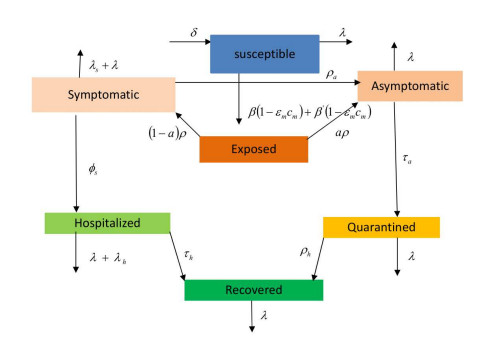
In this article, the Caputo fractional derivative operator of different orders 0<α≤1 is applied to formulate the fractional-order model of the COVID-19 pandemic. The existence and boundedness of the solutions of the model are investigated by using the Gronwall-Bellman inequality. Further, the uniqueness of the model solutions is established by using the fixed-point theory. The Laplace Adomian decomposition method is used to obtain an approximate solution of the nonlinear system of fractional-order differential equations of the model with a different fractional-order α for every compartment in the model. Finally, graphical presentations are presented to show the effects of other fractional parameters α on the obtained approximate solutions.
Citation: Ihtisham Ul Haq, Nigar Ali, Hijaz Ahmad, Taher A. Nofal. On the fractional-order mathematical model of COVID-19 with the effects of multiple non-pharmaceutical interventions[J]. AIMS Mathematics, 2022, 7(9): 16017-16036. doi: 10.3934/math.2022877
[1] | Mohammad Sajid, Biplab Dhar, Ahmed S. Almohaimeed . Differential order analysis and sensitivity analysis of a CoVID-19 infection system with memory effect. AIMS Mathematics, 2022, 7(12): 20594-20614. doi: 10.3934/math.20221129 |
[2] | Murugesan Sivashankar, Sriramulu Sabarinathan, Vediyappan Govindan, Unai Fernandez-Gamiz, Samad Noeiaghdam . Stability analysis of COVID-19 outbreak using Caputo-Fabrizio fractional differential equation. AIMS Mathematics, 2023, 8(2): 2720-2735. doi: 10.3934/math.2023143 |
[3] | Muhammad Farman, Ali Akgül, Kottakkaran Sooppy Nisar, Dilshad Ahmad, Aqeel Ahmad, Sarfaraz Kamangar, C Ahamed Saleel . Epidemiological analysis of fractional order COVID-19 model with Mittag-Leffler kernel. AIMS Mathematics, 2022, 7(1): 756-783. doi: 10.3934/math.2022046 |
[4] | Sadam Hussain, Muhammad Sarwar, Kottakkaran Sooppy Nisar, Kamal Shah . Controllability of fractional differential evolution equation of order γ∈(1,2) with nonlocal conditions. AIMS Mathematics, 2023, 8(6): 14188-14206. doi: 10.3934/math.2023726 |
[5] | Sivaranjani Ramasamy, Thangavelu Senthilprabu, Kulandhaivel Karthikeyan, Palanisamy Geetha, Saowaluck Chasreechai, Thanin Sitthiwirattham . Existence, uniqueness and controllability results of nonlinear neutral implicit ABC fractional integro-differential equations with delay and impulses. AIMS Mathematics, 2025, 10(2): 4326-4354. doi: 10.3934/math.2025200 |
[6] | Yujun Cui, Chunyu Liang, Yumei Zou . Existence and uniqueness of solutions for a class of fractional differential equation with lower-order derivative dependence. AIMS Mathematics, 2025, 10(2): 3797-3818. doi: 10.3934/math.2025176 |
[7] | Shao-Wen Yao, Muhammad Farman, Maryam Amin, Mustafa Inc, Ali Akgül, Aqeel Ahmad . Fractional order COVID-19 model with transmission rout infected through environment. AIMS Mathematics, 2022, 7(4): 5156-5174. doi: 10.3934/math.2022288 |
[8] | Hawsar HamaRashid, Hari Mohan Srivastava, Mudhafar Hama, Pshtiwan Othman Mohammed, Musawa Yahya Almusawa, Dumitru Baleanu . Novel algorithms to approximate the solution of nonlinear integro-differential equations of Volterra-Fredholm integro type. AIMS Mathematics, 2023, 8(6): 14572-14591. doi: 10.3934/math.2023745 |
[9] | Sara Salem Alzaid, Badr Saad T. Alkahtani . Real-world validation of fractional-order model for COVID-19 vaccination impact. AIMS Mathematics, 2024, 9(2): 3685-3706. doi: 10.3934/math.2024181 |
[10] | Isra Al-Shbeil, Abdelkader Benali, Houari Bouzid, Najla Aloraini . Existence of solutions for multi-point nonlinear differential system equations of fractional orders with integral boundary conditions. AIMS Mathematics, 2022, 7(10): 18142-18157. doi: 10.3934/math.2022998 |
In this article, the Caputo fractional derivative operator of different orders 0<α≤1 is applied to formulate the fractional-order model of the COVID-19 pandemic. The existence and boundedness of the solutions of the model are investigated by using the Gronwall-Bellman inequality. Further, the uniqueness of the model solutions is established by using the fixed-point theory. The Laplace Adomian decomposition method is used to obtain an approximate solution of the nonlinear system of fractional-order differential equations of the model with a different fractional-order α for every compartment in the model. Finally, graphical presentations are presented to show the effects of other fractional parameters α on the obtained approximate solutions.
The outbreak of the coronavirus started in December 2019 in China [1]. It has been categorized as a pandemic by the World Health Organization. This new virus causes a severe acute respiratory illness, which can lead to death, that has been spread worldwide. This badly affects the general health system and the economic growth of the developing and underdeveloped countries. Because of the beginning of the first outbreaks, it is assessed that the worldwide disease burden due to COVID-19 amounts to over 91.2 million people infected globally and 1.9 million deaths worldwide [1,2]. From the coronavirus across 215 countries has brought the whole word the series threat [3]. It has been confirmed that large number of huge reported COVID-19 cases tend to be observed as mild to moderate respiratory disorders with symptoms, like coughing, fever, and breathing difficulty. This virus transmits mostly through beads from the nose or mouth when an infected individual sneezes or coughs. When the uninfected one breathes the droplets from the air, they will be presented with the risk of getting the infection. As a result, the best technique to control the spread of the coronavirus is to keep it away from people. Thus, there have been research works to interpret the mechanism to prevent the spread of the coronavirus and examine the impacts of different drugs and non-drug mediation. Mathematical modeling is an importing technique for investigating the underlying dynamics of a virus disperse and developing a control technique when enough data about the infection is scant. Models have also been utilized to predict the infection elements and estimate the efficiency of the intervention strategies disease spreading [4,5,6,7]. In this way different models have been developed that focus on minimizing the spread of coronavirus [8,9,10], such as the isolation of victims, maintaining social distancing, face maskes, washing hands with soap, all official and unofficial gatherings like sports club, games matches, schools, colleges and universities. Mumbu et al. [10] and Verma et al. [11] proposed a model with seven compartments, which include the susceptible population, exposed population, infected population, asymptotic population and recovered population. This model shows the coronavirus infection India and South Korea countries.
Fractional order epidemic models are more helpful and realistic as a tool to evaluate the dynamics of a contagious disease than classical integer order models [12,13]. Because classical mathematical models do not provide a high degree of accuracy for modeling these diseases, fractional differential equations were developed to handle such problems, which have many applications in applied fields related to optimization problems, production problems, artificial intelligence, robotics, cosmology, medical diagnoses, and many more [14,15]. The fractional differential equation has been employed in the mathematical modelling of biological phenomena for many decades. Because mathematical models are effective tools for studying infectious diseases, recently, a lot of scientists have been investing mathematical models of the COVID-19 pandemic with fractional order derivatives; they have produced excellent results [16,17].
Recently, Srivastav et al. [18] developed another compartmental model of the COVID-19 pandemic, particularly for the effects of face masks, hospitalization, and quarantine as follows:
{.S=δ−β(1−εmem)SIs−β′(1−εmem)SIa−λS.E=β1(1−εmem)SIa+β′(1−εmem)SIa−σE−λE.Is=(1−a)σE+ρaIa−(λs+λ)Is−ϕsIs.Ia=aσE−τaIa−ρaIa−λIa.H=ϕsIs−(τh+λh+λ)H.Qh=τaIa−ρhQh−λQ.R=ρhQh+τhH−λR. | (1.1) |
The transmission dynamics of Model (1.1) are represented in the Figure 1 flowchart.
In this model, they have divided the whole population N(t) into seven epidemiological groups: S(t) (susceptible population), E(t) (exposed population), Is(t) (symptomatic population), Ia(t) (asymptomatic population), H(t) (hospitalized population), Qh(t) (quarantined population) and R(t) (recovered population). They categorized the infectious peoples into two subgroups Ia and Is. They assumed that as quickly as symptoms appear, an Ia individual would join the Is class. The biological interpretations of parameters are given in Table 1.
Parameters | Biological Interpretations |
δ | Recruitment rate for the susceptible population |
β′ | Rate of infection of S among Ia population |
β | Rate of infection of S among Is population |
ϵm | Effectiveness of face masks |
em | Masks compliance |
λ | Human mortality rate due to natural causes |
σ | Rate of incubation |
a | Fraction of those exposed who do not exhibit clinical symptoms |
ρa | Rate of progression from Ia to Is class |
ρh | Recovery rate for H individuals |
λs | Disease induced mortality rate for Is class |
τa | Q rate for Ia class |
τh | Recovery rate for R class |
λh | Disease induced mortality rate for H class |
ϕs | Rate of H for Is individuals |
Their model focused on the effects of face masks, hospitalization of the Ia population and quarantine of Is individuals on the spread of the coronavirus. Recently many researchers have proposed models of the COVID-19 pandemic based on the Caputo fractional derivative; they obtained some good results; for details see [19,20]. Consequently, inspired by the previously mentioned work, here we study Model (1.1) under the conditions of using fractional-order derivatives. For α∈(0,1],
{c0Dαt[S(t)]=δ−β(1−εmem)SIs−β′(1−εmem)SIa−λSc0Dαt[E(t)]=β(1−εmem)SIa+β′(1−εmem)SIa−σE−λEc0Dαt[Is(t)]=(1−a)σE+ρaIa−(λs+λ)Is−ϕsIsc0Dαt[Ia(t)]=aσE−τaIa−ρaIa−λIc0Dαt[H(t)]=ϕsIs−(τh+λh+λ)Hc0Dαt[Qh(t)]=τaIa−ρhQh−λQc0Dαt[R(t)]=ρhQh+τhH−λR. | (1.2) |
We apply the following initial conditions:
S(0)=S0=K1,E(0)=E0=K2,Is(0)=Is0=K3,Ia(0)=Ia0=K4,H(0)=H0=K5,Qh(0)=Qh0=K6,R(0)=R0=K7. |
We believe that a good mathematical model will assist health authorities in taking preventative steps against the spread of the new coronavirus disease. Therefore, we first introduce a Caputo fractional-order model and then derive the existence and non-negativity of the Caputo fractional order model solutions by applying the fractional Gronwall-Bellman inequality using the Laplace transform method(LTM). The uniqueness of the solutions of the model is founded on the use of fixed-point theory. Then, we expand the Laplace Adomian decomposition method (LADM) for numerical simulations. The coupling of the Adomian decomposition method and LTM leads to a powerful technique known as Laplace Adomian decomposition. With the application of LTM, we transform a CFDE into algebraic equations. The nonlinear terms in the first two equations are decomposed in terms of Adonmain polynomials. We see that this iterative method works efficiently for a stochastic system as well as deterministic differential equation. More explicitly, we can also use it for a system of linear and nonlinear ordinary differential equations and partial differential equations of an integer and fractional order. In the above process, no perturbation is required. It is to be proved that the LADM is a better technique than the standard ADM method [21,22,23,24].
The rest of the paper is organized as follows: Section 1 is the introduction. Section 2 includes the basic definitions. The existence and non-negativity of the solution are discussed in Section 3. Section 4 is devoted to the uniqueness of the solution. The LAD method is applied to the soolution of the model in Section 5, and the numerical discussion is considered in Section 6. The last section is the conclusion of our work.
Definition 2.1. [25] The CFD of order γ∈(K−1,K] of U(t) is defined as
c0Dγt{U(t)}=1Γ(K−γ)∫t0(t−η)K−γ−1U(K)(η)dη, |
where K=[γ]+1 and [γ] represents the integral parts of γ.
Definition 2.2. Magin [26] The Riemann-Liouville fractional integration of order 0<γ≤1 of the continuous function U is defined as
Iγ(U(t))=1Γ(γ)∫t0(t−η)γ−1U(η)dη,0<η<∞. |
Definition 2.3. [27] The Laplace transform of G(t), as denoted by G(S) is defined as
G(S)=∫∞0e−StG(t)dt. |
Definition 2.4. Mittag-Leffler function (MLF) is defined as
Eα,β(U)=∞∑K=0UKΓ(αK+β), |
and
Eα,1(U)=∞∑K=0UKΓ(αK+1), |
where α and β are greater than zero and the convergence of the MLF is described in [28,29].
Lemma 2.1. [30] The Laplace transform of the Caputo FDO is
L{c0Dγt(U(t))}=SKL{U(t)}−N−1∑K=0Sγ−K−1U(K)(t0), |
where K−1<γ<K, K=[γ]+1 and [γ] shows theinteger part of γ.
Lemma 2.2. [31] The following result holds for Caputo FDO
Iγ[c0DγtU](t)=U(t)+N−1∑K=0UK(0)ΓK+1UK |
for any γ>0,K∈N, where K=[γ]+1 and [γ]shows the integer part of γ.
This section is dedicated to proving the positivity and boundedness of the solution of System (1.2). The size of each class of the population varies over time, and the whole population N(t) is given by
N=S+E+Is+Ia+H+Qh+R. |
Applying Caputo fractional order derivatives and using the linearity property of the Caputo operator, we get
c0Dαt{N(t)}=c0Dαt[S(t)]+c0Dαt[E(t)]+c0Dαt[Is(t)]+c0Dαt[Ia(t)]+c0Dαt[H(t)]+c0Dαt[Qh(t)]+c0Dαt[R(t)]=δ−λN(t)−λsIs−λhH, |
c0Dαt[N(t)]−λN(t)≤δ. | (3.1) |
Applying the LTM to solve the FGB inequality given by Eq (3.1) with initial conditions N(t0)≥0, we obtain
L{c0DαtN(t)−λN(t)}≤L{δ}, |
L{N(t)}≤δS(λ+Sα)+P−1∑K=0Sα−K−1NK(t0)(Sα+λ). | (3.2) |
Splitting Eq (3.2) into partial fractions, we get
L{N(t)}≤δ(1S−1S(1+λSα))+P−1∑K=0NK(t0)SK+1(1+λSα). | (3.3) |
By using binomial series expansion, we get
1(1+λSα)=∞∑K=0(−λSα)K. |
Therefore, Eq (3.3) becomes
L{N(t)}≤δ(1S−∞∑K=0(−λ)KSKα+1)+K−1∑P=0∞∑K=0(−λ)KNK(t0)SαK+P+1. | (3.4) |
Applying the inverse Laplace transform to Eq (3.4) gives
N(t)≤δ(1−∞∑K=0(−λ)KtKαΓ(Kα+1))+K−1∑P=0∞∑K=0(−μλ)NP(t0)tKα+PΓ(Kα+P+1)≤δ(1−∞∑K=0(−λtα)KΓ(Kα+1))+K−1∑P=0∞∑K=0(−λtα)KNP(t0)tPΓ(Kα+P+1). |
Now, we apply the definition of MLF
N(t)≤δ(1−∞∑K=0(−λtα)K)Γ(Kα+1))+Eα,P+1(−λtα)tPNP(t0), | (3.5) |
where Eq (3.5), Eα,1(−λtα) and Eα,K+1(−λtα) represent the series of entire function. Hence the solutions of Model (1.2) are bounded.
Thus,
{(S(t),E(t),Is(t),Ia(t),H(t),Qh(t),R(t))∈R7+,0≤N(t)≤δ(1−Eα,1(−λtα)+Eα,P+1(−λtα)tPNP(t0)}. |
Using the fixed point theory, we will discuss the uniqueness of the solutions of the Caputo fractional-order Model (1.2). For this need, we will follow the following procedure.
Applying the initial conditions and the fractional integral to System (1.2), we obtain
S(t)=K1+1Γ(α)∫t0(t−η)α−1[δ−β(1−ϵmcm)SIs−β′(1−ϵmcm)SIa−λS]dη,E(t)=K2+1Γ(α)∫t0(t−η)α−1[β(1−ϵmcm)SIs+β′(1−ϵmcm)SIa−σE−λE]dη,Is(t)=K3+1Γ(α)∫t0(t−η)α−1[(1−a)σE+ρaIa−(λs+λ)−ϕsIs]dη,Ia(t)=K4+1Γ(α)∫t0(t−η)α−1[aσE−τaIa−ρaIa−λI]dη,H(t)=K5+1Γ(α)∫t0(t−η)α−1[ϕsIs−(τh+λh+λ)H]dη,Qh(t)=K6+1Γ(α)∫t0(t−η)α−1[τaIa−ρhQh−λQ]dη,R(t)=K7+1Γ(α)∫t0(t−η)α−1[ρhQh+τhHh−λR]dη. | (4.1) |
It is assumed that
U(t)={SEIaIsHQhR, | (4.2) |
K={K1K2K3K4K5K6K7. | (4.3) |
We define the functions under the integral signs in Eq (4.1) as
G(t,U(t))={F1(S,E,Is,Ia,H,Qh,R)=δ−β(1−ϵmcm)SIs−β′(1−ϵmcm)SIa−λSF2(S,E,Is,Ia,H,Qh,R)=β(1−ϵmcm)SIs+β′(1−ϵmcm)SIa−σE−λEF3(S,E,Is,Ia,H,Qh,R)=(1−a)σE+ρaIa−(λs+λ)−ϕsIsF4(S,E,Is,Ia,H,Qh,R)=aσE−τaIa−ρaIa−λIF5(S,E,Is,Ia,H,Qh,R)=ϕsIs−(τh+λh+λ)HF6(S,E,Is,Ia,H,Qh,R)=τaIa−ρhQh−λQF7(S,E,Is,Ia,H,Qh,R)=ρhQh+τhHh−λR. | (4.4) |
Substituting Eq (4.4) into Eq (4.1), we get
S(t)=K1+1Γ(α)∫t0(t−η)α−1F1(S,E,Is,Ia,H,Qh,R)dη,E(t)=K2+1Γ(α)∫t0(t−η)α−1F2(S,E,Is,Ia,H,Qh,R)dη,Is(t)=K3+1Γ(α)∫t0(t−η)α−1F3(S,E,Is,Ia,H,Qh,R)dη,Ia(t)=K4+1Γ(α)∫t0(t−η)α−1F4(S,E,Is,Ia,H,Qh,R)dη,H(t)=K5+1Γ(α)∫t0(t−η)α−1F5(S,E,Is,Ia,H,Qh,R)dη,Qh(t)=K6+1Γ(α)∫t0(t−η)α−1F6(S,E,Is,Ia,H,Qh,R)dη,R(t)=K7+1Γ(α)∫t0(t−η)α−1F7(S,E,Is,Ia,H,Qh,R)dη. | (4.5) |
So, System (4.5) becomes
U(t)=K+1Γ(α)∫t0(t−η)α−1G(η,U(η))dη. | (4.6) |
Consider a Banach space Θ=C[0,T]xC[0,T], with a norm
‖S,E,Is,Ia,H,Qh,R‖=maxt∈[0,T]|S+E+Is+Ia+H+Qh+R|. |
Let Ψ:Θ→Θ be a mapping defined as
Ψ[U(t)]=K+1Γ(α)∫t0(t−η)α−1G(η,U(η))dη. | (4.7) |
Theorem 4.1. System (4.4) has at least one solution if there existconstants MC>0, and NC>0, such that
|G(t,U(t))|≤MC|U(t)|+NC. | (4.8) |
Proof. To show that the operator Ψ is bounded on Ω:{U∈Ω|Λ≥‖U‖}, where
Λ≥maxt∈[0,T]K+(MCTα/Γ(α+1))K−(NCTα/Γ(α+1)), | (4.9) |
is a closed and convex subset of Θ. Now, take
‖Ψ(U)‖=maxt∈[0,T]|K+1Γ(α)∫t0(t−η)α−1G(η,U(η))dη|≤|K|+1Γ(α)∫t0(t−η)α−1|G(η,U(η))|dη≤|K|+1Γ(α)∫t0(t−η)α−1|MC|U(t)|+NC|dη≤|K|+|MC|U(t)|+NC|TαΓ(α+1)≤Λ. | (4.10) |
It means that U∈Θ⇒Ψ(Ω)⊆Ω, which shows that d is bounded. Next, to show that Ψ is completely continuous, let t1<t2∈[0,T] and take
‖Ψ(U)(t2)−Ψ(U)(t1)‖=|1Γ(α)∫t20(t2−η)α−1G(η,U(η))dη−1Γ(α)∫t10(t1−η)α−1G(η,U(η))dη|≤[tα1−tα2]|MC|U(t)|+NC|Γ(α+1). | (4.11) |
This shows that ‖Ψ(U)(t2)−Ψ(U)(t1)‖→0,as t2→t1. Hence, the operator Ψ is completely continuous according to the Arzelá-Ascoli theorem. Therefore, the given system, System (4.4) has at least one solution according to Schauder's fixed-point theorem.
Next, the fixed-point theorem of Banach was used to demonstrate that System (4.4) has a unique solution.
Theorem 4.2. System (4.4) has a unique solution if there exists a constantCC>0, such that for each U1(t),U2(t)∈Θ,such that
|G(t,U1(t))−G(t,U2(t))|≤CC|U1(t)−U2(t)|. | (4.12) |
Proof. Let U1(t),U2(t)∈Θ. Take
|Ψ(U1)−Ψ(U2)|=maxt∈[0,T]|1Γ(α)∫t0(t−η)α−1G(η,U1(η))dη−1Γ(α)∫t0(t−η)α−1G(η,U2(η))dη|≤TαΓ(α+1)CC|U1−U2|. |
Hence, Ψ is the contraction. System (4.4) has a unique solution based on the Banach contraction theorem.
Here, we discuss the use of the Laplace Adomian algorithm for the nonlinear and linear fractional differential equations. The nonlinear terms in this model are SIs and SIa. Further, δ,β,β′,εm,em,σ,a,ρa,ϕs,τa,τh,ρh,λ,λs, and λh are known constants.
Applying the Laplace transform to Model (1.2), we get
L{c0Dαt[S(t)]}=L{δ−β(1−εmem)SIs−β′(1−εmem)SIa−λS},L{c0Dαt[E(t)]}=L{β(1−εmem)SIa+β′(1−εmem)SIa−σE−λE},L{c0Dαt[Is(t)]}=L{(1−a)σE+ρaIa−(λs+λ)Is−ϕsIs},L{c0Dαt[Ia(t)]}=L{aσE−τaIa−ρaIa−λIa},L{c0Dαt[H(t)]}=L{ϕsIs−(τh+λh+λ)H},L{c0Dαt[Qh(t)]}=L{τaIa−ρhQh−λQh},L{c0Dαt[R(t)]}=L{ρhQh+τhH−λR}. | (5.1) |
Using the differentiation property of the LT and given initial conditions given in Eq (5.1), we get
{SαL{S(t)}−Sα−1S(0)=L{δ−β(1−εmem)SIs−β′(1−εmem)SIa−λS}SαL{E(t)}−Sα−1E(0)=L{β(1−εmem)SIa+β′(1−εmem)SIa−σE−λE}SαL{Is(t)}−Sα−1Is(0)=L{(1−a)σE+ρaIa−(λs+λ)Is−ϕsIs}SαL{Ia(t)}−Sα−1Ia(0)=L{aσE−τaIa−ρaIa−λIa}SαL{H(t)}−Sα−1H(0)=L{ϕsIs−(τh+λh+λ)H}SαL{Q(t)}−Sα−1Qh(0)=L{τaIa−ρhQh−λQh}SαL{R(t)}−Sα−1R(0)=L{ρhQh+τhH−λR}. | (5.2) |
Now, applying the inverse Laplace transform to System (5.2) and the initial conditions, we get
{S(t)=K1+L−1[1Sα−1L{δ−β(1−εmem)SIs−β′(1−εmem)SIa−λS}]E(t)=K2+L−1[1Sα−1L{β(1−εmem)SIa+β′(1−εmem)SIa−σE−λE}]Is(t)=K3+L−1[1Sα−1L{(1−a)σE+ρaIa−(λs+λ)Is−ϕsIs}]Ia(t)=K4+L−1[1Sα−1L{aσE−τaIa−ρaIa−λIa}]H(t)=K5+L−1[1Sα−1L{ϕsIs−(τh+λh+λ)H}]Q(t)=K6+L−1[1Sα−1L{τaIa−ρhQh−λQh}]R(t)=K7+L−1[1Sα−1L{ρhQh+τhH−λR}]. | (5.3) |
Now, we assume that the solution of S(t),E(t),Is(t),Ia(t),H(t),Qh(t) and R(t) in the form of infinite series is defined as
{S(t)=∞∑P=0SP(t)E(t)=∞∑P=0EP(t)Is(t)=∞∑P=0Is,P(t)Ia(t)=∞∑P=0Ia,P(t)H(t)=∞∑P=0HP(t)Qh(t)=∞∑P=0Qh,PR(t)=∞∑P=0RP(t). | (5.4) |
The nonlinear terms of the fractional order model are SIs and SIa. The nonlinear terms are decomposed by using an Adomian polynomial, as follow:
S(t)Is(t)=∞∑P=0CP,SIa(t)=∞∑P=0BP, | (5.5) |
where CP and BP are called Adomian polynomials, and they depend upon S0Is0,S1Is1,...SmIsm and S0Ia0,S1Ia1,...SmIam, respectively. We can calculate the polynomials by using the following formula:
CP=1Γ(P+1)dPdtP[P∑K=0λKSKP∑K=0λKIs,K]⇂λ=0,BP=1Γ(P+1)dPdtP[P∑K=0λKSKP∑K=0λKIa,K]⇂λ=0. |
Substituting Eqs (5.4) and (5.5) into Eq (5.3), we get
{L{S1(t)}=k1S+1Sα−1L{δ−β(1−εmem)C0−β′(1−εmem)B0−λS}L{E1(t)}=k2S+1Sα−1L{β(1−εmem)C0+β′(1−εmem)B0−σE−λE0}L{Is1(t)}=k3S+1Sα−1L{(1−a)σE0+ρaIa0−(λs+λ)Is−ϕsIs0}L{Ia1(t)}=k4S+1Sα−1L{aσE0−τaIa0−ρaIa0−λIa0}L{H1(t)}=k5S+1Sα−1L{ϕsIs0−(τh+λh+λ)H0}L{Qh1(t)}=k6S+1Sα−1L{τaIa0−ρhQh0−λQh0}L{R1(t)}=k7S+1Sα−1L{ρhQh0+τhH0−λR0}. | (5.6) |
The subsequent terms are
{L{S2(t)}=K1S+1Sα−1L{δ−β(1−εmem)C1−β′(1−εmem)B1−λS1}L{E2(t)}=K2S+1Sα−1L{β(1−εmem)C1+β′(1−εmem)B1−σE1−λE1}L{Is2(t)}=K3S+1Sα−1L{(1−a)σE1+ρaIa1−(λs+λ)Is1−ϕsIs1}L{Ia2(t)}=K4S+1Sα−1L{aσE1−τaIa1−ρaIa1−λIa1}L{H2(t)}=K5S+1Sα−1L{ϕsIs1−(τh+λh+λ)H1}L{Qh2(t)}=K6S+1Sα−1L{τaIa1−αhQh1−λQh1}L{R2(t)}=K7S+1Sα−1L{ρhQh1+τhH1−λR1}. | (5.7) |
{L{Sn+1(t)}=K1S+1Sα−1L{δ−β(1−εmem)Cn−β′(1−εmem)Bn−λSn}L{En+1(t)}=K2S+1Sα−1L{β(1−εmem)Cn+β′(1−εmem)Bn−σEn−λEn}L{Is,n+1(t)}=K3S+1Sα−1L{(1−a)σEn+ρaIan−(λs+λ)Is,n−ϕsIsn}L{Ia,n+1(t)}=K4S+1Sα−1L{aσEn−τaIan−ρaIan−λIan}L{Hn+1(t)}=K5S+1Sα−1L{ϕsIsn−(τh+λh+λ)Hn}L{Qh,n+1(t)}=K6S+1Sα−1L{τaIan−ρhQhn−λQhn}L{Rn+1(t)}=K7S+1Sα−1L{ρhQhn+τhHn−λR}. | (5.8) |
By applying the initial conditions, we obtained C0=S0Is0=K1K3, B0=S0Iao=K1K4.
Then, applying the inverse Laplace transform to Eq (5.8), we obtain the values for Eq (5.8) recursively.
S1(t)=K1+{δ−β(1−εmem)K1K3−β′(1−εmem)K1K4−λK1}tαΓ(α+1),E1(t)=K2+{β(1−εmem)K1K3+β′(1−εmem)K1K4−σK2−λK2}tαΓ(α+1),Is,1(t)=K3+{(1−a)σK2+ρaK4−(λs+λ)K3−ϕsK4}tαΓ(α+1),Ia,1(t)=K4+{aσK2−τaK4−ρaK4−λK4}tαΓ(α+1),H1(t)=K5+{ϕsK3−(τh+λh+λ)K5}tαΓ(α+1),Qh,1(t)=K6+{τaK4−ρhK6−λK6}tαΓ(α+1),R1(t)=K7+{ρhK5+τhK5−λK7}tαΓ(α+1). | (5.9) |
Similarly, we get the following series
![]() |
![]() |
(5.10) |
We noted that the result obtained via LADM is the same as the ADM [32,33].
Now, we considered a graphical representation of the obtained approximate solutions given by Eq (5.10). Here, we calculated only three terms for the required approximate solutions of System (1.2). The values of the parameters have either been taken from suitable sources or were assumed. The importance of these parameters is given in Table 2.
Name | Parameters values | References |
δ | 0.23 | Assumed |
β′ | 0.000516 | Assumed |
λs | 0.0062 | Assumed |
ϕs | 0.06 | [34] |
ρa | 0.07 | [34] |
εm | 0.5 | [35] |
em | 0.2 | [36] |
σ | 0.28 | [37] |
a | 0.66 | [37] |
λh | 0.0045 | Assumed |
λ | 0.000428 | Demographic |
τh | 1/14 | [38,39] |
In Figures 2–8, we present the approximate solutions of the Caputo fractional-order model given by Model (1.2) by using the LADM corresponding to different fractional-order values of α∈(0,1]. Figure 2 demonstrates that the susceptible population rapidly decreases when the value of α decreases. The graph in Figure 3 shows the exposed class when the value of α goes down, the rate of increase slowly reduces. The graph in Figure 5 shows that the number of asymptomatic populations decreases for different values of α. Therefore, it can be seen in Figure 8 that the people are recover very quickly with different fractional values of α. We also notice that the combined effects of face masks and the hospitalization of asymptomatic individuals on the asymptomatic populations which consequently decreases very rapidly Figure (5). The plot shows that the asymptomatic population disappears completely in a shorter time if the face masks are used together with the hospitalization of confirmed infected people.
Figure 9 shows all the seven compartments of Model (1.2) when the integer order α=1, and considering the data from Table 2. As compered to traditional derivatives of integer order, fractional order derivatives give more accuracy.
This study investigated the caputo fractional-order model of the COVID-19 pandemic, wherein we modeled the effects of quarantine, hospitalization, and face mask use on the COVID-19 pandemic. The existence and non-negativity of the solutions of Model (1.2) have been demonstrated by solving the FGB inequality using the LTM. We proved the uniqueness of the solution of the Caputo fractional order model. We found that the LADM is a powerful technique capable of heading linear and nonlinear Caputo fractional differential equations. This method produces the same solution as the ADM with the correct choice of initial approximation. From these examples, we can see that this method is considered effective for solving many Caputo fractional-order models. Finally, we derived the graphical results of the obtained approximate solutions presented in Eq (5.10) with different values of the fractional-order α∈(0,1].
The authors received financial support from Taif University Researchers Supporting Project (TURSP-2020/031)at Taif University, Taif, Saudi Arabia.
The authors declare that they have no competing interests.
[1] |
M. Garcia, N. Lipskiy, J. Tyson, R. Watkins, E. Stein, T. Kinley, Centers for disease control and prevention 2019 novel coronavirus disease (COVID-19) information management: Addressing national health-care and public health needs for standardized data definitions and codified vocabulary for data exchange, J. Am. Med. Inf. Assoc., 27 (2020), 1476–1487. https://doi.org/10.1093/jamia/ocaa141 doi: 10.1093/jamia/ocaa141
![]() |
[2] | Report 9–Impact of non-pharmaceutical interventions (NPIs) to reduce COVID-19 mortality and healthcare demand, MRC Centre for Global Infectious Disease Analysis COVID-19, 2020. Available from: https://www.imperial.ac.uk/mrc-global-infectious-disease-analysis/covid-19/report-9-impact-of-npis-on-covid-19/. |
[3] |
P. Roy, K. R. Upadhyay, J. Caur, Modeling Zika transmission dynamics: Prevention and control, J. Biol. Syst., 28 (2020), 719–749. https://doi.org/10.1142/S021833902050014X doi: 10.1142/S021833902050014X
![]() |
[4] |
S. Dilshad, N. Singh, M. Atif, A. Hanif, N. Yaqub, W. A. Farooq, et al., Automated image classification of chest X-rays of COVID-19 using deep transfer learning, Results Phys., 28 (2021), 104529. https://doi.org/10.1016/j.rinp.2021.104529 doi: 10.1016/j.rinp.2021.104529
![]() |
[5] |
M. Nicola, Z. Alsafi, C. Sohrabi, A. Kerwan, A. Al-Jabir, C. Iosifidis, et al., The socio-economic implications of the coronavirus pandemic (COVID-19): A review, Int. J. Surg., 78 (2020), 185–193. https://doi.org/10.1016/j.ijsu.2020.04.018 doi: 10.1016/j.ijsu.2020.04.018
![]() |
[6] |
I. A. Bashir, B. A. Nasidi, Fractional order model for the role of mild cases in the transmission of COVID-19, Chaos Solition. Fract., 142 (2021), 110374–110383. https://doi.org/10.1016/j.chaos.2020.110374 doi: 10.1016/j.chaos.2020.110374
![]() |
[7] |
S. Ahmada, A. Ullaha, Q. Mdallal, H. Khan, K. Shaha, A. Khan, Fractional order mathematical modeling of COVID-19 transmission, Chaos Solition. Fract., 139 (2020), 110256–110263. https://doi.org/10.1016/j.chaos.2020.110256 doi: 10.1016/j.chaos.2020.110256
![]() |
[8] |
T. Chen, J. Rui, Q. Wang, Z. Zhao, J. Cui, L. Yin, A mathematical model for simulating the phase-based transmissibility of a novel coronavirus, Infect. Dis. Poverty, 9 (2020), 24. https://doi.org/10.1186/s40249-020-00640-3 doi: 10.1186/s40249-020-00640-3
![]() |
[9] |
K. Nisar, S. Ahmad, A. Ullah, K. Shah, H. Alrabaiahc, M. Arfana, Mathematical analysis of SIRD model of COVID-19 with Caputo fractional derivative based on real data, Results Phys., 21 (2021), 103772–103780. https://doi.org/10.1016/j.rinp.2020.103772 doi: 10.1016/j.rinp.2020.103772
![]() |
[10] |
A. J. Mumbu, A. K. Hugo, Mathematical modelling on COVID-19 transmission impacts with preventive measures: A case study of Tanzania, Adv. Differ. Equ., 14 (2020), 748–766. https://doi.org/10.1080/17513758.2020.1823494 doi: 10.1080/17513758.2020.1823494
![]() |
[11] |
R. Verma, S. P. Tiwari, R. Upadhyay, Transmission dynamics of epidemic spread and outbreak of Ebola in West Africa: Fuzzy modeling and simulation, J. Biol. Dyn., 60 (2019), 637–671. https://doi.org/10.1007/s12190-018-01231-0 doi: 10.1007/s12190-018-01231-0
![]() |
[12] |
M. A. Khan, S. Ullah, K. O. Okosun, K. Shah, A fractional order pine wilt disease model with Caputo-Fabrizio derivative, Adv. Differ. Equ., 2018 (2018), 410. https://doi.org/10.1186/s13662-018-1868-4 doi: 10.1186/s13662-018-1868-4
![]() |
[13] |
I. Area, H. Batarfi, J. Losada, J. J. Nieto, W. Shammakh, Á. Torres, On a fractional order Ebola epidemic model, Adv. Differ. Equ., 2015 (2015), 278. https://doi.org/10.1186/s13662-015-0613-5 doi: 10.1186/s13662-015-0613-5
![]() |
[14] |
M. Dulǎu, A. Gligor, T. M. Dulău, Fractional order controllers versus integer order controllers, Procedia Eng., 181 (2017), 538–545. https://doi.org/10.1016/j.proeng.2017.02.431 doi: 10.1016/j.proeng.2017.02.431
![]() |
[15] |
D. Sain, B. M. Mohan, A simple approach to mathematical modelling of integer order and fractional order fuzzy PID controllers using one-dimensional input space and their experimental realization, J. Franklin Inst., 358 (2021), 3726–3756. https://doi.org/10.1016/j.jfranklin.2021.03.010 doi: 10.1016/j.jfranklin.2021.03.010
![]() |
[16] |
M. Higazy, Novel fractional order SIDARTHE mathematical model of COVID-19 pandemic, Chaos Solition. Fract., 138 (2020), 110007. https://doi.org/10.1016/j.chaos.2020.110007 doi: 10.1016/j.chaos.2020.110007
![]() |
[17] |
N. H. Tuan, H. Mohammadi, S. Rezapour, A mathematical model for COVID-19 transmission by using the Caputo fractional derivative, Chaos Solition. Fract., 140 (2020), 110107. https://doi.org/10.1016/j.chaos.2020.110107 doi: 10.1016/j.chaos.2020.110107
![]() |
[18] |
A. Srivastav, P. Tiwari, P. Srivastava, M. Ghosh, Y. Kang, A mathematical model for the impacts of face mask, hospitalization and quarantine on the dynamics of COVID-19 in India: Deterministic vs. stochastic, Math. Biosci. Eng., 18 (2021), 182–213. https://doi.org/10.3934/mbe.2021010 doi: 10.3934/mbe.2021010
![]() |
[19] |
K. Shah, T. A. jawad, I. Mahariq, F. Jarad, Qualitative analysis of a mathematical model in the time of COVID-19, BioMed Res. Int., 2020 (2020), 5098598. https://doi.org/10.1155/2020/5098598 doi: 10.1155/2020/5098598
![]() |
[20] |
Z. Zhang, A. Zeb, O. F. Egbelowo, V. S. Erturk, Dynamics of a fractional order mathematical model for COVID-19 epidemic, Adv. Differ. Equ., 2020 (2020), 420. https://doi.org/10.1186/s13662-020-02873-w doi: 10.1186/s13662-020-02873-w
![]() |
[21] |
H. Jafari, C. M. Khalique, M. Nazari, Application of the Laplace decomposition method for solving linear and nonlinear fractional diffusion–wave equations, Appl. Math. Lett., 24 (2011), 1799–1805. https://doi.org/10.1016/j.aml.2011.04.037 doi: 10.1016/j.aml.2011.04.037
![]() |
[22] |
F. Haq, K. Shah, G. Rahman, M. Shahzada, Numerical solution of fractional order smoking model via Laplace Adomian decomposition method, Alex. Eng. J., 57 (2018), 1061–1069. https://doi.org/10.1016/j.aej.2017.02.015 doi: 10.1016/j.aej.2017.02.015
![]() |
[23] |
M. Z. Mohamed, T. M. Elzaki, Comparison between the Laplace decomposition method and Adomian decomposition in time-space fractional nonlinear fractional differential equations, Appl. Math., 9 (2018), 84309. https://doi.org/10.4236/am.2018.94032 doi: 10.4236/am.2018.94032
![]() |
[24] |
M. De la Sen, Positivity and stability of the solutions of Caputo fractional linear time-invariant systems of any order with internal point delays, Abstr. Appl. Anal., 2011 (2011), 161246. https://doi.org/10.1155/2011/161246 doi: 10.1155/2011/161246
![]() |
[25] |
S. Bushnaq, T. Saeed, D. F. M. Torres, A. Zeb, Control of COVID-19 dynamics through a fractional-order model, Alex. Eng. J., 60 (2021), 3587–3592. https://doi.org/10.1016/j.aej.2021.02.022 doi: 10.1016/j.aej.2021.02.022
![]() |
[26] |
R. Magin, Fractional calculus in bioengineering, Crit. Rev. Biomed. Eng., 32 (2006), 1–104. https://doi.org/10.1615/critrevbiomedeng.v32.i1.10 doi: 10.1615/critrevbiomedeng.v32.i1.10
![]() |
[27] | Introduction to Differential Equations, The Hong Kong University of Science and Technology, 2003. Available from: https://hostnezt.com/cssfiles/appliedmaths/Introduction20to20Differential20Equations20By20Jeffrey20R.0Chasnov.pdf. |
[28] |
I. Podlubny, A. Chechkin, T. Skovranek, Y. Q. Chen, M. V. Jara, Matrix approach to discrete fractional calculus Ⅱ: Partial fractional differential equations, J. Comput. Phys., 228 (1019), 3137–3153. https://doi.org/10.1016/j.jcp.2009.01.014 doi: 10.1016/j.jcp.2009.01.014
![]() |
[29] | J. Sabatier, O. P. Agrawal, J. A. Tenreiro Machado, Advances in fractional calculus, Theoretical Developments and Applications in Physics and Engineering, 4 Eds., Berlin: Springer, 2007. https://doi.org/10.1007/978-1-4020-6042-7 |
[30] |
M. S. Abdo, K. Shah, H. A. Wahash, S. K. Panchal, On a comprehensive model of the novel coronavirus (COVID-19) under Mittag-Leffler derivative, Chaos Solition. Fract., 135 (2020), 109867. https://doi.org/10.1016/j.chaos.2020.109867 doi: 10.1016/j.chaos.2020.109867
![]() |
[31] |
S. Kumar, A. Yildirim, Y. Khan, L. Wei, A fractional model of the diffusion equation and its analytical solution using Laplace transform, Sci. Iran., 19 (2012), 1117–1123. https://doi.org/10.1016/j.scient.2012.06.016 doi: 10.1016/j.scient.2012.06.016
![]() |
[32] |
H. Khan, R. Shah, P. Kumam, D. Baleanu, M. Arif, A two-step Laplace decomposition method for solving nonlinear partial differential equations, Int. J. Phys. Sci., 6 (2011), 4102–4109. https://doi.org/10.5897/IJPS11.146 doi: 10.5897/IJPS11.146
![]() |
[33] |
J. H. He, Variational iteration method–a kind of non-linear analytical technique: Some examples, Int. J. Non-Linear Mech., 34 (1999), 699–708. https://doi.org/10.1016/S0020-7462(98)00048-1 doi: 10.1016/S0020-7462(98)00048-1
![]() |
[34] |
D. Aldila, M. Z. Ndii, B. M. Samiadji, Optimal control on COVID-19 eradication program in Indonesia under the effect of community awareness, Math. Biosci. Eng., 17 (2020), 6355–6389. http://dx.doi.org/10.3934/mbe.2020335 doi: 10.3934/mbe.2020335
![]() |
[35] |
A. Davies, K. Thompson, K. Giri, G. Kafatos, J. Walker, A. Bennett, Testing the efficacy of homemade masks: Would they protect in an influenza pandemic, Disaster Med. Public Health Prep., 7 (2013), 413–418. https://doi.org/10.1017/dmp.2013.43 doi: 10.1017/dmp.2013.43
![]() |
[36] |
C. J. Noakes, P. Sleigh, Mathematical models for assessing the role of airflow on the risk of airborne infection in hospital wards, J. R. Soc. Interface, 6 (2009), S791–S800. https://doi.org/10.1098/rsif.2009.0305.focus doi: 10.1098/rsif.2009.0305.focus
![]() |
[37] |
M. A. Khan, A. Atangana, Modeling the dynamics of novel coronavirus (2019-nCov) with fractional derivative, Alex. Eng. J., 59 (2020), 2379–2389. https://doi.org/10.1016/j.aej.2020.02.033 doi: 10.1016/j.aej.2020.02.033
![]() |
[38] |
F. Zhou, T. Yu, R. Du, G. Fan, Y. Liu, Z. Liu, et al., Clinical course and risk factors for mortality of adult inpatients with COVID-19 in Wuhan, China: A retrospective cohort study, Lancet, 395 (2020), 1054–1062. https://doi.org/10.1016/S0140-6736(20)30566-3 doi: 10.1016/S0140-6736(20)30566-3
![]() |
[39] |
B. Tang, X. Wang, Q. Li, N. L. Bragazzi, S. Tang, Y. Xiao, et al., Estimation of the transmission risk of the 2019-nCoV and its implication for public health interventions, J. Clin. Med., 9 (2020), 462. https://doi.org/10.3390/jcm9020462 doi: 10.3390/jcm9020462
![]() |
1. | Victoria May P. Mendoza, Renier Mendoza, Youngsuk Ko, Jongmin Lee, Eunok Jung, Managing bed capacity and timing of interventions: a COVID-19 model considering behavior and underreporting, 2022, 8, 2473-6988, 2201, 10.3934/math.2023114 | |
2. | V. Rexma Sherine, P. Chellamani, Rashad Ismail, N. Avinash, G. Britto Antony Xavier, Estimating the Spread of Generalized Compartmental Model of Monkeypox Virus Using a Fuzzy Fractional Laplace Transform Method, 2022, 14, 2073-8994, 2545, 10.3390/sym14122545 | |
3. | Aaqid Mohi-Ud Din Bhat, Younis Ahmad Bhat, A Multi-compartment Mathematical Model for HIV–AIDS Transmission and Dynamics, 2023, 2731-8095, 10.1007/s40995-022-01397-0 | |
4. | Ihtisham Ul Haq, Numan Ullah, Nigar Ali, Kottakkaran Sooppy Nisar, A New Mathematical Model of COVID-19 with Quarantine and Vaccination, 2022, 11, 2227-7390, 142, 10.3390/math11010142 | |
5. | Victoria May P. Mendoza, Renier Mendoza, Jongmin Lee, Eunok Jung, Adjusting non-pharmaceutical interventions based on hospital bed capacity using a multi-operator differential evolution, 2022, 7, 2473-6988, 19922, 10.3934/math.20221091 | |
6. | Ihtisham Ul Haq, Nigar Ali, Hijaz Ahmad, Analysis of a chaotic system using fractal-fractional derivatives with exponential decay type kernels, 2022, 2, 2767-8946, 185, 10.3934/mmc.2022019 | |
7. | Islam M Elbaz, MA Sohaly, H El-Metwally, On the extinction of stochastic Susceptible-Infected-Susceptible epidemic with distributed delays, 2023, 42, 1461-3484, 1866, 10.1177/14613484231181956 | |
8. | Vivek MİSHRA, Sarit MAİTRA, Mihir DASH, Saurabh Kumar AGRAWAL, Praveen AGARWAL, Chaos and Control of COVID-19 Dynamical System, 2023, 5, 2687-4539, 233, 10.51537/chaos.1320492 | |
9. | Ibad Ullah, Nigar Ali, Ihtisham Ul Haq, Imtiaz Ahmad, Mohammed Daher Albalwi, Md. Haider Ali Biswas, Kareem T. Elgindy, Analysis of COVID-19 Disease Model: Backward Bifurcation and Impact of Pharmaceutical and Nonpharmaceutical Interventions, 2024, 2024, 1687-0425, 1, 10.1155/2024/6069996 | |
10. | Asma Rashid Butt, Aitzaz Ahmad Saqib, Abu Bakar, Dilber Uzun Ozsahin, Hijaz Ahmad, Bandar Almohsen, Investigating the fractional dynamics and sensitivity of an epidemic model with nonlinear convex rate, 2023, 54, 22113797, 107089, 10.1016/j.rinp.2023.107089 | |
11. | Ibad ullah, Nigar Ali, Ihtisham Ul Haq, Mohammed Daher Albalwi, Shah Muhammad, Mohammad Shuaib, Comprehensive analysis of COVID-19 transmission dynamics: mathematical modeling, stability analysis, and optimal control strategies, 2024, 99, 0031-8949, 075035, 10.1088/1402-4896/ad562c | |
12. | A. Venkatesh, M. Manivel, K. Arunkumar, M. Prakash Raj, S. D. Purohit, A fractional mathematical model for vaccinated humans with the impairment of Monkeypox transmission, 2024, 1951-6355, 10.1140/epjs/s11734-024-01211-5 | |
13. | Samuel Okyere, Joseph Ackora-Prah, Ebenezer Bonyah, Bennedict Barnes, Maxwell Akwasi Boateng, Ishmael Takyi, Samuel Akwasi Adarkwa, Hypertension and COVID-19 fractional derivative model with double dose vaccination, 2023, 12, 2046-1402, 495, 10.12688/f1000research.133768.1 | |
14. | Shashikant Waghule, Dinkar Patil, Amjad Shaikh, Kottakkaran Sooppy Nisar, Application of the Atangana–Baleanu operator in Caputo sense for numerical solutions of the time-fractional Burgers–Fisher equation using finite difference approaches, 2024, 12, 26668181, 100998, 10.1016/j.padiff.2024.100998 | |
15. | Ihtisham ul Haq, Nigar Ali, Hijaz Ahmad, Ramadan Sabra, M. Daher Albalwi, Imtiaz Ahmad, Mathematical analysis of a coronavirus model with Caputo, Caputo–Fabrizio–Caputo fractional and Atangana–Baleanu–Caputo differential operators, 2025, 18, 1793-5245, 10.1142/S1793524523500857 |
Parameters | Biological Interpretations |
δ | Recruitment rate for the susceptible population |
β′ | Rate of infection of S among Ia population |
β | Rate of infection of S among Is population |
ϵm | Effectiveness of face masks |
em | Masks compliance |
λ | Human mortality rate due to natural causes |
σ | Rate of incubation |
a | Fraction of those exposed who do not exhibit clinical symptoms |
ρa | Rate of progression from Ia to Is class |
ρh | Recovery rate for H individuals |
λs | Disease induced mortality rate for Is class |
τa | Q rate for Ia class |
τh | Recovery rate for R class |
λh | Disease induced mortality rate for H class |
ϕs | Rate of H for Is individuals |
Parameters | Biological Interpretations |
δ | Recruitment rate for the susceptible population |
β′ | Rate of infection of S among Ia population |
β | Rate of infection of S among Is population |
ϵm | Effectiveness of face masks |
em | Masks compliance |
λ | Human mortality rate due to natural causes |
σ | Rate of incubation |
a | Fraction of those exposed who do not exhibit clinical symptoms |
ρa | Rate of progression from Ia to Is class |
ρh | Recovery rate for H individuals |
λs | Disease induced mortality rate for Is class |
τa | Q rate for Ia class |
τh | Recovery rate for R class |
λh | Disease induced mortality rate for H class |
ϕs | Rate of H for Is individuals |
Name | Parameters values | References |
δ | 0.23 | Assumed |
β′ | 0.000516 | Assumed |
λs | 0.0062 | Assumed |
ϕs | 0.06 | [34] |
ρa | 0.07 | [34] |
εm | 0.5 | [35] |
em | 0.2 | [36] |
σ | 0.28 | [37] |
a | 0.66 | [37] |
λh | 0.0045 | Assumed |
λ | 0.000428 | Demographic |
τh | 1/14 | [38,39] |