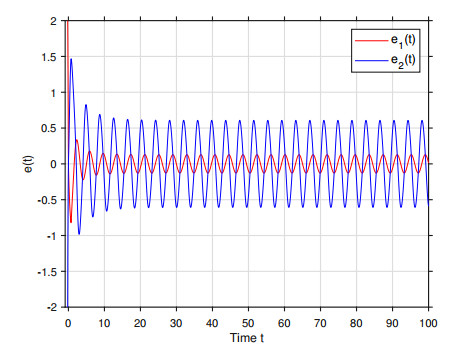
In this paper, the exponential synchronization problem of a class of neural networks with mixed delays under impulsive control is studied. Combining the impulsive comparison principle and the concept of an average impulsive interval, two impulsive differential inequalities with mixed delays are discussed, and the sufficient conditions for the existence of exponential decay are obtained. Based on two different impulsive control strategies, and then by means of the Lyapunov function, the inequality technique, and these two new inequalities, a set of sufficient conditions are derived to ensure the synchronization of the drive and response systems. In order to prove the effectiveness of the proposed control scheme, two numerical examples are given to prove its practicability and effectiveness.
Citation: Wanshun Zhao, Kelin Li, Yanchao Shi. Exponential synchronization of neural networks with mixed delays under impulsive control[J]. Electronic Research Archive, 2024, 32(9): 5287-5305. doi: 10.3934/era.2024244
[1] | Shuang Liu, Tianwei Xu, Qingyun Wang . Effect analysis of pinning and impulsive selection for finite-time synchronization of delayed complex-valued neural networks. Electronic Research Archive, 2025, 33(3): 1792-1811. doi: 10.3934/era.2025081 |
[2] | Yilin Li, Jianwen Feng, Jingyi Wang . Mean square synchronization for stochastic delayed neural networks via pinning impulsive control. Electronic Research Archive, 2022, 30(9): 3172-3192. doi: 10.3934/era.2022161 |
[3] | Chengbo Yi, Jiayi Cai, Rui Guo . Synchronization of a class of nonlinear multiple neural networks with delays via a dynamic event-triggered impulsive control strategy. Electronic Research Archive, 2024, 32(7): 4581-4603. doi: 10.3934/era.2024208 |
[4] | Jun Guo, Yanchao Shi, Shengye Wang . Synchronization analysis of delayed quaternion-valued memristor-based neural networks by a direct analytical approach. Electronic Research Archive, 2024, 32(5): 3377-3395. doi: 10.3934/era.2024156 |
[5] | Jun Guo, Yanchao Shi, Weihua Luo, Yanzhao Cheng, Shengye Wang . Exponential projective synchronization analysis for quaternion-valued memristor-based neural networks with time delays. Electronic Research Archive, 2023, 31(9): 5609-5631. doi: 10.3934/era.2023285 |
[6] | Tianyi Li, Xiaofeng Xu, Ming Liu . Fixed-time synchronization of mixed-delay fuzzy cellular neural networks with $ L\acute{e}vy $ noise. Electronic Research Archive, 2025, 33(4): 2032-2060. doi: 10.3934/era.2025090 |
[7] | Minglei Fang, Jinzhi Liu, Wei Wang . Finite-/fixed-time synchronization of leakage and discrete delayed Hopfield neural networks with diffusion effects. Electronic Research Archive, 2023, 31(7): 4088-4101. doi: 10.3934/era.2023208 |
[8] | Yawei Liu, Guangyin Cui, Chen Gao . Event-triggered synchronization control for neural networks against DoS attacks. Electronic Research Archive, 2025, 33(1): 121-141. doi: 10.3934/era.2025007 |
[9] | Yong Zhao, Shanshan Ren . Synchronization for a class of complex-valued memristor-based competitive neural networks(CMCNNs) with different time scales. Electronic Research Archive, 2021, 29(5): 3323-3340. doi: 10.3934/era.2021041 |
[10] | Xiangwen Yin . A review of dynamics analysis of neural networks and applications in creation psychology. Electronic Research Archive, 2023, 31(5): 2595-2625. doi: 10.3934/era.2023132 |
In this paper, the exponential synchronization problem of a class of neural networks with mixed delays under impulsive control is studied. Combining the impulsive comparison principle and the concept of an average impulsive interval, two impulsive differential inequalities with mixed delays are discussed, and the sufficient conditions for the existence of exponential decay are obtained. Based on two different impulsive control strategies, and then by means of the Lyapunov function, the inequality technique, and these two new inequalities, a set of sufficient conditions are derived to ensure the synchronization of the drive and response systems. In order to prove the effectiveness of the proposed control scheme, two numerical examples are given to prove its practicability and effectiveness.
Originating from the groundbreaking M-P model devised by McCulloch and Pitts [1], neural networks (NNs) have evolved into powerful machine learning algorithms [2]. Their ubiquitous application across diverse fields stems from their remarkable aptitude for learning and discerning patterns within data. Whether in associative memory tasks that need to process a large amount of information[3], or in automatic control systems that require precise control, NNs have shown their unique advantages. Concurrently, NNs occupy an indispensable position in realms such as pattern recognition, signal processing[4,5,6], secure communication, and tackling intricate optimization challenges [7,8]. Because of its characteristics, it has attracted the attention of scholars.
In the specific implementation, a time delay is widespread and inevitable, which is due to the limited transmission of information between systems[9,10]. On the other hand, NNs usually have spatial characteristics because numerous parallel pathways exist. The dissimilar axis-cylinder size and length, as well as the propagation velocity distribution along these pathways, brings about a propagation distribution delay[11,12]. Numerous scholars engaged in profound discourses, thereby embracing the perspective that the presence of a time delay inherently influenced the dynamic behavior exhibited by NNs, such as oscillation, divergence, or instability, and other complex dynamic behaviors [13,14]. The above phenomena is harmful to NNs and practical applications. Therefore, it is a useful theoretical research and practical application to introduce a time delay into the modeling of neural networks.
Synchronization is a dynamic behavior of NNs, whereby two or more systems have the same behavior under coupling or external control. Synchronization is widely used in secure communication[15,16], image encryption[17,18], and image protection[19]. Many scholars studied various types of synchronizations according to various situations, see [20,21,22,23].Therefore, the study of NN synchronization has both theoretical and practical values.
Designing a suitable controller to achieve synchronization is a challenge. Based on the time interval operation, the intermittent controller automatically switches the working / resting state of the equipment, optimizes energy consumption, and reduces costs. Because of its energy saving effect and practicability, it has attracted wide attention and application, see[24,25,26,27,28]. Impulse control turns a continuous system into a discontinuous one at the impulse moment. Both pulse control and intermittent control exert infleunces at the corresponding time. However, compared with intermittent control, pulse control is a time rather than a time period, which helps reduce the cost of control. Therefore, impulsive control is widely used in synchronization problems. In [29], the exponential synchronization problem of delayed coupled NNs was studied by means of a Razumikhin-type inequality. In [30], the exponential synchronization problem of stochastic coupled NNs was studied by using the stochastic impulsive differential inequality. In [31], the quasi-synchronization of NNs was studied using the Lyapunov function and the impulsive comparison principle. In [32], the quasi-synchronization problem of directed coupled heterogeneous NNs was studied using the extended pulse comparison principle and the saturated nonlinear dead-zone function. In [33], the μ-synchronization of coupled NNs was studied by constructing a new impulsive differential inequality. In [34], the quasi-synchronization problem of the quasi-synchronization of multi-layer delayed NNs was studied using the Lyapunov function and the pulse comparison principle. In [35], the synchronization problem of nonlinear, delayed, semi-Markov, jump NNs was studied using the stochastic Lyapunov function and the Razumikhin technique. In [36], the synchronization problem of coupled delayed inertial NNs was studied using the improved pulse comparison principle. In [37], the synchronization problem of hybrid coupled NNs was studied using delay impulsive differential inequalities. For example, in the above cited literature, the main proof of [33] and [36] is to transform the original problem into an impulsive differential inequality by means of the Lyapunov function. The forms of the two are similar. [33] proved the impulsive differential inequality first, while [36] was based on the improved impulsive comparison principle. Inspired by this, we can first discuss the transformed impulsive differential inequalities. This is the source of the idea of the impulsive inequality in this paper.
The main results of this paper focus on the following aspects:
(ⅰ) Two delay impulsive differential inequalities are given; and
(ⅱ) The exponential synchronization problem of two different impulsive control strategies is considered.
The subsequent sections of this paper are organized in the following manner: Section 2 presents an overview of the model and introduces the prerequisite knowledge; Section 3 delves into the presentation of sufficient conditions to achieve exponential synchronization in impulsive neural networks that incorporate mixed delays; in Section 4, two numerical examples are provided to demonstrate the practical efficacy of the proposed approach; and finally, Section 5 concludes the paper with a summary of the key findings.
Hereof, unless otherwise elucidated, we will use the following notation. We define R as the set of real numbers, N as the set of positive integers, and Rn as the set of n-dimensional real vectors equipped with the Euclidean norm ||⋅||. Additionally, we let Rm×n represent the set of m×n real matrices. The n×n unit matrix is represented by E. When A and B are regular intersection matrices, the notationA>B (respectively, A<B) indicates that A−B is a positive definite (respectively, a negative definite). The transpose and the inverse of matrix A are denoted by AT and A−1, respectively. The maximal and minimal eigenvalues of symmetric A are λmax(A) and λmin(A). The function σ(⋅) denotes the spectral radius of a matrix. For any interval J⊂R, PC = {ψ:J→Rn that are continuous everywhere except at a finite number of points t, where ψ(t+),ψ(t−) exist and ψ(t+)=ψ(t)}. For ς(t)∈PC([−τ,0],Rn), define ||ς(t)||=√ςT(t)ς(t).
The driving system considered in this paper is a NN with mixed delays, which is defined as follows:
{dx(t)dt=−˜Dx(t)+˜Af(x(t))+˜Bf(x(t−τ(t)))+˜C∫tt−hf(x(s))ds+˜I(t),x(t)=˜ϕ(t), −ϱ<t<0, | (2.1) |
where the neuron state vector x=(x1,x2,…,xn)T and matrices ˜D>0, ˜A, ˜B, ˜C represent the diagonal matrix, connection weight matrix, delayed weight matrix, and distributively delayed connection weight matrix of the neural network, respectively. The activation function is f(⋅)=(f1(⋅),f2(⋅),…,fn(⋅))T. Time-varying delays τ(t) satisfy 0≤τ(t)≤τ. The distributed-delay is h, and ϱ=max{τ,h}. ˜ϕ(t)∈PC([−ϱ,0],Rn). with components ˜ϕi(t),i=1,2,…,n.
The response system is as follows:
{dy(t)dt=−˜Dy(t)+˜Af(y(t))+˜Bf(y(t−τ(t)))+˜C∫tt−hf(y(s))ds+˜I(t)+˜u(t),y(t)=˜φ(t), −ϱ<t<0, | (2.2) |
where ˜φ(t)=(˜φ1(t),˜φ2(t),…,˜φn(t))T, has components ˜φi(t) that belong to PC([−ϱ,0],Rn).
To achieve the article's objective, the error e(t)=y(t)−x(t) is defined.
Combining networks (2.1) and (2.2), the error system is formulated as follows:
{de(t)dt=−˜De(t)+˜Ag(e(t))+˜Bg(e(t−τ(t)))+˜C∫tt−hg(e(s))ds+˜u(t),e(t)=˜ψ(t), −ϱ<t<0, | (2.3) |
where g(e(t))=f(y(t))−f(x(t)), ˜ψ(t)=˜φ(t)−˜ϕ(t).
The impulsive control protocol has been designed in the following manner:
˜u(t)=˜K1e(t)+˜K2g(e(t))−μ∞∑k=1˜Rke(t)δ(t−tk), | (2.4) |
where the gain matrices are represented by ˜K1 and ˜K2 in this context. ˜Rk>0 is the control gain. The fixed impulsive moments tk (for k∈N) form a strictly increasing sequence that satisfy 0=t0<t1<t2<…,tk<… with the property that the sequence diverges to infinity, (i.e., limt→∞tk=+∞). The Dirac impulsive function is denoted by δ(⋅).
Remark 2.1. In [38], the controller only considered the feedback of the activation function in t and t−τ(t). In [34], the controller was composed of the linear term of the error function and the dirac impulsive function. In [35], the controller was only composed of the dirac impulsive function. The controller in this paper considers the non-delay term of the error function and the dirac impulsive function.
By combining Eqs (2.3) and (2.4), we obtain a rephrased version of the error system:
{de(t)dt=−(˜D−˜K1)e(t)+(˜A+˜K2)g(e(t))+˜Bg(e(t−τ(t)))+˜C∫tt−hg(e(s))ds, t≠tk,:=−ˆDe(t)+ˆAg(e(t))+˜Bg(e(t−τ(t)))+˜C∫tt−hg(e(s))ds, t≠tk,Δe(tk)=e(t+k)−e(t−k)=−μ˜Rke(t−k), t=tk,e(t)=˜ψ(t), −ϱ<t<0. | (2.5) |
Definition 2.1. [38] The systems (2.1) and (2.2) were deemed to be exponentially synchronized if there existed positive constants λ and M≥1, such that for every t>0, the norm of the error function ||e(t)|| between the two systems satisfied the inequality ||e(t)||≤sup−τ≤s≤0||φ(s)−ϕ(s)||Me−λt. Here, λ serves as the convergence rate (or degree), which characterizes the exponential decline in the synchronization error as time progresses.
Definition 2.2. [31] The average impulsive interval of the impulsive sequence ζ={t1,t2,…,tk,…} was equal to Ta, if there existed the positive integer N0 and the positive scalar Ta such that for all 0≤t≤T, the number of impulsive times Nζ(T,t) of the sequence ζ within the time interval (t,T) satisfied the following inequality:
T−tTa−N0≤Nζ(T,t)≤T−tTa+N0, 0≤t≤T. |
Here, Nζ(T,t) represents the count of impulsive instants which belong to the sequence ζ that strictly occur between the times t and T.
Lemma 2.1. [31] Let τ1(t) and τ2(t) be functions which satisfy 0≤τ1(t),τ2(t)≤τ for all t. Consider the function G(t,u,ˉu1):R+×R×R→R, which is nondecreasing in its third argument ˉu1 for fixed values of t and u. Additionally, let Ik(u):R→R be a function that is nondecreasing in u. If there exists a positive constant α>0 such that
{D+u(t)≤G(t,u(t),u(t−τ1(t)))+α∫tt−τ2(t)u(s)ds, t≠tk,u(tk)≤Ik(u(t−k), k∈N, | (2.6) |
{D+v(t)>G(t,v(t),v(t−τ1(t)))+α∫tt−τ2(t)v(s)ds, t≠tk,v(tk)≥Ik(v(t−k), k∈N, | (2.7) |
then u(t)≤v(t) holds for −τ≤t≤0, and under the assumption that certain properties related to the right upper derivativeD+u(t) hold, it can be shown that u(t)≤v(t) also holds for all t>0.
Lemma 2.2. [32] Given any real, symmetric, and positive definite matrix M of an appropriate dimension, a positive scalar η>0, and a vector function ω(⋅):[a,b]→Rn such that the relevant integrals are well-defined, the following inequality holds:
[∫baω(s)ds]TM[∫baω(s)ds]≤(b−a)∫baωT(s)Mω(s)ds. |
In order to study the exponential synchronization of NNs with mixed discrete delays and finite distributed delays, the following impulsive differential inequalities which involve discrete delays and finite distributed delays are first established.
Lemma 2.3. Let u(t)∈PC([−ϱ,+∞),R) with u(t)≥0; for the impulsive sequence ζ={t1,t2,…,tk,…}, consider the following differential inequalities:
{D+u(t)≤αu(t)+θ1u(t−τ(t))+θ2∫tt−hv(s)ds, t≠tk,u(tk)≤βu(t−k), k∈N,u(s)=φ(s)≥0,s∈[−ϱ,0], | (2.8) |
where α>0, θ1>0, θ2>0,0≤τ(t)≤τ, for t∈R, 0<β<1 for k∈N, ϱ=max{τ,h}. If α+lnβTa+θ1βN0+θ2hβN0<0, then ∃λ>0 with the following property:
u(t)≤β−N0sup−ϱ≤s≤0||φ(s)||2e−λ0t,t≥0, |
where λ0 satisfies λ0+α+lnβTa+θ1eλ0τβN0+eλ0h−1λ0θ2βN0=0.
Proof. The following comparison system is considered:
{˙˜u(t)=α˜u(t)+θ1˜u(t−τ(t))+θ2∫tt−h˜u(s)ds+ε, t≠tk,˜u(tk)=β˜u(t−k), k∈N,˜u(s)=φ(s)+ε, −ϱ≤s≤0. | (2.9) |
For any ε>0, ˜u(t) denotes the unique solution. According to Lemma 2.1, we have the inequality ˜u(t)≥u(t)≥0 holding for −ϱ≤s≤0. Consequently, it follows that ˜u(t)≥u(t) also holds for t≥0. Leveraging the formula for the variation of parameters, we can describe ˜u(t) as follows:
˜u(t)=W(t,0)˜u(0)+∫t0W(t,s)[θ1˜u(s−τ(s))+θ2∫tt−h˜u(r)dr+ε]ds. | (2.10) |
The function W(t,s) defined for allt,s≥0, serves as the Cauchy matrix for the subsequent linear impulsive system:
{˙˜u(t)=α˜u(t), t≠tk,˜u(tk)=β˜u(t−k), k∈N. | (2.11) |
Definition 2.2 states that it can acquire the following:
W(t,s)≤eα(t−s)Πs<tk<tβ=eα(t−s)βNζ(s,t)≤eα(t−s)βt−sTa−N0=β−N0e(α+lnβTa)(t−s). | (2.12) |
Let q=β−N0sup−ϱ≤s≤0||φ(s)||2. The result of substituting (2.12) into (2.10) is as follows:
˜u(t)≤qe(α+lnβTa)t+∫t0β−N0e(α+lnβTa)(t−s)[θ1˜u(s−τ(s))+θ2∫ss−h˜u(r)dr+ε]ds, | (2.13) |
Denote G(λ)=λ+α+lnβTa+θ1eλτβN0+eλh−1λθ2βN0. Then, we have the following:
G(0+)=α+lnβTa+θ1βN0+limλ→0+(eλh−1λθ2βN0)=α+lnβTa+θ1βN0+θ2hβN0<0. |
For any λ∈(0,+∞), it is evident that G(+∞)>0 and G′(λ)>0. As a result, the equation G(λ)=0 admits a unique positive solution, denoted by λ0, where λ0>0.
Since ε>0,λ0>0,α+lnβTa+θ1βN0+θ2hβN0<0 and 0<β<1, we have the following:
˜u(s)<q<qe−λ0s+εσ0,−ϱ≤s≤0, | (2.14) |
where ϖ=−(α+lnβTa+θ1βN0+θ2hβN0)βN0.
Next, we prove that
˜u(t)≤qe−λ0t+εϖ,t>0. | (2.15) |
There exists a t∗>0 such that, if (2.15) is false, then
˜u(t∗)>qe−λ0t∗+εϖ | (2.16) |
and
˜u(t)≤qe−λ0t+εϖ, t<t∗. | (2.17) |
Combine (2.15) with (2.17), we have the following:
˜u(t∗)≤qe(α+lnβTa)t∗+∫t∗0β−N0e(α+lnβTa)(t∗−s)θ1(qe−λ0(s−τ(s))+εϖ)ds+∫t∗0β−N0e(α+lnβTa)(t∗−s)θ2∫ss−h(qe−λ0r+εϖ)drds+ε∫t∗0β−N0e(α+lnβTa)(t∗−s)ds≤qe(α+lnβTa)t∗+∫t∗0β−N0e(α+lnβTa)(t∗−s)θ1(qe−λ0(s−τ)+εϖ)ds+∫t∗0β−N0e(α+lnβTa)(t∗−s)θ2∫ss−h(qe−λ0r+εϖ)drds+ε∫t∗0β−N0e(α+lnβTa)(t∗−s)ds≤qe(α+lnβTa)t∗+β−N0e(α+lnβTa)t∗∫t∗0e−(λ0+α+lnβTa)sqθ1eλ0τds+εϖ∫t∗0β−N0e(α+lnβTa)(t∗−s)θ1ds+β−N0e(α+lnβTa)t∗∫t∗0e−(λ0+α+lnβTa)sqθ2eλ0h−1λ0ds+εϖ∫t∗0β−N0e(α+lnβTa)(t∗−s)θ2hds+ε∫t∗0β−N0e(α+lnβTa)(t∗−s)ds≤qe(α+lnβTa)t∗+qe(α+lnβTa)t∗∫t∗0e−(λ0+α+lnβTa)s(−λ0−α−lnβTa)ds+ε(θ1+θ2h+ϖ)ϖ∫t∗0β−N0e(α+lnβTa)(t∗−s)ds≤qe(α+lnβTa)t∗+qe−λ0t∗−qe(α+lnβTa)t∗+−ε(α+lnβTa)βN0ϖβ−N0−(α+lnβTa)(1−e(α+lnβTa)t∗)≤qe−λ0t∗+εϖ, | (2.18) |
which contracts with (2.16). Therefore, (2.15) holds true.
Let ε→0; then
u(t)≤˜u(t)≤qe−λ0t=β−N0sup−ϱ≤s≤0||φ(s)||2e−λ0t, t≥0. | (2.19) |
This completes the proof.
Lemma 2.4. Let u(t)∈PC([−ϱ,+∞),R) with u(t)≥0; for the impulsive sequence ζ={t1,t2,…,tk,…}, consider the following differential inequalities:
{D+u(t)≤αu(t)+θ1u(t−τ(t))+θ2∫tt−hv(s)ds, t≠tk,u(tk)≤βu(t−k), k∈N,u(s)=φ(s)≥0,s∈[−ϱ,0], | (2.20) |
where α<0, θ1>0, θ2>0,0≤τ(t)≤τ, for t∈R, β≥1 for k∈N, ϱ=max{τ,h}. If α+lnβTa+θ1βN0+θ2hβN0<0, then ∃λ>0 with the following property:
u(t)≤βN0sup−ϱ≤s≤0||φ(s)||2e−λ0t,t≥0, |
where λ0 satisfies λ0+α+lnβTa+θ1eλ0τβN0+eλ0h−1λ0θ2βN0=0.
Proof. The proof process follows a similar approach to that of Lemma 2.4, specifically involving the Cauchy matrix as follows:
W(t,s)≤eα(t−s)Πs<tk<tβ=eα(t−s)βNζ(s,t)≤eα(t−s)βt−sTa+N0=βN0e(α+lnβTa)(t−s). | (2.21) |
This completes the proof.
Remark 2.2. In [33], our focus was on the impulsive differential inequality that incorporated multiple discrete delays. Subsequently, in [37], we delved into the delayed impulsive differential inequality characterized by finite distributed delays. Furthermore, in [39], we explored the scenario where the integral term within the impulsive inequality assumed the form of a kernel function. However, this paper presents a comprehensive study of the impulsive differential inequality that encompasses both discrete delays and finite distributed delays, with no imposed constraints on the intensity of the impulse.
The following presumptions are made throughout this paper:
(A1) For every u1,u2∈R,u1≠u2, where Fj, j=1,2,…,n are real positive constants, the activation functions fj(⋅), where j ranges from 1 to n, are bounded functions that satisfy a specific Lipschitz condition:
|fj(u1)−fj(u2)|≤Fj|u1−u2|; and |
(A2) β=σ((E−μ˜Rk)T(E−μ˜Rk)).
Within this section, we explore the attainment of exponential synchronization between systems (2.1) and (2.2) through the construction of an appropriate Lyapunov functional.
|gi(ei(t))|≤Fi(ei(t)), which indicates that gi(⋅) in system (2.5) is constrained from (A1). Thus, for any positive diagonal matrices A, the function g(e(t)) satisfies the following inequality:
gT(e(t))Ag(e(t))≤eT(t)FTAFe(t), | (3.1) |
where F=diag{F1,F2,…,Fn}.
Theorem 3.1. Assume that the assumptions (A1) and (A2) are satisfied. Then, systems (2.1) and (2.2) are exponentially synchronized if there exist real constants α>0, θ1>0, θ2>0, along with an n×n−matrix P>0, four diagonal positive definite n×n−matrix Σi>0,i=1,2,3, and an M such that the subsequent conditions are fulfilled:
Case Ⅰ: The impulsive strength is within the range 0<β<1:
(a1)
Ξ=[ξ11000FTΣ2F−θ1P000Σ3−1hM]<0, |
where ξ11=−PˆD−ˆDTP+PˆAΣ−11ˆATP+FTΣ1F+P˜BΣ−12˜BTP+P˜CΣ−13˜CTP−αP.
(b1)
FTMF−θ2P<0, and |
(c1)
α+lnβTa+θ1βN0+θ2hβN0<0. |
Case Ⅱ: The impulsive strength is within the range β≥1:
The three real constants in the theorem satisfy the relation α<0, θ1>0, θ2>0, conditions (a2) and (b2) are identical to conditions (a1) and (b1) respectively, as defined in Case Ⅰ, and
(c2)
α+lnβTa+(θ1+θ2h)βN0<0. |
Proof. We build the subsequent Lyapunov function as follows:
V(t)=eT(t)Pe(t), | (3.2) |
where P is strictly positive definite matrix.
˙V(t)=2eT(t)P˙e(t)=2eT(t)P{−ˆDe(t)+ˆAg(e(t))+˜Bg(e(t−τ(t)))+˜C∫tt−hg(e(s))ds}=−2eT(t)PˆDe(t)+2eT(t)PˆAg(e(t))+2eT(t)P˜Bg(e(t−τ(t)))+2eT(t)P˜C∫tt−hg(e(s))ds≤eT(t)(−PˆD−ˆDTP)e(t)+eT(t)PˆAΣ−11ˆATPe(t)+gT(e(t))Σ1g(e(t))+eT(t)P˜BΣ−12˜BTPe(t)+gT(e(t−τ(t)))Σ2g(e(t−τ(t)))+eT(t)P˜CΣ−13˜CTPe(t)+[∫tt−hg(e(s))ds]TΣ3∫tt−hg(e(s))ds≤eT(t)(−PˆD−ˆDTP)e(t)+eT(t)PˆAΣ−11ˆATPe(t)+eT(t)FTΣ1Fe(t)+eT(t)P˜BΣ−12˜BTPe(t)+eT(t−τ(t))FTΣ2Fe(t−τ(t))+eT(t)P˜CΣ−13˜CTPe(t)+[∫tt−hg(e(s))ds]TΣ3∫tt−hg(e(s))ds=eT(t)(−PˆD−ˆDTP+PˆAΣ−11ˆATP+FTΣ1F+P˜BΣ−12˜BTP+P˜CΣ−13˜CTP)e(t)+eT(t−τ(t))FTΣ2Fe(t−τ(t))+[∫tt−hg(e(s))ds]TΣ3∫tt−hg(e(s))ds. | (3.3) |
According to Eqs (a1)–(c1), the following inequality is valid for all t≠tk, where k∈N.
˙V(t)≤αeT(t)Pe(t)+θ1eT(t−τ(t))Pe(t−τ(t))+θ2∫tt−heT(s)Pe(t)ds≤αV(t)+θ1V(t−τ(t))+θ2∫tt−hV(s)ds. | (3.4) |
Otherwise,
V(tk)=eT(tk)Pe(tk)=eT(t−k)(E−μ˜Rk)TP(E−μ˜Rk)e(t−k)≤σ((E−μ˜Rk)T(E−μ˜Rk))eT(t−k)Pe(t−k)≤βV(t−k) | (3.5) |
holds for k∈N.
By integrating the conditions (a1)–(c1) with Lemma 2.3, we derive the subsequent conclusion:
λmin(P)||eT(t)e(t)||≤V(t)≤βN0sup−ϱ≤s≤0||˜φ(s)−˜ϕ(s)||2e−λ0t,t≥0, | (3.6) |
quasi,
||e(t)||≤√βN0λmin(P)sup−ϱ≤s≤0||˜φ(s)−˜ϕ(s)||e−λ02t,t≥0. | (3.7) |
Systems (2.1) and (2.2) exhibit an exponential synchronization, which conforms to the criteria specified in Definition 2.1.
In a similar fashion, utilizing the constraints (a2)–(c2) in conjunction with Lemma 2.4, we can ascertain that systems (2.1) and (2.2) exhibit an exponential synchronization.
The proof is completed.
Replace the impulse control mentioned in Eq (2.4) with the following impulsive control
˜u(t)=−˜Le(t)−μ∞∑k=1˜Rke(t)δ(t−tk), | (3.8) |
where the notation L=diag(l1,l2,…,ln), li>0 designates the feedback control gain matrix. The impulsive gain is μ. The control gain is Rk>0.
Remark 3.1. This kind of controller is widely used, which can be seen in [34], and the secondary controller can be used to expand the existing conclusions.
Consider the error function e(t)=y(t)−x(t), which quantifies the deviation between the outputs of networks (2.1) and (2.2). When these networks are interconnected, and the error dynamics are governed by Eq (3.8), the resulting error system is derived as outlined below:
{de(t)dt=−˜De(t)+˜Ag(e(t))+˜Bg(e(t−τ(t)))+˜C∫tt−hg(e(s))ds−˜Le(t), t≠tk,:=−ˆDe(t)+˜Ag(e(t))+˜Bg(e(t−τ(t)))+˜C∫tt−hg(e(s))ds, t≠tk,Δe(tk)=e(t+k)−e(t−k)=−μ˜Rke(t−k), t=tk,e(t)=˜ψ(t), −ϱ<t<0. | (3.9) |
By repeating the previous proof process, we can obtain the following theorem:
Theorem 3.2. Assume that the assumptions (A1) and (A2) are satisfied. Then, systems (2.1) and (2.2) are exponentially synchronized if exist real constants α>0, θ1>0, θ2>0, an n×n−matrix P>0, three diagonal positive definite n×n−matrix Σi>0,i=1,2,3, and an M such that the subsequent conditions are fulfilled:
Case Ⅰ: The impulsive strength is within the range 0<β<1:
(a1)
ˆΞ=[ˆξ11000FTΣ2F−θ1P000Σ3−1hM]<0, |
where ˆξ11=−PˆD−ˆDTP+P˜AΣ−11˜ATP+FTΣ1F+P˜BΣ−12˜BTP+P˜CΣ−13˜CTP−αP. (b1)
FTMF−θ2P<0, and |
(c1)
α+lnβTa+θ1+θ2hβN0<0. |
Case Ⅱ: The impulsive strength is within the range β≥1:
The three real constants in the theorem satisfy the relation α<0, θ1>0, θ2>0, conditions (a2) and (b2) are same as the conditions (a1) and (b1) in Case Ⅰ, and
(c2)
α+lnβTa+(θ1+θ2h)βN0<0. |
Proof. If we assume that V(t)=eT(t)Pe(t), and P is a positive integer martix, then we may show a method analogous to Theorem 3.1. The proof is completed.
Example 4.1. Consider the following model:
{dei(t)dt=−diei(t)+∑2j=1aijgj(ej(t))+∑2j=1bijgj(ej(t−τ(t))+∑2j=1cij∫tt−0.9fj(ej(s))ds+k1i∗ei(t)+k2i∗gi(ei(t),t≠tk,t≥0△ei(tk)=ei(t+k)−ei(t−k)=−μRke(t−k),t=tk, | (4.1) |
where the coefficients and functions are taken as follows:
D=[7.5006], A=[60.15−3.26], B=[−2.40.12−0.26−2.2], |
C=[2.5002], K1=[−1.500−3.3], K2=[−2.100−2.2]. |
g1(x)=g2(x)=tanh(x), τ(t)=0.8. |
In order to see the effect of control more clearly, we first consider the situation without control, see Figure 1.
Case(1). Let μ=0.9, P=E, Rk=E, tk=tk−1+0.02k,k∈N, and β=0.01. It is easy to calculate that ϱ=0.9, Fi=1,i=1,…,n, Ta=0.02,N0=1. By employing the MATLAB toolbox, we are capable of obtaining the subsequent details:
α=150, θ1=θ2=0.1, |
Σ1=[50000300], Σ2=Σ3=[0.09000.09]. |
Then, the system after the controller is made by MATLAB (Figure 2).
Case(2). Let μ=2.1, P=E, Rk=E, tk=tk−1+0.5k,k∈N, and β=1.21. It is easy to calculate that ϱ=0.9, Fi=1,i=1,…,n, Ta=0.5,N0=1. By employing the MATLAB toolbox, we are capable of obtaining the subsequent details:
α=−6, θ1=θ2=2, |
Σ1=[5000200], Σ2=Σ3=[1.9001.9] |
Then, the system after the controller is made by MATLAB (Figure 3).
Example 4.2. Consider the following model:
{dei(t)dt=−diei(t)+∑2j=1aijgj(ej(t))+∑2j=1bijgj(ej(t−τ(t))+∑2j=1cij∫tt−0.9fj(ej(s))ds−li∗ei(t),t≠tk,t≥0△ei(tk)=ei(t+k)−ei(t−k)=−μRke(t−k),t=tk,k∈N, | (4.2) |
where the coefficients and functions are defined as follows:
D=[2.5002], A=[20.15−1.23.5], B=[−1.70.12−0.26−1], |
C=[2.5002], L=[4.5005], |
g1(x)=g2(x)=12(|x+1|−|x−1|), τ(t)=0.8. |
In order to see the effect of control more clearly, we first consider the situation without control, see Figure 4.
Case(1). Let μ=0.9, P=E, Rk=E, tk=tk−1+0.04k,k∈N, and β=0.01. It is easy to calculate that ϱ=0.9, Fi=1,i=1,…,n, Ta=0.04,N0=1. By employing the MATLAB toolbox, we are capable of obtaining the subsequent details:
α=95, θ1=θ2=0.1, |
Σ1=[10010], Σ2=Σ3=[0.09000.09]. |
Then, the system after the controller is made by MATLAB (Figure 5).
Case(2). Let μ=2.1, P=E, Rk=E, tk=tk−1+0.5k,k∈N, and β=1.21. It is easy to calculate that ϱ=0.9, Fi=1,i=1,…,n, Ta=0.5,N0=1. By employing the MATLAB toolbox, we are capable of obtaining the subsequent details:
α=−6, θ1=θ2=2, |
Σ1=[5000200], Σ2=Σ3=[1.9001.9]. |
Then, the system after the controller is made by MATLAB (Figure 6).
This paper delved into the exponential synchronization challenge posed by NNs that incorporated time-varying delays alongside finite distributed delays. To tackle this, a hybrid impulsive controller was devised. Initially, two impulsive differential inequalities were rigorously proven. Subsequently, in the course of proving the main theorem, the original synchronization problem was strategically reframed into a category of impulsive differential inequalities through the application of an apt Lyapunov function. This transformation facilitated the derivation of sufficient corresponding conditions that leveraged lemmas. Ultimately, the validity and efficacy of the obtained conclusions were showcased through two illustrative numerical examples which focused on error analysis.
The attained conclusions affirmed the system's attainment of objectives, yet post-achievement, the control process persisted in the resource exhaustion. Consequently, contemplating a novel impulsive control strategy is advisable to halt the control upon the goal fulfillment. Furthermore, the model overlooked network couplings, which hindered its applicability to broader contexts.
The authors declare they have not used Artificial Intelligence (AI) tools in the creation of this article.
This work was supported by the National Natural Science Foundation of China under Grant 61573010, the Opening Project of Sichuan Province University Key Laboratory of Bridge Non-Destruction Detecting and Engineering Computing under Grant 2021QYJ06.
The authors declare there is no conflicts of interest.
[1] |
W. McCulloch, W. Pitts, A logical calculus of the ideas immanent in nervous activity, Bull. Math. Biol., 52 (1990), 99–115. https://doi.org/10.1016/S0092-8240(05)80006-0 doi: 10.1016/S0092-8240(05)80006-0
![]() |
[2] |
J. J. Hopfield, Neural networks and physical systems with emergent collective computational abilities, Proc. Natl. Acad. Sci. U.S.A., 79 (1982), 2554–2558. https://doi.org/10.1073/pnas.79.8.2554 doi: 10.1073/pnas.79.8.2554
![]() |
[3] |
C. M. Marcus, R. M. Westervelt, Stability of analog neural networks with delay, Phys. Rev. A, 39 (1989), 347–359. https://doi.org/10.1103/PhysRevA.39.347 doi: 10.1103/PhysRevA.39.347
![]() |
[4] | L.O. Chua, L. Yang, Cellular neural network: applications, EEE Trans Circuits Syst., 35 (1988), 1273–1290. https://doi.org/110.1109/31.7601 |
[5] |
N. Takahshi, A new sufficient condition for complete stability of cellular neural networks with delays, IEEE Transactions on Circuits & Systems I Fundamental Theory & Applications, 47.6 (2000), 793–799. https://doi.org/10.1109/81.852931 doi: 10.1109/81.852931
![]() |
[6] |
N. Takahshi, L.O. Chua, On the complete stability of non-symmetric cellular neural networks, IEEE Transactions on Circuits & Systems I Fundamental Theory & Applications, 145 (1998), 754–758. https://doi.org/10.1109/81.703843 doi: 10.1109/81.703843
![]() |
[7] |
Q. Song, Exponential stability of recurrent neural networks with both time-varying delays and general activation functions via LMI approach, Neurocomputing, 71 (2008), 2823–2830. https://doi.org/10.1016/j.neucom.2007.08.024 doi: 10.1016/j.neucom.2007.08.024
![]() |
[8] |
S. Arik, Stability analysis of delayed neural networks, IEEE Trans. Circuits Syst. I: Fundam. Theory Appl., 47 (2000), 1089–1092. https://doi.org/10.1109/81.855465 doi: 10.1109/81.855465
![]() |
[9] |
X. You, S. Dian, R. Guo, S. Li, Exponential stability analysis for discrete-time quaternion-valued neural networks with leakage delay and discrete time-varying delays, Neurocomputing, 430 (2021), 71–81. https://doi.org/10.1016/j.neucom.2020.12.021 doi: 10.1016/j.neucom.2020.12.021
![]() |
[10] |
A. Pratap, R. Raja, J. Cao, G. Rajchakit, E. A. Fuad, Further synchronization in finite time analysis for time-varying delayed fractional order memristive competitive neural networks with leakage delay, Neurocomputing, 317 (2018), 110–126. https://doi.org/10.1016/j.neucom.2018.08.016 doi: 10.1016/j.neucom.2018.08.016
![]() |
[11] |
F. Miaadi, X. Li, Impulsive effect on fixed-time control for distributed delay uncertain static neural networks with leakage delay, Chaos, Solitons Fractals, 142 (2021), 110389. https://doi.org/10.1016/j.chaos.2020.110389 doi: 10.1016/j.chaos.2020.110389
![]() |
[12] |
K. P. Lakshmi, T. Senthilkumar, Robust exponential synchronization results for uncertain infinite time varying distributed delayed neural networks with flexible delayed impulsive control, Math. Comput. Simul., 209 (2023), 267–281. https://doi.org/10.1016/j.matcom.2023.02.009 doi: 10.1016/j.matcom.2023.02.009
![]() |
[13] | S. Haykin, Neural networks and learning machines, in Pearson Education India, 2009. |
[14] | S. Niculescu, Delay Effects on Stability: A Robust Control Approach, Springer-Verlag, New York, 2001. https://doi.org/10.1007/1-84628-553-4 |
[15] |
X. Yang, Z. Yang, X. Nie, Exponential synchronization of discontinuous chaotic systems via delayed impulsive control and its application to secure communication, Commun. Nonlinear Sci. Numer. Simul., 19 (2014), 1529–1543. https://doi.org/10.1016/j.cnsns.2013.09.012 doi: 10.1016/j.cnsns.2013.09.012
![]() |
[16] |
Y. Wang, W. Cheng, J. Feng, S. Zang, H. Cheng, Z. Peng, et al., Silicon photonic secure communication using artificial neural network, Chaos, Solitons Fractals, 163 (2022), 112524. https://doi.org/10.1016/j.chaos.2022.112524 doi: 10.1016/j.chaos.2022.112524
![]() |
[17] |
O. Deqiang, S. Jie, J. Haijun, N. Sing Kiong, S. Heng Tao, Impulsive synchronization of coupled delayed neural networks with actuator saturation and its application to image encryption, Neural Networks, 128 (2020), 158–171. https://doi.org/10.1016/j.neunet.2020.05.016 doi: 10.1016/j.neunet.2020.05.016
![]() |
[18] |
J. Liu, L. Shu, Q. Chen, S. Zhong, Fixed-time synchronization criteria of fuzzy inertial neural networks via Lyapunov functions with indefinite derivatives and its application to image encryption, Fuzzy Sets Syst., 459 (2023), 22–42. https://doi.org/10.1016/j.fss.2022.08.002 doi: 10.1016/j.fss.2022.08.002
![]() |
[19] |
W. Wang, Y. Sun, M. Yuan, Z. Wang, J. Cheng, D. Fan, et al., Projective synchronization of memristive multidirectional associative memory neural networks via self-triggered impulsive control and its application to image protection, Chaos Solitons Fractals, 150 (2021), 111110. https://doi.org/10.1016/j.chaos.2021.111110 doi: 10.1016/j.chaos.2021.111110
![]() |
[20] |
W. Yao, C. Wang, Y. Sun, S. Gong, H. Lin, Event-triggered control for robust exponential synchronization of inertial memristive neural networks under parameter disturbance, Neural Networks, 164 (2023), 67–80. https://doi.org/10.1016/j.neunet.2023.04.024 doi: 10.1016/j.neunet.2023.04.024
![]() |
[21] |
Z. Jiang, F. Huang, H. Shao, S. Cai, X. Lu, S. Jiang, Time-varying finite-time synchronization analysis of attack-induced uncertain neural networks, Chaos, Solitons Fractals, 175 (2023), 113954. https://doi.org/10.1016/j.chaos.2023.113954 doi: 10.1016/j.chaos.2023.113954
![]() |
[22] |
S. Zhao, K. Li, W. Hu, Y. Wang, Finite-time synchronization of discontinuous fuzzy neural networks with mixed time-varying delays and impulsive disturbances, Results Control Optim., 12 (2023), 100253. https://doi.org/10.1016/j.rico.2023.100253 doi: 10.1016/j.rico.2023.100253
![]() |
[23] |
J. Xiao, Y. Hu, Z. Zeng, A. Wu, S. Wen, Fixed/predefined-time synchronization of memristive neural networks based on state variable index coefficient, Neurocomputing, 560 (2023), 126849. https://doi.org/10.1016/j.neucom.2023.126849 doi: 10.1016/j.neucom.2023.126849
![]() |
[24] |
W. Mao, S. You, Y. Jiang, X. Mao, Stochastic stabilization of hybrid neural networks by periodically intermittent control based on discrete-time state observations, Nonlinear Anal. Hybrid Syst, 48 (2023), 101331. https://doi.org/10.1016/j.nahs.2023.101331 doi: 10.1016/j.nahs.2023.101331
![]() |
[25] |
W. Zhou, Y. Hu, X. Liu, J. Cao, Finite-time adaptive synchronization of coupled uncertain neural networks via intermittent control, Physica A, 596 (2022), 127107. https://doi.org/10.1016/j.physa.2022.127107 doi: 10.1016/j.physa.2022.127107
![]() |
[26] |
J. Chen, B. Chen, Z. Zeng, Exponential quasi-synchronization of coupled delayed memristive neural networks via intermittent event-triggered control, Neural Networks, 141 (2021), 98–106. https://doi.org/10.1016/j.neunet.2021.01.013 doi: 10.1016/j.neunet.2021.01.013
![]() |
[27] |
J. Cai, J. Feng, J. Wang, Y. Zhao, Quasi-synchronization of neural networks with diffusion effects via intermittent control of regional division, Neurocomputing, 409 (2020), 146–156. https://doi.org/10.1016/j.neucom.2020.05.037 doi: 10.1016/j.neucom.2020.05.037
![]() |
[28] |
Y. Yang, Y. He, M. Wu, Intermittent control strategy for synchronization of fractional-order neural networks via piecewise Lyapunov function method, J. Franklin Inst., 356 (2019), 4648–4676. https://doi.org/10.1016/j.jfranklin.2018.12.020 doi: 10.1016/j.jfranklin.2018.12.020
![]() |
[29] |
S. Ling, H. Shi, H. Wang, P. X. Liu, Exponential synchronization of delayed coupled neural networks with delay-compensatory impulsive control, ISA Trans., 144 (2024), 133–144. https://doi.org/10.1016/j.isatra.2023.11.015 doi: 10.1016/j.isatra.2023.11.015
![]() |
[30] |
L. Wang, L. Li, Q. Cui, Z. Wang, Exponential synchronization of stochastic coupled neural networks with stochastic delayed impulsive effect, Neurocomputing, 604 (2024), 128262. https://doi.org/10.1016/j.neucom.2024.128262 doi: 10.1016/j.neucom.2024.128262
![]() |
[31] |
L. Shi, J. Li, H. Jiang, J. Wang, Quasi-synchronization of neural networks via non-fragile impulsive control: Multi-layer and memristor-based, Neurocomputing, 596 (2024), 128024. https://doi.org/10.1016/j.neucom.2024.128024 doi: 10.1016/j.neucom.2024.128024
![]() |
[32] |
W. Sun, Z. Tang, J. Feng, J. H. Park, Quasi-synchronization of heterogeneous neural networks with hybrid time delays via sampled-data saturating impulsive control, Chaos, Solitons Fractals, 182 (2024), 114788. https://doi.org/10.1016/j.chaos.2024.114788 doi: 10.1016/j.chaos.2024.114788
![]() |
[33] |
H. Fan, Y. Xiao, K. Shi, H. Wen, Y. Zhao, μ-synchronization of coupled neural networks with hybrid delayed and non-delayed impulsive effects, Chaos, Solitons Fractals, 173 (2023), 113620. https://doi.org/10.1016/j.chaos.2023.113620 doi: 10.1016/j.chaos.2023.113620
![]() |
[34] |
L. Shi, J. Li, H. Jiang, J. Wang, Quasi-synchronization of multi-layer delayed neural networks with parameter mismatches via impulsive control, Chaos, Solitons Fractals, 175 (2023), 113994. https://doi.org/10.1016/j.chaos.2023.113994 doi: 10.1016/j.chaos.2023.113994
![]() |
[35] |
Y. Lin, A. Lindquist, Synchronization of nonlinear delayed semi-Markov jump neural networks via distributed delayed impulsive control, Syst. Control Lett., 174 (2023), 105489. https://doi.org/10.1016/j.sysconle.2023.105489 doi: 10.1016/j.sysconle.2023.105489
![]() |
[36] |
K. Udhayakumar, S. Shanmugasundaram, A. Kashkynbayev, K. Janani, R. Rakkiyappan, Saturated and asymmetric saturated impulsive control synchronization of coupled delayed inertial neural networks with time-varying delays, Appl. Math. Modell., 113 (2023), 528–544. https://doi.org/10.1016/j.apm.2022.09.011 doi: 10.1016/j.apm.2022.09.011
![]() |
[37] |
X. Zhang, C. Li, H. Li, J. Xu, Delayed distributed impulsive synchronization of coupled neural networks with mixed couplings, Neurocomputing, 507 (2022), 117–129. https://doi.org/10.1016/j.neucom.2022.07.045 doi: 10.1016/j.neucom.2022.07.045
![]() |
[38] |
X. Li, M. Bohner, Exponential synchronization of chaotic neural networks with mixed delays and impulsive effects via output coupling with delay feedback, Mathematical and Computer Modelling, 52.5-6 (2010) 643–653. https://doi.org/10.1016/j.mcm.2010.04.01 doi: 10.1016/j.mcm.2010.04.01
![]() |
[39] |
F. Jiang, J. Shen, X. Li, The LMI method for stationary oscillation of interval neural networks with three neuron activations under impulsive effects, Nonlinear Anal. Real World Appl., 14 (2013), 1404–1416. https://doi.org/10.1016/j.nonrwa.2012.10.004 doi: 10.1016/j.nonrwa.2012.10.004
![]() |
1. | Yawei Liu, Guangyin Cui, Chen Gao, Event-triggered synchronization control for neural networks against DoS attacks, 2025, 33, 2688-1594, 121, 10.3934/era.2025007 | |
2. | Shuang Liu, Tianwei Xu, Qingyun Wang, Effect analysis of pinning and impulsive selection for finite-time synchronization of delayed complex-valued neural networks, 2025, 33, 2688-1594, 1792, 10.3934/era.2025081 |