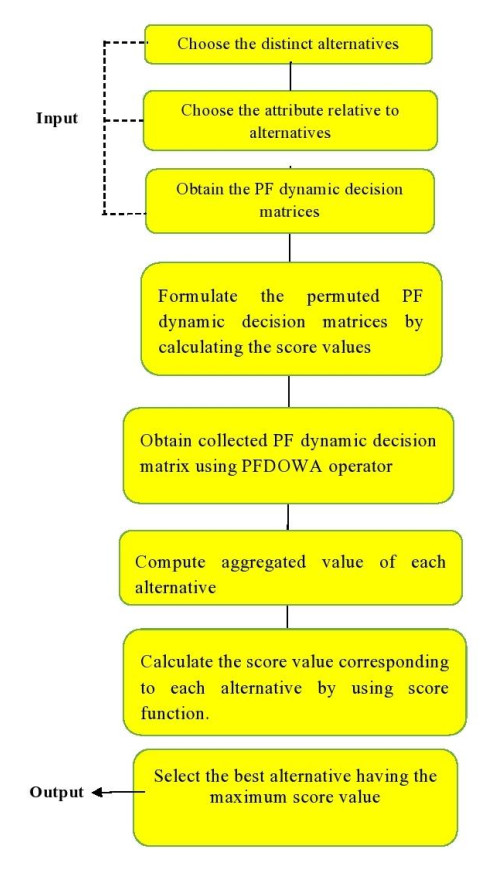
The prevalence of cardiovascular disease (CVD) is a major issue in world health. There is a compelling desire for precise and effective methods for making decisions to determine the most effective technique for treating CVD. Here, we focused on the urgent matter at hand. Pythagorean fuzzy dynamic settings are exceptionally proficient at capturing ambiguity because they can handle complex problem specifications that involve both Pythagorean uncertainty and periodicity. In this article, we introduced a pair of novel aggregation operators: The Pythagorean fuzzy dynamic ordered weighted averaging (PFDOWA) operator and the Pythagorean fuzzy dynamic ordered weighted geometric (PFDOWG) operator, and we proved various structural properties of these concepts. Using these operators, we devised a systematic methodology to handle multiple attribute decision-making (MADM) scenarios incorporating Pythagorean fuzzy data. Moreover, we endeavored to address a MADM problem, where we discerned the most efficacious strategy for the management of CVD through the application of the proposed operators. Finally, we undertook an exhaustive comparative analysis to evaluate the ability of the suggested methods in connection with several developed procedures, therefore demonstrating the reliability of the generated methodologies.
Citation: Mehwish Shehzadi, Hanan Alolaiyan, Umer Shuaib, Abdul Razaq, Qin Xin. Crafting optimal cardiovascular treatment strategy in Pythagorean fuzzy dynamic settings[J]. AIMS Mathematics, 2024, 9(11): 31495-31531. doi: 10.3934/math.20241516
[1] | Esmail Hassan Abdullatif Al-Sabri, Muhammad Rahim, Fazli Amin, Rashad Ismail, Salma Khan, Agaeb Mahal Alanzi, Hamiden Abd El-Wahed Khalifa . Multi-criteria decision-making based on Pythagorean cubic fuzzy Einstein aggregation operators for investment management. AIMS Mathematics, 2023, 8(7): 16961-16988. doi: 10.3934/math.2023866 |
[2] | Hifza, Muhammad Gulistan, Zahid Khan, Mohammed M. Al-Shamiri, Muhammad Azhar, Asad Ali, Joseph David Madasi . A new fuzzy decision support system approach; analysis and applications. AIMS Mathematics, 2022, 7(8): 14785-14825. doi: 10.3934/math.2022812 |
[3] | Misbah Rasheed, ElSayed Tag-Eldin, Nivin A. Ghamry, Muntazim Abbas Hashmi, Muhammad Kamran, Umber Rana . Decision-making algorithm based on Pythagorean fuzzy environment with probabilistic hesitant fuzzy set and Choquet integral. AIMS Mathematics, 2023, 8(5): 12422-12455. doi: 10.3934/math.2023624 |
[4] | Akarachai Satirad, Ronnason Chinram, Aiyared Iampan . Pythagorean fuzzy sets in UP-algebras and approximations. AIMS Mathematics, 2021, 6(6): 6002-6032. doi: 10.3934/math.2021354 |
[5] | Abdul Razaq, Ghaliah Alhamzi . On Pythagorean fuzzy ideals of a classical ring. AIMS Mathematics, 2023, 8(2): 4280-4303. doi: 10.3934/math.2023213 |
[6] | Nadia Khan, Sehrish Ayaz, Imran Siddique, Hijaz Ahmad, Sameh Askar, Rana Muhammad Zulqarnain . Sustainable practices to reduce environmental impact of industry using interaction aggregation operators under interval-valued Pythagorean fuzzy hypersoft set. AIMS Mathematics, 2023, 8(6): 14644-14683. doi: 10.3934/math.2023750 |
[7] | Li Li, Mengjing Hao . Interval-valued Pythagorean fuzzy entropy and its application to multi-criterion group decision-making. AIMS Mathematics, 2024, 9(5): 12511-12528. doi: 10.3934/math.2024612 |
[8] | Saleem Abdullah, Muhammad Qiyas, Muhammad Naeem, Mamona, Yi Liu . Pythagorean Cubic fuzzy Hamacher aggregation operators and their application in green supply selection problem. AIMS Mathematics, 2022, 7(3): 4735-4766. doi: 10.3934/math.2022263 |
[9] | Supriya Bhunia, Ganesh Ghorai, Qin Xin . On the fuzzification of Lagrange's theorem in $ (\alpha, \beta) $-Pythagorean fuzzy environment. AIMS Mathematics, 2021, 6(9): 9290-9308. doi: 10.3934/math.2021540 |
[10] | Wei Lu, Yuangang Li, Yixiu Kong, Liangli Yang . Generalized triangular Pythagorean fuzzy weighted Bonferroni operators and their application in multi-attribute decision-making. AIMS Mathematics, 2023, 8(12): 28376-28397. doi: 10.3934/math.20231452 |
The prevalence of cardiovascular disease (CVD) is a major issue in world health. There is a compelling desire for precise and effective methods for making decisions to determine the most effective technique for treating CVD. Here, we focused on the urgent matter at hand. Pythagorean fuzzy dynamic settings are exceptionally proficient at capturing ambiguity because they can handle complex problem specifications that involve both Pythagorean uncertainty and periodicity. In this article, we introduced a pair of novel aggregation operators: The Pythagorean fuzzy dynamic ordered weighted averaging (PFDOWA) operator and the Pythagorean fuzzy dynamic ordered weighted geometric (PFDOWG) operator, and we proved various structural properties of these concepts. Using these operators, we devised a systematic methodology to handle multiple attribute decision-making (MADM) scenarios incorporating Pythagorean fuzzy data. Moreover, we endeavored to address a MADM problem, where we discerned the most efficacious strategy for the management of CVD through the application of the proposed operators. Finally, we undertook an exhaustive comparative analysis to evaluate the ability of the suggested methods in connection with several developed procedures, therefore demonstrating the reliability of the generated methodologies.
The abbreviations used in this study are summarized as below:
Description | Abbreviation |
Cardiovascular disease | CVD |
Fuzzy set | FS |
Intuitionistic fuzzy set | IFS |
Pythagorean fuzzy set | PFS |
Pythagorean fuzzy sets | PFSs |
Pythagorean fuzzy dynamic ordered weighted averaging operator | PFDOWA |
Pythagorean fuzzy dynamic ordered weighted geometric operator | PFDOWG |
Multi-attribute decision-making | MADM |
Pythagorean fuzzy ordered weighted averaging operator | PFOWA |
Pythagorean fuzzy ordered weighted geometric operator | PFOWG |
Pythagorean fuzzy weighted averaging operator | PFWA |
Pythagorean fuzzy weighted geometric operator | PFWG |
Aggregation operators | AOs |
MADM constitutes a pivotal component of the decision-making process (DM). Its objective is to choose the most rational option from a spectrum of choices, taking into account a multitude of conflicting criteria. The MADM method is recognized as a notable approach due to its immediate application, disregarding the reluctance of individuals with decision-making difficulties and the complexity of decision-making scenarios. The significance of this technique is increasing because of its ability to effectively address real-world issues in several fields. In recent years, healthcare issues have become a central focus for researchers employing decision-making approaches [1,2,3]. The growing importance of MADM is due to its capacity to effectively address real-world problems across a variety of domains through the use of AOs. These operators are explicitly designed to scrutinize each value within the original set and combine all values into a singular entity within the same set. Prior to the advent of AOs, selections were solely based on entire collections. Nevertheless, the concept of belonging to a set is often imprecise, particularly in disciplines such as biology, social sciences, language and linguistics, psychology, economics, and other social sciences. In these fields, traditional mathematical techniques appear somewhat insufficient. To address this challenge, Zadeh [4] proposed the notion of partial membership in a set, which he termed a fuzzy set. Kahne [5] developed a significant decision-making approach that can be used to assess potential solutions by considering numerous factors with different levels of importance. An approach for identifying the optimal choice using fuzzy logic was presented [6]. Fuzzy sets' fundamental operations were investigated in [7]. The AOs for fuzzy sets were first introduced by Yager in [8]. Atanassov [9] introduced the notion of IFS to further develop the concept of fuzzy sets. Szmidt and Kaeprzyk [10] developed a method for solving group decision problems using the intuitionistic fuzzy (IF) environment. Li [11] did a study on fundamental linear programming techniques and methodologies for MADM in an uncertain environment. Xu and Yager [12] developed many geometric AOs for IFS. In 2007, several basic arithmetic aggregation algorithms were created [13] using an IFS framework. [14] suggested the utilization of generalized AOs on IFS as a possible solution to the MADM problem. The researchers in [15] aimed to tackle challenges related to group decision-making in IFS environments by utilizing AOs. Huang [16] proposed a DM method for IFS using Hamacher AOs. Verma [17] was the first to use the Bonferroni mean operators for IFS. In order to tackle MADM issues, the Aczel-Alsina AOs of IFS were introduced by [18].
The condition μ+η≤1 restricts the possibility of having membership and non-membership grades in IFS. To prevent this scenario, Yager [19,20] proposed (PFSs) as a successor to IFSs. PFSs are designed to handle imprecision and complicated uncertainty by imposing the constraint μ2+η2≤1, where μ represents membership grade and η represents non-membership grade. Moreover, the Pythagorean fuzzy number (PFN) was proposed by Zhang and Xu [21] to describe an object's dual characteristics. PFSs generally permit and incorporate a higher amount of ambiguity than IFSs. Yager [22] demonstrated the development of several AOs, such as the weighted power geometric and PFOWA operators. A similarity measure and two AOs form the basis of an innovative method suggested by Zhang [23] in a Pythagorean fuzzy (PF) setting. Peng and Yaun [24] explored the basic characteristics of Pythagorean fuzzy AOs. Wei [25] introduced a collection of generalized Pythagorean fuzzy AOs and highlighted their applications in decision-making. Zeng et al. [26] later integrated AOs and distance measurements to develop the PF weighted and ordered weighted distance operators. For more developments about PFSs, we refer to [27,28,29]. A set of generalized Pythagorean fuzzy geometric AOs was proposed by Garg [30], which incorporated Einstein's operations. Furthermore, Garg [31,32] examined the utilization of the PF environment in diverse decision-making scenarios. Liang et al. [33] devised a PF Bonferroni mean AO and presented a proficient method for its computation to address decision-making problems. To go deeper into this specific area of expertise, one might examine [34,35,36,37].
All the aforementioned decision-making challenges involve the consideration of decision information with simultaneous inputs. However, certain domains of decision-making, such as multi-period dynamic investment, medical diagnosis, dynamic personal selection, and dynamic military system efficiency evaluation, entail the collection of data at various time intervals. Xu and Yager [38] first discovered this particular kind of information in their study on dynamic MADM problems. They presented an application of MADM problems utilizing the uncertain IF dynamic weighted averaging (UIFDWA) operator and the IF dynamic weighted averaging (IFDWA) operator to obtain dynamic or uncertain dynamic IF information. In addition, the IFDWA and UIFDWA operators were employed to address two dynamic MADM challenges when the attributes' arguments are utilized in IFNs or IVIFNs. Dynamic MADM problems involving the utilization of IVIFNs or IFN attribute values were examined by Wei [39]. He introduced weighted geometric AOs comprised of various periods to gather dynamic IFS data, including the IF dynamic weighted geometric (IFDWG) operator and the uncertain IF dynamic weighted geometric (UIFDWG) operator. The scholarly discourse on PFSs has rapidly gained popularity among numerous researchers, as evidenced by the considerable focus they have garnered across diverse studies [40,41,42,43,44].
PFSs sustain higher levels of uncertainty than IFSs, underscoring their suitability for addressing scenarios marked by heightened complexity and ambiguity in MADM challenges. Ordered weighted AOs do not depend on preassigned weights designated to certain attributes. These operators allow decision-makers to include the uncertainty and imprecision associated with real-world scenarios by rearranging the input values to accurately reflect their importance in the decision-making process. Moreover, ordered weighted AOs with varying time intervals offer a strong tool for temporal aggregation and decision-making. Researchers have focused mainly on issues where all of the initially selected data was collected at the same time for decision-making. Nonetheless, it is common in many decision-making environments to collect relevant data at several time points for the underlying selection. In the context of the MADM, the term "time interval" refers to how the decision-maker prefers to receive information throughout various time periods. It is accomplished by employing a time varying function. By combining components into the dynamic paradigm, it becomes feasible to analyze variations in membership degrees as time passes and examine changes within particular time frames. This aspect improves decision-making accuracy, provides insight into modifications, and evaluates the dynamics of fuzzy sets. To address these problems, a variety of techniques are needed. The PFDOWA and PFDOWG operators are essential components of decision-making models because they allow for dynamic modifications and accurately depict complex interactions among systems affected by imprecision and uncertainty. These operators aid in prioritizing pertinent, current data and provide insights into both short- and long-term trends by varying weights over time. In addition, the ordered weighted AOs have already been proposed for PF settings. However, these operators are not capable of handling uncertainty at different time periods. As a result, it is critical to provide a detailed explanation of these operators for PF sets in order to effectively address such scenarios and fill the gap. This motivates us to study dynamic ordered weighted AOs in the framework of PFSs. The dynamic nature of the operator, together with the PFS, is an essential part of our strategy. The key motivations for this study are listed below:
a) Dynamic operators provide exceptional flexibility when dealing with ambiguous data.
b) These operators exhibit exceptional proficiency in switching diverse data into a single value, consequently effectively addressing the complicated aspects of decision-making in an ever-changing environment.
c) In dynamic contexts characterized by ever-changing conditions, PFSs present an advanced representation that can accommodate emerging preferences or circumstances.
The major contributions of this study are explained as follows:
ⅰ. We introduce two innovative AOs, the PFDOWA operator and the PFDOWG operator. We design these operators to apply to procedures that use PF data in decision-making.
ⅱ. We thoroughly examine the essential characteristics of PFDOWA and PFDOWG operators, such as idempotency, boundedness, and monotonicity.
ⅲ. By utilizing the PFDOWA and PFDOWG operators, an organized approach for addressing MADM problems is proposed. This demonstrates the practical significance of these operators.
ⅳ. The suggested method is used to tackle a specific MADM issue that is focused on treating CVD. It underscores the significance of PFDOWA and PFDOWG operators in the context of decision-making processes.
ⅴ. To determine the efficacy of the proposed methods in comparison to a variety of established techniques, we perform a comprehensive comparative analysis. These comparison results demonstrate the consistency and validity of the developed methodology.
To accomplish the work presented in this article, the remaining portion of this study is organized as follows: In Section 2, we provide a few basic definitions that are crucial for understanding the major findings presented in this research work. Section 3 contains an introduction to the study of PFDOWA and PFDOWG operators and establishes the key structural aspects of these phenomena. Section 4 presents a step-by-step methodology for tackling the MADM problem through the utilization of PF dynamic information, employing the suggested operators. In Section 5, we illustrate the validity of defined approaches in the framework of solving the MADM problem of selecting an efficient treatment method for CVD. Additionally, we include a comparative study to demonstrate the effectiveness and practicality of these novel approaches in contrast with established approaches. In Section 6, we describe the conclusions drawn from the complete research.
In this section, we give key definitions that are required for a complete understanding of the subject matter covered in this article.
Definition 1. ([9]) Assuming that Z is a universe of discourse, an IFS J is described as follows:
J={z,μJ(z),ηJ(z)|z∈Z}, |
where μJ:Z→[0,1] and ηJ:Z→[0,1] represent the membership function and non-membership function, respectively, that admit the criteria 0≤μJ(z)+ηJ(z)≤1. The hesitation margin of the IFS J can be characterized as follows: πJ(z)=1−μJ(z)−ηJ(z).
Definition 2. ([22]). Let Z be a universal set. A PFS P can be characterized in the following manner:
P={z,μP(z),ηP(z)|z∈Z}, |
where, μP:Z→[0,1] and ηP:Z→[0,1], are called membership and non-membership functions respectively, under a few restrictions: 0≤μP(z)≤1, 0≤ηP(z)≤1 and 0≤μ2P(z)+η2P(z)≤1∀z∈Z. Additionally, the degree of indeterminacy of (PFS) P is specified asπP(z)=√1−μ2P(z)−η2P(z), such that0≤πP(z)≤1∀z∈Z.
Now, we specify the membership and non-membership degrees of z∈Z as z=(μ,η). The aforementioned description of z is referred to as a PF number.
Definition 3. ([22]) Assume that ϕ is a collection of PFNs represented as ἆi=(μi,ηi) where, i=1,2,…,n and ω=(ω1,ω2,…,ωn)T is the weight vector linked to ἆi with condition ωi∈[0,1] and ∑ni=1ωi=1. The Pythagorean fuzzy weighted averaging AO is a mapping PFWA:ϕn→ϕ, defined by the rule:
PFWA(ἆ1,ἆ2,…,ἆn)=⊕ni=1ωiἆi=(√1−n∏i=1(1−μ2i)ωi,n∏i=1ηωii). |
Definition 4. ([27]) Assume that ϕ is a collection of PFNs represented as ἆi=(μi,ηi) where, i=1,2,…,n and ω=(ω1,ω2,…,ωn)T is the weight vector of ἆi with that that conditions ωi∈[0,1] and ∑ni=1ωi=1. The Pythagorean fuzzy weighted geometric AO is a function PFWG:ϕn→ϕ, defined by the rule:
PFWG(ἆ1,ἆ2,…,ἆn)=⊕ni=1(ἆi)ωi=(n∏i=1μωii,√1−n∏i=1(1−η2i)ωi). |
Definition 5. ([20]) Assume that ϕ is a collection of PFNs represented as ἆi=(μi,ηi) where, i=1,2,…,n and ω=(ω1,ω2,…,ωn)T is the weight vector of ἆi with the conditions that ωi∈[0,1] and ∑ni=1ωi=1. The Pythagorean fuzzy ordered weighted averaging AO is a mapping PFOWA:ϕn→ϕ, defined by the rule:
PFOWA(ἆ1,ἆ2,…,ἆn)=⊕ni=1ωiἆϱ(i)=(√1−n∏i=1(1−μ2ϱ(i))ωi,n∏i=1ηωiϱ(i)). |
Notice, ϱ(1),ϱ(2),…,ϱ(n) is the permutation of {1,2,…,n} in a certain manner, satisfying condition ἆϱ(i−1)≥ἆϱ(i).
Definition 6. ([45]) Assume that ϕ is a collection of PFNs represented as ἆi=(μi,ηi) where, i=1,2,…,n and ω=(ω1,ω2,…,ωn)T is the weight vector linked to ἆi with the conditions ωi∈[0,1] and ∑ni=1ωi=1. The PFOWG operator is a function PFOWG:ϕn→ϕ, defined by the rule:
PFOWG(ἆ1,ἆ2,…,ἆn)=⊕ni=1(ἆϱ(i))ωi=(n∏i=1μωiϱ(i),√1−n∏i=1(1−η2ϱ(i))ωi). |
Definition 7. ([21]) For any PFN ἆ=(μ,η) two vital functions are outlined as follows:
ⅰ. The score function S(ἆ) is computed in the following manner: S(ἆ)=μ2−η2, where, S(ἆ)∈[−1,1].
ⅱ. The definition of the accuracy function H(ἆ) is described as follows: H(ἆ)=μ2+η2, where, H(ἆ)∈[0,1].
Definition 8. ([38]) Let t be a time variable, then Jt=(μt,ηt) is an IF variable where, μt∈[0,1],ηt∈[0,1] with restriction μt+ηt≤1.
In the context of IF variable Jt=(μt,ηt) if a time sequence is known t=(t1,t2,…,tp), then notation Jt1,Jt2,…,Jtp represents a collection of p IF numbers considered at p different time periods.
In this section, we examine the dynamic operations that occur inside the PF framework. Furthermore, we present the concepts of dynamic ordered weighted AOs for PFNs, namely PFDOWA and PFDOWG, and analyze their key features.
Definition 9. Let t symbolize a variable that represents time. Within this framework, we establish ἆt=(μt,ηt)as a PF variable where, μt∈[0,1],ηt∈[0,1] with constraint μ2t+η2t≤1.
For a PF variable ἆt=(μt,ηt) if we have a time sequence t=(t1,t2,…,tp) then ἆt1,ἆt2,…,ἆtp represents p PFNs, associated with distinct time periods.
The following definition introduces us to dynamic operational laws applied to PFNs.
Definition 10. Assume two PFNs ἆt1=(μt1,ηt1) and ἆt2=(μt2,ηt2). The fundamental ordering principles governing the interrelationship of operational laws in PFNs can be characterized as follows:
1- ἆt1≤ἆt2, if μt1≤μt2 and ηt1≤ηt2
2- ἆt1=ἆt2, if and only if ἆt1≤ἆt2 and ἆt2≤ἆt1
Definition 11. Consider ἆt=(μt,ηt), ἆt1=(μt1,ηt1) and ἆt2=(μt2,ηt2) represent three PFNs and Υ>0. The dynamic operations for these PFNs are described as follows:
ⅰ. ἆt1⊕ἆt2=(√μ2t1+μ2t2−μ2t1μ2t2,ηt1ηt2)
ⅱ. ἆt1⊕ἆt2=(μt1μt2,√η2t1+η2t2−η2t1η2t2)
ⅲ. λἆt=(√1−(1−μ2t)Υ,ηΥt)
ⅳ. ἆtΥ=(μΥt,√1−(1−η2t)Υ)
Definition 12. Assume that ϕ is a collection of PFNs represented as ἆtř=(μtř,ηtř) where, ř=1,2.3,…,p and Υt=[Υt1,Υt2,…,Υtp]Tis the weight vector linked to the time periods tř such that ∑př=1Υtř=1 and Υtř∈[0,1]. In this context, the PFDOWA is a mapping PFDOWA:ϕp→ϕ defined by the rule:
PFDOWA(ἆt1,ἆt2,…,ἆtp)=⊕př=1Υtřἆϱ(tř)=(√1−∏př=1(1−μ2ϱ(tř))Υtř,∏př=1ηΥtřϱ(tř)) |
In the upcoming theorem, we will demonstrate that the aggregated value of PFNs at different time periods, using the PFDOWA operator, results in a new PFN.
Theorem 1. Consider p numbers of PFNs expressed as ἆtř=(μtř,ηtř) at p different time periods tř, where ř=1,2,3,…,p, and the associated weight vector is Υt=[Υt1,Υt2,….,Υtp]T with some restrictions ∑př=1Υtř=1 and Υtř∈[0,1]. The aggregated value of these PFNs using the PFDOWA operator is also a PFN. It can be described as follows:
PFDOWA(ἆt1,ἆt2,…,ἆtp)=(√1−∏př=1(1−μ2ϱ(tř))Υ(tř),∏př=1ηΥ(tř)ϱ(tř)) |
Proof. The technique of mathematical induction is used to establish the proof of this theorem. The proof begins by examining the basic case when p=2
PFDOWA(ἆt1,ἆt2)=Υt1ἆϱ(t1)⊕Υt2ἆϱ(t2) |
By separating the components Υt1ἆϱ(t1) and Υt2ἆϱ(t2) utilizing definition 12, we derive the subsequent equations:
Υt1ἆϱ(t1)=[√1−(1−μ2ϱ(t1))Υt1,ηΥt1ϱ(t1)]Υt2ἆϱ(t2)=[√1−(1−μ2ϱ(t2))Υt2,ηΥt2ϱ(t2)] |
Thus,
Υt1.ἆϱ(t1)⊕Υt2.ἆϱ(t2)=[√1−(1−μ2ϱ(t1))Υt1,ηΥt1ϱ(t1)]⊕[√1−(1−μ2ϱ(t2))Υt2,ηΥt2ϱ(t2)]=[√1−(1−μ2ϱ(t1))Υt1(1−μ2ϱ(t2))Υt2,ηΥt1ϱ(t1)ηΥt2ϱ(t2)] |
Consequently,
PFDOWA(ἆt1,ἆt2)=[√1−2∏ř=1(1−μ2ϱ(tř))Υtř,2∏ř=1ηΥtřϱ(tř)] |
Hence, the result is true for p=2.
Now, we assume that the given result is true for p=n>2, then we have,
PFDOWA(ἆt1,ἆt2,…,ἆtn)=[√1−n∏ř=1(1−μ2ϱ(tř))Υtř,n∏ř=1ηΥtřϱ(tř)] |
Now we prove the result for p=n+1
PFDOWA(ἆt1,ἆt2,…,ἆtn,ἆtn+1)=Υt1ἆϱ(t1)⊕Υt2ἆϱ(t2)⊕...⊕Υtnἆϱ(tn)⊕Υtn+1ἆϱ(tn+1)=[√1−n∏ř=1(1−μ2ϱ(tř))Υtř,n∏ř=1ηΥtřϱ(tř)]⊕[√1−(1−μ2ϱ(tn+1))Υtn+1,(ηϱ(tn+1))Υtn+1] |
It follows that
PFDOWA(ἆt1,ἆt2,…,ἆtn,ἆtn+1)=[√1−n+1∏ř=1(1−μ2ϱ(tř))Υtř,n+1∏ř=1ηΥtřϱ(tř)] |
Hence, we can deduce that the result is applicable to all positive integer values of p.
Example 1. Consider ἆt1=(0.30,0.80), ἆt2=(0.40,0.70), ἆt3=(0.20,0.80) and ἆt4=(0.70,0.60) represents four PFNs and Υt=(0.2,0.1,0.3,0.4)T is the weight vector linked to time periods tř where ř=1,2,3,4. First we compute the scores values of ἆtř by applying definition 7 as follows:
S(ἆt1)=−0.55,S(ἆt2)=−0.33,S(ἆt3)=−0.6,S(ἆt4)=0.13 |
then the permuted values of PFNs are organized as follows:
ἆϱ(t1)=(0.70,0.60),ἆϱ(t2)=(0.40,0.70),ἆϱ(t3)=(0.30,0.80),ἆϱ(t4)=(0.20,0.80) |
In view of definition 12, the aggregated values of the above PFNs is calculated as follows:
PFDOWA(ἆt1,ἆt2,ἆt3,ἆt4)=(√1−4∏ř=1(1−μ2ϱ(tř))Υtř,4∏ř=1ηΥtřϱ(tř)) |
PFDOWA(ἆt1,ἆt2,ἆt3,ἆt4)=(√1−(1−0.72)0.2(1−0.42)0.1(1−0.32)0.3(1−0.22)0.4,(0.6)0.2(0.7)0.1(0.8)0.3(0.8)0.4) |
It follows that
PFDOWA(ἆt1,ἆt2,ἆt3,ἆt4)=(0.423,0.745) |
In the upcoming theorem, we establish the idempotency property of the PFDOWA operator, showing that if all PFNs are equal for a given time period, the aggregated result will also equal that PFN.
Theorem 2. Consider the p number of PFNs represented as ἆ(tř)=(μ(tř),η(tř)), existing at p different time periods tř, and let Υt=(Υt1,Υt2,…,Υtp)Tbe the weight vector linked to time periods tř with the condition ∑př=1Υtř=1 and Υtř∈[0,1], where ř=1,2,…,p. If ἆϱ(tř)=ἆϱ(tj) are mathematically equal for all ř and for some j∈{1,2,…,p} where, ἆϱ(tj)=(μϱ(tj),ηϱ(tj)). Then
PFDOWA(ἆt1,ἆt2,…,ἆtp)=ἆϱ(tj) |
Proof. Given that ἆϱ(tř)=ἆϱ(tj) for all ř=1,2,…,p and for some j∈{1,2,…,p}, which implies that μϱ(tř)=μϱ(tj) and ηϱ(tř)=ηϱ(tj). Thus, we have
PFDOWA(ἆt1,ἆt2,…,ἆtp)=(√1−(1−μ2ϱ(tj))∑př=1Υtř,η∑př=1Υtřϱ(tj))=(√1−(1−μ2ϱ(tj)),ηϱ(tj))=(√μ2ϱ(tj),ηϱ(tj))=(μϱ(tj),ηϱ(tj)) |
Consequently,
PFDOWA(ἆt1,ἆt2,…,ἆtp)=ἆϱ(tj) |
In the upcoming theorem, we establish the boundedness property of the PFDOWA operator, demonstrating that the aggregated value of PFNs lies between the defined lower and upper bounds.
Theorem 3. Consider ἆ−=(mintř(μϱ(tř)),maxtř(ηϱ(tř))) and ἆ+=(maxtř(μϱ(tř)),mintř(ηϱ(tř))) represent the lower bound and upper bound of PFNs ἆtř=(μtř,ηtř) where, ř=1,2,…,p. Let Υt=(Υt1,Υt2,…,Υtp)Tbe the corresponding weight vector of time periods tř such that Υtř∈[0,1] satisfying the condition ∑př=1Υtř=1. Then
ἆ−≤PFDOWA(ἆt1,ἆt2,…,ἆtp)≤ἆ+. |
Proof. Consider the outcome when employing the PFDOWA operator on a PFNs collection, indicated as PFDOWA(ἆt1,ἆt2,…,ἆtp)=(μt,ηt),
In view of the given conditions, we have
mintř{μϱ(tř)}≤μϱ(tř)≤maxtř{μϱ(tř)}⟹mintř{μ2ϱ(tř)}≤μ2ϱ(tř)≤maxtř{μ2ϱ(tř)}⟹1−maxtř{μ2ϱ(tř)}≤1−μ2ϱ(tř)≤1−mintř{μ2ϱ(tř)}⟹p∏ř=1(1−maxtř{μ2ϱ(tř)})Υtř≤p∏ř=1(1−μ2ϱ(tř))Υtř≤p∏ř=1(1−mintř{μ2ϱ(tř)})Υtř⟹(1−maxtř{μ2ϱ(tř)})∑př=1Υtř≤p∏ř=1(1−μ2ϱ(tř))Υtř≤(1−mintř{μ2ϱ(tř)})∑př=1Υtř⟹(1−maxtř{μ2ϱ(tř)})≤p∏ř=1(1−μ2ϱ(tř))Υtř≤(1−mintk{μ2ϱ(tk)})⟹mintř{μ2ϱ(tř)}≤1−p∏ř=1(1−μ2ϱ(tř))Υtř≤maxtř{μ2ϱ(tř)}⟹√mintř{μ2ϱ(tř)}≤√1−p∏ř=1(1−μ2ϱ(tř))Υtř≤√maxtř{μ2ϱ(tř)} |
Consequently,
mintř{μϱ(tř)}≤μt≤maxtř{μϱ(tř)} | (3.1) |
Moreover, in view of given conditions we have
maxtř{ηϱ(tř)}≤ηϱ(tř)≤mintř{ηϱ(tř)}⟹p∏ř=1(maxtř{ηϱ(tř)})Υtř≤p∏ř=1(ηϱ(tř))Υtř≤p∏ř=1(mintř{ηϱ(tř)})Υtř⟹(maxtř{ηϱ(tř)})∑př=1Υtř≤p∏ř=1(ηϱ(tř))Υtř≤(mintř{ηϱ(tř)})∑nř=1Υtř⟹maxtř{ηϱ(tř)}≤ηt≤mintř{ηϱ(tř)}. | (3.2) |
In view of definition 12 and by comparing (3.1) and (3.2) we obtain the following expression:
ἆ−≤PFDOWA(ἆt1,ἆt2,…,ἆtp)≤ἆ+. |
In the upcoming theorem, we will establish the monotonicity property of the PFDOWA operator, showing that if one set of PFNs dominates another, their aggregated values will preserve this ordering.
Theorem 4. Let ἆtř=(μtř,ηtř) and ἆ'tř=(μ'tř,η'tř) where, ř=1,2,…,p, be any two collection of PFNs. Consider Υt=(Υt1,Υt2,…,Υtp)Tsignifies the weight vector linked to time periods tř, such that Υtř∈[0,1] and ∑př=1Υř=1. If μϱ(tř)≤μ'ϱ(tř) and ηϱ(tř)≥η'ϱ(tř) then we can establish that:
PFDOWA(ἆt1,ἆt2,…,ἆtp)≤PFDOWA(ἆ't1,ἆ't2,…,ἆ'tp) |
Proof. The application of PFDOWA operator yields:
PFDOWA(ἆt1,ἆt2,…,ἆtp)=(μt,ηt)andPFDOWA(ἆ't1,ἆ't2,…,ἆ'tp)=(μ't,η't) |
Since μϱ(tř)≤μ'ϱ(tř), which shows that μ2ϱ(tř)≤μ2'ϱ(tř), this implies that
1−μ2ϱ(tř)≥1−μ2'ϱ(tř)⇒p∏ř=1(1−μ2ϱ(tř))Υtř≥p∏ř=1(1−μ2'ϱ(tř))Υtř⇒1−p∏ř=1(1−μ2ϱ(tř))Υtř≤1−p∏ř=1(1−μ2'ϱ(tř))Υtř⇒√1−p∏ř=1(1−μ2ϱ(tř))Υtř≤√1−p∏ř=1(1−μ2'ϱ(tř))Υtř |
It follows that
μt≤μ't | (3.3) |
Moreover, by taking into account ηϱ(tř)≥η'ϱ(tř), we have
p∏ř=1ηΥtřϱ(tř)≥p∏ř=1ηΥtř'ϱ(tř) |
It follows that
⇒ηt≥η't | (3.4) |
Consequently, in view of definition 12 and by comparing (3.3) and (3.4), we obtain the following:
PFDOWA(ἆt1,ἆt2,…,ἆtp)≤PFDOWA(ἆ't1,ἆ't2,…,ἆ'tp). |
Definition 13. Assume that ϕ is a collection of PFNs represented as ἆtř=(μtř,ηtř) where, ř=1,2.3,…,p and Υt=[Υt1,Υt2,…,Υtp]Tis the weight vector linked to the time periods tř such that ∑př=1Υtř=1 and Υtř∈[0,1]. The PFDOWG operator is a mapping PFDOWG:ϕp→ϕ specified by the rule:
PFDOWG(ἆt1,ἆt2,…,ἆtp)=⊕př=1(ἆϱ(tř))Υtř=(p∏ř=1μΥ(tř)ϱ(tř),√1−p∏ř=1(1−η2ϱ(tř))Υ(tř)) |
In the upcoming theorem, we will demonstrate that the aggregated value of PFNs at different time periods, using the PFDOWG operator, results in a new PFN.
Theorem 5. Consider p numbers of PFNs expressed as ἆtř=(μtř,ηtř) at p different time periods tř where, ř=1,2,3,…,p, and the associated weight vector is Υt=[Υt1,Υt2,….,Υtp]T with some restrictions ∑př=1Υtř=1 and Υtř∈[0,1]. The aggregated value of these PFNs using PFDOWG operator is also a PFN. It can be described as follows:
PFDOWG(ἆt1,ἆt2,…,ἆtp)=[p∏ř=1μΥtřϱ(tř),√1−p∏ř=1(1−η2ϱ(tř))Υtř] |
Example 2. Consider ἆt1=(0.70,0.40), ἆt2=(0.80,0.30), ἆt3=(0.50,0.70) and ἆt4=(0.50,0.40) represent four PFNs and Υt=(0.2,0.1,0.3,0.4)T is the weight vector linked to time periods tř where ř=1,2,3,4. First we compute the scores values of ἆtř by applying definition 7 as follows:
S(ἆt1)=0.33,S(ἆt2)=0.55,S(ἆt3)=−0.24,S(ἆt4)=0.09 |
Then the permuted values of PFNs are given as:
ἆϱ(t1)=(0.80,0.30),ἆϱ(t2)=(0.70,0.40),ἆϱ(t3)=(0.50,0.40),ἆϱ(t4)=(0.50,0.70) |
In view of definition 13, the aggregated values of above PFNs in the context of PFDOWG operator is calculated as follows:
PFDOWG(ἆt1,ἆt2,ἆt3,ἆt4)=(4∏ř=1μΥ(tř)ϱ(tř),√1−4∏ř=1(1−η2ϱ(tř))Υ(tř)) |
PFDOWG(ἆt1,ἆt2,ἆt3,ἆt4)=((0.8)0.2(0.7)0.1(0.5)0.3(0.5)0.4),√1−(1−0.32)0.2(1−0.42)0.1(1−0.42)0.3(1−0.72)0.4 |
It follows that
PFDOWG(ἆt1,ἆt2,ἆt3,ἆt4)=(0.568,0.548). |
In the upcoming theorem, we establish the idempotency property of the PFDOWG operator, showing that if all PFNs are equal for a given time period, the aggregated result will also equal that PFN.
Theorem 6. Consider the collection of p number of PFNs represented as ἆ(tř)=(μ(tř),η(tř)), existing at p different time periods tř, and let Υt=(Υt1,Υt2,…,Υtp)Tbe the weight vector linked to time periods tř with the condition ∑př=1Υtř=1 and Υtř∈[0,1], where ř=1,2,…,p. If ἆϱ(tř)=ἆϱ(tj) are mathematically equal for all ř and for some j∈{1,2,…,p} where, ἆϱ(tj)=(μϱ(tj),ηϱ(tj)). Then
PFDOWG(ἆt1,ἆt2,…,ἆtp)=ἆϱ(tj) |
In the upcoming theorem, we establish the boundedness property of the PFDOWG operator, demonstrating that the aggregated value of PFNs lies between the defined lower and upper bounds.
Theorem 7. Consider ἆ−=(minř(μϱ(tř)),maxř(ηϱ(tř))) and ἆ+=(maxř(μϱ(tř)),minř(ηϱ(tř))) be the lower bound and upper bound of PFNs ἆϱ(tř)=(μϱ(tř),ηϱ(tř)) where, ř=1,2,…,p. Let Υt=(Υt1,Υt2,…,Υtp)Tbe the weight vector associated with time periods tř such that Υtř∈[0,1] meeting the restriction ∑př=1Υtř=1. Then
ἆ−≤PFDOWG(ἆt1,ἆt2,…,ἆtp)≤ἆ+. |
In the upcoming theorem, we establish the monotonicity property of the PFDOWG operator, showing that if one set of PFNs dominates another, their aggregated values will preserve this ordering.
Theorem 8. Let ἆtř=(μtř,ηtř) and ἆ'tř=(μ'tř,η'tř) where, ř=1,2,…,p, be any two collection of PFNs. Let Υt=(Υt1,Υt2,…,Υtp)Trepresents the weight vector linked to time periods tř, such that Υtř∈[0,1] and ∑př=1Υř=1. If μϱ(tř)≤μ'ϱ(tř) and ηϱ(tř)≥η'ϱ(tř) then we can establish that:
PFDOWG(ἆt1,ἆt2,…,ἆtp)≤PFDOWG(ἆ't1,ἆ't2,…,ἆ'tp). |
In this section, we present a new approach for solving MADM issues. This method utilizes PF dynamic information and applies the recently defined PF dynamic ordered weighted AOs.
1) Let us consider a finite set of alternatives represented as Ȳ={Ȳ1,Ȳ2,…,Ȳm}.
2) Let a collection of attributes be indicated as Ջ={Ջ1,Ջ2,…,Ջn} and the related weight vector is ς=(ς1,ς2,…,ςn)T such that ςj∈[0,1] and ∑nj=1ςj=1 where j=1,2,…,n.
3) In addition, we incorporate the notion of distinct time periods tř where, ř=1,2,…,p, and these time periods are characterized by an associated weight vector Υt=[Υt1,Υt2,…,Υtp]T, where Υtř∈[0,1] and ∑př=1Υtř=1.
4) Consider Rtř=[Eij(tř)]m×n=(μij(tř),ηij(tř))m×n indicates the PF dynamic decision matrices of time periods tř, where μij(tř) represents the ratings to which the alternative Ȳi fulfills the attribute Ջj at time period tř, and ηij(tř) reflects the degree that the alternative Ȳi fails to satisfy with the attribute Ջj at time period tř. In addition, the following restrictions apply to these values:
μijϱ(tř)∈[0,1],ηijϱ(tř)∈[0,1]and(μijϱ(tř))2+(ηijϱ(tř))2≤1. |
Based on the aforementioned decision information, we formulate a methodology for assessing and selecting the most favorable alternative. The process involves the following steps:
Step 1. Obtain the PF dynamic decision matrices Rtř=[Eij(tř)]m×n=(μij(tř),ηij(tř))m×n that indicate various alternatives associated with attributes.
Step 2. To acquire the permuted PF dynamic decision matrices Rϱ(tř)=[Eijϱ(tř)]m×n=(μijϱ(tř),ηijϱ(tř))m×n we implement the subsequent two stages:
• Obtain the score values for each attribute Ջj relating to each alternative Ȳi within each matrix Rtř during the time period tř using definition 7.
• Formulate the permuted PF dynamic decision matrices by organizing the calculated values from the preceding step for each attribute Ջj, related to each alternative Ȳi within each matrix Rtř, in a descending order during the time period tř.
Step 3. Utilize the recently developed PFDOWA operator in the following manner:
PFDOWA(Eijϱ(t1),Eijϱ(t2),…,Eijϱ(tp))=Eijϱ(tř)=(μijϱ(tř),ηijϱ(tř)) |
It implies that
PFDOWA(Eijϱ(t1),Eijϱ(t2),…,Eijϱ(tp))=(√1−p∏ř=1(1−μ2ijϱ(tř))Υtř,p∏ř=1ηΥtřijϱ(tř)) |
Here, i varies from 1 to m and and j varies from 1 to n. This technique integrates all the PF decision matrices to a unified PF decision matrix as R=[Eij]m×n=(μij,ηij)m×n.
Step 4. Determine the combined value Ei=(μi,ηi) of the alternatives Ȳi by applying the PFWA operator to the collective decision matrix as follows:
PFWA(Ei1,Ei2,…,Ein)=Ei=(μi,ηi) |
It follows that:
PFWA(Ei1,Ei2,…,Ein)=(√1−n∏j=1(1−μ2ij)ςj,n∏j=1ηςjij) |
Step 5. Applying definition 7, compute the scores S(Ei) of the PF preference values Ei for all the alternatives Ȳi.
Step 6. Obtain the score values S(Ei), write all Ȳi in decreasing sequence and select the best one.
Figure 1 demonstrates the flowchart of developed approach for addressing MADM issues through the PFDOWA operator.
Step 1. Obtain the PF dynamic decision matrices Rtř=[Eij(tř)]m×n=(μij(tř),ηij(tř))m×n that indicate various alternatives associated with attributes.
Step 2. To acquire the permuted PF dynamic decision matrices Rϱ(tř)=[Eijϱ(tř)]m×n=(μijϱ(tř),ηijϱ(tř))m×n we implement the subsequent two stages:
• Acquire the score values for each attribute Ջj related to each alternative Ȳi within each matrix Rtř during the time period tř using definition 7.
• Formulate the permuted PF dynamic decision matrices by arranging the calculated values from the preceding step for each attribute Ջj, related to each alternative Ȳi within each matrix Rtř, in a descending order during the time period tř.
Step 3. Utilize the recently developed PFDOWG operator in the following manner:
PFDOWG(Eijϱ(t1),Eijϱ(t2),…,Eijϱ(tp))=Eijϱ(tř)=(μijϱ(tř),ηijϱ(tř)) |
It implies that
PFDOWG(Eijϱ(t1),Eijϱ(t2),…,Eijϱ(tp))=(p∏ř=1(μijϱ(tř))Υtř,√1−p∏ř=1(1−η2ijϱ(tř))Υtř) |
Here, i varies from 1 to m and and j varies from 1 to n. This technique integrates all the PF decision matrices to a unified PF decision matrix as R=[Eij]m×n=(μij,ηij)m×n.
Step 4. Calculate the aggregated value Ei=(μi,ηi) of the alternatives Ȳi by applying PFWG operator on collective decision matrix as follows:
PFWG(Ei1,Ei2,…,Ein)=Ei=(μi,ηi) |
It follows that:
PFWG(Ei1,Ei2,…,Ein)=(n∏ř=1(μiř)ςř,√1−n∏ř=1(1−η2iř)ςř). |
Step 5. Applying definition 7, compute the scores S(Ei) of the PF preference values Ei for all the alternatives Ȳi.
Step 6. Obtain the score values S(Ei), write all Ȳi in decreasing sequence and select the best one.
Figure 2 demonstrates the flowchart of developed approach for addressing MADM issues through the PFDOWG operator.
In this section of the article, we solve a MADM problem within the context of algorithm developed in section 4 and establish a comparative analysis to showcase the validity of this novel approach with the existing methodologies.
Illnesses affecting the heart and blood vessels are commonly known as cardiovascular illnesses. In the United States, abnormal saturated fat is present in over half of the population. There are two possibilities for managing CVD: Changing your lifestyle or getting medication from your doctor. CVD is easier to treat the earlier it is identified. In the US as well as throughout the world, CVD is the main cause of death. Heart disease is responsible for 655,000 deaths in America each year. In the United States, about 50% of people have some form of cardiovascular disease. Both men and women are impacted. Indeed, one in every three women dies from cardiovascular disease. People of different age groups, races, and socioeconomic backgrounds are affected by it. Tobacco, excessive drinking, insufficient nutrition, and a sedentary lifestyle are the main psychological risk factors linked to cardiovascular illness and death. Behavioral risk factors may result in the development of high cholesterol levels, high blood sugar levels, elevated blood fatty acids, weight gain, or obesity in adults. These intermediate risk variables suggest a heightened likelihood of experiencing a heart attack, bleeding, or cardiac arrest, along with related outcomes. In order to mitigate the risks linked to CVD, it is advisable to quit smoking, reduce salt intake, increase consumption of fruits and vegetables, engage in regular exercise, and avoid excessive alcohol use. To encourage the adoption and maintenance of healthy habits, it is critical to enact health policies that make healthy options more accessible and affordable. CVDs are influenced by additional underlying causes. Globalization, urbanization, and population aging are the major components that exert influence on financial, social, and cultural aspects. Genetic factors, anxiety, and poverty are additional risk factors for CVD. Treatment for hypertension, diabetes, and high blood cholesterol is essential to mitigate cardiovascular risk and prevent strokes and heart attacks in individuals with these conditions.
Studies have highlighted significant advancements in CVD detection and treatment. Innovative imaging techniques for liver and cardiovascular conditions have enhanced disease management [46], while histone modifications have emerged as potential therapeutic targets in calcific aortic valve disease [47]. Intravascular ultrasonography has also shown promise in treating carotid stenosis [48]. Research into hypoxia-induced signaling [49] and new approaches in electrocardiogram classifications [50] further underscore the need for comprehensive strategies to reduce CVD risks and improve outcomes.
Various methodologies exist for the management and prevention of CVD all over the world. A brief description of some important approaches to preventing CVD. These approaches include.
1) An array of healthy lifestyle choices can contribute to the enhancement of cardiovascular health and the mitigation of illness risk. These choices encompass refraining from smoking, sustaining an optimal weight by adhering to a balanced diet, and engaging in consistent physical activity. Consistent physical exercise aids in weight management while concurrently enhancing cardiovascular health. Many health advantages may be obtained from a diet abundant in fruits, vegetables, lean meats, and whole grains, which can also successfully decrease blood pressure. The cessation of smoking markedly diminishes the chance of developing cardiovascular disease.
2) Pharmacological interventions play a vital role in preventing and managing CVD risks, encompassing antihypertensive agents, statins, and antiplatelet medications. These techniques of therapy are widely accepted by physicians, who need strict attention to the suggested regimens to assure their effectiveness.
3) Primary safeguarding represents a proactive strategy that aims to prevent the development of cardiovascular disease in asymptomatic individuals. This all-encompassing approach entails the implementation of a healthy nutritional plan, consistent engagement in physical activity, and periodic screening for potential risk factors, such as high cholesterol levels and blood pressure.
4) Secondary mitigation stands as a pivotal aspect within the comprehensive cardiovascular disease approach. This requirement involves impeding the progression of CVD among individuals who are already suffering from the disease. Such endeavors necessitate the enactment of prudent lifestyle changes, the discerning application of pharmaceutical treatments, and vigilant oversight by healthcare professionals to observe progress and mitigate unexpected adverse reactions.
5) Within the domain of cardiovascular disease prevention and management, the meticulous management of risk factors is of the highest priority. Individuals can markedly diminish their vulnerability to CVD by efficiently managing situations such as diabetes, hypertension, and elevated cholesterol levels. The orchestration of this preventive regimen involves pharmaceutical adjustments, modifications to one's lifestyle, and vigilant medical supervision.
6) An integral facet of the multidimensional approach to combating CVD encompasses community-based initiatives. These endeavors encompass a diverse array of activities, comprising public health campaigns, support groups and educational programs, all aimed at enhancing public awareness of CVD and fostering healthier lifestyles
Based on data available in 2019, cardiovascular disease appeared as the predominant cause of death in Asia, accounting for approximately eleven million fatalities. Around 39 percent of these possible fatalities from CVD could have been prevented. The amount of premature deaths from CVD was greater than the number of premature deaths from CVD in the US (23%), Europe (22%), and worldwide (34%). Attributable to CVDs, the global mortality toll witnessed a substantial escalation, rising from approximately 12.1 million in 1990, evenly distributed between genders, to 18.6 million in 2019.
The exacerbation of patient treatment expenses and challenges within Pakistan's pharmaceutical industry in recent decades can be attributed to an inequitable distribution of medical supplies, inefficiencies within the healthcare sector, and deficiencies in the health framework. Diseases are evolving towards increased severity, and the twenty-first century's socioeconomic globalization has brought about significant and enduring enhancements in individuals' living standards. The burgeoning discord between ecological considerations and human expansion is increasingly apparent. Numerous urban areas in Pakistan are grappling with severe climatic conditions, posing heightened challenges for the medical sector. The prevailing environmental issues are currently impeding the healthcare industry in Pakistan. Presently, the number of patients diagnosed with CVD is escalating at an accelerated rate. The inclination of individuals to seek medical counsel and aid at larger hospitals is notably elevated in comparison to the inferior care settings and treatment offerings found at smaller clinics. Lahore hospital, being the largest medical facility in Pakistan, is equipped with state-of-the-art medical equipment and ample resources. Given a considerable increase in workload over the past several decades, the Lahore hospital has been unable to adequately meet the increased demands. Within the framework of Lahore hospital, the establishment of a medical system with hierarchies is regarded as a viable strategy to alleviate the strain induced by the substantial influx of patients. The objective is to systematically categorize the degree of treatment complexity according to the type of disease. The attainment of medical qualifications from diverse institutions equips practitioners to adeptly address a diverse spectrum of ailments. A fundamental aspect of the hierarchical healthcare system is the classification of diseases into different severity categories. There is a hierarchical medical care system in place, and instead of sending all patients to a Grade Ⅲ or Class A institution, people with different medical conditions can choose from numerous tiers of institutions. Determining diverse levels of illness severity is an essential initial step in building the hierarchical framework. In order to help build the medical system's hierarchical structure, we aim to classify the different stages of CVD.
The crucial step is to carefully choose a group of experts who possess a deep comprehension of the problem's importance. Considering that this article mainly entails medical therapy, we have primarily focused on selecting physicians who have experience in this subject. The consultants have been assigned the responsibility of managing CVD by employing a range of different methods and key features in the treatment process.
Let {Ȳ1,Ȳ2,Ȳ3,Ȳ4,Ȳ5} represent the set of alternatives for the treatment of CVD;
ⅰ. Ȳ1: Angioplasty;
ⅱ. Ȳ2: Lifestyle modifications;
ⅲ. Ȳ3: Blood pressure management;
ⅳ. Ȳ4: Medication;
ⅴ. Ȳ5: Coronary Artery Bypass Grafting (ABG);
Let {Ջ1,Ջ2,Ջ3,Ջ4} represent the set of attributes, each of which contributes to the medical care of CVD.
ⅰ. Ջ1: Efficiency;
ⅱ. Ջ2: Reliability;
ⅲ. Ջ3: Expertise required;
ⅳ. Ջ4: Sensitivity;
A medical team wants to analyze the five possible alternative Ȳi values, where i ranges from 1 to 5, utilizing PF information. In accordance with the aforementioned four attributes Ջ1, Ջ2,Ջ3 and Ջ4 at the time periods t1, t2, and t3. Here t1 designates the period 1990–1999, t2 is pans from 2000 to 2009, and t3 portrays the progression from 2010 to 2019. The medical team has assigned weight values to the three time periods, represented by the weight vector Υt=[0.2,0.3,0.5]T, where ∑3ř=1Υtř=1 and the weight vector of attributes isς=[0.15,0.16,0.5,0.19]T where, ∑4j=1ςj=1. The medical expert opinion to legitimate the alternative Ȳi with respect to the time period tř is summarized in the f PF dynamic decision matrices Rt1, Rt2 and Rt3(See Tables 2–4).
Ջ1 | Ջ2 | Ջ3 | Ջ4 | |
Ȳ1 | (0.8, 0.3) | (0.4, 0.2) | (0.9, 0.2) | (0.5, 0.6) |
Ȳ2 | (0.6, 0.5) | (0.7, 0.3) | (0.4, 0.6) | (0.5, 0.4) |
Ȳ3 | (0.5, 0.5) | (0.8, 0.3) | (0.6, 0.3) | (0.5, 0.6) |
Ȳ4 | (0.9, 0.2) | (0.6, 0.5) | (0.7, 0.3) | (0.4, 0.5) |
Ȳ5 | (0.3, 0.7) | (0.5, 0.4) | (0.8, 0.2) | (0.6, 0.5) |
Ջ1 | Ջ2 | Ջ3 | Ջ4 | |
Ȳ1 | (0.6, 0.3) | (0.5, 0.3) | (0.8, 0.3) | (0.7, 0.2) |
Ȳ2 | (0.7, 0.3) | (0.8, 0.2) | (0.5, 0.4) | (0.6, 0.5) |
Ȳ3 | (0.9, 0.2) | (0.6, 0.5) | (0.7, 0.3) | (0.4, 0.5) |
Ȳ4 | (0.5, 0.5) | (0.8, 0.3) | (0.5, 0.6) | (0.6, 0.3) |
Ȳ5 | (0.4, 0.5) | (0.5, 0.3) | (0.6, 0.3) | (0.7, 0.4) |
Ջ1 | Ջ2 | Ջ3 | Ջ4 | |
Ȳ1 | (0.6, 0.3) | (0.5, 0.2) | (0.7, 0.4) | (0.3, 0.4) |
Ȳ2 | (0.4, 0.5) | (0.6, 0.5) | (0.7, 0.3) | (0.9, 0.2) |
Ȳ3 | (0.6, 0.5) | (0.3, 0.7) | (0.5, 0.4) | (0.8, 0.2) |
Ȳ4 | (0.7, 0.4) | (0.5, 0.3) | (0.4, 0.5) | (0.6, 0.3) |
Ȳ5 | (0.7, 0.4) | (0.7, 0.3) | (0.6, 0.5) | (0.4, 0.8) |
Within the context of the PFDOWA and PFDOWG operators, the given MADM problem is solved as follows:
Step 1. The permuted PF dynamic decision matrix Rϱ(t1)=[Eijϱ(t1)]5×4=(μijϱ(t1),ηijϱ(t1))5×4 is computed as follows:
ⅰ. Apply definition 7 to ascertain the score values of all four attributes of each alternative of decision matrix Rt1 at the time period t1.
• For alternative Ȳ1, we have
S(E11)=0.55,S(E12)=0.12,S(E13)=0.77,S(E14)=−0.11 |
• For alternative Ȳ2, we have
S(E21)=0.11,S(E22)=0.40,S(E23)=−0.2,S(E24)=0.09 |
• For alternative Ȳ3, we have
S(E31)=0,S(E32)=0.55,S(E33)=0.27,S(E34)=−0.11 |
• For alternative Ȳ4, we have
S(E41)=0.77,S(E42)=0.11,S(E43)=0.40,S(E44)=−0.09 |
• For alternative Ȳ5, we have
S(E51)=−0.40,S(E52)=0.09,S(E53)=0.60,S(E54)=0.11 |
ⅱ. List the values obtained in the previous stage for each option in in decreasing sequence, displayed below:
• For alternative Ȳ1;
S(E13)>S(E11)>S(E12)>S(E14) |
• For alternative Ȳ2;
S(E22)>S(E21)>S(E24)>S(E23) |
• For alternative Ȳ3;
S(E32)>S(E33)>S(E31)>S(E34) |
• For alternative Ȳ4;
S(E41)>S(E43)>S(E42)>S(E44) |
• For alternative Ȳ5;
S(E53)>S(E54)>S(E52)>S(E51) |
Moreover, the permuted PF decision matrix Rϱ(t2)=[Eijϱ(t2)]5×4=(μijϱ(t2),ηijϱ(t2))5×4 is computed as follows:
ⅰ. Apply definition 7 to ascertain the score values of all four attributes of each alternative of decision matrix Rt2 at the time period t2.
• For alternative Ȳ1, we have
S(E11)=0.27,S(E12)=0.16,S(E13)=0.55,S(E14)=0.45 |
• For alternative Ȳ2, we have
S(E21)=0.40,S(E22)=0.60,S(E23)=0.09,S(E24)=0.11 |
• For alternative Ȳ3, we have
S(E31)=0.77,S(E32)=0.11,S(E33)=0.40,S(E34)=−0.09 |
• For alternative Ȳ4, we have
S(E41)=0,S(E42)=0.55,S(E43)=−0.11,S(Ȳ44)=0.27 |
• For alternative Ȳ5, we have
S(E51)=−0.09,S(E52)=0.16,S(E53)=0.27,S(E54)=0.33 |
ⅱ. List the values obtained in the previous stage for each option in in decreasing sequence, displayed below:
• For alternative Ȳ1;
S(E13)>S(E14)>S(E11)>S(E12) |
• For alternative Ȳ2;
S(E22)>S(E21)>S(E24)>S(E23) |
• For alternative Ȳ3;
S(E31)>S(E33)>S(E32)>S(E34) |
• For alternative Ȳ4;
S(E42)>S(E44)>S(E41)>S(E43) |
• For alternative Ȳ5;
S(E54)>S(E53)>S(E52)>S(E51) |
Furthermore, the permuted PF decision matrix Rϱ(t3)=[Eijϱ(t3)]5×4=(μijϱ(t3),ηijϱ(t3))5×4 is computed as follows:
ⅰ. Apply definition 7 to ascertain the score values of all four attributes of each alternative of decision matrix Rt3 at the time period t3.
• For alternative Ȳ1, we have
S(E11)=0.27,S(E12)=0.21,S(E13)=0.33,S(E14)=−0.07 |
• For alternative Ȳ2, we have
S(E21)=−0.09,S(E22)=0.11,S(E23)=0.40,S(E24)=0.77 |
• For alternative Ȳ3, we have
S(E31)=0.11,S(E32)=−0.40,S(E33)=0.09,S(E34)=0.60 |
• For alternative Ȳ4, we have
S(E41)=0.33,S(E42)=0.16,S(E43)=−0.09,S(E44)=0.27 |
• For alternative Ȳ5, we have
S(E51)=0.33,S(E52)=0.40,S(E53)=0.11,S(E54)=0.48 |
ⅱ. List the values obtained in the previous stage for each option in in decreasing sequence, displayed below:
• For alternative Ȳ1;
S(E13)>S(E11)>S(E12)>S(E14) |
• For alternative Ȳ2;
S(E24)>S(E23)>S(E22)>S(E21) |
• For alternative Ȳ3;
S(E34)>S(E31)>S(E33)>S(E32) |
• For alternative Ȳ4;
S(E41)>S(E44)>S(E42)>S(E43) |
• For alternative Ȳ5;
S(E54)>S(E52)>S(E51)>S(E53) |
Step 2. Formulate the permuted PF decision matrices Rϱ(t1), Rϱ(t2), and Rϱ(t2) (See Tables 5–7) using the data acquired from step 1.
Ջ1 | Ջ2 | Ջ3 | Ջ4 | |
Ȳ1 | (0.9, 0.2) | (0.8, 0.3) | (0.4, 0.2) | (0.5, 0.6) |
Ȳ2 | (0.7, 0.3) | (0.6, 0.5) | (0.5, 0.4) | (0.4, 0.6) |
Ȳ3 | (0.8, 0.3) | (0.6, 0.3) | (0.5, 0.5) | (0.5, 0.6) |
Ȳ4 | (0.9, 0.2) | (0.7, 0.3) | (0.6, 0.5) | (0.4, 0.5) |
Ȳ5 | (0.8, 0.2) | (0.6, 0.5) | (0.5, 0.4) | (0.3, 0.7) |
Ջ1 | Ջ2 | Ջ3 | Ջ4 | |
Ȳ1 | (0.8, 0.3) | (0.7, 0.2) | (0.6, 0.3) | (0.5, 0.3) |
Ȳ2 | (0.8, 0.2) | (0.7, 0.3) | (0.6, 0.5) | (0.5, 0.4) |
Ȳ3 | (0.9, 0.2) | (0.7, 0.3) | (0.6, 0.5) | (0.4, 0.5) |
Ȳ4 | (0.8, 0.3) | (0.6, 0.3) | (0.5, 0.5) | (0.5, 0.6) |
Ȳ5 | (0.7, 0.4) | (0.6, 0.3) | (0.5, 0.3) | (0.4, 0.5) |
Ջ1 | Ջ2 | Ջ3 | Ջ4 | |
Ȳ1 | (0.7, 0.4) | (0.6, 0.3) | (0.5, 0.2) | (0.3, 0.5) |
Ȳ2 | (0.9, 0.2) | (0.7, 0.3) | (0.6, 0.5) | (0.4, 0.5) |
Ȳ3 | (0.8, 0.2) | (0.6, 0.5) | (0.5, 0.4) | (0.3, 0.7) |
Ȳ4 | (0.7, 0.4) | (0.6, 0.3) | (0.5, 0.3) | (0.4, 0.5) |
Ȳ5 | (0.7, 0.3) | (0.7, 0.4) | (0.6, 0.5) | (0.4, 0.8) |
Step 3. We aggregate all of the PF permuted decision matrices Rt1, Rt2and Rt3 using the PFDOWA operator to obtain a collective PF decision matrix R, as shown in Table 8.
Ջ1 | Ջ2 | Ջ3 | Ջ4 | |
Ȳ1 | (0.78, 0.32) | (0.68, 0.27) | (0.52, 0.23) | (0.42, 0.45) |
Ȳ2 | (0.85, 0.22) | (0.68, 0.33) | (0.58, 0.48) | (0.43, 0.48) |
Ȳ3 | (0.84, 0.22) | (0.63, 0.39) | (0.53, 0.45) | (0.38, 0.61) |
Ȳ4 | (0.79, 0.32) | (0.62, 0.3) | (0.52, 0.39) | (0.43, 0.53) |
Ȳ5 | (0.72, 0.30) | (0.65, 0.38) | (0.55, 0.41) | (0.42, 0.68) |
Step 4. Compute the aggregated value Ei=(μi,ηi) of the alternatives Ȳi where, i=1,2,3,4,5 by employing the PFWA operator on Table 8. The outcomes of this procedure are listed in Table 9.
Ei | |
Ȳ1 | (0.596, 0.278) |
Ȳ2 | (0.644, 0.402) |
Ȳ3 | (0.607, 0.419) |
Ȳ4 | (0.586, 0.385) |
Ȳ5 | (0.582, 0.426) |
Step 5. To compute the scores S(Ei) of the entire PF preference values Ei for each alternative Ȳi, utilize definition 7.
S(E1)=(0.277)S(E2)=(0.253)S(E3)=(0.193)S(E4)=(0.195)S(E5)=(0.157) |
Step 6. In the light of the score values S(Ei) obtained from the previous step the alternatives Ȳi are to be ranked as follows: Ȳ1>Ȳ2>Ȳ4>Ȳ3>Ȳ5.
Step 7. The aforementioned discussion concludes that "Angioplasty" is the efficient strategy to cure the CVD.
Figure 3 shows the hierarchical ordering of alternatives as determined by the PFDOWA operator.
Similarly, the PFDOWG operator resolves the previously mentioned MADM problem employing the subsequent method:
Step 1. To get a collective PF decision matrix R (see Table 10), we aggregate all the PF permuted decision matrices Rtř employing the PFDOWG operator.
Ջ1 | Ջ2 | Ջ3 | Ջ4 | |
Ȳ1 | (0.77, 0.34) | (0.67, 0.27) | (0.51, 0.24) | (0.39, 0.47) |
Ȳ2 | (0.83, 0.22) | (0.68, 0.35) | (0.58, 0.48) | (0.43, 0.50) |
Ȳ3 | (0.83, 0.22) | (0.63, 0.42) | (0.53, 0.45) | (0.36, 0.63) |
Ȳ4 | (0.77, 0.34) | (0.62, 0.3) | (0.52, 0.42) | (0.43, 0.53) |
Ȳ5 | (0.72, 0.32) | (0.64, 0.40) | (0.55, 0.43) | (0.38, 0.72) |
Step 2. Compute the aggregated value Ei=(μi,ηi) of the alternatives Ȳi where, i=1,2,3,4,5 by employing the PFWG operator on Table 10. The Table 11 presents the computed values.
Ei | |
Ȳ1 | (0.534, 0.318) |
Ȳ2 | (0.593, 0.439) |
Ȳ3 | (0.541, 0.468) |
Ȳ4 | (0.547, 0.419) |
Ȳ5 | (0.547, 0.498) |
Step 3. Utilizing definition 7 to calculate the scores S(Ei) of the overall PF preference values Ei for each of the alternatives Ȳi.
S(E1)=(0.184)S(E2)=(0.159)S(E3)=(0.074)S(E4)=(0.124)S(E5)=(0.051) |
Step 4. In view of the score values S(Ei) the alternatives Ȳi are to be ranked in descending order as follows: Ȳ1>Ȳ2>Ȳ4>Ȳ3>Ȳ5 and the optimal alternative is to be chosen.
Step 5. The aforementioned discussion concludes that "Angioplasty" is the best strategy to cure the CVD.
Figure 4 shows the hierarchical ordering of alternatives as determined by the PFDOWG operator.
In the subsequent section, we compare our suggested operators to those developed in [22,38,39,45,51] to evaluate their effectiveness and reliability for the MADM issue. We utilize many approaches, such as the IFDWA, IFDWG, PFDOWA, and PFDOWG operators, to gather and consolidate similar data. The results produced by these operators are compiled in Table 12, and the outcomes provided according to their ranking are presented in Table 13.
IFDWA [38] | IFDWG [39] | PFDOWA | PFDOWG | |
E(Ȳ1) | (0.688, 0.407) | (0.618, 0.326) | (0.596, 0.278) | (0.534, 0.318) |
E(Ȳ2) | (0.654, 0.378) | (0.597, 0.395) | (0.644, 0.402) | (0.593, 0.439) |
E(Ȳ3) | (0.592, 0.396) | (0.581, 0.464) | (0.607, 0.419) | (0.541, 0.468) |
E(Ȳ4) | (0.577, 0.405) | (0.535, 0.434) | (0.586, 0.385) | (0.547, 0.419) |
E(Ȳ5) | (0.615, 0.403) | (0.550, 0.422) | (0.582, 0.426) | (0.547, 0.498) |
S(E1) | S(E2) | S(E3) | S(E4) | S(E5) | Ranking order | |
IFDWA | 0.281 | 0.276 | 0.196 | 0.172 | 0.212 | Ȳ1>Ȳ2>Ȳ5>Ȳ3>Ȳ4 |
IFDWG | 0.292 | 0.202 | 0.117 | 0.101 | 0.128 | Ȳ1>Ȳ2>Ȳ5>Ȳ3>Ȳ4 |
PFDOWA | 0.277 | 0.253 | 0.193 | 0.195 | 0.157 | Ȳ1>Ȳ2>Ȳ4>Ȳ3>Ȳ5 |
PFDOWG | 0.184 | 0.159 | 0.074 | 0.124 | 0.051 | Ȳ1>Ȳ2>Ȳ4>Ȳ3>Ȳ5 |
Comparison 1. The suggested techniques are genuine and suitable for MADM challenges. The primary reasoning for the superiority of our suggested methods lies in the fact that these models exhibit a more comprehensive framework, which serves as an expansion of the techniques developed in [22]. In addition, the methods developed in [22] are inadequate for the analysis of the data in Tables 2–4 because they cannot handle the problems related to decision-making that are reliant on time, whereas the models proposed in this study can handle data from many time intervals, making them more adaptable.
Comparison 2. The incorporation of the techniques presented in this study within the context of the dynamic PF environment enhances their efficacy. This is due to the fact that they assess many time periods and provide a more precise assessment of the data under consideration. The approaches delineated in [38,39] can be considered a specific case of the innovative methods introduced in our current investigation because they have a more restricted area of application compared to the methods presented in this study within the framework of PF knowledge. Thus, the newly proposed approaches provide a wider array of comprehensive alternatives for identifying and mitigating ambiguity than the methods outlined in [38,39].
Comparison 3. A significant quantity of data is lost because the aggregation techniques explained in [45,51] do not include time intervals, whereas the proposed aggregation techniques are dynamic because they can handle data from many time intervals, making them more adaptable.
The above discussion highlights that the mathematical frameworks of the proposed operators utilizing PF knowledge with time intervals are highly effective and substantial. This establishes the novel techniques' efficiency over other strategies.
We seek to unveil innovative strategies for addressing complex decision-making challenges through the application of a dynamic PF framework. Although the literature has already established various useful operators, none of these specifically take into account the issue of time duration in PF settings. Consequently, utilizing a dynamic PF model proves to be a more efficient method for elucidating information associated with time-dependent considerations, as it adeptly handles two-dimensional data within an integrated framework. In light of these factors, we have implemented a new collection of operators, specifically PFDOWA and PFDOWG, within the framework of the PF environment. We have examined several features of these operators. Furthermore, we have suggested step-by-step mathematical mechanisms to address dynamic PF MADM issues using innovative strategies. Moreover, we have demonstrated the practical application of these newly devised methodologies in discerning the optimal strategy for treating cardiovascular disease in a patient. Finally, we have compared these novel approaches with existing methods to show their reliability and importance.
Despite the advantages offered by the methodologies proposed in this article, several limitations remain:
ⅰ. These techniques are incapable of dealing with situations in which the sum of the squares of the membership and non-membership values surpasses 1.
ⅱ. Due to their limitation to accepting just two parameters, these techniques are incapable of managing model cases that involve picture and spherical fuzzy information.
The principal objective of forthcoming research is to address the constraints recognized in the present study by formulating methodologies applicable to generalized settings, specifically within the contexts of interval-valued Pythagorean fuzzy, complex Pythagorean fuzzy, bipolar fuzzy, spherical fuzzy, and picture fuzzy frameworks. Another goal of future research will be to create a comprehensive decision-analysis tool that employs PF dynamic aggregation operators in order to maximize their significance and efficiency. The strategies suggested in this article will be flexible and applicable to a range of situations, such as the creation of more flexible financial plans, real-time social media activity monitoring online, dynamic military management assessment, dynamic and private shortlisting procedures, addressing the lack of energy in developing countries, and addressing time-dependent MADM issues.
Mehwish Shehzadi: Original draft preparation, Formal analysis, Investigation; Hanan Alolaiyan: Writing and review, Validation of results, Investigation; Umer Shuaib: Conceptualization, Supervision, Methodology; Abdul Razaq: Investigation, Writing and review, Formal analysis; Qin Xin: Investigation, Writing and review, Methodology.
This project is supported by the Researchers Supporting Project Number (RSP2024R317) King Saud University, Riyadh, Saudi Arabia.
All the authors declare no conflict of interest.
Proof (Theorem 5). The technique of mathematical induction is used to establish the proof of this theorem.
For p=2. We have
PFDOWG(ἆt1,ἆt2)=ἆϱ(t1)Υt1⊕ἆϱ(t2)Υt2 |
Utilizing definition 12, we get
ἆϱ(t1)Υt1=[μΥt1ϱ(t1),√1−(1−η2ϱ(t1))Υt1]ἆϱ(t2)Υt2=[μΥt2ϱ(t2),√1−(1−η2ϱ(t2))Υt2] |
Thus,
ἆϱ(t1)Υt1⊕ἆϱ(t2)Υt2=[μΥt1ϱ(t1),√1−(1−η2ϱ(t1))Υt1]⊕[μΥt2ϱ(t2),√1−(1−η2ϱ(t2))Υt2]=[μΥt1ϱ(t1)μΥt2ϱ(t2),√1−(1−η2ϱ(t1))Υt1((1−η2ϱ(t2))Υt2)] |
In consequence,
PFDOWG(ἆt1,ἆt2)=[2∏ř=1μΥtřϱ(tř),√1−2∏ř=1(1−η2ϱ(tř))Υtř] |
Hence, the result is true for p=2.
Furthermore, we assume that the given result is true for =n>2, then we have:
PFDOWG(ἆt1,ἆt2,…,ἆtn)=⊕nř=1ἆϱ(tř)Υtř[n∏ř=1μΥtřϱ(tř),√1−n∏ř=1(1−η2ϱ(tř))Υtř] |
Now we prove the result p=n+1. It can be expressed as
PFDOWG(ἆt1,ἆt2,…,ἆtn,ἆtn+1)=⊕nř=1ἆϱ(tř)Υtř⊕ἆϱ(tn+1)Υtn+1=[∏nř=1μΥtřϱ(tř),√1−∏nř=1(1−η2ϱ(tř))Υtř]⊗[(μϱ(tn+1))Υtn+1,√1−(1−η2ϱ(tn+1))Υtn+1] |
It follows that
PFDOWG(ἆt1,ἆt2,…,ἆtn,ἆtn+1)=[n+1∏ř=1μΥtřϱ(tř),√1−n+1∏ř=1(1−η2ϱ(tř))Υtř,] |
Hence, the result holds for each p∈Z+.
Proof (Theorem 6). Given that ἆϱ(tř)=ἆϱ(tj) for all ř=1,2,…,p and for some j∈{1,2,…,p} which implies that μϱ(tř)=μϱ(tj) and ηϱ(tř)=ηϱ(tj).
PFDOWG(ἆt1,ἆt2,…,ἆtp)=(p∏ř=1μΥtřϱ(tř),√1−p∏ř=1(1−η2ϱ(tř))Υtř)=(μ∑př=1Υtřϱ(tj),√1−(1−η2ϱ(tj))∑př=1Υtř)=(μϱ(tj),√1−(1−η2ϱ(tj)))=(μϱ(tj),√η2ϱ(tj))=(μϱ(tj),ηϱ(tj)) |
Consequently,
PFDOWG(ἆt1,ἆt2,…,ἆtp)=ἆϱ(tj) |
The proofs of Theorems 7 and 8 can be derived by employing analogous reasoning to that used in the proofs of Theorems 3 and 4, respectively.
[1] |
D. Alghazzawi, A. Noor, H. Alolaiyan, H. A. El-Wahed Khalifa, A. Alburaikan, S. Dai, et al., A comprehensive study for selecting optimal treatment modalities for blood cancer in a Fermatean fuzzy dynamic environment, Sci. Rep., 14 (2024), 1896. https://doi.org/10.1038/s41598-024-51942-7 doi: 10.1038/s41598-024-51942-7
![]() |
[2] |
X. Gao, X. Cai, Y. Yang, Y. Zhou, W. Zhu, Diagnostic accuracy of the HAS-BLED bleeding score in VKA- or DOAC-treated patients with atrial fibrillation: a systematic review and meta-analysis, Front. Cardiovasc. Med., 8 (2021), 757087. https://doi:10.3389/fcvm.2021.757087 doi: 10.3389/fcvm.2021.757087
![]() |
[3] |
M. I. Faraz, G. Alhamzi, A. Imtiaz, I. Masmali, U. Shuaib, A. Razaq, et al., A decision-making approach to optimize COVID-19 treatment strategy under a conjunctive complex fuzzy environment, Symmetry, 15 (2023), 1370. https://doi.org/10.3390/sym15071370 doi: 10.3390/sym15071370
![]() |
[4] |
L. A. Zadeh, Fuzzy sets, Information and control, 8 (1965), 338–353. https://doi.org/10.1016/S0019-9958(65)90241-X doi: 10.1016/S0019-9958(65)90241-X
![]() |
[5] |
S. Kahne, A contribution to the decision making in environmental design, Proc. IEEE, 63 (1975), 518–528. https://doi.org/10.1109/PROC.1975.9779 doi: 10.1109/PROC.1975.9779
![]() |
[6] |
R. Jain, A procedure for multiple-aspect decision making using fuzzy sets, Int. J. Syst. Sci., 8 (1977), 1–7. https://doi.org/10.1080/00207727708942017 doi: 10.1080/00207727708942017
![]() |
[7] |
D. Dubois, H. Prade, Operations on fuzzy numbers, Int. J. Syst. Sci., 9 (1978), 613–626. https://doi.org/10.1080/00207727808941724 doi: 10.1080/00207727808941724
![]() |
[8] |
R. R. Yager, Aggregation operators and fuzzy systems modeling, Fuzzy Set. Syst., 67 (1994), 129–145. https://doi.org/10.1016/0165-0114(94)90082-5 doi: 10.1016/0165-0114(94)90082-5
![]() |
[9] | K. T. Atanassov, Intuitionistic fuzzy sets, In: Intuitionistic fuzzy sets, Heidelberg: Physica, 1999, 1–137. https://doi.org/10.1007/978-3-7908-1870-3_1 |
[10] | E. Szmidt, J. Kacprzyk, Intuitionistic fuzzy sets in group decision making, Notes on Intuitionistic Fuzzy Sets, 2 (1996), 15–32. |
[11] |
D.-F. Li, Multiattribute decision making models and methods using intuitionistic fuzzy sets, J. Comput. Syst. Sci., 70 (2005), 73–85. https://doi.org/10.1016/j.jcss.2004.06.002 doi: 10.1016/j.jcss.2004.06.002
![]() |
[12] |
Z. Xu, R. R. Yager, Some geometric aggregation operators based on intuitionistic fuzzy sets, Int. J. Gen. Syst., 35 (2006), 417–433. https://doi.org/10.1080/03081070600574353 doi: 10.1080/03081070600574353
![]() |
[13] |
Z. Xu, Intuitionistic fuzzy aggregation operators, IEEE Trans. Fuzzy Syst., 15 (2007), 1179–1187. https://doi.org/10.1109/TFUZZ.2006.890678 doi: 10.1109/TFUZZ.2006.890678
![]() |
[14] |
H. Zhao, Z. Xu, M. Ni, S. Liu, Generalized aggregation operators for intuitionistic fuzzy sets, Int. J. Intell. Syst., 25 (2010), 1–30. https://doi.org/10.1002/int.20386 doi: 10.1002/int.20386
![]() |
[15] |
Y. Xu, H. Wang, The induced generalized aggregation operators for intuitionistic fuzzy sets and their application in group decision making, Appl. Soft Comput., 12 (2012), 1168–1179. https://doi.org/10.1016/j.asoc.2011.11.003 doi: 10.1016/j.asoc.2011.11.003
![]() |
[16] |
J.-Y. Huang, Intuitionistic fuzzy Hamacher aggregation operators and their application to multiple attribute decision making, J. Intell. Fuzzy Syst., 27 (2014), 505–513. https://doi.org/10.3233/IFS-131019 doi: 10.3233/IFS-131019
![]() |
[17] |
R. Verma, Generalized Bonferroni mean operator for fuzzy number intuitionistic fuzzy sets and its application to multiattribute decision making, Int. J. Intell. Syst., 30 (2015), 499–519. https://doi.org/10.1002/int.21705 doi: 10.1002/int.21705
![]() |
[18] |
T. Senapati, G. Chen, R. R. Yager, Aczel–Alsina aggregation operators and their application to intuitionistic fuzzy multiple attribute decision making, Int. J. Intell. Syst., 37 (2022), 1529–1551. https://doi.org/10.1002/int.22684 doi: 10.1002/int.22684
![]() |
[19] | R. R. Yager, Pythagorean fuzzy subsets, In: 2013 joint IFSA world congress and NAFIPS annual meeting (IFSA/NAFIPS), Edmonton, AB, Canada, 2013, 57–61. https://doi.org/10.1109/IFSA-NAFIPS.2013.6608375 |
[20] |
R. R. Yager, A. M. Abbasov, Pythagorean membership grades, complex numbers, and decision making, Int. J. Intell. Syst., 28 (2013), 436–452. https://doi.org/10.1002/int.21584 doi: 10.1002/int.21584
![]() |
[21] |
X. Zhang, Z. Xu, Extension of TOPSIS to multiple criteria decision making with Pythagorean fuzzy sets, Int. J. Intell. Syst., 29 (2014), 1061–1078. https://doi.org/10.1002/int.21676 doi: 10.1002/int.21676
![]() |
[22] |
R. R. Yager, Pythagorean membership grades in multicriteria decision making, IEEE Trans. Fuzzy Syst., 22 (2013), 958–965. https://doi.org/10.1109/TFUZZ.2013.2278989 doi: 10.1109/TFUZZ.2013.2278989
![]() |
[23] |
X. Zhang, A novel approach based on similarity measure for Pythagorean fuzzy multiple criteria group decision making, Int. J. Intell. Syst., 31 (2016), 593–611. https://doi.org/10.1002/int.21796 doi: 10.1002/int.21796
![]() |
[24] |
X. Peng, H. Yuan, Fundamental properties of Pythagorean fuzzy aggregation operators, Fund. Inform., 147 (2016), 415–446. https://doi.org/10.3233/FI-2016-1415 doi: 10.3233/FI-2016-1415
![]() |
[25] |
G. Wei, Pythagorean fuzzy interaction aggregation operators and their application to multiple attribute decision making, J. Intell. Fuzzy Syst., 33 (2017), 2119–2132. https://doi.org/10.3233/JIFS-162030 doi: 10.3233/JIFS-162030
![]() |
[26] |
S. Zeng, J. Chen, X. Li, A hybrid method for Pythagorean fuzzy multiple-criteria decision making, Int. J. Inf. Tech. Decis., 15 (2016), 403–422. https://doi.org/10.1142/S0219622016500012 doi: 10.1142/S0219622016500012
![]() |
[27] |
X. Peng, Y. Yang, Some results for Pythagorean fuzzy sets, Int. J. Intell. Syst., 30 (2015), 1133–1160. https://doi.org/10.1002/int.21738 doi: 10.1002/int.21738
![]() |
[28] |
X. Peng, Y Yang, Pythagorean fuzzy Choquet integral based MABAC method for multiple attribute group decision making, Int. J. Intell. Syst., 31 (2016), 989–1020. https://doi.org/10.1002/int.21814 doi: 10.1002/int.21814
![]() |
[29] |
S. Zeng, Pythagorean fuzzy multiattribute group decision making with probabilistic information and OWA approach, Int. J. Intell. Syst., 32 (2017), 1136–1150. https://doi.org/10.1002/int.21886 doi: 10.1002/int.21886
![]() |
[30] |
H. Garg, Generalised Pythagorean fuzzy geometric interactive aggregation operators using Einstein operations and their application to decision making, J. Exp. Theor. Artif. Intell., 30 (2018), 763–794. https://doi.org/10.1080/0952813X.2018.1467497 doi: 10.1080/0952813X.2018.1467497
![]() |
[31] |
H. Garg, Some methods for strategic decision‐making problems with immediate probabilities in Pythagorean fuzzy environment, Int. J. Intell. Syst., 33 (2018), 687–712. https://doi.org/10.1002/int.21949 doi: 10.1002/int.21949
![]() |
[32] |
H. Garg, Linguistic Pythagorean fuzzy sets and its applications in multiattribute decision‐making process, Int. J. Intell. Syst., 33 (2018), 1234–1263. https://doi.org/10.1002/int.21979 doi: 10.1002/int.21979
![]() |
[33] |
D. Liang, Y. Zhang, Z. Xu, A. P. Darko, Pythagorean fuzzy Bonferroni mean aggregation operator and its accelerative calculating algorithm with the multithreading, Int. J. Int. Syst., 33 (2018), 615–633. https://doi.org/10.1002/int.21960 doi: 10.1002/int.21960
![]() |
[34] |
S. Naz, S. Ashraf, M. Akram, A novel approach to decision-making with Pythagorean fuzzy information, Mathematics, 6 (2018), 95. https://doi.org/10.3390/math6060095 doi: 10.3390/math6060095
![]() |
[35] |
M. Akram, A. Habib, F. Ilyas, J. M. Dar, Specific types of Pythagorean fuzzy graphs and application to decision-making, Math. Comput. Appl., 23 (2018), 42. https://doi.org/10.3390/mca23030042 doi: 10.3390/mca23030042
![]() |
[36] |
M. Akram, J. M. Dar, A. Farooq, Planar graphs under Pythagorean fuzzy environment, Mathematics, 6 (2018), 278. https://doi.org/10.3390/math6120278 doi: 10.3390/math6120278
![]() |
[37] |
M. Akram, J. M. Dar, S. Naz, Certain graphs under Pythagorean fuzzy environment, Complex Intell. Syst., 5 (2019), 127–144. https://doi.org/10.1007/s40747-018-0089-5 doi: 10.1007/s40747-018-0089-5
![]() |
[38] |
Z. Xu, R. R. Yager, Dynamic intuitionistic fuzzy multi-attribute decision making, Int. J. Approx. Reason., 48 (2008), 246–262. https://doi.org/10.1016/j.ijar.2007.08.008 doi: 10.1016/j.ijar.2007.08.008
![]() |
[39] |
G. W. Wei, Some geometric aggregation functions and their application to dynamic multiple attribute decision making in the intuitionistic fuzzy setting, Int. J. Uncertain. Fuzz., 17 (2009), 179–196. https://doi.org/10.1142/S0218488509005802 doi: 10.1142/S0218488509005802
![]() |
[40] |
J. Zhou, W. Su, T. Baležentis, D. Streimikiene, Multiple criteria group decision-making considering symmetry with regards to the positive and negative ideal solutions via the Pythagorean normal cloud model for application to economic decisions, Symmetry, 10 (2018), 140. https://doi.org/10.3390/sym10050140 doi: 10.3390/sym10050140
![]() |
[41] |
C. Huang, M. Lin, Z. Xu, Pythagorean fuzzy MULTIMOORA method based on distance measure and score function: its application in multicriteria decision making process, Knowl. Inf. Syst., 62 (2020), 4373–4406. https://doi.org/10.1007/s10115-020-01491-y doi: 10.1007/s10115-020-01491-y
![]() |
[42] |
M. Lin, C. Huang, R. Chen, H. Fujita, X. Wang, Directional correlation coefficient measures for Pythagorean fuzzy sets: their applications to medical diagnosis and cluster analysis, Complex Intell. Syst., 7 (2021), 1025–1043. https://doi.org/10.1007/s40747-020-00261-1 doi: 10.1007/s40747-020-00261-1
![]() |
[43] |
Z. Zhang, Y. Li, X. Wang, Y. Liu, W. Tang, W. Ding, et al., Investigating river health across mountain to urban transitions using Pythagorean fuzzy cloud technique under uncertain environment, J. Hydrol., 620 (2023), 129426. https://doi.org/10.1016/j.jhydrol.2023.129426 doi: 10.1016/j.jhydrol.2023.129426
![]() |
[44] |
G. Alhamzi, S. Javaid, U. Shuaib, A. Razaq, H. Garg, A. Razzaque, Enhancing interval-valued Pythagorean fuzzy decision-making through Dombi-based aggregation operators, Symmetry, 15 (2023), 765. https://doi.org/10.3390/sym15030765 doi: 10.3390/sym15030765
![]() |
[45] |
K. Rahman, S. Abdullah, A. Ali, F. Amin, Pythagorean fuzzy ordered weighted averaging aggregation operator and their application to multiple attribute group decision-making, EURO J. Decis. Process., 8 (2020), 61–77. https://doi.org/10.1007/s40070-020-00110-z doi: 10.1007/s40070-020-00110-z
![]() |
[46] |
T. Sun, J. Lv, X. Zhao, W. Li, Z. Zhang, L. Nie, In vivo liver function reserve assessments in alcoholic liver disease by scalable photoacoustic imaging, Photoacoustics, 34 (2023), 100569. https://doi.org/10.1016/j.pacs.2023.100569 doi: 10.1016/j.pacs.2023.100569
![]() |
[47] |
Y. Huang, C. Wang, T. Zhou, F. Xie, Z. Liu, H. Xu, et al., Lumican promotes calcific aortic valve disease through H3 histone lactylation, Eur. Heart J., 45 (2024), 3871–3885. https://doi.org/10.1093/eurheartj/ehae407 doi: 10.1093/eurheartj/ehae407
![]() |
[48] |
P.-C. Fu, J.-Y. Wang, Y. Su, Y.-Q. Liao, S.-L. Li, G.-L. Xu, et al., Intravascular ultrasonography assisted carotid artery stenting for treatment of carotid stenosis: two case reports, World J. Clin. Cases, 11 (2023), 7127–7135. https://doi.org/10.12998/wjcc.v11.i29.7127 doi: 10.12998/wjcc.v11.i29.7127
![]() |
[49] |
Y. Zhao, W. Xiong, C. Li, R. Zhao, H. Lu, S. Song, et al., Hypoxia-induced signaling in the cardiovascular system: pathogenesis and therapeutic targets, Sig. Transduct. Target. Ther., 8 (2023), 431. https://doi.org/10.1038/s41392-023-01652-9 doi: 10.1038/s41392-023-01652-9
![]() |
[50] |
P. Bing, Y. Liu, W. Liu, J. Zhou, L. Zhu, Electrocardiogram classification using TSST-based spectrogram and ConViT, Front. Cardiovasc. Med., 9 (2022), 983543. https://doi.org/10.3389/fcvm.2022.983543 doi: 10.3389/fcvm.2022.983543
![]() |
[51] | K. Rahman, S. Abdullah, F. Husain, M. A. Khan, M. Shakeel, Pythagorean fuzzy ordered weighted geometric aggregation operator and their application to multiple attribute group decision making, J. Appl. Environ. Biol. Sci., 7 (2017), 67–83. |
Ջ1 | Ջ2 | Ջ3 | Ջ4 | |
Ȳ1 | (0.8, 0.3) | (0.4, 0.2) | (0.9, 0.2) | (0.5, 0.6) |
Ȳ2 | (0.6, 0.5) | (0.7, 0.3) | (0.4, 0.6) | (0.5, 0.4) |
Ȳ3 | (0.5, 0.5) | (0.8, 0.3) | (0.6, 0.3) | (0.5, 0.6) |
Ȳ4 | (0.9, 0.2) | (0.6, 0.5) | (0.7, 0.3) | (0.4, 0.5) |
Ȳ5 | (0.3, 0.7) | (0.5, 0.4) | (0.8, 0.2) | (0.6, 0.5) |
Ջ1 | Ջ2 | Ջ3 | Ջ4 | |
Ȳ1 | (0.6, 0.3) | (0.5, 0.3) | (0.8, 0.3) | (0.7, 0.2) |
Ȳ2 | (0.7, 0.3) | (0.8, 0.2) | (0.5, 0.4) | (0.6, 0.5) |
Ȳ3 | (0.9, 0.2) | (0.6, 0.5) | (0.7, 0.3) | (0.4, 0.5) |
Ȳ4 | (0.5, 0.5) | (0.8, 0.3) | (0.5, 0.6) | (0.6, 0.3) |
Ȳ5 | (0.4, 0.5) | (0.5, 0.3) | (0.6, 0.3) | (0.7, 0.4) |
Ջ1 | Ջ2 | Ջ3 | Ջ4 | |
Ȳ1 | (0.6, 0.3) | (0.5, 0.2) | (0.7, 0.4) | (0.3, 0.4) |
Ȳ2 | (0.4, 0.5) | (0.6, 0.5) | (0.7, 0.3) | (0.9, 0.2) |
Ȳ3 | (0.6, 0.5) | (0.3, 0.7) | (0.5, 0.4) | (0.8, 0.2) |
Ȳ4 | (0.7, 0.4) | (0.5, 0.3) | (0.4, 0.5) | (0.6, 0.3) |
Ȳ5 | (0.7, 0.4) | (0.7, 0.3) | (0.6, 0.5) | (0.4, 0.8) |
Ջ1 | Ջ2 | Ջ3 | Ջ4 | |
Ȳ1 | (0.9, 0.2) | (0.8, 0.3) | (0.4, 0.2) | (0.5, 0.6) |
Ȳ2 | (0.7, 0.3) | (0.6, 0.5) | (0.5, 0.4) | (0.4, 0.6) |
Ȳ3 | (0.8, 0.3) | (0.6, 0.3) | (0.5, 0.5) | (0.5, 0.6) |
Ȳ4 | (0.9, 0.2) | (0.7, 0.3) | (0.6, 0.5) | (0.4, 0.5) |
Ȳ5 | (0.8, 0.2) | (0.6, 0.5) | (0.5, 0.4) | (0.3, 0.7) |
Ջ1 | Ջ2 | Ջ3 | Ջ4 | |
Ȳ1 | (0.8, 0.3) | (0.7, 0.2) | (0.6, 0.3) | (0.5, 0.3) |
Ȳ2 | (0.8, 0.2) | (0.7, 0.3) | (0.6, 0.5) | (0.5, 0.4) |
Ȳ3 | (0.9, 0.2) | (0.7, 0.3) | (0.6, 0.5) | (0.4, 0.5) |
Ȳ4 | (0.8, 0.3) | (0.6, 0.3) | (0.5, 0.5) | (0.5, 0.6) |
Ȳ5 | (0.7, 0.4) | (0.6, 0.3) | (0.5, 0.3) | (0.4, 0.5) |
Ջ1 | Ջ2 | Ջ3 | Ջ4 | |
Ȳ1 | (0.7, 0.4) | (0.6, 0.3) | (0.5, 0.2) | (0.3, 0.5) |
Ȳ2 | (0.9, 0.2) | (0.7, 0.3) | (0.6, 0.5) | (0.4, 0.5) |
Ȳ3 | (0.8, 0.2) | (0.6, 0.5) | (0.5, 0.4) | (0.3, 0.7) |
Ȳ4 | (0.7, 0.4) | (0.6, 0.3) | (0.5, 0.3) | (0.4, 0.5) |
Ȳ5 | (0.7, 0.3) | (0.7, 0.4) | (0.6, 0.5) | (0.4, 0.8) |
Ջ1 | Ջ2 | Ջ3 | Ջ4 | |
Ȳ1 | (0.78, 0.32) | (0.68, 0.27) | (0.52, 0.23) | (0.42, 0.45) |
Ȳ2 | (0.85, 0.22) | (0.68, 0.33) | (0.58, 0.48) | (0.43, 0.48) |
Ȳ3 | (0.84, 0.22) | (0.63, 0.39) | (0.53, 0.45) | (0.38, 0.61) |
Ȳ4 | (0.79, 0.32) | (0.62, 0.3) | (0.52, 0.39) | (0.43, 0.53) |
Ȳ5 | (0.72, 0.30) | (0.65, 0.38) | (0.55, 0.41) | (0.42, 0.68) |
Ei | |
Ȳ1 | (0.596, 0.278) |
Ȳ2 | (0.644, 0.402) |
Ȳ3 | (0.607, 0.419) |
Ȳ4 | (0.586, 0.385) |
Ȳ5 | (0.582, 0.426) |
Ջ1 | Ջ2 | Ջ3 | Ջ4 | |
Ȳ1 | (0.77, 0.34) | (0.67, 0.27) | (0.51, 0.24) | (0.39, 0.47) |
Ȳ2 | (0.83, 0.22) | (0.68, 0.35) | (0.58, 0.48) | (0.43, 0.50) |
Ȳ3 | (0.83, 0.22) | (0.63, 0.42) | (0.53, 0.45) | (0.36, 0.63) |
Ȳ4 | (0.77, 0.34) | (0.62, 0.3) | (0.52, 0.42) | (0.43, 0.53) |
Ȳ5 | (0.72, 0.32) | (0.64, 0.40) | (0.55, 0.43) | (0.38, 0.72) |
Ei | |
Ȳ1 | (0.534, 0.318) |
Ȳ2 | (0.593, 0.439) |
Ȳ3 | (0.541, 0.468) |
Ȳ4 | (0.547, 0.419) |
Ȳ5 | (0.547, 0.498) |
IFDWA [38] | IFDWG [39] | PFDOWA | PFDOWG | |
E(Ȳ1) | (0.688, 0.407) | (0.618, 0.326) | (0.596, 0.278) | (0.534, 0.318) |
E(Ȳ2) | (0.654, 0.378) | (0.597, 0.395) | (0.644, 0.402) | (0.593, 0.439) |
E(Ȳ3) | (0.592, 0.396) | (0.581, 0.464) | (0.607, 0.419) | (0.541, 0.468) |
E(Ȳ4) | (0.577, 0.405) | (0.535, 0.434) | (0.586, 0.385) | (0.547, 0.419) |
E(Ȳ5) | (0.615, 0.403) | (0.550, 0.422) | (0.582, 0.426) | (0.547, 0.498) |
S(E1) | S(E2) | S(E3) | S(E4) | S(E5) | Ranking order | |
IFDWA | 0.281 | 0.276 | 0.196 | 0.172 | 0.212 | Ȳ1>Ȳ2>Ȳ5>Ȳ3>Ȳ4 |
IFDWG | 0.292 | 0.202 | 0.117 | 0.101 | 0.128 | Ȳ1>Ȳ2>Ȳ5>Ȳ3>Ȳ4 |
PFDOWA | 0.277 | 0.253 | 0.193 | 0.195 | 0.157 | Ȳ1>Ȳ2>Ȳ4>Ȳ3>Ȳ5 |
PFDOWG | 0.184 | 0.159 | 0.074 | 0.124 | 0.051 | Ȳ1>Ȳ2>Ȳ4>Ȳ3>Ȳ5 |
Ջ1 | Ջ2 | Ջ3 | Ջ4 | |
Ȳ1 | (0.8, 0.3) | (0.4, 0.2) | (0.9, 0.2) | (0.5, 0.6) |
Ȳ2 | (0.6, 0.5) | (0.7, 0.3) | (0.4, 0.6) | (0.5, 0.4) |
Ȳ3 | (0.5, 0.5) | (0.8, 0.3) | (0.6, 0.3) | (0.5, 0.6) |
Ȳ4 | (0.9, 0.2) | (0.6, 0.5) | (0.7, 0.3) | (0.4, 0.5) |
Ȳ5 | (0.3, 0.7) | (0.5, 0.4) | (0.8, 0.2) | (0.6, 0.5) |
Ջ1 | Ջ2 | Ջ3 | Ջ4 | |
Ȳ1 | (0.6, 0.3) | (0.5, 0.3) | (0.8, 0.3) | (0.7, 0.2) |
Ȳ2 | (0.7, 0.3) | (0.8, 0.2) | (0.5, 0.4) | (0.6, 0.5) |
Ȳ3 | (0.9, 0.2) | (0.6, 0.5) | (0.7, 0.3) | (0.4, 0.5) |
Ȳ4 | (0.5, 0.5) | (0.8, 0.3) | (0.5, 0.6) | (0.6, 0.3) |
Ȳ5 | (0.4, 0.5) | (0.5, 0.3) | (0.6, 0.3) | (0.7, 0.4) |
Ջ1 | Ջ2 | Ջ3 | Ջ4 | |
Ȳ1 | (0.6, 0.3) | (0.5, 0.2) | (0.7, 0.4) | (0.3, 0.4) |
Ȳ2 | (0.4, 0.5) | (0.6, 0.5) | (0.7, 0.3) | (0.9, 0.2) |
Ȳ3 | (0.6, 0.5) | (0.3, 0.7) | (0.5, 0.4) | (0.8, 0.2) |
Ȳ4 | (0.7, 0.4) | (0.5, 0.3) | (0.4, 0.5) | (0.6, 0.3) |
Ȳ5 | (0.7, 0.4) | (0.7, 0.3) | (0.6, 0.5) | (0.4, 0.8) |
Ջ1 | Ջ2 | Ջ3 | Ջ4 | |
Ȳ1 | (0.9, 0.2) | (0.8, 0.3) | (0.4, 0.2) | (0.5, 0.6) |
Ȳ2 | (0.7, 0.3) | (0.6, 0.5) | (0.5, 0.4) | (0.4, 0.6) |
Ȳ3 | (0.8, 0.3) | (0.6, 0.3) | (0.5, 0.5) | (0.5, 0.6) |
Ȳ4 | (0.9, 0.2) | (0.7, 0.3) | (0.6, 0.5) | (0.4, 0.5) |
Ȳ5 | (0.8, 0.2) | (0.6, 0.5) | (0.5, 0.4) | (0.3, 0.7) |
Ջ1 | Ջ2 | Ջ3 | Ջ4 | |
Ȳ1 | (0.8, 0.3) | (0.7, 0.2) | (0.6, 0.3) | (0.5, 0.3) |
Ȳ2 | (0.8, 0.2) | (0.7, 0.3) | (0.6, 0.5) | (0.5, 0.4) |
Ȳ3 | (0.9, 0.2) | (0.7, 0.3) | (0.6, 0.5) | (0.4, 0.5) |
Ȳ4 | (0.8, 0.3) | (0.6, 0.3) | (0.5, 0.5) | (0.5, 0.6) |
Ȳ5 | (0.7, 0.4) | (0.6, 0.3) | (0.5, 0.3) | (0.4, 0.5) |
Ջ1 | Ջ2 | Ջ3 | Ջ4 | |
Ȳ1 | (0.7, 0.4) | (0.6, 0.3) | (0.5, 0.2) | (0.3, 0.5) |
Ȳ2 | (0.9, 0.2) | (0.7, 0.3) | (0.6, 0.5) | (0.4, 0.5) |
Ȳ3 | (0.8, 0.2) | (0.6, 0.5) | (0.5, 0.4) | (0.3, 0.7) |
Ȳ4 | (0.7, 0.4) | (0.6, 0.3) | (0.5, 0.3) | (0.4, 0.5) |
Ȳ5 | (0.7, 0.3) | (0.7, 0.4) | (0.6, 0.5) | (0.4, 0.8) |
Ջ1 | Ջ2 | Ջ3 | Ջ4 | |
Ȳ1 | (0.78, 0.32) | (0.68, 0.27) | (0.52, 0.23) | (0.42, 0.45) |
Ȳ2 | (0.85, 0.22) | (0.68, 0.33) | (0.58, 0.48) | (0.43, 0.48) |
Ȳ3 | (0.84, 0.22) | (0.63, 0.39) | (0.53, 0.45) | (0.38, 0.61) |
Ȳ4 | (0.79, 0.32) | (0.62, 0.3) | (0.52, 0.39) | (0.43, 0.53) |
Ȳ5 | (0.72, 0.30) | (0.65, 0.38) | (0.55, 0.41) | (0.42, 0.68) |
Ei | |
Ȳ1 | (0.596, 0.278) |
Ȳ2 | (0.644, 0.402) |
Ȳ3 | (0.607, 0.419) |
Ȳ4 | (0.586, 0.385) |
Ȳ5 | (0.582, 0.426) |
Ջ1 | Ջ2 | Ջ3 | Ջ4 | |
Ȳ1 | (0.77, 0.34) | (0.67, 0.27) | (0.51, 0.24) | (0.39, 0.47) |
Ȳ2 | (0.83, 0.22) | (0.68, 0.35) | (0.58, 0.48) | (0.43, 0.50) |
Ȳ3 | (0.83, 0.22) | (0.63, 0.42) | (0.53, 0.45) | (0.36, 0.63) |
Ȳ4 | (0.77, 0.34) | (0.62, 0.3) | (0.52, 0.42) | (0.43, 0.53) |
Ȳ5 | (0.72, 0.32) | (0.64, 0.40) | (0.55, 0.43) | (0.38, 0.72) |
Ei | |
Ȳ1 | (0.534, 0.318) |
Ȳ2 | (0.593, 0.439) |
Ȳ3 | (0.541, 0.468) |
Ȳ4 | (0.547, 0.419) |
Ȳ5 | (0.547, 0.498) |
IFDWA [38] | IFDWG [39] | PFDOWA | PFDOWG | |
E(Ȳ1) | (0.688, 0.407) | (0.618, 0.326) | (0.596, 0.278) | (0.534, 0.318) |
E(Ȳ2) | (0.654, 0.378) | (0.597, 0.395) | (0.644, 0.402) | (0.593, 0.439) |
E(Ȳ3) | (0.592, 0.396) | (0.581, 0.464) | (0.607, 0.419) | (0.541, 0.468) |
E(Ȳ4) | (0.577, 0.405) | (0.535, 0.434) | (0.586, 0.385) | (0.547, 0.419) |
E(Ȳ5) | (0.615, 0.403) | (0.550, 0.422) | (0.582, 0.426) | (0.547, 0.498) |
S(E1) | S(E2) | S(E3) | S(E4) | S(E5) | Ranking order | |
IFDWA | 0.281 | 0.276 | 0.196 | 0.172 | 0.212 | Ȳ1>Ȳ2>Ȳ5>Ȳ3>Ȳ4 |
IFDWG | 0.292 | 0.202 | 0.117 | 0.101 | 0.128 | Ȳ1>Ȳ2>Ȳ5>Ȳ3>Ȳ4 |
PFDOWA | 0.277 | 0.253 | 0.193 | 0.195 | 0.157 | Ȳ1>Ȳ2>Ȳ4>Ȳ3>Ȳ5 |
PFDOWG | 0.184 | 0.159 | 0.074 | 0.124 | 0.051 | Ȳ1>Ȳ2>Ȳ4>Ȳ3>Ȳ5 |