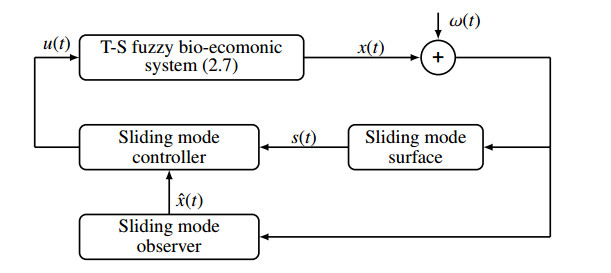
Optimization techniques can be used to find the optimal combination of inputs and parameters and help identify the most efficient solution. Aggregation operators (AOs) play a prominent role in discernment between two circulations of prospect and pull out anxieties from that insight. The most fundamental objective of this research is to extend the interaction AOs to the interval-valued Pythagorean fuzzy hypersoft set (IVPFHSS), the comprehensive system of the interval-valued Pythagorean fuzzy soft set (IVPFSS). The IVPFHSS adroitly contracts with defective and ambagious facts compared to the prevalent Pythagorean fuzzy soft set and interval-valued intuitionistic fuzzy hypersoft set (IVIFHSS). It is the dominant technique for enlarging imprecise information in decision-making (DM). The most important intention of this exploration is to intend interactional operational laws for IVPFHSNs. We extend the AOs to interaction AOs under IVPFHSS setting such as interval-valued Pythagorean fuzzy hypersoft interactive weighted average (IVPFHSIWA) and interval-valued Pythagorean fuzzy hypersoft interactive weighted geometric (IVPFHSIWG) operators. Also, we study the significant properties of the proposed operators, such as Idempotency, Boundedness, and Homogeneity. Still, the prevalent multi-criteria group decision-making (MCGDM) approaches consistently carry irreconcilable consequences. Meanwhile, our proposed MCGDM model is deliberate to accommodate these shortcomings. By utilizing a developed mathematical model and optimization technique, Industry 5.0 can achieve digital green innovation, enabling the development of sustainable processes that significantly decrease environmental impact. The impacts show that the intentional model is more operative and consistent in conducting inaccurate data based on IVPFHSS.
Citation: Nadia Khan, Sehrish Ayaz, Imran Siddique, Hijaz Ahmad, Sameh Askar, Rana Muhammad Zulqarnain. Sustainable practices to reduce environmental impact of industry using interaction aggregation operators under interval-valued Pythagorean fuzzy hypersoft set[J]. AIMS Mathematics, 2023, 8(6): 14644-14683. doi: 10.3934/math.2023750
[1] | Ismail Aydogdu . On double cyclic codes over Z2+uZ2. AIMS Mathematics, 2024, 9(5): 11076-11091. doi: 10.3934/math.2024543 |
[2] | Fatma Zehra Uzekmek, Elif Segah Oztas, Mehmet Ozen . (θi,λ)-constacyclic codes and DNA codes over Z4+uZ4+u2Z4. AIMS Mathematics, 2024, 9(10): 27908-27929. doi: 10.3934/math.20241355 |
[3] | Shakir Ali, Amal S. Alali, Kok Bin Wong, Elif Segah Oztas, Pushpendra Sharma . Cyclic codes over non-chain ring R(α1,α2,…,αs) and their applications to quantum and DNA codes. AIMS Mathematics, 2024, 9(3): 7396-7413. doi: 10.3934/math.2024358 |
[4] | Turki Alsuraiheed, Elif Segah Oztas, Shakir Ali, Merve Bulut Yilgor . Reversible codes and applications to DNA codes over F42t[u]/(u2−1). AIMS Mathematics, 2023, 8(11): 27762-27774. doi: 10.3934/math.20231421 |
[5] | Wei Qi . The polycyclic codes over the finite field Fq. AIMS Mathematics, 2024, 9(11): 29707-29717. doi: 10.3934/math.20241439 |
[6] | Sami Alabiad, Yousef Alkhamees . On classification of finite commutative chain rings. AIMS Mathematics, 2022, 7(2): 1742-1757. doi: 10.3934/math.2022100 |
[7] | Hongfeng Wu, Li Zhu . Repeated-root constacyclic codes of length p1pt2ps and their dual codes. AIMS Mathematics, 2023, 8(6): 12793-12818. doi: 10.3934/math.2023644 |
[8] | Shakir Ali, Amal S. Alali, Atif Ahmad Khan, Indah Emilia Wijayanti, Kok Bin Wong . XOR count and block circulant MDS matrices over finite commutative rings. AIMS Mathematics, 2024, 9(11): 30529-30547. doi: 10.3934/math.20241474 |
[9] | Yousef Alkhamees, Badr Alhajouj . Structure of a chain ring as a ring of matrices over a Galois ring. AIMS Mathematics, 2022, 7(9): 15824-15833. doi: 10.3934/math.2022866 |
[10] | Yang Zhang, Jizhu Nan . A note on the degree bounds of the invariant ring. AIMS Mathematics, 2024, 9(5): 10869-10881. doi: 10.3934/math.2024530 |
Optimization techniques can be used to find the optimal combination of inputs and parameters and help identify the most efficient solution. Aggregation operators (AOs) play a prominent role in discernment between two circulations of prospect and pull out anxieties from that insight. The most fundamental objective of this research is to extend the interaction AOs to the interval-valued Pythagorean fuzzy hypersoft set (IVPFHSS), the comprehensive system of the interval-valued Pythagorean fuzzy soft set (IVPFSS). The IVPFHSS adroitly contracts with defective and ambagious facts compared to the prevalent Pythagorean fuzzy soft set and interval-valued intuitionistic fuzzy hypersoft set (IVIFHSS). It is the dominant technique for enlarging imprecise information in decision-making (DM). The most important intention of this exploration is to intend interactional operational laws for IVPFHSNs. We extend the AOs to interaction AOs under IVPFHSS setting such as interval-valued Pythagorean fuzzy hypersoft interactive weighted average (IVPFHSIWA) and interval-valued Pythagorean fuzzy hypersoft interactive weighted geometric (IVPFHSIWG) operators. Also, we study the significant properties of the proposed operators, such as Idempotency, Boundedness, and Homogeneity. Still, the prevalent multi-criteria group decision-making (MCGDM) approaches consistently carry irreconcilable consequences. Meanwhile, our proposed MCGDM model is deliberate to accommodate these shortcomings. By utilizing a developed mathematical model and optimization technique, Industry 5.0 can achieve digital green innovation, enabling the development of sustainable processes that significantly decrease environmental impact. The impacts show that the intentional model is more operative and consistent in conducting inaccurate data based on IVPFHSS.
In recent years, human beings have been faced with the problem of resource shortage and environmental deterioration. Therefore, more and more people are interested in the analysis of predator-prey bio-economic systems. The authors proposed a singular bio-economic system with the invasion alien species in [1]. The stability and sliding mode control of a single bio-economic system was analyzed in [2]. The fuzzy stochastic optimal guaranteed cost control of bio-economic singular Markovian jump systems (MJSs) was studied in [3]. A singular bio-economic MJS with commodity prices as Markov chains was proposed in [4]. The exponential stability in mean square for MJSs was discussed in [31]. The problem of bifurcation and control for the biological economic system had been considered in [5]. The optimal harvest of the abstract population model with interval biological parameters was proposed in [6]. The optimal controller was designed by Pontryagin's maximum principle to obtain the optimal harvest. An elastic fuzzy controller was designed in [7] for a class of singular stochastic bio-economic fishery models with variable economic benefits. The fixed-time synchronization and energy consumption of Kuramoto-oscillator networks with multilayer distributed control are studied in [29]. Finite-time synchronization and H∞ synchronization of coupled complex-valued memristive neural networks (CCVMNNs) with or without parameter uncertainty are analyzed in [35]. In order to protect the ecological environment and ensure the synchronous development of economy, it is necessary to research the bio-economic systems.
Particularly, singular system models hold broader applicability in the realm of the bio-economic systems. In [8], a deterministic two-species predator-prey model with prey herd behavior, mutual disturbance and the effect of fear was introduced. In [9], the stability of a nonlinear population system with a weighted total size of scale structure and migration in a polluted environment was studied, where fertility and mortality depend on the density in different ways. Furthermore, the stability of biological and population systems was studied in [10]. It can be seen that the application of singular systems in the field of bioeconomy is worth exploring. In the biological system, it will be interfered by many noises, which can be divided into Gaussian noise or non Gaussian noise. Among the non Gaussian noises studied, white noise in stochastic systems is a special problem that needs to be studied. In [11], a new stochastic average method was used to analyze the impact vibration system with Gaussian white noise.
Sliding mode control, also known as variable structure control is a kind of special nonlinear control, and nonlinearity is expressed as control discontinuity. The advantage of sliding mode control mentioned in [12] is that it can overcome the uncertainty of the system, and has strong robustness to disturbance and unmodeled dynamics. In recent years, as we learned in [13,14,15,16], variable structure control had been successfully applied in various projects, such as robotics, aerospace, power systems and so on. In addition, variable structure control had achieved many results through fuzzy sliding mode control in [17]. The finite-time synchronization (FTS) of the prediction of the synchronization time and energy consumption is discussed for multilayer fractional-order networks (MFONs) in [33]. Therefore, we will continue to study variable structure control of the singular bio-economic systems.
In practice, biological systems are subject to fluctuations in the natural environment. Most of natural phenomena are not certain; they are stochastic. Therefore, it is very difficult to predict the dynamics of biological systems with deterministic methods. Therefore, the stochastic differential equation model is used to predict various dynamic analyses of biological systems to describe the behavior more in line with biological population activities. When existing stochastic factors and disturbance from white noise interfere, the model is changed from a biological model to a singular stochastic model. In [18], the influence of stochastic disturbance on a dynamic system was studied when white noise persists. In [19], the stability for stochastic singular systems with state-dependent noise in both continuous-time and discrete-time cases was discussed. The condition for the existence and uniqueness of the solution to stochastic singular systems is given. Furthermore, in [20], the stochastic Itˆo singular system is transformed into a deterministic standard singular system by means of the H-representation method and the new sufficient conditions for the stability of systems considered are derived in terms of strict linear matrix inequalities. In real life, the system state may be difficult to measure due to high cost or technical limitations. As an alternative method, state observers can overcome this difficulty. In [21], an observer-based nonlinear state delay system sliding mode controller was designed. H. Y. Li, et al. [22] studied observer-based adaptive sliding mode control for nonlinear MJSs. The observer based integral sliding mode control for stochastic systems was studied in [23] and the state observer was designed. The constraints of stochastic Itˆo regular systems were eliminated by designing linear sliding surfaces in [24]. In stochastic systems, the integral sliding mode control scheme requires very strict assumptions and we need to eliminate them. A new integral sliding mode control approach was proposed in [25]. The state of the system is difficult to obtain in practice. Inspired by control inputs and measurement outputs in [26], an observer is constructed to solve this problem in this paper.
The main contributions of this manuscript include:
(1) We first consider the capture of alien species and juvenile native species and we develop a more general exotic bioeconomic model of alien species invasion, taking into account the effects of stochastic environmental noise.
(2) For the error system (i.e., model(3.2)) and observer system, we design two ingenious integral sliding surfaces including the sliding mode observer (SMO) gain matrix and singular matrix. It more effectively handles various uncertainties and white noise in the system, thereby improving the robustness of the system.
(3) In this paper, we design a sliding mode controller, which is more suitable for biological systems to maintain the accessibility of sliding mode surfaces. A self-feedback term is innovatively introduced into the sliding variables to eliminate the restrictive assumptions often encountered in the sliding mode control of stochastic Ito systems.
Notations: For the Itô-type stochastic differential equation dζ=f(ζ,t)dt+g(ζ,t)dω where ζ∈Rn is the state, ω is the standard Brownian motion ∈Rn, and both f(ζ,t) and g(ζ,t) are locally Lipschitz in ζ and piecewise continuous in t. The differential operator L of V(ζ,t) is defined for the Itô-type stochastic system as LV(ζ,t)=∂V∂t+∂V∂ζf+12Tr{gT∂2V∂ζ2g}.
The following model is presented in [27]:
{˙x1(t)=αx2(t)−r1x1(t)−βx1(t)−ηx21(t)˙x2(t)=βx1(t)−r2x2(t), | (2.1) |
where x1(t) and x2(t) represent population density of immature species and mature species, respectively; α,r1,β denotes the intrinsic growth rate, death rate and transition rate of the immature population, respectively; r2 is the death rate of the mature population and the growth of the immature population is restricted by population density, which is reflected by −ηx21(t).
In the development of fishery, we mainly catch adult fish for profit and release young fish when we catch them. At the same time, we make the following assumptions:
Assumption 1. When the living conditions are the same, the population density of early alien species is not affected by the external environment.
Assumption 2. The competition between native species and alien species is far less than the internal competition of alien species.
Considering the impact of harvesting effort on a biological system, a singular bio-economic model was established in [28]:
{˙x1(t)=αx2(t)−r1x1(t)−βx1(t)−ηx21(t)−E(t)x1(t)˙x2(t)=βx1(t)−r2x2(t)0=E(t)(ρx1(t)−c)−m(t), | (2.2) |
where E(t) represents the harvesting effort of immature population, ρ represents the price coefficient of individual population, c represents the cost coefficient, cE(t) represents the total cost, and m(t) represents the net profit. Based on the system in [30], we can establish the following singular bio-economic model:
{˙x1(t)=αx2(t)−r1x1(t)−βx1(t)−ηx21(t)−E(t)x1(t)˙x2(t)=βx1(t)−r2x2(t)˙x3(t)=ax3(t)−hx4(t)−E(t)x3(t)˙x4(t)=β1x3(t)−θ1x2(t)−θ2x4(t)0=E(t)(ρ1x1(t)+ρ2x3(t)−c)−m(t), | (2.3) |
where x3(t) represents the population density of alien species at the time t. x4(t) represents its harvesting capacity. a represents the growth rate of alien invasive species. η represents the limiting rate of the growth of alien invasive species on the adult fish population. hx4(t) represents the purification amount of alien species. β1x3(t) represents the fixation purification of alien species. θ1 represents the impact of purification on adult fish populations. θ2x4(t) represents the cost of capturing alien invasive species. E(t)x3(t) represents the harvesting effort amount of alien species and ρ1 and ρ2 represent the price coefficient of x1(t) and x3(t) individual population. There are many stochastic factors in the real environment that interfere with the changes of immature species density and mature species density. Considering these factors, we introduce randomness into the model (2.3) by replacing the parameters r1,r2. First, suppose that the mortality of immature species r1 and mature species r2 will be affected by white noise, by r1→r1−α1ξ(t) and r2→r2−α2ξ(t). Second, it is assumed that the population density will also be directly affected by the external stochastic excitation ω(t). If the system is unstable, the expected performance can be achieved by controlling the capture of alien species. Therefore, we add u(t) to the fourth equation of system (2.3).
{˙x1(t)=αx2(t)−r1x1(t)−βx1(t)−ηx21(t)−E(t)x1(t)+α1x1(t)ξ(t)+x1(t)ω(t)˙x2(t)=βx1(t)−r2x2(t)+α2x2(t)ξ(t)˙x3(t)=ax3(t)−hx4(t)˙x4(t)=β1x3(t)−θ1x2(t)−θ2x4(t)+u(t)0=E(t)(ρx1(t)+ρ2x3(t)−c)−m(t), | (2.4) |
where α1, α2 represent the intensity of the white noises and ξ(t) and ω(t) are independent, in possession of the zero mean value and standard variance Gauss white noises. i.e., E[ω(t)]=0,E[ω(t)ω(t+τ)]=δ(τ), δ(x) represents the Dirac function.
Remark 1. Biological system modeling can not only use the Takagi and Sugeno (T-S) fuzzy method, but also use the adaptive T-S neural network method to deal with biological population problems. In particular, a novel hybrid photovoltaic maximum power point tracker structure based on an adaptive T-S fuzzy radial basis function neural network approach is proposed in [34]. Compared with the T-S fuzzy method, it has higher tracking efficiency and minimum tracking deviation, but it will make the system more complicated, which is not conducive to manual intervention in a biological system.
In this section, we use the T-S fuzzy method to express the nonlinear system as multiple local linear systems to make the analysis easier[32]. System (2.4) can be written as:
Edx(t)=[Ax(t)+BU(t)]dt+Jx(t)dw(t), | (2.5) |
where
A=[z1(t)α00−x1(t)βz2(t)00000a−h−x3(t)0−θ1β1−θ20z3(t)0z4(t)0−c],E=[1000001000001000001000000],J=[1000000000000000000000000],B=[000000000000000000100000−m(t)],U(t)=[111u(t)1]T,x(t)=[x1(t)x2(t)x3(t)x4(t)E(t)]T, |
where z1(t)=−r1−β−ηx1(t)+α1ξ(t), z2(t)=−x1(t), z3(t)=ρ1E(t), z4(t)=ρ2E(t), z5(t)=−x3(t). Thus,
maxz1(t)=−r1−β−ηminx1(t)+α1ξ(t),minz1(t)=−r1−β−ηmaxx1(t)+α1ξ(t),maxz2(t)=−minx1(t),minz2(t)=−maxx1(t),maxz3(t)=ρ1maxE(t),minz3(t)=ρ1minE(t),maxz4(t)=ρ2maxE(t),minz4(t)=ρ2minE(t),maxz5(t)=−minx3(t),minz5(t)=−maxx3(t). |
Using the maximum and minimum values, z1(t), z2(t), z3(t), z4(t) and z5(t) can be represented by
z1(t)=M11(z1(t))maxz1(t)+M12(z1(t))minz1(t),z2(t)=M21(z2(t))maxz2(t)+M22(z2(t))minz2(t),z3(t)=M31(z3(t))maxz3(t)+M32(z3(t))minz3(t),z4(t)=M41(z4(t))maxz4(t)+M42(z4(t))minz4(t),z5(t)=M51(z5(t))maxz5(t)+M52(z5(t))minz5(t), |
where Mi1+Mi2=1,i=1,2,3,4,5 and Mij represent the membership functions. Ai is the coefficient matrix of linear system after fuzzy and Bi is the control input matrix. The system (2.5) can be written as
Edx(t)=32∑i=1hi(z(t))[(Aix(t)+BiU(t))dt+Jx(t)dw(t)], | (2.6) |
where hi(z(t)) are the weight functions defined by
hi(z(t))=r∏j=1ωij(zj(t))g∑i=1r∏j=1ωij(zj(t)),hi(z(t))≥0,32∑i=1hi(z(t))=1. |
The ωij(zj(t)) is called the grade of membership funcition zj(t) in ωij.
For notational simplicity, 32∑i=1hi(z(t))Ai and 32∑i=1hi(z(t))Bi will be written as ˉA and ˉB, respectively. The T-S fuzzy stochastic singular bio-economic system (2.6) is shown as follows:
{Edx(t)=[ˉAx(t)+ˉBU(t)]dt+Jx(t)dω(t)y(t)=Cx(t). | (2.7) |
A schematic diagram of the T-S fuzzy bio-economic systems is shown in Figure 1.
In this paper, we mainly study the population density of alien species. However, in practice, due to various factors and the rapid reproduction of alien species, it is difficult to measure the real population density. Therefore, the design of the state observer usually provides an approximate estimate for the original system. However, it can be observed that since the sliding mode observer has a discontinuous control law, it can deal with the case where the population density is externally excited. Therefore, the observer (3.1) is proposed in this paper.
The functions are as follows:
{Edˆx(t)=[ˉAˆx(t)+ˉB(U(t)−Us(t))+G(y(t)−ˆy(t))]dtˆy(t)=Cˆx(t), | (3.1) |
where ˆx(t)∈Rn represents the state estimation of x(t), ˆy(t) represents the output vector of the observer, Us(t) represents the input vector of observer and G represents the observer gain to be designed in the sequel.
Define e(t)=x(t)−ˆx(t), which represents state estimation error. Subtracting system (3.1) from system (2.7), according to the systems (2.7) and (3.1), we can obtain (3.2) as follows:
Ede(t)=[(ˉA−GC)e(t)+ˉBUs(t)]dt+Jx(t)dw(t). | (3.2) |
We first construct the sliding surface for the error system (3.2). The sliding surface is obtained by making s(t)=0, where the sliding variable s(t) is defined as
s(t)=SEe(t)−SEe(t0)+∫tt0(SGCe(ν)−Qs(ν))dν, | (3.3) |
where S∈Rn×n is a known matrix satisfying det(SˉB)≠0 and rank[SEC]=rankC and G is the observer gain. Q is a known positive definite matrix used to adjust the convergence rate and eliminate the constraint SˉJ=0.
Remark 2. We limit rank[SEC]=rankC, so that there is a matrix H satisfying SE=HC, so the sliding variable s(t) can be converted into
s(t)=H(y(t)−ˆy(t))−H(y(0)−ˆy(0))+∫tt0(SG(y(ν)−ˆy(ν))−Qs(ν))dν, |
which is accessible.
Compared with the sliding variable without self feedback term, the advantages of the sliding variable (3.6) are mainly reflected in two points: First, the self-feedback term −Qs(t) allows an increase in the stability margin and makes the sliding variable more robust to disturbances beyond the sliding surface. Second, the self-feedback term is also responsible for eliminating the restrictive constraint SJ=0, stabilizing the sliding variable and reducing chattering. From (3.2) and (3.3), it follows that
ds(t)=SEde(t)+SGCe(t)−Qs(t)=S[(ˉA−GC)e(t)+ˉBUs(t)]+SJx(t)dw(t)+SGCe(t)−Qs(t)=S[ˉAe(t)+ˉBUs(t)]−Qs(t)+SJx(t)dw(t)=Ls(t)+SJx(t)dw(t). | (3.4) |
When the system is constrained on the sliding surface, we can get E{s(t)}=0, so from (3.4), we get Ls(t)=0. The equivalent control law can be given by Useq(t)=−(SˉB)−1SˉAe(t). Substituting Useq(t) into (3.2), the sliding mode dynamic can be obtained as
Ede(t)=(˜A−GC)e(t)dt+[Je(t)+Jˆx(t)]dw(t), | (3.5) |
where ˜A=(I−ˉB(SˉB)−1S)ˉA.
According to (3.4), the dynamics of sliding variable s(t) includes a product term −Qs(t), which involves a Hurwitz matrix −Q and sliding variable itself, which means that the term −Qs(t) contained in the sliding variable (3.3) has a stabilizing effect on the sliding variable. It can be seen that by introducing −Qs(t) into the sliding variable s(t) and selecting an appropriate positive definite matrix Q, the convergence speed of the sliding variable can be improved to a certain extent.
By using the state variables of the designed observer, the stability of the system is achieved, and the expected performance is achieved. Therefore, s0=0 can be used to design the observer for (3.1), where
s0(t)=S0Eˆx(t)−S0Eˆx(t0)−S0∫tt0(ˉA+ˉBK)ˆx(ν)dν | (3.6) |
and K is the parameter matrix, so that E,ˉA+ˉB are admissible and S0=ˉBTX∈Rm×n is the unknown matrix to be determined later.
From (3.4) and (3.6), it follows that
.s0(t)=S0E˙ˆx(t)−S0(ˉA+ˉBK)ˆx(t)=S0[ˉAˆx(t)+ˉB(U(t)−Us(t))+GCe(t)]−S0(ˉA+ˉBK)ˆx(t)=S0ˉB(U(t)−Us(t)−Kˆx(t))+S0GCe(t)=Ls0(t). | (3.7) |
The equivalent control law can be obtained
Ueq=Kˆx(t)+Us(t)−(S0ˉB)−1S0GCe(t). |
Further, substituting the equivalent control law into the observer (3.1), we can get the following equation
E˙ˆx(t)=(A+ˉBK)ˆx(t)+(I−ˉB(S0ˉB)−1S0)GCe(t). | (3.8) |
The dynamics of the resultant sliding motion are given
{E˙ˆx(t)=(A+ˉBK)ˆx(t)+(I−ˉB(S0ˉB)−1S0)GCe(t)E˙e(t)=(˜A−GC)e(t)+[Je(t)+Jˆx(t)]˙w(t) |
ˆA=[ˉA+ˉBKI−ˉB(S0B)−1S0GC0ˉA−GC]. |
In order to analyze the mean-square exponentially admissibility of (3.5) and (3.8) simultaneously, the SMO system (3.1) can track the original system (2.7) and the estimation state variables can converge to zero. Thus, we consider the following augmented system:
ˆE˙xe(t)=ˆAxe(t)dt+ˆJxe(t)˙w(t), | (3.9) |
where
ˆE=(E00E),xe(t)=[ˆx(t)e(t)],ˆJ=[00JJ]. | (3.10) |
Theorem 3.1. The closed-loop system (3.9) is mean-square exponentially admissible if there are matrices K,G,X and Y, and a positive scalar α such that the following inequalities hold:
ETX=XTE≥0 | (3.11) |
ETY=YTE≥0, | (3.12) |
[Λi+αJT(E+)TETYE+J−XT(ˉBK)−(ˉBK)TXαΦi]<0, | (3.13) |
where
Λi=(ˉA+ˉBK)TX+XT(ˉA+ˉBK)+JT(E+)TETYE+J. | (3.14) |
Φi=(ˉA−GC)TY+YT(ˉA−GC). | (3.15) |
Proof. First, we prove that system (3.9) is impulse free, regular and has a unique solution.
Let
ˆZ=[X00αY]. | (3.16) |
Using (3.10)–(3.12) and (3.16), we have the following equation
ˆETˆZ=[ETX00αETY]=[XTE00αYTE]=ˆZTˆE≥0. | (3.17) |
Singular invertible matrix results in
ˆE+=[E+00E+]. | (3.18) |
Calculated by (3.10) and (3.18),
ˆATˆZ+ˆZTˆA+ˆJT(ˆE+)TˆETˆZˆE+ˆJ=[Λi+αJT(E+)TETYE+J−XT(ˉBK)−(ˉBK)TXαΦi], | (3.19) |
where Λi and Φi are defined in (3.14) and (3.15). It can be seen from Eq (3.16) that the matrix (3.19) is negative definite; that is,
ˆATˆZ+ˆZTˆA+ˆJT(ˆE+)TˆETˆZˆE+ˆJ<0. | (3.20) |
There are non-singular matrixs M and N such that
MˆEN=[Ir000],MˆAN=[ˆA11ˆA12ˆA21ˆA22],MˆJN=[ˆJ11ˆJ1200],M−TˆZN=[ˆZ11ˆZ12ˆZ21ˆZ22],N−1xe(t)=[ˆx1(t)ˆx2(t)]. | (3.21) |
From (3.17), we can get NTˆETMTM−TˆZN=NTˆZTM−1MˆEN≥0; that is,
[Ir000][ˆZ11ˆZ12ˆZ21ˆZ22]=[ˆZ11TˆZ12TˆZ21TˆZ22T][Ir000]≥0. | (3.22) |
By (3.22), we get
ˆZ11=ˆZ11T,ˆZ12=0,M−TˆZN=[ˆZ110ˆZ21ˆZ22], | (3.23) |
then
NTˆETˆZN=(NTˆETMT)(M−TˆZN)=[ˆZ11000]. | (3.24) |
It follows that (3.20) implies
ˆATˆZ+ˆZTˆA<0. | (3.25) |
Moreover, pre-multiplying and post-multiplying Eq (3.25) by NT and N gives us
[Δ1Δ2∗ˆA22TˆZ22+ˆZ22TˆA22]<0. | (3.26) |
Since Δ1 and Δ2 are independent of the results discussed below, the actual expressions for these two variables are omitted. From (3.26), we have ˆA22TˆZ22+ˆZ22TˆA22<0, which makes it easy to find ˆA22 as nonsingular. Therefore, from Eq (3.10), we can obtain the constrained equivalent transformation form of (3.9).
{˙ˆx1(t)=(ˆA11−ˆA12ˆA22−1ˆA21)ˆx1(t)+(ˆJ11−ˆJ12ˆA22−1ˆA21)ˆx1(t)˙w(t)˙ˆx2(t)=−ˆA22−1ˆA21ˆx1(t). | (3.27) |
We get system (3.9) as regular and impulse-free. Based on the stochastic theory, the system (3.9) has a unique solution.
Next, we will prove that the system (3.9) is the mean-square exponential stability. Select the Lyapunov function candidate as V(xe(t),t)=xeT(t)ˆETˆZxe(t).
Let W(t)=eεtV(xe(t),t) and using (3.1), we can obtain
˙W(t)=εeεtV(xe(t),t)+eεtLV(xe(t),t)+2eεtxeT(t)ˆZTˆJxe(t). | (3.28) |
Integrating both sides and then taking expectation yields
E(W(t))=E(W(t0))+∫tt0εeενEV(xe(ν),ν)dν+∫tt0eενEV(xe(ν),ν)dν. | (3.29) |
By (2.3), we make
V(xe(t),t)=xTe(t)ˆETˆZxe(t)=ˆxT1(t)ˆZ11ˆx1(t), | (3.30) |
which means that
λmin(ˆZ11)‖ˆx1(t)‖2≤V(xe(t),t)≤λmax(ˆZ11)‖ˆx1(t)‖2. | (3.31) |
On the basis of (3.31), one can obtain
E(V(xe(t),t))≤λmax(ˆZ11)E‖ˆx1(t)‖2. | (3.32) |
E(W(t))=eεtEV(xe(t),t)≥λmin(ˆZ11)eεtE(‖ˆx1(t)‖2). | (3.33) |
In terms of ˆx2(t)=−ˆA22−1ˆA21ˆx1(t) given by (3.27), one can find positive scalars f1 and f2 such that
f1‖ˆx1(t)‖2≤‖ˆx2(t)‖2≤f2‖ˆx1(t)‖2. | (3.34) |
It is noted that
‖N−1xe(t)‖2=‖ˆx1(t)‖2+‖ˆx2(t)‖2. | (3.35) |
By (3.34) and (3.35), we have
(f1+1)‖ˆx1(t)‖2≤‖N−1xe(t)‖2≤(f2+1)‖ˆx1(t)‖2, | (3.36) |
which indicates
(f1+1)λmax(N−TN−1)‖ˆx1(t)‖2≤‖xe(t)‖2≤(f2+1)λmin(N−TN−1)‖ˆx1(t)‖2. | (3.37) |
From (3.2) and (3.20), we can find a positive scalar η so that
LV(xe(t),t)≤−η‖xe(t)‖2. | (3.38) |
Substituting (3.37) into (3.38) and then taking expectation, one has
E(LV(xe(t),t))≤−ϕE(‖ˆx1(t)‖2), | (3.39) |
where ϕ=η(f1+1)λmax(N−TN−1). Substituting (3.32), (3.33) and (3.39) into (3.29), one has
λmin(ˆZ11)eεtE‖ˆx1(t)‖2≤E(W(t0))+∫tt0(ελmax(ˆZ11)−ϕ)eενE‖ˆx1(ν)‖2dν. | (3.40) |
If ε is chosen as ε≤ϕλmax(ˆZ11), (3.40) becomes
λmin(ˆZ11)eεtE(‖ˆx1(t)‖2)≤E(W(t0)), | (3.41) |
which indicates
E(‖ˆx1(t)‖2)≤κe−εt, | (3.42) |
where κ=E(W(t0))λmin(ˆZ11). Using (3.34) and (3.42), one has
E(‖ˆx2(t)‖2)≤f2κe−εt. | (3.43) |
It can be seen that system (3.9) is mean-square exponentially admissible.
Theorem 3.2. Select the appropriate matrix Q>0, K and sliding mode controller
Us(t)=(SˉB)−1SˉAˆx(t)−ρ(SˉB)−1s(t)‖s(t)‖, | (3.44) |
where ρ=θ‖SˉA‖+∂. If (3.13) is feasible, then for all ‖x(t)‖≤θ, the sliding mode controller Us(t) given as above will guarantee that state trajectories of error system (3.2) are attracted to a small ball region around the sliding surface s(t)=0 from the beginning almost surely.
Proof. We can get
ds(t)=[S(ˉAe(t)+ˉBUs(t))−Qs(t)]dt+SJx(t)dw(t). | (3.45) |
Select the Lyapunov function as V(s(t))=12sT(t)s(t). According to the formula of Itˆo, it is as follows:
dV(s(t))=LV(s(t))dt+sT(t)SJx(t) dw(t), | (3.46) |
where
LV(s(t))=sT(t)[S(ˉAe(t)+ˉBUs(t))−Qs(t)]+12xT(t)JTSTSJx(t)=−ρ‖s(t)‖+sT(t)SˉAx(t)−sT(t)Qs(t)+12xT(t)JTSTSJx(t)≤−ρ‖s(t)‖+θ‖SˉA‖‖s(t)‖−λmin(Q)‖s(t)‖2+12λmax(JTSTSJ)‖x(t)‖2. | (3.47) |
The reachability condition LV(s(t))≤α1‖s(t)‖ is satisfied if the following holds
λmin(Q)‖s(t)‖2−(α1+θ‖SˉA‖−ρ)‖s(t)‖−12λmax(JTSTSJ)‖x(t)‖2≥0, | (3.48) |
where ρ≥α1+θ‖SˉA‖. It can be concluded that the solution of parabolic Eq (3.48) can be divided into two cases: One is that there is a unique solution ‖s(t)‖=0, and the other is that there are two different solutions
‖s(t)‖1=(α1−∂)2λmin(Q)−Ξ,‖s(t)‖2=(α1−∂)2λmin(Q)+Ξ, |
where Ξ=√(α1−∂)2+2λmin(Q)λmax(JTSTSJ)‖x(t)‖22λmin(Q), ‖s(t)‖=0 can be regarded as a special case of different solutions. Therefore, we only need to analyze the case of two different solutions. According to the parabolic equation in geometry, as long as ‖s(t)‖≥‖s(t)‖2 is met, (3.48) is true and ‖s(t)‖≤‖s(t)‖1 is discarded due to ‖s(t)‖≥0 and ‖s(t)‖1≤0.Thus, can get
LV(s(t))≤α1‖s(t)‖,∀‖s(t)‖≥(α1−∂)2λmin(Q)+Ξ. |
This means that the sliding variable s(t) is limited to a ball region
Υ(s(t),Q)={s(t)|‖s(t)‖≤(α1−∂)2λmin(Q)+Ξ} |
for the beginning almost surely. As a result, the almost sure attractiveness of ball region Υ(s(t),Q) is proved.
Theorem 3.3. Select the appropriate matrix Q>0, K and sliding mode controller
U(t)=((SˉB)−1SˉA+K)ˆx(t)−(S0ˉB)−1S0G(y(t)−ˆy(t))−ξ(S0ˉB)−1s0(t)‖s0(t)‖, | (3.49) |
where ξ=ρ‖(SˉB)−1‖‖S0ˉB‖+μ and ρ=θ‖SˉA‖+∂. If (3.12) is feasible, for all ‖x(t)‖≤θ, the sliding mode controller U(t) given above will ensure that the state track of the observer system (3.1) is almost certainly pulled to the sliding surface s0(t)=0 from the beginning.
Proof. We can get
˙s0(t)=S0ˉB(U(t)−Us(t)−Kˆx(t))+S0GCe(t). | (3.50) |
Select the Lyapunov function as V(s0(t))=12s0T(t)s0(t). According to the formula of Itˆo, we have
˙V(s0(t))=LV(s0(t)), | (3.51) |
where
LV(s0(t))=sT0(t)[S0ˉB(U(t)−Us(t)−Kˆx(t))]+sT0(t)S0GCe(t)=sT0(t)[S0ˉB(−ξ(S0ˉB)−1s0(t)‖s0(t)‖+ρ(SˉB)−1s(t)‖s(t)‖)]=−ξ‖s0(t)‖+ρsT0(t)S0ˉB(SˉB)−1s(t)‖s(t)‖≤−ξ‖s0(t)‖+ρ‖s(t)‖‖S0ˉB‖‖(SˉB)−1‖‖s0(t)‖≤−μ‖s0(t)‖. | (3.52) |
It can be concluded that the sliding variable s0(t) has been almost always on the sliding surface s0(t)=0 since the beginning.
Remark 3. In this paper, we add the product term −Qs(t) to the sliding variable s(t) in (3.3) to counteract the 12xT(t)JTSTSJx(t) caused by the presence of random noise. Therefore, it is no longer necessary to consider limiting SJ=0. In addition, when SJ=0 is met, the ideal sliding mode appears, and the ball area Y(s(t),Q) becomes the sliding surface s(t) itself. If this happens, the additional robustness of the self feedback item −Qs(t) will also make the proposed integral sliding variable s(t) better than the existing sliding variable.
Human activities have led to the destruction of the ecological environment of a large number of biological species. For instance, the ocean has been affected by land erosion, which has destroyed the spawning grounds and living environment of young fish. Environmental pollution has also had an increasingly serious impact on people's production and daily life. It is urgent to control and deal with the invasion of alien species.
In the economic globalization today, invasive species has increasingly become a global hot issue. Since the 1980s, alien species have shown a faster growth trend in China. Up to now, nearly 50 new invasive species have been added, and more than 20 dangerous invasive species have successively erupted in large areas in China.
We choose the following parameters according to the case of Nile tilapia:
α=0.4r1=0.5β=0.5η=0.1α1=0.1r2=0.1α2=0.1a=0.6h=0.01β1=0.4θ1=0.1θ2=0.2c=3ρ1=ρ2=4. |
We have:
{˙x1(t)=0.4x2(t)−0.5x1(t)−0.5x1(t)−0.1x21(t)−E(t)x1(t)+0.1x1(t)ξ(t)+x1(t)ω(t)˙x2(t)=0.5x1(t)−0.1x2(t)+0.6x2(t)ξ(t)˙x3(t)=0.6x3(t)−0.01x4(t)−E(t)x3(t)˙x4(t)=0.4x3(t)−0.1x2(t)−0.2x4(t)+u(t)0=E(t)(4x1(t)+4x3(t)−3)−m(t), |
where
x1(t)∈[−8,8],x2(t)∈[0,6],x3(t)∈[0,4],x4(t)∈[−6,0],E(t)∈[−6,2], |
then z1(t), z2(t), z3(t), z4(t) and z5(t) are obtained as follows:
maxz1(t)=−0.9,minz1(t)=−1.4maxz2(t)=0,minz2(t)=−10maxz3(t)=20,minz3(t)=0maxz4(t)=20,minz4(t)=0maxz5(t)=0,minz5(t)=−3. |
According to the above fuzzy rules given, the fuzzy model is carried out as (2.6). For the expression of A1, A2, ...A32, see the appendix. The other expression is as follows:
E=[1000001000001000001000000],Bi=[0000000000000000001000000],i=1,⋯32,Ji=[1000000000000000000000000],i=1,⋯32C=[−0.401−21020−10],M11=z1(t)+1.40.5,M12=−0.9−z1(t)0.5,M21=z2(t)+1010,M22=−z1(t)10,M31=z3(t)20,M32=20−z3(t)20. |
Set the initial conditions of system (2.6) and observer system to x(0)=[−7.930.8−7.8−4.9]T and ˆx(0)=[−7.930.8−7.8−4.9]T.
The state trajectory of the open loop system (2.6) can be clearly seen in Figure 2, which is not mean-square exponentially admissible. Next, in order to stabilize the system (2.6), a sliding mode control strategy is designed.
It can be obtained that
X=[0.6294−0.8049−0.6848−0.71620.31150.8116−0.44300.9412−0.1565−0.9286−0.74600.09380.91430.83150.69830.82680.9150−0.02920.58440.86800.26470.92980.60060.91900.3575],G=[5.15484.12094.8626−9.3633−2.1555−4.46153.1096−9.0766−6.5763−8.0574]×106. |
To design sliding mode control (SMC) laws Us(t) and U(t), take appropriate matrices
S=[−0.22.5−1.2−1.8−2.4],Q=2,K=[−1.6469−0.8775−0.9795−0.5521−0.9967−1.3897−0.7631−0.8912−1.3594−1.9195−0.6342−1.5310−1.2926−1.3102−0.6808−1.9004−1.5904−1.4187−0.3252−1.1705−0.0689−0.3737−1.5094−0.2380−0.4476] |
and θ=1.5,∂=0.001,μ=0.001. Figure 3 shows the trajectory of the closed-loop system, from which it can be found that the state variable of the closed-loop system can converge to zero in a very short time, that is, the observer is asymptotically stable. Figure 4 show the sliding mode surface, respectively. Therefore, the proposed observer and control scheme are effective. To reduce the chattering, by replacing s(t)‖s(t)‖ and s0(t)‖s0(t)‖ by s(t)‖s(t)‖+0.01 and s0(t)‖s0(t)‖+0.01, as can be seen from Figures 3–6, the simulation results have less chattering and faster convergence. It can be seen that the method of introducing self feedback −Qs(t) is improved compared with the existing method, which shows that the self feedback item can play a stable role. Therefore, it should be weighed when selecting Q.
In this paper, observer-based integral sliding mode control for singular bio-economic system with stochastic fluctuations is studied. A sliding mode observer is designed and an observer based integral sliding mode control method is proposed. By introducing a term consisting of a sliding variable and a negative definite matrix, a new integral sliding surface is constructed. From the results, it can be concluded that the newly introduced term can eliminate restrictive assumptions and has a stabilizing effect on sliding variables. Finally, an example is given to illustrate the effectiveness of the results obtained.
In biology, up to now, we still need to invest in a lot of manpower and material resources to control the density of alien species. The observer-based integral sliding mode control method proposed by us can effectively control the population density of alien invasive species to ensure that native species and invasive species can coexist within a certain range.
The authors declare they have not used Artificial Intelligence (AI) tools in the creation of this article.
The authors declare there is no conflict of interest in this paper.
A1=[−0.90.400−100.50000000.6−0.01−30−0.10.4−0.200000−3],A2=[−0.90.400−100.50000000.6−0.01−30−0.10.4−0.2000000],A3=[−0.90.400−100.50000000.6−0.0100−0.10.4−0.2000200−3],A4=[−0.90.400−100.50000000.6−0.0100−0.10.4−0.20002000],A5=[−0.90.400−100.50000000.6−0.0100−0.10.4−0.2020000−3],A6=[−0.90.400−100.50.9000000.6−0.0100−0.10.4−0.20200000],A7=[−0.90.400−100.50000000.6−0.0100−0.10.4−0.20200200−3],A8=[−0.90.400−100.50000000.6−0.0100−0.10.4−0.202002000],A9=[−0.90.40000.55000000.6−0.01−30−0.10.4−0.200000−3],A10=[−0.90.40000.55000000.6−0.01−30−0.10.4−0.2000000],A11=[−0.90.40000.55000000.6−0.0100−0.10.4−0.2000200−3],A12=[−0.90.40000.55000000.6−0.0100−0.10.4−0.20002000],A13=[−0.90.40000.55000000.6−0.0100−0.10.4−0.2020000−3],A14=[−0.90.40000.55000000.6−0.0100−0.10.4−0.20200000],A15=[−0.90.40000.55000000.6−0.0100−0.10.4−0.20200200−3],A16=[−0.90.40000.55000000.6−0.0100−0.10.4−0.202002000],A17=[−1.40.400−100.50000000.6−0.01−30−0.10.4−0.200000−3],A18=[−1.40.400−100.50000000.6−0.01−30−0.10.4−0.2000000],A19=[−1.40.400−100.50000000.6−0.0100−0.10.4−0.2000200−3],A20=[−1.40.400−100.50000000.6−0.0100−0.10.4−0.20002000],A21=[−1.40.400−100.50000000.6−0.0100−0.10.4−0.2020000−3],A22=[−1.40.400−100.50.9000000.6−0.0100−0.10.4−0.20200000],A23=[−1.40.400−100.50000000.6−0.0100−0.10.4−0.20200200−3],A24=[−1.40.400−100.50000000.6−0.0100−0.10.4−0.202002000],A25=[−1.40.40000.55000000.6−0.01−30−0.10.4−0.200000−3],A26=[−1.40.40000.55000000.6−0.01−30−0.10.4−0.2000000],A27=[−1.40.40000.55000000.6−0.0100−0.10.4−0.2000200−3],A28=[−1.40.40000.55000000.6−0.0100−0.10.4−0.20002000],A29=[−1.40.40000.55000000.6−0.0100−0.10.4−0.2020000−3],A30=[−1.40.40000.55000000.6−0.0100−0.10.4−0.20200000],A31=[−1.40.40000.55000000.6−0.0100−0.10.4−0.20200200−3],A32=[−1.40.40000.55000000.6−0.0100−0.10.4−0.202002000], |
[1] |
L. A. Zadeh, Fuzzy sets, Inform. Control, 8 (1965), 338–353. https://doi.org/10.1016/S0019-9958(65)90241-X doi: 10.1016/S0019-9958(65)90241-X
![]() |
[2] |
F. Xiao, CaFtR: A fuzzy complex event processing method, Int. J. Fuzzy Syst., 24 (2022), 1098–1111. https://doi.org/10.1007/s40815-021-01118-6 doi: 10.1007/s40815-021-01118-6
![]() |
[3] |
I. B. Turksen, Interval valued fuzzy sets based on normal forms, Fuzzy Set. Syst., 20 (1986), 191–210. https://doi.org/10.1016/0165-0114(86)90077-1 doi: 10.1016/0165-0114(86)90077-1
![]() |
[4] |
K. Atanassov, Intuitionistic fuzzy sets, Fuzzy Set.. Syst., 20 (1986), 87–96. https://doi.org/10.1016/S0165-0114(86)80034-3 doi: 10.1016/S0165-0114(86)80034-3
![]() |
[5] |
W. Wang, X. Liu, Intuitionistic fuzzy geometric aggregation operators based on Einstein operations, Int. J. Intell. Syst., 26 (2011), 1049–1075. https://doi.org/10.1002/int.20498 doi: 10.1002/int.20498
![]() |
[6] |
F. Xiao, A distance measure for intuitionistic fuzzy sets and its application to pattern classification problems, IEEE T. Syst. Man Cy.-S., 51 (2019), 3980–3992. https://doi.org/10.1109/TSMC.2019.2958635 doi: 10.1109/TSMC.2019.2958635
![]() |
[7] | K. T. Atanassov, Interval-valued intuitionistic fuzzy sets, In: Intuitionistic Fuzzy Sets, Physica, Heidelberg, 35 (1999). https://doi.org/10.1007/978-3-7908-1870-3_2 |
[8] |
F. Xiao, EFMCDM: Evidential fuzzy multicriteria decision making based on belief entropy, IEEE T. Fuzzy Syst., 28 (2019), 1477–1491. https://doi.org/10.1109/TFUZZ.2019.2936368 doi: 10.1109/TFUZZ.2019.2936368
![]() |
[9] |
R. R. Yager, Pythagorean membership grades in multi-criteria decision making, IEEE T. Fuzzy Syst., 22 (2013), 958–965. https://doi.org/10.1109/TFUZZ.2013.2278989 doi: 10.1109/TFUZZ.2013.2278989
![]() |
[10] |
M. J. Khan, M. I. Ali, P. Kumam, W. Kumam, M. Aslam, J. C. R. Alcantud, Improved generalized dissimilarity measure‐based VIKOR method for Pythagorean fuzzy sets, Int. J. Intell. Syst., 37 (2022), 1807–1845. https://doi.org/10.1002/int.22757 doi: 10.1002/int.22757
![]() |
[11] |
K. Rahman, S. Abdullah, R. Ahmed, M. Ullah, Pythagorean fuzzy Einstein weighted geometric aggregation operator and their application to multiple attribute group decision making, J. Intell. Fuzzy Syst., 33 (2017), 635–647. https://doi.org/10.3233/JIFS-16797 doi: 10.3233/JIFS-16797
![]() |
[12] |
C. Huang, M. Lin, Z. Xu, Pythagorean fuzzy MULTIMOORA method based on distance measure and score function: Its application in multicriteria decision making process, Knowl. Inform. Syst., 62 (2020), 4373–4406. https://doi.org/10.1007/s10115-020-01491-y doi: 10.1007/s10115-020-01491-y
![]() |
[13] |
X. Zhang, Z. Xu, Extension of TOPSIS to multiple criteria decision making with Pythagorean fuzzy sets, Int. J. Intell. Syst., 29 (2014), 1061–1078. https://doi.org/10.1002/int.21676 doi: 10.1002/int.21676
![]() |
[14] |
M. Lin, C. Huang, R. Chen, H. Fujita, X. Wang, Directional correlation coefficient measures for Pythagorean fuzzy sets: Their applications to medical diagnosis and cluster analysis, Complex Intell. Syst., 7 (2021), 1025–1043. https://doi.org/10.1007/s40747-020-00261-1 doi: 10.1007/s40747-020-00261-1
![]() |
[15] |
G. Wei, M. Lu, Pythagorean fuzzy power aggregation operators in multiple attribute decision making, Int. J. Intell. Syst., 33 (2018), 169–186. https://doi.org/10.1002/int.21946 doi: 10.1002/int.21946
![]() |
[16] |
M. Akram, K. Zahid, J.C. R. Alcantud, A new outranking method for multicriteria decision making with complex Pythagorean fuzzy information, Neural Comput. Appl., 34 (2022), 8069–8102. https://doi.org/10.1007/s00521-021-06847-1 doi: 10.1007/s00521-021-06847-1
![]() |
[17] |
F. Xiao, W. Ding, Divergence measure of Pythagorean fuzzy sets and its application in medical diagnosis, Appl. Soft Comput., 79 (2019), 254–267. https://doi.org/10.1016/j.asoc.2019.03.043 doi: 10.1016/j.asoc.2019.03.043
![]() |
[18] |
L. Wang, N. Li, Pythagorean fuzzy interaction power Bonferroni means aggregation operators in multiple attribute decision making, Int. J. Intell. Syst., 35 (2020), 150–183. https://doi.org/10.1002/int.22204 doi: 10.1002/int.22204
![]() |
[19] |
M. Lin, J. Wei, Z. Xu, R. Chen, Multiattribute group decision-making based on linguistic Pythagorean fuzzy interaction partitioned bonferroni mean aggregation operators, Complexity, 2018 (2018). https://doi.org/10.1155/2018/9531064 doi: 10.1155/2018/9531064
![]() |
[20] |
M. R. Khan, K. Ullah, Q. Khan, Multi-attribute decision-making using Archimedean aggregation operator in T-spherical fuzzy environment, Rep. Mech. Eng., 4 (2023), 18–38. https://doi.org/10.31181/rme20031012023k doi: 10.31181/rme20031012023k
![]() |
[21] |
M. Akram, F. Wasim, J. C. R. Alcantud, A. N. Al-Kenani, Multi-criteria optimization technique with complex Pythagorean fuzzy n-soft information, Int. J. Comput. Intell. Syst., 14 (2021), 1–24. https://doi.org/10.1007/s44196-021-00008-x doi: 10.1007/s44196-021-00008-x
![]() |
[22] |
X. Zhang, A novel approach based on similarity measure for Pythagorean fuzzy multiple criteria group decision making, Int. J. Intell. Syst., 31 (2016), 593–611. https://doi.org/10.1002/int.21796 doi: 10.1002/int.21796
![]() |
[23] |
M. Riaz, H. M. A. Farid, Picture fuzzy aggregation approach with application to third-party logistic provider selection process, Rep. Mech. Eng., 3 (2022), 227–236. https://doi.org/10.31181/rme20023062022r doi: 10.31181/rme20023062022r
![]() |
[24] |
M. Lin, X. Li, R. Chen, H. Fujita, J. Lin, Picture fuzzy interactional partitioned Heronian mean aggregation operators: an application to MADM process, Artif. Intell. Rev., 55 (2022), 1171–1208. https://doi.org/10.1007/s10462-021-09953-7 doi: 10.1007/s10462-021-09953-7
![]() |
[25] |
X. Peng, Y. Yang, Fundamental properties of interval‐valued Pythagorean fuzzy aggregation operators, Int. J. Intell. Syst., 31 (2016), 444–487. https://doi.org/10.1002/int.21790 doi: 10.1002/int.21790
![]() |
[26] |
M. Lin, C. Huang, Z. Xu, R. Chen, Evaluating IoT platforms using integrated probabilistic linguistic MCDM method, IEEE Internet Things, 7 (2020), 11195–11208. https://doi.org/10.1109/JIOT.2020.2997133 doi: 10.1109/JIOT.2020.2997133
![]() |
[27] |
K. Rahman, S. Abdullah, M. Shakeel, M. S. Ali Khan, M. Ullah, Interval-valued Pythagorean fuzzy geometric aggregation operators and their application to group decision making problem, Cogent Math., 4 (2017), 1338638. https://doi.org/10.1080/23311835.2017.1338638 doi: 10.1080/23311835.2017.1338638
![]() |
[28] |
M. Lin, X. Li, L. Chen, Linguistic q‐rung orthopair fuzzy sets and their interactional partitioned Heronian mean aggregation operators, Int. J. Intell. Syst., 35 (2020), 217–249. https://doi.org/10.1002/int.22136 doi: 10.1002/int.22136
![]() |
[29] |
D. Molodtsov, Soft set theory—first results, Comput. Math. Appl., 37 (1999), 19–31. https://doi.org/10.1016/S0898-1221(99)00056-5 doi: 10.1016/S0898-1221(99)00056-5
![]() |
[30] |
P. K. Maji, R. Biswas, A. R. Roy, Soft set theory, Comput. Math. Appl., 45 (2003), 555–562. https://doi.org/10.1016/S0898-1221(03)00016-6 doi: 10.1016/S0898-1221(03)00016-6
![]() |
[31] | P. K. Maji, R. Biswas, A. R. Roy, Fuzzy soft sets, J. Fuzzy Math., 9 (2001), 589–602. |
[32] | P. K. Maji, R. Biswas, A. R. Roy, Intuitionistic fuzzy soft sets, J. Fuzzy Math., 9 (2001), 677–692. |
[33] | R. Arora, H. Garg, A robust aggregation operators for multi-criteria decision-making with intuitionistic fuzzy soft set environment, Sci. Iran., 25 (2018), 931–942. |
[34] |
A. K. Das, FP-intuitionistic multi fuzzy N-soft set and its induced FP-hesitant N soft set in decision-making, Decis. Mak. Appl. Manag. Eng., 5 (2022), 67–89. https://doi.org/10.31181/dmame181221045d doi: 10.31181/dmame181221045d
![]() |
[35] |
Y. Jiang, Y. Tang, Q. Chen, H. Liu, J. Tang, Interval-valued intuitionistic fuzzy soft sets and their properties, Comput. Math. Appl., 60 (2010), 906–918. https://doi.org/10.1016/j.camwa.2010.05.036 doi: 10.1016/j.camwa.2010.05.036
![]() |
[36] |
R. M. Zulqarnain, X. L. Xin, M. Saqlain, W. A. Khan, TOPSIS method based on the correlation coefficient of interval-valued intuitionistic fuzzy soft sets and aggregation operators with their application in decision-making, J. Math., 2021 (2021), 1–16. https://doi.org/10.1155/2021/6656858 doi: 10.1155/2021/6656858
![]() |
[37] | X. D. Peng, Y. Yang, J. Song, Y. Jiang, Pythagorean fuzzy soft set and its application, Comput. Eng., 41 (2015), 224–229. |
[38] |
R. M. Zulqarnain, I. Siddique, A. Iampan, D. Baleanu, Aggregation operators for interval-valued Pythagorean fuzzy soft set with their application to solve multi-attribute group decision making problem, CMES-Comput. Model. Eng. Sci., 131 (2022), 1717–1750. https://doi.org/10.32604/cmes.2022.019408 doi: 10.32604/cmes.2022.019408
![]() |
[39] | F. Smarandache, Extension of soft set to hypersoft set, and then to plithogenic hypersoft set, Neutrosophic Sets Sy., 22 (2018), 168–170. |
[40] |
A. U. Rahman, M. Saeed, H. A. E. W. Khalifa, W. A. Afifi, Decision making algorithmic techniques based on aggregation operations and similarity measures of possibility intuitionistic fuzzy hypersoft sets, AIMS Math., 7 (2022), 3866–3895. https://doi.org/10.3934/math.2022214 doi: 10.3934/math.2022214
![]() |
[41] |
A. U. Rahman, M. Saeed, S. S. Alodhaibi, H. Abd, Decision making algorithmic approaches based on parameterization of neutrosophic set under hypersoft set environment with fuzzy, intuitionistic fuzzy and neutrosophic settings, CMES-Comput. Model. Eng. Sci., 12 (2021), 743–777. https://doi.org/10.32604/cmes.2021.016736 doi: 10.32604/cmes.2021.016736
![]() |
[42] |
M. Saeed, M. Ahsan, A. U. Rahman, M. H. Saeed, A. Mehmood, An application of neutrosophic hypersoft mapping to diagnose brain tumor and propose appropriate treatment, J. Intell. Fuzzy Syst., 41 (2021), 1677–1699. https://doi.org/10.3233/JIFS-210482 doi: 10.3233/JIFS-210482
![]() |
[43] |
R. M. Zulqarnain, I. Siddique, R. Ali, D. Pamucar, D. Marinkovic, D. Bozanic, Robust aggregation operators for intuitionistic fuzzy hypersoft set with their application to solve MCDM problem, Entropy, 23 (2021), 688. https://doi.org/10.3390/e23060688 doi: 10.3390/e23060688
![]() |
[44] | R. M. Zulqarnain, X. L. Xin, M. Saeed, A development of Pythagorean fuzzy hypersoft set with basic operations and decision-making approach based on the correlation coefficient, In: Theory and Application of Hypersoft Set, Pons Publishing House Brussels., 2021 (2021), 85–106. |
[45] |
R. M. Zulqarnain, I. Siddique, R. Ali, F. Jarad, A. Iampan, Aggregation operators for interval-valued Pythagorean fuzzy hypersoft set with their application to solve MCDM problem, CMES-Comput. Model. Eng. Sci., 135 (2022), 619–651. https://doi.org/10.32604/cmes.2022.022767 doi: 10.32604/cmes.2022.022767
![]() |
[46] |
K. Chatterjee, D. Pamucar, E. K. Zavadskas, Evaluating the performance of suppliers based on using the R'AMATELMAIRCA method for green supply chain implementation in electronics industry, J. Clean. Prod., 184 (2018), 101–129. https://doi.org/10.1016/j.jclepro.2018.02.186 doi: 10.1016/j.jclepro.2018.02.186
![]() |
[47] |
Z. Stevic, D. Pamucar, M. Vasiljevic, G. Stojic, S. Korica, Novel integrated multicriteria model for supplier selection: Case study construction company, Symmetry, 9 (2017), 279. https://doi.org/10.3390/sym9110279 doi: 10.3390/sym9110279
![]() |
[48] |
G. Stojic, Z. Stevic, J. Antucheviciene, D. Pamucar, M. Vasiljevic, A novel rough WASPAS approach for supplier selection in a company manufacturing PVC carpentry products, Information, 9 (2018), 121. https://doi.org/10.3390/info9050121 doi: 10.3390/info9050121
![]() |
[49] |
Z. Stevic, D. Pamucar, A. Puska, P. Chatterjee, Sustainable supplier selection in healthcare industries using a new MCDM method: Measurement of alternatives and ranking according to COmpromise solution (MARCOS), Comput. Ind. Eng., 140 (2020), 106231. https://doi.org/10.1016/j.cie.2019.106231 doi: 10.1016/j.cie.2019.106231
![]() |
[50] |
W. Wang, X. Liu, Y. Qin, Interval-valued intuitionistic fuzzy aggregation operators, J. Syst. Eng. Electron., 23 (2012), 574–580. https://doi.org/10.1109/JSEE.2012.00071 doi: 10.1109/JSEE.2012.00071
![]() |
[51] | Z. Xu, J. Chen, On geometric aggregation over interval-valued intuitionistic fuzzy information, In: Fourth international conference on fuzzy systems and knowledge discovery (FSKD 2007), Haikou, China, 2007,466–471. https://doi.org/10.1109/FSKD.2007.427 |
[52] | S. Khan, H. F. Ashraf, Analysis of Pakistan's electric power sector, In: Blekinge Institute of Technology, Department of Electrical Engineering, 2015. Available from: https://www.diva-portal.org/smash/get/diva2: 917526/FULLTEXT01.pdf. |
[53] | D. Anderson, F. Britt, D. Favre, The seven principles of supply chain management, Supply Chain Manag. Rev., 1 (1997), 21–31. |
[54] |
X. Y. Deng, Y. Hu, Y. Deng, S. Mahadevan, Supplier selection using AHP methodology extended by D numbers, Expert Syst. Appl., 41 (2014), 156–167. https://doi.org/10.1016/j.eswa.2013.07.018 doi: 10.1016/j.eswa.2013.07.018
![]() |
[55] |
G. W. Dickson, An analysis of vendor selection: Aystems and decisions, J. Purch., 1 (1966), 5–17. https://doi.org/10.1111/j.1745-493X.1966.tb00818.x doi: 10.1111/j.1745-493X.1966.tb00818.x
![]() |
[56] |
Y. Wind, P. E. Green, P. J. Robinson, The determinants of vendor selection: The evaluation function approach, J. Purch., 8 (1968), 29–41. https://doi.org/10.1111/j.1745-493X.1968.tb00592.x doi: 10.1111/j.1745-493X.1968.tb00592.x
![]() |
[57] |
W. Ho, X. Xu, P. K. Dey, Multi-criteria decision making approaches for supplier evaluation and selection: A literature review, Eur. J.. Oper. Res., 202 (2010), 16–24. https://doi.org/10.1016/j.ejor.2009.05.009 doi: 10.1016/j.ejor.2009.05.009
![]() |
[58] |
C. A. Weber, J. R. Current, W. C. Benton, Vendor selection criteria and methods, Eur. J.. Oper. Res., 50 (1991), 2–18. https://doi.org/10.1016/0377-2217(91)90033-R doi: 10.1016/0377-2217(91)90033-R
![]() |
[59] |
A. Amid, S. H. Ghodsypour, C. Brien, A weighted max-min model for fuzzy multiobjective supplier selection in a supply chain, Int. J. Prod. Econ., 131 (2011), 139–145. https://doi.org/10.1016/j.ijpe.2010.04.044 doi: 10.1016/j.ijpe.2010.04.044
![]() |
[60] |
F. Jolai, S. A. Yazdian, K. Shahanaghi, M. A. Khojasteh, Integrating fuzzy TOPSIS and multiperiod goal programming for purchasing multiple products from multiple suppliers, J. Purch. Supply Manag., 17 (2011), 42–53. https://doi.org/10.1016/j.pursup.2010.06.004 doi: 10.1016/j.pursup.2010.06.004
![]() |
[61] |
M. Sevkli, S. C. L. Koh, S. Zaim, M. Demirbag, E. Tatoglu, Hybrid analytical hierarchy process model for supplier selection, Ind. Manage. Data Syst., 108 (2008), 122–142. https://doi.org/10.1108/02635570810844124 doi: 10.1108/02635570810844124
![]() |
[62] |
A. Anastasiadis, S. Konstantinopoulos, G. Kondylis, G. A. Vokas, M. J. Salame, Carbon tax, system marginal price and environmental policies on smart microgrid operation, Manag. Environ. Qual., 29 (2018), 76–88. https://doi.org/10.1108/MEQ-11-2016-0086 doi: 10.1108/MEQ-11-2016-0086
![]() |
[63] |
K. Govindan, R. Sivakumar, Green supplier selection and order allocation in a low-carbon paper industry: Integrated multi-criteria heterogeneous decision-making and multiobjective linear programming approaches, Ann. Oper. Res., 238 (2016), 243–276. https://doi.org/10.1007/s10479-015-2004-4 doi: 10.1007/s10479-015-2004-4
![]() |
[64] |
J. D. Qin, X. W. Liu, W. Pedrycz, An extended TODIM multi-criteria group decision making method for green supplier selection in interval type-2 fuzzy environment, Eur. J. Oper. Res., 258 (2017), 626–638. https://doi.org/10.1016/j.ejor.2016.09.059 doi: 10.1016/j.ejor.2016.09.059
![]() |
[65] |
M. Davood, H. G. Seyed, H. Ashkan, A game theoretic analysis in capacity-constrained supplier selection and cooperation by considering the total supply chain inventory costs, Int. J. Prod. Econ., 181 (2016), 87–97. https://doi.org/10.1016/j.ijpe.2015.11.016 doi: 10.1016/j.ijpe.2015.11.016
![]() |
[66] |
X. Tong, Z. J. Wang, A group decision framework with intuitionistic preference relations and its application to low carbon supplier selection, Int. J. Environ. Res. Pub. He., 13 (2016), 923. https://doi.org/10.3390/ijerph13090923 doi: 10.3390/ijerph13090923
![]() |
[67] |
S. Zeng, X. Peng, T. Baležentis, D. Streimikiene, Prioritization of low-carbon suppliers based on Pythagorean fuzzy group decision making with self-confidence level, Econ. Res.-Ekon. Istraaz., 32 (2019), 1073–1087. https://doi.org/10.1080/1331677X.2019.1615971 doi: 10.1080/1331677X.2019.1615971
![]() |