensively employed to simulate the formation of pavement rutting according to JTG E20-2011. To prepare the specimens of the wheel tracking test, each mixture was poured into a rectangular slab with the dimensions of 300 mm × 300 mm × 50 mm, as shown in Figure 4. Following that, the specimen was subjected to repeated wheel pressure of 0.7 MPa with a loading speed of 42 cycles/min for 60 min. The test was carried out at 60℃ and the whole load period was 60 min. Eventually, dynamic stability (DS) was obtained to evaluate the anti-rutting performance of asphalt mixtures, which is calculated by using the rutting depth (RD) at 45 and 60 min, as shown in Eq (3).
(3) |
where d2 and d1 are the RDs at the loading time of 60 and 45 min, respectively.
The dispersed state of additives in asphalt will strongly influence their compatibility. In general, uniform fluorescent particles stand for desirable compatibility and accordingly indicate better modification effects on asphalt binder. Figure 5 presents four FM images of ARA modified asphalt. It is obvious that fluorescent particles appear in Figure 5(b)–(d), which are dispersed ARAs. Comparing various ARAs, the fluorescent particles in the image of ARA-A modified asphalt are relatively regular and uniform with large quantities. It indicates that ARA-A additives have formed a uniformly dispersed system with asphalt, behaving the beneficial compatibility. However, ARA-B modified asphalt indicates a slight difference with that of ARA-A, namely, less quantities of particles and larger sizes, so the ARA-B additive has relatively poor compatibility with asphalt. Notably, irregular fluorescent particles appear in ARA-C modified asphalt, showing elongated strip-type fluorescent particles, which may be attributed to the large size of the ARA-C additive, as described in Figure 5(d). However, it presents a large area of fluorescent particles in the ARA-C image, which implies the compatibility between the ARA-C and asphalt is acceptable. As a result, the compatibility between the asphalt and various additives fulfills the sequence: ARA-A > ARA-C > ARA-B.
The MSCR test was confirmed as a satisfactory approach for investigating the anti-rutting performance from the binder scale, in which the indicators R and Jnr highly correlate to the road performance [17]. A higher R and lower Jnr indicate better anti-rutting performance at a high temperature. Figure 6 presents shear strain-time curves of modified asphalts. The curves were obtained by applying 3.2 kPa. It is indicated that the incorporation of ARA-B additive leads to the minimum shear strain, followed by ARA-C and ARA-A, respectively. It implies that the deformation resistance under high temperature follows the sequence: ARA-B > ARA-C > ARA-A. Interestingly, the recoverable strain for ARA-B modified asphalt is obviously less than other two modified asphalts. This means the ARA-B modified asphalt has more sufficient elasticity characteristics.
The recovery rate R and non-recoverable creep compliance Jnr of different asphalts are presented in Figure 7. A remarkable increase of recovery rate is observed for the asphalt incorporating ARAs, with a maximum R up to 84.5% under 0.1 kPa and a maximum R up to 60.6% under 3.2 kPa. Notably, the recovery rates vary slightly for three types of modified asphalts. Similarly, a significant decrease in non-recoverable creep compliance is presented in Figure 7(b). It indicates that the resistance to unrecoverable deformation is dramatically improved for three modified asphalts.
Based on the fluorescence microscopy experimental results, it is clear that the compatibility between the additive and the asphalt is not a decisive basis for determining the anti-rutting performance of modified asphalts. In fact, large quantities of ARAs are difficult to directly blend with asphalt, so the distribution characteristics of additives would not have a strong correlation with high-temperature anti-rutting performance. Under the circumstances, an in-depth investigation into the effects of additives on mixtures remains to be developed.
Wheel tracking test is widely applied to determine the anti-rutting performance of mixtures. After incorporating anti-rutting additives, asphalt mixtures generally have remarkable resistance to deformation, thereby the rutting depth obtained in wheel tracking test tends to be extremely small, nearly no more than 1mm. In particular, the deformation d1 and d2 would vary slightly during the loading period, which is hard to be detected by the machine sensor with low accuracy. The dynamic stability DS will then be affected, obtaining a deviated DS. As a result, the experimental errors caused by sensor accuracy demand in-depth investigation.
To calculate the DS error, a DS1 is previously assumed, so the difference between d1 and d2 was obtained. Following that, given that the error might simultaneously happen to d1 and d2 or solely happen to one, the incorrect DS2 was determined. Based on DS1 and DS2, the relative errors of these two conditions were obtained, as shown in Figure 8(a)(b). It is found that the relative error gradually rises up with the increase of tested DS. Notably, the relative error due to both d1 and d2 increases more quickly than that due to one of them. Considering the uncertainty of errors, the possibility of both d1 and d2 having errors is bound to be high. For sensor accuracy ± 0.01 mm, when the DS of asphalt mixtures becomes 5250 cycle/mm, the relative error exceeds 20%, which is judged to have been an unacceptable error. For sensor accuracy ± 0.001 mm, when the DS is 53,000 cycle/mm, its relative error is regarded as an unacceptable error, exceeding 20%. It can be recognized that improving sensor accuracy would contribute to the reduction of test errors.
The findings imply that high-accuracy testing sensors are preferable to be employed for wheel tracking testing. Furthermore, the variability of DS should be focused to ensure the result errors are within an acceptable range.
The rutting depth curve acquired from the wheel tracking test records the development process of rutting. As shown in Figure 9, the rutting deformation of various asphalt mixtures increases with time, including those modified with ARA-A, ARA-B and ARA-C. A deformation-strengthened type curve is observed for the relationship between rutting depth and time. The rutting depth first sharply increases during 0–10 min, then it increases at a slow rate during 10–60 min. The accumulation rutting depth of anti-rutting asphalt mixtures is rather small, for all modified SUP-13. The ARA-B modified SUP-13 has the minimum accumulation rutting depth, not exceeding 0.45 mm, which is mainly due to the modification of binders by additives and their reinforcement formed with aggregates.
DS is a significant indicator to evaluate the anti-rutting performance of asphalt mixtures and reflect their resistance at the stable stage during rutting increase. A higher DS indicates the better high temperature performance. From Figure 10, it can be found that the DS of asphalt mixtures fulfills the sequence: SUP13-ARA-B > SUP13-ARA-A > SUP13-ARA-C > SUP13. Compared with SUP13, three modified asphalt mixtures have extremely larger DS, showing strong resistance to the rutting distress. Notably, the DS demonstrates larger variability for three modified asphalt mixtures, which is due to the error caused by sensor accuracy. Nonetheless, the maximum coefficient of variation of different modified asphalt mixtures is 16.5%, which is within 20%, so it is confirmed that the indicator DS is capable of reflecting the high-temperature performance of anti-rutting asphalt mixtures.
As described before, the ARA-A additive has the most desirable compatibility with the asphalt, followed by ARA-C and ARA-B, respectively. In most cases, the better compatibility implies more complete modification effects on the asphalt, thus leading to higher dynamic stability of the asphalt mixture. Nevertheless, three additives present differences in road performance. The main reasons could be traced back to the dry mixing process for preparing asphalt mixtures. The incorporation of ARAs into mixtures will not only interact with asphalt but also change their physical morphology [12], so ARAs mainly develop their functions via physical modification, instead of chemical reaction with aggregates.
Three ARAs in asphalt mixtures differ in their dynamic stability, which is mostly due to the various forms in which they are presented. The ARAs primarily achieve their function via two approaches. First, the melted ARA modifies the asphalt in a dispersed system. Second, the ARA, in a viscous-flow state, also has the effects of interlocking, filling voids, cementing and wrapping the aggregate, as presented in Figure 11. Accordingly, several stiff network structures are formed to confine the relative movement of mineral aggregates, as well as enhance the overall performance of the asphalt mixture.
The variation of road performance of different asphalt mixtures is due to the distribution form of ARAs. Large quantities of ARA-A additives are melted into the asphalt-additive system, so its leading intention is to improve the properties of asphalt. In contrast, a few quantities of ARA-C are melted into the asphalt, where it plays a more significant function in filling mineral voids and enhancing the overall performance of the asphalt mixture. More importantly, the latter has a greater impact on the dynamic stability of asphalt mixtures at a high temperature.
This study aimed to evaluate the effects of ARAs on the high-temperature performance of asphalt and asphalt mixtures. The FM test, MSCR test and wheel tracking test were conducted to reveal the performance of ARAs and their interactions with asphalt mixtures. The following main conclusions were drawn.
1) By incorporating ARA, a remarkable increase of recovery rate and a significant decrease of non-recoverable creep compliance were observed for the asphalt.
2) Fluorescent particles were uniformly presented with large quantities in the FM image of ARA-A modified asphalt, which implies good compatibility. The compatibility between the asphalt and various additives fulfill the sequence: ARA-A > ARA-C > ARA-B.
3) Due to differences in dispersion characteristics, the compatibility between the additive and the asphalt binder was not the only leading factor that correlated with their high-temperature anti-rutting performance.
4) ARAs hugely raise the dynamic stability of mixtures. Melted ARAs modify the asphalt to improve the performance of asphalt mixtures. Further, viscous-flow additives contribute to the modification of asphalt mixtures by interlock action, filling voids, cementing and wrapping the aggregate.
In the future, extensive research efforts will be made to investigate the comprehensive performances of asphalt mixtures due to the incorporation of ARAs. Further, developing a quantitative relationship between the performance and the distribution form of ARAs will be performed in the follow-up studies.
The authors declare they have not used Artificial Intelligence (AI) tools in the creation of this article.
This research was funded by the Opening Project of National Engineering Research Center of Advanced Road Materials (Grant No. 22990169).
The authors declare there is no conflict of interest.
[1] | S. Chakraborty, K. Mali, S. Chatterjee, S. Banerjee, A. Sah, S. Pathak, et al., Bio-medical image enhancement using hybrid metaheuristic coupled soft computing tools, in 2017 IEEE 8th Annual Ubiquitous Computing, Electronics and Mobile Communication Conference (UEMCON), (2017), 231–236. https://doi.org/10.1109/UEMCON.2017.8249036 |
[2] |
N. Du, Q. Luo, Y. Du, Y. Zhou, Color image enhancement: A metaheuristic Chimp optimization algorithm, Neural Process. Lett., 54 (2022), 4769–4808. https://doi.org/10.1007/s11063-022-10832-7 doi: 10.1007/s11063-022-10832-7
![]() |
[3] |
W. Wang, C. Zhang, Bifurcation of a feed forward neural network with delay and application in image contrast enhancement, Math. Biosci. Eng., 17 (2020), 387–403. https://doi.org/10.3934/mbe.2020021 doi: 10.3934/mbe.2020021
![]() |
[4] | S. Chakraborty, A. Raman, S. Sen, K. Mali, S. Chatterjee, H. Hachimi, Contrast optimization using elitist metaheuristic optimization and gradient approximation for biomedical image enhancement, in 2019 Amity International Conference on Artificial Intelligence (AICAI), (2019), 712–717. https://doi.org/10.1109/AICAI.2019.8701367 |
[5] |
M. J. Horry, S. Chakraborty, B. Pradhan, M. Fallahpoor, H. Chegeni, M. Paul, Factors determining generalization in deep learning models for scoring COVID-CT images, Math. Biosci. Eng., 18 (2021), 9264–9293. https://doi.org/10.3934/mbe.2021456 doi: 10.3934/mbe.2021456
![]() |
[6] |
R. Janarthanan, E. A. Refaee, K. Selvakumar, M. A. Hossain, R. Soundrapandiyan, M. Karuppiah, Biomedical image retrieval using adaptive neuro-fuzzy optimized classifier system, Math. Biosci. Eng., 19 (2022), 8132–8151. https://doi.org/10.3934/mbe.2022380 doi: 10.3934/mbe.2022380
![]() |
[7] |
J. R. Tang, N. A. M. Isa, Bi-histogram equalization using modified histogram bins, Appl. Soft Comput., 55 (2017), 31–43. https://doi.org/10.1016/j.asoc.2017.01.053 doi: 10.1016/j.asoc.2017.01.053
![]() |
[8] |
U. K. Acharya, S. Kumar, Genetic algorithm based adaptive histogram equalization (GAAHE) technique for medical image enhancement, Optik, 230 (2021), 166273. https://doi.org/10.1016/j.ijleo.2021.166273 doi: 10.1016/j.ijleo.2021.166273
![]() |
[9] |
T. Rahman, A. Khandakar, Y. Qiblawey, A. Tahir, S. Kiranyaz, S. B. A. Kashem, et al., Exploring the effect of image enhancement techniques on COVID-19 detection using chest X-ray images, Comput. Biol. Med., 132 (2021), 104319. https://doi.org/10.1016/j.compbiomed.2021.104319 doi: 10.1016/j.compbiomed.2021.104319
![]() |
[10] |
A. Qayyum, W. Sultani, F. Shamshad, R. Tufail, J. Qadir, Single-shot retinal image enhancement using untrained and pretrained neural networks priors integrated with analytical image priors, Comput. Biol. Med., 148 (2022), 105879. https://doi.org/10.1016/j.compbiomed.2022.105879 doi: 10.1016/j.compbiomed.2022.105879
![]() |
[11] |
R. Kumar, A. K. Bhandari, Spatial mutual information based detail preserving magnetic resonance image enhancement, Comput. Biol. Med., 146 (2022), 105644. https://doi.org/10.1016/j.compbiomed.2022.105644 doi: 10.1016/j.compbiomed.2022.105644
![]() |
[12] |
M. Jalali, H. Behnam, M. Shojaeifard, Echocardiography image enhancement using texture-cartoon separation, Comput. Biol. Med., 134 (2021), 104535. https://doi.org/10.1016/j.compbiomed.2021.104535 doi: 10.1016/j.compbiomed.2021.104535
![]() |
[13] |
K. G. Dhal, S. Ray, A. Das, S. Das, A survey on nature-inspired optimization algorithms and their application in image enhancement domain, Arch. Comput. Methods Eng., 26 (2019), 1607–1638. https://doi.org/10.1007/s11831-018-9289-9 doi: 10.1007/s11831-018-9289-9
![]() |
[14] | S. Goel, A. Verma, N. Kumar, Gray level enhancement to emphasize less dynamic region within image using genetic algorithm, in 2013 3rd IEEE International Advance Computing Conference (IACC), (2013), 1171–1176. https://doi.org/10.1109/IAdCC.2013.6514393 |
[15] |
S. Suresh, S. Lal, Modified differential evolution algorithm for contrast and brightness enhancement of satellite images, Appl. Soft Comput., 61 (2017), 622–641. https://doi.org/10.1016/j.asoc.2017.08.019 doi: 10.1016/j.asoc.2017.08.019
![]() |
[16] |
A. K. Bhandari, A. Kumar, S. Chaudhary, G. K. Singh, A new beta differential evolution algorithm for edge preserved colored satellite image enhancement, Multidimension. Syst. Signal Process., 28 (2017), 495–527. https://doi.org/10.1007/s11045-015-0353-4 doi: 10.1007/s11045-015-0353-4
![]() |
[17] |
H. K. Verma, S. Pal, Modified sigmoid function based gray scale image contrast enhancement using particle swarm optimization, J. Inst. Eng. India Ser. B, 97 (2016), 243–251. https://doi.org/10.1007/s40031-014-0175-z doi: 10.1007/s40031-014-0175-z
![]() |
[18] |
S. K. Ghosh, B. Biswas, A. Ghosh, A novel approach of retinal image enhancement using PSO system and measure of fuzziness, Procedia Comput. Sci., 167 (2020), 1300–1311. https://doi.org/10.1016/j.procs.2020.03.446 doi: 10.1016/j.procs.2020.03.446
![]() |
[19] |
H. Gao, W. Zeng, Color image enhancement based on Ant Colony Optimization Algorithm, Telkomnika, 13 (2015), 155–163. http://doi.org/10.12928/telkomnika.v13i1.1274 doi: 10.12928/telkomnika.v13i1.1274
![]() |
[20] | H. Singh, A. Kumar, L. K. Balyan, A sine-cosine optimizer-based gamma corrected adaptive fractional differential masking for satellite image enhancement, in Harmony Search and Nature Inspired Optimization Algorithms, Springer, (2019), 633–645. https://doi.org/10.1007/978-981-13-0761-4_61 |
[21] |
Y. Feng, S. Deb, G. G. Wang, A. H. Alavi, Monarch butterfly optimization: a comprehensive review, Expert Syst. Appl., 168 (2021), 114418. https://doi.org/10.1016/j.eswa.2020.114418 doi: 10.1016/j.eswa.2020.114418
![]() |
[22] |
S. Li, H. Chen, M. Wang, A. A. Heidari, S. Mirjalili, Slime mould algorithm: A new method for stochastic optimization, Future Gener. Comput. Syst., 111 (2020), 300–323. https://doi.org/10.1016/j.future.2020.03.055 doi: 10.1016/j.future.2020.03.055
![]() |
[23] |
Y. Feng, G. G. Wang, A binary moth search algorithm based on self-learning for multidimensional knapsack problems, Future Gener. Comput. Syst., 126 (2022), 48–64. https://doi.org/10.1016/j.future.2021.07.033 doi: 10.1016/j.future.2021.07.033
![]() |
[24] |
A. A. Heidari, S. Mirjalili, H. Faris, I. Aljarah, M. Mafarja, H. Chen, Harris hawks optimization: Algorithm and applications, Future Gener. Comput. Syst., 97 (2019), 849–872. https://doi.org/10.1016/j.future.2019.02.028 doi: 10.1016/j.future.2019.02.028
![]() |
[25] |
I. Ahmadianfar, A. A. Heidari, S. Noshadian, H. Chen, A. H. Gandomi, INFO: An efficient optimization algorithm based on weighted mean of vectors, Expert Syst. Appl., 195 (2022), 116516. https://doi.org/10.1016/j.eswa.2022.116516 doi: 10.1016/j.eswa.2022.116516
![]() |
[26] |
Y. Yang, H. Chen, A. A. Heidari, A. H. Gandomi, Hunger games search: Visions, conception, implementation, deep analysis, perspectives, and towards performance shifts, Expert Syst. Appl., 177 (2021), 114864. https://doi.org/10.1016/j.eswa.2021.114864 doi: 10.1016/j.eswa.2021.114864
![]() |
[27] |
J. Tu, H. Chen, M. Wang, A. H. Gandomi, The colony predation algorithm, J. Bionic Eng., 18 (2021), 674–710. https://doi.org/10.1007/s42235-021-0050-y doi: 10.1007/s42235-021-0050-y
![]() |
[28] |
H. Su, D. Zhao, A. A. Heidari, L. Liu, X. Zhang, M. Mafarja, et al., RIME: A physics-based optimization, Neurocomputing, 532 (2023), 183–214. https://doi.org/10.1016/j.neucom.2023.02.010 doi: 10.1016/j.neucom.2023.02.010
![]() |
[29] |
M. O. Oloyede, A. J. Onumanyi, H. Bello-Salau, K. Djouani, A. Kurien, Exploratory analysis of different metaheuristic optimization methods for medical image enhancement, IEEE Access, 10 (2022), 28014–28036. https://doi.org/10.1109/ACCESS.2022.3158324 doi: 10.1109/ACCESS.2022.3158324
![]() |
[30] |
J. Tang, J. Kim, E. Peli, Image enhancement in the JPEG domain for people with vision impairment, IEEE. Trans. Biomed. Eng., 51 (2004), 2013–2023. https://doi.org/10.1109/TBME.2004.834264 doi: 10.1109/TBME.2004.834264
![]() |
[31] |
J. Tang, X. Liu, Q. Sun, A direct image contrast enhancement algorithm in the wavelet domain for screening mammograms, IEEE. J. Sel. Top. Signal Process., 3 (2009), 74–80. https://doi.org/10.1109/JSTSP.2008.2011108 doi: 10.1109/JSTSP.2008.2011108
![]() |
[32] | X. Liu, J. Tang, X. Zhang, A multiscale image enhancement method for calcification detection in screening mammograms, in 2009 16th IEEE International Conference on Image Processing (ICIP), (2009), 677–680. https://doi.org/10.1109/ICIP.2009.5414077 |
[33] |
K. Lan, G. Li, Y. Jie, R. Tang, L. Liu, S. Fong, Convolutional neural network with group theory and random selection particle swarm optimizer for enhancing cancer image classification, Math. Biosci. Eng., 18 (2021), 5573–5591. https://doi.org/10.3934/mbe.2021281 doi: 10.3934/mbe.2021281
![]() |
[34] | S. Fong, K. Lan, P. Sun, S. Mohammed, J. Fiaidhi, A time-series pre-processing methodology for biosignal classification using statistical feature extraction, in Proceedings of the 10th IASTED International Conference on Biomedical Engineering (Biomed'13), (2013), 207–214. https://doi.org/10.2316/P.2013.791-100 |
[35] |
K. Lan, J. Zhou, X. Jiang, J. Wang, S. Huang, J. Yang, et al., Group theoretic particle swarm optimization for multi-level threshold lung cancer image segmentation, Quant. Imaging. Med. Surg., 13 (2023), 1312–1322. https://doi.org/10.21037/qims-22-295 doi: 10.21037/qims-22-295
![]() |
1. | Eyob Mersha Woldamanuel, Shonak Bansal, Hybrid Simulated Annealing‐Evaporation Rate‐Based Water Cycle Algorithm Application for Medical Image Enhancement, 2024, 2024, 2090-0147, 10.1155/2024/3328299 | |
2. | M.Y. Bogatyrev, 2024, 10, 2618-9305, 10.17537/icmbb24.47 | |
3. | Mohamed Abdel-Basset, Reda Mohamed, Mohamed Elhoseny, 2024, 9780443133145, 67, 10.1016/B978-0-443-13314-5.00009-6 | |
4. | Yibo Han, Pei Hu, Zihan Su, Lu Liu, John Panneerselvam, An Advanced Whale Optimization Algorithm for Grayscale Image Enhancement, 2024, 9, 2313-7673, 760, 10.3390/biomimetics9120760 | |
5. | İbrahim Yildirim, Mustafa Hakan Bozkurt, Hamdi Tolga Kahraman, Sefa Aras, Dental X-Ray image enhancement using a novel evolutionary optimization algorithm, 2025, 142, 09521976, 109879, 10.1016/j.engappai.2024.109879 |
Properties | Result | Technical standard | Test method |
Density (g∙cm-3) | 1.036 | - | T0603 |
Penetration (25℃, 0.1 mm) | 71 | 60~80 | T0604 |
Softening point (℃) | 47 | > 46 | T0606 |
Ductility (10℃, cm) | 76 | > 20 | T0605 |
Technical indices | Test results | requirements | Test methods |
Crushing value (%) | 21 | ≤ 26 | T 0316 |
Los Angeles Abrasion (%) | 16.8 | ≤ 28 | T 0317 |
Water absorption (%) | 1.5 | ≤ 2.0 | T 0304 |
Robustness (%) | 3.5 | ≤ 12 | T 0314 |
Soft stone content (%) | 1.2 | ≤ 3.0 | T 0320 |
Elongated particles content (%) | 10.5 | ≤ 15 | T 0312 |
Technical indices | Test results | Requirements | Test methods |
Mud content (%) | 2.1 | ≤ 3 | T 0333 |
Apparent relative density | 2.635 | ≥ 2.50 | T 0304 |
Sand equivalent (%) | 76.7 | ≥ 60 | T 0334 |
Robustness (%) | 4.2 | ≤ 12 | T 0340 |
Technical indices | Test result | Requirements | Test methods |
Density (g•cm-3) | 2.68 | > 2.5 | T0352 |
Hydrophilic coefficient | 0.60 | < 1 | T0353 |
Moisture content (%) | 0.18 | < 1 | T0103 |
BET specific surface area (m2/g) | 0.49 | - | - |
Properties | Result | Technical standard | Test method |
Density (g∙cm-3) | 1.036 | - | T0603 |
Penetration (25℃, 0.1 mm) | 71 | 60~80 | T0604 |
Softening point (℃) | 47 | > 46 | T0606 |
Ductility (10℃, cm) | 76 | > 20 | T0605 |
Technical indices | Test results | requirements | Test methods |
Crushing value (%) | 21 | ≤ 26 | T 0316 |
Los Angeles Abrasion (%) | 16.8 | ≤ 28 | T 0317 |
Water absorption (%) | 1.5 | ≤ 2.0 | T 0304 |
Robustness (%) | 3.5 | ≤ 12 | T 0314 |
Soft stone content (%) | 1.2 | ≤ 3.0 | T 0320 |
Elongated particles content (%) | 10.5 | ≤ 15 | T 0312 |
Technical indices | Test results | Requirements | Test methods |
Mud content (%) | 2.1 | ≤ 3 | T 0333 |
Apparent relative density | 2.635 | ≥ 2.50 | T 0304 |
Sand equivalent (%) | 76.7 | ≥ 60 | T 0334 |
Robustness (%) | 4.2 | ≤ 12 | T 0340 |
Technical indices | Test result | Requirements | Test methods |
Density (g•cm-3) | 2.68 | > 2.5 | T0352 |
Hydrophilic coefficient | 0.60 | < 1 | T0353 |
Moisture content (%) | 0.18 | < 1 | T0103 |
BET specific surface area (m2/g) | 0.49 | - | - |