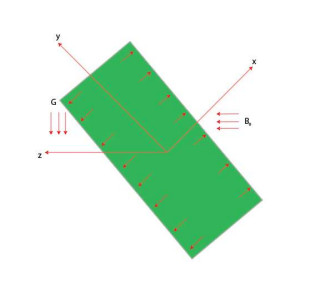
Citation: Asit Dey, Tapan Senapati, Madhumangal Pal, Guiyun Chen. A novel approach to hesitant multi-fuzzy soft set based decision-making[J]. AIMS Mathematics, 2020, 5(3): 1985-2008. doi: 10.3934/math.2020132
[1] | Taqi A. M. Shatnawi, Nadeem Abbas, Wasfi Shatanawi . Comparative study of Casson hybrid nanofluid models with induced magnetic radiative flow over a vertical permeable exponentially stretching sheet. AIMS Mathematics, 2022, 7(12): 20545-20564. doi: 10.3934/math.20221126 |
[2] | Kiran Sajjan, N. Ameer Ahammad, C. S. K. Raju, M. Karuna Prasad, Nehad Ali Shah, Thongchai Botmart . Study of nonlinear thermal convection of ternary nanofluid within Darcy-Brinkman porous structure with time dependent heat source/sink. AIMS Mathematics, 2023, 8(2): 4237-4260. doi: 10.3934/math.2023211 |
[3] | Adnan, Waseem Abbas, Sayed M. Eldin, Mutasem Z. Bani-Fwaz . Numerical investigation of non-transient comparative heat transport mechanism in ternary nanofluid under various physical constraints. AIMS Mathematics, 2023, 8(7): 15932-15949. doi: 10.3934/math.2023813 |
[4] | Abdul Rauf, Nehad Ali Shah, Aqsa Mushtaq, Thongchai Botmart . Heat transport and magnetohydrodynamic hybrid micropolar ferrofluid flow over a non-linearly stretching sheet. AIMS Mathematics, 2023, 8(1): 164-193. doi: 10.3934/math.2023008 |
[5] | Nadeem Abbas, Wasfi Shatanawi, Taqi A. M. Shatnawi . Innovation of prescribe conditions for radiative Casson micropolar hybrid nanofluid flow with inclined MHD over a stretching sheet/cylinder. AIMS Mathematics, 2025, 10(2): 3561-3580. doi: 10.3934/math.2025164 |
[6] | Yasir Khan, Sohaib Abdal, Sajjad Hussain, Imran Siddique . Numerical simulation for thermal enhancement of H2O + Ethyl Glycol base hybrid nanofluid comprising GO+(Ag,AA7072,MoS2) nano entities due to a stretched sheet. AIMS Mathematics, 2023, 8(5): 11221-11237. doi: 10.3934/math.2023568 |
[7] | Subhan Ullah, Hassan Ali Ghazwani, Dolat Khan, Zareen A. Khan . Heat transfer augmentation of Jeffery–Hamel hybrid nanofluid in a stretching convergent/divergent channel through porous medium. AIMS Mathematics, 2025, 10(1): 388-402. doi: 10.3934/math.2025018 |
[8] | Kiran Sajjan, Nehad Ali Shah, N. Ameer Ahammad, C.S.K. Raju, M. Dinesh Kumar, Wajaree Weera . Nonlinear Boussinesq and Rosseland approximations on 3D flow in an interruption of Ternary nanoparticles with various shapes of densities and conductivity properties. AIMS Mathematics, 2022, 7(10): 18416-18449. doi: 10.3934/math.20221014 |
[9] | Haifaa Alrihieli, Musaad S. Aldhabani, Ghadeer M. Surrati . Enhancing the characteristics of MHD squeezed Maxwell nanofluids via viscous dissipation impact. AIMS Mathematics, 2023, 8(8): 18948-18963. doi: 10.3934/math.2023965 |
[10] | S. R. Mishra, Subhajit Panda, Mansoor Alshehri, Nehad Ali Shah, Jae Dong Chung . Sensitivity analysis on optimizing heat transfer rate in hybrid nanofluid flow over a permeable surface for the power law heat flux model: Response surface methodology with ANOVA test. AIMS Mathematics, 2024, 9(5): 12700-12725. doi: 10.3934/math.2024621 |
List of symbols:
W,V,U | Velocity components (ms−1) | y,x,z | Space coordinates (m) |
ν | Kinematic viscosity (m2s−1) | β | Casson fluid number |
ρ | Fluidic density (Kgm−3) | βi,βe | Ion slip and Hall parameters |
T | Temperature (K) | T∞ | Ambient temperature (K) |
μ | Viscosity (Kgm−1s−1) | G | Gravitational acceleration (N) |
β,γ | Buoyancy parameters | B0 | Magnetic induction (A.Kgs−2) |
σ | Electrical conductivity (Sm−1) | n | Power law index number |
a,b | Constants | Tw | Wall temperature (K) |
η | Independent variable | Pr | Prandtl number |
M | Magnetic number | ϕ3,ϕ1,ϕ2 | Volume fractions |
λ | Stretching ratio number | Cf | Skin friction coefficient |
Nu | Nusselt number | TH | Tri-hybrid nanofluid |
bf | Base fluid | EG | Ethylene glycol |
Al2O3 | Aluminum oxide | SiO2 | Silicon dioxide |
w1,w3,w2,w4,w5 | Weight functions | ∞ | Infinity |
K | Thermal conductivity (Wm−1) | PDEs | Partial differential equations |
ODEs | Ordinary differential equations | sp1,sp3,sp2 | Solid nanoparticles |
θω | Temperature ratio number | Nr | Thermal radiation number |
ϵ | Porosity parameter (m2) | fs | Inertia coefficient |
k1 | Permeability of porous (m2) | Fr | Forchheirmer number |
σ∗,k∗ | Stefan-Boltzmann constants | α | Thermal diffusivity (m2s−1) |
Nanofluid is an engineered colloidal mixture regarding base fluid with single nanoparticles. Such behavior of fluid is applicable in cooling process, thermal process, thermal enhancement, thermal reduction and microelectronics. Deep exploration regarding case of mixture of base fluid with two kind's nanoparticles is termed as hybrid nanofluid. Moreover, Casson fluid behaves like a non-Newtonian liquid and shear thinning. Such type of non-Newtonian rheology is applicable in petroleum products, syrup drugs, production of plastic materials and engineering field etc. Rheology related to Casson fluid was investigated by Casson [1]. He has investigated that Casson model displays role like a shear thinning, plastic liquid model and high shear viscosity. Chabani et al. [2] discussed features of hybrid nanoparticles involving Lorentz force in triangular enclosure. They have studied convective flow considering the Darcy Forchheirmer model and included that flow is reduced when Lorentz force is implemented. Chu et al. [3] investigated a study related to hybrid nanofluid in the presence of magnetohydrodynamics for unsteady flow in two parallel plates including shape effects. They considered engine oil as base fluid and model is numerical solved by numerical scheme. Selimefendigil et al. [4] studied the role of various shapes regarding nanoparticles in ventilated cavities considering artificial neural networks. They implemented a finite element approach to find numerical results. It was included that maximum temperature was obtained using L-shaped cavity. Saleem et al. [5] estimated performance of several shapes of nanoparticles in horizontal surface considering base fluid (water). They have used a shooting approach to find parametric study on temperature and flow profiles. It was established that mass transport rate is enhanced versus erratic motion. Saleem et al. [6] established modeling related to Eyring-Powell material considering magnetized effect in mass species and heat transfer involving chemical reaction using finite element approach. Algehyne et al. [7] studied thermal features of Maxwell fluid in hybrid particles over a stretching frame. They have utilized finite element approach to find computational study and parametric study. It was investigated that highest thermal performance can be achieved by hybrid nanofluid rather than nanofluid. Imran et al. [8] performed thermal aspects of the solar collector using approach of hybrid nanoparticles via several shape effects implementing a numerical approach. Several studies regarding hybrid nanofluid are mentioned in [9]. Khan et al. [10] discussed features of Lorentz force in Casson fluid inserting dust particles along with hybrid nanoparticles past a stretchable sheet using an analytic approach.
Ternary hybrid nanofluid is a mixture of three kinds of nanoparticles in base fluid. It is an efficient approach to achieve the highest temperature rather than nanofluid and hybrid nanofluid. The process regarding tri-hybrid nanoparticles is most efficient for development of thermal enhancement and thermal reduction. Sarada et a. [11] estimated enhancement process in energy efficient using applications of ternary hybrid nanoparticles in curved stretching sheet implementing shooting approach. Nazir et al. [12] visualized comparison enhancement into different fluids using Hall currents inserting mixture of multiple nanoparticles in base fluid via finite element methodology. Dezfulizadeh et al. [13] discussed impacts of Lorentz force in MHD flow using exergy an efficiency approach in the presence of ternary hybrid nanofluid. Oke [14] determined performance of tri-hybrid nanofluid in mass dissuasion and thermal energy considering base fluid (EG) past a rotating surface. Xiu et al. [15] studied dynamic behavior of ternary-hybrid nanoparticles in mass diffusion and thermal field considering small and large volume of nanoparticles with platelet, spherical and cylindrical nanoparticles over wedge using shooting approach. They have found that tri-hybrid nanofluid was observed very significantly for thermal performance rather than hybrid nanofluid. Animasaun and Asogwa [16] discussed comparative influences among alumina and water-based nanoparticles including based cupric nanoparticles (water-based) over stretching surface. Rasool et al. [17] experienced multiple aspects based on radiation and viscous dissipation using theory of non-Fourier's in second grade rheology using analytic approach on porous surface. Ashraf et al. [18] captured thermal features of magnetohydrodynamic flow in peristaltic fluid inserting magnetite nanoparticles in blood using the D-quadrature algorithm.
Nowadays, several industrial applications in pigments, electronics, cosmetics, food processing and utilizing engineering process metal oxide nanomaterials such as silicon dioxide, aluminum oxide and titanium dioxide. The retention and transport of nanoparticles (Al2O3,SiO2,TiO2) ware utilized to resolve issues of petroleum engineering and environmental. Nanoparticles (Al2O3,SiO2,TiO2) were introduced for enhancement of oil recovery by Hendraningrat et al. [19]. Al2O3 nanoparticles have higher boiling and melting points which are useful for enhancement of thermal inertia (see Song et al. [20]). In addition, Al2O3 nanoparticles are applicable to enhance the strengthens of smoothness, ceramics, creep resistance and crack hardness. Dynamic features of thermal transport in the presence of partially ionized liquid subjected to magnetic field is significantly exaggerated by magnetic field. Animasaun et al. [21] investigated that the Hall effect occurs when a magnetic field is applied perpendicular to the current flow through a thin sheet. As a result, an electric field is created parallel to the magnetic and current fields and directly proportional to the product of the magnetic induction and current density. Animasaun et al. [22] discussed comparison impacts among 47 nm and 36 nm alumina nanoparticles in base fluid (water) under action of Hall currents. Farooq et al. [23] developed model of entropy production rate in mass diffusion and thermal field under effects of Hall currents, viscous dissipation, magnetic field and Joule heating in cylindrical tubules.
The purpose of the present flow problem is to study characterizations of Casson fluid involving Lorentz force and thermal radiation (non-linear) over a 3D vertical surface accompanied with buoyancy force, Forchheirmer porous model and Joule heating. In additionally, mixture of three kinds of nanoparticles (Al2O3,SiO2,TiO2) in ethylene glycol is inserted to visualize thermal performance among cylindrical and platelet nanoparticles. The current problem fills the gap while not adequately studied with the following points.
● Analysis of heat transfer in Casson liquid under effects of Joule heating and viscous dissipation, thermal radiation (non-linear), Hall and ion slip forces using Darcy's Forchheirmer theory past a 3D surface has not been investigated yet.
● Previous to this investigation, no comparative analysis on Casson fluid among cylindrical and platelet nanoparticles over 3D surface using Forchheirmer porous model has been existed in literature works computed by finite element method.
● In existence literature, no investigations have been reported including ternary hybrid nanoparticles and shape effects rather than [24] to visualize comparative analysis among cylindrical and platelet nanoparticles.
Existence works demonstrate that development of Casson liquid inserting ternary hybrid nanofluid using thermal properties of various shapes of nanoparticles over 3-dimensional vertical using Forchheirmer porous model frame is not studied yet. Moreover, ion slip and Hall currents are considered with viscous dissipation and variable thermal conductivity. Present complex development is numerically handled by a finite element approach. In addition, section Ⅰ, section Ⅱ, section Ⅳ and section Ⅴ are based on literature review, problem modeling, numerical approach, results and conclusions, respectively.
A 3D model of Casson fluid is developed over a stretching surface using theories of generalized Ohm's law and Darcy's Forchheirmer model. Three types of nanoparticles are inserted into EG (ethylene glycol) while various shapes of nanoparticles (cylindrical and platelet) are addressed. Motion of nanoparticles is produced using movement of wall velocity. Buoyancy force is considered whereas viscous dissipation and thermal radiation (non-linear) are added in the energy equation. Suspension of nanoparticles regarding aluminum oxide, titanium oxide and silicon dioxide in working fluid is considered. Reduced form regarding Navier-Stoke equations is obtained and physical model is considered by Figure 1. Thermal radiation in terms of non-linear is utilized to determine thermal fields. Surface is assumed as non-conducting in terms of electrically and non-isothermal. Figure 1 is 3D vertical surface in y-, x- and z-components while non-uniform magnetic field is considered along z-component and gravitational force acts along downward direction. Partially flow over surface is induced by bidirectional movement of wall velocities Uw and Vw at z=0. A 3D surface lies in plane at z=0 and flow occupies region in field at z>0. Wall temperature and ambient temperature are considered as Tw and T∞. Further, it is studied that ethylene glycol is shear thinning [25], non-Newtonian liquid [26] and plasma [26]. Casson fluid model is also known as non-Newtonian liquid and shear thinning [1]. Table 1 reveals thermal properties of various nanoparticles. So, its rheology is prescribed by the Casson fluid stress tensor relation.
k | ρ | σ | |
Al2O3 | 32.9 | 6310 | 5.96×107 |
SiO2 | 1.4013 | 2270 | 3.5×106 |
TiO2 | 8.953 | 4250 | 2.4×106 |
EG | 0.144 | 884 | 0.125×10−11 |
Conservation laws for energy equation, momentum equation for incompressible and steady flow [24] using Casson liquid are
Ux+Vy+Wz=0, | (1) |
UUx+VUy+WUz=μTHρTH(1+1β)Uzz−μTHρTHfsU−fs(k1)1/2U2+(B0)2(y+x)n−1σTHρTH[(1+βiβe)2+β2e][βeV−(1+βeβi)U]+GβTH(T−T∞), | (2) |
UVx+VVy+WVz=μTHρTH(1+1β)Vzz−μTHρTHfsV−fs(k1)1/2V2−(B0)2(y+x)n−1σTHρTH[(1+βiβe)2+β2e][βeU+(1+βeβi)V]+GγTH(T−T∞), | (3) |
UTx+VTy+WTz=(B0)2(y+x)n−1σTHρTH[(1+βiβe)2+β2e](U2+V2)+(αTz+16T3σ∗3k∗(ρCp)fTz)z+μTH(ρCp)TH(1+1β)[(Uz)2+(Vz)2], | (4) |
Boundary conditions [24] of developing model are defined as
U=a(x+y)n,V=b(x+y)n,W=0,asz=0,U=0,T→T∞,V=0,asz→∞,T=Tw=T∞+A1T0(y+x)2n. | (5) |
Similarity variables [24] are delivered as
U=a(y+x)nF′,V=b(y+x)nG′,η=√aνf(y+x)n−12z,W=−√aνf(x+y)n−12{n+12(F+G)+n−12η(F′+G′)}. | (6) |
Equations (1)–(4) into dimensionless form [24] using variables similarity are
(1+1β)F′′′−A1[−n(F′+G′)F′+n+12(F+G)F′′]−ϵF′−νfνTHFrF′2+M2(1−ϕ1)2.5(1−ϕ2)2.5(1−ϕ3)−2.5[(1+βiβe)2+(βe)2][βeG′−(1+βeβi)F′]+α1θ=0, | (7) |
(1+1β)G′′′+A1[n(F′+G′)G′−n+12(F+G)G′′]−ϵG′−νfνTHFrG′2−M2(1−ϕ1)2.5(1−ϕ2)2.5(1−ϕ3)−2.5[(1+βiβe)2+(βe)2][βeF′+(1+βeβi)G′]+α2θ=0, | (8) |
((1+Nr(1+(θω−1)θ′)3))′+kf(ρcp)THkTH(ρcp)fPr[n+12θ′(F+G)−nθ(F′+G′)]+kfkTHPrEcM2(1+βiβe)2+(βe)2[(F′)2+(G′)2]=0, | (9) |
BCs in term of dimensionless [24] are
F(0)=F′(∞)=0,F′(0)=1,(0)=G′(∞)=0,G′(0)=λ,θ(0)=1=0,θ(∞)=0. | (10) |
Correlations regarding ternary hybrid nanofluid [28] for density, thermal conductivity and viscosity are defined as
A1=(1−ϕ1−ϕ2−ϕ3)+ϕ1ρsp1ρf+ϕ2ρsp2ρf+ϕ3ρsp3ρf, | (11) |
μTH=μNf1ϕ1+μNf2ϕ2+μNf3ϕ3,KTH=KNf1ϕ1+KNf2ϕ2+KNf3ϕ3ϕ, | (12) |
ρTH=(1−ϕ1−ϕ2−ϕ3)ρbf+ϕ1ρsp1+ϕ2ρsp2+ϕ2ρsp2, | (13) |
(ρCp)TH=(1−ϕ1−ϕ2−ϕ3)(ρCp)bf+ϕ1(ρCp)sp1+ϕ2(ρCp)sp2+ϕ3(ρCp)sp3, | (14) |
Thermal conductivity and viscosity models for cylindrical and platelet (see Figure 2) [28] are defined as
μNf2μbf=1+904.4ϕ2+13.5ϕ,Knf1=Kbf[Ksp2+3.9Kbf−3.9ϕ(Kbf−Ksp2)Ksp2+3.9Kbf+ϕ(Kbf−Ksp2)], | (15) |
μNf3μbf=1+612.6ϕ2+37.1ϕ,Knf1=Kbf[Ksp3+4.7Kbf−4.7ϕ(Kbf−Ksp3)Ksp3+4.7Kbf+ϕ(Kbf−Ksp3)]. | (16) |
Wall shear stresses due to ternary hybrid nanofluid [24] are derived as
Cf=τzx|z=0ρTH(uw)2,Re1/2Cf=(1+ϕ3)−2.5(n+1)1/2(1+ϕ2)2.5(1+ϕ1)2.5(1+1β)F′′(0), | (17) |
Cg=τzy|z=0ρTH(uw)2,Re1/2Cg=(1+ϕ3)−2.5(n+1)1/2(1+ϕ2)2.5(1+ϕ1)2.5(1+1β)G′′(0). | (18) |
Temperature gradient due to ternary hybrid nanofluid [24] is
Nu=−KTH(y+x)Tz|z=0(Tw−T∞)Kf,NuRe−1/2−KTHKfθ′(0). | (19) |
Finite element method [6,7] is employed to construct numerical solution of desired problem. It is described under.
Step 1: Firstly, the problem domain is transformed into a finite number of elements and derived linear type polynomial over each element. Weak form can be achieved from strong form using weighted residual function. Weighted residuals [6,7] are derived as
∫ηe+1ηew1[F′−H]dη=0,∫ηe+1ηew2[G′−T]dη=0, | (20) |
∫ηe+1ηew3[(1+1β)H′′−A1(−n(H+T)H+n+12(F+G)H′)+M2(1−ϕ1)2.5(1−ϕ2)2.5(1+βiβe)2+(βe)2(βeT−(1+βeβi)H)+α1θ]dη=0, | (21) |
∫ηe+1ηew4[(1+1β)T′′+A1(n(H+T)T−n+12(F+G)T′)+α2θ+M2(1−ϕ1)2.5(1−ϕ2)2.5(1+βiβe)2+(βe)2(βeH+(1+βeβi)T)]dη=0, | (22) |
∫ηe+1ηew5[θ′′+kfkTHPrEcM2(1+βiβe)2+(βe)2{(H)2+(H)2}−kf(ρcp)THkTH(ρcp)fPr{n+12θ′(F+G)−nθ(H+T)}]dη=0. | (23) |
Here, w3,w1,w2,w4 and w5 are weighted functions. Unknown variables and shape functions [6] are defined as
F=∑2j=1Fiψj,T=∑2j=1Tiψj,G=∑2j=1Giψj,H=∑2j=1Hiψj,θ=∑2j=1θiψj, | (24) |
ψj=(−1)i−1(η−ηj−1ηi−ηj−1). | (25) |
Step 2: Boundary integral vectors, stiffness matrices and force vectors are derived. After it, the global stiffness matrix is derived. In additionally, Picard linearization approach is utilized for achieving linear form of algebraic equations. Derivation of stiffness elements are
K11ij=∫ηe+1ηeψi(dψjdη)dη,K13ij=−∫ηe+1ηeψi(dψjdη)dη,K12ij=K14ij=K15ij=0, | (26) |
K21ij=∫ηe+1ηeψi(dψjdη)dη,K24ij=−∫ηe+1ηeψi(dψjdη)dη,K22ij=K23ij=K25ij=0, | (27) |
K33ij=∫ηe+1ηe[−(1+1β)dψidηdψjdη−A1(−n(−H+−T)ψiψj+n+12(−F+−G)ψiψj)−M2(1−ϕ1)2.5(1−ϕ2)2.5(1+βiβe)2+(βe)2((1+βeβi)ψiψj)]dη, | (28) |
K34ij=∫ηe+1ηe(βeψiψj)dη,K35ij=∫ηe+1ηeα1(ψiψj)dη,K31ij=0,K32ij=0,B5i=0, | (29) |
K34ij=∫ηe+1ηe[−A1(n(−H+−T)ψiψj−((−F+−G)ψidψjdη)n+12)−M2(1−ϕ1)2.5(1−ϕ2)2.5(1+βiβe)2+(βe)2((1+βeβi)ψiψj)]dη,B4i=0,K42ij=0, | (30) |
K55ij=∫ηe+1ηe[−(1+Nr(1+(θω−1))3)′3−θ′2dψidηdψjdη−kf(ρcp)THkTH(ρcp)fn(−H+−T)ψiψj−kf(ρcp)THkTH(ρcp)fPr−Gψidψjdη+kf(ρcp)THkTH(ρcp)fPr(n+12)−Fψidψjdη]dη, | (31) |
K53ij=∫ηe+1ηe[kfkTHPrEcM2(1+βiβe)2+(βe)2−Hψiψj]dη,K41ij=K51ij=0,K52ij=0,B1i=0,K43ij=0, | (32) |
K54ij=∫ηe+1ηe[kfkTHPrEcM2(1+βiβe)2+(βe)2−Gψiψj]dη,B2i=0,B3i=0,K35ij=∫ηe+1ηeα1(ψiψj)dη, | (33) |
Step 3: System of linear equations is solved in form of iteratively under numerically tolerance (10−5). Convergence and error analysis is mentioned below
Err=|δi−δi−1|,Max|δi−δi−1|≤10−8. | (34) |
Step 4: MAPLE 18 is used to design programming of finite element approach. Mesh free study is recorded in Table 2. Flow chart of numerical methodology is addressed by Figure 3. Validation results of present model is verified with already published work [24] considering absence of ternary hybrid nanofluid, power law index number, Darcy's Forchheirmer model and Casson fluid. Validation of numerical results with published work is mentioned in Table 3.
e | F′(ηmax2) | G′(ηmax2) | θ(ηmax2) |
30 | 0.5172614186 | 0.4141116019 | 0.5331053851 |
60 | 0.5009743321 | 0.3991196957 | 0.5166109650 |
90 | 0.4955010944 | 0.3940814743 | 0.5110865969 |
120 | 0.4927563199 | 0.3915549156 | 0.5083195950 |
150 | 0.4911066420 | 0.3900362436 | 0.5066578927 |
180 | 0.4900077513 | 0.3890241819 | 0.5055494665 |
210 | 0.4892201185 | 0.3882992913 | 0.5047574611 |
240 | 0.4886298879 | 0.3877558788 | 0.5041632925 |
270 | 0.4881717384 | 0.3873337477 | 0.5037009996 |
300 | 0.4888027764 | 0.3879944431 | 0.5033311740 |
n | λ | Khan et al. [24] | present results for −F′′(0) | Khan et al. [24] | present results for −G′′(0) |
0.0 | 1.0 | 1.0 | 0.0 | 0.0 | |
1 | 0.5 | 1.224745 | 0.2210013530 | 0.612372 | 0.6124360703 |
1.0 | 1.414214 | 0.4111910216 | 1.414214 | 0.4145020304 | |
0.0 | 1.624356 | 0.6238906653 | 0.0 | 0.0 | |
3 | 0.5 | 1.989422 | 0.9893026033 | 0.994711 | 0.9950661609 |
1.0 | 2.297182 | 0.2969018879 | 2.297182 | 2.2960670811 |
The parametric study is captured to determine flow behavior and transport of thermal energy into a mixture of ternary hybrid nanoparticles in base and working fluids. The graphical outcomes are displayed in Figures 4–12. It is noticed that ternary hybrid nanofluid is a mixture of aluminum dioxide, titanium dioxide, silicon dioxide in base fluid and working fluid. Hybrid nanofluid is considered a mixture of silicon dioxide, titanium dioxide while nanofluid is a mixture of titanium dioxide in Casson fluid. In additionally, thermal and velocity fields are plotted versus different parameters implementing ranges of 0.5≤β≤4, 0.1≤n≤3, 0.0≤M≤2.0, ,0.0≤βe≤3.0, ,0.0≤βi≤4.0, ,204≤Pr≤208, 0.0≤Ec≤3.0, 0.0≤α1≤1.5, 0.0≤ϕ1≤0.04, 0.0≤λ≤0.5, 0.0≤ϕ2≤0.07, 0.0≤ϕ2≤0.07, 0.0≤ϕ3≤0.06, 0.0≤α1≤2.0, 0.0≤ϵ≤1.5, 0.0≤Fr≤3.0, 0.0≤Nr≤3.0 and 0.0≤θω≤2.0.
Figures 4–7 are plotted to determine the effect of several parameters on flow involving dispersion of ternary hybrid nanoparticles. It is visualized that platelet nanoparticles are represented by solid curves on graphs and dot curves are associated with cylindrical nanoparticles on graphs. Figure 4 clearly reveal that there is reduction into flow (y- and x-directions) when β is changed. The effect of the Casson parameter for cylindrical nanoparticles is less than the effect of the Casson number for platelet nanoparticles. This effect is created using concept of Casson fluid in momentum equations whereas existence of β is formulated considering tensor of Casson fluid in momentum equations. Flow becomes reduced in y- and x-directions because of β is appeared in form of 1β. Therefore, an inverse proportional relation can be investigated versus velocity fields. Consequently, flow slows down in terms of y- and x-directions. Casson fluid behaves as a shear thinning and non-Newtonian liquid. Flow becomes significant for higher values of β rather flow for minimize values of β. Thickness for momentum boundary layers is declined when β is gradually enhanced. It is studied that current analysis is transformed into Newtonian model for β→∞ while other values of β is case of non-Newtonian model. In momentum equation, β occurs in the denominator. Hence, an inclination in β results velocity field is enhanced. Role βe (Hall parameter) is predicted on flow behavior in term of y- and x-directions. An enhancement is studied into flow (x-and y-direction) versus variation in βe. It is dimensionless parameter which is formulated using the theory of generalized Ohm's law in current analysis. Further, flow for platelet nanoparticles is produced significantly rather than flow for cylindrical particles. Figure 5 visualize the role of ion slip number (βi) on velocity fields in terms of y- and x-directions. Similar impact is conducted when βi is increased. It is observed that ethylene glycol was predicted as plasma and its behavior of flow is exposed as a non-uniform magnetic field. An interaction among plasma and magnetic field produces ion slip and Hall currents which is responsible for ion slip and Hall forces. Applied magnetic field is opposite against action of ion slip and Hall forces. Hence, Lorentz force reduces when βe and βi are increased. Physically, magnetic field was placed perpendicular along the z-direction of the surface and against flow. Therefore, flow was reduced by applying higher values of Lorentz force because Lorentz force is addressed as opposite against applied magnetic field. Thickness based on flow curves for the case of platelet nanoparticles is higher than thickness generated by cylindrical nanoparticles. Role of Fr on flow in y- and x-directions is addressed by Figure 7. It was found that velocity distribution in y- and x-directions is decreased in presence of higher numerical values of Fr. It is noticed that Fr is dimensionless parameter which is produced considering Forchheirmer-porous theory. Mathematically, Fr is inversely proportional versus velocity field. Consequently, an inclination in Fr results flow increases in y- and x-directions. Thickness for the momentum layers is declined when Fr is changed. Flow for case of Fr=0 is greater than flow for case Fr≠0.
Figures 8–11 reveal impact of temperature profile carrying three kind nanoparticles having cylindrical and platelet shapes against change in Nr,Fr,βe and βi. Reduction into temperature versus implication of Nr has been estimated by Figure 8. Highest performance of thermal process for case of platelet nanoparticles has been obtained rather than for cylindrical particles. It is addressed that Nr is termed as dimensionless which is produced using the concept of non-uniform thermal radiation in energy equation. It was noticed that thermally energy reduces when Nr is distributed. Physically, heat energy moves away from z=0 along with thermal radiations. Therefore, temperature decreases at walls of plate. Thickness of thermally layers for case of platelet nanoparticles is greater than thermally thickness for case of cylindrical nanoparticles. Observations of Fr on thermally field was investigated by Figure 9 including ternary hybrid nanoparticles. Maximum production of thermal field was estimated versus distribution in Fr. Thermal enhancement for the case Fr=0 is less than thermal enhancement for case of Fr≠0. Thermal production for cylindrical particles in presence of variable thermal conductivity is less than thermal production for platelet particles. Figures 10 and 11 are plotted to estimate distribution into thermal energy using parameters regarding ion slip (βi) and Hall parameters (βe). Reduction into thermal distribution was visualized using higher values of ion slip (βi) and Hall parameters (βe). Ion slip (βi) and Hall (βe) are opposite against Lorentz force. Further, collision rate into nanoparticles is inversely directly proportional to ion slip and Hall forces result in reduction into magnetic intensity. This reduction in magnetic intensity produces declination on thermal profile. Platelet nanoparticles experience significant thermal energy rather than experience of thermal energy for cylindrical nanoparticles. Physically, βi and βe are associated in the denominator of the Joule heating term in the energy equation. Consequent, heat energy generates because of the Joule heating process. Figure 12 reveals comparison analysis among alumina-silica-titania nanoparticles/EG, silica-titania nanoparticles/EG, silica-titania nanoparticles/EG, titania nanoparticles/EG and working fluid. It was observed that alumina-silica-titania nanoparticles/EG experiences higher thermal energy rather than for the cases of silica-titania nanoparticles/EG, silica-titania nanoparticles/EG, titania nanoparticles/EG and working fluid. Therefore, ternary hybrid nanofluid is observed as most significant for producing maximum production of thermal energy as well as reduction in thermal energy.
Table 4 is prepared to determine production of wall shear stresses and thermal rate using change in Hall parameter, magnetic parameter, ion slip parameter, Casson parameter and Fr. It was numerically investigated that production of thermal rate ingresses when Hall parameter, magnetic parameter, ion slip parameter are changed. However, the same behavior is investigated versus β and thermal rate decreases versus change in Fr. Wall shear stresses are inversely proportional against distribution in ion slip parameter, Casson number and Hall parameter. But inverse behavior for case of wall shear stresses is estimated when Lorentz force is changed.
Parameters | Distribution in parameters | −Re1/2Cf | −Re1/2Cg | −Re−1/2Nu |
0.0 | 0.2625008163 | 0.4236006667 | 1.652101988 | |
Fr | 0.5 | 0.2701304207 | 0.4424323033 | 1.634134933 |
1.4 | 0.2905110133 | 0.4903136669 | 1.610193943 | |
1.5 | 0.2192892414 | 0.2595109874 | 1.751988063 | |
β | 2.0 | 0.2403202308 | 0.2706220343 | 1.730933030 |
3.5 | 0.2700293493 | 0.2903119971 | 1.711018177 | |
0.0 | 0.2233998059 | 0.2824407947 | 1.747439085 | |
M | 0.6 | 0.2346098044 | 0.2903302023 | 1.754403388 |
1.2 | 0.2472144519 | 0.3184495583 | 1.780274025 | |
0.0 | 0.2437500727 | 0.2688997575 | 1.754913084 | |
βe | 0.4 | 0.2242905241 | 0.2214389298 | 1.764917691 |
0.8 | 0.2146742280 | 0.2116991296 | 1.794919036 | |
0.0 | 0.2741394839 | 0.2805626046 | 1.754917615 | |
βi | 0.4 | 0.2341541906 | 0.2304551660 | 1.7843013073 |
0.8 | 0.2141711628 | 0.2202741977 | 1.7899183003 |
Thermal aspects of Casson fluid containing ternary hybrid nanofluid over stretching sheet utilizing Darcy's Forchheirmer theory are addressed. Various shapes regarding nanoparticles (platelet, cylindrical and spherical) are implemented in EG. Thermal radiation (non-linear) is utilized in the energy equation considering effects of viscous dissipation and variable wall velocity. Performance of several parameters on flow and temperature profile is addressed and numerical study is tackled by FEM. Key findings of the problem are listed below.
Hall and ion slip numbers play a remarkable role in controlling momentum and thermal boundary layers. However, declination into motion has been investigated versus β and Fr.
Temperature decreases against variation in βe and βi but opposite trend is noticed in thermal layers against distribution in Fr.
Ethylene glycol is studied as partially ionized and shear thinning and its interaction with magnetic field produces ion slip and Hall currents. Due to this process, EG experiences ion slip and Hall forces which are against Lorentz force. Therefore, flow of EG was accelerated versus change in βe and βi.
Convergence of current analysis is ensured using finite element methodology.
Maximum production for thermal energy can be achieved rather than nanofluid and hybrid nanofluid. This process is applicable in coolants (in automobiles), fuel dynamics, solar system, microelectronics, temperature reduction, cooling process and pharmaceutical process.
It was included that platelet nanoparticles experience more heat energy rather than thermal energy for cylindrical nanoparticles. Similar behavior was analyzed in velocity fields.
Nusselt number increases versus change in ion slip, Hall and M parameters but thermal rate decreases versus distribution in Fr.
This research was supported by the Postdoctoral Research Fellowship Training Program from Khon Kaen University (PD2565-02-05).
The authors declare that they have no conflicts of interest.
[1] |
M. Akram, A. Adeel, J. C. R. Alcantud, Group decision-making methods based on hesitant N-soft sets, Expert Syst. Appl., 115 (2019), 95-105. doi: 10.1016/j.eswa.2018.07.060
![]() |
[2] |
J. C. R. Alcantud, A. Giarlotta, Necessary and possible hesitant fuzzy sets: A novel model for group decision making, Inform. Fusion, 46 (2019), 63-76. doi: 10.1016/j.inffus.2018.05.005
![]() |
[3] |
M. Ali, F. Feng, X. Liu, et al. On some new operations in soft set theory, Comput. Math. Appl., 57 (2009), 1547-1553. doi: 10.1016/j.camwa.2008.11.009
![]() |
[4] |
B. Bedregal, R. Reiser, H. Bustince, et al. Aggregation functions for typical hesitant fuzzy elements and the action of automorphisms, Inform. Sci., 255 (2014), 82-99. doi: 10.1016/j.ins.2013.08.024
![]() |
[5] |
N. Cagman, S. Enginoglu, Soft set theory and uni-int decision making, Eur. J. Oper. Res., 207 (2010), 848-855. doi: 10.1016/j.ejor.2010.05.004
![]() |
[6] |
N. Cagman, S. Enginoglu, Soft matrix theory and its decision making, Comput. Math. Appl., 59 (2010), 3308-3314. doi: 10.1016/j.camwa.2010.03.015
![]() |
[7] |
D. Chen, E. C. C. Tsang, D. S. Yeung, et al. The parameterization reduction of soft sets and its applications, Comput. Math. Appl., 49 (2005), 757-763. doi: 10.1016/j.camwa.2004.10.036
![]() |
[8] |
J. Chen, X. Huang, J. Tang, Distance measures for higher order dual hesitant fuzzy sets, Comput. Appl. Math., 37 (2018), 1784-1806. doi: 10.1007/s40314-017-0423-3
![]() |
[9] |
N. Chen, Z. S. Xu, M. M. Xia, Interval-valued hesitant preference relations and their applications to group decision making, Knowl. Based Syst., 37 (2013), 528-540. doi: 10.1016/j.knosys.2012.09.009
![]() |
[10] | A. Dey, M. Pal, Genelalised multi-fuzzy soft set and its application in decision making, Pac. Sci. Rev. A, 17 (2015), 23-28. |
[11] | A. Dey, M. Pal, On hesitant multi-fuzzy soft topology, Pac. Sci. Rev. B, 1 (2015), 124-130. |
[12] |
F. Feng, Y. B. Jun, X. Liu, et al. An adjustable approach to fuzzy soft set based decision making, J. Comput. Appl. Math., 234 (2010), 10-20. doi: 10.1016/j.cam.2009.11.055
![]() |
[13] |
F. Feng, Y. Li, N. Cagman, Generalized uni-int decision making schemes based on choice value soft sets, Eur. J. Oper. Res., 220 (2012), 162-170. doi: 10.1016/j.ejor.2012.01.015
![]() |
[14] |
X. Guan, Y. Li, F. Feng, A new order relation on fuzzy soft sets and its application, Soft Comput., 17 (2013), 63-70. doi: 10.1007/s00500-012-0903-8
![]() |
[15] |
J. Ignatius, S. Motlagh, M. Sepehri, et al. Hybrid models in decision making under uncertainty: The case of training provider evaluation, J. Intell. Fuzzy Syst., 21 (2010), 147-162. doi: 10.3233/IFS-2010-0443
![]() |
[16] | P. Kakati, S. Borkotokey, S. Rahman, et al. Interval neutrosophic hesitant fuzzy Einstein Choquet integral operator for multicriteria decision making, Artif. Intell. Rev., (2019), 1-36. |
[17] |
Z. Kong, L. Gao, L. Wang, A fuzzy soft set theoretic approach to decision making problems, J. Comput. Appl. Math., 223 (2009), 540-542. doi: 10.1016/j.cam.2008.01.011
![]() |
[18] |
Z. Kong, L. Wang, Z. Wu, Application of fuzzy soft set in decision making problems based on grey theory, J. Comput. Appl. Math., 236 (2011), 1521-1530. doi: 10.1016/j.cam.2011.09.016
![]() |
[19] |
D. C. Liang, D. Liu, A novel risk decision making based on decision-theoretic rough sets under hesitant fuzzy information, IEEE Trans. Fuzzy Syst., 23 (2015), 237-247. doi: 10.1109/TFUZZ.2014.2310495
![]() |
[20] |
D. C. Liang, Z. S. Xu, D. Liu, Three-way decisions based on decision-theoretic rough sets with dual hesitant fuzzy information, Inform. Sci., 396 (2017), 127-143. doi: 10.1016/j.ins.2017.02.038
![]() |
[21] |
D. C. Liang, M. W. Wang, Z. S. Xu, et al. Risk appetite dual hesitant fuzzy three-way decisions with todim, Inform. Sci., 507 (2020), 585-605. doi: 10.1016/j.ins.2018.12.017
![]() |
[22] | P. K. Maji, R. Biswas, A. R. Roy, Fuzzy soft sets, J. Fuzzy Math., 9 (2001), 589-602. |
[23] |
P. K. Maji, R. Biswas, A. R. Roy, An application of soft sets in a decision making problem, Comput. Math. Appl., 44 (2002), 1077-1083. doi: 10.1016/S0898-1221(02)00216-X
![]() |
[24] |
P. Majumdar, S. K. Samanta, Generalised fuzzy soft sets, Comput. Math. Appl., 59 (2010), 1425-1432. doi: 10.1016/j.camwa.2009.12.006
![]() |
[25] | D. A. Molodtsov, Soft set theory-first results, Comput. Math. Appl., 37 (1999), 19-31. |
[26] | S. Naz, M. Akram, Novel decision-making approach based on hesitant fuzzy sets and graph theory, Comput. Appl. Math., 38 (2019), 7. |
[27] |
B. Ozkan, E. Ozceylan, M. Kabak, et al. Evaluating the websites of academic departments through SEO criteria: A hesitant fuzzy linguistic MCDM approach, Artif. Intell. Rev., 53 (2020), 875-905. doi: 10.1007/s10462-019-09681-z
![]() |
[28] |
X. Qi, D. Liang, J. Zhang, Multiple attribute group decision making based on generalized power aggregation operators under interval-valued dual hesitant fuzzy linguistic environment, Int. J. Mach. Learn. Cybern., 7 (2016), 1147-1193. doi: 10.1007/s13042-015-0445-3
![]() |
[29] |
Z. Ren, C. Wei, A multi-attribute decision-making method with prioritization relationship and dual hesitant fuzzy decision information, Int. J. Mach. Learn. Cybern., 8 (2017), 755-763. doi: 10.1007/s13042-015-0356-3
![]() |
[30] |
R. M. Rodryguez, L. Martynez, V. Torra, et al. Hesitant fuzzy sets: state of the art and future directions, Int. J. Intell. Syst., 29 (2014), 495-524. doi: 10.1002/int.21654
![]() |
[31] |
A. R. Roy, P. K. Maji, A fuzzy soft set theoretic approach to decision making problems, J. Comput. Appl. Math., 203 (2007), 412-418. doi: 10.1016/j.cam.2006.04.008
![]() |
[32] | S. Sebastian, T. V. Ramakrishnan, Multi-fuzzy sets: An extension of fuzzy sets, Fuzzy Inf. Eng., 1 (2011), 35-43. |
[33] | C. Song, Y. Zhang, Z. Xu, An improved structure learning algorithm of Bayesian Network based on the hesitant fuzzy information flow, Appl. Soft Comput., 82 (2019), 105549. |
[34] | V. Torra, Hesitant fuzzy sets, Int. J. Intell. Syst., 25 (2010), 529-539. |
[35] | F. Wang, X. Li, X. Chen, Hesitant fuzzy soft set and its applications in multicriteria decision making, J. Appl. Math., 2014 (2014), 643785. |
[36] |
G. Wei, X. Zhao, R. Lin, Some hesitant intervalvalued fuzzy aggregation operators and their applications to multiple attribute decision making, Knowl. Based Syst, 46 (2013), 43-53. doi: 10.1016/j.knosys.2013.03.004
![]() |
[37] |
G. Wei, X. Zhao, Induced hesitant interval-valued fuzzy Einstein aggregation operators and their application to multiple attribute decision making, J. Intell. Fuzzy Syst., 24 (2013), 789-803. doi: 10.3233/IFS-2012-0598
![]() |
[38] |
G. Wei, Interval valued hesitant fuzzy uncertain linguistic aggregation operators in multiple attribute decision making, Int. J. Mach. Learn. Cybern., 7 (2016), 1093-1114. doi: 10.1007/s13042-015-0433-7
![]() |
[39] |
M. Xia, Z. S. Xu, Hesitant fuzzy information aggregation in decision making, Int. J. Approx. Reason., 52 (2011), 395-407. doi: 10.1016/j.ijar.2010.09.002
![]() |
[40] |
M. Xia, Z. S. Xu, Some studies on properties of hesitant fuzzy sets, Int. J. Mach. Learn. Cybern., 8 (2017), 489-495. doi: 10.1007/s13042-015-0340-y
![]() |
[41] |
Z. S. Xu, M. Xia, Distance and similarity measures for hesitant fuzzy sets, Inform. Sci., 181 (2011), 2128-2138. doi: 10.1016/j.ins.2011.01.028
![]() |
[42] |
Z. Xu, W. Zhou, Consensus building with a group of decision makers under the hesitant probabilistic fuzzy environment, Fuzzy Optim. Decis. Ma., 16 (2017), 481-503. doi: 10.1007/s10700-016-9257-5
![]() |
[43] |
W. Xue, Z. Xu, H. Wang, et al. Hazard assessment of landslide dams using the evidential reasoning algorithm with multi-scale hesitant fuzzy linguistic information, Appl. Soft Comput., 79 (2019), 74-86. doi: 10.1016/j.asoc.2019.03.032
![]() |
[44] |
Y. Yang, X. Tan, C. Meng, The multi-fuzzy soft set and its application in decision making, Appl. Math. Model., 37 (2013), 4915-4923. doi: 10.1016/j.apm.2012.10.015
![]() |
[45] |
Y. Yang, X. Peng, H. Chen, A decision making approach based on bipolar multi-fuzzy soft set theory, J. Intell. Fuzzy Syst., 27 (2014), 1861-1872. doi: 10.3233/IFS-141152
![]() |
[46] |
D. Yu, D. F. Li, J. M. Merigo, Dual hesitant fuzzy group decision making method and its application to supplier selection, Int. J. Mach. Learn. Cybern., 7 (2016), 819-831. doi: 10.1007/s13042-015-0400-3
![]() |
[47] |
C. Zhang, D. Li, J. Liang, Hesitant fuzzy linguistic rough set over two universes model and its applications, Int. J. Mach. Learn. Cybern., 9 (2018), 577-588. doi: 10.1007/s13042-016-0541-z
![]() |
[48] |
C. Zhang, D. Li, J. Liang, Multi-granularity three-way decisions with adjustable hesitant fuzzy linguistic multigranulation decision-theoretic rough sets over two universes, Inform. Sci., 507 (2020), 665-683. doi: 10.1016/j.ins.2019.01.033
![]() |
[49] |
C. Zhang, D. Li, J. Liang, Interval-valued hesitant fuzzy multi-granularity three-way decisions in consensus processes with applications to multi-attribute group decision making, Inform. Sci., 511 (2020), 192-211. doi: 10.1016/j.ins.2019.09.037
![]() |
[50] |
Z. Zhang, Hesitant fuzzy power aggregation operators and their application to multiple attribute group decision making, Inform. Sci., 234 (2013), 150-181. doi: 10.1016/j.ins.2013.01.002
![]() |
[51] |
Z. Zhang, C. Wang, D. Tian, et al. Induced generalized hesitant fuzzy operators and their application to multiple attribute group decision making, Comput. Ind. Eng., 67 (2014), 116-138. doi: 10.1016/j.cie.2013.10.011
![]() |
[52] | Z. Zhang, H. Dong, S. Lan, Possibility multi-fuzzy soft set and its application in decision making, J. Intell. Fuzzy Syst., 27 (2013), 2115-2125. |
[53] | H. Zhang, L. Xiong, W. Ma, On interval-valued hesitant fuzzy soft sets, Math. Probl. Eng., 2015 (2015), 254764. |
[54] |
F. Zhang, J. Ignatius, C. P. Lim, et al. A new method for ranking fuzzy numbers and its application to group decision making, Appl. Math. Model., 38 (2014), 1563-1582. doi: 10.1016/j.apm.2013.09.002
![]() |
[55] | W. Zhou, Z. Xu, Hesitant fuzzy linguistic portfolio model with variable risk appetite and its application in the investment ratio calculation, Appl. Soft Comput., 84 (2019), 105719. |
1. | Muhammad Sohail, Umar Nazir, Kanit Mukdasai, Manoj Singh, Abha Singh, Chandika Rama Mohan, Ahmed M. Galal, Sayed M. Eldin, Transportation of Fe3O4-SiO2-Al2O3/EO and SiO2-Al2O3/EO nanoparticles in magnetized Reiner–Philippoff liquid, including modified fluxes via Galerkin algorithm: Significance of EMHD, 2023, 11, 2296-424X, 10.3389/fphy.2023.1133550 | |
2. | KANG-JIA WANG, SHUAI LI, STUDY ON THE LOCAL FRACTIONAL (3+1)-DIMENSIONAL MODIFIED ZAKHAROV–KUZNETSOV EQUATION BY A SIMPLE APPROACH, 2024, 32, 0218-348X, 10.1142/S0218348X24500919 | |
3. | Naila Shaheen, Muhammad Ramzan, Seifedine Kadry, Mohamed Abbas, C Ahamed Saleel, Unsteady ternary hybrid-nanofluid flow over an expanding/shrinking cylinder with multiple slips: a Yamada–Ota model implementation, 2023, 34, 0957-4484, 365711, 10.1088/1361-6528/acdaa0 | |
4. | N. Sultana, S. Shaw, S. Mondal, M.K. Nayak, S. Nazari, Abir Mouldi, Ali J. Chamkha, Hall and ion-slip current efficacy on thermal performance of magnetic power-law hybrid nanofluid using modified Fourier’s law, 2024, 15, 20904479, 102838, 10.1016/j.asej.2024.102838 | |
5. | D. K. Almutairi, Analysis for bioconvection due to magnetic induction of Casson nanoparticles subject to variable thermal conductivity, 2024, 14, 2045-2322, 10.1038/s41598-024-59478-6 | |
6. | Vinutha K, Shilpa B, Koushik V. Prasad, Naveen Kumar R, Punith Gowda R. J., Taseer Muhammad, Raman Kumar, Karthik K, Dynamics of Pollutant Discharge Concentration, Nanoparticle Diameter and Solid–Fluid Interfacial Layer on Nanofluid Flow Past a Convergent/Divergent Channel, 2024, 14, 2191-1630, 2218, 10.1007/s12668-024-01554-2 | |
7. | Liu Yuhao, Qu Pu, Li Qiang, Numerical simulation and experiment of double chamber brake based on CFD, 2023, 13, 2045-2322, 10.1038/s41598-023-45010-9 |
k | ρ | σ | |
Al2O3 | 32.9 | 6310 | 5.96×107 |
SiO2 | 1.4013 | 2270 | 3.5×106 |
TiO2 | 8.953 | 4250 | 2.4×106 |
EG | 0.144 | 884 | 0.125×10−11 |
e | F′(ηmax2) | G′(ηmax2) | θ(ηmax2) |
30 | 0.5172614186 | 0.4141116019 | 0.5331053851 |
60 | 0.5009743321 | 0.3991196957 | 0.5166109650 |
90 | 0.4955010944 | 0.3940814743 | 0.5110865969 |
120 | 0.4927563199 | 0.3915549156 | 0.5083195950 |
150 | 0.4911066420 | 0.3900362436 | 0.5066578927 |
180 | 0.4900077513 | 0.3890241819 | 0.5055494665 |
210 | 0.4892201185 | 0.3882992913 | 0.5047574611 |
240 | 0.4886298879 | 0.3877558788 | 0.5041632925 |
270 | 0.4881717384 | 0.3873337477 | 0.5037009996 |
300 | 0.4888027764 | 0.3879944431 | 0.5033311740 |
n | λ | Khan et al. [24] | present results for −F′′(0) | Khan et al. [24] | present results for −G′′(0) |
0.0 | 1.0 | 1.0 | 0.0 | 0.0 | |
1 | 0.5 | 1.224745 | 0.2210013530 | 0.612372 | 0.6124360703 |
1.0 | 1.414214 | 0.4111910216 | 1.414214 | 0.4145020304 | |
0.0 | 1.624356 | 0.6238906653 | 0.0 | 0.0 | |
3 | 0.5 | 1.989422 | 0.9893026033 | 0.994711 | 0.9950661609 |
1.0 | 2.297182 | 0.2969018879 | 2.297182 | 2.2960670811 |
Parameters | Distribution in parameters | −Re1/2Cf | −Re1/2Cg | −Re−1/2Nu |
0.0 | 0.2625008163 | 0.4236006667 | 1.652101988 | |
Fr | 0.5 | 0.2701304207 | 0.4424323033 | 1.634134933 |
1.4 | 0.2905110133 | 0.4903136669 | 1.610193943 | |
1.5 | 0.2192892414 | 0.2595109874 | 1.751988063 | |
β | 2.0 | 0.2403202308 | 0.2706220343 | 1.730933030 |
3.5 | 0.2700293493 | 0.2903119971 | 1.711018177 | |
0.0 | 0.2233998059 | 0.2824407947 | 1.747439085 | |
M | 0.6 | 0.2346098044 | 0.2903302023 | 1.754403388 |
1.2 | 0.2472144519 | 0.3184495583 | 1.780274025 | |
0.0 | 0.2437500727 | 0.2688997575 | 1.754913084 | |
βe | 0.4 | 0.2242905241 | 0.2214389298 | 1.764917691 |
0.8 | 0.2146742280 | 0.2116991296 | 1.794919036 | |
0.0 | 0.2741394839 | 0.2805626046 | 1.754917615 | |
βi | 0.4 | 0.2341541906 | 0.2304551660 | 1.7843013073 |
0.8 | 0.2141711628 | 0.2202741977 | 1.7899183003 |
k | ρ | σ | |
Al2O3 | 32.9 | 6310 | 5.96×107 |
SiO2 | 1.4013 | 2270 | 3.5×106 |
TiO2 | 8.953 | 4250 | 2.4×106 |
EG | 0.144 | 884 | 0.125×10−11 |
e | F′(ηmax2) | G′(ηmax2) | θ(ηmax2) |
30 | 0.5172614186 | 0.4141116019 | 0.5331053851 |
60 | 0.5009743321 | 0.3991196957 | 0.5166109650 |
90 | 0.4955010944 | 0.3940814743 | 0.5110865969 |
120 | 0.4927563199 | 0.3915549156 | 0.5083195950 |
150 | 0.4911066420 | 0.3900362436 | 0.5066578927 |
180 | 0.4900077513 | 0.3890241819 | 0.5055494665 |
210 | 0.4892201185 | 0.3882992913 | 0.5047574611 |
240 | 0.4886298879 | 0.3877558788 | 0.5041632925 |
270 | 0.4881717384 | 0.3873337477 | 0.5037009996 |
300 | 0.4888027764 | 0.3879944431 | 0.5033311740 |
n | λ | Khan et al. [24] | present results for −F′′(0) | Khan et al. [24] | present results for −G′′(0) |
0.0 | 1.0 | 1.0 | 0.0 | 0.0 | |
1 | 0.5 | 1.224745 | 0.2210013530 | 0.612372 | 0.6124360703 |
1.0 | 1.414214 | 0.4111910216 | 1.414214 | 0.4145020304 | |
0.0 | 1.624356 | 0.6238906653 | 0.0 | 0.0 | |
3 | 0.5 | 1.989422 | 0.9893026033 | 0.994711 | 0.9950661609 |
1.0 | 2.297182 | 0.2969018879 | 2.297182 | 2.2960670811 |
Parameters | Distribution in parameters | −Re1/2Cf | −Re1/2Cg | −Re−1/2Nu |
0.0 | 0.2625008163 | 0.4236006667 | 1.652101988 | |
Fr | 0.5 | 0.2701304207 | 0.4424323033 | 1.634134933 |
1.4 | 0.2905110133 | 0.4903136669 | 1.610193943 | |
1.5 | 0.2192892414 | 0.2595109874 | 1.751988063 | |
β | 2.0 | 0.2403202308 | 0.2706220343 | 1.730933030 |
3.5 | 0.2700293493 | 0.2903119971 | 1.711018177 | |
0.0 | 0.2233998059 | 0.2824407947 | 1.747439085 | |
M | 0.6 | 0.2346098044 | 0.2903302023 | 1.754403388 |
1.2 | 0.2472144519 | 0.3184495583 | 1.780274025 | |
0.0 | 0.2437500727 | 0.2688997575 | 1.754913084 | |
βe | 0.4 | 0.2242905241 | 0.2214389298 | 1.764917691 |
0.8 | 0.2146742280 | 0.2116991296 | 1.794919036 | |
0.0 | 0.2741394839 | 0.2805626046 | 1.754917615 | |
βi | 0.4 | 0.2341541906 | 0.2304551660 | 1.7843013073 |
0.8 | 0.2141711628 | 0.2202741977 | 1.7899183003 |