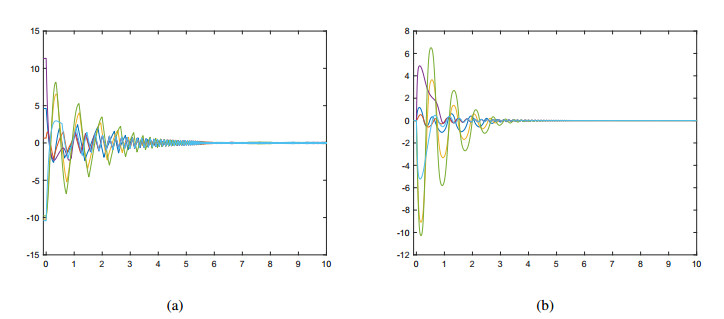
This article focuses on achieving fixed-time synchronization (FxTS) of nonlinear coupled memristive neural networks (NCMMN) with time delays. We propose a novel integrable sliding-mode manifold (SMM) and develop two control strategies (chattering or non-chattering) to achieve FxTS. By selecting appropriate parameters, some criteria are established to force the dynamics of NCMMN to reach the designed SMM within a fixed time and remain on it thereafter. Additionally, they provide estimations for the settling time (TST). the validity of our results is demonstrated through several numerical examples.
Citation: Xingting Geng, Jianwen Feng, Yi Zhao, Na Li, Jingyi Wang. Fixed-time synchronization of nonlinear coupled memristive neural networks with time delays via sliding-mode control[J]. Electronic Research Archive, 2023, 31(6): 3291-3308. doi: 10.3934/era.2023166
[1] | Huijun Xiong, Chao Yang, Wenhao Li . Fixed-time synchronization problem of coupled delayed discontinuous neural networks via indefinite derivative method. Electronic Research Archive, 2023, 31(3): 1625-1640. doi: 10.3934/era.2023084 |
[2] | Chao Yang, Juntao Wu, Zhengyang Qiao . An improved fixed-time stabilization problem of delayed coupled memristor-based neural networks with pinning control and indefinite derivative approach. Electronic Research Archive, 2023, 31(5): 2428-2446. doi: 10.3934/era.2023123 |
[3] | Xiangwen Yin . A review of dynamics analysis of neural networks and applications in creation psychology. Electronic Research Archive, 2023, 31(5): 2595-2625. doi: 10.3934/era.2023132 |
[4] | Yong Zhao, Shanshan Ren . Synchronization for a class of complex-valued memristor-based competitive neural networks(CMCNNs) with different time scales. Electronic Research Archive, 2021, 29(5): 3323-3340. doi: 10.3934/era.2021041 |
[5] | Tianyi Li, Xiaofeng Xu, Ming Liu . Fixed-time synchronization of mixed-delay fuzzy cellular neural networks with $ L\acute{e}vy $ noise. Electronic Research Archive, 2025, 33(4): 2032-2060. doi: 10.3934/era.2025090 |
[6] | Yu-Jing Shi, Yan Ma . Finite/fixed-time synchronization for complex networks via quantized adaptive control. Electronic Research Archive, 2021, 29(2): 2047-2061. doi: 10.3934/era.2020104 |
[7] | Shuang Liu, Tianwei Xu, Qingyun Wang . Effect analysis of pinning and impulsive selection for finite-time synchronization of delayed complex-valued neural networks. Electronic Research Archive, 2025, 33(3): 1792-1811. doi: 10.3934/era.2025081 |
[8] | Minglei Fang, Jinzhi Liu, Wei Wang . Finite-/fixed-time synchronization of leakage and discrete delayed Hopfield neural networks with diffusion effects. Electronic Research Archive, 2023, 31(7): 4088-4101. doi: 10.3934/era.2023208 |
[9] | Qianqian Zhang, Mingye Mu, Heyuan Ji, Qiushi Wang, Xingyu Wang . An adaptive type-2 fuzzy sliding mode tracking controller for a robotic manipulator. Electronic Research Archive, 2023, 31(7): 3791-3813. doi: 10.3934/era.2023193 |
[10] | Moutian Liu, Lixia Duan . In-phase and anti-phase spikes synchronization within mixed Bursters of the pre-Bözinger complex. Electronic Research Archive, 2022, 30(3): 961-977. doi: 10.3934/era.2022050 |
This article focuses on achieving fixed-time synchronization (FxTS) of nonlinear coupled memristive neural networks (NCMMN) with time delays. We propose a novel integrable sliding-mode manifold (SMM) and develop two control strategies (chattering or non-chattering) to achieve FxTS. By selecting appropriate parameters, some criteria are established to force the dynamics of NCMMN to reach the designed SMM within a fixed time and remain on it thereafter. Additionally, they provide estimations for the settling time (TST). the validity of our results is demonstrated through several numerical examples.
In 1971, as the fourth basic circuit element, memristor was proposed by Chua [1]. After a while, it did not attract widespread attention. In 2008, the research team from the Hewlett-Packard (HP) laboratory successfully verified the memristor, which was proposed in 1971, by using the TiO2 material as the main body and extracting both ends from platinum electrodes made of metal respectively [2]. Memristors have better properties than resistors because it has the memory function, which makes itself have widespread applications in many fields, such as information processing, industrial automation, combinatorial optimization and knowledge acquisition [3,4,5,6,7]. Over the past few years, there have been comprehensive researches on neural networks, including image and signal processing, secure communication, pattern recognition, and associative memories [8,9,10,11,12,13,14]. As a result, there has been an increasing focus on memristive neural networks (MNN) and their potential applications. Many studies have been conducted to investigate the dynamic behavior of MNNs. For instance, [15] examined memristive Cohen-Grossberg NNs with stochastic parameter perturbations. In [16], a general class of MNNs with time delays was investigated. Additionally, [17] considered stochastic MNNs subjected to deception attacks.
Synchronization becomes a hot topic in the studies on MNN. Based on different control mechanisms, asymptotic synchronization of MNN has been studied in [18,19,20]. However, synchronisation time is an important indicator of control performance. As a result, finite-time synchronisation is proposed, which has better disturbance rejection properties [21,22,23] and TST is a finite number. Afterward, the paper [24] investigated finite-time synchronization of complex chaotic systems with network transmission mode. Another paper, [25] discussed the finite-time stabilizability and instabilizability of delayed MNN. In [26], Tang et al. studied the finite-time cluster synchronization of discontinuous Lur'e networks. In fact, it is important to note that TST of finite-time synchronization is heavily dependent on the initial values, which can limit practical applications. To address this issue, the concept of FxTS was introduced in [27] for those systems in an unknown environment (initial values are unavailable). Then, Mishra et al. researched consensus for second-order multi-agent systems by designing SMM within a fixed-time time in [28]. [29] addressed cluster synchronization through fixed-time pinning control. However, controllers in the works mentioned above have the signal function, which may lead to chatter and thus shorten the service life of the controller to a certain extent. To overcome the shortcoming, Yang et al. put forward a non-chattering controller without sign(⋅) to research FxTS in [30]. The articles referenced as [31] and [32] respectively discuss FxTS of coupled MNN using event-triggered control.
There has been extensive research on sliding mode control (SMC). The main advantage of SMC is that it has strong robustness to system with uncertainties and external interference. It only needs to estimate the boundary of the disturbance without measuring its specific value, and it is easy to be realized. The dynamic performance of SMC is determined by SMM. The most commonly used SMM is linear. A typical feature of such a linear SMC system is that the convergence of the system state at the equilibrium point is asymptotic rather than within a fixed time. Usually, the gain of the controller is enhanced to enable faster synchronization of the system. However, noting the actual system control limitation, a high-gain sliding mode controller is difficult to obtain, and the gain may cause system instability. To solve this problem, the nonlinear sliding mode is developed. As is known to all, the design of SMC is a two-step process: (1) Design a SMM having the properties we expect. (2) Design the corresponding controller to provide he dynamics of the system trajectory to SMM in a finite time. Once the system reaches the designed SMM, it exhibits the desired robust performance. Numerous studies have focused on the development of SMC techniques, as evidenced by a large body of research [33,34]. In [35], Corradini et al. proposed a novel nonsingular terminal SMC approach for stabilizing second-order systems in fixed time. Additionally, to achieve FxTS of complex networks, a second-order SMC strategy was presented in [36]. In view of disturbances influence, finite-time synchronization and FxTS of MNN were discussed via SMC in [37]. Considering the extensive application of MNN and the advantages of FxTS control, it is imperative to study FxTS of MNN with non-chattering control. Furthermore, FxTS of NCMNNs with non-chattering SMC is more challenging. Therefore, in this paper, we will also study the FxTS of NCMNNs by constructing a non-chattering controller.
Inspired by the above, this paper will study FxTS of NCMNNs with time delays. Main contributions of this article are listed below: (1) To achieve FxTS, SMM designed do not contain signal functions, which is different from [37]. (2) Two kinds of sliding-mode control strategies (chattering or non-chattering) are designed to achieve fixed-time synchronization. In contrast to [28,29,37], the non-chattering controller designed in this paper does not contain signal functions, thus avoiding the drawback of shortening the controller lifetime due to controller chattering. (3) Using the irrational number π and trigonometric function, the estimation of TST is more accurate than most existing results.
The remaining of this paper is formed as below. Section 2 presents the preliminaries, while Section 3 introduces the main findings. In Section 4, several numerical examples and simulations are provided. Finally, Section 5 offers the conclusion.
Notations: N={1,2,…,N} and N={1,2,…,n}, where N and n are positive integers. The n×1 column vector of all ones is denoted by 1. Rn and Rn×n are the set consisting of all n-dimensional real vectors and n×n real matrices, respectively. R stands for real number set. Given a vector ˜x=[˜x1,˜x2,…,˜xn]T, define ˜xh=[˜xh1,˜xh2,…,˜xhn]T, |˜x|=[|˜x1|,|˜x2|,…,|˜xn|]T and Sign(˜x(t))=diag{sign(˜x1(t)),sign(˜x2(t)),…,sign(˜xn(t))}. Let ||⋅|| be 2-norm of a matrix or a vector.
Consider CMNNs containing N nodes as below
˙vi(t)=−Cvi(t)+A(vi(t))f(vi(t))+B(vi(t))f(vi(t−τ(t)))+N∑j=1gij(g(vj(t))−g(vi(t))), | (2.1) |
where vi(t)=(vi1(t),vi2(t),…,vin(t))T∈Rn is the state vector of the i-th node, i∈N, C=diag{c1,c2,…,cn}>0; the coupled matrix G=(gij)N×N satisfies gii=0 and gij=gji≥0, for i≠j; τ(t) is time-varying delay satisfying 0≤τ(t)≤τ. The activation function f: Rn×[0,+∞)→Rn. In addition, A(vi(t))=(ars(fs(vis(t))−vir(t)))n×n≜(ars(vir(t)))n×n and B(vi(t))=(brs(fs(vis(t−τ(t)))−vir(t)))n×n≜(brs(vir(t)))n×n denote the connection weight matrix without and with delayed time, respectively, in which
ars(vir(t))={a′rs,D−firs(t)<0,a″rs,D−firs(t)>0,ars(t−),D−firs(t)=0,brs(vir(t))={b′rs,D−firs(t−τ(t))<0,b″rs,D−firs(t−τ(t))>0, s,r∈N,brs(t−),D−firs(t−τ(t))=0, |
and D−firs(t) means the left derivation of firs(t) in t. Defined the Laplacian matrix L=D−G=[lij]N×N∈RN×N with
lij={−gij,i≠j,N∑j=1,j≠igij,i=j. |
vi(˜ϖ)=ϕvi(˜ϖ)=(ϕvi1(˜ϖ),ϕvi2(˜ϖ),…,ϕvin(˜ϖ))T∈C([−τ(t),0],Rn) is the initial value of system (2.1), for i∈N. The dynamics of the corresponding response system (2.2) of the drive system (2.1) is
˙di(t)=−Cdi(t)+A(di(t))f(di(t))+B(di(t))f(di(t−τ(t)))+N∑j=1gij(g(dj(t))−g(di(t)))+ui(t), | (2.2) |
where ui(t)=(ui1(t),ui2(t),…,uin(t))T is the controller to be designed. Similarly, A(di(t))=(a∗rs(fs(dis(t))−dir(t)))n×n≜(a∗rs(dir(t)))n×n and B(di(t))=(a∗rs(fs(dis(t))−dir(t)))n×n≜(a∗rs(dir(t)))n×n
a∗rs(vir(t))={a∗′rs,D−f∗irs(t)<0,a∗″rs,D−f∗irs(t)>0,a∗rs(t−),D−f∗irs(t)=0,b∗rs(vir(t))={b∗′rs,D−f∗irs(t−τ(t))<0,b∗″rs,D−f∗irs(t−τ(t))>0,b∗rs(t−),D−f∗irs(t−τ(t))=0. |
The initial value of system (2.2) is given by di(˜ϖ)=ϕdi(˜ϖ)=(ϕdi1(˜ϖ),ϕdi2(˜ϖ),…,ϕdin(˜ϖ))T∈C([−τ(t),0],Rn).
Let hi(t)≜di(t)−vi(t), i∈N. Subtracting (2.2) from (2.1), it is obvious that
˙hi(t)=−Chi(t)+A(di(t))˜f(hi(t))+B(di(t))˜f(hi(t−τ(t)))−N∑j=1lij˜g(hj(t))+(A(di(t))−A(vi(t)))f(vi(t))+(B(di(t))−B(vi(t)))f(vi(t−τ(t)))+ui(t), |
where ˜f(hi(t))≜f(di(t))−f(vi(t)), ˜g(hj(t))≜g(dj(t))−g(vj(t)), h(t)=[hT1(t),hT2(t),…,hTN(t)]T∈RNn.
Definition 1. [37] The NCMNNs (2.1) and (2.2) can achieve FxTS via controllers designed if there exists a finite positive constant T such that limt→T||di(t)−vi(t)||=0 and ||di(t)−vi(t)||≡0 when t≥T, for ∀i∈N, where the estimation of TST T depends on some parameters of controllers designed and network.
Lemma 1. [38] For error system (2.3), if the C-regular function V(h(t)):RNn→R meets
ddtV(h(t))≤−ϵVδ(h(t))−κVθ(h(t)), |
where θ∈[0,1), δ>1, ϵ,κ>0, then the origin is fixed-time stable, that is, NCMNNs (2.1) and (2.2) can realize FxTS, and TST T1 is estimated to be
T(h0)≤T1=π(δ−θ)κ(κϵ)ςcsc(ςπ), |
where ς=1−θδ−θ.
Lemma 2. [39] Given a vector z∈Rn. If 0<ι<ω holds, then
||z||ω≤||z||ι≤n1ι−1ω||z||ω, |
where ||z||ω=(∑ni=1|zi|ω)1ω and ||z||ι=(∑ni=1|zi|ι)1ι.
Assumption 1. [40] As for the nonlinear functions fk(⋅) and gk(⋅), assume there exist constants ϖk,mk,ρk>0 such that
|fk(ˆv)|≤ϖk, |fk(ˆv)−fk(ˆy)|≤mk|ˆv−ˆy|, |
and
|gk(ˆv)−gk(ˆy)|≤ρk|ˆv−ˆy|, |
where ˆv,ˆy∈R, k∈N.
In this section, we present two novel SMM. Moreover, two corresponding different kinds of SMC are designed to achieve FxTS of SMM.
First, SMM is described as follows:
Ω={s(t)|s(t)=(sT1(t),sT2(t),…,sTN(t))T=0}, |
where si(t)=(si1(t),si2(t),…,sin(t))T∈Rn. Its sliding variable as
si(t)=kihi(t)+∫t0Sign(hi(τ))(ϵ|hi(τ)|δ+κ|hi(τ)|θ)dτ, |
where ki>0, ϵ,κ>0, δ>1 and 0≤θ<1 are constants.
Construct the following controller
ui(t)=−1kiSign(hi(t))(ϵ|hi(t)|δ+κ|hi(t)|θ)−Sign(si(t))ηi(t), | (3.1) |
where ηi(t)=γi||hi(t)||1+∑j∈Niξj||hj(t)||1+φi1+ϵ1|si(t)|δ1+κ1|si(t)|θ1, the parameters γi>0, ξj>0, j∈Ni, φi>0. And ϵ1>0, κ1>0, 0≤θ1<1, δ1>1.
Suppose the trajectories of error system (2.3) can reach SMM Ω within fixed-time T1 under controller (3.1). In other words, one has
si(t)=˙si(t)=0, |
for all t≥T1, i.e.
˙si(t)=ki˙hi(t)+Sign(hi(t))(ϵ|hi(t)|δ+κ|hi(t)|θ)=0. | (3.2) |
Then, dynamics of the error system on SMM is
˙hi(t)=−1kiSign(hi(t))(ϵ|hi(t)|δ+κ|hi(t)|θ). | (3.3) |
Theorem 1. Suppose Assumption 1 holds. If the following inequality is satisfied
||C||+||ˆA∗||||M||−γi≤0, (H1)∑j∈Ni(|lij|||Φ||−ξj)≤0, (H2)||ˆB∗||||W||+||ˉA||||W||+||ˉB||||W||−φi≤0, (H3) |
where ˆA∗=(ˆa∗kl)n×n, ˆa∗kl=max{|a∗′kl|,|a∗″kl|}, ˆB∗=(ˆb∗kl)n×n, ˆb∗kl=max{|b∗′kl|,|b∗″kl|}, ˉA=(ˉakl)n×n, ˉakl=max{|a∗′kl−a′kl|,|a∗′kl−a″kl|,|a∗″kl−a′kl|,|a∗″kl−a″kl|}, ˉB=(ˉakl)n×n, ˉbkl=max{b∗′kl−b′kl|,|b∗′kl−b″kl|,|b∗″kl−b′kl|,|b∗″kl−b″kl|}, M=diag{m1,m2,⋯,mn}, W=diag{ϖ1,ϖ2,⋯,ϖn}, Φ=diag{ρ1,ρ2,⋯,ρn}, then the following two conclusions are true.
(a) Under the control of controller (3.1), the error system (2.3) is capable of achieving SMM within T1 and maintaining its stability thereafter. TST can be estimated using the following equation
T1=T{1}1=π(δ1−θ1)κ∗1(κ∗1ϵ∗1)ς1csc(ς1π), |
where ˇk=mini∈N{ki}, ϵ∗1=2(nN2)1−δ12ˇkϵ1, κ∗1=2θ1+12ˇkκ1, ς1=1−θ1δ1−θ1.
(b) For system (3.3), it is fixed-time stable. And TST can be estimated as T2
T2=T{1}2=ˆkπ(δ−θ)κ(κϵ)ςcsc(ςπ), |
where ˆk=maxi∈N{ki}, ς=1−θδ−θ.
Proof: Our conclusion (a) will be proved at first. Construct the Lyapunov function as
V1(t)=12sT(t)s(t). |
Calculate the derivative of V1(t) with respect to time t
˙V1(t)=N∑i=1sTi(t)(ki˙hi(t)−Sign(hi(t))(−ϵ|hi(t)|δ−κ|hi(t)|θ))=N∑i=1kisTi(t)(−Chi(t)+A(di(t))˜f(hi(t))+B(di(t))˜f(hi(t−τ(t)))−N∑j=1lij˜g(hj(t))+(A(di(t))−A(vi(t)))f(vi(t))+(B(di(t))−B(vi(t)))f(vi(t−τ(t)))−Sign(si(t))ηi(t)), | (3.4) |
where
−sTi(t)Chi(t)≤||C||||si(t)||||hi(t)||. | (3.5) |
Using the boundary of the active function, it is obvious
sTi(t)B(di(t))˜f(hi(t−τ(t)))=n∑k=1n∑l=1b∗kl(dik(t))sik(t)˜fl(hil(t−τ(t)))≤n∑k=1n∑l=1ˆb∗klϖl|sik(t)|≤|si(t)|TˆB∗W1≤n12||si(t)||||ˆB∗||||W||. | (3.6) |
From Assumption 1, the following inequalities hold
sTi(t)A(di(t))˜f(hi(t))=n∑k=1n∑l=1a∗kl(dik(t))sik(t)˜fl(hil(t))≤n∑k=1n∑l=1ˆa∗klml|sik(t)||hil(t)|=|si(t)|TˆA∗M|hi(t)|≤||si(t)||||ˆA∗||||M||||hi(t)||, | (3.7) |
and
sTi(t)(A(di(t))−A(vi(t)))f(vi(t))=n∑k=1n∑l=1(akl(dik(t))−akl(vik(t)))sik(t)fl(vil(t))≤|si(t)|TˉAW1≤n12||si(t)||||ˉA||||W||, | (3.8) |
and
sTi(t)(B(di(t))−B(vi(t)))f(vi(t−τ(t)))=n∑k=1n∑l=1(bkl(dik(t))−bkl(vik(t)))sik(t)fl(vil(t−τ(t)))≤|si(t)|TˉBW1≤n12||si(t)||||ˉB||||W||, | (3.9) |
and
−N∑i=1N∑j=1lijsTi(t)˜g(hj(t))≤N∑i=1∑j∈Ni|lij|n∑k=1ρk|sik(t)||hjk(t)|=N∑i=1∑j∈Ni|lij||si(t)|TΦ|hj(t)|≤N∑i=1∑j∈Ni|lij|||si(t)||||Φ||||hj(t)||. | (3.10) |
Because of Lemma 2, one has
−N∑i=1sTi(t)Sign(si(t))ηi(t)=−N∑i=1|si(t)|T(γi||hi(t)||1+∑j∈Niξj||hj(t)||1+φi1+ϵ1|si(t)|δ1+κ1|si(t)|θ1)≤−N∑i=1(γi||hi(t)||||si(t)||+∑j∈Niξj||hj(t)||||si(t)||+φi||si(t)||)−N∑i=1|si(t)|T(ϵ1|si(t)|δ1+κ1|si(t)|θ1). | (3.11) |
In the light of (3.4)–(3.11), there is
˙V1(t)≤N∑i=1ki((||C||+||ˆA∗||||M||−γi)||hi(t)||||si(t)||+∑j∈Ni(|lij|||Φ||−ξj)||si(t)||||hj(t)||+n12(||ˆB∗||||W||+||ˉA||||W||+||ˉB||||W||−φi)||si(t)||)−N∑i=1ki|si(t)|T(ϵ1|sik(t)|δ1+κ1|sik(t)|θ1)≤−N∑i=1ki|sik(t)|(ϵ1|sik(t)|δ1+κ1|sik(t)|θ1)≤−ϵ∗1V1+δ121(t)−κ∗1V1+θ121(t). |
According to Lemma 1, SMM is reached in fixed time T1.
Next, the conclusion (b) will be verified. The Lyapunov function is chosen as
V2(t)=N∑i=1n∑k=1|hik(t)|. |
Computing the derivative of V2(t) with respect to time t, we have
˙V2(t)=N∑i=1n∑k=1sign(hik(t))˙hik(t)≤−1ˆk(ϵVδ2(t)+κVθ2(t)). |
From Lemma 1, system (3.3) is stable in fixed time T2.
Remark 1. If Assumptions 1 and (H1)−(H3) hold, NCMNNs (2.2) can be synchronized to NCMNNs (2.1) in fixed time T′ under sliding-mode controller (3.1). In addition, the corresponding TST can be estimated as T′=T1+T2.
Remark 2. The first term in the controller (3.1) is used to counteract the integral term in the sliding mode dynamics, and the second term is to force the error system (2.3) to reach SMM in T2.
Consider linear CMNNs with time delays and dynamics of the corresponding error system is
˙hi(t)=−Chi(t)+A(di(t))˜f(hi(t))+B(di(t))˜f(hi(t−τ(t)))−N∑j=1lijhj(t)+(A(di(t))−A(vi(t)))f(vi(t))+(B(di(t))−B(vi(t)))f(vi(t−τ(t)))+ui(t). | (3.12) |
Corollary 1. Suppose Assumption 1 holds. System (3.12) can be stable in fixed time T′ under controller (3.1) if conditions (H1),(H3) in Theorem 1 and
∑j∈Ni(|lij|−ξj)≤0 (H4) |
can be satisfied.
If the system parameters of the nonlinear coupled network are state-independent, the corresponding error system dynamics can be expressed as
˙hi(t)=−Chi(t)+A˜f(hi(t))+B˜f(hi(t−τ(t)))−N∑j=1lij˜g(hj(t))+ui(t). | (3.13) |
Corollary 2. Suppose Assumption 1 holds. Then, system (3.13) is stable in T′ under controller (3.1) if (H2) and the following (H5) and (H4) hold
||C||+||ˆA||||M||−γi≤0, (H5)||ˆB||||W||−φi≤0, (H6) |
where ˆA=(|aij|)n×n, ˆB=(|bij|)n×n.
Remark 3. Since what we can observe is mainly the corresponding nonlinear function of state variables, nonlinearly coupled networks are more practical. And networks studied are parameter mismatched, which is also more realistic. Besides, we always hope to achieve our desired goal within finite or even fixed time. Therefore, FxTS of the NCMNN discussed in this article possess great meanings in application.
Remark 4. Controller (3.1) contains the signal function, which plays important roles for fixed-time strategy. However, sign(⋅) in the designed controllers will shorten the service life of the machine and cause undesirable oscillations. Therefore, a novel controller will be designed to overcome this shortcoming.
A novel SMC protocol will be presented to force NCMNNs (2.1) and (2.2) to achieve synchronization in this subsection. The sliding-mode function can be designed as
si(t)=kihi(t)+∫t0(ϵ3hqpi(τ)+κ3hmhi(τ))dτ, |
where ki>0, ϵ3,κ3>0, m,h,p,q>0 are all odd numbers, and m<h,q>p. Then SMM can be obtained
Ω′={s(t)|s(t)=(sT1(t),sT2(t),…,sTN(t))T=0}, |
where si(t)=(si1(t),si2(t),…,sin(t))T∈Rn.
The controller is constructed as
ui(t)={−1ki(ϵ3hqpi(t)+κ3hmhi(t))−(ϵ2sq1p1i(t)+κ2sm1h1i(t))−si(t)||si(t)||η∗i(t),si(t)≠0,0,si(t)=0, | (3.14) |
where η∗i(t)=γi||hi(t)||+∑j∈Niξj||hj(t)||+φi, the parameters ϵ2>0, κ2>0, m<h,m1<h1, q>p, q1>p1 and m,m1,h,h1,p,p1,q,q1>0 are all odd numbers.
Remark 5. Note that controller (3.14) is continuous and does not contain the function sign(⋅). As we all know, the signal function in the controllers is indispensable in the study of finite-time synchronization or FxTS [24,25,26,27,28,29]. In this paper, the function sign(⋅) is replaced by two odd ratios so that FxTS can be realized. Besides, from controller (3.14), we can see that our designed controller does not use global information, but only uses the neighbor information of node i, which makes the controller we designed more practical. In this paper, FxTS will be investigated under controller (3.14) without the signal function.
When the error system reaches SMM, it means
˙si(t)=ki˙hi(t)+ϵ3hqpi(t)+κ3hmhi(t)=0. | (3.15) |
Then, the error system on SMM is
˙hi(t)=−1ki(ϵ3hqpi(t)+κ3hmhi(t)). | (3.16) |
Theorem 2. Let Assumption 1 hold, then the following conclusions are true.
(a) System (2.3) can arrive at SMM in fixed time T3 under controller (3.14) if inequalities (H1)−(H3) can be satisfied. TST can be estimated as T3:
T3=T{1}3=h1p1π(h1q1−m1p1)κ∗2(κ∗2ϵ∗2)ς2csc(ς2π), |
where ϵ∗2=2(nN2)p1−q12p1ˇkϵ2, κ∗2=2m1+h12h1ˇkκ2, ς2=p1(h1−m1)h1q1−m1p1.
(b) For system (3.16), it is fixed-time stable. TST can be estimated as T4:
T4=T{1}4=hpπκ∗3(hq−mp)(κ∗3ϵ∗3)ς3csc(ς3π), |
where ϵ∗3=(nN2)p−q2p2ϵ3ˆk, κ∗3=2m+h2hκ3ˆk, ς3=p(h−m)hq−mp.
Proof: First of all, the conclusion (a) can be proved. The Lyapunov function can be selected as
V3(t)=12sT(t)s(t). |
When si(t)≠0, we have
−N∑i=1sTi(t)si(t)||si(t)||η∗i(t)=−N∑i=1||si(t)||(γi||hi(t)||+∑j∈Niξj||hj(t)||+φi). |
Similarly to Theorem 1, one has
˙V3(t)=N∑i=1sTi(t)(ki˙hi(t)+ϵ3hqpi(t)+κ3hmhi(t))≤−ˇkN∑i=1n∑k=1sik(t)(ϵ2sq1p1ik(t)+κ2sm1h1ik(t))≤−ϵ∗2Vq1+p12p13(t)−κ∗2Vm1+h12h13(t). |
Based on Lemma 1, system (2.3) will reach SMM in fixed time T3 under controller (3.14).
Then, the proofs of the conclusion (b) are given as below. The Lyapunov function is
V4(t)=12hT(t)h(t), |
which follows
˙V4(t)=N∑i=1hTi(t)˙hi(t)=−N∑i=11kihTi(t)(ϵ3hqpi(t)+κ3hmhi(t))≤−ϵ∗3Vq+p2p4(t)−κ∗3Vm+h2h4(t). |
From Lemma 1, system (3.16) is stable in T4.
Remark 6. If Assumption 1 and (H1)−(H3) can be satisfied, NCMNNs (2.2) can be synchronized to NCMNNs (2.1) in fixed time T″ under controller (3.14). TST can be estimated as T″=T3+T4. Note that in controller (3.14), if si(t)=0 for some point t, then system (2.3) can arrive at SMM and there is no more need for control input.
Corollary 3. Suppose Assumption 1 holds. System (3.12) can stable in fixed time T″ with controller (3.14) if (H1), (H3), (H4) can be satisfied.
Corollary 4. Suppose Assumption 1 holds, and then, system (3.13) can be stable in fixed time T″ with controller (3.14) if (H2),(H5),(H6) can be satisfied.
Remark 7. The idea of replacing the role of the signal function with two odd ratios qp was proposed in [30]. However, this paper focuses on networks with matched parameter. Moreover, the ability of the designed controller in this paper is stronger than one in [30] for resisting external interference. Therefore, this controller in [30] cannot be used in the NCMNNs considered in this paper.
Remark 8. Unlike [31,32], based on SMM, this paper studies FxTS of NCMNNs, where TST dose not depend on the initial values of controlled network. And a non-chattering sliding-time control strategy is adopted in this paper to realize FxTS of NCMNNs. Actually, due to the limited communication resources, event-triggered mechanism [33,34] research on FxTS is becoming more and more popular, which is also our concern in the future.
Consider three-neuron NCMNNs with time delay examples
˙di(t)=−Cdi(t)+A(di(t))f(di(t))+B(di(t))f(di(t−τ(t)))+N∑j=1gij(g(dj(t))−g(di(t)))+ui(t), |
where d(t)=(dT1(t),rT2(t),rT3(t))T, di(t)=(di1,di2)T, C=diag{1,1}. The time delay can be chosen as τ(t)=et1+et. The weight matrices of CMNNs are as follows
A(di(t))=(a∗11(di1(t))a∗12(di1(t))a∗21(di2(t))a∗22(di2(t))),B(di(t))=(b∗11(di1(t))b∗12(di1(t))b∗21(di2(t))b∗22(di2(t))), |
where a∗11(di1)=1, a∗21(di2)=1, b∗12(di1)=−1, b∗21(di2)=1, and
a∗12(di1(t))={2,D−fi12(t)<0,6,D−fi12(t)>0,a∗12(t−),D−fi12(t)=0,a∗22(di2(t))={3.5,D−fi22(t)<0,4.2,D−fi22(t)>0,a∗22(t−),D−fi22(t)=0, |
b∗11(di1(t))={2,D−fi11(t−1)<0,−1,D−fi11(t−1)>0,b∗11(t−),D−fi11(t−1)=0,b∗22(di2(t))={−2,D−fi22(t−1)<0,3,D−fi22(t−1)>0,b∗22(t−),D−fi22(t−1)=0. |
The coupled matrix of the three-node network is
G=(010101010). |
Then corresponding Laplacian matrix is
L=(1−10−12−10−11). |
The activation functions are defined as fi(v)=(fi1(v1),fi2(v2))T, i=1,2,3, fil(⋅)=|(⋅)+1|−|(⋅)−1|2, l=1,2, where v=(v1,v2)T, such that ml=ϖl=1. And the nonlinear coupled function is selected as gi(v)=(gi1(v1),gi2(v2))T, gi1(v1)=2v1+0.2sinv1, gi2(v2)=5v2+0.5cosv2, such as ρi1=2.2, ρi2=5.5.
The weight matrices of corresponding drive system are as follows
A(vi(t))=(a11(vi1(t))a12(vi1(t))a21(vi2(t))a22(vi2(t))),B(vi(t)=(b11(vi1(t))b12(vi1(t))b21(vi2(t))b22(vi2(t))), |
where a11(vi1)=5, a21(vi2)=1, b12(vi1)=2.1, b22(vi2)=−0.3, and
a12(vi1(t))={−0.3,D−fi12(t)<0,−0.5,D−fi12(t)>0,a12(t−),D−fi12(t)=0,a22(vi2(t))={1.4,D−fi22(t)<0,1.6,D−fi22(t)>0,a22(t−),D−fi22(t)=0, |
b11(vi1(t))={2.8,D−fi11(t−1)<0,2.7,D−fi11(t−1)>0,b11(t−),D−fi11(t−1)=0,b21(vi2(t))={0.5,D−fi21(t−1)<0,0.2,D−fi21(t−1)>0,b22(t−),D−fi21(t−1)=0. |
Example 1. To achieve FxTS of corresponding error system, the sliding-mode function is designed as
si(t)=kihi(t)+∫t0Sign(hi(τ))(ϵ|hi(τ)|δ+κ|hi(τ)|θ)dτ. |
Then, for controller (3.1), choose ki=1, ϵ=1.4, δ=2, κ=2, θ=0.4, ϵ1=1.5, δ1=1.6, κ1=2, θ1=0.4, γi=5, ξj=10, φi=15.
Figure 1(a) and (b) describe the trajectory of the error system and the sliding mode variable, respectively. From Figure 1, we can observe that both the error and sliding mode variables tend to zero within T′=7, which means that the error system dynamics can reach SMM within T′=7 and the error tends to zero along SMM. Besides, TST can be estimated as T′=9.1 from Theorem 1, which is larger than the actual time required.
Moreover, we can calculate that synchronization can be achieved within 29.3 when k=1 in Lemma 6 [39]. Compared with the estimation of TST used in [39], it is obvious that the estimated time in this article is more accurate than one in [39].
Example 2. The signal function in the designed controllers as coefficients will shorten the service life of the machine and cause undesirable oscillations. Therefore, another controller (3.14) is presented to overcome these difficulties.
And the corresponding sliding mode variable is designed as
si(t)=kihi(t)+∫t0(ϵ3hqpi(τ)+κ3hmhi(τ))dτ. |
Choose ϵ3=1.5, qp=97, κ3=2, mh=13, ϵ2=5, q1p1=97, κ2=3, m1h1=13.
It can be seen from (a) in Figure 2 that drive and response NCMNNs can be synchronized under controller (3.14) within T″=9. (b) shows the trajectory of sliding mode variable tends to zero within T″=9. However, according to Theorem 2, TST can be estimated as T″=10.4, which is larger than the actual time required. Furthermore, TST can be estimated as T″=143.2 in [39]. Therefore, the estimated time in this article is more accurate than one in [39].
Remark 9. In the process of simulations, we can find that there is a unified upper bound on TST of FxTS with arbitrary initial values, which further shows that FxTS does not depend on the initial value. Therefore, FxTS has a wide range of applications in various fields.
This paper discusses the use of SMC to achieve FxTS of NCMNNs with time delays. The controllers are established using a novel integral SMC technology, which has several advantages. Two different control strategies, chattering and non-chattering, are employed to achieve FxTS, meaning that the error system's orbit can reach designed SMM in a fixed time and remain there thereafter. And the dynamics of the error system on SMM can converge to zero within a fixed time. In addition, TST can be estimated more accurately than most existing literature. However, in some sense, the controllers designed in this paper are complicated, and improvements about controllers should be made in our future work. Meanwhile, it is more interesting and challenging to increase the accuracy of estimation of TST.
This work was supported in part by the National Natural Science Foundation of China under Grant 61873171, in part by the Natural Science Foundation of Guangdong Province, China under Grant 2019A1515012192.
The authors declare that they have no known competing financial interests or personal relationships that could have appeared to influence the work reported in this paper.
[1] | L. Chua, Memristor the missing circuit elemen, IEEE Trans. Circuit Theory, 18 (1971), 507–519. |
[2] |
D. Strukov, G. Snider, D. Stewart, R. Williams, The missing memristor found, Nature, 453 (2008), 80–83. https://doi.org/10.1038/nature06932 doi: 10.1038/nature06932
![]() |
[3] |
W. Lin, G. Chen, Large memory capacity in chaotic artificial neural networks: A view of the anti-integrable limit, IEEE Trans. Neural Netw., 20 (2009), 1340–1351. https://doi.org/10.1109/TNN.2009.2024148 doi: 10.1109/TNN.2009.2024148
![]() |
[4] |
V. Yuriy, D. Massimiliano, Experimental demonstration of associative memory with memristive neural networks, Neural Netw., 23 (2010), 881–886. https://doi.org/10.1016/j.neunet.2010.05.001 doi: 10.1016/j.neunet.2010.05.001
![]() |
[5] |
M. Zhao, Algebraic criteria for reachable set estimation of delayed memristive neural networks, IET Control Theory Appl., 13 (2019), 1736–1743. https://doi.org/10.1049/iet-cta.2018.5959 doi: 10.1049/iet-cta.2018.5959
![]() |
[6] |
S. Adhikari, C. Yang, H. Kim, L. Chua, Memristor bridge synapse-based neural network and its learning, IEEE Trans. Neural Netw. Learn. Syst., 23 (2012) 1426–1435. https://doi.org/10.1109/TNNLS.2012.2204770 doi: 10.1109/TNNLS.2012.2204770
![]() |
[7] |
J. Hu, Y. Yang, H. Liu, Non-fragile set-membership estimation for sensor-saturated memristive neural networks via weighted try-once-discard protocol, IET Control Theory Appl., 14 (2020), 1671–1680. https://doi.org/10.1049/iet-cta.2020.0219 doi: 10.1049/iet-cta.2020.0219
![]() |
[8] |
C. Zhou, C. Wang, W. Yao, H. Lin, Observer-based synchronization of memristive neural networks under DOS attacks and actuator saturation and its application to image encryption, Appl. Math. Comput., 425 (2022), 127080. https://doi.org/10.1016/j.amc.2022.127080 doi: 10.1016/j.amc.2022.127080
![]() |
[9] |
J. Cao, W. Jun, Global asymptotic and robust stability of recurrent neural networks with time delays, IEEE Trans. Circuits-I, 52 (2005), 417–426. https://doi.org/10.1109/TCSI.2004.841574 doi: 10.1109/TCSI.2004.841574
![]() |
[10] |
X. Wang, H. Su, F. Zhang, G. Chen, A robust distributed interval observer for LTI systems, IEEE Trans. Autom. Control, 68 (2023), 1337–1352. https://doi.org/10.1109/TAC.2022.3151586 doi: 10.1109/TAC.2022.3151586
![]() |
[11] |
R. Saber, J. Fax, R. Murray, Consensus and cooperation in networked multi-agent systems, Proc. IEEE, 95 (2007), 215–233. https://doi.org/10.1109/JPROC.2006.887293 doi: 10.1109/JPROC.2006.887293
![]() |
[12] |
X. Wang, X. Wang, H. Su, J. Lam, Reduced-order interval observer based consensus for masswith time-varying interval uncertainties, Automatica, 135 (2022), 109989. https://doi.org/10.1016/j.automatica.2021.109989 doi: 10.1016/j.automatica.2021.109989
![]() |
[13] |
J. Wang, C. Xu, J. Feng, M. Chen, X. Wang, Y. Zhao, Synchronization in moving pulse-coupled oscillator networks, IEEE Trans. Circuits-I, 62 (2015), 2544–2554. https://doi.org/10.1109/TCSI.2015.2477576 doi: 10.1109/TCSI.2015.2477576
![]() |
[14] |
Y. Bao, Y. Zhang, B. Zhang, B. Wang, Resilient fixed-time synchronization of neural networks under DOS attacks, J. Frank. Inst., 360 (2023), 555–573. https://doi.org/10.1016/j.jfranklin.2022.09.038 doi: 10.1016/j.jfranklin.2022.09.038
![]() |
[15] |
W. Yao, C. Wang, Y. Sao, C. Zhou, H. Lin, Exponential multistability of memristive Cohen-Grossberg neural networks with stochastic parameter perturbations, Appl. Math. Comput., 386 (2020), 125483. https://doi.org/10.1016/j.amc.2020.125483 doi: 10.1016/j.amc.2020.125483
![]() |
[16] |
A. Wu, Z. Zeng, Exponential stabilization of memristive neural networks with time delays, IEEE Trans. Neural Netw. Learn. Syst., 23 (2012), 1919–1929. https://doi.org/10.1109/TNNLS.2012.2219554 doi: 10.1109/TNNLS.2012.2219554
![]() |
[17] |
Z. Chao, C. Wang, W. Yao, Quasi-synchronization of stochastic memristive neural networks subject to deception attacks, Nonlinear Dynam., 2022 (2022), 1–20. https://doi.org/10.1007/s11071-022-07925-2 doi: 10.1007/s11071-022-07925-2
![]() |
[18] |
W. Lu, T. Chen, G. Chen, Synchronization analysis of linearly coupled systems described by differential equations with a coupling delay, Physica D, 221 (2006), 118–134. https://doi.org/10.1016/j.physd.2006.07.020 doi: 10.1016/j.physd.2006.07.020
![]() |
[19] |
Z. Guo, J. Wang, Z. Yan, Global exponential synchronization of two memristor-based recurrent neural networks with time delays via static or dynamic coupling, IEEE Trans. Syst. Manand Cybern., 45 (2015), 235–249. https://doi.org/10.1109/TSMC.2014.2343911 doi: 10.1109/TSMC.2014.2343911
![]() |
[20] |
C. Zhou, C. Wang, Y. Sun, W. Yao, H. Lin, Cluster output synchronization for memristive neural networks, Inform. Sciences, 589 (2022), 459–477. https://doi.org/10.1016/j.ins.2021.12.084 doi: 10.1016/j.ins.2021.12.084
![]() |
[21] | S. Bhat, D. Bernstein, Finite-time stability of homogeneous systems, in Proceedings of the American control conference, (1997), 2513–2514. https://doi.org/10.1109/ACC.1997.609245 |
[22] |
V. Haimo, Finite-time controllers, SIAM J. Control Optim., 24 (1986), 760–770. https://doi.org/10.1137/0324047 doi: 10.1137/0324047
![]() |
[23] |
Y. Hong, J. Huang, Y. Xu, On an output feedback finite-time stabilization problem, IEEE Trans. Autom. Control, 46, (2001), 305–309. https://doi.org/10.1109/9.905699 doi: 10.1109/9.905699
![]() |
[24] |
X. Chen, T. Huang, J. Cao, Finite-time multi-switching sliding mode synchronisation for multiple uncertain complex chaotic systems with network transmission mode, IET Control Theory Appl., 13 (2019), 1246–1257. https://doi.org/10.1049/iet-cta.2018.5661 doi: 10.1049/iet-cta.2018.5661
![]() |
[25] |
L. Wang, Y. Shen, Finite-time stabilizability and instabilizability of delayed memristive neural networks with nonlinear discontinuous controller, IEEE Trans. Neur. Netw. Learn. Syst., 26 (2015), 2914–2924. https://doi.org/10.1109/TNNLS.2015.2460239 doi: 10.1109/TNNLS.2015.2460239
![]() |
[26] | Z. Tang, J. Park, H. Bao, Finite-time cluster synchronization of discontinuous Lur'e networks via pinning control, in 2016 35th Chinese Control Conference (CCC), (2016), 7206–7210. https://doi.org/10.1109/ChiCC.2016.7554497 |
[27] |
E. Cruz-Zavala, J. Moreno, L. Fridman, Uniform robust exact differentiator, IEEE Trans. Autom. Control, 56 (2011), 2727–2733. https://doi.org/10.1109/TAC.2011.2160030 doi: 10.1109/TAC.2011.2160030
![]() |
[28] |
J. Mishra, C. Li, M. Jalili, X. Yu, Robust second-order consensus using a fixed-time convergent sliding surface in multiagent systems, IEEE Trans. Cybern., 50 (2020), 846–855. https://doi.org/10.1109/TCYB.2018.2875362 doi: 10.1109/TCYB.2018.2875362
![]() |
[29] |
X. Liu, T. Chen, Finite-time and fixed-time cluster synchronization with or without pinning control, IEEE Trans. Cybern., 48 (2018), 240–252. https://doi.org/10.1109/TCYB.2016.2630703 doi: 10.1109/TCYB.2016.2630703
![]() |
[30] |
X. Yang, J. Lam, D. Ho, Fixed-time synchronization of complex networks with impulsive effects via nonchattering control, IEEE Trans. Autom. Control, 62 (2017), 5511–5521. https://doi.org/10.1109/TAC.2017.2691303 doi: 10.1109/TAC.2017.2691303
![]() |
[31] |
J. Cai, J. Feng, J. Wang, Y. Zhao, Tracking consensus of multi-agent systems under switching topologies via novel SMC: An event-triggered approach, IEEE Trans. Netw. Sci. Eng., 9 (2022), 2150–2163. https://doi.org/10.1109/TNSE.2022.3155405 doi: 10.1109/TNSE.2022.3155405
![]() |
[32] |
X. Liu, X. Su, P. Shi, C. Shen, Observer-based sliding mode control for uncertain fuzzy systems via event-triggered strategy, IEEE Trans. Fuzzy Syst., 27 (2019), 2190–2201. https://doi.org/10.1109/TFUZZ.2019.2895804 doi: 10.1109/TFUZZ.2019.2895804
![]() |
[33] |
Y. Bao, Y. Zhang, B. Zhang, Fixed-time synchronization of coupled memristive neural networks via event-triggered control, Appl. Math. Comput., 411 (2021), 126542. https://doi.org/10.1016/j.amc.2021.126542 doi: 10.1016/j.amc.2021.126542
![]() |
[34] |
Y. Bao, Y. Zhang, Fixed-time dual-channel event-triggered secure quasi-synchronization of coupled memristive neural networks, J. Frank. Inst., 358 (2021), 10052–10078. https://doi.org/10.1016/j.jfranklin.2021.10.023 doi: 10.1016/j.jfranklin.2021.10.023
![]() |
[35] |
M. Corradini, A. Cristofaro, Nonsingular terminal sliding-mode control of nonlinear planar systems with global fixed-time stability guarantees, Automatica, 95 (2018), 561–565. https://doi.org/10.1016/j.automatica.2018.06.032 doi: 10.1016/j.automatica.2018.06.032
![]() |
[36] |
Z. Wang, H. Wu, Projective synchronization in fixed time for complex dynamical networks with nonidentical nodes via second-order sliding mode control strategy, J. Frankl. Inst., 355 (2018), 7306–7334. https://doi.org/10.1016/j.jfranklin.2018.07.018 doi: 10.1016/j.jfranklin.2018.07.018
![]() |
[37] |
L. Wang, Z. Zeng, M. Ge, A disturbance rejection framework for finite-time and fixed-time stabilization of delayed memristive neural networks, IEEE Trans. Syst., Man, Cybern., Syst., 51 (2021), 905–915. https://doi.org/10.1109/TSMC.2018.2888867 doi: 10.1109/TSMC.2018.2888867
![]() |
[38] |
C. Hu, H. He, H. Jiang, Fixed/preassigned-time synchronization of complex networks via improving fixed-time stability, IEEE Trans. Cybern., 51 (2021), 2882–2892. https://doi.org/10.1109/TCYB.2020.2977934 doi: 10.1109/TCYB.2020.2977934
![]() |
[39] |
N. Li, X. Wu, J. Feng, Y. Xu, J. Lu, Fixed-time synchronization of coupled neural networks with discontinuous activation and mismatched parameters, IEEE Trans. Neural Netw. Learn. Syst., 32 (2021), 2470–2482. https://doi.org/10.1109/TNNLS.2020.3005945 doi: 10.1109/TNNLS.2020.3005945
![]() |
[40] |
Z. Guo, S. Yang, J. Wang, Global exponential synchronization of multiple memristive neural networks with time delay via nonlinear coupling, IEEE Trans. Neural Netw. Learn. Syst., 26 (2015), 1300–1311. https://doi.org/10.1109/TNNLS.2014.2354432 doi: 10.1109/TNNLS.2014.2354432
![]() |
1. | Yanli Huang, Aobo Li, General decay anti-synchronization and H∞ anti-synchronization of derivative coupled delayed memristive neural networks with constant and delayed state coupling, 2024, 139, 10075704, 108313, 10.1016/j.cnsns.2024.108313 | |
2. | Yan-Li Huang, Ao-Bo Li, General decay anti-synchronization of coupled delayed memristive neural networks with constant and time-varying distributed-delay coupling, 2025, 618, 09252312, 129058, 10.1016/j.neucom.2024.129058 | |
3. | Cheng-Jun 成俊 Xie 解, Xiang-Qing 向清 Lu 卢, Finite time hybrid synchronization of heterogeneous duplex complex networks via time-varying intermittent control, 2025, 34, 1674-1056, 040601, 10.1088/1674-1056/adacc6 |