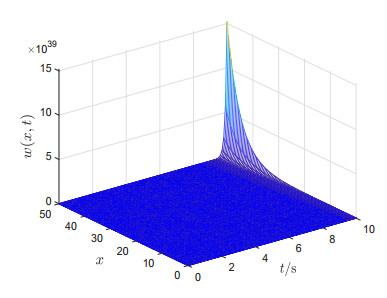
Citation: Weiguo Liu, Yan Jiang, Zhi Li. Rate of convergence of Euler approximation of time-dependent mixed SDEs driven by Brownian motions and fractional Brownian motions[J]. AIMS Mathematics, 2020, 5(3): 2163-2195. doi: 10.3934/math.2020144
[1] | Xiaowei Shao, Li Chen, Junli Chen, Dexin Zhang . Prescribed-time control for spacecraft formation flying with uncertainties and disturbances. AIMS Mathematics, 2024, 9(1): 1180-1198. doi: 10.3934/math.2024058 |
[2] | Lichao Feng, Mengyuan Dai, Nan Ji, Yingli Zhang, Liping Du . Prescribed-time stabilization of nonlinear systems with uncertainties/disturbances by improved time-varying feedback control. AIMS Mathematics, 2024, 9(9): 23859-23877. doi: 10.3934/math.20241159 |
[3] | Yihang Kong, Xinghui Zhang, Yaxin Huang, Ancai Zhang, Jianlong Qiu . Prescribed-time adaptive stabilization of high-order stochastic nonlinear systems with unmodeled dynamics and time-varying powers. AIMS Mathematics, 2024, 9(10): 28447-28471. doi: 10.3934/math.20241380 |
[4] | Bin Hang, Weiwei Deng . Finite-time adaptive prescribed performance DSC for pure feedback nonlinear systems with input quantization and unmodeled dynamics. AIMS Mathematics, 2024, 9(3): 6803-6831. doi: 10.3934/math.2024332 |
[5] | Yanxia Hu, Qian Liu . On traveling wave solutions of a class of KdV-Burgers-Kuramoto type equations. AIMS Mathematics, 2019, 4(5): 1450-1465. doi: 10.3934/math.2019.5.1450 |
[6] | Miao Xiao, Zhe Lin, Qian Jiang, Dingcheng Yang, Xiongfeng Deng . Neural network-based adaptive finite-time tracking control for multiple inputs uncertain nonlinear systems with positive odd integer powers and unknown multiple faults. AIMS Mathematics, 2025, 10(3): 4819-4841. doi: 10.3934/math.2025221 |
[7] | Xia Song, Lihua Shen, Fuyang Chen . Adaptive backstepping position tracking control of quadrotor unmanned aerial vehicle system. AIMS Mathematics, 2023, 8(7): 16191-16207. doi: 10.3934/math.2023828 |
[8] | Aceng Sambas, Ardashir Mohammadzadeh, Sundarapandian Vaidyanathan, Ahmad Faisal Mohamad Ayob, Amiral Aziz, Mohamad Afendee Mohamed, Ibrahim Mohammed Sulaiman, Mohamad Arif Awang Nawi . Investigation of chaotic behavior and adaptive type-2 fuzzy controller approach for Permanent Magnet Synchronous Generator (PMSG) wind turbine system. AIMS Mathematics, 2023, 8(3): 5670-5686. doi: 10.3934/math.2023285 |
[9] | Muhammad Usman, Hidayat Ullah Khan, Zareen A Khan, Hussam Alrabaiah . Study of nonlinear generalized Fisher equation under fractional fuzzy concept. AIMS Mathematics, 2023, 8(7): 16479-16493. doi: 10.3934/math.2023842 |
[10] | Feiting Fan, Xingwu Chen . Dynamical behavior of traveling waves in a generalized VP-mVP equation with non-homogeneous power law nonlinearity. AIMS Mathematics, 2023, 8(8): 17514-17538. doi: 10.3934/math.2023895 |
Recently, boundary control for uncertain systems described by wave equations with dynamic boundary has been extensively investigated (see e.g., [1,2,3,4,5,6,7,8,9,10,11,12,13] and references therein). However, most of the uncertainties considered in these works come from external disturbances [3,5,6,7,8,9,10,11] and constant parametric uncertainties [1,2,4,12,13], few uncertainties are about unknown spatially varying or time-varying parameters, which are inevitable in practice. It is worth pointing out that [14,15,16,17] studied the cases with unknown spatially varying parameters in the hyperbolic PDEs therein, but the control schemes are developed for first-order hyperbolic PDEs with static boundary conditions and are not applicable to the second-order hyperbolic PDEs with dynamic boundary conditions. Moreover, in [1,4,12,13], adaptive technique was utilized to compensate system uncertainties, but the control objectives achieved are relatively conservative. Specifically, the control objectives are reduced that the distributed states in the closed-loop system are ultimately uniformly bounded in the sense of certain norm in [1], and the closed-loop systems are stable in the sense of certain norms in [4,12,13] (in these works, the performance of the states of the systems cannot be derived from these norms). Therefore, when a parameter involved in the wave equation is spatially varying and unknown, how to compensate the serious parametric uncertainties and design a desired boundary controller to achieve delicate objective with prescribed performance are challenging problems and worthy of thorough investigation.
In this paper, we consider the boundary control for the following wave equation with dynamic boundary condition and multiple parametric uncertainties:
{wtt(x,t)=a(x)wxx(x,t)+a′(x)wx(x,t)−b(x)wt(x,t),wx(0,t)=qwt(0,t),wtt(L,t)=λa(L)wx(L,t)−ηwt(L,t)+1Mu(t), | (1.1) |
where w:[0,L]×R+→R is the system state; u:R+→R is the control input of the system; w(x,0)=w0(x) and wt(x,0)=w1(x) are the initial values of the system; a(x) is an increasing function satisfying a(0)≥L2; L is a positive constant denoting the length of the wave equation domain; M is a positive constant; b:[0,L]→R+ is an unknown spatially varying parameter, called in-domain damping coefficient; η is an unknown positive constant, called boundary damping coefficient; q and λ are unknown positive constants.
The objective of this paper is to design an adaptive boundary controller for system (1.1) with dynamic boundary and rather essential uncertainties, to guarantee that all states of the resulting closed-loop system are bounded, and the original system state ultimately converges to an arbitrary prescribed small neighborhood of the origin, that is, |w(x,t)|≤ε, ∀t≥Tfor someT>0, where ε is a positive constant representing the prescribed accuracy. To achieve the objective, the following assumption is imposed on the unknown parameters b(x), q, λ and η:
Assumption 1. There exist known constants b_, ¯b, q_, ¯q, λ_, ¯λ, η_ and ¯η such that
0<b_≤b(x)≤¯b,∀x∈[0,1],0<q_≤q≤¯q,λ_≤λ≤¯λ,η_≤η≤¯η. |
Boundary control has received considerable attention for system (1.1) and its variants in recent decades, since this class of systems has wide application background in engineering, such as crane systems [18,19], oilwell drillstring systems [1,20,21], sea cable problems [22,23] and cable elevators [24,25]. It is worth stressing that compared with the systems concerned in the above related literature, system (1.1) has two distinctive features: (i) Unknown in-domain damping coefficient and unknown boundary damping coefficient are allowed, and particularly, the in-domain damping coefficient is spatially varying. In fact, [1,18,19,24] rule out any dampings in the systems; [20,21,23,25] require that both the in-domain and boundary damping coefficients are known constants; [22] only allows that the boundary damping coefficient is unknown and constant. Remarkably, [26,27] investigated the more general cases where the in-domain damping coefficients are spatially varying, but the coefficients are required to be known. (ii) Dynamic boundary condition is considered in the present paper. This makes that the control schemes proposed by infinite-dimensional backstepping method in [14,15,16,17] (where unknown spatially varying parameters are allowed in the systems therein) cannot be applied to solve the control problem in the paper, since the explicit expression for the key kernel function in the infinite-dimensional backstepping method is rather difficult to construct in the presence of dynamic boundary condition [28,29]. Thus, one of the main contributions of the paper is that the system under discussion allows serious unknowns and is more practical than those in the existing related works.
This paper is devoted to developing an adaptive prescribed performance control scheme for system (1.1) with dynamic boundary condition, unknown in-domain spatially varying damping coefficient and unknown boundary constant damping coefficient. One key point in the control design is to construct the ingenious updating laws which not only can effectively counteract the serious unknowns but also are easily integrated with the control design for dynamic boundary condition. Specifically, adaptive technique based projection operator and backstepping method developed for ODEs (see e.g., [30,31,32]) are applied to compensate the multiple parametric uncertainties and simultaneously to design the desired controller, since the dynamic boundary condition at the control end can be written as a two-dimensional ODE system by letting X1(t)=w(L,t) and X2(t)=˙X1(t). Based on this, an adaptive controller is successfully designed in two steps, which guarantees that all states of the resulting closed-loop system are bounded, and furthermore the original system state ultimately converges to an arbitrarily prescribed small neighborhood of the origin. Therefore, the other main contributions of the paper is that a new adaptive control strategy, which is essentially different from those in [1,4,12,13], is proposed to achieve delicate control objective with prescribed convergence rate for uncertain systems described by wave equations with dynamic boundary condition.
The remainder of the paper proceeds as follows. In Section 2, the desired adaptive controller and dynamic compensators are designed. Section 3 summarizes the main results of the paper. Section 4 gives a simulation example, and Section 5 addresses some concluding remarks. The paper ends with a supplementary which gives several useful inequalities.
{Notations. Throughout the paper, R denotes the set of all real numbers; R+ denotes the set of all nonnegative real numbers; L2(0,L) denotes the space of all measurable functions on [0,L] with the property that ∫L0|f(x)|2dx<+∞; Hi(0,L) denotes the usual Sobolev space of functions in L2(0,L) with derivatives up to ith order also in L2(0,L).
This section is devoted to designing an appropriate adaptive controller for system (1.1). Particularly, ingenious updating laws and delicate Lyapunov function are constructed not only to deal with multiple parametric uncertainties but also to guarantee the stabilization with prescribed performance. Based on this, an adaptive controller is designed for system (1.1) by the idea of backstepping method for ODEs, since the boundary condition that the control u(t) involved in can be rewritten as a two-dimensional ODE system.
Letting X1(t)=w(L,t) and X2(t)=˙X1(t). Then, system (1.1) can be rewritten as follows:
{{wtt(x,t)=a(x)wxx(x,t)+a′(x)wx(x,t)−b(x)wt(x,t),wx(0,t)=qwt(0,t),w(L,t)=X1(t),{˙X1(t)=X2(t),˙X2(t)=λa(L)wx(L,t)−ηX2(t)+1Mu(t). | (2.1) |
We now design the adaptive controller by two steps using the backstepping method for ODEs.
Step 1. Consider the control design for the following subsystem peeled from (2.1):
{wtt(x,t)=a(x)wxx(x,t)+a′(x)wx(x,t)−b(x)wt(x,t),wx(0,t)=qwt(0,t),w(L,t)=X1(t),˙X1(t)=X2(t)−X∗2(t)+X∗2(t), | (2.2) |
where X∗2(t) is the control input of system (2.2).
Let
E1(t)=12∫L0(a(x)w2x(x,t)+w2t(x,t))dx. | (2.3) |
Then, for system (2.2), we introduce the following energy function:
V1(t)=E1(t)+X21(t). | (2.4) |
By the first equation in (2.2) and integration by parts, it can be easily verified that
˙V1(t)=∫L0(a(x)wx(x,t)wxt(x,t)+wt(x,t)wtt(x,t))dx+2X1(t)˙X1(t)=∫L0(a(x)wx(x,t)wxt(x,t)+wt(x,t)(a(x)wxx(x,t)+a′(x)wx(x,t)−b(x)wt(x,t)))dx+2X1(t)˙X1(t)=∫L0(a(x)wx(x,t)wxt(x,t)+(a(x)wx(x,t))xwt(x,t)−b(x)w2t(x,t))dx+2X1(t)˙X1(t)=(a(x)wx(x,t)wt(x,t))|L0−∫L0b(x)w2t(x,t)dx+2X1(t)˙X1(t)=a(L)wx(L,t)wt(L,t)−a(0)wx(0,t)wt(0,t)−∫L0b(x)w2t(x,t)dx+2X1(t)˙X1(t). |
Noting that w(L,t)=X1(t), wx(0,t)=qwt(0,t) and ˙X1(t)=X2(t)−X∗2(t)+X∗2(t) from (2.2), there holds
˙V1(t)=(X2(t)−X∗2(t)+X∗2(t))(a(L)wx(L,t)+2X1(t))−qa(0)w2t(0,t)−∫L0b(x)w2t(x,t)dx. | (2.5) |
We choose
X∗2(t)=−k1(a(L)wx(L,t)+2X1(t)), | (2.6) |
where k1 is a positive design parameter to be determined later. Then, substituting (2.6) into (2.5), and using Assumption 1, we arrive at
˙V1(t)≤(X2(t)−X∗2(t))(a(L)wx(L,t)+2X1(t))−k1(a(L)wx(L,t)+2X1(t))2−q_a(0)w2t(0,t)−b_∫L0w2t(x,t)dx. | (2.7) |
Step 2. To design an appropriate controller u(t) in this step, we define
E2(t)=∫L0(x−L)wx(x,t)wt(x,t)dx, |
by which, (2.3), Young's inequality and noting a(x)≥L2, we have
|E2(t)|≤12∫L0(L2w2x(x,t)+w2t(x,t))dx≤E1(t). | (2.8) |
Then, for the following entire system:
{{wtt(x,t)=a(x)wxx(x,t)+a′(x)wx(x,t)−b(x)wt(x,t),wx(0,t)=qwt(0,t),w(L,t)=X1(t),{˙X1(t)=˘X2(t)+X∗2(t),˙˘X2(t)=λa(L)wx(L,t)−ηwt(L,t)+1Mu(t)−˙X∗2(t), | (2.9) |
its energy function is constructed as
V2(t)=E1(t)+12X21(t)+12˘X22(t)+12γ1˜λ2(t)+12γ2˜η2(t)+δE2(t), | (2.10) |
where ˘X2(t)=X2(t)−X∗2(t); ˜λ(t)=λ−ˆλ(t) and ˜η(t)=η−ˆη(t) are the parameter estimate errors with ˆλ(t) and ˆη(t) being the estimates of unknown parameters λ and η, respectively; γ1, γ2 and δ<1 are positive constants to be determined later.
Following similar arguments to the proof of (2.7), and by (2.6) and (2.9), we obtain
˙V2(t)=(a(x)wx(x,t)wt(x,t))|L0−∫L0b(x)w2t(x,t)dx+X1(t)˙X1(t)+˘X2(t)(λa(L)wx(L,t)−ηwt(L,t)+1Mu(t)−˙X∗2(t))−1γ1˜λ(t)˙ˆλ(t)−1γ2˜η(t)˙ˆη(t)+δ˙E2(t)=(˘X2(t)+X∗2(t))(a(L)wx(L,t)+X1(t))−qa(0)wt2(0,t)−∫L0b(x)w2t(x,t)dx+˘X2(t)(λa(L)wx(L,t)−ηwt(L,t)+1Mu(t)+k1(a(L)wxt(L,t)+2˙X1(t)))−1γ1˜λ(t)˙ˆλ(t)−1γ2˜η(t)˙ˆη(t)+δ˙E2(t)≤˘X2(t)(a(L)wx(L,t)+X1(t))−k1(a(L)wx(L,t)+X1(t))2−k1X1(t)a(L)wx(L,t)−k1X21(t)−q_a(0)wt2(0,t)−b_∫L0w2t(x,t)dx+˘X2(t)(ˆλ(t)a(L)wx(L,t)−ˆη(t)wt(L,t)+1Mu(t)+k1(a(L)wxt(L,t)+2˙X1(t)))+˜λ(t)(a(L)wx(L,t)˘X2(t)−1γ1˙ˆλ(t))−˜η(t)(wt(L,t)˘X2(t)+1γ2˙ˆη(t))+δ˙E2(t). | (2.11) |
Then, by Young's inequality, there holds
{|˘X2(a(L)wx(L,t)+X1(t))|≤c02(a(L)wx(L,t)+X1(t))2+12c0˘X22(t),k1|X1(t)a(L)wx(L,t)|≤k12(a(L)wx(L,t)+X1(t))2, | (2.12) |
where c0 is a positive constant to be determined later. Substituting (2.12) into (2.11) yields
˙V2(t)≤−k1−c02(a(L)wx(L,t)+X1(t))2+12c0˘X22(t)−k1X21(t)−q_a(0)wt2(0,t)−b_∫L0w2t(x,t)dx+˘X2(t)(ˆλ(t)a(L)wx(L,t)−ˆη(t)wt(L,t)+1Mu(t)+k1(a(L)wxt(L,t)+2˙X1(t)))+˜λ(t)(a(L)wx(L,t)˘X2(t)−1γ1˙ˆλ(t))−˜η(t)(wt(L,t)˘X2(t)+1γ2˙ˆη(t))+δ˙E2(t). | (2.13) |
For the last term on the right-hand side of (2.13), by Assumption 1, integration by parts, Young's inequality and the increasing property of a(x), it can be derived similar to the proof of the equality above (A3) in Lemma 2.1 in [10] that
˙E2(t)=12Lw2t(0,t)−12∫L0w2t(x,t)dx+12La(0)w2x(0,t)+12∫L0(x−L)a′(x)w2x(x,t)dx−12∫L0a(x)w2x(x,t)dx−∫L0(x−L)b(x)wx(x,t)wt(x,t)dx≤−12∫L0w2t(x,t)dx−12∫L0(a(x)−(x−L)a′(x))w2x(x,t)dx+12L(1+q2a(0))w2t(0,t)+c1¯b2∫L0L2w2x(x,t)dx+¯b2c1∫L0w2t(x,t)dx≤−12(1−¯bc1)∫L0w2t(x,t)dx−1−c1¯b2∫L0a(x)w2x(x,t)dx+12L(1+¯q2a(0))w2t(0,t), | (2.14) |
where 0<c1<1¯b.
Substituting (2.14) into (2.13) results in
˙V2(t)≤−k1−c02(a(L)wx(L,t)+X1(t))2+12c0˘X22(t)−k1X21(t)−(b_+δ2(1−¯bc1))∫L0w2t(x,t)dx−δ2(1−c1¯b)∫L0a(x)w2x(x,t)dx+˘X2(t)(ˆλ(t)a(L)wx(L,t)−ˆη(t)wt(L,t)+1Mu(t)+k1(a(L)wxt(L,t)+2˙X1(t)))+˜λ(t)(a(L)wx(L,t)˘X2(t)−1γ1˙ˆλ(t))−˜η(t)(wt(L,t)˘X2(t)+1γ2˙ˆη(t))−(q_a(0)−δL2(1+¯q2a(0)))w2t(0,t). |
By choosing
{0<c0≤k1,0<δ<σ={min{2q_a(0)L(1+¯q2a(0)),2L3−3L2−1(1+2L)L2},¯b≤c1<1¯b,min{2b_c1¯b−c1,2q_a(0)L(1+¯q2a(0)),2L3−3L2−1(1+2L)L2},¯b≥1,or0<¯b<1and0<c1<¯b, | (2.15) |
there holds
˙V2(t)≤12c0˘X22(t)−k1X21(t)−(b_+δ2(1−¯bc1))∫L0w2t(x,t)dx−δ2(1−c1¯b)∫L0a(x)w2x(x,t)dx+˘X2(t)(ˆλ(t)a(L)wx(L,t)−ˆη(t)wt(L,t)+1Mu(t)+k1(a(L)wxt(L,t)+2˙X1(t)))+˜λ(t)(a(L)wx(L,t)˘X2(t)−1γ1˙ˆλ(t))−˜η(t)(wt(L,t)˘X2(t)+1γ2˙ˆη(t)). | (2.16) |
To ensure the desired stability of the resulting closed-loop system, the adaptive controller and the updating laws of ˆλ(t) and ˆη(t) are designed as follows:
u(t)=−k1M(a(L)wxt(L,t)+2˙X1(t))−k2M˘X2(t)−Ma(L)ˆλ(t)wx(L,t)+Mˆη(t)wt(L,t)=−k1M(a(L)wxt(L,t)+2wt(L,t))−k2M(wt(L,t)+k1(a(L)wx(L,t)+2w(L,t)))−Ma(L)ˆλ(t)wx(L,t)+Mˆη(t)wt(L,t), | (2.17) |
and
{˙ˆλ(t)=Proj[λ_,¯λ]{γ1a(L)wx(L,t)˘X2(t),ˆλ(t)},ˆλ(0)∈[λ_,¯λ],˙ˆη(t)=Proj[η_,¯η]{−γ2wt(L,t)˘X2(t),ˆη(t)},ˆη(0)∈[η_,¯η], | (2.18) |
where k2 is a design parameter satisfying k2>12c0; γi (i=1,2) are positive constants to be determined later, called adaptation gains; Proj[⋅,⋅]{⋅,⋅} is a projection operator defined as
Proj[θ_,¯θ]{τ,ˆθ}={0,ˆθ=θ_andτ<0,0,ˆθ=¯θandτ>0,τ,else. | (2.19) |
It is worth pointing out that the projection operator defined by (2.19) possesses some nice properties presenting in the following Lemma, which will play an important role in the stability analysis in Section 3, especially in guaranteeing the convergence of the system state.
Lemma 1. [15,30] For projection operator defined by (2.19), there hold
(i) For ˆθ(0)∈[θ_,¯θ], the solution of ˙ˆθ=Proj[θ_,¯θ]{τ,ˆθ} remains in [θ_,¯θ];
(ii) If θ_≤ˆθ≤¯θ and θ_≤θ≤¯θ, then −˜θProj[θ_,¯θ]{τ,ˆθ}≤−˜θτ.
Substituting (2.17) and (2.18) into (2.16), and then using claims (i) and (ii) in Lemma 1, it follows that
˙V2(t)≤−k1X21(t)−(k2−12c0)˘X22(t)−(b_+δ2(1−¯bc1))∫L0w2t(x,t)dx−δ2(1−c1¯b)∫L0a(x)w2x(x,t)dx. | (2.20) |
This completes the design procedure of the desired adaptive controller.
In this section, we aim to analyze the stability of the closed-loop system resulting from (1.1), (2.17) and (2.18), which is summarized in the following theorem.
Theorem 1. Consider system (1.1) under Assumption 1. If the positive design parameters ki's and γj's are chosen to satisfy k1≥c0, k2>12c0 and 1γ1(λ_−¯λ)2+1γ2(η_−¯η)2≤ε24(1+2L) with c0 being a certain positive constant and ε being an arbitrary pre-specified positive constant. Then, the adaptive controller described by (2.17) and (2.18) guarantees that for any initial values w0(x)∈H1(0,L), w1(x)∈L2(0,L), ˆλ(0)∈[λ_,¯λ] and ˆη(0)∈[η_,¯η], the resulting closed-loop system state w(x,t) is bounded on [0,L]×[0,+∞) while ˆλ(t) and ˆη(t) are bounded on [0,+∞), and furthermore,
{limt→∞supx∈[0,L]|w(x,t)|≤ε,x∈[0,L],ˆλ(t)∈[λ_,¯λ],ˆη(t)∈[η_,¯η],t∈[0,+∞). | (3.1) |
Proof. By Assumption 1, claim (i) of Lemma 1, ˆλ(0)∈[λ_,¯λ] and ˆη(0)∈[η_,¯η], we can see that ˆλ(t)∈[λ_,¯λ],ˆη(t)∈[η_,¯η] on [0,+∞), and hence the boundedness of ˆλ(t) and ˆη(t) on [0,+∞) as well as the last relation in (3.1) are proved.
We next prove that for x∈[0,L] and an arbitrary pre-specified positive constant ε, the system state w(x,t) satisfies limt→∞supx∈[0,L]|w(x,t)|≤ε. By (2.20) and denoting l=min{2b_+δ(1−¯bc1),δ(1−c1¯b)}, there holds
˙V2(t)≤−lE1(t)−k1X21(t)−(k2−12c0)˘X22(t). |
From (2.8), we have E1(t)+δE2(t)≤(1+δ)E1(t). This, together with (2.10), results in
˙V2(t)≤−l1+δ(E1(t)+δE2(t))−k1X21(t)−(k2−12c0)˘X22(t)≤−ϱV2(t)+ϱ2γ1˜λ2(t)+ϱ2γ2˜η2(t), |
where ϱ=min{l1+δ,2k1,2k2−1c0}. From this, it can be deduced that
V2(t)≤V2(0)e−ϱt+12γ1(λ_−¯λ)2+12γ2(η_−¯η)2. | (3.2) |
By (2.10), (3.2) and w(L,t)=X1(t), we have
|w(L,t)|2≤2V2(0)e−ϱt+1γ1(λ_−¯λ)2+1γ2(η_−¯η)2. |
Noting that there exists a sufficiently large T, such that for t∈[T,+∞), there holds
|V2(0)e−ϱt|≤ε28(1+2L), | (3.3) |
which, together with
1γ1(λ_−¯λ)2+1γ2(η_−¯η)2≤ε24(1+2L), | (3.4) |
implies
lim supt→+∞|w(L,t)|2≤ε22(1+2L). | (3.5) |
On the other hand, by (2.8), there holds (1−δ)E1(t)≤E1(t)+δE2(t), which together with (3.2) yields
(1−δ)E1(t)≤V2(t)≤V2(0)e−ϱt+12γ1(λ_−¯λ)2+12γ2(η_−¯η)2. |
Thus, by (3.3) and (3.4), we can prove that
lim supt→+∞∫L0a(x)w2x(x,t)dx≤ε22(1−δ)(1+2L). | (3.6) |
Then, by Agmon's inequality (see Lemma A.1 in Supplementary), we get
|w(x,t)|2≤|w(L,t)|2+2√∫L0w2(x,t)dx√∫L0w2x(x,t)dx, |
which, together with Poincaré's inequality (see Lemma A.2 in Supplementary), Young's inequality and a(x)≥L2, results in
|w(x,t)|2≤|w(L,t)|2+2√∫L0w2x(x,t)dx√2Lw2(L,t)+4L2∫L0w2x(x,t)dx≤(1+2L)|w(L,t)|2+(1+4L2)L2∫L0a(x)w2x(x,t)dx. | (3.7) |
Substituting (3.5) and (3.6) into (3.7), we can deduce that
limt→∞supx∈[0,L]|w(x,t)|2≤ε22+(1+4L2)ε22L2(1−δ)(1+2L),x∈[0,L]. |
Then, noting from (2.15), there holds
δ<2L3−3L2−1(1+2L)L2, |
from which, it follows that
limt→∞supx∈[0,L]|w(x,t)|2≤ε2,x∈[0,L]. |
Thus, we achieve the first relation in (3.1). This completes the proof of the theorem.
Remark 1. From (3.2), we can see the necessity of introducing the term −2k1X1(t) in (2.6). Actually, if −2k1X1(t) is replaced by −k1X1(t), the term −k1X1(t)a(L)wx(L,t)−k1X21(t) is no longer appears in (2.11). Then, by (2.20), inequality (3.2) becomes V2(t)≤V2(0). By which, (2.10), Poincaré's inequality and Agmon's inequality, we can see that only the boundedness of w(x,t), ˆλ(t) and ˆη(t) can be derived. Thus, when −2k1X1(t) is changed into −k1X1(t), the key result limt→∞supx∈[0,L]|w(x,t)|≤ε of the paper, that is, the original system state ultimately converges to an arbitrary prescribed small neighborhood of the origin can not be established.
Remark 2. It is worth pointing out that for unknown parameters b(x) and q, their updating laws can be explicitly constructed, although it is enough to guarantee the main results of the paper stated in Theorem 1 only by using their upper and lower bounds. In fact, when (2.4) is replaced by the following one:
V1(t)=E1(t)+X21(t)+12ϑ1∫L0˜b2(x,t)dx+12ϑ2˜q2(t), |
where ˜b(x,t)=b(x)−ˆb(x,t) and ˜q(t)=q−ˆq(t) with ˆb(x,t) and ˆq(t) being the estimates of unknown parameters b(x) and q, respectively, ϑ1 and ϑ2 are two positive constants to be determined later, then by choosing
{ˆbt(x,t)=Proj[b_,¯b]{−ϑ1w2t(x,t),ˆb(x,t)},ˆb(x,0)∈[b_,¯b],˙ˆq(t)=Proj[q_,¯q]{−ϑ2a(0)w2t(0,t),ˆq(t)},ˆq(0)∈[q_,¯q], |
and using claims (i), (ii) in Lemma 1, it follows that
˙V1(t)=(X2(t)−X∗2(t)+X∗2(t))(a(L)wx(L,t)+2X1(t))−∫L0ˆb(x,t)w2t(x,t)dx−∫L0˜b(x,t)(1ϑ1ˆbt(x,t)+w2t(x,t))dx−a(0)ˆq(t)w2t(0,t)−˜q(t)(1ϑ2˙ˆq(t)+a(0)w2t(0,t))≤(X2(t)−X∗2(t))(a(L)wx(L,t)+2X1(t))−k1(a(L)wx(L,t)+2X1(t))2−∫L0ˆb(x,t)w2t(x,t)dx−a(0)ˆq(t)w2t(0,t). |
Thus, by Assumption 1 and claim (i) in Lemma 1, we can see that (2.7) still holds, by which and a similar argument as in deriving limt→∞supx∈[0,L]|w(x,t)|≤ε, it follows that the main results as stated in Theorem 1 also can be established by choosing 1ϑ1(b_−¯b)2+1ϑ2(q_−¯q)2+1γ1(λ_−¯λ)2+1γ2(η_−¯η)2≤ε24(1+2L).
This section is devoted to validate the effectiveness of the theoretical results for system (1.1). From references [18,19], it can be seen that system (1.1) models a motorized platform moving along an horizontal bench with a flexible cable attaching to the platform and holding a load mass, the state/solution w(x,t) is the horizontal displacement at time t of the point whose curvilinear abscissa is x denoting the arc length along the cable, and control u(t) is the force applied by the motor to the platform, seeing [18,19] for more detailed explanations.
To design the desired adaptive controller, the parameters of the system are given as follows: L=50m, M=20kg, a(x)=gx+gmρ with m=1500kg, ρ=5kg/m and g=9.8m/s2. Obviously, a(x)=9.8x+2940 is an increasing function satisfying a(0)=2940≥L2=2500. The initial conditions w0(x) and w1(x) are respectively chosen as
w0(x)=w(x,0)=2.5cos(100πx)−1.05, |
and
w1(x)=wt(x,0)={−12.75,0≤x≤L2,0,else. |
The unknown parameters b(x),q,λ and η are supposed to be b(x)=x15+2.15, q=0.01, λ=0.25 and η=7.5, respectively. Then, we assume that b_=0.1, ¯b=6, q_=0.001, ¯q=2, λ_=0.01, ¯λ=0.6, η_=5 and ¯η=9. Clearly, system (1.1) satisfies Assumption 1.
Choose the design parameters k1=0.25, k2=52, γ1=5.75×104 and γ2=2.0×106. Obviously, k1≥c0>0 and k2>12c0 by letting c0=0.2. Moreover, set ε=0.1, it can be verified that 1γ1(λ_−¯λ)2+1γ2(η_−¯η)2=0.1405×10−4<ε24(1+2L)=0.25×10−4. Then, by (2.17), (2.18) and choosing the initial conditions ˆλ(0)=0.3∈[0.01,0.6] and ˆη(0)=5.75∈[5,9], the following adaptive controller is designed for system (1.1):
u(t)=−5(3430wxt(L,t)+2wt(L,t))−1040(wt(L,t)+0.25(3430wx(L,t)+2w(L,t)))−68600ˆλ(t)wx(L,t)+20ˆη(t)wt(L,t), |
with ˆλ(t) and ˆη(t) being given by
{˙ˆλ(t)=Proj[λ_,¯λ]{1.97225×108wx(L,t)˘X2(t),ˆλ(t)},˙ˆη(t)=Proj[η_,¯η]{−2.0×106wt(L,t)˘X2(t),ˆη(t)}, |
˘X2(t)=wt(L,t)+0.25(3430wx(L,t)+2w(L,t)).
By using the implicit backward Euler method and explicit central difference method (see e.g., Pages 407 and 415 of [34], respectively) with the grid sizes are taken as Nx=100 and Nt=50000 in MATLAB software, four figures are obtained for the resulting closed-loop system signals. Specifically, Figure 1 shows the trajectory of the original system state w(x,t) with u(t)=0, from which we can see that the value of w(x, t) becomes very large as time increases; Figure 2 shows the trajectory of the original system state w(x,t), from which we can see that w(x,t) ultimately converges to an arbitrarily small neighborhood of the origin. Figures 3 and 4 show the trajectories of the estimates of unknown parameters λ and η, respectively, from which we can see that ˆλ(t)∈[0.01,0.6] and ˆη(t)∈[5,9].
In this paper, an adaptive prescribed performance control scheme has been developed for wave equations with dynamic boundary condition and multiple parametric uncertainties. To deal with these uncertainties, adaptive technique based projection operator is applied to construct the corresponding compensation mechanisms, which are integrated with the control design for dynamic boundary. Compared with the related literature, our design scheme can achieve the desired control objective, while dealing with rather serious parametric uncertainties. Remark that in this paper, the lower and upper bounds of the unknown parameters b(x), q, λ and η are required to be known, and time varying parametric uncertainties are excluded in the considered system, hence, one of the future work is to consider the case with more essential uncertainties, such as b(x), q, λ and η with unknown bounds or being unknown time varying parameters. Moreover, only one-dimensional wave equation is considered and b(x) is required to be positive for x∈[0,L] in the system in question, another future work is to study the case for PDE system described by multi-dimensional wave equation with b(x) being negative for x∈[0,L] (i.e., anti-damping case). Besides these, we shall explore how to achieve a further result that the original system state converges to zero for wave equations with serious uncertainties and how to generalize the proposed control scheme for wave equation to other PDEs, such as KdV and DMBBM equations presented in [35,36].
The authors declare they have not used Artificial Intelligence (AI) tools in the creation of this article.
This work was supported by the National Natural Science Foundations of China (Grant Nos. 62003197 and 61773332).
The authors declare that they have no conflict of interest.
Useful inequalities
The following two lemmas provide several useful inequalities. The proofs of these two lemmas are similar to those of Lemmas B.1 and B.2 in [33] and hence are omitted here.
Lemma A.1. (Agmon's Inequality) For any w∈H1(0,L), the following inequalities hold:
{maxx∈[0,L]w2(x)≤w2(0)+2√∫L0w2(x)dx∫L0w2x(x)dx,maxx∈[0,L]w2(x)≤w2(L)+2√∫L0w2(x)dx∫L0w2x(x)dx. |
Lemma A.2. (Poincaré's Inequality) For any w∈H1(0,L), the following inequalities hold:
{∫L0w2(x)dx≤2Lw2(0)+4L2∫L0w2x(x)dx,∫L0w2(x)dx≤2Lw2(L)+4L2∫L0w2x(x)dx. |
[1] | X. Mao, Stochastic Differential Equations and Applications, Chichester, UK: Horwood, 1997. |
[2] |
P. Guasoni, No arbitrage with transaction costs, with fractional Brownian motion and beyond, Math. Financ., 16 (2006), 569-582. doi: 10.1111/j.1467-9965.2006.00283.x
![]() |
[3] | A. A. Kilbas, H. M. Srivastava, J. J. Trujillo, Theory and applications of fractional differential equations, Amsterdam: Elsevier Science B.V., 2006. |
[4] | Zh. Li, W. Zhan, L. Xu, Stochastic differential equations with time-dependent coefficients driven by fractional Brownian motion, Physica A, Volume 530, 15 September 2019, 121565. |
[5] |
G. Denk, R. Winkler, Modelling and simulation of transient noise in circuit simulation, Math. Comp. Model. Dynm., 13 (2007), 383-394. doi: 10.1080/13873950500064400
![]() |
[6] |
S. C. Kou, Stochastic modeling in nanoscale biophysics: subdiffusion within proteins, Ann. Appl. Stat., 2 (2008), 501-535. doi: 10.1214/07-AOAS149
![]() |
[7] |
F. E. Benth, On arbitrage-free pricing of weather derivatives based on fractional Brownian motion, Appl. Math. Financ., 10 (2003), 303-324. doi: 10.1080/1350486032000174628
![]() |
[8] |
P. Cheridito, Mixed fractional Brownian motion, Bernoulli, 7 (2001), 913-934. doi: 10.2307/3318626
![]() |
[9] |
J. L. da Silva, M. Erraoui, E. H. Essaky, Mixed stochastic differential equations: Existence and uniqueness result, J. Theor. Probab., 31 (2018), 1119-1141. doi: 10.1007/s10959-016-0738-9
![]() |
[10] | Y. Krvavych, Yu. Mishura, Exponential formula and Girsanov theorem for mixed semilinear stochastic differential equations, Birkhäuser Verlag Basel/Switzerland: Trends in Mathematics, 2001. |
[11] |
Yu. S. Mishura, S. V. Posashkova, Stochastic differential equations driven by a Wiener process and fractional Brownian motion: Convergence in Besov space with respect to a parameter, Comput. Math. Appl., 62 (2011), 1166-1180. doi: 10.1016/j.camwa.2011.02.032
![]() |
[12] |
G. Shevchenko, T. Shalaiko, Malliavin regularity of solutions to mixed stochastic differential equations, Stat. Probabil. Lett., 83 (2013), 2638-2646. doi: 10.1016/j.spl.2013.08.013
![]() |
[13] |
J. Guerra, D. Nualart, Stochastic differential equations driven by fractional Brownian motion and standard Brownian motion, Stoch. Anal. Appl., 26 (2008), 1053-1075. doi: 10.1080/07362990802286483
![]() |
[14] | Yu. S. Mishura, G. M. Shevchenko, Mixed stochastic differential equations with long-range dependence: Existence, uniqueness and convergence of solutions, Comput. Math. Appl., 64 (2012), 3217-3227. |
[15] |
W. G. Liu, J. W. Luo, Modified Euler approximation of stochastic differential equation driven by Brownian motion and fractional Brownian motion, Commun. Stat. Theor. M., 46 (2017), 7427-7443. doi: 10.1080/03610926.2016.1152487
![]() |
[16] | Yu. S. Mishura, G. M. Shevchenko, Rate of convergence of Euler approximation of solution to mixed stochastic differential equation involving Brownian motion and fractional Brownian motion, Rand. Opera. Stoch. Equ., 19 (2011), 387-406. |
[17] | D. Nualart, A. Răşcanu, Differential equation driven by fractional Brownian motion, Collect. Math., 53 (2002), 55-81. |
[18] |
Yu. S. Mishura, G. M. Shevchenko, The rate of convergence for Euler approximations of solutions of stochastic differential equations driven by fractional Brownian motion, Stochastics, 80 (2008), 489-511. doi: 10.1080/17442500802024892
![]() |
[19] |
G. Shevchenko, Mixed fractional stochastic differential equations with jumps, Stochastics, 86 (2014), 203-217. doi: 10.1080/17442508.2013.774404
![]() |
[20] | P. E. Kloeden, E. Platen, Numerical Solution of Stochastic Differential Equations, Berlin: Springer, 1992. |
[21] | S. G. Samko, A. A. Kilbas, O. I. Marichev, Fractional integrals and derivatives, In: Theory and Applications, Gordon and Breach Science Publishers, Yvendon, xxxvi+976 pp. ISBN: 2-88124-864-0, 1993. |
[22] |
L. C. Young, An inequality of Hölder type connected with Stieltjes integration, Acta. Math. Djursholm, 67 (1936), 251-282. doi: 10.1007/BF02401743
![]() |
[23] |
M. Zähle, Integration with respect to fractal functions and stochastic calculus I, Probab. Theory. Rel., 111 (1998), 333-374. doi: 10.1007/s004400050171
![]() |
[24] | Yu. S. Mishura, G. M. Shevchenko, Existence and uniqueness of the solution of stochastic differential equation involving wiener process and fractional Brownian motion with Hurst Index H > 1/2, Commun. Stat. Theor. M., 40 (2011), 3492-3508. |
[25] |
Y. Z. Hu, K. Le, A multiparameter Garsia-Rodemich-Rumsey inequality and some applications, Stoch. Proc. Appl., 123 (2013), 3359-3377. doi: 10.1016/j.spa.2013.04.019
![]() |
[26] |
E. Alòs, D. Nualart, Stochastic integration with respect to the fractional Brownian motion, Stoch. Stoch. Rep., 75 (2003), 129-152. doi: 10.1080/1045112031000078917
![]() |
[27] |
D. Nualart, B. Saussereau, Malliavin calculus for stochastic differential equations driven by a fractional Brownian motion, Stoch. Proc. Appl., 119 (2009), 391-409. doi: 10.1016/j.spa.2008.02.016
![]() |
[28] |
A. Neuenkirch, I. Nourdin, Exact rate of convergence of some approximation schemes associated to SDEs driven by a fractional Brownian motion, J. Theor. Probab., 20 (2007), 871-899. doi: 10.1007/s10959-007-0083-0
![]() |
[29] | S. Cambanis, Y. Hu, Exact convergence rate of the Euler-Maruyama scheme, with application to sampling design, Stoch. Stoch. Rep., 59 (1996), 211-240. |
[30] |
G. M. J. Schoenmakers, P. E. Kloeden, Robust option replication for a Black-Scholes model extended with nondeterministic trends, J. Appl. Math. Stoch. Analy., 12 (1999), 113-120. doi: 10.1155/S104895339900012X
![]() |