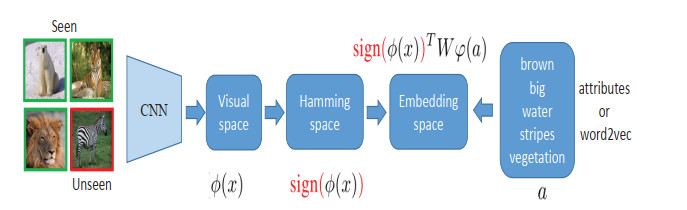
In this study, we introduced several types of higher-order difference equations involving q-SINE Euler (QSE) and q-COSINE Euler (QCE) polynomials. Depending on the parameters selected, these higher-order difference equations exhibited properties of trigonometric functions or related Euler numbers. Approximate root construction focused on the QSE polynomial, which was the solution of the q-difference equations obtained earlier. We also showed the structure of the approximate roots of higher-order polynomials among the QSE polynomials, understood them, and considered the associated conjectures.
Citation: Jung Yoog Kang, Cheon Seoung Ryoo. Exploring variable-sensitive q-difference equations for q-SINE Euler polynomials and q-COSINE-Euler polynomials[J]. AIMS Mathematics, 2024, 9(6): 16753-16772. doi: 10.3934/math.2024812
[1] | Yu Wang . Bi-shifting semantic auto-encoder for zero-shot learning. Electronic Research Archive, 2022, 30(1): 140-167. doi: 10.3934/era.2022008 |
[2] | Li Sun, Bing Song . Feature adaptive multi-view hash for image search. Electronic Research Archive, 2023, 31(9): 5845-5865. doi: 10.3934/era.2023297 |
[3] | Shixiong Zhang, Jiao Li, Lu Yang . Survey on low-level controllable image synthesis with deep learning. Electronic Research Archive, 2023, 31(12): 7385-7426. doi: 10.3934/era.2023374 |
[4] | Xingsi Xue, Miao Ye . Interactive complex ontology matching with local and global similarity deviations. Electronic Research Archive, 2023, 31(9): 5732-5748. doi: 10.3934/era.2023291 |
[5] | Hui-Ching Wu, Yu-Chen Tu, Po-Han Chen, Ming-Hseng Tseng . An interpretable hierarchical semantic convolutional neural network to diagnose melanoma in skin lesions. Electronic Research Archive, 2023, 31(4): 1822-1839. doi: 10.3934/era.2023094 |
[6] | Shuangjie Yuan, Jun Zhang, Yujia Lin, Lu Yang . Hybrid self-supervised monocular visual odometry system based on spatio-temporal features. Electronic Research Archive, 2024, 32(5): 3543-3568. doi: 10.3934/era.2024163 |
[7] | Guozhong Liu, Qiongping Tang, Changnian Lin, An Xu, Chonglong Lin, Hao Meng, Mengyu Ruan, Wei Jin . Semantic segmentation of substation tools using an improved ICNet network. Electronic Research Archive, 2024, 32(9): 5321-5340. doi: 10.3934/era.2024246 |
[8] | Wu Zeng, Heng-liang Zhu, Chuan Lin, Zheng-ying Xiao . A survey of generative adversarial networks and their application in text-to-image synthesis. Electronic Research Archive, 2023, 31(12): 7142-7181. doi: 10.3934/era.2023362 |
[9] | Chengyong Yang, Jie Wang, Shiwei Wei, Xiukang Yu . A feature fusion-based attention graph convolutional network for 3D classification and segmentation. Electronic Research Archive, 2023, 31(12): 7365-7384. doi: 10.3934/era.2023373 |
[10] | Jing Lu, Longfei Pan, Jingli Deng, Hongjun Chai, Zhou Ren, Yu Shi . Deep learning for Flight Maneuver Recognition: A survey. Electronic Research Archive, 2023, 31(1): 75-102. doi: 10.3934/era.2023005 |
In this study, we introduced several types of higher-order difference equations involving q-SINE Euler (QSE) and q-COSINE Euler (QCE) polynomials. Depending on the parameters selected, these higher-order difference equations exhibited properties of trigonometric functions or related Euler numbers. Approximate root construction focused on the QSE polynomial, which was the solution of the q-difference equations obtained earlier. We also showed the structure of the approximate roots of higher-order polynomials among the QSE polynomials, understood them, and considered the associated conjectures.
Content-based image retrieval (CBIR) has been widely studied in the past decade [1]. Due to computational and memory constraints, these methods are unable to deal with large-scale data. In recent years, the large-scale of and ever-growing nature of online image data makes approximate nearest neighbor (ANN) search popular in image semantic retrieval tasks[2,3,4,5]. For ANN search, most research efforts have been devoted to developing two promising binarization solutions, such as learning to hash (L2H) [6,7,8,9,10,11,12,13] and learning to quantization (L2Q) [4,5,14,15,16,17,18]. By encoding real-valued images into binary codes, hashing based methods or quantization based methods can achieve efficient storage and retrieval of image data in a large-scale database.
L2H based methods mainly aim to map high-dimensional data into a low-dimensional Hamming space while preserving the data similarities or the semantic information. L2Q based methods mainly aim to approximate feature representation using a quantizer (i.e., sign funciton) [4,5,11,14] or approximate the high-dimensional data with a set of learned quantizers (i.e., different codebooks) [15,16,17,18]. Recent studies [5,16,17,18] indicate that L2Q based methods perform generally better than L2H methods for image semantic retrieval tasks. The reason may be that L2Q methods can control the quantization error until the statistically minimized error is arrived. Therefore, L2Q methods can generate higher quality of binary codes than L2H methods. Generally speaking, the encoding time and retrieval efficiency of quantization methods are slightly more costly than hashing methods [16].
It should be noticed that existing ANN search approaches are based on the hypothesis that the concepts of both database samples and query samples are seen at the training stage. However, the hypothesis can be violated with the explosive growth of web data because a fast growing number of images with the new semantic concepts spring up on the web. For the fast growing new concepts, it seems almost impossible to annotate sufficient training data timely, and unrealistic to retrain the model over and over again. Existing ANN search approaches yield poor retrieval performance because they tend to recognize the images of unseen categories as one of the seen categories. Therefore, the generalization ability of the model is essential for solving the retrieval problem of the unseen concepts.
To alleviate the problem mentioned above, zero-shot learning (ZSL) techniques [19,20,21] assume both seen classes and unseen classes share a common semantic space where all the classes reside. The shared semantic space can be characterized by attributes [22], word2vec [23] or WordNet [24]. In the zero-shot classification task, the image classes in the training set and the test set are referred to as seen classes and unseen classes respectively. During the test phase, the image from the unseen class is assigned to the nearest class embedding vector in the shared space by a simple nearest neighbor search strategy. Although ZSL techniques have achieved progress in zero-shot image classification, zero-shot image retrieval has not yet been well explored.
Recently, zero-shot learning techniques have been introduced into learning to hash to improve the generalization ability of the hashing model [25]. SitNet [25] incorporates a semantic embedding loss and a regularized center loss into a multi-task architecture to capture the semantic structure in the semantic space. To facilitate knowledge transferring and reduce the quantization error in the training process, some quantization based methods [26,27] propose to simultaneously transfer the semantic information to binary codes and control the quantization error between low-dimensional feature representations and learned binary codes. However, a significant disadvantage of these methods is that the minimization of the quantization error in the training process is still unsatisfactory. Moreover, the inconsistency of the visual space and semantic space has not been considered sufficiently, which can increase the risk of the overfitting the seen classes and reduce the expansibility of the training model to the unseen classes [28]. Last but not least, the works in [26,27] utilize the semantic space as the embedding space, which means projecting the visual feature vectors or hash codes into the semantic space. This will shrink the variance of the projected data points and thus result in higher hubness (i.e., the projected data points will be closer to each other on average) [20]. In turn, the hubness problem in the semantic space can decrease the semantic transfer ability of the visual feature vectors or hash codes for the zero-shot image retrieval task.
In this paper, we propose a novel deep quantization network with visual-semantic alignment (VSAQ) for efficient zero-shot image retrieval. Specifically, we design a deep quantization network architecture which consists of the following components: 1) an image feature network to generate discriminative and polymeric image representations for facilitating the visual-semantic alignment and guiding the semantic embedding more easily; 2) a semantic embedding network to maximize the compatibility score between the image and semantic vectors for knowledge transfer; 3) a quantization loss layer to control the quantization error of image representation and generate high quality of binary codes for visual-semantic alignment and alleviating the hubness problem. We compare the proposed method with several state-of-the-art methods on several benchmark datasets and the experimental results validate the superiority of the proposed method.
The remainder of this paper is organized as follows: related work is reviewed in Section 2 and we illustrate the proposed method in Section 3. Evaluation on three commonly used benchmark datasets is described in Section 4, followed by conclusions in Section 5.
Due to the ever-growing amount of image data on the internet, hashing has become a popular technique for image retrieval. Generally, we can divide existing hashing approaches into two categories: data-independent and data-dependent hashing methods. Data-independent hashing methods map the data points from the original feature space into a binary code space by using random projections as hash functions. Representative data-independent hashing methods include Locality Sensitive Hashing (LSH) [3]. These methods provide theoretical guarantees for mapping the nearby data points into the same hash codes with high probabilities. However, they need long binary codes to achieve high precision. Data-dependent hashing methods learn hash functions and compact binary codes from training data. Typical data-dependent hashing methods include spectral hashing (SH) [6], anchor graph hashing (AGH) [7], supervised hashing with kernels (KSH) [8], supervised discrete hashing (SDH) [9] and column sampling based discrete supervised hashing (COSDISH) [10]. Recently, benefiting from the power of deep convolutional networks, deep hashing methods which integrate feature learning and hash-code learning into the same end-to-end framework have been proposed to further improve the semantic retrieval performance. Typical deep hashing methods include convolutional neural network hashing deep pairwise supervised hashing (DPSH) [5], deep supervised discrete hashing (DSDH) [29], deep supervised hashing (DSH) [13], and deep hashing network (DHN) [12]. Although there has been success in semantic image retrieval, most existing hashing methods fail on zero-shot image retrieval, due to the low generalization ability of learned hashing models for unseen concepts.
Quantization-based methods attempt to control the quantization error of the feature representations using a quantizer (i.e., sign funciton) [4,5,11,14,30] or approximate the high-dimensional data with a set of learned quantizers (i.e., different codebooks) [15,16,17,18]. For example, [4,5,14] and [30] try to minimize the Euclidean distance and the cosine distance between continuous representations and their signed binary codes respectively. Alternatively, [11] utilizes a sequence of smoothing activation functions to gradually approach the sign function. Although the quantization error can be controlled using a single quantizer, it is not statistically minimized for generating high-quality binary codes. To further reduce the quantization error, [15,16,17,18] utilize the vector quantization (VQ) technique [31] to improve the accuracy and efficiency of the quantification process. Benefiting from the power of VQ, the retrieval performance has been improved significantly. However, these methods focus on traditional image retrieval (i.e., the concepts of all samples are seen in the training set), and how to integrate them into zero-shot image retrieval is still an open problem.
Zero-shot learning recognizes unseen or novel classes that did not appear in the training stage [19,20,21]. The zero-shot learning framework learns a compatible visual-semantic embedding space and utilizes the learned embedding space as an intermediate to accomplish the zero-shot image classification task. The method in [20] utilizes a latent space as the visual-semantic embedding space and introduces the least square loss between the embedded visual features and the embedded semantic vectors to cope with the hubness problem. The method in [21] utilizes the semantic space as the visual-semantic embedding space and introduce an image feature structure constraint and a semantic embedding structure constraint to learn structure-preserving image features and improve the generalization ability of the learned embedding space respectively. Recently, some works [25,26,27] attempt to utilize the zero-shot learning for solving the zero-shot image retrieval problem. The method in [26] projects the binary codes to the semantic space with the ridge regression formulation, which can exacerbate the hubness problem. However, the quantization error is not statistically minimized and the inconsistency of the visual space and semantic space has not been considered sufficiently.
We follow the definition of zero-shot image retrieval in [25,26]. The training set is defined as S≡{xsi,ysi,asi}nsi=1. Each image xsi∈XS is associated with a corresponding class label ysi∈YS. Similarly, the test set is defined as U≡{xuj,yuj,auj}nui=j. Each image xuj∈XU is associated with a corresponding class label yuj∈YU. The side information matrix A∈Rr×(|YS|+|YU|) is obtained from the user-defined attributes or word2vec to transfer knowledge across concepts. The side information of image xsi can be denoted as asi=Aysi, which corresponds to the ysi-th column of A. According to the setting of zero-shot learning, YS∩YU=∅, i.e., the seen classes are disjoint from the unseen classes. The goal of zero-shot hashing is to predict the binary codes of images from both seen classes and unseen classes.
As illustrated in Figure 2, the proposed architecture mainly consists of three different components: 1) the image feature network (FNet) for learning discriminative and polymeric image representations; 2) the embedding network (Enet) for learning an embedding space to associate the visual information with the semantic information; and 3) the quantization loss layer for controlling coding quality, aligning the visual and semantic information and alleviating the hubness problem.
The image feature network (FNet) aims to learn the semantic image representations with discrimination and polymerization. We adopt AlexNet [32] as the base network using the layers from conv1 to fc7 and replace fc8 with a q-dimensional fully-connected layer (4096-128). In addition, the tanh(⋅) activation function and an L2 Normalization Layer are added to enhance the nonlinear representation ability and constrain the range of the output features. Inspired by [33], a variant of the softmax loss is utilized to increase the discrimination of inter-class features and the compactness of intra-class features as follows:
Lf=−1nn∑i=1logexp(γ1⟨ϕf(xsi),ˆck⟩)|YS|∑j=1exp(γ1⟨ϕf(xsi),ˆcj⟩) | (3.1) |
where ˆcj denotes the centroid of the features associated with the j-th class, and γ1 is set to 10 in all experiments. The ϕf(x) refers to the output of the FNet. Under the guidance of the label information YS of the seen classes, the FNet can learn semantic-preserving image representations. In addition, the following image embedding network can learn the visual-semantic embedding space more easily with the help of such semantic-preserving image representations. Finally, it can assist the visual-semantic alignment more easily.
The embedding network (ENet) aims to learn an embedding space to associate the visual information with the semantic information. According to most of previous ZSL methods, we utilize the semantic space of A as the visual-semantic embedding space, i.e., projecting the outputs of FNet into the semantic space. Therefore, the ENet is constructed by an r-dimensional fully-connected layer (128-d) followed by the tanh(⋅) activation function and an L2 Normalization Layer, where r denotes the length of the semantic vectors. We use the following inner product to define the compatibility score between the visual embedding ϕe(x) and the semantic vector ay. Similar to traditional image classification tasks, we replace the classification score with the compatibility score in the following softmax loss:
Le=−1nn∑i=1logexp(γ2⟨ϕe(xsi),ˆask⟩)|AS|∑j=1exp(γ2⟨ϕe(xsi),ˆasj⟩) | (3.2) |
where ϕe(xsi) denotes the output of the ENet, ˆasj denotes the L2-normalized side information (attribute or word2vec) associated with the j-th class and γ2 is set to 10 in all experiments.
The acquirement of the semantic information is independent of visual samples. Therefore, the class structures between the visual space and semantic space are usually inconsistent. For example, the concepts of 'cat' and 'dog' locate quite close to each other in the semantic space, while the appearance features of 'cat' and 'dog' are far away from each other in the visual space. If we only use the semantic space as the visual-semantic embedding space, the mapped visual embeddings can be collapsed to hubs [34], i.e., nearest neighbours to many other projected visual feature representation vectors. To alleviate the hubness problem, we map the semantic information to the visual space and align the projected semantic vectors with the visual features in the visual space using a collective quantization framework.
Specifically, we use a matrix W∈Rr×q to map the L2-normalized semantic vectors to the visual space. The semantic image representations ϕf(xi) and the corresponding mapped semantic vectors WTˆaj are quantized using two codebooks C=[C1,⋯,CM] and D=[D1,⋯,DM] respectively. Each sub-codebook Cm (or Dm) consists of K codewords Cm=[Cm1,⋯,CmK] where the k-th codeword Cmk corresponds to a q-dimensional vector. The basic idea for visual-semantic alignment is to learn two codebooks to quantize the visual features and the corresponding mapped semantic vectors into binary codes and enforce the binary codes to be the same between them. The loss function can be written as:
Lq=1nn∑i=1‖ϕf(xsi)−M∑m=1Cmbmi‖2+1nn∑i=1(‖WTˆAysi−M∑m=1Dmbmi‖2+λ‖W‖2),s.t.,‖bmi‖0=1,bmi∈{0,1}K, | (3.3) |
where λ>0 is a balancing parameter, and ˆAysi is the L2-normalized semantic vector of i-th image. ‖⋅‖0 refers to the ℓ0-norm which returns the number of the vector's non-zero values. The constraint indicates that {bmi}Km=1 are the one-of-K encodings which means only one of the codeword per sub-codebook in codebooks C and D can be activated to approximate the semantic image representations ϕf(x) and the corresponding mapped semantic vectors WTˆaj. Each one-of-K encodings {bmi}Mm=1 can be compressed in log2K bits. We can obtain compact binary codes with B=Mlog2K bits by concatenating all M compressed encodings. The one-of-K encodings {bmi}Mm=1 play the key role to align the visual space and the semantic space, thus the consistency of the class structures can be guaranteed in the two spaces.
The final objective function for training the whole network is constructed by aggregating all the loss functions as follows:
L=Lf+αLe+βLq | (3.4) |
where α and β are two hyperparameters to balance the influence of different terms.
Approximate nearest neighbor search with the inner product distance is a powerful tool for quantization techniques. Given an unseen image query xq and the binary codes of database points {bn=[b1n;⋯;bMn]}Nn=1, we first use the trained image feature network to obtain the image representations. Following the asymmetric search method in [16,17,18], we adopt the asymmetric quantizer distance (AQD) to compute the inner-product similarity between the unseen query xuq and database point xn as follows:
AQD(xuq,xn)=M∑m=1ϕf(xuq)T(M∑m=1Cmbmn) | (3.5) |
where ∑Mm=1Cmbmn is used to approximate the image representation of the database point xn. Given an unseen query xuq, the inner-products between ϕf(xuq) and all M codebooks {Cm}Mm=1 and all K possible values of bmn can be pre-computed and stored in a M×K lookup table. Therefore, the computation of AQD between the unseen query and all database points can be speed up. Considering computational complexity, it is slightly more costly than the Hamming distance, since M table lookups and additions are involved.
The optimization problem contains four sets of variables including the network parameters Θ, the centroid of the features ˆC={ˆc1,⋯,ˆc|YS|}, the projection matrix W, the codebooks C and D, and the binary codes B=[b1,⋯,bn]. In the following optimization process, we adopt an alternating optimization strategy that updates one variable while holding fixed all other variables iteratively.
Updating Θ. We adopt the standard back-propagation algorithm with automatic differentiation techniques in Pytorch [35] to update the network parameters Θ.
Updating ˆC. We can update {ˆci}|YS|i=1 as follows:
ˆcj=1|{ysi∈j}nsi=1|∑ysi∈jϕf(xsi) | (4.1) |
where {ysi∈j}nsi=1 denotes the set of samples from class j.
Updating W. We can update the projection matrix W by optimizing the following subproblem
minWn∑i=1‖WTˆAysi−M∑m=1Dmbmi‖2+λ‖W‖2. | (4.2) |
We can obtain an analytic solution for this unconstrained quadratic problem as follows:
W=(ˆAYSYSTˆAT+λI)−1ˆAYSBTDT | (4.3) |
where YS=[ys1,⋯,ysn]∈{0,1}(|YS|+|YU|)×n is the label matrix of training images with each column corresponding to a one-hot vector and I is an identity matrix.
Updating C. We rewrite the optimization problem w.r.t. the dictionary C in matrix formulation as follows:
minC‖Φf−CB‖2 | (4.4) |
where Φf=[ϕf(xs1),⋯,ϕf(xsn)]. We can update C with the following analytic solution
C=ΦfBT(BBT)−1. | (4.5) |
Updating D. Similarly to the update method for C, we can update D with the following analytic solution
D=WTˆAYSBT(BBT)−1. | (4.6) |
Updating B. We can decompose the optimization problem for B into n subproblems, since {bi}ni=1 are independent of each other. For bi, the subproblem can be written as
minbi‖ϕf(xsi)−M∑m=1Cmbmi‖2+‖WTˆAysi−M∑m=1Dmbmi‖2 | (4.7) |
which can be further simplified as
minbi‖[ϕf(xsi)WTˆAysi]−M∑m=1[CmDm]bmi‖2. | (4.8) |
Generally, the above optimization problem is NP-hard. We adopt the iterated conditional modes (ICM) algorithm [36] to solve M indicators {bmi}Mm=1 alternatively. Specifically, fixing {bm′i}m′≠m, we check all the elements in [CmDm] exhaustively and find the element such that the obective function is minimized. Then, the corresponding entry of bmi is updated to 1 and the rest is updated to 0. The ICM algorithm is guaranteed to converge until the maximum iterations reached. The algorithm is summarized in Algorithm 1.
Algorithm 1 VSAQ algorithm |
Input: Training set S≡{xsi,ysi,asi}nsi=1; Output: Parameter Θ of the deep neural networks. Initialization: Initialize network parameter Θ, mini-batch size M, the iteration number T; 1: for epoch=1,2,…,T do 2: Update W according to Eq (4.2); 3: Update C according to Eq (4.5); 4: Update D according to Eq (4.6); 5: Update B according to Eq (4.8); 6: Update the parameter Θ by using backpropagation; 7: end for |
We evaluate and compare the proposed method with state-of-the-art baselines on several benchmark datasets. The proposed method is implemented with the open-source deep learning toolbox Pytorch [35]. All the experiments are carried out on a server with an Intel(R) Xeon(R) E5-2620 v4@2.10GHz CPU, 128GB RAM and two GeForce TITAN X GPUs with 24GB memory.
Three widely used datasets including Animals with Attributes [37], CIFAR10 [32] and ImageNet [38] are adopted to evaluate the proposed method and other baselines.
Animals with Attributes: contains 30,475 images from 50 animal categories. Each class is provided with 85 semantic attributes.
CIFAR-10: consists of 60,000 color images. The image size is 32×32 pixels. Each image is associated with one of the ten classes with each class containing 6000 images.
ImageNet: consists of 1.2 million images labeled with 1000 categories/synsets for the Large Scale Visual Recognition Challenge 2012 (ILSVRC2012).
Following the settings in [25,26], we construct the zero-shot scenario by splitting the benchmark datasets into seen classes and unseen classes. Specifically, for the Animals with Attributes (AwA) dataset, we randomly split the 50 animal categories into five groups with each group containing ten categories. In turn, we use one group as the unseen classes and the remaining groups as the seen classes. Therefore, we can obtain 5 different seen-unseen splits. We utilize 85-dim attribute vectors as the semantic vector. For the CIFAR10 dataset, we use one category as the unseen class and the remaining categories as the seen classes. Consequently, we can obtain 10 different seen-unseen splits. The 300-dimensional semantic vector is extracted from class names using the word2vec tool. For the ImageNet dataset, we randomly select a subset of ImageNet with 100 categories, which gives us about 130,000 images for evaluation. The 100 selected categories have the semantic vector from word2vec. We use 10 categories as seen classes and the remaining 90 categories as unseen classes, and thus we can obtain 10 different seen-unseen splits. Similar to CIFAR10, we use the word2vec tool to extract 300-dimensional semantic vectors from class names. For all three datasets, we randomly take 1000 images from the unseen categories as the query set. The remaining images from the remaining unseen categories images and all the seen categories images are treated as the retrieval database. For training, we randomly select 10,000 images from the seen categories as the training set.
We use the widely used mean Average Precision (mAP) based on Hamming ranking as the evaluation metric. The final experimental results are averaged over the different seen-unseen splits for all datasets.
We compare the proposed method with the following state-of-the-art hashing methods. These methods fall into two categories: 1) Hashing methods for traditional image retrieval: Iterative Quantization (ITQ) [4], supervised discrete hashing (SDH) [9], deep pairwise supervised hashing (DPSH) [5], deep supervised discrete hashing (DSDH) [29]; 2) zero-shot hashing methods: zero-shot hashing via transferring supervised knowledge (TSK) [26] and zero-shot hashing with discrete similarity transfer network (SitNet) [25]. We implement SitNet with Pytorch by ourselves. For the other compared methods, we adopt the public codes and suggested parameters from the their papers. For the non-CNN hashing methods, we adopt the pre-trained AlexNet model for extracting the 4096-dimensional CNN features as image representations for fair comparison.
We implement the VSAQ model via Pytorch. For the Animals with Attributes and CIFAR-10 datasets, the initial learning rate was set to 0.001. For the ImageNet dataset, the initial learning rate was set to 0.01. As the last fully connected layers in FNet and ENet are training from scratch, the learning rates of these layers are set to 10 times the other layers. We set the batch size to 128 and train the model for 10 epochs. The dimension of image representations q is set to 128 following [17]. The hyperparameters are set as α=1,β=10,λ=0.01 across all the following experiments.
The zero-shot image retrieval performances on AwA in terms of MAP with respect to different code lengths (i.e., {8,16,32,48}) are shown in Table 1. We find that our VSAQ method outperforms all other baseline methods by a large margin in terms of MAP, especially from 8 to 32 bits. In addition, we find that the unsupervised hashing method ITQ achieves comparable results with some supervised hashing method SDH. This demonstrates that the generalization ability of existing supervised hashing is limited for unseen concepts. The existing state-of-the-art deep hashing methods, including DPSH and DSDH, perform poorly on the zero-shot retrieval task over the AwA dataset. The main reason can be that the trained CNN compatible with the label information can fall into the risk of overfitting the seen classes, which reduces the expansibility of the training model to the unseen classes. We also find that TSK performs worse, especially at the lower bits (e.g., 8 and 16 bits). The main reason is that the hubness problem is exacerbated by projecting the binary codes to the semantic space with the ridge regression formulation [20], which will decrease the semantic transfer ability of hash codes in turn. To alleviate such a problem, the proposed VSAQ model utilizes the visual space as the embedding space for learning compact binary codes. In addition, we adopt a collective quantization technique for visual-semantic alignment which can improve the generalization ability of the proposed model.
Method | AwA | |||
8 bits | 16 bits | 32 bits | 48 bits | |
ITQ | 0.0886 | 0.1359 | 0.1723 | 0.2024 |
SDH | 0.0966 | 0.1370 | 0.1835 | 0.2122 |
DPSH | 0.0726 | 0.1080 | 0.1435 | 0.1525 |
DSDH | 0.0808 | 0.1081 | 0.1320 | 0.1469 |
TSK | 0.0349 | 0.0591 | 0.1320 | 0.1617 |
SitNet | 0.1036 | 0.1651 | 0.1870 | 0.2121 |
VSAQ | 0.1948 | 0.2099 | 0.2187 | 0.2218 |
The performances of the proposed VSAQ and other baselines on CIFAR-10 with different code length are illustrated in Table 2. From Table 2, we can find that VSAQ consistently outperforms other baselines at all bits by a large margin. For example, VSAQ surpasses SitNet with the second best performance by 3 to 4 percent. Even though the code length is short, VSAQ still achieves superior retrieval performance compared to the baselines with longer code length. It can be attributed to the lower quantization error controlled by the quantization technique. The deep hashing methods DPSH and DSDH perform better than the non-deep hashing methods ITQ and SDH, which demonstrates that CNNs can utilize the proper supervision to discover the complicated semantic similarity structure. VSAQ utilizes the label information to learn the semantic image representations with discriminative and polymeric structure, which can assist the visual-semantic alignment more easily. The unsupervised hashing mehtod ITQ achieves comparable performance with TSK which demonstrates that the generalization ability degenerates due to the existing hubness problem.
Method | CIFAR-10 | |||
8 bits | 16 bits | 32 bits | 48 bits | |
ITQ | 0.1507 | 0.1736 | 0.1871 | 0.1972 |
SDH | 0.1226 | 0.1331 | 0.1553 | 0.2068 |
DPSH | 0.2176 | 0.2205 | 0.2280 | 0.2261 |
DSDH | - | - | - | - |
TSK | 0.1507 | 0.1759 | 0.1740 | 0.2132 |
SitNet | 0.2208 | 0.2303 | 0.2351 | 0.2471 |
VSAQ | 0.2615 | 0.2682 | 0.2670 | 0.2867 |
The performances of the proposed VSAQ and other baselines on ImageNet with different code length are demonstrated in Table 3. As we can see, the proposed VSAQ model outperforms the baseline approaches by significant margins. For example, VSAQ surpasses SitNet with the second best performance by 2 to 9 percent. It clearly demonstrates that the VSAQ model generalizes better for unseen concepts compared with other state-of-the-art methods, which validates the effectiveness of the proposed method for zero-shot image retrieval.
Method | ImageNet | |||
8 bits | 16 bits | 32 bits | 48 bits | |
ITQ | 0.0507 | 0.0732 | 0.1123 | 0.1357 |
SDH | 0.0400 | 0.0727 | 0.1107 | 0.1312 |
DPSH | 0.0409 | 0.0524 | 0.0712 | 0.0881 |
DSDH | - | - | - | - |
TSK | 0.0162 | 0.0206 | 0.0247 | 0.0609 |
SitNet | - | - | - | - |
VSAQ | 0.1472 | 0.1516 | 0.1579 | 0.1614 |
The proposed VSAQ model consists of three components: an image feature loss layer Lf for learning discriminative and polymeric image representations, a semantic embedding loss layer Le for maximizing the compatibility score between the image and semantic vectors for knowledge transfer, and a quantization loss layer Lq for visual-semantic alignment. The quantization loss layer Lq is an essential part of generating binary codes. To study the contribution of different components for the zero-shot image retrieval performance, we compare the proposed method with the following submodels: 1) Lf+Lq (VSQA-1); 2) Le+Lq (VSQA-2); 3) Lf+Le+L1q (VSQA-3), where L1q refers to the first term in Eq (3.3), i.e., only considering the visual features for quantization. Table 4 illustrates the experimental results of different submodels. From Table 4, we can see that the combination of the image feature loss, the semantic embedding loss and the quantization loss achieves the best performance. The results demonstrate that the proposed framework improves the zero-shot image retrieval performance indeed. Comparing the performance of VSQA-3 and VSQA, we can find that the visual-semantic alignment will help the knowledge transfer from the seen concepts to the unseen concepts. Though the comparisons of VSQA-2 and VSQA, we can find that the discriminative and polymeric image representations will improve the performance a lot, which means that it can assist the visual-semantic alignment and semantic embedding more easily. Comparing the performance of VSQA-1 and VSQA, we can find that the knowledge transfer ability can be significantly improved by the semantic embedding.
Method | AwA | CIFAR-10 | ImageNet | |||||||||
12 bits | 24 bits | 32 bits | 48 bits | 12 bits | 24 bits | 32 bits | 48 bits | 12 bits | 24 bits | 32 bits | 48 bits | |
VSAQ | 0.1948 | 0.2099 | 0.2187 | 0.2218 | 0.2615 | 0.2682 | 0.2670 | 0.2867 | 0.1472 | 0.1516 | 0.1579 | 0.1614 |
VSAQ-1 | 0.1830 | 0.1849 | 0.1923 | 0.2012 | 0.2360 | 0.2487 | 0.2538 | 0.2539 | 0.1288 | 0.1319 | 0.1386 | 0.1497 |
VSAQ-2 | 0.1911 | 0.1956 | 0.2026 | 0.2089 | 0.2412 | 0.2533 | 0.2613 | 0.2665 | 0.1296 | 0.1365 | 0.1463 | 0.1495 |
VSAQ-3 | 0.1816 | 0.1736 | 0.1825 | 0.1998 | 0.2278 | 0.2324 | 0.2405 | 0.2487 | 0.1053 | 0.1150 | 0.1194 | 0.1256 |
In this paper, we propose a novel deep quantization network with visual-semantic alignment for efficient zero-shot image retrieval. In the proposed deep architecture, we use the label information and the sematic vector to supervise the image feature extraction and improve the compatibility between the image representations and the semantic vectors respectively. The semantic vectors are mapped to the visual space and aligned with the corresponding image representations via a collective quantization framework for alleviating the hubness problem. The experimental results on three datasets show that the proposed model outperforms the state-of-the-art methods on zero-shot image retrieval tasks. In the future work, we will investigate the zero-shot multi-label image (i.e., an image is assigned with multiple categories) retrieval task.
I would like to thank all anonymous reviewers for their constructive comments through each stage of the process.
All authors declare that they have no conflicts of interest.
[1] | W. H. Abdi, On q-Laplace transforms, Proc. Natl. Acad. Sci. India, 29 (1961), 389–408. |
[2] | G. Bangerezako, An Introduction to q-Difference Equations, Preprint, Bujumbura: University of Burundi, 2007. |
[3] | L. Carlitz, q-Bernoulli numbers and polynomials, Duke Math. J., 15 (1948), 987–1000. |
[4] | L. Carlitz, q-Bernoulli and Eulerian numbers, Trans. Amer. Math. Soc., 76 (1954), 332–350. |
[5] | T. Ernst, A Comprehensive Treatment of q-Calculus, New York: Springer Science, Business Media, 2012. |
[6] | J. A. Ganie, A. A. Bhat, S. A. Wani, Natural transform of two variables in q-calculus with applications, Bollettino dell'Unione Matematica Italian, 17 (2024), 101–117. |
[7] | R. N. Goldman, P. Simenov, Y. Simsek, Generating Functions For The Q-Bernstein Bases, Siam J. Discrete Math., 28 (2014), 1009–1025. |
[8] | H. F. Jackson, q-Difference equations, Am. J. Math., 32 (1910), 305–314. |
[9] | H. F. Jackson, On q-functions and a certain difference operator, Earth Environ. Sci. Trans. R. Soc. Edinb., 46 (2013), 253–281. |
[10] | A. Kemp, Certain q-analogues of the binomial distribution, Sankhya Indian J. Stat. Ser. A, 64 (2002), 293–305. |
[11] | N. Kilar, Y. Simsek, Computational formulas and identities for new classes of Hermite-based Milne–Thomson type polynomials: Analysis of generating functions with Euler's formula, Math. Appl. Sci., 44 (2021), 6731–6762. |
[12] | N. Kilar, Y. Simsek, H. M. Srivastava, Recurrence relations, associated formulas, and combinatorial sums for some parametrically generalized polynomials arising from an analysis of the Laplace transform and generating functions, Ramanujan J., 61 (2023), 731–756. |
[13] |
N. Kilar, Y. Simsek, Identities and relations for Hermite-based Milne–Thomson polynomials associated with Fibonacci and Chebyshev polynomials, RACSAM, 115 (2021), 28. https://doi.org/10.1007/s13398-020-00968-3 doi: 10.1007/s13398-020-00968-3
![]() |
[14] |
J. Konvalina, A unified interpretation of the binomial coefficients, the Stirling numbers, and the Gaussian coefficients, Am. Math. Mon., 107 (2000), 901–910. https://doi.org/10.1080/00029890.2000.12005290 doi: 10.1080/00029890.2000.12005290
![]() |
[15] |
T. Kim, C. S. Ryoo, Some identities for Euler and Bernoulli polynomials and their zeros, Axioms, 7 (2018), 56. https://doi.org/10.3390/axioms7030056 doi: 10.3390/axioms7030056
![]() |
[16] |
J. Y. Kang, C. S. Ryoo, Various structures of the roots and explicit properties of q-cosine Bernoulli polynomials and q-sine Bernoulli polynomials, Mathematics, 8 (2020), 463. https://doi.org/10.3390/math8040463 doi: 10.3390/math8040463
![]() |
[17] | Q. Luo, H. M. Srivastava, q-extensions of some relationships between the Bernoulli and Euler polynomials, Taiwan. J. Math., 15 (2011), 241–257. |
[18] |
S. Picoli Jr., R. S. Mendes, L. C. Malacarne, R. P. B. Santos, q-distributions in complex systems: A brief review, Braz. J. Phys., 39 (2009), 468–474. https://doi.org/10.1590/S0103-97332009000400023 doi: 10.1590/S0103-97332009000400023
![]() |
[19] |
C. S. Ryoo, J. Y. Kang, Explicit Properties of q-Cosine and q-Sine Euler Polynomials Containing Symmetric Structures, Symmetry, 12 (2020), 1274. https://doi.org/10.3390/sym12081247 doi: 10.3390/sym12081247
![]() |
[20] |
C. S. Ryoo, J. Y. Kang, Various Types of q-Differential Equations of Higher Order for q-Euler and q-Genocchi Polynomials, Mathematics, 10 (2022), 1181. https://doi.org/10.3390/math10071181 doi: 10.3390/math10071181
![]() |
[21] |
P. S. Rodrigues, G. Wachs-Lopes, R. M. Santos, E. Coltri, G. A. Giraldi, A q-extension of sigmoid functions and the application for enhancement of ultrasound images, Entropy, 21 (2019), 430. https://doi.org/10.3390/e21040430 doi: 10.3390/e21040430
![]() |
[22] | E. Schrödinger, A method for determining quantum mechanical eigenvalues and eigenfunctions, Proc. Roy. Irish. Acad. A, 46 (1940), 9–16. |
[23] | K. Victor, C. Pokman, Quantum Calculus Universitext, New York: Springer, 2002. |
1. | Huadong Sun, Zhibin Zhen, Yinghui Liu, Xu Zhang, Xiaowei Han, Pengyi Zhang, Embedded Zero-Shot Image Classification Based on Bidirectional Feature Mapping, 2024, 14, 2076-3417, 5230, 10.3390/app14125230 |
Method | AwA | |||
8 bits | 16 bits | 32 bits | 48 bits | |
ITQ | 0.0886 | 0.1359 | 0.1723 | 0.2024 |
SDH | 0.0966 | 0.1370 | 0.1835 | 0.2122 |
DPSH | 0.0726 | 0.1080 | 0.1435 | 0.1525 |
DSDH | 0.0808 | 0.1081 | 0.1320 | 0.1469 |
TSK | 0.0349 | 0.0591 | 0.1320 | 0.1617 |
SitNet | 0.1036 | 0.1651 | 0.1870 | 0.2121 |
VSAQ | 0.1948 | 0.2099 | 0.2187 | 0.2218 |
Method | CIFAR-10 | |||
8 bits | 16 bits | 32 bits | 48 bits | |
ITQ | 0.1507 | 0.1736 | 0.1871 | 0.1972 |
SDH | 0.1226 | 0.1331 | 0.1553 | 0.2068 |
DPSH | 0.2176 | 0.2205 | 0.2280 | 0.2261 |
DSDH | - | - | - | - |
TSK | 0.1507 | 0.1759 | 0.1740 | 0.2132 |
SitNet | 0.2208 | 0.2303 | 0.2351 | 0.2471 |
VSAQ | 0.2615 | 0.2682 | 0.2670 | 0.2867 |
Method | ImageNet | |||
8 bits | 16 bits | 32 bits | 48 bits | |
ITQ | 0.0507 | 0.0732 | 0.1123 | 0.1357 |
SDH | 0.0400 | 0.0727 | 0.1107 | 0.1312 |
DPSH | 0.0409 | 0.0524 | 0.0712 | 0.0881 |
DSDH | - | - | - | - |
TSK | 0.0162 | 0.0206 | 0.0247 | 0.0609 |
SitNet | - | - | - | - |
VSAQ | 0.1472 | 0.1516 | 0.1579 | 0.1614 |
Method | AwA | CIFAR-10 | ImageNet | |||||||||
12 bits | 24 bits | 32 bits | 48 bits | 12 bits | 24 bits | 32 bits | 48 bits | 12 bits | 24 bits | 32 bits | 48 bits | |
VSAQ | 0.1948 | 0.2099 | 0.2187 | 0.2218 | 0.2615 | 0.2682 | 0.2670 | 0.2867 | 0.1472 | 0.1516 | 0.1579 | 0.1614 |
VSAQ-1 | 0.1830 | 0.1849 | 0.1923 | 0.2012 | 0.2360 | 0.2487 | 0.2538 | 0.2539 | 0.1288 | 0.1319 | 0.1386 | 0.1497 |
VSAQ-2 | 0.1911 | 0.1956 | 0.2026 | 0.2089 | 0.2412 | 0.2533 | 0.2613 | 0.2665 | 0.1296 | 0.1365 | 0.1463 | 0.1495 |
VSAQ-3 | 0.1816 | 0.1736 | 0.1825 | 0.1998 | 0.2278 | 0.2324 | 0.2405 | 0.2487 | 0.1053 | 0.1150 | 0.1194 | 0.1256 |
Method | AwA | |||
8 bits | 16 bits | 32 bits | 48 bits | |
ITQ | 0.0886 | 0.1359 | 0.1723 | 0.2024 |
SDH | 0.0966 | 0.1370 | 0.1835 | 0.2122 |
DPSH | 0.0726 | 0.1080 | 0.1435 | 0.1525 |
DSDH | 0.0808 | 0.1081 | 0.1320 | 0.1469 |
TSK | 0.0349 | 0.0591 | 0.1320 | 0.1617 |
SitNet | 0.1036 | 0.1651 | 0.1870 | 0.2121 |
VSAQ | 0.1948 | 0.2099 | 0.2187 | 0.2218 |
Method | CIFAR-10 | |||
8 bits | 16 bits | 32 bits | 48 bits | |
ITQ | 0.1507 | 0.1736 | 0.1871 | 0.1972 |
SDH | 0.1226 | 0.1331 | 0.1553 | 0.2068 |
DPSH | 0.2176 | 0.2205 | 0.2280 | 0.2261 |
DSDH | - | - | - | - |
TSK | 0.1507 | 0.1759 | 0.1740 | 0.2132 |
SitNet | 0.2208 | 0.2303 | 0.2351 | 0.2471 |
VSAQ | 0.2615 | 0.2682 | 0.2670 | 0.2867 |
Method | ImageNet | |||
8 bits | 16 bits | 32 bits | 48 bits | |
ITQ | 0.0507 | 0.0732 | 0.1123 | 0.1357 |
SDH | 0.0400 | 0.0727 | 0.1107 | 0.1312 |
DPSH | 0.0409 | 0.0524 | 0.0712 | 0.0881 |
DSDH | - | - | - | - |
TSK | 0.0162 | 0.0206 | 0.0247 | 0.0609 |
SitNet | - | - | - | - |
VSAQ | 0.1472 | 0.1516 | 0.1579 | 0.1614 |
Method | AwA | CIFAR-10 | ImageNet | |||||||||
12 bits | 24 bits | 32 bits | 48 bits | 12 bits | 24 bits | 32 bits | 48 bits | 12 bits | 24 bits | 32 bits | 48 bits | |
VSAQ | 0.1948 | 0.2099 | 0.2187 | 0.2218 | 0.2615 | 0.2682 | 0.2670 | 0.2867 | 0.1472 | 0.1516 | 0.1579 | 0.1614 |
VSAQ-1 | 0.1830 | 0.1849 | 0.1923 | 0.2012 | 0.2360 | 0.2487 | 0.2538 | 0.2539 | 0.1288 | 0.1319 | 0.1386 | 0.1497 |
VSAQ-2 | 0.1911 | 0.1956 | 0.2026 | 0.2089 | 0.2412 | 0.2533 | 0.2613 | 0.2665 | 0.1296 | 0.1365 | 0.1463 | 0.1495 |
VSAQ-3 | 0.1816 | 0.1736 | 0.1825 | 0.1998 | 0.2278 | 0.2324 | 0.2405 | 0.2487 | 0.1053 | 0.1150 | 0.1194 | 0.1256 |