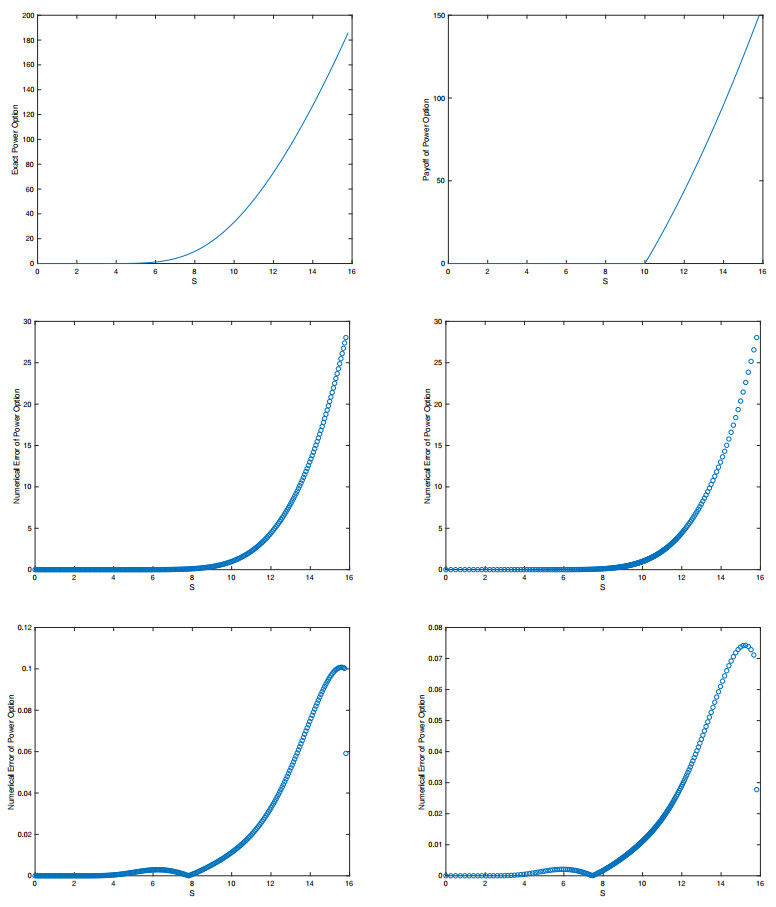
In this article, we will prove some new conformable fractional Hilbert-type dynamic inequalities on time scales. These inequalities generalize some known dynamic inequalities on time scales, unify and extend some continuous inequalities and their corresponding discrete analogues. Our results will be proved by using some algebraic inequalities, conformable fractional Hölder inequalities, and conformable fractional Jensen's inequalities on time scales.
Citation: Ahmed A. El-Deeb, Dumitru Baleanu, Nehad Ali Shah, Ahmed Abdeldaim. On some dynamic inequalities of Hilbert's-type on time scales[J]. AIMS Mathematics, 2023, 8(2): 3378-3402. doi: 10.3934/math.2023174
[1] | Min-Ku Lee, Jeong-Hoon Kim . Closed-form approximate solutions for stop-loss and Russian options with multiscale stochastic volatility. AIMS Mathematics, 2023, 8(10): 25164-25194. doi: 10.3934/math.20231284 |
[2] | Din Prathumwan, Thipsuda Khonwai, Narisara Phoochalong, Inthira Chaiya, Kamonchat Trachoo . An improved approximate method for solving two-dimensional time-fractional-order Black-Scholes model: a finite difference approach. AIMS Mathematics, 2024, 9(7): 17205-17233. doi: 10.3934/math.2024836 |
[3] | Boling Chen, Guohe Deng . Analytic approximations for European-style Asian spread options. AIMS Mathematics, 2024, 9(5): 11696-11717. doi: 10.3934/math.2024573 |
[4] | Zhongdi Cen, Jian Huang, Aimin Xu . A posteriori grid method for a time-fractional Black-Scholes equation. AIMS Mathematics, 2022, 7(12): 20962-20978. doi: 10.3934/math.20221148 |
[5] | Ashish Awasthi, Riyasudheen TK . An accurate solution for the generalized Black-Scholes equations governing option pricing. AIMS Mathematics, 2020, 5(3): 2226-2243. doi: 10.3934/math.2020147 |
[6] | Jafar Biazar, Fereshteh Goldoust . Multi-dimensional Legendre wavelets approach on the Black-Scholes and Heston Cox Ingersoll Ross equations. AIMS Mathematics, 2019, 4(4): 1046-1064. doi: 10.3934/math.2019.4.1046 |
[7] | Chao Yue, Chuanhe Shen . Fractal barrier option pricing under sub-mixed fractional Brownian motion with jump processes. AIMS Mathematics, 2024, 9(11): 31010-31029. doi: 10.3934/math.20241496 |
[8] | Yao Fu, Sisi Zhou, Xin Li, Feng Rao . Multi-assets Asian rainbow options pricing with stochastic interest rates obeying the Vasicek model. AIMS Mathematics, 2023, 8(5): 10685-10710. doi: 10.3934/math.2023542 |
[9] | Azadeh Ghanadian, Taher Lotfi . Approximate solution of nonlinear Black–Scholes equation via a fully discretized fourth-order method. AIMS Mathematics, 2020, 5(2): 879-893. doi: 10.3934/math.2020060 |
[10] | Kazem Nouri, Milad Fahimi, Leila Torkzadeh, Dumitru Baleanu . Numerical method for pricing discretely monitored double barrier option by orthogonal projection method. AIMS Mathematics, 2021, 6(6): 5750-5761. doi: 10.3934/math.2021339 |
In this article, we will prove some new conformable fractional Hilbert-type dynamic inequalities on time scales. These inequalities generalize some known dynamic inequalities on time scales, unify and extend some continuous inequalities and their corresponding discrete analogues. Our results will be proved by using some algebraic inequalities, conformable fractional Hölder inequalities, and conformable fractional Jensen's inequalities on time scales.
The well-known Black-Scholes (B-S) equation [1] for multi-asset option pricing is the following d-dimensional partial differential equation (PDE):
∂v(S,t)∂t+12d∑i,j=1σiσjρijSiSj∂2v(S,t)∂Si∂Sj+rd∑i=1Si∂v(S,t)∂Si−rv(S,t)=0, | (1.1) |
for (S,t)∈(0,∞)d×[0,T) with the final condition v(S,T)=vT(S), where v(S,t) is the value of the option in the multi-asset S=(S1,S2,…,Sd) at time t. T is the maturity time, σi is the volatility of underlying asset Si, ρij is the correlation between i-th and j-th assets, and r is the risk-free interest rate.
However most of the financial derivatives specially linear ones have analytical solutions, but they are not often easy to implement. Therefore the numerical methods would be suitable to find numerical approximations of them. Various numerical methods have been considered for one and multi-dimensional Black-Scholes equations. For instance, mesh free methods [9], high-order option pricing schemes [10,14], alternating direction implicit schemes [2,7] and etc. Furthermore, not only the numerical methods are so common to implement for the financial derivatives, but also they have been implemented for other variety problems and equations frequently [3,11,12,15].
One of the most common and simple numerical methods to implement for solving PDEs is FDMs. The main problem of FDMs for pricing multi-asset options with non-linear payoffs is significant numerical errors around their boundary points and also the strike price K. Several studies have been done to achieve an accurate numerical solution of multi-asset options. Jeong et al. [8] proposed a useful remedy to reduce numerical error around Smax by using the standard Monte Carlo simulation at this boundary point. Here we improved their methodology by using the antithetic variate method in Monte Carlo simulation [4], and furthermore a space transformation [13,16] that causes more nodal points around the strike price to get more robust solution.
Two-dimensional B-S equation with two assets x=S1 and y=S2 is the following PDE:
∂u∂τ=12σ2xx2∂2u∂x2+12σ2yy2∂2u∂y2+σxσyρ∂2u∂x∂y+rx∂u∂x+ry∂u∂y−ru, | (2.1) |
for (x,y,τ)∈Ω×[0,T) with the initial condition u(x,y,0)=u0(x,y) which is the Eq (1.1) with d=2 and a variable changing τ=T−t in the truncated domain Ω=(0,xmax)×(0,ymax). Suppose LBS is the operator of the right hand side of Eq (2.1). Now we discretize the time interval with a uniform time step Δτ=T/Nτ and the following grid stretching transformation that makes non-uniform space discretization of the interval [xmin,xmax] with more nodal points around K:
x=K(1+sinh(c2w+c1(1−w))15), for w∈[0,1], | (2.2) |
where c1=arcsinh(15(xmin−K)/K) and c2=arcsinh(15(xmax−K)/K). Therefore x(w)∈[xmin,xmax] for any w∈[0,1]. The grid stretching transformation by locating more nodal points around the strike price K, leads to reduce the numerical errors around K for the options with non-smooth payoffs which can be applied to even higher order finite difference methods in both single and multi-asset Black-Scholes equations. At first for the x-direction, we discretize the interval [0,1] uniformly to Nx sub-intervals, then we use the transformation (2.2) in [0,xmax] and define hxi=xi+1−xi for i=0,…,Nx−1. we discretize the interval [0,ymax] to Ny sub-intervals similarly. Let for i=0,…,Nx, j=0,…,Ny and n=0,…,Nτ define unij≈u(xi,yj,τn) and use the operator splitting method for solving Eq (2.1) with the following two discrete fractional time step equations:
un+12ij−unijΔτ=LxBS(αun+12ij+βunij), | (2.3) |
un+1ij−un+12ijΔτ=LyBS(αun+1ij+βun+12ij), | (2.4) |
where α≥0, β≥0 and α+β=1. We can choose different sets of values of α and β to achieve us implicit or explicit schemes in both (2.3) and (2.4). These schemes have first-order accuracy in general [5]. Here we take the implicit scheme by choosing α=1 and β=0 for both Eqs (2.3) and (2.4) which leads to a consistent and stable scheme and thereby the scheme is convergent with the first order of accuracy in time. Furthermore, with the operators (LxBSu)n+12ij and (LyBSu)n+1ij as follows, we obtain the second order of accuracy in space as the central finite difference approximations have been used for the space derivatives.
(LxBSu)n+12ij=(σxxi)22Dxxun+12ij+σxσyρxiyj2Dxyunij+rxiDxun+12ij−r2un+12ij, | (2.5) |
(LyBSu)n+1ij=(σyyj)22Dyyun+1ij+σxσyρxiyj2Dxyun+12ij+ryjDyun+1ij−r2un+1ij, | (2.6) |
where the derivatives approximations are defined as
Dxxuij≈2hxi−1(hxi−1+hxi)ui−1,j−2hxi−1hxiuij+2hxi(hxi−1+hxi)ui+1,j, | (2.7) |
Dxyuij≈ui+1,j+1−ui−1,j+1−ui+1,j−1+ui−1,j−1hxihyj+hxi−1hyj+hxihyj−1+hxi−1hyj−1, | (2.8) |
Dxuij≈−hxihxi−1(hxi−1+hxi)ui−1,j+hxi−hxi−1hxi−1hxiuij+hxi−1hxi(hxi−1+hxi)ui+1,j. | (2.9) |
By substituting above derivatives in Eq (2.3), the following equations will be achieved:
αiun+12i−1,j=βiun+12i,j+γiun+12i+1,j=fij, i=1,…,Nx, | (2.10) |
where αi, βi and γi are the lower, main and upper diagonal of the tridiagonal matrix of the above system of equations respectively and fij is the constant vector of the system which are defined as:
αi=−(σxxi)2hxi−1(hxi−1+hxi)+rxihxihxi−1(hxi−1+hxi), | (2.11) |
βi=1Δτ+(σxxi)2hxi−1hxi−rxi(hxi−hxi−1)hxi−1hxi+r2, | (2.12) |
γi=−(σxxi)2hxi(hxi−1+hxi)−rxihxi−1hxi(hxi−1+hxi), | (2.13) |
fnij=12σxσyρxiyjDxyunij−1Δτunij, | (2.14) |
for fixed j and the linear boundary condition at the boundaries. For instance u0j=2u1j−u2j, for j=1,…,Ny and similarly for uNx,j, ui0 and ui,Ny or at the end point of the domain, we apply the Monte Carlo simulation with the antithetic variate method (AMC) or the standard Monte Carlo simulation (MC). The solution of the above system of equations will be the vector u121:Nx,j. Similarly by substituting the derivatives approximations with respect to y in (2.4), we have another system of equations to solve for finding the value of the two-asset option.
Note that with the AMC and the MC simulations we obtain the option value VAMC and VMC respectively at time t=0 and (x,y)=(xmax,ymax) as follow:
VAMC=e−rTMM∑m=1[12(Λ(Smax)+Λ(˜Smax))], | (2.15) |
VMC=e−rTMM∑m=1(Λ(Smax)), | (2.16) |
where
Smax=[xmax×e((r−Φ22)T+Σ(1,:).z.√T),ymax×e((r−Φ22)T+Σ(2,:).z.√T)], | (2.17) |
˜Smax=[xmax×e((r−Φ22)T−Σ(1,:).z.√T),ymax×e((r−Φ22)T−Σ(2,:).z.√T)], | (2.18) |
and M is the number of replications in the antithetic and the standard Monte Carlo simulation which in this work we choose M=106, Λ is a payoff function, z, Σ and Φ are the random vector from a standard normal distribution, the volatility matrix and a vector with the size of multi-asset respectively. For instance, for the case of two-asset Σ and Φ are as follows:
Σ=[σ11σ12σ21σ22], | (2.19) |
Φ=√diag(Σ×Σ′)=[√σ11×σ12√σ21×σ22], | (2.20) |
where the elements of the volatility matrix will be obtained by solving the following system of equations:
σx=√σ211+σ212, | (2.21) |
σy=√σ221+σ222, | (2.22) |
ρ=σ11σ21+σ12σ22√(σ211+σ212)(σ221+σ222). | (2.23) |
Then with the exponential interpolation between V (which is VAMC or VMC) and the payoff value u0Nx,Ny, we obtain the option price at the end point of the domain for every time step:
unNx,Ny=u0Nx,Ny(Vu0Nx,Ny)nΔτT, n=0,…,Nτ. | (2.24) |
Here we solve numerically the B-S equation in one and two-dimensional space for different options with these parameters: T=1, K1=K2=100, r=0.03, σx=σy=0.3, ρ=0.5.
A power call option has a payoff max(xp−K,0) where p∈R+ is a power. The closed-form solution of the power option is given by [18]:
u(x,τ)=xpe(p−1)(r+pσ2/2)τN(d1)−Ke−rτN(d2) | (3.1) |
where
d1=ln(xp√K)+(r+(p−0.5)σ2)τσ√τ,d2=d1−pσ√τ. | (3.2) |
Note that the power option with p=1 is the call option. Figure 1 shows the comparison of the numerical errors of one-asset power call option with different schemes: The equidistance discretization in space with the linear boundary condition (Equi&L), the grid stretching discretization around the strike price with linear boundary condition (GS&L), the equidistance discretization in space with Monte Carlo simulation at Smax (Equi&MC) and the grid stretching discretization with the antithetic Monte Carlo simulation at Smax (GS&AMC).
Table 1 shows the RMSE of the power option with Nx=250 and Δτ=1720 for p=1, p=2 and p=3 in the whole domain Ω=(0,Smax) and the interesting domain around the strike price Ωe=[0.7p√K,1.3p√K] for the different schemes and different Smax. Keep in mind that in the grid stretching transformation (2.2) we must substitute p√K instead of K. We can see the MC and AMC simulation at the end point of the domain reduce RMSE significantly for bigger p and none of the GS, MC and AMC improve the accuracy of the numerical approximation for the call option (p=1).
Smax | 150 | 200 | 250 | 300 | 350 | 400 | 450 | |
Equi&L | 0.3452 | 0.0271 | 0.0024 | 0.0009 | 0.0008 | 0.0008 | 0.0008 | |
p=1 | GS&L | 0.2737 | 0.0165 | 0.0022 | 0.0017 | 0.0016 | 0.0016 | 0.0015 |
Ω | Equi&MC | 0.0457 | 0.0172 | 0.0072 | 0.0511 | 0.0148 | 0.0038 | 0.0419 |
GS&AMC | 0.0457 | 0.0033 | 0.0032 | 0.0023 | 0.0023 | 0.0017 | 0.0024 | |
Equi&L | 0.1505 | 0.0015 | 0.0015 | 0.0016 | 0.0017 | 0.0018 | 0.0019 | |
p=1 | GS&L | 0.1122 | 0.0027 | 0.0024 | 0.0022 | 0.0021 | 0.0021 | 0.0020 |
ΩK | Equi&MC | 0.0563 | 0.0016 | 0.0015 | 0.0016 | 0.0017 | 0.0018 | 0.0019 |
GS&AMC | 0.0472 | 0.0023 | 0.0024 | 0.0022 | 0.0021 | 0.0021 | 0.0020 | |
Smax | √150 | √200 | √250 | √300 | √350 | √400 | √450 | |
Equi&L | 6.8424 | 6.8064 | 7.7089 | 8.9340 | 10.2820 | 11.6811 | 13.1029 | |
p=2 | GS&L | 8.4653 | 6.1009 | 5.8106 | 6.0578 | 6.4852 | 6.9842 | 7.5149 |
Ω | Equi&MC | 0.2272 | 0.0685 | 0.0319 | 0.0205 | 0.0457 | 0.0926 | 0.0863 |
GS&AMC | 0.3311 | 0.0253 | 0.0244 | 0.0345 | 0.0266 | 0.0364 | 0.0348 | |
Equi&L | 10.4749 | 5.5909 | 2.9152 | 1.7040 | 1.0414 | 0.6631 | 0.4350 | |
p=2 | GS&L | 9.6261 | 4.6061 | 2.3610 | 1.3371 | 0.8022 | 0.5133 | 0.3308 |
ΩK | Equi&MC | 0.3478 | 0.0661 | 0.0193 | 0.0170 | 0.0115 | 0.0161 | 0.0106 |
GS&AMC | 0.3765 | 0.0245 | 0.0165 | 0.0203 | 0.0146 | 0.0117 | 0.0081 | |
Smax | 3√150 | 3√200 | 3√250 | 3√300 | 3√350 | 3√400 | 3√450 | |
Equi&L | 19.3431 | 22.0844 | 25.8076 | 29.9848 | 34.3954 | 38.9355 | 43.5521 | |
p=3 | GS&L | 28.5178 | 25.4178 | 25.0462 | 25.8940 | 27.3175 | 29.0375 | 30.9201 |
Ω | Equi&MC | 1.2650 | 0.6127 | 0.4785 | 0.3064 | 0.3175 | 0.2029 | 0.1936 |
GS&AMC | 1.9696 | 0.9038 | 0.5715 | 0.3920 | 0.3462 | 0.2689 | 0.2511 | |
Equi&L | 31.1024 | 33.1054 | 28.4051 | 21.9288 | 17.3308 | 14.1742 | 11.8378 | |
p=3 | GS&L | 32.8550 | 28.7798 | 23.0077 | 17.4396 | 13.9633 | 11.2298 | 9.3862 |
ΩK | Equi&MC | 2.0303 | 0.9183 | 0.6883 | 0.4127 | 0.3427 | 0.2148 | 0.1607 |
GS&AMC | 2.2680 | 1.0233 | 0.6413 | 0.4100 | 0.3167 | 0.2264 | 0.1849 |
Now we consider a European call option on the maximum of two assets with the payoff u(x,y,0)=max{max{x,y}−K,0}. Then the following exact analytical solutions of the option [17] will be compared to the numerical solutions.
u(x,y,τ)=xM(d1,d;ρ1)+yM(d2,−d+σ√τ;ρ2)−Ke−rτ[1−M(−d1+σ1√τ,−d2+σ2√τ;ρ)], | (3.3) |
where M is the cumulative bivariate normal distribution function and defined as
M(a,b;ρ)=12π√1−ρ2∫b−∞∫a−∞exp(−x2−2ρxy+y22(1−ρ2))dxdy, | (3.4) |
and other parameters are as follows:
d1=ln(xK)+(r+σ2x2)Tσx√T,d2=ln(yK)+(r+σ2y2)Tσy√T,d=ln(xy)+σ22Tσ√T, | (3.5) |
σ=√σ2x+σ2y−2ρσxσy, ρ1=(σx−ρσy)/σ, ρ2=(σy−ρσx)/σ. | (3.6) |
Figure 2 shows the comparison of the numerical solutions of the call option on the maximum of two assets with the different schemes and (xmax,ymax)=(250,250).
Now in Table 2 we compare the RMSE of these four schemes in the whole region Ω=(0,xmax)×(0,ymax) and in the most interesting region ΩK=(0.7K,1.3K)×(0.7K,1.3K) with different domain sizes (xmax,ymax)=(L,L) and Nx=Ny=L at t=0.
L | 150 | 200 | 250 | 300 | |
Equi&L | 12.1428 | 16.9562 | 22.3907 | 28.4710 | |
GS&L | 9.1971 | 5.7377 | 5.4052 | 5.5654 | |
Ω | Equi&MC | 2.6188 | 3.0868 | 3.8931 | 4.6910 |
GS&AMC | 2.2287 | 1.5009 | 1.7865 | 2.0569 | |
Equi&L | 8.5065 | 0.7793 | 0.0690 | 0.0084 | |
GS&L | 6.1609 | 0.4476 | 0.0354 | 0.0051 | |
ΩK | Equi&MC | 2.1547 | 0.2287 | 0.0225 | 0.0048 |
GS&AMC | 1.9339 | 0.1858 | 0.0178 | 0.0040 |
A European call on the minimum of two assets has the payoff u(x,y,0)=max{min{x,y}−K,0} and the closed form solution as follows [17]
u(x,y,τ)=xM(d1,−d;−ρ1)+yM(d2,d−σ√τ;−ρ2)−Ke−rτM(d1−σ1√τ,d2−σ2√τ;ρ). | (3.7) |
where its parameters are the same as the European call option on the maximum of two assets. In Figure 3 and Table 3 we compare the four above mentioned schemes for this two-asset option.
L | 150 | 200 | 250 | 300 | |
Equi&L | 11.4121 | 16.5529 | 21.9750 | 28.0137 | |
GS&L | 8.7458 | 5.6337 | 5.1434 | 5.1969 | |
Ω | Equi&MC | 0.9972 | 1.4289 | 1.8595 | 3.7692 |
GS&AMC | 0.8470 | 0.7205 | 0.6078 | 0.5893 | |
Equi&L | 8.2864 | 0.7763 | 0.0666 | 0.0062 | |
GS&L | 5.9848 | 0.4443 | 0.0330 | 0.0030 | |
ΩK | Equi&MC | 1.0381 | 0.1382 | 0.0128 | 0.0029 |
GS&AMC | 0.6490 | 0.0951 | 0.0087 | 0.0016 |
Here we consider a two-asset correlation call option wich pays off:
u(x,y,0)={y−K2 ,if x>K10 ,otherwise | (3.8) |
This option has been priced by [18]:
u(x,y,τ)=yM(dy+σy√τ,dx+ρσy√τ;ρ)−Ke−rτM(dy,dx;ρ), | (3.9) |
where ρ is the correlation coefficient between the returns on the two assets and
dx=ln(xK)+(r−σ2x2)Tσx√T, dy=ln(yK)+(r−σ2y2)Tσy√T. | (3.10) |
Now we solve numerically this option. Figure 4 shows the comparison of the numerical solutions of two-asset correlation call option with the different schemes.
Table 4 shows the RMSE of the equidistance discretization (Equi) and the grid stretching discretization around the strike price with Monte Carlo simulation at the last point of the boundary (xmax,ymax)=(L,L) (GS&MC)with different L in the whole region Ω=[0,L]×[0,L] and the most interesting region ΩK=[0.7K,1.3K]×[0.7K,1.3K] respectively.
L | 150 | 200 | 250 | 300 | |
Equi&L | 0.8040 | 0.5688 | 0.6639 | 0.7581 | |
GS&L | 0.7340 | 0.6739 | 0.9008 | 0.9729 | |
Ω | Equi&MC | 0.2578 | 0.3740 | 0.5793 | 0.8419 |
GS&AMC | 0.1999 | 0.5024 | 0.8245 | 0.8788 | |
Equi&L | 0.2386 | 0.1022 | 0.1023 | 0.1023 | |
GS&L | 0.1803 | 0.0213 | 0.0181 | 0.0108 | |
ΩK | Equi&MC | 0.2582 | 0.1255 | 0.1047 | 0.1026 |
GS&AMC | 0.2355 | 0.0474 | 0.0171 | 0.0110 |
A butterfly option can be regarded as a combination of two long calls with strikes K1 and K2 and two short calls both with strike K=(K1+K2)/2. Thus, for given values K1,K2>0 with K1<K2, the payoff of a two-asset European butterfly option is:
u(x,y,0)=max{max{x,y}−K1,0}+max{max{x,y}−K2,0}−2max{max{x,y}−K,0}, | (3.11) |
and has the following analytical solution:
u(x,y,τ)=x[M(d(1)1,d;ρ1)−2M(d1,d;ρ1)+M(d(2)1,d;ρ1)]+y[M(d(1)2,−d+σ√τ;ρ2)−2M(d2,−d+σ√τ;ρ2)+M(d(2)2,−d+σ√τ;ρ2)]−K1e−rτ[1−M(−d(1)1+σx√τ,−d(1)2+σy√τ;ρ)]+2Ke−rτ[1−M(−d1+σx√τ,−d2+σy√τ;ρ)]−K2e−rτ[1−M(−d(2)1+σx√τ,−d(2)2+σy√τ;ρ)], |
where the M(a,b;ρ) is the cumulative bivariate normal distribution function (3.4) and other parameters are defined in (3.5) and (3.6). where d(i)1 and d(i)2 will be obtained by substituting Ki in 3.5 for i=1,2.
Here we consider a two-asset butterfly option with K1=50, K2=150. Figure 5 shows the comparison of the numerical solutions of the two-asset butterfly option with the equidistance and the grid stretching discretization schemes. Since u0Nx,Ny=0 we can not use the Monte Carlo simulation at the end point of the domain in (2.24).
Now in Table 5 we compare the RMSE of the equidistance discretization (Equi) and the grid stretching discretization (GS) for the space in the whole region Ω=(xmax,ymax) and the most interesting region ΩK=[0.7K1,1.3K2]×[0.7K1,1.3K2] with different domain sizes (xmax,ymax)=(L,L) and Nx=Ny=L at t=0. We can see in this option the GS scheme does not have any superiority over Equi for the bigger domain.
L | 200 | 250 | 300 | 350 | |
Equi | 1.0136 | 0.2986 | 0.2065 | 0.1753 | |
Ω | GS | 0.5842 | 0.2733 | 0.2258 | 0.1946 |
Equi | 0.8978 | 0.0174 | 0.0043 | 0.0042 | |
ΩK | GS | 0.5099 | 0.0098 | 0.0052 | 0.0049 |
The payoff function of a two-asset cash-or-nothing option is given by
u(x,y,0)={c,if x≥K1,y≥K20,otherwise, | (3.12) |
which c is a constant. The closed form solution for the two-asset cash-or-nothing option is as follows
u(x,y,τ)=ce−rτM(dx,dy;ρ), | (3.13) |
where M(a,b;ρ) is the cumulative bivariate normal distribution function (3.4) and dx and dy are the same as (3.10). Now we consider a two-asset cash-or-nothing call option with c=100, then the exact analytical solutions of the option [6] will be compared to the numerical solutions. Figure 6 shows the comparison of the numerical solutions of the option with the different four schemes and also Table 6 demonstrates the RMSE of the four schemes with different domain sizes (xmax,ymax)=(L,L), Nx=Ny=L and Δτ=1720 in the whole region Ω and the in interesting region ΩK. Since the payoff of this option is constant at the end point of the domain, we can see that the MC and AMC simulations do not have any superiority for the bigger domain.
L | 150 | 200 | 250 | 300 | |
Equi&L | 3.6561 | 1.1174 | 0.9870 | 0.9458 | |
GS&L | 3.0292 | 0.6828 | 0.5302 | 0.3400 | |
Ω | Equi&MC | 1.3850 | 1.0302 | 0.9847 | 0.9462 |
GS&AMC | 1.1707 | 0.6454 | 0.5294 | 0.3400 | |
Equi&L | 0.0429 | 0.0218 | 0.0217 | 0.0217 | |
GS&L | 0.0237 | 0.0051 | 0.0042 | 0.0024 | |
ΩK | Equi&MC | 0.0250 | 0.0217 | 0.0217 | 0.0217 |
GS&AMC | 0.0047 | 0.0050 | 0.0042 | 0.0024 |
We applied the FDM for solving numerically one and two-dimensional B-S equations with different space discretization and boundary conditions. Also, RMSE has been applied for estimating error of the method as it is common to use and consider the numerical errors for all nodal points of the region. Numerical experiments illustrated that by applying the antithetic Monte Carlo simulation for the end point of the space domain and discretization with grid stretching to put more nodal points around the non-smooth point of the payoff function, we obtained more accurate numerical solutions in the considered exotic options with non-constant payoff at the end point of the space domain. These remedies can be considered and implemented for other options, higher dimensional models, nonlinear B-S equations and also other financial derivatives.
The authors would like to thank the reviewers for their helpful suggestions and comments, which improved the quality of this article.
The authors declare that they have no conflicts of interest.
[1] | G. H. Hardy, J. E. Littlewood, G. Polya, Inequalities, Cambridge Univ. Press, Cambridge, 1952. |
[2] |
B. G. Pachpatte, A note on Hilbert type inequality, Tamkang J. Math., 29 (1998), 293–298. https://doi.org/10.5556/j.tkjm.29.1998.4258 doi: 10.5556/j.tkjm.29.1998.4258
![]() |
[3] |
G. D. Handley, J. J. Koliha, J. Pecaric, A Hilbert type inequality, Tamkang J. Math., 31 (2000), 311–316. https://doi.org/10.5556/j.tkjm.31.2000.389 doi: 10.5556/j.tkjm.31.2000.389
![]() |
[4] |
C. J. Zhao, W. S. Cheung, Inverses of new Hilbert-Pachpatte-type inequalities, J. Inequal. Appl., 2006 (2006), 97860. https://doi.org/10.1155/JIA/2006/97860 doi: 10.1155/JIA/2006/97860
![]() |
[5] |
B. G. Pachpatte, On some new inequalities similar to Hilbert's inequality, J. Math. Anal. Appl., 226 (1998), 166–179. https://doi.org/10.1006/jmaa.1998.6043 doi: 10.1006/jmaa.1998.6043
![]() |
[6] |
A. A. El-Deeb, H. A. El-Sennary, P. Agarwal, Some opial-type inequalities with higher order delta derivatives on time scales, RACSAM Rev. R. Acad. A, 114 (2020), 29. https://doi.org/10.1007/s13398-019-00749-7 doi: 10.1007/s13398-019-00749-7
![]() |
[7] |
A. A. El-Deeb, Z. A. Khan, Certain new dynamic nonlinear inequalities in two independent variables and applications, Bound. Value Probl., 2020 (2020), 31. https://doi.org/10.1186/s13661-020-01338-z doi: 10.1186/s13661-020-01338-z
![]() |
[8] |
B. C. Yang, S. H. Wu, Q. Chen, A new extension of Hardy-Hilbert's inequality containing kernel of double power functions, Mathematics, 8 (2020), 894. https://doi.org/10.3390/math8060894 doi: 10.3390/math8060894
![]() |
[9] |
A. A. El-Deeb, H. Xu, A. Abdeldaim, G. Wang, Some dynamic inequalities on time scales and their applications, Adv. Differ. Equ., 2019,130. https://doi.org/10.1186/s13662-019-2023-6 doi: 10.1186/s13662-019-2023-6
![]() |
[10] |
A. A. El-Deeb, On some generalizations of nonlinear dynamic inequalities on time scales and their applications, Appl. Anal. Discret. Math., 13 (2019), 440–462. https://doi.org/10.2298/AADM170406010E doi: 10.2298/AADM170406010E
![]() |
[11] |
Y. Tian, A. A. El-Deeb, F. Meng, Some nonlinear delay Volterra-Fredholm type dynamic integral inequalities on time scales, Discrete Dyn. Nat. Soc., 2018 (2018), 5841985. https://doi.org/10.1155/2018/5841985 doi: 10.1155/2018/5841985
![]() |
[12] |
A. A. El-Deeb, W. S. Cheung, A variety of dynamic inequalities on time scales with retardation, J. Nonlinear Sci. Appl., 11 (2018), 1185–1206. https://doi.org/10.22436/jnsa.011.10.07 doi: 10.22436/jnsa.011.10.07
![]() |
[13] | A. A. El-Deeb, H. A. Elsennary, E. R. Nwaeze, Generalized weighted Ostrowski, trapezoid and Gruss type inequalities on time scales, Fasc. Math., 2018,123–144. |
[14] |
A. Abdeldaim, A. A. El-Deeb, P. Agarwal, H. A. El-Sennary, On some dynamic inequalities of Steffensen type on time scales, Math. Method. Appl. Sci., 41 (2018), 4737–4753. https://doi.org/10.1002/mma.4927 doi: 10.1002/mma.4927
![]() |
[15] |
A. A. El-Deeb, Some Gronwall-Bellman type inequalities on time scales for Volterra-Fredholm dynamic integral equations, J. Egypt. Math. Soc., 26 (2018), 1–17. https://doi.org/10.21608/JOMES.2018.9457 doi: 10.21608/JOMES.2018.9457
![]() |
[16] |
A. A. El-Deeb, S. D. Makharesh, D. Baleanu, Dynamic Hilbert-type inequalities with fenchel-legendre transform, Symmetry, 12 (2020), 582. https://doi.org/10.3390/sym12040582 doi: 10.3390/sym12040582
![]() |
[17] | M. Kh. Fatma, A. A. El-Deeb, A Abdeldaim, Z. A Khan, On some generalizations of dynamic Opial-type inequalities on time scales, Adv. Differ. Eq., 2019 (2019), 1–14. |
[18] |
A. A. El-Deeb, M. Kh. Fatma, G. A. F. Ismail, Z. A. Khan, Weighted dynamic inequalities of Opial-type on time scales, Adv. Differ. Eq., 2019 (2019), 393. https://doi.org/10.1186/s13662-019-2325-8 doi: 10.1186/s13662-019-2325-8
![]() |
[19] |
B. C. Yang, S. H. Wu, A. Z. Wang, A new Hilbert-type inequality with positive homogeneous kernel and its equivalent forms, Symmetry, 12 (2020), 342. https://doi.org/10.3390/sym12030342 doi: 10.3390/sym12030342
![]() |
[20] | R. Agarwal, D. O'Regan, S. Saker, Dynamic inequalities on time scales, Springer, Cham, 2014. |
[21] |
S. H. Saker, A. A. El-Deeb, H. M. Rezk, R. P. Agarwal, On Hilbert's inequality on time scales, Appl. Anal. Discrete Math., 11 (2017), 399–423. https://doi.org/10.2298/AADM170428001S doi: 10.2298/AADM170428001S
![]() |
[22] |
S. Rashid, Z. Hammouch, H. Aydi, A. G. Ahmad, A. M. Alsharif, Novel computations of the time-fractional fisher's model via generalized fractional integral operators by means of the elzaki transform, Fractal Fract., 5 (2021). https://doi.org/10.3390/fractalfract5030094 doi: 10.3390/fractalfract5030094
![]() |
[23] |
S. S. Zhou, S. Rashid, S. Parveen, A. O. Akdemir, Z. Hammouch, New computations for extended weighted functionals within the hilfer generalized proportional fractional integral operators, AIMS Math., 6 (2021), 4507–4525. https://doi.org/10.3934/math.2021267 doi: 10.3934/math.2021267
![]() |
[24] |
A. A. El-Deeb, D. Baleanu, Some new dynamic Gronwall-Bellman-Pachpatte type inequalities with delay on time scales and certain applications, J. Inequal. Appl., 2020 (2022). https://doi.org/10.1186/s13660-022-02778-0 doi: 10.1186/s13660-022-02778-0
![]() |
[25] |
A. A. El-Deeb, O. Moaaz, D. Baleanu, S. S. Askar, A variety of dynamic α-conformable Steffensen-type inequality on a time scale measure space, AIMS Math., 7 (2022), 11382–11398. https://doi.org/10.3934/math.2022635 doi: 10.3934/math.2022635
![]() |
[26] |
A. A. El-Deeb, E. Akin, B. Kaymakçalan, Generalization of Mitrinović-Pečarić inequalities on time scales, Rocky MT J. Math., 51 (2021), 1909–1918. https://doi.org/10.1216/rmj.2021.51.1909 doi: 10.1216/rmj.2021.51.1909
![]() |
[27] |
A. A. El-Deeb, S. D. Makharesh, E. R. Nwaeze, O. S. Iyiola, D. Baleanu, On nabla conformable fractional Hardy-type inequalities on arbitrary time scales, J. Inequal. Appl., 2021 (2021), 192. https://doi.org/10.1186/s13660-021-02723-7 doi: 10.1186/s13660-021-02723-7
![]() |
[28] |
T. Abdeljawad, S. Rashid, Z. Hammouch, I. Işcan, Y. M. Chu, Some new simpson-type inequalities for generalized p-convex function on fractal sets with applications, Adv. Differ. Eq., 2020 (2020), 1–26. https://doi.org/10.1186/s13662-020-02955-9 doi: 10.1186/s13662-020-02955-9
![]() |
[29] |
H. G. JiLe, S. Rashid, M. A. Noor, A. Suhail, Y. M. Chu, Some unified bounds for exponentially tgs-convex functions governed by conformable fractional operators, AIMS Math., 5 (2020), 6108–6123. https://doi.org/10.3934/math.2020392 doi: 10.3934/math.2020392
![]() |
[30] |
S. Rashid, Z. Hammouch, H. Kalsoom, R. Ashraf, Y. M. Chu, New investigation on the generalized k-fractional integral operators, Front. Phys., 8 (2020). https://doi.org/10.3389/fphy.2020.00025 doi: 10.3389/fphy.2020.00025
![]() |
[31] |
M. A. Noor, K. I. Noor, S. Rashid, Some new classes of preinvex functions and inequalities, Mathematics, 7 (2019). https://doi.org/10.3390/math7010029 doi: 10.3390/math7010029
![]() |
[32] |
A. Abdeldaim, A. A. El-Deeb, On generalized of certain retarded nonlinear integral inequalities and its applications in retarded integro-differential equations, Appl. Math. Comput., 256 (2015), 375–380. https://doi.org/10.1016/j.amc.2015.01.047 doi: 10.1016/j.amc.2015.01.047
![]() |
[33] | M. Bohner, A. Peterson, Dynamic equations on time scales: An introduction with applications, Birkhauser, Boston, Mass, USA, 2001. |
[34] |
R. Khalil, M. A. Horani, A. Yousef, M. Sababheh, A new definition of fractional derivative, J. Comput. Appl. Math., 264 (2014), 65–70. https://doi.org/10.1016/j.cam.2014.01.002 doi: 10.1016/j.cam.2014.01.002
![]() |
[35] |
T. Abdeljawad, On conformable fractional calculus, J. Comput. Appl. Math., 279 (2015), 57–66. https://doi.org/10.1016/j.cam.2014.10.016 doi: 10.1016/j.cam.2014.10.016
![]() |
[36] |
N. Benkhettou, S. Hassani, D. F. Torres, A conformable fractional calculus on arbitrary time scales, J. King Saud Univ. Sci., 28 (2016), 93–98. https://doi.org/10.1016/j.jksus.2015.05.003 doi: 10.1016/j.jksus.2015.05.003
![]() |
[37] | B. Bendouma, A. Hammoudi, A nabla conformable fractional calculus on time scales, Elec. J. Math. Anal. Appl., 7 (2019), 202–216. |
[38] |
R. Bibi, M. Bohner, J. Pečarić, S. Varošanec, Minkowski and Beckenbach-Dresher inequalities and functionals on time scales, J. Math. Inequal., 7 (2013), 299–312. https://doi.org/10.7153/jmi-07-28 doi: 10.7153/jmi-07-28
![]() |
[39] |
C. J. Zhao, W. S. Cheung, On new Hilbert-Pachpatte type integral inequalities, Taiwan. J. Math., 2010. 1271–1282. https://doi.org/10.11650/twjm/1500405943 doi: 10.11650/twjm/1500405943
![]() |
[40] |
A. A. El-Deeb, S. D. Makharesh, B. Almarri, Some new inverse Hilbert inequalities on time scales, Symmetry, 14 (2022), 2234. https://doi.org/10.3390/sym14112234 doi: 10.3390/sym14112234
![]() |
[41] | A. A. El-Deeb, J Awrejcewicz, Diamond-α Hardy-type inequalities on time scales, Symmetry, 2047. |
[42] | A. A. El-Deeb, A. A. El-Bary, J. Awrejcewicz, On some dynamic (ΔΔ)∇ Gronwall-Bellman-Pachpatte-type inequalities on time scales and its applications, Symmetry, 14 (1902). |
[43] | A. A. El-Deeb, D. Baleanu, J. Awrejcewicz, (Δ∇)∇-Pachpatte dynamic inequalities associated with Leibniz integral rule on time scales with applications, Symmetry, 14 (1867). |
1. | Y. Ma, C.Z. Shi, Y.C. Hon, Generalized Finite Integration Method with Laplace transform for European option pricing under Black–Scholes and Heston models, 2024, 164, 09557997, 105751, 10.1016/j.enganabound.2024.105751 |
Smax | 150 | 200 | 250 | 300 | 350 | 400 | 450 | |
Equi&L | 0.3452 | 0.0271 | 0.0024 | 0.0009 | 0.0008 | 0.0008 | 0.0008 | |
p=1 | GS&L | 0.2737 | 0.0165 | 0.0022 | 0.0017 | 0.0016 | 0.0016 | 0.0015 |
Ω | Equi&MC | 0.0457 | 0.0172 | 0.0072 | 0.0511 | 0.0148 | 0.0038 | 0.0419 |
GS&AMC | 0.0457 | 0.0033 | 0.0032 | 0.0023 | 0.0023 | 0.0017 | 0.0024 | |
Equi&L | 0.1505 | 0.0015 | 0.0015 | 0.0016 | 0.0017 | 0.0018 | 0.0019 | |
p=1 | GS&L | 0.1122 | 0.0027 | 0.0024 | 0.0022 | 0.0021 | 0.0021 | 0.0020 |
ΩK | Equi&MC | 0.0563 | 0.0016 | 0.0015 | 0.0016 | 0.0017 | 0.0018 | 0.0019 |
GS&AMC | 0.0472 | 0.0023 | 0.0024 | 0.0022 | 0.0021 | 0.0021 | 0.0020 | |
Smax | √150 | √200 | √250 | √300 | √350 | √400 | √450 | |
Equi&L | 6.8424 | 6.8064 | 7.7089 | 8.9340 | 10.2820 | 11.6811 | 13.1029 | |
p=2 | GS&L | 8.4653 | 6.1009 | 5.8106 | 6.0578 | 6.4852 | 6.9842 | 7.5149 |
Ω | Equi&MC | 0.2272 | 0.0685 | 0.0319 | 0.0205 | 0.0457 | 0.0926 | 0.0863 |
GS&AMC | 0.3311 | 0.0253 | 0.0244 | 0.0345 | 0.0266 | 0.0364 | 0.0348 | |
Equi&L | 10.4749 | 5.5909 | 2.9152 | 1.7040 | 1.0414 | 0.6631 | 0.4350 | |
p=2 | GS&L | 9.6261 | 4.6061 | 2.3610 | 1.3371 | 0.8022 | 0.5133 | 0.3308 |
ΩK | Equi&MC | 0.3478 | 0.0661 | 0.0193 | 0.0170 | 0.0115 | 0.0161 | 0.0106 |
GS&AMC | 0.3765 | 0.0245 | 0.0165 | 0.0203 | 0.0146 | 0.0117 | 0.0081 | |
Smax | 3√150 | 3√200 | 3√250 | 3√300 | 3√350 | 3√400 | 3√450 | |
Equi&L | 19.3431 | 22.0844 | 25.8076 | 29.9848 | 34.3954 | 38.9355 | 43.5521 | |
p=3 | GS&L | 28.5178 | 25.4178 | 25.0462 | 25.8940 | 27.3175 | 29.0375 | 30.9201 |
Ω | Equi&MC | 1.2650 | 0.6127 | 0.4785 | 0.3064 | 0.3175 | 0.2029 | 0.1936 |
GS&AMC | 1.9696 | 0.9038 | 0.5715 | 0.3920 | 0.3462 | 0.2689 | 0.2511 | |
Equi&L | 31.1024 | 33.1054 | 28.4051 | 21.9288 | 17.3308 | 14.1742 | 11.8378 | |
p=3 | GS&L | 32.8550 | 28.7798 | 23.0077 | 17.4396 | 13.9633 | 11.2298 | 9.3862 |
ΩK | Equi&MC | 2.0303 | 0.9183 | 0.6883 | 0.4127 | 0.3427 | 0.2148 | 0.1607 |
GS&AMC | 2.2680 | 1.0233 | 0.6413 | 0.4100 | 0.3167 | 0.2264 | 0.1849 |
L | 150 | 200 | 250 | 300 | |
Equi&L | 12.1428 | 16.9562 | 22.3907 | 28.4710 | |
GS&L | 9.1971 | 5.7377 | 5.4052 | 5.5654 | |
Ω | Equi&MC | 2.6188 | 3.0868 | 3.8931 | 4.6910 |
GS&AMC | 2.2287 | 1.5009 | 1.7865 | 2.0569 | |
Equi&L | 8.5065 | 0.7793 | 0.0690 | 0.0084 | |
GS&L | 6.1609 | 0.4476 | 0.0354 | 0.0051 | |
ΩK | Equi&MC | 2.1547 | 0.2287 | 0.0225 | 0.0048 |
GS&AMC | 1.9339 | 0.1858 | 0.0178 | 0.0040 |
L | 150 | 200 | 250 | 300 | |
Equi&L | 11.4121 | 16.5529 | 21.9750 | 28.0137 | |
GS&L | 8.7458 | 5.6337 | 5.1434 | 5.1969 | |
Ω | Equi&MC | 0.9972 | 1.4289 | 1.8595 | 3.7692 |
GS&AMC | 0.8470 | 0.7205 | 0.6078 | 0.5893 | |
Equi&L | 8.2864 | 0.7763 | 0.0666 | 0.0062 | |
GS&L | 5.9848 | 0.4443 | 0.0330 | 0.0030 | |
ΩK | Equi&MC | 1.0381 | 0.1382 | 0.0128 | 0.0029 |
GS&AMC | 0.6490 | 0.0951 | 0.0087 | 0.0016 |
L | 150 | 200 | 250 | 300 | |
Equi&L | 0.8040 | 0.5688 | 0.6639 | 0.7581 | |
GS&L | 0.7340 | 0.6739 | 0.9008 | 0.9729 | |
Ω | Equi&MC | 0.2578 | 0.3740 | 0.5793 | 0.8419 |
GS&AMC | 0.1999 | 0.5024 | 0.8245 | 0.8788 | |
Equi&L | 0.2386 | 0.1022 | 0.1023 | 0.1023 | |
GS&L | 0.1803 | 0.0213 | 0.0181 | 0.0108 | |
ΩK | Equi&MC | 0.2582 | 0.1255 | 0.1047 | 0.1026 |
GS&AMC | 0.2355 | 0.0474 | 0.0171 | 0.0110 |
L | 200 | 250 | 300 | 350 | |
Equi | 1.0136 | 0.2986 | 0.2065 | 0.1753 | |
Ω | GS | 0.5842 | 0.2733 | 0.2258 | 0.1946 |
Equi | 0.8978 | 0.0174 | 0.0043 | 0.0042 | |
ΩK | GS | 0.5099 | 0.0098 | 0.0052 | 0.0049 |
L | 150 | 200 | 250 | 300 | |
Equi&L | 3.6561 | 1.1174 | 0.9870 | 0.9458 | |
GS&L | 3.0292 | 0.6828 | 0.5302 | 0.3400 | |
Ω | Equi&MC | 1.3850 | 1.0302 | 0.9847 | 0.9462 |
GS&AMC | 1.1707 | 0.6454 | 0.5294 | 0.3400 | |
Equi&L | 0.0429 | 0.0218 | 0.0217 | 0.0217 | |
GS&L | 0.0237 | 0.0051 | 0.0042 | 0.0024 | |
ΩK | Equi&MC | 0.0250 | 0.0217 | 0.0217 | 0.0217 |
GS&AMC | 0.0047 | 0.0050 | 0.0042 | 0.0024 |
Smax | 150 | 200 | 250 | 300 | 350 | 400 | 450 | |
Equi&L | 0.3452 | 0.0271 | 0.0024 | 0.0009 | 0.0008 | 0.0008 | 0.0008 | |
p=1 | GS&L | 0.2737 | 0.0165 | 0.0022 | 0.0017 | 0.0016 | 0.0016 | 0.0015 |
Ω | Equi&MC | 0.0457 | 0.0172 | 0.0072 | 0.0511 | 0.0148 | 0.0038 | 0.0419 |
GS&AMC | 0.0457 | 0.0033 | 0.0032 | 0.0023 | 0.0023 | 0.0017 | 0.0024 | |
Equi&L | 0.1505 | 0.0015 | 0.0015 | 0.0016 | 0.0017 | 0.0018 | 0.0019 | |
p=1 | GS&L | 0.1122 | 0.0027 | 0.0024 | 0.0022 | 0.0021 | 0.0021 | 0.0020 |
ΩK | Equi&MC | 0.0563 | 0.0016 | 0.0015 | 0.0016 | 0.0017 | 0.0018 | 0.0019 |
GS&AMC | 0.0472 | 0.0023 | 0.0024 | 0.0022 | 0.0021 | 0.0021 | 0.0020 | |
Smax | √150 | √200 | √250 | √300 | √350 | √400 | √450 | |
Equi&L | 6.8424 | 6.8064 | 7.7089 | 8.9340 | 10.2820 | 11.6811 | 13.1029 | |
p=2 | GS&L | 8.4653 | 6.1009 | 5.8106 | 6.0578 | 6.4852 | 6.9842 | 7.5149 |
Ω | Equi&MC | 0.2272 | 0.0685 | 0.0319 | 0.0205 | 0.0457 | 0.0926 | 0.0863 |
GS&AMC | 0.3311 | 0.0253 | 0.0244 | 0.0345 | 0.0266 | 0.0364 | 0.0348 | |
Equi&L | 10.4749 | 5.5909 | 2.9152 | 1.7040 | 1.0414 | 0.6631 | 0.4350 | |
p=2 | GS&L | 9.6261 | 4.6061 | 2.3610 | 1.3371 | 0.8022 | 0.5133 | 0.3308 |
ΩK | Equi&MC | 0.3478 | 0.0661 | 0.0193 | 0.0170 | 0.0115 | 0.0161 | 0.0106 |
GS&AMC | 0.3765 | 0.0245 | 0.0165 | 0.0203 | 0.0146 | 0.0117 | 0.0081 | |
Smax | 3√150 | 3√200 | 3√250 | 3√300 | 3√350 | 3√400 | 3√450 | |
Equi&L | 19.3431 | 22.0844 | 25.8076 | 29.9848 | 34.3954 | 38.9355 | 43.5521 | |
p=3 | GS&L | 28.5178 | 25.4178 | 25.0462 | 25.8940 | 27.3175 | 29.0375 | 30.9201 |
Ω | Equi&MC | 1.2650 | 0.6127 | 0.4785 | 0.3064 | 0.3175 | 0.2029 | 0.1936 |
GS&AMC | 1.9696 | 0.9038 | 0.5715 | 0.3920 | 0.3462 | 0.2689 | 0.2511 | |
Equi&L | 31.1024 | 33.1054 | 28.4051 | 21.9288 | 17.3308 | 14.1742 | 11.8378 | |
p=3 | GS&L | 32.8550 | 28.7798 | 23.0077 | 17.4396 | 13.9633 | 11.2298 | 9.3862 |
ΩK | Equi&MC | 2.0303 | 0.9183 | 0.6883 | 0.4127 | 0.3427 | 0.2148 | 0.1607 |
GS&AMC | 2.2680 | 1.0233 | 0.6413 | 0.4100 | 0.3167 | 0.2264 | 0.1849 |
L | 150 | 200 | 250 | 300 | |
Equi&L | 12.1428 | 16.9562 | 22.3907 | 28.4710 | |
GS&L | 9.1971 | 5.7377 | 5.4052 | 5.5654 | |
Ω | Equi&MC | 2.6188 | 3.0868 | 3.8931 | 4.6910 |
GS&AMC | 2.2287 | 1.5009 | 1.7865 | 2.0569 | |
Equi&L | 8.5065 | 0.7793 | 0.0690 | 0.0084 | |
GS&L | 6.1609 | 0.4476 | 0.0354 | 0.0051 | |
ΩK | Equi&MC | 2.1547 | 0.2287 | 0.0225 | 0.0048 |
GS&AMC | 1.9339 | 0.1858 | 0.0178 | 0.0040 |
L | 150 | 200 | 250 | 300 | |
Equi&L | 11.4121 | 16.5529 | 21.9750 | 28.0137 | |
GS&L | 8.7458 | 5.6337 | 5.1434 | 5.1969 | |
Ω | Equi&MC | 0.9972 | 1.4289 | 1.8595 | 3.7692 |
GS&AMC | 0.8470 | 0.7205 | 0.6078 | 0.5893 | |
Equi&L | 8.2864 | 0.7763 | 0.0666 | 0.0062 | |
GS&L | 5.9848 | 0.4443 | 0.0330 | 0.0030 | |
ΩK | Equi&MC | 1.0381 | 0.1382 | 0.0128 | 0.0029 |
GS&AMC | 0.6490 | 0.0951 | 0.0087 | 0.0016 |
L | 150 | 200 | 250 | 300 | |
Equi&L | 0.8040 | 0.5688 | 0.6639 | 0.7581 | |
GS&L | 0.7340 | 0.6739 | 0.9008 | 0.9729 | |
Ω | Equi&MC | 0.2578 | 0.3740 | 0.5793 | 0.8419 |
GS&AMC | 0.1999 | 0.5024 | 0.8245 | 0.8788 | |
Equi&L | 0.2386 | 0.1022 | 0.1023 | 0.1023 | |
GS&L | 0.1803 | 0.0213 | 0.0181 | 0.0108 | |
ΩK | Equi&MC | 0.2582 | 0.1255 | 0.1047 | 0.1026 |
GS&AMC | 0.2355 | 0.0474 | 0.0171 | 0.0110 |
L | 200 | 250 | 300 | 350 | |
Equi | 1.0136 | 0.2986 | 0.2065 | 0.1753 | |
Ω | GS | 0.5842 | 0.2733 | 0.2258 | 0.1946 |
Equi | 0.8978 | 0.0174 | 0.0043 | 0.0042 | |
ΩK | GS | 0.5099 | 0.0098 | 0.0052 | 0.0049 |
L | 150 | 200 | 250 | 300 | |
Equi&L | 3.6561 | 1.1174 | 0.9870 | 0.9458 | |
GS&L | 3.0292 | 0.6828 | 0.5302 | 0.3400 | |
Ω | Equi&MC | 1.3850 | 1.0302 | 0.9847 | 0.9462 |
GS&AMC | 1.1707 | 0.6454 | 0.5294 | 0.3400 | |
Equi&L | 0.0429 | 0.0218 | 0.0217 | 0.0217 | |
GS&L | 0.0237 | 0.0051 | 0.0042 | 0.0024 | |
ΩK | Equi&MC | 0.0250 | 0.0217 | 0.0217 | 0.0217 |
GS&AMC | 0.0047 | 0.0050 | 0.0042 | 0.0024 |