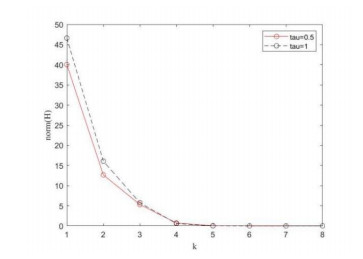
Here, we provide a new method to solve the time-fractional diffusion equation (TFDE) following the spectral tau approach. Our proposed numerical solution is expressed in terms of a double Lucas expansion. The discretization of the technique is based on several formulas about Lucas polynomials, such as those for explicit integer and fractional derivatives, products, and certain definite integrals of these polynomials. These formulas aid in transforming the TFDE and its conditions into a matrix system that can be treated through a suitable numerical procedure. We conduct a study on the convergence analysis of the double Lucas expansion. In addition, we provide a few examples to ensure that the proposed numerical approach is applicable and efficient.
Citation: Waleed Mohamed Abd-Elhameed, Abdullah F. Abu Sunayh, Mohammed H. Alharbi, Ahmed Gamal Atta. Spectral tau technique via Lucas polynomials for the time-fractional diffusion equation[J]. AIMS Mathematics, 2024, 9(12): 34567-34587. doi: 10.3934/math.20241646
[1] | Ahmed A. El-Deeb, Osama Moaaz, Dumitru Baleanu, Sameh S. Askar . A variety of dynamic α-conformable Steffensen-type inequality on a time scale measure space. AIMS Mathematics, 2022, 7(6): 11382-11398. doi: 10.3934/math.2022635 |
[2] | Mohammed S. El-Khatib, Atta A. K. Abu Hany, Mohammed M. Matar, Manar A. Alqudah, Thabet Abdeljawad . On Cerone's and Bellman's generalization of Steffensen's integral inequality via conformable sense. AIMS Mathematics, 2023, 8(1): 2062-2082. doi: 10.3934/math.2023106 |
[3] | Ahmed A. El-Deeb, Dumitru Baleanu, Nehad Ali Shah, Ahmed Abdeldaim . On some dynamic inequalities of Hilbert's-type on time scales. AIMS Mathematics, 2023, 8(2): 3378-3402. doi: 10.3934/math.2023174 |
[4] | Ahmed A. El-Deeb, Samer D. Makharesh, Sameh S. Askar, Dumitru Baleanu . Bennett-Leindler nabla type inequalities via conformable fractional derivatives on time scales. AIMS Mathematics, 2022, 7(8): 14099-14116. doi: 10.3934/math.2022777 |
[5] | Elkhateeb S. Aly, Y. A. Madani, F. Gassem, A. I. Saied, H. M. Rezk, Wael W. Mohammed . Some dynamic Hardy-type inequalities with negative parameters on time scales nabla calculus. AIMS Mathematics, 2024, 9(2): 5147-5170. doi: 10.3934/math.2024250 |
[6] | Marwa M. Ahmed, Wael S. Hassanein, Marwa Sh. Elsayed, Dumitru Baleanu, Ahmed A. El-Deeb . On Hardy-Hilbert-type inequalities with α-fractional derivatives. AIMS Mathematics, 2023, 8(9): 22097-22111. doi: 10.3934/math.20231126 |
[7] | Bingxian Wang, Mei Xu . Asymptotic behavior of some differential inequalities with mixed delays on time scales and their applications. AIMS Mathematics, 2024, 9(6): 16453-16467. doi: 10.3934/math.2024797 |
[8] | Jian-Mei Shen, Saima Rashid, Muhammad Aslam Noor, Rehana Ashraf, Yu-Ming Chu . Certain novel estimates within fractional calculus theory on time scales. AIMS Mathematics, 2020, 5(6): 6073-6086. doi: 10.3934/math.2020390 |
[9] | Awais Younus, Khizra Bukhsh, Manar A. Alqudah, Thabet Abdeljawad . Generalized exponential function and initial value problem for conformable dynamic equations. AIMS Mathematics, 2022, 7(7): 12050-12076. doi: 10.3934/math.2022670 |
[10] | Haytham M. Rezk, Mohammed Zakarya, Amirah Ayidh I Al-Thaqfan, Maha Ali, Belal A. Glalah . Unveiling new reverse Hilbert-type dynamic inequalities within the framework of Delta calculus on time scales. AIMS Mathematics, 2025, 10(2): 2254-2276. doi: 10.3934/math.2025104 |
Here, we provide a new method to solve the time-fractional diffusion equation (TFDE) following the spectral tau approach. Our proposed numerical solution is expressed in terms of a double Lucas expansion. The discretization of the technique is based on several formulas about Lucas polynomials, such as those for explicit integer and fractional derivatives, products, and certain definite integrals of these polynomials. These formulas aid in transforming the TFDE and its conditions into a matrix system that can be treated through a suitable numerical procedure. We conduct a study on the convergence analysis of the double Lucas expansion. In addition, we provide a few examples to ensure that the proposed numerical approach is applicable and efficient.
The Weighted Complementarity Problem (WCP), which is to find a pair of (x,s,y)∈Rn×Rn×Rm such that
x⩾0,s⩾0,xs=w,F(x,s,y)=0, | (1.1) |
where, F:R2n+m→Rn+m is a continuously differentiable function, w∈Rn+ is the given weight vector, xs:=x∘s is the componentwise product of the vectors x and s. When w=0, WCP (1.1) reduces to the classical Nonlinear Complementarity Problem (NCP). At present, there are many effective algorithms [1,2,3,4,5] that can solve NCP. For examples, Newton method [1], Quasi-Newton method [2], L-M method [3,4], Neural-Networks method [5] etc. If
F(x,s,y)=Px+Qs+Ry−a, | (1.2) |
problem (1.1) is the Linear Weighted Complementarity Problem (LWCP) studied in this paper, which is to find a pair of (x,s,y)∈Rn×Rn×Rm such that
x⩾0,s⩾0,xs=w,Px+Qs+Ry=a, | (1.3) |
where, P∈R(n+m)×n,Q∈R(n+m)×n,R∈R(n+m)×m,a∈Rn+m, are given matrices and vector. In addition, when
F(x,s,y)=(∇f(x)−s−ATyAx−b), | (1.4) |
problem (1.1) is the perturbed Karush-Kuhn-Tucker(KKT) condition for the following Nonlinear Programming(NLP)
minf(x),s.t.Ax=b,x≥0. | (1.5) |
Problem(1.3) was introduced by Potra [6] in 2012 and has been widely studied for its important applications in management, market equilibrium, etc. Many equilibrium problems can also be transformed into LWCP to solve, such as the famous Fisher market equilibrium problem [7], and the quadratic programming and weighted center problem [6].
In recent years, many effective algorithms have been proposed to solve problem (1.1) or (1.3) [8,9,10,11,12,13]. For examples, Chi et al. [9] proposed the full-Newton step infeasible interior-point method for solving LWCP. Zhang et al. [12] proposed the smoothing Newton type method for solving LWCP. Tang et al. [13] proposed the nonmonotone L-M method for NWCP. The interior point method depends on the choice of initial value. The classical Newton method needs the positive definite of Hessian matrix, otherwise, it is difficult to guarantee that the Newton direction is descending. The L-M method does not depend on the choice of initial values, nor does it require the positive definiteness of the Hessian matrix. Therefore, this paper mainly considers using L-M method to solve problem (1.3). Motivated by [13], we consider using a nonmonotone L-M method to solve LWCP.
LWCP is a more general complementary model. For the solution of this model, we hope to use the WCP functions obtained by the extension of NCP functions. However, due to the existence of weighting term, not all NCP functions can be directly extended to WCP functions. For NCP function in the form of FB function, many scholars have extended it to WCP function. In this paper, motivated by the smoothed penalty function for [14], we construct a smoothng function for WCP. And then use L-M method to approximate the equivalent reconstruction equations of problem (1.3). The comparison experiment of random generation shows the feasibility and effectiveness of our algorithm.
The following notations will be used throughout this paper. The superscript T denotes transpose. R denotes real numbers, Rn represents the set of all n dimensional real column vectors. The matrix I denotes the identity matrix, and ‖ denotes 2-norm. All vectors in this article are column vectors.
In this section, we study a class of complementary functions with participation weights and discuss its properties. Based on this weighted complementary function, the equivalent reconstruction equations of problem (1.3) are given.
Definition 2.1. For a fixed c \geqslant 0 , a function \phi :{R^2} \to R is called a weighted complementarity function [13], if it satisfies
{\phi ^c}(a, b) = 0 \Leftrightarrow a \geqslant 0, b \geqslant 0, ab = c. | (2.1) |
When c = 0 , {\phi ^c}(a, b) reduces to the NCP function.
In this paper, to solve the LWCP (1.3), we hope to use the WCP functions obtained by the extension of NCP functions. However, due to the existence of weighting term, not all NCP functions can be directly generalized to WCP functions. For example, the two piecewise NCP functions given in [2]:
\phi \left( {a, b} \right) = \left\{ {\begin{array}{*{20}{c}} {3a - \left( {\frac{{{a^2}}}{b}} \right), b \geqslant a > 0, or3b > - a \geqslant 0;} \\ {3a - \left( {\frac{{{b^2}}}{a}} \right), a > b > 0, or3a > - b \geqslant 0;} \\ {9a + 9b, else.} \end{array}} \right. | (2.2) |
\phi \left( {a, b} \right) = \left\{ {\begin{array}{*{20}{c}} {{k^2}a, b \geqslant k\left| a \right|;} \\ {2kb - \left( {\frac{{{b^2}}}{a}} \right), a > \frac{{\left| b \right|}}{k};} \\ {2{k^2}a + 2kb + \left( {\frac{{{b^2}}}{a}} \right), a < - \frac{{\left| b \right|}}{k};} \\ {{k^2}a + 4kb, b \leqslant - k\left| a \right|.} \end{array}} \right. | (2.3) |
For FB function, many scholars have extended it to WCP function. For example, Liu et al. [11] based on the symmetric disturbance FB function in [15] constructed:
{\phi _c}(\mu , a, b) = \left( {1 + \mu } \right)\left( {a + b} \right) - \sqrt {{{\left( {a + \mu b} \right)}^2} + {{\left( {\mu a + b} \right)}^2} + 2c + 2{\mu ^2}} , | (2.4) |
where, c is a given nonnegative vector.
Zhang[12] proposed:
{\phi _\theta }(\mu , a, b, c) = \sqrt {{a^2} + {b^2} - 2\theta ab + 2\left( {1 + \theta } \right)c + 2\mu } - a - b, | (2.5) |
where, \theta \in \left( { - 1, 1} \right], c is a given nonnegative vector.
In addition, [13] provides another smooth function:
\phi _{_{\tau , q}}^c(a, b) = {\left( {a + b} \right)^q} - {\left( {\sqrt {{a^2} + {b^2} + \left( {\tau - 2} \right)ab + \left( {4 - \tau } \right)c} } \right)^q}, | (2.6) |
where, c is a given nonnegative vector, \tau \in \left[ {0, 4} \right) is a constant, q > 1 is an odd integer. Compared with (2.4) and (2.5), (2.6) does not need to introduce the smoothing factor \mu . By controlling the value of q , smoothing can be achieved. This smoothing method will be used to smooth the new WCP function given below.
\phi _\tau ^c(a, b) = a + b - \sqrt {\tau {{(a - b)}^2} + (1 - \tau )({a^2} + {b^2}) + 2(1 + \tau )c} , | (2.7) |
where, c is a given nonnegative vector, \tau \in \left[ {0, 1} \right] is a constant.
Since Eq (2.7) is not smooth, we make the following smoothing treatment:
\phi _{\tau , q}^c(a, b) = {(a + b)^q} - {(\sqrt {\tau {{\left( {a - b} \right)}^2} + \left( {1 - \tau } \right)\left( {{a^2} + {b^2}} \right) + 2(1 + \tau )c} )^q}, | (2.8) |
where, c is a given nonnegative vector, \tau \in \left[ {0, 1} \right] is a constant, q > 1 is an odd integer.
Theorem 2.1. Let \phi _{\tau , q}^c be defined by (2.8) with \tau \in \left[ {0, 1} \right] and q > 1 being a positive odd interger. Then \phi _\tau ^q is a family of WCP functions, i.e.,
\phi _{\tau , q}^c(a, b) = 0 \Leftrightarrow a \geqslant 0, b \geqslant 0, ab = c. | (2.9) |
Proof. Since for any \alpha , \beta \in R and any positive odd interger q , there is {\alpha ^q} = {\beta ^q} \Leftrightarrow \alpha = \beta . So we have
\begin{array}{l} \phi _{\tau , q}^c(a, b) = 0 \Leftrightarrow {(a + b)^q} = {(\sqrt {\tau {{(a - b)}^2} + (1 - \tau )({a^2} + {b^2}) + 2(1 + \tau )c} )^q} \\ \;\; \;\;\;\;\;\;\;\; \Leftrightarrow a + b = \sqrt {\tau {{(a - b)}^2} + (1 - \tau )({a^2} + {b^2}) + 2(1 + \tau )c} \\ \;\; \;\;\;\;\;\;\;\; \Leftrightarrow \phi _\tau ^c(a, b) = 0. \end{array} | (2.10) |
That is to say, we only need to prove that \phi _\tau ^c(a, b) is a family of WCP functions. On the one hand, we fist suppose that \forall a, b \in R satisfy, \phi _\tau ^c(a, b) = 0 i.e.,
\sqrt {\tau {{(a - b)}^2} + (1 - \tau )({a^2} + {b^2}) + 2(1 + \tau )c} = a + b. | (2.11) |
By squaring the two sides of (2.11), we have 2(1 + \tau )ab = 2(1 + \tau )c, which together with \tau \in [0, 1] . yields ab = c. By substituing ab = c into (2.2), we have \sqrt {{a^2} + {b^2} + 2ab} = a + b \geqslant 0. Since c = ab \geqslant 0, it follows that a \geqslant 0, b \geqslant 0. On the other hand, we suppose that a \geqslant 0, b \geqslant 0, ab = c, then a + b \geqslant 0 and
\sqrt {\tau {{(a - b)}^2} + (1 - \tau )({a^2} + {b^2}) + 2(1 + \tau )c} = \sqrt {{a^2} + {b^2} + 2ab} = \left| {a + b} \right| = a + b. | (2.12) |
Which implies that \phi _\tau ^c(a, b) = 0.
Lemma 2.1. Let \phi _{\tau , q}^c be defined by (2.8) with \tau \in [0, 1] and q > 1 being a positive odd interger. Let
h_\tau ^c(a, b) = \sqrt {\tau {{(a - b)}^2} + (1 - \tau )({a^2} + {b^2}) + 2(1 + \tau )c} . | (2.13) |
Then
(ⅰ)When q > 1 , \phi _{\tau , q}^c is continuously differentiable at any \left( {a, b} \right) \in {R^2} with
\nabla \phi _{\tau , q}^c = \left[ {\begin{array}{*{20}{c}} {\frac{{\partial \phi _{\tau , q}^c}}{{\partial a}}} \\ {\frac{{\partial \phi _{\tau , q}^c}}{{\partial b}}} \end{array}} \right], | (2.14) |
where
\begin{array}{l} \frac{{\partial \phi _{\tau , q}^c}}{{\partial a}} = q[{(a + b)^{q - 1}} - h_\tau ^c{(a, b)^{q - 2}}(a - \tau b)], \\ \frac{{\partial \phi _{\tau , q}^c}}{{\partial b}} = q[{(a + b)^{q - 1}} - h_\tau ^c{(a, b)^{q - 2}}(b - \tau a)]. \end{array} |
(ⅱ)When q > 3 , \phi _{\tau , q}^c is twice continuously differentiable at any \left( {a, b} \right) \in {R^2} with
{\nabla ^2}\phi _{\tau , q}^c(a, b) = \left[ {\begin{array}{*{20}{c}} {\frac{{{\partial ^2}\phi _{\tau , q}^c}}{{\partial {a^2}}}}&{\frac{{{\partial ^2}\phi _{\tau , q}^c}}{{\partial a\partial b}}} \\ {\frac{{{\partial ^2}\phi _{\tau , q}^c}}{{\partial b\partial a}}}&{\frac{{{\partial ^2}\phi _{\tau , q}^c}}{{\partial {b^2}}}} \end{array}} \right], | (2.15) |
where
\begin{array}{l} \frac{{{\partial ^2}\phi _\tau ^q}}{{\partial {a^2}}} = q\left\{ {(q - 1){{(a + b)}^{q - 2}} - {h_\tau }{{(a, b, c)}^{q - 4}}[(q - 2){{(a - \tau b)}^2} + {h_\tau }{{(a, b, c)}^2}]} \right\}, \\ \frac{{{\partial ^2}\phi _{\tau , q}^c}}{{\partial {b^2}}} = q\left\{ {(q - 1){{(a + b)}^{q - 2}} - h_\tau ^c{{(a, b)}^{q - 4}}[(q - 2){{(b - \tau a)}^2} + h_\tau ^c{{(a, b)}^2}]} \right\}, \end{array} |
\frac{{{\partial ^2}\phi _{\tau , q}^c}}{{\partial a\partial b}} = \frac{{{\partial ^2}\phi _{\tau , q}^c}}{{\partial b\partial a}} = q\left\{ {(q - 1){{(a + b)}^{q - 2}} - h_\tau ^c{{(a, b)}^{q - 4}}[(q - 2)(a - \tau b)(b - \tau a) - \tau h_\tau ^c{{(a, b)}^2}]} \right\}. |
Lemma 2.2. Let \phi _{\tau , q}^c be defined by (2.8) with \tau \in [0, 1] and q > 1 being a positive odd interger. Defining the closed and convex set \Omega \left( u \right): = \left\{ {u \in {R^2}\left| {\left\| u \right\|} \right. \leqslant \theta } \right\} , where \theta is a positive constant. Then:
(ⅰ)When q > 1 , \phi _{\tau , q}^c is Lipschitz continuous on \Omega \left( u \right) for any \theta > 0 .
(ⅱ)When q > 3 , \nabla \phi _{\tau , q}^c is Lipschitz continuous on \Omega \left( u \right) for any \theta > 0 .
Since \phi _{\tau , q}^c and \nabla \phi _{\tau , q}^c are bounded on the set \Omega \left( u \right) , therefore the conclusion (ⅰ) and (ⅱ) can be obtained from the Mean-Value-Theorem.
Given weight vector w \in R_ + ^n , let z: = (x, s, y) \in {R^{2n + m}} and
H(z) = H(x, s, y): = \left( {\begin{array}{*{20}{c}} {F(x, s, y)} \\ {\Phi _{\tau , q}^w(x, s)} \end{array}} \right), | (2.16) |
where
\Phi _{\tau , q}^w(x, s) = \left( {\begin{array}{*{20}{c}} {\begin{array}{*{20}{c}} {\phi _{\tau , q}^{{w_1}}({x_1}, {s_1})} \\ \vdots \end{array}} \\ {\phi _{\tau , q}^{{w_n}}({x_n}, {s_n})} \end{array}} \right). | (2.17) |
Then the solution of LWCP (1.3) is equivalent to the approximate solution of the system of equations H(z) = 0 .
Lemma2.3. Let H\left( z \right):{R^{2n + m}} \to {R^{2n + m}}, \Phi _{\tau , q}^w:{R^{2n}} \to {R^n} be defined by (2.16) and (2.17), respectively. Then:
(ⅰ) \Phi _{\tau , q}^w\left( {x, s} \right) is continuously differentiable at any z = \left( {x, s, y} \right) \in {R^{2n + m}} .
(ⅱ) H\left( z \right) is continuously differentiable at any z = \left( {x, s, y} \right) \in {R^{2n + m}} with its Jacobian
H'\left( z \right) = \left( {\begin{array}{*{20}{c}} {\begin{array}{*{20}{c}} {{{F'}_x}}&{{{F'}_s}}&{{{F'}_y}} \end{array}} \\ {\begin{array}{*{20}{c}} {{D_1}}&{{D_2}}&0 \end{array}} \end{array}} \right), | (2.18) |
where
\begin{array}{l} {D_1} = diag\left\{ {q\left[ {{{\left( {{x_i} + {s_i}} \right)}^{q - 1}} - h_\tau ^{{w_i}}{{\left( {{x_i}, {s_i}} \right)}^{q - 2}}\left( {{x_i} - \tau {s_i}} \right)} \right]} \right\}, i = 1, 2, \cdots , n. \\ {D_2} = diag\left\{ {q\left[ {{{\left( {{x_i} + {s_i}} \right)}^{q - 1}} - h_\tau ^{{w_i}}{{\left( {{x_i}, {s_i}} \right)}^{q - 2}}\left( {{s_i} - \tau {x_i}} \right)} \right]} \right\}, i = 1, 2, \cdots , n. \\ h_\tau ^{{w_i}}({x_i}, {s_i}) = \sqrt {\tau {{({x_i} - {s_i})}^2} + (1 - \tau )({x_i}^2 + {s_i}^2) + 2(1 + \tau ){w_i}} , i = 1, 2, \cdots , n. \end{array} |
Let H(z) be defined by (2.16), then its value function M:{R^{2n + m}} \to {R_ + } can be defined as:
M(z): = \frac{1}{2}{\Vert H\left(z\right)\Vert }^{2}. | (2.19) |
Obviously, the solution of LWCP (1.3) is also equivalent to the approximate solution of the system of equations M(z) = 0. In addition, the following conclusion can be obtained from the Lemma 2.3.
Lemma 2.4. Let M:{R^{2n + m}} \to {R_ + } be defined by (2.19), then M(z) is continuously differentiable at any z \in {R^{2n + m}} , and \nabla M(z) = H'{\left( z \right)^T}H\left( z \right).
In this section, based on the WCP function in Section 2, we will give the smooth L-M type algorithm and its convergence.
Algorithm3.1 (A smooth L-M method)
Step 0: Choose \theta , \sigma , \gamma , \delta \in \left( {0, 1} \right) and {z^0}: = \left( {{x^0}, {s^0}, {y^0}} \right) \in {R^{2n + m}} , let 0 \leqslant \varepsilon \leqslant 1 , and {C_0} = M\left( {{z^0}} \right) . Choose a sequence \left\{ {{\eta _k}\left| {\forall k \geqslant 0, } \right.{\eta _k} \in \left( {0, 1} \right)} \right\} , set k: = 0.
Step 1: Compute H({z^k}) . If \left\| {H({z^k})} \right\| \leqslant \varepsilon then stop.
Step 2: Let {\mu _k}: = \theta {\left\| {H\left( {{z^k}} \right)} \right\|^2} . Compute the search direction {d_k} \in {R^{2n + m}} by
\nabla M({z^k}) + \left( {{H^{'}}{{({z^k})}^T}{H^{'}}({z^k}) + {\mu _k}I} \right){d_k} = 0. | (3.1) |
Step 3: If {d_k} satisfies
\left\| {H({z^k} + {d_k})} \right\| \leqslant \sigma \left\| {H({z^k})} \right\|. | (3.2) |
Then let {\alpha _k}: = 1 , and go to step 5. Otherwise, go to step 4.
Step 4: Set {j_k} be the smallest nonnegative integer j satisfying
M({z^k} + {\delta ^j}{d_k}) \leqslant {C_k} - \gamma {\left\| {{\delta ^j}{d_k}} \right\|^2}. | (3.3) |
let {\alpha _k}: = {\delta ^{{j_k}}} , and go to step 5.
Step 5: Set {z^{k + 1}}: = {z^k} + {\alpha _k}{d_k} and
{Q_{k + 1}}: = {\eta _k}{Q_k}, {C_{k + 1}}: = \frac{{{\eta _k}{Q_k}{C_k} + M\left( {{z^{k + 1}}} \right)}}{{{Q_{k + 1}}}}. | (3.4) |
Step 6: Let k: = k + 1 , and go to step 1.
Existing L-M type methods [16,17,18] are usually designed based on the Armijo line search. While algorithm 3.1 adopts a nonmonotone derivate free line search. The choice of {\eta _k} controls the degree of nonmonotoicity. If {\eta _k} \equiv 0 , then the line search is monotone.
Theorem3.1. Let \left\{ {{z^k}} \right\} be the sequence generated by Algorithm 3.1. Then, \left\{ {{z^k}} \right\} satisfying M({z^k}) \leqslant {C_k} for all k \geqslant 0 .
Proof. By Algorithm 3.1 {C_0} = M\left( {{z^0}} \right). We first assume that M\left( {{z^k}} \right) \leqslant {C_k} . If \nabla M\left( {{z^k}} \right) = 0, then Algorithm 3.1 terminates. Otherwise \nabla M\left( {{z^k}} \right) \ne 0 which implies that H\left( {{z^k}} \right) \ne 0 , hence {\mu _k} = \theta {\left\| {H\left( {{z^k}} \right)} \right\|^2} > 0 . So the matrix H'{\left( {{z^k}} \right)^T}H'\left( {{z^k}} \right) + {\mu _k}I is positive definite. Thus the search direction {d_k} in step 3 is well-defined and {d_k} \ne 0 . Since \nabla M\left( {{z^k}} \right) \ne 0 , we have
\nabla M{\left( {{z^k}} \right)^T}{d_k} = - {d_k}^T\left( {H'{{\left( {{z^k}} \right)}^T}H'\left( {{z^k}} \right) + {\mu _k}I} \right){d_k} < 0. | (3.5) |
This implies that {d_k} is a descent direction of M\left( {{z^k}} \right) at the point {z^k} . Next we will prove that at least one step size is obtained by step 4. Inversely, we assume that for any j , M\left( {{z^k} + {\delta ^j}{d_k}} \right) > {C_k} - \gamma {\left\| {{\delta ^j}{d_k}} \right\|^2} , then
M\left( {{z^k} + {\delta ^j}{d_k}} \right) > {C_k} - \gamma {\left\| {{\delta ^j}{d_k}} \right\|^2} \geqslant M\left( {{z^k}} \right) - \gamma {\left\| {{\delta ^j}{d_k}} \right\|^2}, | (3.6) |
thereby
\frac{{M\left( {{z^k} + {\delta ^j}{d_k}} \right) - M\left( {{z^k}} \right) + \gamma {{\left\| {{\delta ^j}{d_k}} \right\|}^2}}}{{{\delta ^j}}} > 0. | (3.7) |
By letting j \to \infty in (3.7), we have \nabla M{\left( {{z^k}} \right)^T}{d_k} \geqslant 0 , which contradicts (3.5). Therefore, we can always get {z^{k + 1}} by Step 3 or Step 4. If {z^{k + 1}} is generated by step 3, i.e., \left\| {H\left( {{z^k} + {d_k}} \right)} \right\| \leqslant \sigma \left\| {H\left( {{z^k}} \right)} \right\| , then \frac{1}{2}{\left\| {H\left( {{z^k} + {d_k}} \right)} \right\|^2} \leqslant \frac{1}{2}{\sigma ^2}{\left\| {H\left( {{z^k}} \right)} \right\|^2} , so M\left( {{z^{k + 1}}} \right) \leqslant {\sigma ^2}M\left( {{z^k}} \right) . And because, \sigma \in \left( {0, 1} \right) , therefore, we have M\left( {{z^{k + 1}}} \right) \leqslant {\sigma ^2}M\left( {{z^k}} \right) < M\left( {{z^k}} \right) \leqslant {C_k} . If {z^{k + 1}} is generated by step 4, we can get M\left( {{z^{k + 1}}} \right) \leqslant {C_k} directly. So, from(3.4), we can get that {C_k} \geqslant \frac{{{\eta _k}{Q_k}M\left( {{z^{k + 1}}} \right) + M\left( {{z^{k + 1}}} \right)}}{{{Q_{k + 1}}}} = M\left( {{z^{k + 1}}} \right) . Hence, we conclude that M({z^k}) \leqslant {C_k} for all k \geqslant 0 .
Next, we first suppose that \nabla M\left( {{z^k}} \right) \ne 0 for all k \geqslant 0 . In order to discuss the convergence of algorithm 3.1, we need the following lemma.
Lemma 3.1. Let \left\{ {{z^k}} \right\} be the sequence generated by Algorithm 3.1, then there exists a nonnegative constant {C^ * } such that
\mathop {\lim }\limits_{k \to \infty } M\left( {{z^k}} \right) = \mathop {\lim }\limits_{k \to \infty } {C_k} = {C^ * }. | (3.8) |
Proof. By Theorem3.1, we can get 0 \leqslant M\left( {{z^k}} \right) \leqslant {C_k} for all k \geqslant 0 and {C_{k + 1}} \leqslant \frac{{{\eta _k}{Q_k}{C_k} + {C_k}}}{{{Q_{k + 1}}}} = {C_k}. Hence, by The Monotone Bounded Theorem, there exists a nonnegative constant {C^ * } such that \mathop {\lim }\limits_{k \to \infty } {C_k} = {C^ * } . By the definition of {Q_k} , we have
{Q_{k + 1}} = 1 + \mathop \Sigma \limits_{i = 0}^k \mathop \Pi \limits_{j = 0}^i {\eta _{k - j}} \leqslant 1 + \mathop \Sigma \limits_{i = 0}^k \eta _{\max }^{i + 1} \leqslant \mathop \Sigma \limits_{i = 0}^\infty \eta _{\max }^i = \frac{1}{{1 - {\eta _{\max }}}}. | (3.9) |
Hence, we conclude that {\eta _k}{Q_k} \leqslant \frac{{{\eta _{\max }}}}{{1 - {\eta _{\max }}}} is bounded, which together with \mathop {\lim }\limits_{k \to \infty } {C_k} = {C^ * } yields \mathop {\lim }\limits_{k \to \infty } {\eta _{k - 1}}{Q_{k - 1}}\left( {{C_k} - {C_{k - 1}}} \right) = 0. So, it follows from (3.4) that
\begin{array}{l} M\left( {{z^{k + 1}}} \right) = {Q_{k + 1}}{C_{k + 1}} - {\eta _k}{Q_k}{C_k} = \left( {{\eta _k}{Q_k} + 1} \right){C_{k + 1}} - {\eta _k}{Q_k}{C_k} \\ \;\;\;\;\;\;\;\;\;\;\;\;\;= {\eta _k}{Q_k}\left( {{C_{k + 1}} - {C_k}} \right) + {C_{k + 1}}. \end{array} | (3.10) |
Hence
\mathop {\lim }\limits_{k \to \infty } M\left( {{z^k}} \right) = \mathop {\lim }\limits_{k \to \infty } \left[ {{\eta _{k - 1}}{Q_{k - 1}}\left( {{C_k} - {C_{k - 1}}} \right) + {C_k}} \right] = {C^ * }. | (3.11) |
We complete the proof.
Theorem3.2. Let \left\{ {{z^k}} \right\} be the sequence generated by Algorithm 3.1. Then any accumulation point {z^ * } of \left\{ {{z^k}} \right\} is a stationary point of M\left( z \right) .
Proof. By Lemma 3.1, we have \mathop {\lim }\limits_{k \to \infty } M\left( {{z^k}} \right) = \mathop {\lim }\limits_{k \to \infty } {C_k} = {C^ * }, {C^ * } \geqslant 0 . If {C^ * } = 0 , then \mathop {\lim }\limits_{k \to \infty } H\left( {{z^k}} \right) = 0 which together with Lemma 2.4 yields \nabla M\left( {{z^ * }} \right) = 0 . In the following, we discuss the case of {C^ * } > 0 . Set N: = \left\{ {k\left| {\left\| {H\left( {{z^k} + {d_k}} \right)} \right\| \leqslant \sigma \left\| {H\left( {{z^k}} \right)} \right\|} \right.} \right\} . Then N must be a finite set, otherwise M\left( {{z^{k + 1}}} \right) \leqslant {\sigma ^2}M\left( {{z^k}} \right) holds for infinitely many k . By letting k \to \infty with k \in N , we can have {C^ * } \leqslant {\sigma ^2}{C^ * } and 1 \leqslant {\sigma ^2} which contradicts \sigma \in \left( {0, 1} \right) . Therefore, we can suppose that there exists an index \bar k > 0 such that \left\| {H\left( {{z^k} + {d_k}} \right)} \right\| > \sigma \left\| {H\left( {{z^k}} \right)} \right\| for all k \geqslant \bar k . Thereby, there exists a {j_k} such that M\left( {{z^{k + 1}}} \right) \leqslant {C_k} - \gamma {\left\| {{\delta ^{{j_k}}}{d_k}} \right\|^2} , i.e.,
\gamma {\left\| {{\delta ^{{j_k}}}{d_k}} \right\|^2} \leqslant {C_k} - M\left( {{z^{k + 1}}} \right). | (3.12) |
Next, we suppose that {z^ * } is the limit of the subsequence {\left\{ {{z^k}} \right\}_{k \in K}} \subset \left\{ {{z^k}} \right\} where K \in \left\{ {0, 1, 2, \cdots } \right\} , i.e., \mathop {\lim }\limits_{k\left( { \in K} \right) \to \infty } {z^k} = {z^ * } . Hence, by the continuity, we have {C^ * } = M\left( {{z^ * }} \right) = \frac{1}{2}{\left\| {H\left( {{z^ * }} \right)} \right\|^2} . By \mathop {\lim }\limits_{k \to \infty } {\mu _k} = \mathop {\lim }\limits_{k \to \infty } \theta {\left\| {H\left( {{z^k}} \right)} \right\|^2} = \mathop {\lim }\limits_{k \to \infty } 2\theta M\left( {{z^k}} \right) = 2\theta {C^ * } , we can get that
\mathop {\lim }\limits_{k\left( { \in K} \right) \to \infty } \left[ {H'{{\left( {{z^k}} \right)}^T}H'\left( {{z^k}} \right) + {\mu _k}I} \right] = H'{\left( {{z^ * }} \right)^T}H'\left( {{z^ * }} \right) + 2\theta {C^ * }I. | (3.13) |
According to the proof process of theorem 3.1, the matrix H'{\left( {{z^k}} \right)^T}H'\left( {{z^k}} \right) + {\mu _k}I is a symmetric positive definite matrix. In addition, because of {C^ * } > 0 , the matrix H'{\left( {{z^ * }} \right)^T}H'\left( {{z^ * }} \right) + 2\theta {C^ * }I is also symmetric positive definite matrix. Hence, we have
\mathop {\lim }\limits_{k\left( { \in K} \right) \to \infty } {\left[ {H'{{\left( {{z^k}} \right)}^T}H'\left( {{z^k}} \right) + {\mu _k}I} \right]^{ - 1}} = {\left[ {H'{{\left( {{z^ * }} \right)}^T}H'\left( {{z^ * }} \right) + 2\theta {C^ * }I} \right]^{ - 1}}. | (3.14) |
and
\mathop {\lim }\limits_{k\left( { \in K} \right) \to \infty } {d_k} = {d^ * } = - {\left[ {H'{{\left( {{z^ * }} \right)}^T}H'\left( {{z^ * }} \right) + 2\theta {C^ * }I} \right]^{ - 1}}\nabla M\left( {{z^ * }} \right). | (3.15) |
By (3.5), we can get
\nabla M{\left( {{z^ * }} \right)^T}{d^ * } = \mathop {\lim }\limits_{k\left( { \in K} \right) \to \infty } \nabla M{\left( {{z^k}} \right)^T}{d^k} \leqslant 0. | (3.16) |
By letting k \to \infty with k \in N in (3.12), we have \mathop {\lim }\limits_{k\left( { \in K} \right) \to \infty } \left\| {{\delta ^{{j_k}}}{d_k}} \right\| = 0 . If {\delta ^{{j_k}}} > 0 , then \mathop {\lim }\limits_{k\left( { \in K} \right) \to \infty } {d_k} = {d^ * } = 0 which together with (3.15) yields \nabla M\left( {{z^ * }} \right) = 0 . Otherwise, \mathop {\lim }\limits_{k\left( { \in K} \right) \to \infty } {\delta ^{{j_k}}} = 0 . From step 4 and Theorem 3.1
M({z^k} + {\delta ^{{j_k} - 1}}{d_k}) > {C_k} - \gamma {\left\| {{\delta ^{{j_k} - 1}}{d_k}} \right\|^2} \geqslant M({z^k}) - \gamma {\left\| {{\delta ^{{j_k} - 1}}{d_k}} \right\|^2}, | (3.17) |
i.e.,
\frac{{M({z^k} + {\delta ^{{j_k} - 1}}{d_k}) - M({z^k})}}{{{\delta ^{{j_k} - 1}}}} + \gamma {\left\| {{\delta ^{{j_k} - 1}}{d_k}} \right\|^2} > 0. | (3.18) |
Now that M\left( z \right) is continuously differentiable at {z^ * } , so we have
\nabla M{\left( {{z^ * }} \right)^T}{d^ * } \geqslant 0. | (3.19) |
Then, from (3.16), we can get \nabla M{\left( {{z^ * }} \right)^T}{d^ * } = 0 and
\begin{array}{l} {\left( {{d^ * }} \right)^T}\left( {H'{{\left( {{z^ * }} \right)}^T}H'\left( {{z^ * }} \right) + 2\theta {C^ * }I} \right){d^ * } = - \nabla M{\left( {{z^ * }} \right)^T}{\left[ {H'{{\left( {{z^ * }} \right)}^T}H'\left( {{z^ * }} \right) + 2\theta {C^ * }I} \right]^{ - 1}}\left( {H'{{\left( {{z^ * }} \right)}^T}H'\left( {{z^ * }} \right) + 2\theta {C^ * }I} \right){d^ * } \\ \;\;\;\;\;\;\;\;\;\;\;\;\;\; \;\;\;\;\;\;\;\;\;\;\;\;\;\;\;\;\;\;\;\;\;\;\;\;\;\;\;\;\;\;\;\;\;\;\;\;\;\;\;= - \nabla M{\left( {{z^ * }} \right)^T}{d^ * } = 0. \end{array} |
Since the matrix H'{\left( {{z^ * }} \right)^T}H'\left( {{z^ * }} \right) + 2\theta {C^ * }I is a positive matrix, so we have
{d^ * } = - {\left[ {H'{{\left( {{z^ * }} \right)}^T}H'\left( {{z^ * }} \right) + 2\theta {C^ * }I} \right]^{ - 1}}\nabla M{\left( {{z^ * }} \right)^T} = 0. | (3.20) |
Now that the matrix {\left[ {H'{{\left( {{z^ * }} \right)}^T}H'\left( {{z^ * }} \right) + 2\theta {C^ * }I} \right]^{ - 1}} is also positive matrix, we can get \nabla M\left( {{z^ * }} \right) = 0.
In this section, we carry out some numerical experiments on the LWCP by Algorithm 3.1. All experiments were conducted on a ThinkPad480 with a 1.8GHz CPU and 8.0GB RAM. The codes are run in MATLAB R2018b under Win10.
We first generate the matrices P, Q, R and vector a by following way:
P = \left( {\begin{array}{*{20}{c}} A \\ M \end{array}} \right), Q = \left( {\begin{array}{*{20}{c}} 0 \\ I \end{array}} \right), P = \left( {\begin{array}{*{20}{c}} 0 \\ { - {A^T}} \end{array}} \right), a = \left( {\begin{array}{*{20}{c}} b \\ { - f} \end{array}} \right), | (4.1) |
where A \in {R^{m \times n}} is a full row rank matrix with m < n , the matrix M is an n \times n symmetric semidefinite matrix, b \in {R^m}, f \in {R^n}. In our algorithm we set: \gamma = 0.01, \sigma = 0.5, \delta = 0.8, \theta = {10^{ - 4}}. The initial points are choosing as : {x^0} = \left( {1, \cdots , 1} \right), {s^0} = \left( {1, \cdots , 1} \right), {y^0} = \left( {0, \cdots , 0} \right).
In the course of experiments, we generate LWCP (1.3) by the following two ways.
(ⅰ) We take A = randn\left( {m, n} \right) with rank\left( A \right) = m , and M = \frac{{B{B^T}}}{{\left\| {B{B^T}} \right\|}} with B = rand\left( {n, n} \right) . we first generate \hat x = rand\left( {n, 1} \right), f = rand\left( {n, 1} \right) , then we set \hat b: = A\hat x, \hat s = M\hat x + f, w = \hat x\hat s .
(ⅱ) We choose a = \left( {\begin{array}{*{20}{c}} b \\ { - f} \end{array}} \right) - \xi where \xi \in {R^{n + m}} is a noise. We choose M = diag(v) with v = rand\left( {n, 1} \right) . The matrix A and vectors b, f are generated in the same way as (ⅰ). In the course of experiments, we take \xi = {10^{ - 4}}rand(1, 1)p with p: = {\left( {1, 1, 0, \cdots , 0} \right)^T} \in {R^{n + m}} .
First, in order to observe the local convergence of algorithm 3.1, we conducted two sets of random test experiments on LWCP (ⅰ) with n = 1000, m = 500 . Figure 1 gives the convergence curve of \left\| {H\left( {{z^k}} \right)} \right\| at the k -th iteration. We can clearly see that algorithm 3.1 is locally fast, or at least locally superlinear.
Next, we conducted comparative experiments with [13]. In the course of experiments, the parameters in the WCP functions \phi _{\tau , q}^w are respectively taken as \tau = 0.5, q = 3, \tau = 1, q = 3 and \tau = 0.3, q = 3, \tau = 0.8, q = 3 . The numerical results are presented in Tables 1, 2, Figures 2 and 3 respectively. Where AIT, ACPU, ANH are respectively the average number of iterations, the average CPU time (unit seconds), and the average number \left\| {H\left( {{z^k}} \right)} \right\| of iterations at the end of 10 random experiments. LM represents our experimental result, TLM is the experimental result of [13].
m | n | LM | TLM | ||||
AIT | ACPU | ANH | AIT | ACPU | ANH | ||
200 | 500 | 7.9 | 0.6960 | 5.0131×10-12 | 8.0 | 0.7015 | 6.0903×10-13 |
7.8 | 0.6974 | 5.5794×10-12 | 7.7 | 0.6906 | 1.2630×10-11 | ||
7.6 | 0.6703 | 8.5289×10-12 | 7.9 | 0.7025 | 3.5098×10-13 | ||
400 | 800 | 8.1 | 2.4705 | 5.5548×10-13 | 8.8 | 3.1241 | 5.2707×10-13 |
8.2 | 2.5097 | 7.6171×10-13 | 8.9 | 2.6100 | 2.2961×10-13 | ||
8.2 | 2.6300 | 2.4813×10-13 | 8.1 | 2.4039 | 3.6750×10-12 | ||
500 | 1000 | 8.1 | 4.4569 | 1.2136×10-12 | 8.1 | 4.3590 | 2.2894×10-12 |
8.4 | 4.7697 | 3.1192×10-13 | 8.4 | 4.4993 | 4.5153×10-12 | ||
8.2 | 4.8820 | 2.7039×10-12 | 8.4 | 4.4767 | 9.3738×10-13 | ||
600 | 1500 | 7.9 | 11.2160 | 9.7961×10-12 | 8.0 | 11.6639 | 1.0240×10-12 |
8.0 | 11.4230 | 1.0008×10-13 | 8.0 | 11.6522 | 9.3154×10-13 | ||
8.0 | 11.5575 | 1.0238×10-12 | 7.9 | 11.4497 | 1.0559×10-11 | ||
1000 | 1500 | 9.6 | 18.4934 | 5.6351×10-12 | 9.5 | 18.6699 | 1.6880×10-11 |
9.9 | 19.0396 | 5.2759×10-12 | 11.1 | 21.6384 | 5.9206×10-11 | ||
8.4 | 16.3751 | 1.2735×10-11 | 10.9 | 21.3177 | 7.6313×10-12 |
m | n | LM | TLM | ||||
AIT | ACPU | ANH | AIT | ACPU | ANH | ||
200 | 500 | 7.5 | 0.6642 | 1.5973×10-11 | 8.0 | 0.7155 | 9.7275×10-12 |
7.4 | 0.6675 | 8.8485×10-12 | 8.4 | 0.7429 | 8.7482×10-13 | ||
7.6 | 0.6661 | 2.5321×10-12 | 8.1 | 0.7167 | 6.7422×10-13 | ||
400 | 800 | 8.0 | 2.3642 | 2.4919×10-13 | 8.8 | 2.6212 | 4.6000×10-12 |
8.0 | 2.3791 | 4.5892×10-13 | 8.2 | 2.5740 | 2.4604×10-13 | ||
8.2 | 2.4293 | 9.2368×10-13 | 9.0 | 2.6885 | 1.3216×10-12 | ||
500 | 1000 | 8.0 | 4.3592 | 6.2691×10-13 | 8.3 | 4.5328 | 3.5736×10-12 |
8.1 | 4.3174 | 3.1221×10-13 | 8.2 | 4.3540 | 3.2290×10-13 | ||
7.9 | 4.2089 | 9.7440×10-12 | 9.9 | 5.3469 | 6.5691×10-12 | ||
600 | 1500 | 7.9 | 11.3807 | 9.2057×10-12 | 8.9 | 12.9567 | 9.7825×10-13 |
7.8 | 11.2766 | 1.3435×10-11 | 8.0 | 11.6116 | 9.9437×10-13 | ||
8.0 | 11.5494 | 9.8875×10-13 | 9.2 | 13.3792 | 1.0247×10-12 | ||
1000 | 1500 | 9.3 | 17.6422 | 7.8120×10-12 | 8.9 | 17.2609 | 3.4824×10-12 |
8.7 | 16.3247 | 4.9019×10-12 | 8.8 | 17.3407 | 4.7999×10-11 | ||
9.3 | 18.1968 | 7.8112×10-12 | 9.4 | 18.4024 | 1.3738×10-11 |
Tables 1 and 2 show the numerical results for LWCP (ⅰ). Where, the parameters are taken as \tau = 0.5, q = 3;\tau = 1, q = 3 respectively. It can be seen from the table that no matter what value \tau takes, our algorithm 3.1 has less iteration time or higher accuracy than algorithm 1 in [13].
Figures 2 and 3 show the numerical results for solving LWCP (ⅱ). Where, the parameters are respectively taken as \tau = 0.3, q = 3, m = \frac{n}{2};\tau = 0.8, q = 3, m = \frac{n}{2} . It can be seen from the figure that with the increase of dimension, the AIT of algorithm 3.1 fluctuates slightly, but it is always smaller than the AIT in [13]. The ACPU increases steadily and always smaller than the ACPU in [13].
When \tau = 0.6, q = 3, m = \frac{n}{2} , Figure 4 shows the ACPU and AIT comparison line graphs for LWCP (ⅰ) and LWCP (ⅱ) solved by algorithms 3.1 and [13] respectively. It can be seen from the figure that after adding noise to LWCP (ⅰ), the solution speed of both algorithms decreases, but our algorithm still has certain advantages.
In general, the problems generated by numerical experiments converge in a few iterations. The number of iterations varies slightly with the dimension of the problem. Our algorithm is effective for the linear weighted complementarity problem LWCP (1.3), because each problem can be successfully solved in a very short time with a small number of iterations. Numerical results show the feasibility and effectiveness of the algorithm 3.1.
Based on the idea of L-M method, with the help of a new class of WCP functions {\varphi }_{\tau , q}^{c}(a, b), we give the algorithm 3.1 for solving the LWCP (1.3). Under certain conditions, our algorithm can obtain the approximate solution of LWCP (1.3). Numerical experiments show the feasibility and effectiveness of the algorithm 3.1.
The authors declare no conflicts of interest.
[1] | A. A. Kilbas, H. M. Srivastava, J. J. Trujillo, Theory and applications of fractional differential equations, Elsevier, 2006. |
[2] | S. G. Samko, Fractional integrals and derivatives: theory and applications, Gordon and Breach Science Publishers, 1993. |
[3] | I. Podlubny, Fractional differential equations: an introduction to fractional derivatives, fractional differential equations, to methods of their solution and some of their applications, Elsevier, 1998. |
[4] |
D. Albogami, D. Maturi, H. Alshehri, Adomian decomposition method for solving fractional time-Klein-Gordon equations using Maple, Appl. Math., 14 (2023), 411–418. https://doi.org/10.4236/am.2023.146024 doi: 10.4236/am.2023.146024
![]() |
[5] |
K. Sadri, K. Hosseini, E. Hinçal, D. Baleanu, S. Salahshour, A pseudo-operational collocation method for variable-order time-space fractional KdV–Burgers–Kuramoto equation, Math. Methods Appl. Sci., 46 (2023), 8759–8778. https://doi.org/10.1002/mma.9015 doi: 10.1002/mma.9015
![]() |
[6] |
S. M. Sivalingam, V. Govindaraj, A novel numerical approach for time-varying impulsive fractional differential equations using theory of functional connections and neural network, Expert Syst. Appl., 238 (2024), 121750. https://doi.org/10.1016/j.eswa.2023.121750 doi: 10.1016/j.eswa.2023.121750
![]() |
[7] |
W. M. Abd-Elhameed, H. M. Ahmed, Spectral solutions for the time-fractional heat differential equation through a novel unified sequence of Chebyshev polynomials, AIMS Math., 9 (2024), 2137–2166. https://doi.org/10.3934/math.2024107 doi: 10.3934/math.2024107
![]() |
[8] |
M. Izadi, Ş. Yüzbaşı W. Adel, A new Chelyshkov matrix method to solve linear and nonlinear fractional delay differential equations with error analysis, Math. Sci., 17 (2023), 267–284. https://doi.org/10.1007/s40096-022-00468-y doi: 10.1007/s40096-022-00468-y
![]() |
[9] |
H. Alrabaiah, I. Ahmad, R. Amin, K. Shah, A numerical method for fractional variable order pantograph differential equations based on Haar wavelet, Eng. Comput., 38 (2022), 2655–2668. https://doi.org/10.1007/s00366-020-01227-0 doi: 10.1007/s00366-020-01227-0
![]() |
[10] |
Kamran, S. Ahmad, K. Shah, T. Abdeljawad, B. Abdalla, On the approximation of fractal-fractional differential equations using numerical inverse Laplace transform methods, Comput. Model. Eng. Sci., 135 (2023), 3. https://doi.org/10.32604/cmes.2023.023705 doi: 10.32604/cmes.2023.023705
![]() |
[11] |
L. Qing, X. Li, Meshless analysis of fractional diffusion-wave equations by generalized finite difference method, Appl. Math. Lett., 157 (2024), 109204. https://doi.org/10.1016/j.camwa.2024.08.008 doi: 10.1016/j.camwa.2024.08.008
![]() |
[12] |
L. Qing, X. Li, Analysis of a meshless generalized finite difference method for the time-fractional diffusion-wave equation, Comput. Math. Appl., 172 (2024), 134–151. https://doi.org/10.1016/j.camwa.2024.08.008 doi: 10.1016/j.camwa.2024.08.008
![]() |
[13] | T. Koshy, Fibonacci and Lucas numbers with applications, John Wiley & Sons, 2011. |
[14] |
I. Ali, S. Haq, S. F. Aldosary, K. S. Nisar, F. Ahmad, Numerical solution of one-and two-dimensional time-fractional Burgers equation via Lucas polynomials coupled with finite difference method, Alex. Eng. J., 61 (2022), 6077–6087. https://doi.org/10.1016/j.aej.2021.11.032 doi: 10.1016/j.aej.2021.11.032
![]() |
[15] |
I. Ali, S. Haq, K. S. Nisar, S. U. Arifeen, Numerical study of 1D and 2D advection-diffusion-reaction equations using Lucas and Fibonacci polynomials, Arab. J. Math., 10 (2021), 513–526. https://doi.org/10.1007/s40065-021-00330-4 doi: 10.1007/s40065-021-00330-4
![]() |
[16] |
M. N. Sahlan, H. Afshari, Lucas polynomials based spectral methods for solving the fractional order electrohydrodynamics flow model, Commun. Nonlinear Sci. Numer. Simul., 107 (2022), 106108. https://doi.org/10.1016/j.cnsns.2021.106108 doi: 10.1016/j.cnsns.2021.106108
![]() |
[17] |
P. K. Singh, S. S. Ray, An efficient numerical method based on Lucas polynomials to solve multi-dimensional stochastic Itô-Volterra integral equations, Math. Comput. Simul., 203 (2023), 826–845. https://doi.org/10.1016/j.matcom.2022.06.029 doi: 10.1016/j.matcom.2022.06.029
![]() |
[18] |
A. M. S. Mahdy, D. Sh. Mohamed, Approximate solution of Cauchy integral equations by using Lucas polynomials, Comput. Appl. Math., 41 (2022), 403. https://doi.org/10.1007/s40314-022-02116-6 doi: 10.1007/s40314-022-02116-6
![]() |
[19] |
B. P. Moghaddam, A. Dabiri, A. M. Lopes, J. A. T. Machado, Numerical solution of mixed-type fractional functional differential equations using modified Lucas polynomials, Comput. Appl. Math., 38 (2019), 1–12. https://doi.org/10.1007/s40314-019-0813-9 doi: 10.1007/s40314-019-0813-9
![]() |
[20] |
O. Oruç, A new algorithm based on Lucas polynomials for approximate solution of 1D and 2D nonlinear generalized Benjamin–Bona–Mahony–Burgers equation, Comput. Math. Appl., 74 (2017), 3042–3057. https://doi.org/10.1016/j.camwa.2017.07.046 doi: 10.1016/j.camwa.2017.07.046
![]() |
[21] | M. Çetin, M. Sezer, H. Kocayiğit, An efficient method based on Lucas polynomials for solving high-order linear boundary value problems, Gazi Univ. J. Sci., 28 (2015), 483–496. |
[22] |
P. Roul, V. Goura, R. Cavoretto, A numerical technique based on B-spline for a class of time-fractional diffusion equation, Numer. Methods Partial Differ. Equ., 39 (2023), 45–64. https://doi.org/10.1002/num.22790 doi: 10.1002/num.22790
![]() |
[23] |
Y. H. Youssri, A. G. Atta, Petrov-Galerkin Lucas polynomials procedure for the time-fractional diffusion equation, Contemp. Math., 4 (2023), 230–248. https://doi.org/10.37256/cm.4220232420 doi: 10.37256/cm.4220232420
![]() |
[24] |
P. Lyu, S. Vong, A fast linearized numerical method for nonlinear time-fractional diffusion equations, Numer. Algorithms, 87 (2021), 381–408. https://doi.org/10.1007/s11075-020-00971-0 doi: 10.1007/s11075-020-00971-0
![]() |
[25] |
X. M. Gu, H. W. Sun, Y. L. Zhao, X. Zheng, An implicit difference scheme for time-fractional diffusion equations with a time-invariant type variable order, Appl. Math. Lett., 120 (2021), 107270. https://doi.org/10.1016/j.aml.2021.107270 doi: 10.1016/j.aml.2021.107270
![]() |
[26] |
A. Khibiev, A. Alikhanov, C. Huang, A second-order difference scheme for generalized time-fractional diffusion equation with smooth solutions, Comput. Methods Appl. Math., 24 (2024), 101–117. https://doi.org/10.1515/cmam-2022-0089 doi: 10.1515/cmam-2022-0089
![]() |
[27] |
J. L. Zhang, Z. W. Fang, H. W. Sun, Exponential-sum-approximation technique for variable-order time-fractional diffusion equations, J. Appl. Math. Comput., 68 (2022), 323–347. https://doi.org/10.1007/s12190-021-01528-7 doi: 10.1007/s12190-021-01528-7
![]() |
[28] | C. Canuto, M. Y. Hussaini, A. Quarteroni, T. A. Zang, Spectral methods in fluid dynamics, Springer-Verlag, 1988. |
[29] | J. Hesthaven, S. Gottlieb, D. Gottlieb, Spectral methods for time-dependent problems, Cambridge University Press, 2007. |
[30] | J. P. Boyd, Chebyshev and Fourier spectral methods, Courier Corporation, 2001. |
[31] |
W. M. Abd-Elhameed, M. M. Alsyuti, Numerical treatment of multi-term fractional differential equations via new kind of generalized Chebyshev polynomials, Fractal Fract., 7 (2023), 74. https://doi.org/10.3390/fractalfract7010074 doi: 10.3390/fractalfract7010074
![]() |
[32] |
M. M. Alsuyuti, E. H. Doha, S. S. Ezz-Eldien, I. K. Youssef, Spectral Galerkin schemes for a class of multi-order fractional pantograph equations, J. Comput. Appl. Math., 384 (2021), 113157. https://doi.org/10.1016/j.cam.2020.113157 doi: 10.1016/j.cam.2020.113157
![]() |
[33] |
R. M. Hafez, M. A. Zaky, M. A. Abdelkawy, Jacobi spectral Galerkin method for distributed-order fractional Rayleigh–Stokes problem for a generalized second grade fluid, Front. Phys., 7 (2020), 240. https://doi.org/10.3389/fphy.2019.00240 doi: 10.3389/fphy.2019.00240
![]() |
[34] |
W. M. Abd-Elhameed, A. M. Al-Sady, O. M. Alqubori, A. G. Atta, Numerical treatment of the fractional Rayleigh-Stokes problem using some orthogonal combinations of Chebyshev polynomials, AIMS Math., 9 (2024), 25457–25481. https://doi.org/10.3934/math.20241243 doi: 10.3934/math.20241243
![]() |
[35] |
A. A. El-Sayed, S. Boulaaras, N. H. Sweilam, Numerical solution of the fractional-order logistic equation via the first-kind Dickson polynomials and spectral tau method, Math. Methods Appl. Sci., 46 (2023), 8004–8017. https://doi.org/10.1002/mma.7345 doi: 10.1002/mma.7345
![]() |
[36] |
H. Hou, X. Li, A meshless superconvergent stabilized collocation method for linear and nonlinear elliptic problems with accuracy analysis, Appl. Math. Comput., 477 (2024), 128801. https://doi.org/10.1016/j.amc.2024.128801 doi: 10.1016/j.amc.2024.128801
![]() |
[37] |
A. G. Atta, Two spectral Gegenbauer methods for solving linear and nonlinear time fractional cable problems, Int. J. Mod. Phys. C, 35 (2024), 2450070. https://doi.org/10.1142/S0129183124500700 doi: 10.1142/S0129183124500700
![]() |
[38] |
M. M. Khader, M. Adel, Numerical and theoretical treatment based on the compact finite difference and spectral collocation algorithms of the space fractional-order Fisher's equation, Int. J. Mod. Phys. C, 31 (2020), 2050122. https://doi.org/10.1142/S0129183120501223 doi: 10.1142/S0129183120501223
![]() |
[39] |
A. Z. Amin, M. A. Abdelkawy, I. Hashim, A space-time spectral approximation for solving nonlinear variable-order fractional convection-diffusion equations with nonsmooth solutions, Int. J. Mod. Phys. C, 34 (2023), 2350041. https://doi.org/10.1142/S0129183123500419 doi: 10.1142/S0129183123500419
![]() |
[40] |
H. M. Ahmed, New generalized Jacobi polynomial Galerkin operational matrices of derivatives: An algorithm for solving boundary value problems, Fractal Fract., 8 (2024), 199. https://doi.org/10.3390/fractalfract8040199 doi: 10.3390/fractalfract8040199
![]() |
[41] |
İ. Avcı Spectral collocation with generalized Laguerre operational matrix for numerical solutions of fractional electrical circuit models, Math. Model. Numer. Simul. Appl., 4 (2024), 110–132. https://doi.org/10.53391/mmnsa.1428035 doi: 10.53391/mmnsa.1428035
![]() |
[42] |
H. Singh, Chebyshev spectral method for solving a class of local and nonlocal elliptic boundary value problems, Int. J. Nonlinear Sci. Numer. Simul., 24 (2023), 899–915. https://doi.org/10.1515/ijnsns-2020-0235 doi: 10.1515/ijnsns-2020-0235
![]() |
[43] |
M. Abdelhakem, A. Ahmed, D. Baleanu, M. El-Kady, Monic Chebyshev pseudospectral differentiation matrices for higher-order IVPs and BVP: Applications to certain types of real-life problems, Comput. Appl. Math., 41 (2022), 253. https://doi.org/10.1007/s40314-022-01940-0 doi: 10.1007/s40314-022-01940-0
![]() |
[44] |
Priyanka, S. Sahani, S. Arora, An efficient fourth order Hermite spline collocation method for time fractional diffusion equation describing anomalous diffusion in two space variables, Comput. Appl. Math., 43 (2024), 193. https://doi.org/10.1007/s40314-024-02708-4 doi: 10.1007/s40314-024-02708-4
![]() |
[45] |
W. M. Abd-Elhameed, A. Napoli, Some novel formulas of Lucas polynomials via different approaches, Symmetry, 15 (2023), 185. https://doi.org/10.3390/sym15010185 doi: 10.3390/sym15010185
![]() |
[46] |
W. M. Abd-Elhameed, N. A. Zeyada, New formulas including convolution, connection and radicals formulas of k-Fibonacci and k-Lucas polynomials, Indian J. Pure Appl. Math., 53 (2022), 1006–1016. https://doi.org/10.1007/s13226-021-00214-5 doi: 10.1007/s13226-021-00214-5
![]() |
[47] |
W. M. Abd-Elhameed, A. K. Amin, Novel identities of Bernoulli polynomials involving closed forms for some definite integrals, Symmetry, 14 (2022), 2284. https://doi.org/10.3390/sym14112284 doi: 10.3390/sym14112284
![]() |
[48] |
W. M. Abd-Elhameed, Y. H. Youssri, Generalized Lucas polynomial sequence approach for fractional differential equations, Nonlinear Dynam., 89 (2017), 1341–1355. https://doi.org/10.1007/s11071-017-3519-9 doi: 10.1007/s11071-017-3519-9
![]() |
[49] |
Y. H. Youssri, W. M. Abd-Elhameed, A. G. Atta, Spectral Galerkin treatment of linear one-dimensional telegraph type problem via the generalized Lucas polynomials, Arab. J. Math., 11 (2022), 601–615. https://doi.org/10.1007/s40065-022-00374-0 doi: 10.1007/s40065-022-00374-0
![]() |
[50] |
G. J. O. Jameson, The incomplete gamma functions, Math. Gaz., 100 (2016), 298–306. https://doi.org/10.1017/mag.2016.67 doi: 10.1017/mag.2016.67
![]() |
[51] |
F. Zeng, C. Li, A new Crank–Nicolson finite element method for the time-fractional subdiffusion equation, Appl. Numer. Math., 121 (2017), 82–95. https://doi.org/10.1016/j.apnum.2017.06.011 doi: 10.1016/j.apnum.2017.06.011
![]() |
m | n | LM | TLM | ||||
AIT | ACPU | ANH | AIT | ACPU | ANH | ||
200 | 500 | 7.9 | 0.6960 | 5.0131×10-12 | 8.0 | 0.7015 | 6.0903×10-13 |
7.8 | 0.6974 | 5.5794×10-12 | 7.7 | 0.6906 | 1.2630×10-11 | ||
7.6 | 0.6703 | 8.5289×10-12 | 7.9 | 0.7025 | 3.5098×10-13 | ||
400 | 800 | 8.1 | 2.4705 | 5.5548×10-13 | 8.8 | 3.1241 | 5.2707×10-13 |
8.2 | 2.5097 | 7.6171×10-13 | 8.9 | 2.6100 | 2.2961×10-13 | ||
8.2 | 2.6300 | 2.4813×10-13 | 8.1 | 2.4039 | 3.6750×10-12 | ||
500 | 1000 | 8.1 | 4.4569 | 1.2136×10-12 | 8.1 | 4.3590 | 2.2894×10-12 |
8.4 | 4.7697 | 3.1192×10-13 | 8.4 | 4.4993 | 4.5153×10-12 | ||
8.2 | 4.8820 | 2.7039×10-12 | 8.4 | 4.4767 | 9.3738×10-13 | ||
600 | 1500 | 7.9 | 11.2160 | 9.7961×10-12 | 8.0 | 11.6639 | 1.0240×10-12 |
8.0 | 11.4230 | 1.0008×10-13 | 8.0 | 11.6522 | 9.3154×10-13 | ||
8.0 | 11.5575 | 1.0238×10-12 | 7.9 | 11.4497 | 1.0559×10-11 | ||
1000 | 1500 | 9.6 | 18.4934 | 5.6351×10-12 | 9.5 | 18.6699 | 1.6880×10-11 |
9.9 | 19.0396 | 5.2759×10-12 | 11.1 | 21.6384 | 5.9206×10-11 | ||
8.4 | 16.3751 | 1.2735×10-11 | 10.9 | 21.3177 | 7.6313×10-12 |
m | n | LM | TLM | ||||
AIT | ACPU | ANH | AIT | ACPU | ANH | ||
200 | 500 | 7.5 | 0.6642 | 1.5973×10-11 | 8.0 | 0.7155 | 9.7275×10-12 |
7.4 | 0.6675 | 8.8485×10-12 | 8.4 | 0.7429 | 8.7482×10-13 | ||
7.6 | 0.6661 | 2.5321×10-12 | 8.1 | 0.7167 | 6.7422×10-13 | ||
400 | 800 | 8.0 | 2.3642 | 2.4919×10-13 | 8.8 | 2.6212 | 4.6000×10-12 |
8.0 | 2.3791 | 4.5892×10-13 | 8.2 | 2.5740 | 2.4604×10-13 | ||
8.2 | 2.4293 | 9.2368×10-13 | 9.0 | 2.6885 | 1.3216×10-12 | ||
500 | 1000 | 8.0 | 4.3592 | 6.2691×10-13 | 8.3 | 4.5328 | 3.5736×10-12 |
8.1 | 4.3174 | 3.1221×10-13 | 8.2 | 4.3540 | 3.2290×10-13 | ||
7.9 | 4.2089 | 9.7440×10-12 | 9.9 | 5.3469 | 6.5691×10-12 | ||
600 | 1500 | 7.9 | 11.3807 | 9.2057×10-12 | 8.9 | 12.9567 | 9.7825×10-13 |
7.8 | 11.2766 | 1.3435×10-11 | 8.0 | 11.6116 | 9.9437×10-13 | ||
8.0 | 11.5494 | 9.8875×10-13 | 9.2 | 13.3792 | 1.0247×10-12 | ||
1000 | 1500 | 9.3 | 17.6422 | 7.8120×10-12 | 8.9 | 17.2609 | 3.4824×10-12 |
8.7 | 16.3247 | 4.9019×10-12 | 8.8 | 17.3407 | 4.7999×10-11 | ||
9.3 | 18.1968 | 7.8112×10-12 | 9.4 | 18.4024 | 1.3738×10-11 |
m | n | LM | TLM | ||||
AIT | ACPU | ANH | AIT | ACPU | ANH | ||
200 | 500 | 7.9 | 0.6960 | 5.0131×10-12 | 8.0 | 0.7015 | 6.0903×10-13 |
7.8 | 0.6974 | 5.5794×10-12 | 7.7 | 0.6906 | 1.2630×10-11 | ||
7.6 | 0.6703 | 8.5289×10-12 | 7.9 | 0.7025 | 3.5098×10-13 | ||
400 | 800 | 8.1 | 2.4705 | 5.5548×10-13 | 8.8 | 3.1241 | 5.2707×10-13 |
8.2 | 2.5097 | 7.6171×10-13 | 8.9 | 2.6100 | 2.2961×10-13 | ||
8.2 | 2.6300 | 2.4813×10-13 | 8.1 | 2.4039 | 3.6750×10-12 | ||
500 | 1000 | 8.1 | 4.4569 | 1.2136×10-12 | 8.1 | 4.3590 | 2.2894×10-12 |
8.4 | 4.7697 | 3.1192×10-13 | 8.4 | 4.4993 | 4.5153×10-12 | ||
8.2 | 4.8820 | 2.7039×10-12 | 8.4 | 4.4767 | 9.3738×10-13 | ||
600 | 1500 | 7.9 | 11.2160 | 9.7961×10-12 | 8.0 | 11.6639 | 1.0240×10-12 |
8.0 | 11.4230 | 1.0008×10-13 | 8.0 | 11.6522 | 9.3154×10-13 | ||
8.0 | 11.5575 | 1.0238×10-12 | 7.9 | 11.4497 | 1.0559×10-11 | ||
1000 | 1500 | 9.6 | 18.4934 | 5.6351×10-12 | 9.5 | 18.6699 | 1.6880×10-11 |
9.9 | 19.0396 | 5.2759×10-12 | 11.1 | 21.6384 | 5.9206×10-11 | ||
8.4 | 16.3751 | 1.2735×10-11 | 10.9 | 21.3177 | 7.6313×10-12 |
m | n | LM | TLM | ||||
AIT | ACPU | ANH | AIT | ACPU | ANH | ||
200 | 500 | 7.5 | 0.6642 | 1.5973×10-11 | 8.0 | 0.7155 | 9.7275×10-12 |
7.4 | 0.6675 | 8.8485×10-12 | 8.4 | 0.7429 | 8.7482×10-13 | ||
7.6 | 0.6661 | 2.5321×10-12 | 8.1 | 0.7167 | 6.7422×10-13 | ||
400 | 800 | 8.0 | 2.3642 | 2.4919×10-13 | 8.8 | 2.6212 | 4.6000×10-12 |
8.0 | 2.3791 | 4.5892×10-13 | 8.2 | 2.5740 | 2.4604×10-13 | ||
8.2 | 2.4293 | 9.2368×10-13 | 9.0 | 2.6885 | 1.3216×10-12 | ||
500 | 1000 | 8.0 | 4.3592 | 6.2691×10-13 | 8.3 | 4.5328 | 3.5736×10-12 |
8.1 | 4.3174 | 3.1221×10-13 | 8.2 | 4.3540 | 3.2290×10-13 | ||
7.9 | 4.2089 | 9.7440×10-12 | 9.9 | 5.3469 | 6.5691×10-12 | ||
600 | 1500 | 7.9 | 11.3807 | 9.2057×10-12 | 8.9 | 12.9567 | 9.7825×10-13 |
7.8 | 11.2766 | 1.3435×10-11 | 8.0 | 11.6116 | 9.9437×10-13 | ||
8.0 | 11.5494 | 9.8875×10-13 | 9.2 | 13.3792 | 1.0247×10-12 | ||
1000 | 1500 | 9.3 | 17.6422 | 7.8120×10-12 | 8.9 | 17.2609 | 3.4824×10-12 |
8.7 | 16.3247 | 4.9019×10-12 | 8.8 | 17.3407 | 4.7999×10-11 | ||
9.3 | 18.1968 | 7.8112×10-12 | 9.4 | 18.4024 | 1.3738×10-11 |