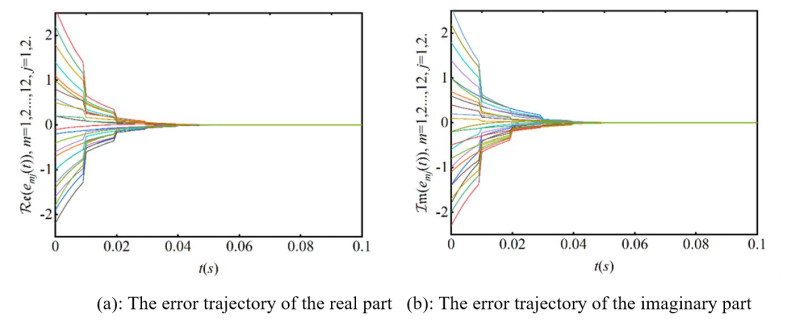
We explored the phenomenon of finite-time synchronization for coupled complex-valued neural networks that were subject to mixed-variable delays. To address this challenge, an impulsive pinning control strategy was proposed. The method presented entailed the dynamic adjustment of specific nodes at distinct impulsive intervals, contingent upon the magnitude of the errors observed in those nodes. Furthermore, an enhanced technique utilizing the sign function was employed to simultaneously manage the real and imaginary components of the complex-valued neural networks. By applying finite-time stability theorems and utilizing complex-valued inequalities, sufficient conditions for achieving finite-time synchronization and determining stability time under the influence of delayed impulsive effects were established. A comprehensive discussion on the interaction between impulsive effects and pinning strategies was also included. It was noted that integrating impulsive effects with pinning ratios enabled precise control over nodes exhibiting significant errors, thereby promoting rapid convergence within finite time frames. Our findings highlight the effectiveness of impulsive pinning control in enhancing synchronization stability, providing significant insights into the practical applications of complex-valued neural networks, particularly in image processing.
Citation: Shuang Liu, Tianwei Xu, Qingyun Wang. Effect analysis of pinning and impulsive selection for finite-time synchronization of delayed complex-valued neural networks[J]. Electronic Research Archive, 2025, 33(3): 1792-1811. doi: 10.3934/era.2025081
[1] | Yilin Li, Jianwen Feng, Jingyi Wang . Mean square synchronization for stochastic delayed neural networks via pinning impulsive control. Electronic Research Archive, 2022, 30(9): 3172-3192. doi: 10.3934/era.2022161 |
[2] | Jiaqi Chang, Xiangxin Yin, Caoyuan Ma, Donghua Zhao, Yongzheng Sun . Estimation of the time cost with pinning control for stochastic complex networks. Electronic Research Archive, 2022, 30(9): 3509-3526. doi: 10.3934/era.2022179 |
[3] | Wanshun Zhao, Kelin Li, Yanchao Shi . Exponential synchronization of neural networks with mixed delays under impulsive control. Electronic Research Archive, 2024, 32(9): 5287-5305. doi: 10.3934/era.2024244 |
[4] | Yu-Jing Shi, Yan Ma . Finite/fixed-time synchronization for complex networks via quantized adaptive control. Electronic Research Archive, 2021, 29(2): 2047-2061. doi: 10.3934/era.2020104 |
[5] | Minglei Fang, Jinzhi Liu, Wei Wang . Finite-/fixed-time synchronization of leakage and discrete delayed Hopfield neural networks with diffusion effects. Electronic Research Archive, 2023, 31(7): 4088-4101. doi: 10.3934/era.2023208 |
[6] | Jun Guo, Yanchao Shi, Shengye Wang . Synchronization analysis of delayed quaternion-valued memristor-based neural networks by a direct analytical approach. Electronic Research Archive, 2024, 32(5): 3377-3395. doi: 10.3934/era.2024156 |
[7] | Chao Yang, Juntao Wu, Zhengyang Qiao . An improved fixed-time stabilization problem of delayed coupled memristor-based neural networks with pinning control and indefinite derivative approach. Electronic Research Archive, 2023, 31(5): 2428-2446. doi: 10.3934/era.2023123 |
[8] | Tianyi Li, Xiaofeng Xu, Ming Liu . Fixed-time synchronization of mixed-delay fuzzy cellular neural networks with $ L\acute{e}vy $ noise. Electronic Research Archive, 2025, 33(4): 2032-2060. doi: 10.3934/era.2025090 |
[9] | Chengbo Yi, Jiayi Cai, Rui Guo . Synchronization of a class of nonlinear multiple neural networks with delays via a dynamic event-triggered impulsive control strategy. Electronic Research Archive, 2024, 32(7): 4581-4603. doi: 10.3934/era.2024208 |
[10] | Yong Zhao, Shanshan Ren . Synchronization for a class of complex-valued memristor-based competitive neural networks(CMCNNs) with different time scales. Electronic Research Archive, 2021, 29(5): 3323-3340. doi: 10.3934/era.2021041 |
We explored the phenomenon of finite-time synchronization for coupled complex-valued neural networks that were subject to mixed-variable delays. To address this challenge, an impulsive pinning control strategy was proposed. The method presented entailed the dynamic adjustment of specific nodes at distinct impulsive intervals, contingent upon the magnitude of the errors observed in those nodes. Furthermore, an enhanced technique utilizing the sign function was employed to simultaneously manage the real and imaginary components of the complex-valued neural networks. By applying finite-time stability theorems and utilizing complex-valued inequalities, sufficient conditions for achieving finite-time synchronization and determining stability time under the influence of delayed impulsive effects were established. A comprehensive discussion on the interaction between impulsive effects and pinning strategies was also included. It was noted that integrating impulsive effects with pinning ratios enabled precise control over nodes exhibiting significant errors, thereby promoting rapid convergence within finite time frames. Our findings highlight the effectiveness of impulsive pinning control in enhancing synchronization stability, providing significant insights into the practical applications of complex-valued neural networks, particularly in image processing.
In recent years, neural networks (NNs) have gained significant attention across various disciplines [1,2]. There has been considerable interest in examining the stability and convergence of real-valued NNs [3,4]. Research on NNs has expanded to include dynamic behaviors such as synchronization, contagion, and cooperative evolution. In reference [5], the authors examined delayed competitive NNs under the framework of progressive synchronization. Subsequently, the focus shifted towards other NNs [6,7]. Researchers have increasingly prioritized finite-time methods due to their advantages, including faster convergence, robustness against disturbances, and resilience to uncertainties. In this context, the authors in [8] introduced a prescribed time control approach for high-order nonlinear systems. The authors in [9] established that a prescribed-time output feedback control algorithm under output constraint occurs in any finite time interval and malicious attacks. The appeal study designs a finite time control algorithm from the point of view of real-valued networks but ignores the importance of complex-valued networks. Therefore, in order to enhance the ability to capture and process phase information from data, complex or complex-valued functions are used in this paper to represent connection weights, activation functions, and state vectors, and the research on complex-valued neural networks (CVNNs) [10] is completed.
Given that most parameters in CVNNs are expressed in complex form, their enhanced performance compared to real-valued NNs positions them to serve distinct functions in specific domains. For instance, a CVNN characterized by orthogonal decision boundaries can effectively address issues related to XOR and symmetry detection [11,12]. In the domain of electromagnetic wave research, phenomena such as wave transmission, phase advancement, delay, reflection, and field superposition can be more intuitively interpreted through the lens of complex numbers [13]. To facilitate the analysis of complex-valued networks, a separation method has been proposed for complex-valued problems [14,15]. Consequently, further investigation into the dynamic evolution of CVNNs is of utmost importance. In the implementation of NNs, the occurrence of time delays is an unavoidable reality. To study the stability and synchronization of CVNNs, many researchers have proposed introducing time delays into the dynamic equations of neural networks [16,17]. Time delay issues are considered critical factors affecting the performance of neural networks, as different types of delays such as discrete delays, time-varying delays, and distributed delays can significantly impact the network's behavior. Research has shown that delays can lead to oscillatory behavior, instability, and even chaotic phenomena, which can adversely affect the synchronization and stability of the network [3,4]. In order to more accurately reflect the time-delay characteristics of real systems and provide a more accurate analysis basis for studying the synchronization, stability, and control strategy design of networks, it is crucial to consider the mixed delay in CVNNs. Therefore, the integration of time delays into CVNNs, along with an examination of their dynamic behaviors to achieve stability within finite time frames, is critically significant. As far as current knowledge extends, methods aimed at enhancing the FTS of CVNNs with mixed-variable delays remain under exploration, which constitutes the primary motivation for this study.
The synchronization dynamics among neurons in NNs play a crucial role in determining the functionality of the network. Different control methods exhibit distinct performance characteristics in relation to synchronization behavior [18,19]. It is noteworthy that impulses act as an effective control mechanism by enabling the relinquishment of continuous monitoring, focusing instead on exerting control at specific moments defined by predetermined impulsive gains. The presence of impulsive effects is a significant factor influencing impulsive control issues. However, not all impulsive effects contribute positively to synchronization; certain effects present within the network or the controller may destabilize the network, while judiciously applied impulsive effects can enhance network convergence [20,21]. Consequently, it is pertinent to investigate the influence of impulsive effects on network stability. Furthermore, pinning control is favored for its ability to selectively manage specific designated nodes rather than imposing control on all nodes across the network. Nonetheless, the adjustability of pinned nodes and the selection criteria for these nodes present challenges within pinning control strategies. Both impulsive and pinning control methods have been extensively utilized in prior research [22]. We propose an impulsive pinning control strategy that integrates the benefits of both pinning and impulsive control, applying it to CVNNs to achieve FTS. Drawing on the insights presented in reference [22], we expand the concept of impulsive pinning control to CVNNs. Additionally, we conduct a thorough analysis of the impulsive and pinning effects on the network. In contrast to the research outlined in reference [14], we incorporate the elements of time delays and impulsive effects into CVNNs.
With respect to control methodologies, the incorporation of a pinning ratio within the impulsive control framework facilitates the dynamic regulation of nodes exhibiting significant errors at discrete time intervals.
(1) The conditions for FTS under mixed delays have been established, and the stability duration corresponding to various types of impulsive gains has been computed.
(2) In the analysis of CVNNs, the use of the sign function method has been proposed, improving the approach of handling real and imaginary components separately. Applying the sign function to both the real and imaginary parts simultaneously simplifies the analysis and enhances the ability to capture the overall behavior of the network.
(3) The moment point of impulsive control is chosen, and the error of the system's state variables is calculated. An algorithm is then used to sort and select nodes with significant errors, with the basis for adjustment determined by the impulsive pinning control ratio ρk. Introducing this pinning ratio within the impulsive control scheme allows for dynamic control of nodes with large errors at discrete instants.
The structure of this paper is organized as follows: In Section 2, we present a detailed introduction to the research topic. In Section 3, we derive the necessary conditions for achieving FTS and calculating the stabilization time by employing the proposed theorems, hypotheses, and methodologies. In Section 4, we offer multiple simulations to evaluate the effectiveness of synchronization, the adaptability of impulsive gains, and the number of pinned nodes. Finally, in Section 5, we summarize the key conclusions of the study.
A class of CVNNs with mixed-variable delays, composed of N identical nodes exhibiting linear diffusive couplings, is considered. The network is described:
˙xm(t)=Dxm(t)+f(xm(t),xm(t−τ1(t)))+c∑Nj=1amjΓxj(t)+c∑Nj=1bmjΓxj(t−τ2(t))+um(t), | (1) |
where xm=(xm1,xm2,…,xmn)H∈Cn represents the matrix of the mth complex-valued neuron variable, which has n dimensions. D=diag{d1,d2,…dn}∈Cn×n and dm denote the neuronal self-inhibition coefficient. c∈C is the neuronal coupling strength. f(⋅)=(f1(⋅),f2(⋅),…,fn(⋅))H∈Cn is the complex-valued activation function. τ1(t) refers to the neuronal internal delay, and τ2(t) denotes the coupling delay between neurons. amj and bmj represent the complex-valued connection weights for non-time-varying and time-varying delays, respectively, and both satisfy the dissipative coupling condition. The internal coupling matrix is Γ=diag{h1,h2,…hn}∈Cn×n. um(t) is the designed impulsive pinning control strategy.
The leader node system s(t) is considered as follows:
˙s(t)=Ds(t)+f(s(t),s(t−τ1(t))), | (2) |
where s(t)=(s1(t),s2(t),…,sn(t))H represents the synchronous trajectory. The matrix D is defined identically to the previously mentioned description. f(s(t),s(t−τ1(t))) and f(xm(t),xm(t−τ1(t))) share the same expression.
Remark 1. Equation (1) proposed in this paper is a simplified version of a more complex model. Depending on the field of application, it can be adapted to different terminologies and forms. For example, understanding the impulsive synchronization in brain networks is crucial for improving diagnostic tools, as it can provide insights into brain disorders [23]. In the engineering field, the same equation can be applied to simulate communication networks, where it plays a vital role in network synchronization and stability analysis [24]. By studying how different layers of the network synchronize, it is possible to optimize communication systems, thereby improving the performance of these networks. In hardware implementations of neural networks, the presence of delays is an inherent issue.
Remark 2. Research indicates that these delays can considerably lead to oscillations, instability, or chaotic behavior [3,4]. Furthermore, the incorporation of delays complicates the analysis of CVNNs, presenting a critical challenge that many researchers are keen to explore [14,18]. Unlike other researchers, we introduce an innovative approach by incorporating a compensation mechanism within the controller to handle delays in the network. By carefully integrating the delay into the control strategy, we effectively compensate for its impact on the system, reducing the interference caused by the delays.
Some fundamental requirements, such as definitions, lemmas, and assumptions, are presented below to prepare for the implementation of FTS.
Definition 1. [25] If the network is Lyapunov stable and there exists T1 > 0 (T1 depends on the initial values), for any m=1,2,...,N such that 1Nlimt→T1N∑m=1‖xm(t)−s(t)‖=0 and when t > T1 then N∑m=1‖xm(t)−s(t)‖/N≡0, the network achieves FTS. Here, T1 is defined as the settling time.
Definition 2. [26] Consider N(T,t) which shows the impulsive count of impulsive sequence {t1,t2,⋯} within a time interval of (t,T). If there exist two constants N0 and Ta such that the inequalities ∀T⩾t⩾0 and
T−tTa−N0⩽N(T,t)⩽T−tTa+N0 |
hold, then it is proposed that the impulsive interval of ζ is less than Ta.
Definition 3. [27] For any z=Re(z)+iIm(z), the function [z]=sgn(Re(z))+isgn(Im(z)) denotes the sign function of z. Similarly, for any complex vector W=(w1,w2,…,wn)H∈Cn, [W]=(sgn(Re(w1))+isgn(Im(w1)),...,sgn(Re(wn))+isgn(Im(wn)))H represents the sign function vector of W.
Assumption 1. The neuron's delay τ1(t) and the coupling delay τ2(t) between neurons are bounded, i.e., 0⩽τ1(t)⩽ˆτ1,0⩽τ2(t)⩽ˆτ2, where ˆτ1,ˆτ2 are positive constants.
Assumption 2. For any x(t),y(t)∈Cn, there exists l1>0, l2>0 such that the nonlinear function f(∙) satisfies (f(x(t),x(t−τ1))−f(y(t),y(t−τ1)))H(f(x(t),x(t−τ1))−f(y(t),y(t−τ1)))≤l1(x(t)−y(t))H(x(t)−y(t)) + l2(x(t−τ1(t))−y(t))H(x(t)−y(t)).
Lemma 1. [22] If V∈V0 and satisfies t≠tk,D+V(t,x)⩽g(t,V(t,x)),t=tk,V(t,x+Δx)⩽Ψi(V(t,x)), then there exists the following comparison system:
{˙v(t)=g(t,V(t,x)),t≠tk,tk>0,v(tk)=Ψi(v(tk−)),k∈N,v(0)=V(0). |
Lemma 2. [14] For any z, the following equation holds true
[z(t)]Hz(t)+z(t)H[z(t)]=2‖z(t)‖1⩾2‖z(t)‖2, |
where for any z=(z1,z2,…,zn)∈Cn, ‖z‖1=n∑m=1|zm|1,‖z‖2=√zHz,, which represent the L1 norm and L2 norm of the vector z respectively, and for zm=x+iy, |zm|=|x|+|y|.
Denote em(t)=xm(t)−s(t)(m=1,2,⋯,N), for amj and bmj satisfying the dissipative coupling condition, that is ∑Nj=1amjΓs(t)=∑Nj=1amjΓs(t−τ2(t))=0. Then, the error system can be simplified as
˙em(t)=Dem(t)+˜f(em(t),em(t−τ1(t)))+c∑Nj=1amjΓej(t)+c∑Nj=1bmjΓej(t−τ2(t))+um(t), | (3) |
where ˜f(em(t),em(t−τ1(t)))=f(xm(t),xm(t−τ1(t)))−f(s(t),s(t−τ1(t))).
Consider the simultaneous requirement for meeting the impulsive conditions and achieving finite-time objectives. Then, our control strategy is bifurcated into two aspects um(t)=u(1)m(t)+u(2)m(t). One aspect is to integrate the principles of impulsive effects into the control strategy, and the other aspect is dedicated to accomplishing finite-time goals. There are many methods for finite-time synchronization, such as achieving synchronization in neural networks through the use of the maximum value method [28] or by applying integral inequality methods [29] alone. Inspired by the approach in reference [30], we extend the finite-time stability theorem, combining inequality methods and various analytical methods, to investigate impulsive control in complex-valued neural networks. The control strategy is outlined as follows:
u(1)m(t)=∑∞k=1μkem(t)ψ(t−tk),u(2)m(t)={−δmem(t)−ζ[em(t)]/[em(t)]‖em(t)‖2‖em(t)‖2,‖em(t)‖≠0,0,‖em(t)‖=0, | (4) |
where μk is the impulsive gain, and (t1,t2,t3,…) is the impulsive sequence, satisfying limk→∞tk=∞, δm is the control gain, ‖em(t)‖2 is the L2 norm of the vector em(t), and ζ is a constant greater than 0.
We establish the impulsive control framework to integrate the concept of pinning, i.e., consider P(tk) as the set of nodes pinned at the moment of the impulse t=tk, and sort these nodes based on the magnitude of synchronization error during the impulsive instance tk. Subsequently, the top lk nodes exhibiting higher control errors are selected and included in the set of controlled nodes P(tk). In pinning control, more attention is given to the nodes with large errors, while the remainder of the nodes rely on the self-regulation of the nodes' coupling relationships. The number of pinned nodes lk is determined by the impulsive pinning control ratio ρk, defined as ρk = (∑i∈P(tk)emH(tk−)em(tk−))/(∑Nm=1emH(tk−)em(tk−)).
Remark 3. Numerous scholars have approached the synchronization issues in CVNNs by considering the real and imaginary components as real-valued systems. Although this method is straightforward and yields effective results, it causes increased computing costs. Therefore, inspired by [14,21], we investigate non-separation techniques that refrain from partitioning the system. Unlike the two referenced articles, we extend the research to delayed neural networks and improve the control method by employing impulsive control, effectively achieving network synchronization.
Remark 4. In the analysis of CVNNs, the use of the sign function often results in oscillations or shaking phenomena due to its discontinuity, which can negatively affect synchronization and stability. To address this, one approach is to replace the standard sign function with a smooth sign function like tanh or sigmoid, which reduces sharp jumps and minimizes oscillations [31]. Additionally, incorporating delay compensation mechanisms such as model predictive control (MPC) can help mitigate the impact of time delays on synchronization [32]. Adjusting the system's gain can also reduce the amplitude of oscillations, while robust control methods offer a way to handle discontinuities and ensure stability [33]. The aforementioned work has significantly contributed to our improved understanding of the tremor phenomenon observed in the running trajectory resulting from symbolic functions. This issue will also serve as a central focus for our future investigations.
In this section, according to the model and method proposed above, the conditions are provided for achieving the FTS of CVNNs under different impulsive gains.
Theorem 1. Based on Assumptions 1 and 2, if there exists δ>0 such that
(ˉdl+dl+‖c‖22λmax(BBH⊗ΓΓH)+1+l1+l21−ˆτ1+11−ˆτ2)IN+ˉcˉhlAH+chlA−2δ⩽0. | (5) |
Therefore, it can be derived that:
(ⅰ): When γ∈(0, 1), the network can reach a stable state within a finite time frame under the influence of synchronous impulses, and the stabilization time is denoted as: T1=Talnγln(ζNTaζNTa−γ−2N0v(0)lnγ).
(ⅱ): When γ=1, the network operates without impulsive effects, yet it can still attain stability within a finite time frame, with the stabilization time denoted as: T1=v(0)ζN.
(ⅲ): When γ>1, the network experiences the influence of desynchronized impulses. After meeting certain conditions, it can still reach a stable state within a finite time: T1=Talnγln(ζNTaζNTa−γ2N0v(0)lnγ),
where dland hl are, respectively, the diagonal elements of the matrices D and Γ, and ˉdl, ˉhl, and ˉcdenote their complex conjugates. δ = diag{ δ1,δ2,...,δN}, γ = 1 + μk(μk+2)ρk,
A=[a11a12⋯a1Na21a22⋯a2N⋮⋮⋱⋮aN1aN2⋯aNN];B=[b11b12⋯b1Nb21b22⋯b2N⋮⋮⋱⋮bN1bN2⋯bNN]. |
Proof. Consider the following Lyapunov function:
V(t)=V1(t)+V2(t)+V3(t).V1(t)=∑Nm=1emH(tk)em(tk),V2(t)=l21−ˆτ1∑Nm=1∫tt−τ1(t)emH(y)em(y)dy,V3(t)=11−ˆτ2∑Nm=1∫tt−τ2(t)emH(y)em(y)dy. | (6) |
For t∈[tk−1,tk),k∈N, take the derivative and substitute it into the error system (3),
˙V(t)=˙V1(t)+˙V2(t)+˙V3(t). | (7) |
By incorporating the impulsive control strategy (4), we have
˙V1(t)=∑Nm=1em(t)H(DH+D)em(t) + ∑Nm=1(fH(em(t),em(t−τ1(t)))em(t)+emH(t)f(em(t),em(t−τ1(t)))) + ∑Nm=1∑Nj=1((camjΓej(t))Hem(t)+emH(t)camjΓej(t)) + ∑Nm=1∑Nj=1((cbmjΓej(t−τ2(t)))Hem(t)+emH(t)cbmjΓej(t−τ2(t))) + ∑Nm=1((u(2)m(t))Hem(t)+emH(t)u(2)m(t)). | (8) |
According to Assumption 2 and xHy+yHx⩽xHx+yHy[26], one obtains
∑Nm=1(fH(em(t),em(t−τ1(t)))em(t)+emH(t)f(em(t),em(t−τ1(t))))⩽∑Nm=1emH(t)em(t) + l1∑Nm=1emH(t)em(t)+l2∑Nm=1emH(t−τ1(t))em(t−τ1(t))=∑nl=1el(t)H(1+l1)INel(t)+∑nl=1el(t−τ1(t))Hl2INel(t−τ1(t)). | (9) |
Applying the same approach along with the operational rules of the Kronecker product, we can derive
∑Nm=1∑Nj=1((cbmjΓej(t−τ2(t)))Hem(t)+emH(t)cbmjΓej(t−τ2(t))) = ∑Nm=1∑Nj=1emH(t)(cB⊗Γ)ej(t−τ2(t)) + ∑Nm=1∑Nj=1em(t)(cB⊗Γ)HejH(t−τ2(t))⩽‖c‖22λmax(BBH⊗ΓΓH)∑Nm=1∑Nj=1emH(t)ej(t) + emH(t−τ2(t))ej(t−τ2(t)) = ∑nl=1elH(t)(‖c‖22λmax(BBH⊗ΓΓH))INel(t)+∑nl=1el(t−τ2(t))HINel(t−τ2(t)). | (10) |
According to the devised control strategy and Lemma 2, the equation can be further transformed into
∑Nm=1((u(2)m(t))Hem(t)+emH(t)u(2)m(t))=−2δm∑Nm=1emH(t)em(t)−ζ∑Nm=1(emH(t)[em(t)]+[em(t)]Tem(t))‖em(t)‖2⩽−2δm∑Nm=1emH(t)em(t)−ζN=−2∑nl=1el(t)Hδel(t)−ζN. | (11) |
Substituting Eqs (9)–(11) into Eq (8), one has
˙V1(t)⩽∑nl=1el(t)H(ˉdl+dl)INel(t)+∑nl=1el(t)H(1+l1)INel(t)−ζN−2∑nl=1el(t)Hδel(t)+∑nl=1elH(t)(ˉcˉhlAH+chlA)el(t)+∑nl=1elH(t)(‖c‖22λmax(BBH⊗ΓΓH))INel(t)+∑nl=1el(t−τ2(t))HINel(t−τ2(t))+∑nl=1el(t−τ1(t))Hl2INel(t−τ1(t)). | (12) |
Under Assumption 1, simplifying ˙V2(t) and ˙V3(t) is also straightforward, i.e.,
˙V2(t)⩽l21−ˆτ1∑Nm=1emH(t)em(t)−l2∑Nm=1emH(t−τ1(t))em(t−τ1(t))=∑nl=1el(t)Hl21−ˆτ1INel(t)−∑nl=1el(t−τ1(t))Hl2INel(t−τ1(t)),˙V3(t)⩽11−ˆτ2∑Nm=1emH(t)em(t)−∑Nm=1emH(t−τ2(t))em(t−τ2(t))=∑nl=1el(t)H11−ˆτ2INel(t)−∑nl=1el(t−τ2(t))HINel(t−τ2(t)). | (13) |
Substituting ˙V1(t), ˙V2(t) and ˙V3(t) into ˙V(t), in conjunction with Theorem 1, yields
˙V(t)⩽∑nl=1el(t)H(ˉdl+dl)INel(t)+∑nl=1el(t)H(1+l1)INel(t)+∑nl=1elH(t)(ˉcˉhlAH+chlA)el(t)+∑nl=1elH(t)(‖c‖22λmax(BBH⊗ΓΓH))INel(t)+∑nl=1el(t−τ1(t))HINel(t−τ1(t))−2∑nl=1el(t)Hδel(t)−ζN+∑nl=1el(t)H11−ˆτ2INel(t)+∑nl=1el(t−τ2(t))Hl2INel(t−τ2(t))+∑nl=1el(t)Hl21−ˆτ1INel(t)−∑nl=1el(t−τ2(t))Hl2INel(t−τ2(t))−∑nl=1el(t−τ1(t))HINel(t−τ1(t))=∑nl=1el(t)H((ˉdl+dl+1+l1+l21−ˆτ1+11−ˆτ2+‖c‖22λmax(BBH⊗ΓΓH))IN+ˉcˉhlAH+chlA−2δ)el(t)−ζN⩽−ζN, | (14) |
where el(t) = (e1lH(t),e2lH(t),⋯,eNlH(t))H.
When t=tk(k=1,2,⋯), one obtains V(tk+)=V1(tk+)+V2(tk+)+V3(tk+).
Hence, according to the pinning rule in Section 2.3, it can be inferred that
V1(tk+) = ∑Nm=1emH(tk+)em(tk+)=∑m∈P(tk+)emH(tk+)em(tk+)+∑m∉P(tk+)emH(tk+)em(tk+) = ∑m∈P(tk−)(1 + μk)2emH(tk−)em(tk−)+∑m∉P(tk−)emH(tk−)em(tk−) = (1 + μk)2ρk∑Nm=1emH(tk−)em(tk−)+(1−ρk)∑Nm=1emH(tk−)em(tk−)=(1 + μk(μk+2)ρk)V1(tk−). | (15) |
In the same way, one has
V2(tk+) = l21−ˆτ1∑Nm=1∫tk+tk+−τ1(t)emH(y)em(y)dy=(1 + μk(μk+2)ρk)V2(tk−),V3(tk+) = 11−ˆτ2∑Nm=1∫tk+tk+−τ2(t)emH(y)em(y)dy=(1 + μk(μk+2)ρk)V3(tk−). | (16) |
Hence,
V(tk+)=V1(tk+)+V2(tk+)+V3(tk+) = (1 + μk(μk+2)ρk)V(tk−) = γV(tk−). | (17) |
Next, From Eqs (14) and (17), it can be seen ˙V(t)⩽−ζN, and V(tk+)=γV(tk−) then design the comparison system as:
{˙V(t)⩽˙v(t)=−ζN,t≠tk,tk>0,v(tk)=γv(tk−),k∈N,v(0)=V(0). | (18) |
According to the comparison theorem, it can be found that V(t)⩽v(t), where v(t) can be obtained from the formula of parameter variation, which has been rigorously demonstrated in this article [34]
v(t)⩽W(t,0)v(t)−ζN∫t0W(t,ϑ)dϑ, | (19) |
where W(t,ϑ), 0⩽ϑ⩽t satisfies
W(t,ϑ) = ∏s⩽tr<tγ=γN(t,ϑ). | (20) |
Substitute Eq (20) into Eq (19) to obtain
v(t) = γN(t,0)v(0)−ζN∫t0γN(t,ϑ)dϑ. | (21) |
According to γ = 1 + μk(μk+2)ρk, as can be seen from the formula, this variable is affected by two parts, namely μk and ρk. The μk is the intensity of the impulsive control that determines the impact of the impulse on the system, and ρk is the pinning control ratio that determines the number of nodes. In view of its impact on the network, from the numerical value, we divided it into three parts for theoretical discussion and analysis. Going deep into its components, we discuss and analyze the pulse intensity and the number of holding nodes in the numerical simulation section.
(Ⅰ) When γ∈(0, 1), or equivalently −1<μk<0, the CVNNs are affected by the synchronous impulses. One has:
v(t)⩽γ(tTa−N0)v(0)−ζN∫t0γ(t−ϑTa+N0)dϑ=(γ−N0v(0)−ζNγN0Talnγ)exp(tTalnγ)+ζNγN0Talnγ. | (22) |
When setting v(t)→0, the stabilization time is denoted as: T1=Talnγln(ζNTaζNTa−γ−2N0v(0)lnγ).
(Ⅱ) When γ=1, or μk=0, the CVNNs experience no impulsive effects. Hence, its stabilization time remains independent of the impulsive gain and pinning ratio, with the stabilization time denoted as T1=v(0)ζN.
(Ⅲ) When γ>1, and 0<μk<1, there exist desynchronized impulses. In this case, it is provided that when Ta>γ2N0v(0)lnγ/(ζN), the CVNNs can attain a stable state within the stabilization time T1=Talnγln(ζNTaζNTa−γ2N0v(0)lnγ).
In this section, multiple numerical simulations employing the impulsive pinning strategy are conducted. Initially, the potential for achieving synchronization through this approach is assessed. Building on the findings from the initial analysis, various parameters, including impulsive gain and the number of pinned nodes, are examined in detail. Furthermore, an analysis of the optimization of synchronization performance within networks influenced by impulsive and pinning effects is presented. Ultimately, a chaotic encryption mechanism is developed to validate the applicability of the proposed method.
An NN consisting of twelve complex-valued two-neuron systems [35] is considered the network (1) to be controlled. With the same complex-valued two-neuron, the leader system (2) is formed. Then in the network (1) xm(t)=(xm1(t),xm2(t))H,m=1,2,...,12 and in the system (2) s(t)=(s1(t),s2(t))H. The other parameters of the controlled network are as follows: D=−(1+i)I2, c=1+i, Γ=(1−i)I2, and B = A/10. The node coupling matrix of the controlled network is written as
A=[−2−i1+i00000000011+i−5−i1−i01+i100000101−i−21+i00000000001+i−3−i0010100001+i00−3−i001000101000−3−i1+i10000000101+i−3−2i001+i000000110−3−i001+i000010000−2+i1−i000000001+i01−i−20000000001+i00−2−2i1+i11001000001+i−4−i]. |
The nonlinear function of the controlled and leader system is defined as
f(m,n)=Mg(m)+Ng(n)=M(tanh(Re(m1))+isin(Im(m1)),tanh(Re(m2))+isin(Im(m2)))H+N(tanh(Re(n1))+isin(Im(n1)),tanh(Re(n2))+isin(Im(n2)))H,M=N=[1.8−3.6i−0.6+1.5i0.85−0.1i1.5−2i]. |
Furthermore, consider the following settings for time delays: the internal delay within each neuron, denoted as τ1(t)=0.02, and the inter-neuronal coupling delay, denoted as τ2(t)=0.1+0.05sint. It is noteworthy that the time delays are bounded, i.e., let ˆτ1=0.5,ˆτ2=0.5, and Assumption 1 is considered reasonable.
After calculation, the nonlinear function f(m,n) is consistent with that of Assumption 2. Subsequently, the control parameters have been set as δm = 60 to ensure CVNN stability, and ζ = 0.01 to satisfy Theorem 1. To select the pinned nodes, the errors across all nodes have been reorganized to yield the sequence: ‖em1(tk)‖⩾‖em2(tk)‖⩾...⩾‖emN(tk)‖ at each impulsive time tk. The impulsive pinning control ratio ρk is selected with the goal of controlling only the eight nodes with larger errors at each impulsive moment.
To ascertain the evolution of the network (1) with different impulsive gains, the simulations are carried out in terms of both synchronous and desynchronized impulses.
When synchronous impulses are imposed on the network, the impulsive gain μk=−0.5, N0 = 3, and the average impulsive interval Ta = 0.01 are opted for simulation purposes. According to Theorem 1, the error curves are depicted in Figure 1.
From Figure 1(a), (b), both the real and imaginary parts exhibit a noticeable reduction in the synchronization error under the influence of synchronous impulses every 0.01 s. After four impulsive interventions, the synchronization errors gradually converge, stabilizing around t = 0.05 s.
When desynchronized impulses are imposed on the network, the impulsive gain μk=0.5, N0 = 3, and the average impulsive interval Ta = 0.02 are opted for simulation purposes. Then, the error curves can be obtained, as depicted in Figure 2.
Figure 2 shows that the network (1) can achieve the FTS under desynchronized impulses. Synchronously, the network can achieve stability in both the real and imaginary parts after approximately 5 impulsive applications, with a stabilization time of approximately 0.15 s. Unlike the effects of synchronous impulses, the error at the desynchronized impulsive moment initially exhibits an upward jump followed by a significant decay. It is a hindering effect that makes the network need a longer time to attain synchronization under the influence of desynchronized impulses. The conclusion is similar to previous research findings [26]. The simulation analysis demonstrates that under the proposed impulsive pinning strategy, controlling the eight nodes with large errors enables the CVNNs to attain the FTS in both synchronous and desynchronized impulsive scenarios.
Both pinning and impulsive selection are essential mechanisms that influence the stability and convergence of dynamic systems. Pinning control directs the system towards a predetermined target state, thereby ensuring stable convergence. In contrast, impulsive control enhances system responsiveness through intermittent adjustments, facilitating rapid adaptation. While pinning control effectively maintains stability, excessive reliance on it may diminish the system's flexibility; impulsive control addresses this drawback by enabling the system to better accommodate sudden changes. Furthermore, impulsive interventions can rectify deviations when pinning control is insufficient, while pinning control serves to mitigate oscillations that may arise from impulsive actions. Together, these two approaches contribute to achieving both stability and adaptability in dynamic environments. By considering the effects of pinning and impulsive selection, it becomes possible to design systems that are more robust, adaptive, and efficient, thus enhancing their performance across a variety of real-world scenarios.
Here, varying impulsive gains from synchronous impulses to desynchronized ones are applied with intervals of 0.02 s. The observed metric represents the norm summation of errors across nodes (differentiated into real and imaginary parts). The heatmap below illustrates the real and imaginary stable values under varying impulsive gains.
Figure 3(a), (b) exhibit a consistent trend, i.e., as the impulsive gain transitions from the synchronous state to the desynchronized state, there is a continuous increase in the network's error at identical time points. This change is particularly noticeable between 0.02 s and 0.04 s. This robustly confirms the viewpoint that synchronous impulses can facilitate the FTS, whereas desynchronized impulses have the opposite effects.
Furthermore, according to Theorem 1, the network's convergence is associated with γ, where γ is correlated with ρk. This can be proved by the value of ρk, as the impulsive pinning control gain designed in this paper can be transformed into the number of control nodes, given by round (N⋅ρk). The number of control nodes directly affects the network's convergence. In other words, the value of γ indirectly influences the network's dynamical properties, which is referred to as the pinning effects. To further observe the influence of the pinning effects on convergence, the resultant approximate time to reach the stable state when controlling the number of different nodes is shown in the blue part of Figure 4, while the change of control time after adding one control node is shown in the orange part.
The left plot of Figure 4 depicts the stability time variation concerning the alteration in the number pinned nodes under synchronous impulsive conditions. Notably, the stability time decreases as the number of pinned nodes increases when the system applies with positive impulses. As the pinned nodes approach the total nodes, the time variation becomes level-off. Consequently, determining an optimal number of pinned nodes is critical to further investigations.
Different from the left plot, under the desynchronized impulsive condition, a growing trend in the stability time is observed with the increase in the number of pinned nodes. This difference is due to the counteractive effects of desynchronized impulses on the network stability, unlike the promoting effects of synchronous impulses. Thus, due to the presence of nodes affected by desynchronized impulses, the network necessitates more time to attain stability.
Finally, the error magnitude at a fixed time point as a metric is used to investigate the impact of synchronous impulsive gains and the number of pinned nodes on the stability. The synchronous analysis results are exhibited in Figure 5.
When considering synchronous impulses, the analytical results are depicted in Figure 5. From Figure 5(a), (b), when the absolute value of impulsive gain is higher, particularly within the range of (–1, –0.7), the network stability is contingent upon the number of pinned nodes. As the number of pinned nodes increases, the CVNNs converge to stability more rapidly.
When the pinned nodes surpass half of the total nodes, their impact on the stability tends to reach a saturation point. In other words, for impulsive gains within a range of (–1, –0.7), the optimal number of pinned nodes approximates half of the total nodes. With the increasing impulsive gain, however, the number of pinned nodes no longer remains the sole determining factor for stability. The relationship between the number of pinned nodes and impulsive gains no longer adheres to a proportional association with stability. For instance, at an impulsive gain of –0.6 with 12 restrained nodes, the stability is notably compromised, whereas around an impulsive gain of –0.4 with 6 restrained nodes, the stability is noticeably improved. Consequently, when the absolute value of synchronous impulsive gains is lower, simply increasing the pinned node number may not guarantee expedited stabilization. Instead, a specific analysis is required to ascertain an optimal choice.
In this section, leveraging the outcomes derived from Theorem 1, we design a chaotic encryption mechanism to demonstrate the practicality of this study in secure image communication. The key details are outlined as follows.
(Ⅰ) Handling color images requiring encryption and decryption involves extracting its pixel matrix, denoted as . Here, represents the rows in the matrix , and represents the columns in the matrix , . For each value of a new matrix is generated, with three matrices representing the pixel matrices for R, G, and B, respectively.
(Ⅱ) Using the synchronized state of a specific node within Eq (1) (which possesses 2 dimensions) generates a chaotic sequence, resulting in the signals , , , . The matrices , are obtained by taking elements in a row-major order ().
(Ⅲ) Image encryption: let denote the pixel matrix of the encrypted image. The encryption rule, denoted as , is derived according to the original pixel matrix and chaotic signals and . Here, represents the arithmetic mean, denotes modulo operation, stands for rounding operation, and indicates the Euclidean norm operation.
(Ⅳ) Image decryption involves the inverse operations of encryption, utilizing synchronized sequences to obtain signals , , , and . Similarly, consider the matrices and , and let represent the pixel matrix of the decrypted image. The decryption rule is denoted as , where signifies the subtraction operation.
(Ⅴ) According to the aforementioned algorithm, the simulated results of the encrypted and decrypted color images, along with their respective histograms, are illustrated in Figures 6 and 7. It can be seen that the algorithm can effectively implement color image encryption and decryption and has high security.
As mentioned, the choice of the number of pinned nodes and the impulsive gains is crucial for achieving effective synchronization performance. Variations in these selections directly influence the complexity of both the encryption and decryption processes. Thus, modifying the control parameters during decryption can be viewed as a strategy for simplifying the key. The issue of key optimization will be explored in greater detail in future research.
Remark 5. The adoption of complex numbers and matrix-based weights in neural networks has the potential to improve their representational abilities. However, this transition also presents several challenges, including increased computational complexity, difficulties in optimization, and diminished interpretability. In domains such as signal processing and communications, where the use of complex-valued data is intrinsic, these modifications can prove to be particularly advantageous. Consequently, the decision to implement such changes should be guided by the specific requirements of the problem at hand and the resources that are available.
We investigate the finite-time synchronization issue associated with complex-valued mixed-delay neural networks. By utilizing the comparison theorem and applying the the Lyapunov function theory, we calculate the time required for networks with mixed delays to achieve stability under both synchronous and desynchronized impulses. To validate the theoretical analysis, several numerical examples and an application in image encryption are provided. In addressing complex-valued problems, we consider non-separable methods as effective approaches. The impulsive pinning strategy proposed here selectively targets nodes exhibiting higher error rates to enhance FTS. The analysis indicates that suitable impulsive gains can promote synchronization, while inappropriate gains may obstruct it. Specifically, when synchronized impulses are applied to the network, an increase in the number of pinned nodes correlates with a reduced time frame to attain stability, whereas desynchronized impulses yield the opposite effect. Last, we analyze the effects of varying impulsive gains and the number of pinned nodes on the overall stability of the network.
The controller described in this paper will cause the tremor phenomenon of the running trajectory due to the use of symbol function, which will affect the synchronous selection, and the effective strategy to solve this problem needs to be studied. In addition, the number of pinned nodes and the change in impulsive gain selection directly affect the complexity of the encryption and decryption process, and we will explore the strategy of simplifying the key in more detail in future research.
The authors declare they have not used Artificial Intelligence (AI) tools in the creation of this article.
This work is supported by the National Natural Science Foundation of China (Nos. 11602146 and 11872304), the Science Foundation of Shanghai (No. 18ZR1438200), and the Youth and Middle-aged Science and Technology Development Program of Shanghai Institute of Technology (No. ZQ2024-10).
The authors declare there is no conflict of interest.
[1] |
X. Tan, C. Xiang, J. Cao, Synchronization of neural networks via periodic self-triggered impulsive control and its application in image encryption, IEEE Trans. Cybern., 52 (2021), 8246–8257. 10.1109/TCYB.2021.3049858 doi: 10.1109/TCYB.2021.3049858
![]() |
[2] |
W. Zhao, K. Li, Y. Shi, Exponential synchronization of neural networks with mixed delays under impulsive control, Electron. Res. Arch., 32 (2024), 5287–5305. https://doi.org/10.3934/era.2024244 doi: 10.3934/era.2024244
![]() |
[3] |
M. Fang, J. Liu, W. Wang, Finite-/fixed-time synchronization of leakage and discrete delayed Hopfield neural networks with diffusion effects, Electron. Res. Arch., 31 (2023), 4088–4101. https://doi.org/10.3934/era.2023208 doi: 10.3934/era.2023208
![]() |
[4] |
J. Cao, L. Wang, Periodic oscillatory solution of bidirectional associative memory networks with delays, Phys. Rev. E, 61 (2000), 1825–1828. https://doi.org/10.1103/PhysRevE.61.1825 doi: 10.1103/PhysRevE.61.1825
![]() |
[5] |
Q. Gan, R. Xu, Synchronization of unknown chaotic delayed competitive neural networks with different time scales based on adaptive control and parameter identification, Nonlinear Dyn., 67 (2012), 1893–1902. https://doi.org/10.1007/s11071-011-0116-1 doi: 10.1007/s11071-011-0116-1
![]() |
[6] |
P. Gao, R. Ye, H. Zhang, I. Stamova, Asymptotic stability and quantitative synchronization of fractional competitive neural networks with multiple restrictions, Math. Comput. Simul., 217 (2024), 338–353. https://doi.org/10.1016/j.matcom.2023.11.005 doi: 10.1016/j.matcom.2023.11.005
![]() |
[7] |
L. Wang, Finite-/fixed-time synchronization of memristor chaotic systems and image encryption application, IEEE Trans. Circuits Syst. I, 68 (2021), 4957–4969. https://doi.org/10.1109/TCSI.2021.3121555 doi: 10.1109/TCSI.2021.3121555
![]() |
[8] |
Y. Yao, Y. Kang, Y. Zhao, P. Li, J. Tan, A novel prescribed-time control approach of state-constrained high-order nonlinear systems, IEEE Trans. Syst. Man Cybern., 54 (2024), 2941–2951. https://doi.org/10.1109/TSMC.2024.3352905 doi: 10.1109/TSMC.2024.3352905
![]() |
[9] |
Y. Yao, Y. Kang, Y. Zhao, P. Li, J. Tan, Prescribed-time output feedback control for cyber-physical systems under output constraints and malicious attacks, IEEE Trans. Cybern., 54 (2024), 6518–6530. https://doi.org/10.1109/TCYB.2024.3418384 doi: 10.1109/TCYB.2024.3418384
![]() |
[10] |
Y. D. Qu, R. Q. Zhang, S. Q. Shen, J. Yu, M. Li, Entanglement detection with complex-valued neural networks, Int. J. Theor. Phys., 62 (2023), 206. https://doi.org/10.1007/s10773-023-05460-3 doi: 10.1007/s10773-023-05460-3
![]() |
[11] |
T. Nitta, Solving the XOR problem and the detection of symmetry using a single complex-valued neuron, Neural Networks, 16 (2003), 1101–1105. https://doi.org/10.1016/S0893-6080(03)00168-0 doi: 10.1016/S0893-6080(03)00168-0
![]() |
[12] |
P. R. Chang, Complex-valued neural network for direction of arrival estimation, Electron. Lett., 30 (1994), 574–575. https://doi.org/10.1049/el:19940400 doi: 10.1049/el:19940400
![]() |
[13] | A. Hirose, Application fields and fundamental merits of complex-valued neural networks, in Complex-Valued Neural Networks: Advances and Applications, Springer, New York, (2013), 1–31. https://doi.org/10.1002/9781118590072.ch1 |
[14] |
K. Xiong, J. Yu, C. Hu, H. Jiang, Synchronization in finite/fixed time of fully complex-valued dynamical networks via nonseparation approach, J. Franklin Inst., 357 (2020), 473–493. https://doi.org/10.1016/j.jfranklin.2019.11.072 doi: 10.1016/j.jfranklin.2019.11.072
![]() |
[15] |
Y. Sheng, H. Gong, Z. Zeng, Global synchronization of complex-valued neural networks with unbounded time-varying delays, Neural Networks, 162 (2023), 309–317. https://doi.org/10.1016/j.neunet.2023.02.041 doi: 10.1016/j.neunet.2023.02.041
![]() |
[16] |
P. Mayavel, The split step theta balanced numerical approximations of stochastic time varying Hopfield neural networks with distributed delays, Results Control Optim., 13 (2023), 100329. https://doi.org/10.1016/j.rico.2023.100329 doi: 10.1016/j.rico.2023.100329
![]() |
[17] |
Q. Li, H. Wei, D. Hua, J. Wang, J. Yang, Stabilization of semi-Markovian jumping uncertain complex-valued networks with time-varying delay, Neural Process. Lett., 56 (2024), 111. https://doi.org/10.1007/s11063-024-11585-1 doi: 10.1007/s11063-024-11585-1
![]() |
[18] |
P. L. K., T. Senthilkumar, Synchronization results for uncertain complex-valued neural networks under delay-dependent flexible impulsive control, Chaos Solitons Fractals, 178 (2024), 114338. https://doi.org/10.1016/j.chaos.2023.114338 doi: 10.1016/j.chaos.2023.114338
![]() |
[19] |
B. Zhen, Y. L. Li, L. J. Pei, L. J. Ouyang, The approximate lag and anticipating synchronization between two unidirectionally coupled Hindmarsh-Rose neurons with uncertain parameters, Electron. Res. Arch., 32 (2024), 5557–5576. https://doi.org/10.3934/era.2024257 doi: 10.3934/era.2024257
![]() |
[20] |
Y. Wang, X. Li, S. Song, Input-to-state stabilization of nonlinear impulsive delayed systems: An observer-based control approach, IEEE/CAA J. Autom. Sin., 9 (2022), 1273–1283. https://doi.org/10.1109/JAS.2022.105422 doi: 10.1109/JAS.2022.105422
![]() |
[21] |
H. Zhu, J. Lu, X. Li, X. Chen, Stability of nonlinear impulsive systems with non-uniformly distributed packet loss, J Franklin Inst., 360 (2023), 13244–13260. https://doi.org/10.1016/j.jfranklin.2023.09.053 doi: 10.1016/j.jfranklin.2023.09.053
![]() |
[22] |
H. Fan, K. Shi, H. Wen, Y. Zhao, Synchronization of multi-weighted complex networks with mixed variable delays and uncertainties via impulsive pinning control, Phys. D, 456 (2023), 133935. https://doi.org/10.1016/j.physd.2023.133935 doi: 10.1016/j.physd.2023.133935
![]() |
[23] |
Z. Liu, M. Luo, Synchronization control of partial-state-based neural networks: Event-triggered impulsive control with distributed actuation delay, Phys. A Stat. Mech. Appl., 251 (2024), 12400. https://doi.org/10.1016/j.eswa.2024.124000 doi: 10.1016/j.eswa.2024.124000
![]() |
[24] |
Y. Li, J. Zhang, J. Lu, J. Lou, Finite-time synchronization of complex networks with partial communication channels failure, Inf. Sci., 634 (2023), 539–549. https://doi.org/10.1016/j.ins.2023.03.077 doi: 10.1016/j.ins.2023.03.077
![]() |
[25] |
S. Liu, T. Xu, Q. Wang, Y. Yang, The impulsive synchronization of multiplex networks with mixed delays and dual uncertainties, Math. Comput. Simul., 219 (2024), 141–163. https://doi.org/10.1016/j.matcom.2023.12.013 doi: 10.1016/j.matcom.2023.12.013
![]() |
[26] |
J. Lu, D. W. C. Ho, A unified synchronization criterion for impulsive dynamical networks, Automatica, 46 (2010), 1215–1221. https://doi.org/10.1016/j.automatica.2010.04.005 doi: 10.1016/j.automatica.2010.04.005
![]() |
[27] |
L. Feng, J. Yu, C. Hu, C. Yang, Nonseparation method-based finite/fixed-time synchronization of fully complex-valued discontinuous neural networks, IEEE Trans. Cybern., 51 (2021), 3212–3223. https://doi.org/10.1109/TCYB.2020.2980684 doi: 10.1109/TCYB.2020.2980684
![]() |
[28] |
Z. Zhang, J. Cao, Finite-time synchronization for fuzzy inertial neural networks by maximum value approach, IEEE Trans. Fuzzy Syst., 30 (2022), 1436–1446. https://doi.org/10.1109/TFUZZ.2021.3059953 doi: 10.1109/TFUZZ.2021.3059953
![]() |
[29] |
Z. Zhang, J. Cao, Novel finite-time synchronization criteria for inertial neural networks with time delays via integral inequality method, IEEE Trans. Neural Networks Learn. Syst., 30 (2019), 1476–1485. https://doi.org/10.1109/TNNLS.2018.2868800 doi: 10.1109/TNNLS.2018.2868800
![]() |
[30] |
J. Jian, L. Duan, Finite-time synchronization for fuzzy neutral-type inertial neural networks with time-varying coefficients and proportional delays, Fuzzy Sets Syst., 381 (2020), 51–67. https://doi.org/10.1016/j.fss.2019.04.004 doi: 10.1016/j.fss.2019.04.004
![]() |
[31] |
E. Lee, J. Lee, Y. Kim, Minimax approximation of sign function by composite polynomial for homomorphic comparison, IEEE Trans. Dependable Secure Comput., 19 (2022), 3711–3727. https://doi.org/10.1109/TDSC.2021.3105111 doi: 10.1109/TDSC.2021.3105111
![]() |
[32] |
L. Qiu, L. Dai, U. Ahsan, C. Fang, Model predictive control for networked multiple linear motors system under dos attack and time delay, IEEE Trans. Ind. Inf., 19 (2023), 790–799. https://doi.org/10.1109/TII.2021.3139127 doi: 10.1109/TII.2021.3139127
![]() |
[33] |
H. Zhang, X. Wang, C. Zhang, P. Yan, Robust synchronization for discrete-time coupled markovian jumping neural networks with mixed time-delays, IEEE Access, 8 (2020), 16099–16110. https://doi.org/10.1109/ACCESS.2020.2966525 doi: 10.1109/ACCESS.2020.2966525
![]() |
[34] | G. Feng, J. Cao, Stability analysis of nonlinear time-delay system with delayed impulsive effects, in 2014 International Joint Conference on Neural Networks (IJCNN), (2014), 307–312. https://doi.org/10.1109/IJCNN.2014.6889393 |
[35] |
A. Abdurahman, M. Abudusaimaiti, H. Jiang, Fixed/predefined-time lag synchronization of complex-valued BAM neural networks with stochastic perturbations, Appl. Math. Comput., 444 (2023), 127811. https://doi.org/10.1016/j.amc.2022.127811 doi: 10.1016/j.amc.2022.127811
![]() |