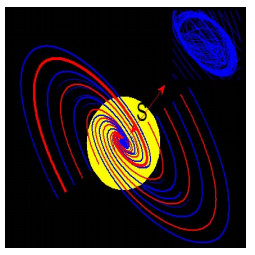
The synchronization problem and the dynamics analysis of neural networks have been thoroughly explored, and there have been many interesting results. This paper presents a review of the issues of synchronization problem, the periodic solution and the stability/stabilization with emphasis on the memristive neural networks and reaction-diffusion neural networks. First, this paper introduces the origin and development of neural networks. Then, based on different types of neural networks, some synchronization problems and the design of the controllers are introduced and summarized in detail. Some results of the periodic solution are discussed according to different neural networks, including bi-directional associative memory (BAM) neural networks and cellular neural networks. From the perspective of memristive neural networks and reaction-diffusion neural networks, some results of stability and stabilization are reviewed comprehensively with latest progress. Based on a review of dynamics analysis of neural networks, some applications in creation psychology are also introduced. Finally, the conclusion and the future research directions are provided.
Citation: Xiangwen Yin. A review of dynamics analysis of neural networks and applications in creation psychology[J]. Electronic Research Archive, 2023, 31(5): 2595-2625. doi: 10.3934/era.2023132
[1] | Shuang Liu, Tianwei Xu, Qingyun Wang . Effect analysis of pinning and impulsive selection for finite-time synchronization of delayed complex-valued neural networks. Electronic Research Archive, 2025, 33(3): 1792-1811. doi: 10.3934/era.2025081 |
[2] | Eduardo Paluzo-Hidalgo, Rocio Gonzalez-Diaz, Guillermo Aguirre-Carrazana . Emotion recognition in talking-face videos using persistent entropy and neural networks. Electronic Research Archive, 2022, 30(2): 644-660. doi: 10.3934/era.2022034 |
[3] | Yong Zhao, Shanshan Ren . Synchronization for a class of complex-valued memristor-based competitive neural networks(CMCNNs) with different time scales. Electronic Research Archive, 2021, 29(5): 3323-3340. doi: 10.3934/era.2021041 |
[4] | Jun Guo, Yanchao Shi, Shengye Wang . Synchronization analysis of delayed quaternion-valued memristor-based neural networks by a direct analytical approach. Electronic Research Archive, 2024, 32(5): 3377-3395. doi: 10.3934/era.2024156 |
[5] | Xingting Geng, Jianwen Feng, Yi Zhao, Na Li, Jingyi Wang . Fixed-time synchronization of nonlinear coupled memristive neural networks with time delays via sliding-mode control. Electronic Research Archive, 2023, 31(6): 3291-3308. doi: 10.3934/era.2023166 |
[6] | Yilin Li, Jianwen Feng, Jingyi Wang . Mean square synchronization for stochastic delayed neural networks via pinning impulsive control. Electronic Research Archive, 2022, 30(9): 3172-3192. doi: 10.3934/era.2022161 |
[7] | Minglei Fang, Jinzhi Liu, Wei Wang . Finite-/fixed-time synchronization of leakage and discrete delayed Hopfield neural networks with diffusion effects. Electronic Research Archive, 2023, 31(7): 4088-4101. doi: 10.3934/era.2023208 |
[8] | Xinzheng Xu, Xiaoyang Zhao, Meng Wei, Zhongnian Li . A comprehensive review of graph convolutional networks: approaches and applications. Electronic Research Archive, 2023, 31(7): 4185-4215. doi: 10.3934/era.2023213 |
[9] | Chao Yang, Juntao Wu, Zhengyang Qiao . An improved fixed-time stabilization problem of delayed coupled memristor-based neural networks with pinning control and indefinite derivative approach. Electronic Research Archive, 2023, 31(5): 2428-2446. doi: 10.3934/era.2023123 |
[10] | Xudong Hai, Chengxu Chen, Qingyun Wang, Xiaowei Ding, Zhaoying Ye, Yongguang Yu . Effect of time-varying delays' dynamic characteristics on the stability of Hopfield neural networks. Electronic Research Archive, 2025, 33(3): 1207-1230. doi: 10.3934/era.2025054 |
The synchronization problem and the dynamics analysis of neural networks have been thoroughly explored, and there have been many interesting results. This paper presents a review of the issues of synchronization problem, the periodic solution and the stability/stabilization with emphasis on the memristive neural networks and reaction-diffusion neural networks. First, this paper introduces the origin and development of neural networks. Then, based on different types of neural networks, some synchronization problems and the design of the controllers are introduced and summarized in detail. Some results of the periodic solution are discussed according to different neural networks, including bi-directional associative memory (BAM) neural networks and cellular neural networks. From the perspective of memristive neural networks and reaction-diffusion neural networks, some results of stability and stabilization are reviewed comprehensively with latest progress. Based on a review of dynamics analysis of neural networks, some applications in creation psychology are also introduced. Finally, the conclusion and the future research directions are provided.
A neural network is a kind of network structure which can be used to deal with practical problems involving multiple nodes and multiple output points, see [1,2,3]. Although both the human brain and artificial neural networks have extremely powerful information processing capabilities, there are many differences between them. Different from information processing by the human brain, robots developed by using neural networks process information in a linear way of thinking, and computers have fast, precise sequential numerical calculations, which leads to the fact that the robot with neural networks outperform humans in some fields.
The concept of neural networks (also called artificial neural networks) was originated by Warren McCulloch and Walter Pitts in 1943. They created a mathematical and algorithm-based computing model of neural networks for the first time, see [4]. It marked the sprout of neural networks. Hebb hypothesized that the change of synaptic weights might control the way neurons excite each other. In 1949, synapses and Hebb learning rules were put forward, which constructed the theoretical knowledge based on the development of neural network algorithms, see [5]. In the late 1950s, Rosenblatt created a perceptron based on a model, which was the first neural network that was physically constructed and had the ability of learning, see [6]. Minsky and Papert pointed out that the single-layer perceptron of Rosenblatt can only learn linearly separable modes but cannot deal with linearly separable problems such as xor, see [7]. In 1984, the Hopfield neural network was first introduced in [8]. The model was described by nonlinear differential equation, simulated by circuit system and analyzed by mathematical tools. After that, there were many interesting results of dynamical behaviour of Hopfield neural network, which played a vital role in information processing and engineering research. Subsequently, [9] proposed the concept of Backpropagation neural networks, which can be applied to solve the problem reflected by multi-layer neural networks. However, there are some disadvantages of Backpropagation neural networks, such as slow convergence. In 1988, [10] was inspired by Hopfield neural networks and developed a large-scale nonlinear analog circuit, called cellular neural networks. Different from Hopfield neural networks, cellular neural networks are more in tune with biological characteristics in the topology of cell connections. Specifically, the activation function of cellular neural networks can be represented by piecewise linear function, which makes it easier to complete the hardware of cellular neural networks. Meanwhile, the BAM neural network model was proposed in terms of the brain's different associative thinking mode. The model used a two-layer network structure to make the state of the network transfer back and forth between the two layers of neurons. [11] and [12] presented the prototype of convolutional neural network (i.e., LeNet-5) and the deep belief network, respectively. Recently, neural network is a very hot topic in various fields. By simulating the principle and process of biological nerve cells, this model describes the mathematical theory and network structure of artificial neurons, and it proves that a single neuron can achieve logical functions. Hence, it opens the era of the research of neural networks.
It is worth mentioning that in 1971, L. O. Chua boldly predicted that there must be a relationship between magnetic flux and charge according to the principle of the completeness of circuit variable combination, and named the circuit element describing the relationship between them as memristor. Since then, the memristor-based neural networks (also called memristive neural networks) have attracted the attention of scholars. Note that some scholars studied continuous memristive neural network, such as [13]. Other scholars were concerned with the model of memristive neural network that is a form of a discontinuous differential equation, which leads to the fact that some theories about neural networks cannot be applied directly. Hence, it is difficult to study the dynamics of memristive neural networks. Since the 1980s, Lyapunov stability theory has been successfully applied to the stability and stabilization of memristive neural networks, and there is a series of achievements, such as [14]. Moreover, memristive neural networks are widely used in associative memory [15], pattern classification with unsupervised learning [16], artificial intelligence [17] and other fields of application and promotion. The stability and stabilization problems of memristive neural networks have brought about widespread attention.
In fact, every kind of movement in the world takes place in a certain space-time environment, such as electrochemical reaction process, propagation of waves, and population migration. Hence, when modeling this kind of system, both time and space variables should be considered in the mathematical model. Also, the diffusion phenomenon is inevitable when electrons move in non-uniform electric field, which can result in structural and dynamic changes in the system model implemented through the circuit system. Therefore, the reaction-diffusion term should be considered in the constructed network model. It is not only the extension of network system research, but also the extension of the practical application of reaction-diffusion neural network. When considering the influence of diffusion phenomenon, the main problems are: the stability and stabilization of neural network, the existence of equilibrium point, the existence of periodic solution, synchronization and other problems. These problems have also aroused wide attention and research.
In addition, there are some applications in creation psychology. The most critical link in the process of literary and artistic creation is the generation of literary and artistic creativity, that is, the generation of new ideas, which involves the common processing of many brain regions. At present, neuroaestheticians have conducted brain scanning experiments on the creation process of literature, painting and music, and they described the results of brain neuroimaging experiments on the generation and evaluation process of literary and artistic creativity. As for the neural basis of creative writing, the experimental results of Natalia P. Bekhtereva et al. showed that verbal creativity was associated with the activation of the dorsal and ventral prefrontal cortex in both hemispheres. To investigate the neural mechanisms of literary creative production, Carolin Shah et al. conducted a functional magnetic resonance imaging study. The experiment observed the participants' creative writing process through brain scanner, which is an important experiment in the field of research on the neural basis of literary creative production. Brain regions involved in cognitive writing processing are also activated during creative writing. These brain regions include language, memory and visual areas, and they are involved in language processing, semantic integration, working memory, episodic memory, memory retrieval, free association and higher cognitive control. Corresponding to the general system of neural mechanism of literary and artistic creation, the creative production of different literary and artistic types is supported by different special nervous systems and has processing characteristics. Different types of creativity have their own distinct processing brain regions. With the development of artificial neural networks [18,19], artificial intelligence literature that relies on the machine chip came into being, which is different from human literature, dependent on the literary heart. By inputting large amounts of literary text, letting the machine process the text through deep learning techniques, the artificial intelligence generates the literature work. Different from human literature, artificial intelligence literature lacks the emotions that is characteristic of humans.
In this paper, we present a review of recent advances on neural networks with emphasis on synchronization, stability and stabilization of neural networks. The rest of the paper is organized as follows. Section 2 introduces the overview of synchronization of neural networks. Section 3 provides the periodic solution of neural networks. Sections 4 and 5 give some interesting results from the perspective of the memristive neural networks and the reaction-diffusion neural networks, respectively. Section 6 introduces some applications in creation psychology. Section 7 presents the conclusion.
Synchronization phenomena are ubiquitous in nature and human society and reveal that various system states reach the same values under some time frame. For example, fireflies flash synchronously, flocks of fish or birds migrate in special formations, and audiences applaud with equal frequency. In the field of engineering and control, the synchronization of chaotic neural networks has played a vital role in secure communication [20] and image encryption [21], which has fascinated numerous researchers over the past decade. In the frame of drive-response synchronization, drive system is usually chaotic and independent of response system, while the design of response system is relevant with the drive system. A control input is usually designed to make the states of response system approach the states of the drive system, namely, the synchronization error between drive and response systems approaches zero under the designed control input. Due to different actual situations, various synchronization types and synchronization control methods are proposed. In this part, we focus on the differences between various synchronization types. Then, we review several synchronization methods and discuss their advantages and shortcomings.
It is common for synchronization type to meet multifarious requirements in practice. It follows from the different sizes of synchronization error that we can define five kinds of synchronization, namely, complete synchronization [22], quasi-synchronization [23], generalized synchronization [24], lag synchronization [25], projective synchronization [26]. Complete synchronization implies that the synchronization error strictly approaches zero after a period of time. Moreover, complete synchronization plays an important role in the process of secure communication based on chaos since the accurate cryptographic information can be obtained only when complete synchronization is achieved [27]. Unfortunately, in the application on the formation control of multiple agents, if state information describes the position information of agents, then complete synchronization may be not suitable since collision avoidance is necessary, while other synchronization types can be applied to reveal the formation information of agents [28]. Unlike complete synchronization, the synchronization error on quasi-synchronization may not approach to zero and stay at a given bound. Meanwhile, in generalized synchronization, the synchronization error can be described by a known function. In other words, there exists a function relationship between the states of drive system and response system. When the function is chosen as a projective function, then the generalized synchronization degrades into projective synchronization. As for lag synchronization, the difference from other synchronization types reflects on time scale. The current states of response system reach the consensus with the historical states of drive system in lag synchronization. In addition, if the synchronization rate is considered, then asymptotic synchronization [29], exponential synchronization [30] and finite-time synchronization [31] can be distinguished. The concept of asymptotic synchronization is more general than the other two concepts and does not specifically restrict the rate of convergence. While exponential synchronization emphasizes that the synchronization rate is exponential. However, it is difficult to estimate the convergence time. Different from exponential synchronization, the finite-time synchronization not only has a desired synchronization rate, but also obtains a settling time depending on initial synchronization error.
Since drive system is independent of response system, if control input is not implemented on response system, then the synchronization between drive system and response system cannot be achieved. In the past few years, some interesting synchronization control strategies have been proposed to achieve the synchronization goal. Next, we review the recent developments in the synchronization of neural networks.
As a class of traditional control methods, state-feedback control has been successfully applied to synchronization of chaotic neural networks due to its simple design and significantly effective control performance, such as [32]. In [33], asymptotic synchronization of impulsive neural networks with time delay is studied, where parametric uncertainties with linear fractional form, which covers norm bounded parameter uncertainties as a special case, are fully considered. In terms of linear matrix inequality approach, some sufficient conditions are proposed, and a delay-dependent state-feedback controller is designed to achieve the synchronization. However, the synchronization rate cannot be estimated, and the feedback control gain is constant and unchanged, which may lead to conservatism such that the obtained results cannot be applied to some complex cases. In order to improve the results in [33], an adaptive state-feedback controller is proposed to achieve the finite-time synchronization of memristive neural networks with mixed time delay in [34], where an adaptive law is designed to deal with memristive connection weights. Based on the Forti Lemma and the Hardy inequality, some interesting synchronization criteria are established, where the estimated settling time depends on adaptive parameters. It is shown that the settling time of synchronization is relevant with the system parameters and the adaptive controller. In this kind of control, the state information of drive system is directly utilized to compel the states of response system to approach the drive system. However, under most applications, it is difficult to obtain the state information, or the state information is not measured. While, output information is usually obtained easily, and some external disturbances are usually unavoidable. In [35], a class of coupled reaction-diffusion neural networks with external disturbance is considered. In order to describe the effect of external disturbance on the synchronization of reaction-diffusion neural networks, the synchronization is investigated in terms of the Lyapunov functional method, where a sampled-based output feedback controller is designed based on the linear matrix inequality technique. This kind of controller can reduce the update rate in the control process of control by sampling output information at some fixed sampling points. Different from [35], the authors in [36] consider the effect of stochastic disturbances on the dynamic of reaction-diffusion neural networks. Utilizing It\^{o} differential formula, some sufficient conditions on the asymptotical synchronization of reaction-diffusion neural networks in mean square are established, where a non-fragile output-feedback controller is designed in terms of linear matrix inequality. In addition, malicious attacks may occur due to the open and available communication networks. In [37], the control effect of output feedback control on the synchronization of neural networks is studied under mixed-type network attacks, where deception, replay, and denial-of-service attacks are modeled in the frame of a unified Markov. Based on the Lyapunov-Krasovskii theory and stochastic analysis techniques, some synchronization criteria are established, where the static output feedback controller can be designed by a convex optimization algorithm.
In practice, the bandwidth of communication channels usually restrict the transmission of signals. To improve the efficiency of communication and avoid information blocking, it is necessary to quantize the transmission signals when those signals are transmitted. In [38,39,40], the control effect of quantized control on the synchronization of neural networks is investigated, and the concept of Filippov solution is used to deal with the quantized function, where stochastic exponential synchronization of memristive neural networks with stochastic perturbations is considered in [38], while [39] considers coupled neutral-type neural networks with mixed delays and output information utilized at quantized controller. Different from [38,39], a more general neural network model, namely, BAM neural networks with discontinuous neuron activation function, are considered in [40]. A new quantized controller is designed to achieve the finite-time synchronization, where the sign function is not used such that the chattering phenomenon does not occur in the designed controller.
When the concerned response system consists of a large number of neural networks, especially large-scale neural networks, then a corresponding controller is designed for every neural networks to achieve the synchronization, which leads to tremendous control costs. Pinning control provides an effective control strategy such that the synchronization of neural networks can be achieved by controlling a part of a response neural networks. In [41], the pinning synchronization of coupled reaction-diffusion neural networks is investigated, where a feedback controller is implemented on the part nodes of response system. Combined with the Kronecker product and the linear matrix inequality, some sufficient conditions are proposed to guarantee the asymptotic synchronization. Further, in [42], exponential synchronization of discontinuous Cohen-Grossberg neural networks is studied based on pinning control, where the discontinuous neural activated function and connection weight coefficient uncertainties are involved. An adaptive pinning control strategy is proposed to deal with uncertainties and achieve the exponential synchronization. One may observe that the connection relationship of neural networks plays an important role in pinning control. Although there exist some uncontrolled network nodes, the pinning network nodes have an effect on the uncontrolled network nodes by the connection relationship to ensure that all network nodes can be synchronized with the drive neural networks.
The above mentioned control methods are continuous, which may leads to the wasting of control resources and limited applications. Intermittent control, as a class of discontinuous control methods, has been extensively studied over the past few years, where the designed controller only works on a control interval and stops working on a rest interval. In [43], a periodically intermittent control method is developed to achieve the exponential synchronization of inertial neural networks. The key of the control method is to deal with the relationship between the dynamics of the response system over the control interval and rest interval. However, the periodical time-triggered control law leads to the conservatism of the obtained results. In order to overcome the shortcoming, the authors in [44] propose an aperiodic intermittent control method and apply the method to the synchronization of memristive neural networks, where the length of each control interval is nonidentical and different. On the basis of [44], [45] further considers the aperiodic intermittent control method to achieve the synchronization of fractional-order neural networks. A piecewise Lyapunov function method is utilized to analyze the dynamical behaviors of drive and response systems such that the obtained results are less conservative than the results in [44].
As another class of discontinuous control methods, impulsive control has fascinated numerous researchers within the past few years. The basic idea of impulsive control is to make states abruptly change to approach the equilibrium state at some discrete instants. Unlike other control methods, impulsive control only needs a small control gain to achieve the desired performance. In [46], some impulsive synchronization criteria on reaction-diffusion neural networks with multiple time-varying delays are established, where impulsive control only depends on current states. Different from [46], a distributed delay is introduced into impulsive control in [47] such that impulsive control not only depends on current states but also is related to historical states. However, the interval of impulsive control is strictly restricted in those results in [46]. This restriction is relaxed in [48], and an average impulsive interval method is utilized such that impulsive control interval is not restricted by a certain constant. Some interesting synchronization results on coupled neural networks are proposed based on inequality technique and comparison principle. On the basis of [48], [49] further considers the effect of impulse saturation on the synchronization of neural networks. A dead-zone function method is used to deal with impulse saturation. Combined with linear matrix inequality, synchronization criteria on coupled neural networks are established, and an optimization algorithm is proposed to estimate the domain of attraction.
In addition, although time-triggered control strategies are characteristic as simple design and easy implementation, they lead to a high frequency of control signal transmission and updates. Under the case of maintaining control performance, how to significantly save control costs and communication resources is an important issue. From the saving control costs point of view, event-triggered control strategy presents a desired feature, where sampling data and updating control information are determined by a preset event triggering condition, which is related to the concerned system as a performance index. Once the event triggering condition is violated, which implies that the system performance is deteriorating, and the current control has a bad effect, then the system states should be sampled, and control information should be updated. In [50], the synchronization of memristive neural networks is investigated based on the event-triggered control, where an event-triggered mechanism with fixed triggering threshold is designed to increase the inter-triggering intervals and improve the control effect. An adaptive control gain update law is designed to deal with uncertain parameters in the controller. In order to further save control cost, double event-triggered control strategies are proposed to achieve the synchronization of chaotic neural networks in [51], where twice event-triggered mechanisms are implemented on the sense-to-controller channel and controller-to-actuator channel, respectively. Moreover, the second event-triggered mechanism is based on the first event-triggered mechanism. In other words, the second triggering instants belong to the subset of the first triggering instants, which further saves control cost and reduces communication burden. Different from the event-triggered mechanism of continuous control, some interesting stability results for nonlinear system based on event-triggered impulsive control strategy is proposed in the sense of Lyapunov in [52]. On the basis of [52], the event-triggered impulsive control strategy is applied to the synchronization of neural networks in [53].
There are many dynamical models of neural networks, each of which has its own unique dynamical behavior and characteristics. The various dynamical models of a neural network may generally have three dynamical behaviors:
1) Convergence: An orbit converges to an equilibrium or stable state as time gets longer and longer, and a part of the orbit converges to a set of equilibrium points (an equilibrium point may be stable or unstable, i.e., the orbit will move away from it), see Figure 1.
2) Oscillation: An orbit asymptotically tends to a periodic orbit which may be stable or unstable, see Figure 2.
3) Chaos: The long-term behavior of an orbital, usually roughly defined, within a bounded range, which is extremely sensitive to initial values, see Figure 3.
Based on the numerical simulation and theoretical analysis of various existing dynamical models of artificial neural networks, as well as people's mental state and some practical application needs, the first kind of convergence dynamical behavior has been studied more and more deeply [54]. It is always believed that dynamical behavior should ultimately be information obtained or generated, and it is natural to expect this information to be a form of capture, that is, the equilibrium point of a stable state, which can be useful to obtain, such as pattern recognition, combinatorial optimization, and so on [55]. However, if the first kind of dynamical behavior is analyzed from the biological neural network, it is quite untrustworthy and may even cause a lot of contradictions. In recent years, from the point of view of neurobiology, it has been found that people's brains can process information and have so many functions, mainly because the neural network dynamic system has the second and third dynamical behaviors [56]. However, most of the studies on the theory and application of neural networks focus on the study of the unique global convergence equilibrium point or stability of neural networks. Because of the complexity of the latter two dynamical behaviors, many mathematical tools cannot be applied and develop very slowly. In particular, the third dynamic behavior, chaos, as a nonlinear dynamic phenomenon, is an internally random and initial value sensitive phenomenon, and the famous "butterfly effect" [57] is a manifestation of its initial value sensitivity. Chaos can be combined with artificial neural network to simulate and interpret human brain, process information, establish the mechanism of transmitting information and truly reflect the internal mechanism of biological nervous system [58,59]. However, chaotic neural network models are usually fixed, and the output is chaotic, which cannot converge to periodic states or fixed points, and it is necessary to exert control over the network to be studied, while the applied control often fails to satisfy the inherent dynamic characteristics of real neurons. Based on the above, there is little research on the third dynamical behavior compared to the other two.
As we all know, periodic phenomena can be seen everywhere in nature, such as day and night cycles, element cycles in chemistry, and so on. Therefore, the exploration of the existence of periodic solutions is one of the most important aspects of ordinary differential theory [60]. In fact, a equilibrium point can be regarded as a special periodic solution of the neural network with any period or zero amplitude, so the study of the periodic solution of the neural networks is more general than that of the equilibrium point. In recent years, there have been many studies on the (almost) periodic solution of various kinds of neural networks, including the existence, uniqueness and stability of the periodic solution [61].
In 1987, B. Kosko extended the single-layer unidirectional associative memory network to a bidirectional dual-layer structure, namely, BAM neural network [62]. BAM neural networks have great potential in pattern recognition, associative memory and so on, so they have received extensive attention. In [63], some sufficient conditions for the existence and asymptotic stability of periodic solutions for BAM neural networks are established. Mawhin extension theorem of coincidence degree and Lyapunov functional method are used to consider BAM neural networks with constant coefficients and constant time delays. In [63], the existence, uniqueness and global exponential stability of periodic solutions of BAM neural networks with time-varying delays are studied by using inequality techniques, Lyapunov functional methods, and fixed-point theorem. The existence and stability of the equilibrium points and periodic solutions of BAM neural networks with periodic coefficients and time-varying delays are studied in [64]. However, most of the activation functions are required to have the properties of boundedness, monotonicity and differentiability, and the Lipschitz condition is usually satisfied. These limitations bring inconvenience to the application of some important theories in engineering technology and physical problems. [65] established a criteria ensuring the existence and global asymptotic stability on periodic solutions for a class of discrete-time Quaternion-valued BAM neural networks by constructing two Lyapunov sequences, without applying the traditional priori estimate approach of periodic solutions or the fixed point theorem approach.
The Wilson-Cowan model, proposed by famous scholars Wilson and Cowan in 1972 [66], is an equation describing the dynamical evolution of neuron populations with different characteristics of neural networks. The model is composed of two nonlinear differential equations, representing the relationship between two groups of neurons that interact to produce excitation and inhibition. Wilson-Cowan networks have attracted much attention in studying the periodic solution of networks. For example, [67] used symmetry properties and Poincare mapping to find the parameter space of the periodic vibration region, and it proved that there are three or more periodic attractors in Wilson-Cowan. In [68], authors investigated the excitatory or inhibitory behavior generated by the interaction of neurons in Wilson-Cowan networks, and they had completely different effects on the activation or inhibition of neurons under periodic input. [69] proposed an extension of classical Wilson-Cowan model, and deduced a dynamical system closely related to the original model. This dynamical system can predict the oscillation and chaotic behavior in the neural network activity of excitable population, which is in sharp contrast to the original Wilson-Cowan model.
Cellular Neural Networks (CNNs) have been deeply studied in many publications since they were proposed by L. O. Chua and L. Yang in 1988 [10]. The research results also show that CNNs have a wide range of applications in pattern recognition, memory and signal processing, image processing and computer technology. [70,71] studied the existence and global stability of periodic solutions of cellular neural networks with time delays. By using the continuation theorem of Mawhin's coincidence degree theory and constructing a suitable Lyapunov function, some sufficient conditions are derived to guarantee the existence and global exponential stability of periodic solutions for quaternion-valued CNNs in [72]. [73] studies the existence, uniqueness and global exponential stability global exponential attractivity of -pseudo almost periodic solutions of CNNs with mixed delays.
In addition to the study of the existence and stability of periodic solutions of neural networks, the generalization of almost periodic functions as periodic functions has also attracted many scholars' interests [74,75]. An almost periodic function is a special kind of bounded continuous function. In real life, almost periodic phenomena can better describe the development of some processes than periodic phenomena, such as mechanical vibration in physics, celestial mechanics, population dynamics in ecosystems and even the law of market supply and demand in economics. These practical problems can usually be transformed into the existence of almost periodic solutions of differential equations and solved by discussing their properties. In real life, there are not only periodic phenomena but also a large number of anti-periodic behaviors. The existence of anti-periodic solutions plays an important role in describing the dynamical behavior of nonlinear differential equations [76,77]. In [76], the existence and global exponential stability of anti-periodic solutions for a class of field neural network systems with impulses are studied. Considering that higher-order neural networks have stronger approximation and faster convergence speed than lower order neural networks, [77] obtains some sufficient conditions for the existence and exponential stability of anti-periodic solutions for high-order Hopfield neural networks with time-varying delays.
This section will give several classes of memristors, some methods or tools to deal with the dynamical behaviors of memristor-based neural networks (also called memristive neural networks) and some results of stability or stabilization, passivity and dissipativity for memristive neural networks.
As a new type of memory device, the memristor is similar to the synapses of human neurons that can realize information storage and information processing somatic function, which provides a completely new design for the next generation of computers architecture. In accordance with the complexity of mathematical model of memristor, memristors can be classified into four types: ideal memristors, generic memristors, ideal generic memristors and extended memristors. There are two points worth mentioning: 1) As pointed out in [78], the ideal memristor fits the original definition of a memristor, where a unique relationship between the flux relevant to the memristor current and the charge related to the memristor voltage is established by a one-port; 2) by some mathematical transformations, the ideal generic memristor can be transformed into the ideal memristor. Hence, the ideal generic memristor is also called the derived memristor of the ideal memristor.
Memristor-based neural networks have become a research hotspot due to the fact that the memristor is nano-sized and has a higher degree of integration. Hence, as an artificial neuron synapse, memristive neural networks have an unparalleled advantage over other components. Compared with the traditional artificial neural networks, there are some advantages [79] of memristive neural networks and some applications, such as associative memory [80], pattern classification with supervised learning [81] and chaotic system based on memristor neural network[82,83,84].
● Memristive neural networks have self-learning function. For example, in the process of image recognition, when entering many different image samples and corresponding recognition results into the memristive neural network, the memristive neural network will gradually learn to recognize similar images through self-learning function. By learning historical data, a specific neural network system with the ability to generalize all data can be trained. In addition, self-learning function is of vital importance for prediction.
● Memristive neural networks have associative storage function. The feedback network of artificial neural network can realize this associative memory function.
● Memristive neural networks have the ability to find optimal solutions at high speed. As we all know, it is a very computation-intensive process to find the optimal solution of a complex problem, but designing a feedback type artificial neural network for a certain problem and giving full play to the high-speed computing power of the computer, the optimal solution can often be found quickly.
● Memristive neural networks have nonlinear processing function. Memristor neural network can simulate human brain thinking, which is a form of nonlinearity, and deal with nonlinear problems.
● Memristive neural networks have the property of adaptivity. Conventional neural network circuits often cannot deal with new patterns, new data, not to mention the ability to self-regulate. On the contrary, memristive neural networks have a strong adaptivity to new patterns and new data.
It is worth noting that a kind of memristive neural networks can be regarded as a class of state-dependent switching systems, whose right is discontinuous. Hence, the classical stability theory and methods of differential equations that is in right continuous sense is invalid. At present, there are two main ways to deal with memristive neural networks: 1) discuss the research directly with the help of some existing analytical techniques and methods, such as [85,86,87]; 2) based on the theory of differential inclusion and set-valued mapping, the dynamical behavior of memristive neural networks are analyzed in the framework of Filippov's right discontinuity stability theory [88,89].
In the following, we will present some interesting results according to different classes of memristive neural networks.
It is worth noting that time-delay is inevitable in reality. Many scholars have fully considered the effect of time-delay on the memristive neural networks, such as [90]. The time-delay considered in memristive neural networks can be divided into several types: constant time-delay, time-varying delay, discrete time-delay, leakage time-delay, distributed time-delay, proportional time-delay, probabilistic time-delay, mixed time-delay, and unbounded time-delay. To be specific, [90] obtained the global uniform asymptotic stability of memristor-based recurrent neural networks in terms of differential inclusion. Subsequently, [91] studied the memristor-based recurrent neural networks and extended global uniform asymptotic stability to the global exponential stability. By constructing a new Lyapunov-Razumikhin function, some sufficient conditions guaranteeing the global exponential stability were proposed, where the presented results in [91] were applicable to both cases with and without time-delay. With the help of linear matrix inequality, [92] proposed a suitable Lyapunov-Krasovskii functional to guarantee the global asymptotic stability of memristive neural networks. In the framework of discontinuous right-hand side, [93] put forward some new criteria for guaranteeing the existence and the global exponential stability of periodic solution. It was also shown from simulations that the proposed algebraic criteria are very easy to verify. [94] investigated fractional-order memristive neural networks and developed set-valued maps and fractional-order differential inclusion to achieve global Mittag-Leffler stabilization. Different from [94], for fractional-order memristive neural networks, [95] presented two types of delay-independent stability criteria by applying the spectral radii of matrices and the maximum modulus principle, respectively. From the perspective of time-delay analysis, to guarantee the dynamical behaviors, some additional restrictions on the time-delay are imposed. For example, for time-varying delay, it and its derivatives are both assumed to be bounded in [96], and a novel Lyapunov function approach was put forward to study the periodic solution for a class of memristive neural networks with time-varying delay. Furthermore, [97] extended the existing results in [96] to the memristive neural networks with unbounded time-delay, where the boundedness of the time-delay was completely dropped, and only the boundedness of the derivative of time-delay is needed. Under such constraint on time-delay, [97] presented some sufficient conditions to guarantee the exponential stabilization of memristive neural networks.
Note that these mentioned results are based in the infinite-time domain. There are some interesting results based in the finite-time domain. For instance, [86] studied the stabilization of delayed memristive neural networks in the finite-time interval and estimated the upper bound of the settling time for delayed memristive neural networks. [98] extended the existing results in [86] to the complex-valued memristive neural networks, where two new inequalities were put forward to design the nonlinear delayed controller. Based on a similar idea, [99] considered proportional delay in memristive neural networks and dealt with the finite-time stabilization problem. [100] proposed two classes of novel hybrid controllers that consist of discontinuous state-feedback controller and discontinuous adaptive controller, which can guarantee the finite-time stabilization of delayed memristive neural networks. [101] considered delayed memristive neural networks involving the external disturbance. By designing sliding-mode surface, [101] achieved the stabilization in the sense of finite-time and fixed-time, respectively.
In the framework of the fuzzy model, there are some meaningful results. [102] extended the existing results to the Takagi-Sugeno fuzzy memristive neural networks. In terms of comparison principle, [102] designed a class of fuzzy state feedback controllers and obtained the stabilization criterion in Filippov sense. Subsequently, in Lagrange sense, [103] came up with some new scale-limited criteria of global exponential stability by utilizing matrix-norm strategies. Based on the results in [102], [104] considered unbounded discrete and distributed time-varying delays and presented exponential stabilization conditions by using theories of fuzzy sets. [105] analyzed the finite-time stabilization of fuzzy memristive neural networks by designing a nonlinear state feedback controller, where the boundedness of the activation function was completely dropped. In addition, many scholars have also designed other types of controllers for fuzzy memristive neural networks. For example, [106] deigned a class of periodically intermittent controllers to guarantee the exponential stabilization, and moreover, such controller was applied to synchronization of fuzzy memristive neural networks. [107] focused on a class of switched fuzzy sampled-data controllers, based on which the stabilization criterion of fuzzy memristive neural networks was guaranteed by using the fuzzy membership functions-dependent Lyapunov-Krasovskii functional. In [108], a fuzzy adaptive event-triggered sampled-data controller was first proposed. Under the mentioned controller, not only the stabilization can be guaranteed, but also the limited communication resources can be saved effectively. Based an event-triggered scheme, [109] designed a class of fuzzy event-triggered controllers and proposed some sufficient conditions of stabilization for complex-valued memristive neural networks. [110] developed the vector ordering approach to guarantee the stabilization of fractional-order quaternion-valued fuzzy memristive neural networks.
In practical applications, memristive neural networks are implemented through a series of electronic devices such as resistors, capacitors, memristors and amplifiers. On the one hand, these electronic devices have their own parameters uncertainty. On the other hand, the signal storage and synaptic transmission between neurons are affected by random factors such as noise. Hence, when analyzing the stability or stabilization of memristor neural networks, it is necessary to consider the effect of these random factors. There are some interesting results. [111] studied memristive neural networks with Markovian jumping and impulses and obtained some criteria of exponential stability in the mean square. [112] discussed a class of stochastic memristive neural networks by using Lyapunov functional and inequality square and presented three sufficient conditions for exponential stability. [113] and [114] solved the exponentially stable in the mean square problem via linear matrix inequalities and intermittent adaptive control, respectively. [115] was concerned with mean square exponential input-to-state stability of stochastic memristive neural networks. [116] studied the stabilization of switched memristive neural networks subject to stochastic disturbance. From the random delay point of view, [117] took advantage of a sequence of Bernoulli distributed random variables and gained the globally exponential stability results. From the random switching topologies point of view, [118] considered memristive neural networks subject to stochastic sensor faults and achieved the asymptotic stability by sampled-data control.
In addition, there are some interesting results involving the potential effect of impulsive signals on the memristive neural networks. According to the effect of impulses on the networks, impulses can be classified into three types: stabilizing impulses, destabilizing impulses and hybrid impulses (combination of stabilizing impulses and destabilizing impulses). From the stabilizing impulses point of view, [119] designed an appropriate impulsive controller to achieve the global exponential stability, which can improve the speed of convergence and reduce the cost of time. [120] studied a class of inertial memristor-based neural networks, where the potential effects of time-delay and impulses on the networks are fully considered. Note that the results in [119,120] are based on the time-triggered mechanism. In terms of the event-triggered mechanism, [121] proposed the event-based impulsive control strategy, where the impulse time sequence is uniquely determined by the designed event-triggered mechanism. These results were applied to synchronization of memristive neural networks. From the destabilizing impulses point of view, [122] constructed a Lyapunov-Krasovskii functional and presented the disturbance suppression condition. From the hybrid impulses point of view, [123] considered stabilizing impulses and destabilizing impulses simultaneously. Based on Lyapunov method, some sufficient conditions of globally exponential stability were obtained.
Due to the diffusion phenomenon of electrons' movement in a nonuniform electromagnetic field, reaction-diffusion neural networks (RDNNs) are put forward and widely investigated. In this section, we will take the system form (e.g., delayed RDNNs, impulsive RDNNs and stochastic RDNNs) and the dynamical behavior (e.g., stability and synchronization) as the main lines to present recent development of RDNNs.
The dynamical analysis of RDNNs is always a hot topic, since it not only casts light on the theoretical mechanism of neural networks but also provides the guide of designing practical neural networks in engineering. Among various dynamical properties of RDNNs, stability and synchronization are fundamental and undoubtedly significant. The synchronization of RDNNs has also successfully been applied to secure communication. In the sequel, recent progress of delayed RDNNs is presented including, the stability and synchronization.
1) Stability. Due to the spatial-temporal complex dynamics in the PDE setting, the stability analysis of RDNNs is quite challenging and attracts the interest of many researchers. In [124], the global exponential stability of recurrent RDNNs with delays and Dirichlet boundary conditions was studied by the Lyapunov function, where the reaction-diffusion has been shown to have a stabilizing effect by a novel Poincaré-type inequality on a cube. Then, the time-varying delays and spatial-temporal output were considered in the model of RDNNs, and robust exponential stability was investigated in [125]. In the circuits of neural networks, the electronic signal transmits in parallel pathways along the length of the axon, and hence the distributed time delays are included in the system of RDNNs. In [126], the distributed time delays were modeled by the Lebesgue-Stieltjes integration of a delay kernel, and the past state was dealt with the truncation method for the globally exponential stability. Then, the global stabilization of fuzzy RDNNs with distributed time delays was achieved by fuzzy feedback controller and fuzzy adaptive controller in [127]. Subsequently, a fuzzy intermittent controller was proposed for the stabilization of uncompensated fuzzy RDNNs to overcome the difficulty caused by unexpected attack [128].
2) Synchronization. The synchronization is one of the most important dynamical properties of neural networks, since it discloses the mechanics of neurons in engineering such as image encryption. To synchronize the delayed RDNNs, plenty of effective control schemes are designed in existing results, such as sampled-data control, pinning control, adaptive control, etc.
Among the various control methods, the sampled-data control has been extensively used to reduce the update rate of controller in the synchronization of delayed RDNNs. In [129], a stochastic sampled-data controller with sampling period satisfying the Bernoulli distribution was proposed for the synchronization of RDNNs with time-varying delays. Then, the output synchronization of RDNNs with distributed time delays was achieved by a spatial sampled-data control which only required the states in partial domain in [35]. [130] considered both the time-varying delays and distributed time delays in RDNNs and designed a quantized sampled-data controller which avoided the overuse of sensors and actuators. For the purpose of communication reduction and energy saving, an event-triggered controller with sampled data was given for the synchronization of RDNNs in [131].
Neural networks comprise massive neuron nodes with nonidentical dynamics. It is impractical to control all the neuron nodes in the implementation of circuits, and thus only partial neuron nodes are controlled for the synchronization of RDNNs. In [132], the sampled-data strategy was designed to control partial nodes for the synchronization of directed RDNNs. Then, two distributed pinning control schemes which only required partial information of pinning neighbors were introduced for the global exponential synchronization of RDNNs with constant delays in [133]. Furthermore, the problem of pinning synchronization of RDNNs with time-varying delays was addressed by adaptive pinning controller which automatically determined the first pinned nodes [134].
When designing the controllers for the synchronization of RDNNs, the controller gain has historically been time-invariant. This may result in the slow convergence of the states when the system is subject to large disturbance. In contrast, the adaptive control can address the issue because of its automatic adjustment. In [135], the authors used the adaptive controller to synchronize the RDNNs with time-varying delays and successfully applied the synchronized systems to image encryption. Then, the adaptive synchronization of RDNNs with multiple time delays was achieved in [136]. Recently, the adaptive controller was also designed based on event-triggered mechanism for the synchronization of RDNNs with random time-varying delays [137].
In the circuits of neural networks, the abrupt changes and instantaneous disturbances are inevitable and modeled as impulse actions. The impulses are subsequently introduced in RDNNs, and the dynamical behavior, including stability and synchronization, of impulsive RDNNs is extensively investigated in recent literature.
1) Stability. Impulsive RDNNs, as a subclass of discontinuous neural networks, consist of continuous dynamics and discrete dynamics. The continuous dynamics is determined by the differential equation with spatial derivative, whereas the discrete dynamics is depicted by the impulsive measurement and impulsive triggered mechanism. According to the effect of impulses for the dynamics of the system, the impulsive systems can be roughly classified into two categories: the systems with impulsive disturbance and impulsive control. In the stability of impulsive RDNNs, the impulses are usually regarded as the disturbance. Thus, the stability issue of impulsive RDNNs with time-varying delays was dealt with in [138]. In [139], the parameter uncertainty was considered in the impulsive RDNNs, and several robust mean square stability criteria were given by Lyapunov functional. Then, the exponential stability of stochastic impulsive RDNNs with distributed time delays was established by a novel impulsive inequality involving infinite delay in [140], which was extended into the scenario of input-to-state stability in [141]. The impulsive RDNNs was further considered in fractional order, and the criteria ensuring the stability of almost periodic solutions were derived in [142]. Recently, the RDNNs were also proven to be robust against hybrid impulsive disturbance, and a novel vector Halanay-type inequality was given to handle the hybrid impulses for the exponential stability of impulsive RDNNs [143].
2) Impulsive synchronization. As stated above, the impulse action sometimes acts as the control factor in impulsive systems, which inspires the impulsive synchronization of RDNNs. The impulsive control advances in the aspect of source saving and disturbance rejection and hence attracts the attention of scholars. In [144], the synchronization of RDNNs with delays was achieved by impulsive controller. Then, the authors of [145] considered the pinning problem of RDNNs and designed a pinning-impulsive controller for the global exponential synchronization. Furthermore, the impulsive control was also used for the synchronization of RDNNs with unbounded distributed time delays in [146], where a novel Wirtinger-type inequality and an impulse-dependent Lyapunov function were proposed to capture the characteristics of the continuous and discrete dynamics. In addition, the impulsive control and pinning impulsive control successfully synchronized the RDNNs with stochastic disturbance involving temporal and spatial feature [147].
The external disturbance, parameter uncertainty, and unexpected attack in neural networks embody the random characteristics. This results in the models of RDNNs driven by stochastic processes such as Markov jump, Brownian motion, infinite dimensional Wiener process, etc. The involvement of stochastic factors will bring essential influence to the dynamical behavior of RDNNs. For instance, the stochastic factor can destabilize the system from the viewpoint of disturbance, and they can also stabilize an unstable deterministic system from the viewpoint of control. The two-sided effect of stochastic factor in RDNNs is attractive and consequently studied in recent literature.
1) Stability. According to the stochastic processes, there are three types of stochastic RDNNs: Markovian RDNNs, RDNNs with Brownian motion, and RDNNs with infinite dimensional Wiener process. The Markovian RDNNs model the RDNNs whose structures suffer random failure governed by Markovian chain. The stability of Markovian RDNNs was established under the condition that the parameters satisfied the matrix inequality related to the transition rate [148]. Then, an adaptive event-triggered scheme was designed for the stabilization of Markovian RDNNs to lower the communication and save energy in [149]. Different from the conventional control input in the state's evolution, [150] proposed an asynchronous control strategy to stabilize the Markovian RDNNs with input in the boundary.
The stochastic RDNNs driven by Brownian motion model the RDNNs subject to time-dependent random noise. The exponential stability in the mean square of such networks was established under the requirement that the diffusion strength of the Brownian noise was not very strong [151]. [152] considered the stochastic RDNNs with infinite time delays, which consist of infinite discrete delays and infinite distributed delays, and presented useful criteria for -type stability. The stability of stochastic RDNNs with distributed time delays can also be ensured by boundary control with low spatial cost [153].
Recently, the spatial stochastic disturbance is considered in the dynamical analysis of RDNNs, since random noise has spatial feature when the electrons move in the nonuniform electromagnetic field. Therefore, many researchers pay attention to the stability analysis of stochastic RDNNs driven by infinite dimensional Wiener process. In [154], the properties of the mild solution of stochastic RDNNs were studied by transforming the network system into an abstract stochastic differential equation in Hilbert space based on semigroup theory. Then, the nonautonomous stochastic reaction-diffusion neural-network models with S-type distributed delays were modeled as nonautonomous stochastic differential equation with infinite delay by evolution system theory, and some easy-to-test conditions were given for the stability of such networks in [155]. Recently, the impulses were also proven to generate the periodic solution and stabilize the mild solution in finite-time sense of stochastic RDNNs in [156].
2) Synchronization. To synchronize the stochastic RDNNs, various controls are input in the network system such as state feedback control, impulsive control, sampled-data control, etc. In [157], a linear feedback controller synchronized the stochastic RDNNs with time-varying delays in the mean-square sense. Then, the mean square exponential stability of stochastic RDNNs with distributed time delays was achieved by impulsive control, which greatly reduced the update rate of control input [158]. In addition, the update rate was also reduced by the sampled-data controller in the synchronization of stochastic RDNNs with time-varying delays in [159]. The authors of [160] considered the limitation of bandwidth and proposed a quantized control scheme to significantly save communication resources in synchronization of stochastic RDNNs. Recently, the finite-time synchronization of stochastic RDNNs was also achieved by a nonfragile time-varying proportional retarded strategy in [161]. Different from previous results, [162] focused on the stochastic RDNNs with multiple disturbances and synchronized the networks by a robust composite technique based on disturbance observer.
There are currently two paths for artificial intelligence to generate literary works. One is the top-down structuralism approach, that is, people program some literary laws, pre-place some content outline and character settings into the writing program, and then artificial intelligence automatically generates literature. An American scholar applies this approach to an automatic script writing program "Universe." The other is the bottom-up functionalist approach, which involves the production of simulated works by inputting large amounts of literary text and having the machine process the text through deep learning techniques, such as some artificial intelligence programs called "Microsoft Xiaobing, " "Tsinghua Jiuge" and so on. One can observe from the generation type of artificial intelligence literature that human literature is the imitative object and learning material of artificial intelligence literature. Therefore, the reference standard to judge the quality of artificial intelligence literature also comes from human literature. However, to evaluate the current artificial intelligence literature by the standard of human literature, the latter is still immature and even failed. From the perspective of ancient Chinese literary theory, the fundamental reason why artificial intelligence literature cannot match human literature is that it has only "machine chip" but no "literary heart."
The machine writing lies in the machine chip that refers to the core part of a machine, which generally includes both hardware and software. Hardware refers to the artificial intelligence chip, and software refers to the program in the chip. The chip is equivalent to the brain of artificial intelligence, which realizes target tasks through algorithms, programs and instructions. However, it does not have the function of independent cognition and emotional activities and does not have subjective spirit and consciousness. It can only simulate human emotions and imagination through coding, calculation and programs. The artificial intelligence literature created by the "machine chip" does not have the spiritual thinking process and temperament sustenance generated by the "literary heart" of human literature. This determines the disadvantage of the current artificial intelligence literature.
Artificial neural networks are inspired by the human nervous system, and the human mind can be regarded as a big black box. Different from artificial intelligence, human beings, as "heart agents, " can sense the relationship between their own actions and "heart" and will try to figure out their own mind, with spontaneous introspection of their own behavior and rules. This is reflected in the field of literature, which is to explore the creative motivation and law of the creative subject. Some scholars focus on human neural networks and attempt to simulate the conditions of emotion. Emotion is a product of a more developed limbic system in the cerebral cortex. The occurrence of emotion should be related to human neurobiochemical mechanism. The mechanism has yet to be uncovered, but scientists have not given up on the path. For example, the program MINDER, which led development in the 1990s, built a model that produced feelings of unease and anxiety, which tries to use programs to mimic human emotions. If artificial intelligence is always based on human brain, with the further development of science and technology, as human beings have more understanding of the thinking mechanism and emotional mechanism of the brain, artificial intelligence may be able to imitate the biochemical mechanism of human emotions and have emotional experience.
Therefore, how to use artificial intelligence machines and develop artificial intelligence literature on the basis of maintaining literary heart and humanity and how to realize human-machine cooperation on the basis of human-machine harmony are urgent problems to be solved at present. We should attach importance to the enlightening and supporting role of artificial intelligence in the field of literature. First of all, it can take advantage of its "extensive view" to act as the intermediary of human literature creation, playing a role in the field of material collection, picture screening, manuscript polishing, literary publicity and so on. The current achievements of artificial intelligence literature are the result of man-machine collaboration, or the outline set by man is described by the machine, or the draft generated by the machine is modified by man, or the man-machine is assisted by the machine, or the machine provides materials and ideas. Now, mature artificial intelligence writing assistants include Give Me Sport, Google, News Cart and so on. The second is a supplementary standard evaluation process for human texts. Artificial intelligence is in some ways better suited to standard evaluation than human beings, because in an ideal state, artificial intelligence has no emotion, as long as the literary texts of different kinds and times are placed in equal numbers, and discriminatory discourse is excluded. In addition, the strengthening of big data technology and computing power has improved the efficiency of artificial intelligence learning. Artificial intelligence far more easily than human beings achieves "extensive view." Third, the development of artificial intelligence literature can stimulate human imagination and the ability of literary criticism. The literary value of artificial intelligence literature lies more in readers' interpretation of it. The process in which readers seem to read "meaning" and "revelation" from obscure or unstructured artificial intelligence literature is a process in which readers participate in re-creation. Human beings can make up for the lack of emotion in artificial intelligence literature with their powerful ability of interpretation and association, and the sentences written by artificial intelligence that break through grammatical inertia or taboos also give new inspiration to human literature creation. Finally, it can help human beings improve the quality of literature and promote the innovation of literature. The style of network literature which provides people with cultural fast food is similar to that of we-media articles, and the writing pattern is serious. Artificial intelligence is good at imitating the article creation with routine, and the author using routine writing is replaced. Therefore, the human writer must be careful to create the content of the text and even find a way to develop a more advanced literary form. When readers are tired of reading similar sets of works repeatedly recommended by artificial intelligence, they will have aesthetic fatigue and will inevitably jump out of the cocoon room of knowledge and seek the edification of high-quality literary works.
If we judge artificial intelligence literature by the aesthetic standard of ancient Chinese literary theory, we will find many defects. It is still premature to worry that human literature will be replaced by artificial intelligence literature in the current situation where artificial intelligence is still weak, that is, artificial intelligence cannot really have emotions and mind. However, with the continuous improvement of artificial intelligence writing technology, artificial intelligence more deeply involved in human literary and artistic creation may lead to completely different effects. On the positive side, as artificial intelligence expands the field of literature, literature will become richer, and its boundaries will continue to expand. Moreover, artificial intelligence can easily produce more works, especially in some special types of writing such as official documents, reducing the burden of workers. However, on the negative side, artificial intelligence will cause some legal, ethical and social problems in the field of literature, such as privacy, copyright and intellectual property rights. It will also cause trust problems between human beings. When people read literary works, they need to first identify whether it's a human or a machine. What's more, when artificial intelligence can replace human to generate art works, one of the most important essential activities of human–literary and artistic activities may shrink or even disappear. Then, we may be living in a sea of machine text and be controlled by artificial intelligence from the mind and culture, and this is worth thinking about.
This paper briefly introduces the development history of neural networks, and it emphatically introduces the synchronization, the periodic solution, the stability/stabilization of neural networks, and creation psychology. This paper points out that neural networks are of great significance for solving large and complex data problems, and they are also widely used in various fields such as medicine and industry. However, neural networks are not universal, that is, according to different problems, one needs to adjust parameters, weights, the number of hidden layers and other methods to train a new model suitable for the problem. In future work, the model of neural networks can be improved according to the needs of specific problems, or even a new model can be proposed, so as to truly realize the grand goal of replacing "brain" with "machine."
The author declares there are no conflicts of interest.
[1] | G. Rajchakit, P. Agarwal, S. Ramalingam, Stability Analysis of Neural Networks, Springer, 2022. https://doi.org/10.1007/978-981-16-6534-9 |
[2] |
G. Rajchakit, R. Sriraman, N. Boonsatit, P. Hammachukiattikul, C. P. Lim, P. Agarwal, Global exponential stability of clifford-valued neural networks with time-varying delays and impulsive effects, Adv. Differ. Equations, 2021 (2021), 1–21. https://doi.org/10.1186/s13662-021-03367-z doi: 10.1186/s13662-021-03367-z
![]() |
[3] |
N. Boonsatit, G. Rajchakit, R. Sriraman, C. P. Lim, P. Agarwal, Finite-/fixed-time synchronization of delayed clifford-valued recurrent neural networks, Adv. Differ. Equations, 2021 (2021), 1–25. https://doi.org/10.1186/s13662-021-03438-1 doi: 10.1186/s13662-021-03438-1
![]() |
[4] |
W. S. McCulloch, W. Pitts, A logical calculus of the ideas immanent in nervous activity, Bull. Math. Biophys., 5 (1943), 115–133. https://doi.org/10.1007/BF02478259 doi: 10.1007/BF02478259
![]() |
[5] | D. O. Hebb, The Organization of Behavior: A Neuropsychological Theory, Psychology Press, 2005. https://doi.org/10.4324/9781410612403 |
[6] |
F. Rosenblatt, The perceptron: a probabilistic model for information storage and organization in the brain, Psychol. Rev., 65 (1958), 386–408. https://doi.org/10.1037/h0042519 doi: 10.1037/h0042519
![]() |
[7] | M. Minsky, S. Papert, An introduction to computational geometry, Cambridge tiass., HIT, 479 (1969), 480. |
[8] |
J. J. Hopfield, Neurons with graded response have collective computational properties like those of two-state neurons, PNAS, 81 (1984), 3088–3092. https://doi.org/10.1073/pnas.81.10.3088 doi: 10.1073/pnas.81.10.3088
![]() |
[9] |
D. E. Rumelhart, G. E. Hinton, R. J. Williams, Learning representations by back-propagating errors, Nature, 323 (1986), 533–536. https://doi.org/10.1038/323533a0 doi: 10.1038/323533a0
![]() |
[10] |
L. O. Chua, L. Yang, Cellular neural networks: Theory, IEEE Trans. Circuits Syst., 35 (1988), 1257–1272. https://doi.org/10.1109/31.7600 doi: 10.1109/31.7600
![]() |
[11] |
Y. LeCun, L. Bottou, Y. Bengio, P. Haffner, Gradient-based learning applied to document recognition, Proc. IEEE, 86 (1998), 2278–2324. https://doi.org/10.1109/5.726791 doi: 10.1109/5.726791
![]() |
[12] |
G. E. Hinton, S. Osindero, Y. W. Teh, A fast learning algorithm for deep belief nets, Neural Comput., 18 (2006), 1527–1554. https://doi.org/10.1162/neco.2006.18.7.1527 doi: 10.1162/neco.2006.18.7.1527
![]() |
[13] |
H. Lin, C. Wang, Y. Sun, T. Wang, Generating-scroll chaotic attractors from a memristor-based magnetized hopfield neural network, IEEE Trans. Circuits Syst. II Express Briefs, 70 (2022), 311–315. https://doi.org/10.1109/TCSII.2022.3212394 doi: 10.1109/TCSII.2022.3212394
![]() |
[14] |
H. Liu, L. Ma, Z. Wang, Y. Liu, F. E. Alsaadi, An overview of stability analysis and state estimation for memristive neural networks, Neurocomputing, 391 (2020), 1–12. https://doi.org/10.1016/j.neucom.2020.01.066 doi: 10.1016/j.neucom.2020.01.066
![]() |
[15] |
Z. Zeng, D. S. Huang, Z. Wang, Pattern memory analysis based on stability theory of cellular neural networks, Appl. Math. Modell., 32 (2008), 112–121. https://doi.org/10.1016/j.apm.2006.11.010 doi: 10.1016/j.apm.2006.11.010
![]() |
[16] |
Z. Wang, S. Joshi, S. Savel'ev, W. Song, R. Midya, Y. Li, et al., Fully memristive neural networks for pattern classification with unsupervised learning, Nat. Electron., 1 (2018), 137–145. https://doi.org/10.1038/s41928-018-0023-2 doi: 10.1038/s41928-018-0023-2
![]() |
[17] |
C. Tsioustas, P. Bousoulas, J. Hadfield, T. P. Chatzinikolaou, I. A. Fyrigos, V. Ntinas, et al., Simulation of low power self-selective memristive neural networks for in situ digital and analogue artificial neural network applications, IEEE Trans. Nanotechnol., 21 (2022), 505–513. https://doi.org/10.1109/TNANO.2022.3205698 doi: 10.1109/TNANO.2022.3205698
![]() |
[18] |
B. Seyfi, A. Rassoli, M. Imeni Markhali, N. Fatouraee, Characterization of the nonlinear biaxial mechanical behavior of human ureter using constitutive modeling and artificial neural networks, J. Appl. Comput. Mech., 8 (2022), 1186–1195. https://doi.org/10.22055/JACM.2020.33703.2272 doi: 10.22055/JACM.2020.33703.2272
![]() |
[19] |
M. Aliasghary, H. Mobki, H. M. Ouakad, Pull-in phenomenon in the electrostatically micro-switch suspended between two conductive plates using the artificial neural network, J. Appl. Comput. Mech., 8 (2022), 1222–1235. https://doi.org/10.22055/JACM.2021.38569.3248 doi: 10.22055/JACM.2021.38569.3248
![]() |
[20] |
H. Guo, J. Zhang, Y. Zhao, H. Zhang, J. Zhao, X. Yang, et al., Accelerated key distribution method for endogenously secure optical communication by synchronized chaotic system based on fiber channel feature, Opt. Fiber Technol., 75 (2023), 103162. https://doi.org/10.1016/j.yofte.2022.103162 doi: 10.1016/j.yofte.2022.103162
![]() |
[21] |
C. Zhou, C. Wang, W. Yao, H. Lin, Observer-based synchronization of memristive neural networks under dos attacks and actuator saturation and its application to image encryption, Appl. Math. Comput., 425 (2022), 127080. https://doi.org/10.1016/j.amc.2022.127080 doi: 10.1016/j.amc.2022.127080
![]() |
[22] |
H. L. Li, C. Hu, L. Zhang, H. Jiang, J. Cao, Complete and finite-time synchronization of fractional-order fuzzy neural networks via nonlinear feedback control, Fuzzy Sets Syst., 443 (2022), 50–69. https://doi.org/10.1016/j.fss.2021.11.004 doi: 10.1016/j.fss.2021.11.004
![]() |
[23] |
W. Chen, Y. Yu, X. Hai, G. Ren, Adaptive quasi-synchronization control of heterogeneous fractional-order coupled neural networks with reaction-diffusion, Appl. Math. Comput., 427 (2022), 127145. https://doi.org/10.1016/j.amc.2022.127145 doi: 10.1016/j.amc.2022.127145
![]() |
[24] |
Y. Shen, X. Liu, Generalized synchronization of delayed complex-valued dynamical networks via hybrid control, Commun. Nonlinear Sci. Numer. Simul., 118 (2023), 107057. https://doi.org/10.1016/j.cnsns.2022.107057 doi: 10.1016/j.cnsns.2022.107057
![]() |
[25] |
A. Abdurahman, M. Abudusaimaiti, H. Jiang, Fixed/predefined-time lag synchronization of complex-valued bam neural networks with stochastic perturbations, Appl. Math. Comput., 444 (2023), 127811. https://doi.org/10.1016/j.amc.2022.127811 doi: 10.1016/j.amc.2022.127811
![]() |
[26] |
H. Pu, F. Li, Fixed-time projective synchronization of delayed memristive neural networks via aperiodically semi-intermittent switching control, ISA Trans., 133 (2023), 302–316. https://doi.org/10.1016/j.isatra.2022.07.022 doi: 10.1016/j.isatra.2022.07.022
![]() |
[27] |
J. Luo, S. Qu, Y. Chen, X. Chen, Z. Xiong, Synchronization, circuit and secure communication implementation of a memristor-based hyperchaotic system using single input controller, Chin. J. Phys., 71 (2021), 403–417. https://doi.org/10.1016/j.cjph.2021.03.009 doi: 10.1016/j.cjph.2021.03.009
![]() |
[28] |
V. L. Freitas, S. Yanchuk, M. Zaks, E. E. Macau, Synchronization-based symmetric circular formations of mobile agents and the generation of chaotic trajectories, Commun. Nonlinear Sci. Numer. Simul., 94 (2021), 105543. https://doi.org/10.1016/j.cnsns.2020.105543 doi: 10.1016/j.cnsns.2020.105543
![]() |
[29] |
J. Xiang, J. Ren, M. Tan, Asymptotical synchronization for complex-valued stochastic switched neural networks under the sampled-data controller via a switching law, Neurocomputing, 514 (2022), 414–425. https://doi.org/10.1016/j.neucom.2022.09.152 doi: 10.1016/j.neucom.2022.09.152
![]() |
[30] |
Z. Dong, X. Wang, X. Zhang, M. Hu, T. N. Dinh, Global exponential synchronization of discrete-time high-order switched neural networks and its application to multi-channel audio encryption, Nonlinear Anal. Hybrid Syst., 47 (2023), 101291. https://doi.org/10.1016/j.nahs.2022.101291 doi: 10.1016/j.nahs.2022.101291
![]() |
[31] | S. Gong, Z. Guo, S. Wen, Finite-time synchronization of T-S fuzzy memristive neural networks with time delay, Fuzzy Sets Syst., In press. https://doi.org/10.1016/j.fss.2022.10.013 |
[32] |
C. Zhou, C. Wang, Y. Sun, W. Yao, H. Lin, Cluster output synchronization for memristive neural networks, Inf. Sci., 589 (2022), 459–477. https://doi.org/10.1016/j.ins.2021.12.084 doi: 10.1016/j.ins.2021.12.084
![]() |
[33] |
K. Subramanian, P. Muthukumar, S. Lakshmanan, State feedback synchronization control of impulsive neural networks with mixed delays and linear fractional uncertainties, Appl. Math. Comput., 321 (2018), 267–281. https://doi.org/10.1016/j.amc.2017.10.038 doi: 10.1016/j.amc.2017.10.038
![]() |
[34] |
X. Li, W. Zhang, J. Fang, H. Li, Finite-time synchronization of memristive neural networks with discontinuous activation functions and mixed time-varying delays, Neurocomputing, 340 (2019), 99–109. https://doi.org/10.1016/j.neucom.2019.02.051 doi: 10.1016/j.neucom.2019.02.051
![]() |
[35] |
B. Lu, H. Jiang, C. Hu, A. Abdurahman, Spacial sampled-data control for output synchronization of directed coupled reaction-diffusion neural networks with mixed delays, Neural Networks, 123 (2020), 429–440. https://doi.org/10.1016/j.neunet.2019.12.026 doi: 10.1016/j.neunet.2019.12.026
![]() |
[36] |
W. Tai, Q. Teng, Y. Zhou, J. Zhou, Z. Wang, Chaos synchronization of stochastic reaction-diffusion time-delay neural networks via non-fragile output-feedback control, Appl. Math. Comput., 354 (2019), 115–127. https://doi.org/10.1016/j.amc.2019.02.028 doi: 10.1016/j.amc.2019.02.028
![]() |
[37] |
A. Kazemy, R. Saravanakumar, J. Lam, Master-slave synchronization of neural networks subject to mixed-type communication attacks, Inf. Sci., 560 (2021), 20–34. https://doi.org/10.1016/j.ins.2021.01.063 doi: 10.1016/j.ins.2021.01.063
![]() |
[38] |
W. Zhang, S. Yang, C. Li, W. Zhang, X. Yang, Stochastic exponential synchronization of memristive neural networks with time-varying delays via quantized control, Neural Networks, 104 (2018), 93–103. https://doi.org/10.1016/j.neunet.2018.04.010 doi: 10.1016/j.neunet.2018.04.010
![]() |
[39] |
X. Yang, Z. Cheng, X. Li, T. Ma, Exponential synchronization of coupled neutral-type neural networks with mixed delays via quantized output control, J. Franklin Inst., 356 (2019), 8138–8153. https://doi.org/10.1016/j.jfranklin.2019.07.006 doi: 10.1016/j.jfranklin.2019.07.006
![]() |
[40] |
R. Tang, X. Yang, X. Wan, Y. Zou, Z. Cheng, H. M. Fardoun, Finite-time synchronization of nonidentical bam discontinuous fuzzy neural networks with delays and impulsive effects via non-chattering quantized control, Commun. Nonlinear Sci. Numer. Simul., 78 (2019), 104893. https://doi.org/10.1016/j.cnsns.2019.104893 doi: 10.1016/j.cnsns.2019.104893
![]() |
[41] |
M. Xu, J. L. Wang, P. C. Wei, Synchronization for coupled reaction-diffusion neural networks with and without multiple time-varying delays via pinning-control, Neurocomputing, 227 (2017), 82–91. https://doi.org/10.1016/j.neucom.2016.10.063 doi: 10.1016/j.neucom.2016.10.063
![]() |
[42] |
Y. Li, B. Luo, D. Liu, Z. Yang, Robust synchronization of memristive neural networks with strong mismatch characteristics via pinning control, Neurocomputing, 289 (2018), 144–154. https://doi.org/10.1016/j.neucom.2018.02.006 doi: 10.1016/j.neucom.2018.02.006
![]() |
[43] |
Q. Tang, J. Jian, Exponential synchronization of inertial neural networks with mixed time-varying delays via periodically intermittent control, Neurocomputing, 338 (2019), 181–190. https://doi.org/10.1016/j.neucom.2019.01.096 doi: 10.1016/j.neucom.2019.01.096
![]() |
[44] |
S. Cai, X. Li, P. Zhou, J. Shen, Aperiodic intermittent pinning control for exponential synchronization of memristive neural networks with time-varying delays, Neurocomputing, 332 (2019), 249–258. https://doi.org/10.1016/j.neucom.2018.12.070 doi: 10.1016/j.neucom.2018.12.070
![]() |
[45] |
Y. Yang, Y. He, M. Wu, Intermittent control strategy for synchronization of fractional-order neural networks via piecewise lyapunov function method, J. Franklin Inst., 356 (2019), 4648–4676. https://doi.org/10.1016/j.jfranklin.2018.12.020 doi: 10.1016/j.jfranklin.2018.12.020
![]() |
[46] |
H. A. Tang, S. Duan, X. Hu, L. Wang, Passivity and synchronization of coupled reaction-cdiffusion neural networks with multiple time-varying delays via impulsive control, Neurocomputing, 318 (2018), 30–42. https://doi.org/10.1016/j.neucom.2018.08.005 doi: 10.1016/j.neucom.2018.08.005
![]() |
[47] |
Z. Xu, D. Peng, X. Li, Synchronization of chaotic neural networks with time delay via distributed delayed impulsive control, Neural Networks, 118 (2019), 332–337. https://doi.org/10.1016/j.neunet.2019.07.002 doi: 10.1016/j.neunet.2019.07.002
![]() |
[48] |
M. Li, X. Li, X. Han, J. Qiu, Leader-following synchronization of coupled time-delay neural networks via delayed impulsive control, Neurocomputing, 357 (2019), 101–107. https://doi.org/10.1016/j.neucom.2019.04.063 doi: 10.1016/j.neucom.2019.04.063
![]() |
[49] |
S. Wu, X. Li, Y. Ding, Saturated impulsive control for synchronization of coupled delayed neural networks, Neural Networks, 141 (2021), 261–269. https://doi.org/10.1016/j.neunet.2021.04.012 doi: 10.1016/j.neunet.2021.04.012
![]() |
[50] |
Y. Zhou, H. Zhang, Z. Zeng, Synchronization of memristive neural networks with unknown parameters via event-triggered adaptive control, Neural Networks, 139 (2021), 255–264. https://doi.org/10.1016/j.neunet.2021.02.029 doi: 10.1016/j.neunet.2021.02.029
![]() |
[51] |
A. Kazemy, J. Lam, X. M. Zhang, Event-triggered output feedback synchronization of master-slave neural networks under deception attacks, IEEE Trans. Neural Networks Learn. Syst., 33 (2022), 952–961. https://doi.org/10.1109/TNNLS.2020.3030638 doi: 10.1109/TNNLS.2020.3030638
![]() |
[52] |
X. Li, D. Peng, J. Cao, Lyapunov stability for impulsive systems via event-triggered impulsive control, IEEE Trans. Autom. Control, 65 (2020), 4908–4913. https://doi.org/10.1109/TAC.2020.2964558 doi: 10.1109/TAC.2020.2964558
![]() |
[53] |
M. Wang, X. Li, P. Duan, Event-triggered delayed impulsive control for nonlinear systems with application to complex neural networks, Neural Networks, 150 (2022), 213–221. https://doi.org/10.1016/j.neunet.2022.03.007 doi: 10.1016/j.neunet.2022.03.007
![]() |
[54] |
Y. Fang, T. G. Kincaid, Stability analysis of dynamical neural networks, IEEE Trans. Neural Networks, 7 (1996), 996–1006. https://doi.org/10.1109/72.508941 doi: 10.1109/72.508941
![]() |
[55] |
K. A. Smith, Neural networks for combinatorial optimization: a review of more than a decade of research, Informs J. Comput., 11 (1999), 15–34. https://doi.org/10.1287/ijoc.11.1.15 doi: 10.1287/ijoc.11.1.15
![]() |
[56] |
T. Zhang, J. Zhou, Y. Liao, Exponentially stable periodic oscillation and mittag-leffler stabilization for fractional-order impulsive control neural networks with piecewise caputo derivatives, IEEE Trans. Cybern., 52 (2022), 9670–9683. https://doi.org/10.1109/TCYB.2021.3054946 doi: 10.1109/TCYB.2021.3054946
![]() |
[57] | E. N. Lorenz, The mechanics of vacillation, J. Atmos. Sci., 20 (1963), 448–465. https://doi.org/10.1175/1520-0469(1963)020 < 0448: TMOV > 2.0.CO; 2 |
[58] | K. Aihara, T. Takabe, M. Toyoda, Chaotic neural networks, Phys. Lett. A, 144 (1990), 333–340. https://doi.org/10.1016/0375-9601(90)90136-C |
[59] |
H. Lin, C. Wang, Q. Deng, C. Xu, Z. Deng, C. Zhou, Review on chaotic dynamics of memristive neuron and neural network, Nonlinear Dyn., 106 (2021), 959–973. https://doi.org/10.1007/s11071-021-06853-x doi: 10.1007/s11071-021-06853-x
![]() |
[60] | T. Yoshizawa, Stability Theory and the Existence of Periodic Solutions and Almost Periodic Solutions, Springer Science & Business Media, 2012. |
[61] |
Y. Li, X. Wang, Almost periodic solutions in distribution of clifford-valued stochastic recurrent neural networks with time-varying delays, Chaos, Solitons Fractals, 153 (2021), 111536. https://doi.org/10.1016/j.chaos.2021.111536 doi: 10.1016/j.chaos.2021.111536
![]() |
[62] |
B. Kosko, Adaptive bidirectional associative memories, Appl. Opt., 26 (1987), 4947–4960. https://doi.org/10.1364/AO.26.004947 doi: 10.1364/AO.26.004947
![]() |
[63] |
J. Cao, New results concerning exponential stability and periodic solutions of delayed cellular neural networks, Phys. Lett. A, 307 (2003), 136–147. https://doi.org/10.1016/S0375-9601(02)01720-6 doi: 10.1016/S0375-9601(02)01720-6
![]() |
[64] |
J. Cao, J. Wang, Global asymptotic stability of a general class of recurrent neural networks with time-varying delays, IEEE Trans. Circuits Syst. I Fundam. Theory Appl., 50 (2003), 34–44. https://doi.org/10.1109/TCSI.2002.807494 doi: 10.1109/TCSI.2002.807494
![]() |
[65] |
D. Li, Z. Zhang, X. Zhang, Periodic solutions of discrete-time quaternion-valued bam neural networks, Chaos, Solitons Fractals, 138 (2020), 110144. https://doi.org/10.1016/j.chaos.2020.110144 doi: 10.1016/j.chaos.2020.110144
![]() |
[66] |
H. R. Wilson, J. D. Cowan, Excitatory and inhibitory interactions in localized populations of model neurons, Biophys. J., 12 (1972), 1–24. https://doi.org/10.1016/S0006-3495(72)86068-5 doi: 10.1016/S0006-3495(72)86068-5
![]() |
[67] |
R. Decker, V. W. Noonburg, A periodically forced wilson–cowan system with multiple attractors, SIAM J. Math. Anal., 44 (2012), 887–905. https://doi.org/10.1137/110823365 doi: 10.1137/110823365
![]() |
[68] |
B. Pollina, D. Benardete, V. W. Noonburg, A periodically forced wilson–cowan system, SIAM J. Appl. Math., 63 (2003), 1585–1603. https://doi.org/10.1137/S003613990240814X doi: 10.1137/S003613990240814X
![]() |
[69] |
V. Painchaud, N. Doyon, P. Desrosiers, Beyond wilson-cowan dynamics: oscillations and chaos without inhibition, Biol. Cybern., 116 (2022), 527–543. https://doi.org/10.1007/s00422-022-00941-w doi: 10.1007/s00422-022-00941-w
![]() |
[70] |
J. Cao, Global exponential stability and periodic solutions of delayed cellular neural networks, J. Comput. Syst. Sci., 60 (2000), 38–46. https://doi.org/10.1006/jcss.1999.1658 doi: 10.1006/jcss.1999.1658
![]() |
[71] |
S. Arik, V. Tavsanoglu, On the global asymptotic stability of delayed cellular neural networks, IEEE Trans. Circuits Syst. I Fundam. Theory Appl., 47 (2000), 571–574. https://doi.org/10.1109/81.841859 doi: 10.1109/81.841859
![]() |
[72] |
Y. Li, J. Qin, Existence and global exponential stability of periodic solutions for quaternion-valued cellular neural networks with time-varying delays, Neurocomputing, 292 (2018), 91–103. https://doi.org/10.1016/j.neucom.2018.02.077 doi: 10.1016/j.neucom.2018.02.077
![]() |
[73] |
D. Békollè, K. Ezzinbi, S. Fatajou, D. E. H. Danga, F. M. Béssémè, Attractiveness of pseudo almost periodic solutions for delayed cellular neural networks in the context of measure theory, Neurocomputing, 435 (2021), 253–263. https://doi.org/10.1016/j.neucom.2020.12.047 doi: 10.1016/j.neucom.2020.12.047
![]() |
[74] |
A. Chen, L. Huang, J. Cao, Existence and stability of almost periodic solution for bam neural networks with delays, Appl. Math. Comput., 137 (2003), 177–193. https://doi.org/10.1016/S0096-3003(02)00095-4 doi: 10.1016/S0096-3003(02)00095-4
![]() |
[75] |
Q. Jiang, Q. R. Wang, Almost periodic solutions for quaternion-valued neural networks with mixed delays on time scales, Neurocomputing, 439 (2021), 363–373. https://doi.org/10.1016/j.neucom.2020.09.063 doi: 10.1016/j.neucom.2020.09.063
![]() |
[76] |
L. Pan, J. Cao, Anti-periodic solution for delayed cellular neural networks with impulsive effects, Nonlinear Anal. Real World Appl., 12 (2011), 3014–3027. https://doi.org/10.1016/j.nonrwa.2011.05.002 doi: 10.1016/j.nonrwa.2011.05.002
![]() |
[77] |
C. Ou, Anti-periodic solutions for high-order hopfield neural networks, Comput. Math. Appl., 56 (2008), 1838–1844. https://doi.org/10.1016/j.camwa.2008.04.029 doi: 10.1016/j.camwa.2008.04.029
![]() |
[78] |
L. Chua, Memristor-the missing circuit element, IEEE Trans. Circuit Theory, 18 (1971), 507–519. https://doi.org/10.1109/TCT.1971.1083337 doi: 10.1109/TCT.1971.1083337
![]() |
[79] | L. S. Zhang, Y. C. Jin, Y. D. Song, An overview of dynamics analysis and control of memristive neural networks with delays, Acta Autom. Sin., 47 (2021), 765–779. |
[80] |
M. Liao, C. Wang, Y. Sun, H. Lin, C. Xu, Memristor-based affective associative memory neural network circuit with emotional gradual processes, Neural Comput. Appl., 34 (2022), 13667–13682. https://doi.org/10.1007/s00521-022-07170-z doi: 10.1007/s00521-022-07170-z
![]() |
[81] | Z. Deng, C. Wang, H. Lin, Y. Sun, A memristive spiking neural network circuit with selective supervised attention algorithm, IEEE Trans. Comput. Aided Des. Integr. Circuits Syst., Early Access, 2022. https://doi.org/10.1109/TCAD.2022.3228896 |
[82] |
H. Lin, C. Wang, C. Xu, X. Zhang, H. H. Iu, A memristive synapse control method to generate diversified multi-structure chaotic attractors, IEEE Trans. Comput. Aided Des. Integr. Circuits Syst., 42 (2023), 942–955. https://doi.org/10.1109/TCAD.2022.3186516 doi: 10.1109/TCAD.2022.3186516
![]() |
[83] |
H. Lin, C. Wang, L. Cui, Y. Sun, X. Zhang, W. Yao, Hyperchaotic memristive ring neural network and application in medical image encryption, Nonlinear Dyn., 110 (2022), 841–855. https://doi.org/10.1007/s11071-022-07630-0 doi: 10.1007/s11071-022-07630-0
![]() |
[84] |
Z. Wen, C. Wang, Q. Deng, H. Lin, Regulating memristive neuronal dynamical properties via excitatory or inhibitory magnetic field coupling, Nonlinear Dyn., 110 (2022), 1–13. https://doi.org/10.1007/s11071-022-07813-9 doi: 10.1007/s11071-022-07813-9
![]() |
[85] |
Z. Guo, J. Wang, Z. Yan, Attractivity analysis of memristor-based cellular neural networks with time-varying delays, IEEE Trans. Neural Networks Learn. Syst., 25 (2013), 704–717. https://doi.org/10.1109/TNNLS.2013.2280556 doi: 10.1109/TNNLS.2013.2280556
![]() |
[86] |
L. Wang, Y. Shen, Finite-time stabilizability and instabilizability of delayed memristive neural networks with nonlinear discontinuous controller, IEEE Trans. Neural Networks Learn. Syst., 26 (2015), 2914–2924. https://doi.org/10.1109/TNNLS.2015.2460239 doi: 10.1109/TNNLS.2015.2460239
![]() |
[87] |
A. Wu, Z. Zeng, Algebraical criteria of stability for delayed memristive neural networks, Adv. Differ. Equations, 2015 (2015), 1–12. https://doi.org/10.1186/s13662-015-0449-z doi: 10.1186/s13662-015-0449-z
![]() |
[88] | J. P. Aubin, A. Cellina, Differential Inclusions: Set-valued Maps and Viability Theory, Springer Science & Business Media, 2012. |
[89] | A. F. Filippov, Differential Equations with Discontinuous Righthand Sides: Control Systems, Springer Science & Business Media, 2013. |
[90] | J. Hu, J. Wang, Global uniform asymptotic stability of memristor-based recurrent neural networks with time delays, in The 2010 International Joint Conference on Neural Networks (IJCNN), IEEE, (2010), 1–8. https://doi.org/10.1109/IJCNN.2010.5596359 |
[91] |
S. Wen, Z. Zeng, T. Huang, Exponential stability analysis of memristor-based recurrent neural networks with time-varying delays, Neurocomputing, 97 (2012), 233–240. https://doi.org/10.1016/j.neucom.2012.06.014 doi: 10.1016/j.neucom.2012.06.014
![]() |
[92] |
K. Mathiyalagan, R. Anbuvithya, R. Sakthivel, J. H. Park, P. Prakash, Reliable stabilization for memristor-based recurrent neural networks with time-varying delays, Neurocomputing, 153 (2015), 140–147. https://doi.org/10.1016/j.neucom.2014.11.043 doi: 10.1016/j.neucom.2014.11.043
![]() |
[93] |
G. Zhang, Y. Shen, Q. Yin, J. Sun, Global exponential periodicity and stability of a class of memristor-based recurrent neural networks with multiple delays, Inf. Sci., 232 (2013), 386–396. https://doi.org/10.1016/j.ins.2012.11.023 doi: 10.1016/j.ins.2012.11.023
![]() |
[94] |
A. Wu, Z. Zeng, Global mittag–leffler stabilization of fractional-order memristive neural networks, IEEE Trans. Neural Networks Learn. Syst., 28 (2015), 206–217. https://doi.org/10.1109/TNNLS.2015.2506738 doi: 10.1109/TNNLS.2015.2506738
![]() |
[95] |
L. Chen, J. Cao, R. Wu, J. T. Machado, A. M. Lopes, H. Yang, Stability and synchronization of fractional-order memristive neural networks with multiple delays, Neural Networks, 94 (2017), 76–85. https://doi.org/10.1016/j.neunet.2017.06.012 doi: 10.1016/j.neunet.2017.06.012
![]() |
[96] |
J. Chen, Z. Zeng, P. Jiang, On the periodic dynamics of memristor-based neural networks with time-varying delays, Inf. Sci., 279 (2014), 358–373. https://doi.org/10.1016/j.ins.2014.03.124 doi: 10.1016/j.ins.2014.03.124
![]() |
[97] |
J. Zhao, Exponential stabilization of memristor-based neural networks with unbounded time-varying delays, Sci. China Inf. Sci., 64 (2021), 1–3. https://doi.org/10.1007/s11432-018-9817-4 doi: 10.1007/s11432-018-9817-4
![]() |
[98] |
Z. Zhang, X. Liu, D. Zhou, C. Lin, J. Chen, H. Wang, Finite-time stabilizability and instabilizability for complex-valued memristive neural networks with time delays, IEEE Trans. Syst. Man Cybern.: Syst., 48 (2017), 2371–2382. https://doi.org/10.1109/TSMC.2017.2754508 doi: 10.1109/TSMC.2017.2754508
![]() |
[99] |
M. Syed Ali, G. Narayanan, Z. Orman, V. Shekher, S. Arik, Finite time stability analysis of fractional-order complex-valued memristive neural networks with proportional delays, Neural Process. Lett., 51 (2020), 407–426. https://doi.org/10.1007/s11063-019-10097-7 doi: 10.1007/s11063-019-10097-7
![]() |
[100] |
Z. Cai, L. Huang, Finite-time stabilization of delayed memristive neural networks: Discontinuous state-feedback and adaptive control approach, IEEE Trans. Neural Networks Learn. Syst., 29 (2017), 856–868. https://doi.org/10.1109/TNNLS.2017.2651023 doi: 10.1109/TNNLS.2017.2651023
![]() |
[101] |
L. Wang, Z. Zeng, M. F. Ge, A disturbance rejection framework for finite-time and fixed-time stabilization of delayed memristive neural networks, IEEE Trans. Syst. Man Cybern.: Syst., 51 (2019), 905–915. https://doi.org/10.1109/TSMC.2018.2888867 doi: 10.1109/TSMC.2018.2888867
![]() |
[102] |
Y. Sheng, H. Zhang, Z. Zeng, Stabilization of fuzzy memristive neural networks with mixed time delays, IEEE Trans. Fuzzy Syst., 26 (2017), 2591–2606. https://doi.org/10.1109/TFUZZ.2017.2783899 doi: 10.1109/TFUZZ.2017.2783899
![]() |
[103] |
Q. Xiao, Z. Zeng, Lagrange stability for T–S fuzzy memristive neural networks with time-varying delays on time scales, IEEE Trans. Fuzzy Syst., 26 (2017), 1091–1103. https://doi.org/10.1109/TFUZZ.2017.2704059 doi: 10.1109/TFUZZ.2017.2704059
![]() |
[104] |
Y. Sheng, F. L. Lewis, Z. Zeng, Exponential stabilization of fuzzy memristive neural networks with hybrid unbounded time-varying delays, IEEE Trans. Neural Networks Learn. Syst., 30 (2018), 739–750. https://doi.org/10.1109/TNNLS.2018.2852497 doi: 10.1109/TNNLS.2018.2852497
![]() |
[105] |
Y. Sheng, F. L. Lewis, Z. Zeng, T. Huang, Lagrange stability and finite-time stabilization of fuzzy memristive neural networks with hybrid time-varying delays, IEEE Trans. Cybern., 50 (2019), 2959–2970. https://doi.org/10.1109/TCYB.2019.2912890 doi: 10.1109/TCYB.2019.2912890
![]() |
[106] |
S. Yang, C. Li, T. Huang, Exponential stabilization and synchronization for fuzzy model of memristive neural networks by periodically intermittent control, Neural Networks, 75 (2016), 162–172. https://doi.org/10.1016/j.neunet.2015.12.003 doi: 10.1016/j.neunet.2015.12.003
![]() |
[107] |
X. Wang, J. H. Park, S. Zhong, H. Yang, A switched operation approach to sampled-data control stabilization of fuzzy memristive neural networks with time-varying delay, IEEE Trans. Neural Networks Learn. Syst., 31 (2019), 891–900. https://doi.org/10.1109/TNNLS.2019.2910574 doi: 10.1109/TNNLS.2019.2910574
![]() |
[108] |
R. Zhang, D. Zeng, J. H. Park, H. K. Lam, S. Zhong, Fuzzy adaptive event-triggered sampled-data control for stabilization of T-S fuzzy memristive neural networks with reaction-diffusion terms, IEEE Trans. Fuzzy Syst., 29 (2020), 1775–1785. https://doi.org/10.1109/TFUZZ.2020.2985334 doi: 10.1109/TFUZZ.2020.2985334
![]() |
[109] |
X. Li, T. Huang, J. A. Fang, Event-triggered stabilization for takagi–sugeno fuzzy complex-valued memristive neural networks with mixed time-varying delays, IEEE Trans. Fuzzy Syst., 29 (2020), 1853–1863. https://doi.org/10.1109/TFUZZ.2020.2986713 doi: 10.1109/TFUZZ.2020.2986713
![]() |
[110] |
H. Wei, R. Li, B. Wu, Dynamic analysis of fractional-order quaternion-valued fuzzy memristive neural networks: Vector ordering approach, Fuzzy Sets Syst., 411 (2021), 1–24. https://doi.org/10.1016/j.fss.2020.02.013 doi: 10.1016/j.fss.2020.02.013
![]() |
[111] |
R. Sakthivel, R. Raja, S. M. Anthoni, Exponential stability for delayed stochastic bidirectional associative memory neural networks with markovian jumping and impulses, J. Optim. Theory Appl., 150 (2011), 166–187. https://doi.org/10.1007/s10957-011-9808-4 doi: 10.1007/s10957-011-9808-4
![]() |
[112] |
J. Li, M. Hu, L. Guo, Exponential stability of stochastic memristor-based recurrent neural networks with time-varying delays, Neurocomputing, 138 (2014), 92–98. https://doi.org/10.1016/j.neucom.2014.02.042 doi: 10.1016/j.neucom.2014.02.042
![]() |
[113] |
Z. Meng, Z. Xiang, Stability analysis of stochastic memristor-based recurrent neural networks with mixed time-varying delays, Neural Comput. Appl., 28 (2017), 1787–1799. https://doi.org/10.1007/s00521-015-2146-y doi: 10.1007/s00521-015-2146-y
![]() |
[114] |
X. Li, J. Fang, H. Li, Exponential stabilisation of stochastic memristive neural networks under intermittent adaptive control, IET Control Theory Appl., 11 (2017), 2432–2439. https://doi.org/10.1049/iet-cta.2017.0021 doi: 10.1049/iet-cta.2017.0021
![]() |
[115] |
D. Liu, S. Zhu, W. Chang, Mean square exponential input-to-state stability of stochastic memristive complex-valued neural networks with time varying delay, Int. J. Syst. Sci., 48 (2017), 1966–1977. https://doi.org/10.1080/00207721.2017.1300706 doi: 10.1080/00207721.2017.1300706
![]() |
[116] |
C. Li, J. Lian, Y. Wang, Stability of switched memristive neural networks with impulse and stochastic disturbance, Neurocomputing, 275 (2018), 2565–2573. https://doi.org/10.1016/j.neucom.2017.11.031 doi: 10.1016/j.neucom.2017.11.031
![]() |
[117] |
H. Liu, Z. Wang, B. Shen, T. Huang, F. E. Alsaadi, Stability analysis for discrete-time stochastic memristive neural networks with both leakage and probabilistic delays, Neural Networks, 102 (2018), 1–9. https://doi.org/10.1016/j.neunet.2018.02.003 doi: 10.1016/j.neunet.2018.02.003
![]() |
[118] |
K. Ding, Q. Zhu, Impulsive method to reliable sampled-data control for uncertain fractional-order memristive neural networks with stochastic sensor faults and its applications, Nonlinear Dyn., 100 (2020), 2595–2608. https://doi.org/10.1007/s11071-020-05670-y doi: 10.1007/s11071-020-05670-y
![]() |
[119] |
S. Duan, H. Wang, L. Wang, T. Huang, C. Li, Impulsive effects and stability analysis on memristive neural networks with variable delays, IEEE Trans. Neural Networks Learn. Syst., 28 (2016), 476–481. https://doi.org/10.1109/TNNLS.2015.2497319 doi: 10.1109/TNNLS.2015.2497319
![]() |
[120] |
W. Zhang, T. Huang, X. He, C. Li, Global exponential stability of inertial memristor-based neural networks with time-varying delays and impulses, Neural Networks, 95 (2017), 102–109. https://doi.org/10.1016/j.neunet.2017.03.012 doi: 10.1016/j.neunet.2017.03.012
![]() |
[121] |
W. Zhu, D. Wang, L. Liu, G. Feng, Event-based impulsive control of continuous-time dynamic systems and its application to synchronization of memristive neural networks, IEEE Trans. Neural Networks Learn. Syst., 29 (2017), 3599–3609. https://doi.org/10.1109/TNNLS.2017.2731865 doi: 10.1109/TNNLS.2017.2731865
![]() |
[122] |
H. Wang, S. Duan, T. Huang, C. Li, L. Wang, Novel stability criteria for impulsive memristive neural networks with time-varying delays, Circuits Syst. Signal Process., 35 (2016), 3935–3956. https://doi.org/10.1007/s00034-015-0240-0 doi: 10.1007/s00034-015-0240-0
![]() |
[123] |
J. Qi, C. Li, T. Huang, Stability of delayed memristive neural networks with time-varying impulses, Cognit. Neurodyn., 8 (2014), 429–436. https://doi.org/10.1007/s11571-014-9286-0 doi: 10.1007/s11571-014-9286-0
![]() |
[124] |
J. G. Lu, Global exponential stability and periodicity of reaction-diffusion delayed recurrent neural networks with dirichlet boundary conditions, Chaos Solitons Fractals, 35 (2008), 116–125. https://doi.org/10.1016/j.chaos.2007.05.002 doi: 10.1016/j.chaos.2007.05.002
![]() |
[125] |
J. L. Wang, H. N. Wu, L. Guo, Passivity and stability analysis of reaction-diffusion neural networks with Dirichlet boundary conditions, IEEE Trans. Neural Networks, 22 (2011), 2105–2116. https://doi.org/10.1109/TNN.2011.2170096 doi: 10.1109/TNN.2011.2170096
![]() |
[126] |
L. Wang, R. Zhang, Y. Wang, Global exponential stability of reaction-diffusion cellular neural networks with S-type distributed time delays, Nonlinear Anal. Real World Appl., 10 (2009), 1101–1113. https://doi.org/10.1016/j.nonrwa.2007.12.002 doi: 10.1016/j.nonrwa.2007.12.002
![]() |
[127] |
L. Wang, M. F. Ge, J. Hu, G. Zhang, Global stability and stabilization for inertial memristive neural networks with unbounded distributed delays, Nonlinear Dyn., 95 (2019), 943–955. https://doi.org/10.1007/s11071-018-4606-2 doi: 10.1007/s11071-018-4606-2
![]() |
[128] |
L. Wang, H. He, Z. Zeng, Intermittent stabilization of fuzzy competitive neural networks with reaction diffusions, IEEE Trans. Fuzzy Syst., 29 (2021), 2361–2372. https://doi.org/10.1109/TFUZZ.2020.2999041 doi: 10.1109/TFUZZ.2020.2999041
![]() |
[129] |
R. Rakkiyappan, S. Dharani, Q. Zhu, Synchronization of reaction-diffusion neural networks with time-varying delays via stochastic sampled-data controller, Nonlinear Dyn., 79 (2015), 485–500. https://doi.org/10.1007/s11071-014-1681-x doi: 10.1007/s11071-014-1681-x
![]() |
[130] |
Z. P. Wang, H. N. Wu, J. L. Wang, H. X. Li, Quantized sampled-data synchronization of delayed reaction-diffusion neural networks under spatially point measurements, IEEE Trans. Cybern., 51 (2021), 5740–5751. https://doi.org/10.1109/TCYB.2019.2960094 doi: 10.1109/TCYB.2019.2960094
![]() |
[131] |
Q. Qiu, H. Su, Sampling-based event-triggered exponential synchronization for reaction-diffusion neural networks, IEEE Trans. Neural Networks Learn. Syst., 34 (2021), 1209–1217. https://doi.org/10.1109/TNNLS.2021.3105126 doi: 10.1109/TNNLS.2021.3105126
![]() |
[132] |
D. Zeng, R. Zhang, J. H. Park, Z. Pu, Y. Liu, Pinning synchronization of directed coupled reaction-diffusion neural networks with sampled-data communications, IEEE Trans. Neural Networks Learn. Syst., 31 (2020), 2092–2103. https://doi.org/10.1109/TNNLS.2019.2928039 doi: 10.1109/TNNLS.2019.2928039
![]() |
[133] |
Z. Guo, S. Wang, J. Wang, Global exponential synchronization of coupled delayed memristive neural networks with reaction–diffusion terms via distributed pinning controls, IEEE Trans. Neural Networks Learn. Syst., 32 (2021), 105–116. https://doi.org/10.1109/TNNLS.2020.2977099 doi: 10.1109/TNNLS.2020.2977099
![]() |
[134] |
Y. Cao, Y. Cao, Z. Guo, T. Huang, S. Wen, Global exponential synchronization of delayed memristive neural networks with reaction-diffusion terms, Neural Networks, 123 (2020), 70–81. https://doi.org/10.1016/j.neunet.2019.11.008 doi: 10.1016/j.neunet.2019.11.008
![]() |
[135] |
L. Shanmugam, P. Mani, R. Rajan, Y. H. Joo, Adaptive synchronization of reaction-diffusion neural networks and its application to secure communication, IEEE Trans. Cybern., 50 (2020), 911–922. https://doi.org/10.1109/TCYB.2018.2877410 doi: 10.1109/TCYB.2018.2877410
![]() |
[136] |
J. L. Wang, Z. Qin, H. N. Wu, T. Huang, Passivity and synchronization of coupled uncertain reaction-diffusion neural networks with multiple time delays, IEEE Trans. Neural Networks Learn. Syst., 30 (2019), 2434–2448. https://doi.org/10.1109/TNNLS.2018.2884954 doi: 10.1109/TNNLS.2018.2884954
![]() |
[137] |
R. Zhang, D. Zeng, J. H. Park, Y. Liu, X. Xie, Adaptive event-triggered synchronization of reaction-diffusion neural networks, IEEE Trans. Neural Networks Learn. Syst., 32 (2021), 3723–3735. https://doi.org/10.1109/TNNLS.2020.3027284 doi: 10.1109/TNNLS.2020.3027284
![]() |
[138] |
J. Pan, X. Liu, S. Zhong, Stability criteria for impulsive reaction-diffusion cohen-grossberg neural networks with time-varying delays, Math. Comput. Modell., 51 (2010), 1037–1050. https://doi.org/10.1016/j.mcm.2009.12.004 doi: 10.1016/j.mcm.2009.12.004
![]() |
[139] |
S. Mongolian, Y. Kao, C. Wang, H. Xia, Robust mean square stability of delayed stochastic generalized uncertain impulsive reaction-diffusion neural networks, J. Franklin Inst., 358 (2021), 877–894. https://doi.org/10.1016/j.jfranklin.2020.04.011 doi: 10.1016/j.jfranklin.2020.04.011
![]() |
[140] |
T. Wei, P. Lin, Y. Wang, L. Wang, Stability of stochastic impulsive reaction-diffusion neural networks with S-type distributed delays and its application to image encryption, Neural Networks, 116 (2019), 35–45. https://doi.org/10.1016/j.neunet.2019.03.016 doi: 10.1016/j.neunet.2019.03.016
![]() |
[141] |
T. Wei, X. Li, V. Stojanovic, Input-to-state stability of impulsive reaction-diffusion neural networks with infinite distributed delays, Nonlinear Dyn., 103 (2021), 1733–1755. https://doi.org/10.1007/s11071-021-06208-6 doi: 10.1007/s11071-021-06208-6
![]() |
[142] |
J. Cao, G. Stamov, I. Stamova, S. Simeonov, Almost periodicity in impulsive fractional-order reaction-diffusion neural networks with time-varying delays, IEEE Trans. Cybern., 51 (2021), 151–161. https://doi.org/10.1109/TCYB.2020.2967625 doi: 10.1109/TCYB.2020.2967625
![]() |
[143] | T. Wei, X. Li, J. Cao, Stability of delayed reaction-diffusion neural-network models with hybrid impulses via vector Lyapunov function, IEEE Trans. Neural Networks Learn. Syst., early access, (2022), 1–12. https://doi.org/10.1109/TNNLS.2022.3143884 |
[144] |
C. Hu, H. Jiang, Z. Teng, Impulsive control and synchronization for delayed neural networks with reaction-diffusion terms, IEEE Trans. Neural Networks, 21 (2010), 67–81. https://doi.org/10.1109/TNN.2009.2034318 doi: 10.1109/TNN.2009.2034318
![]() |
[145] |
X. Yang, J. Cao, Z. Yang, Synchronization of coupled reaction-diffusion neural networks with time-varying delays via pinning-impulsive controller, SIAM J. Control Optim., 51 (2013), 3486–3510. https://doi.org/10.1137/120897341 doi: 10.1137/120897341
![]() |
[146] |
W. H. Chen, S. Luo, W. X. Zheng, Impulsive synchronization of reaction–diffusion neural networks with mixed delays and its application to image encryption, IEEE Trans. Neural Networks Learn. Syst., 27 (2016), 2696–2710. https://doi.org/10.1109/TNNLS.2015.2512849 doi: 10.1109/TNNLS.2015.2512849
![]() |
[147] |
H. Chen, P. Shi, C. C. Lim, Pinning impulsive synchronization for stochastic reaction–diffusion dynamical networks with delay, Neural Networks, 106 (2018), 281–293. https://doi.org/10.1016/j.neunet.2018.07.009 doi: 10.1016/j.neunet.2018.07.009
![]() |
[148] |
Y. Wang, P. Lin, L. Wang, Exponential stability of reaction-diffusion high-order Markovian jump hopfield neural networks with time-varying delays, Nonlinear Anal. Real World Appl., 13 (2012), 1353–1361. https://doi.org/10.1016/j.nonrwa.2011.10.013 doi: 10.1016/j.nonrwa.2011.10.013
![]() |
[149] | R. Zhang, H. Wang, J. H. Park, K. Shi, P. He, Mode-dependent adaptive event-triggered control for stabilization of Markovian memristor-based reaction-diffusion neural networks, IEEE Trans. Neural Networks Learn. Syst., early access, (2021), 1–13. https://doi.org/10.1109/TNNLS.2021.3122143 |
[150] |
X. X. Han, K. N. Wu, Y. Niu, Asynchronous boundary stabilization of stochastic Markov jump reaction-diffusion systems, IEEE Trans. Syst. Man Cybern.: Syst., 52 (2022), 5668–5678. https://doi.org/10.1109/TSMC.2021.3130271 doi: 10.1109/TSMC.2021.3130271
![]() |
[151] |
Q. Zhu, X. Li, X. Yang, Exponential stability for stochastic reaction-diffusion BAM neural networks with time-varying and distributed delays, Appl. Math. Comput., 217 (2011), 6078–6091. https://doi.org/10.1016/j.amc.2010.12.077 doi: 10.1016/j.amc.2010.12.077
![]() |
[152] |
Y. Sheng, H. Zhang, Z. Zeng, Stability and robust stability of stochastic reaction–diffusion neural networks with infinite discrete and distributed delays, IEEE Trans. Syst. Man Cybern.: Syst., 50 (2020), 1721–1732. https://doi.org/10.1109/TSMC.2017.2783905 doi: 10.1109/TSMC.2017.2783905
![]() |
[153] |
X. Z. Liu, K. N. Wu, X. Ding, W. Zhang, Boundary stabilization of stochastic delayed Cohen-Grossberg neural networks with diffusion terms, IEEE Trans. Neural Networks Learn. Syst., 33 (2022), 3227–3237. https://doi.org/10.1109/TNNLS.2021.3051363 doi: 10.1109/TNNLS.2021.3051363
![]() |
[154] |
X. Liang, L. Wang, Y. Wang, R. Wang, Dynamical behavior of delayed reaction-diffusion Hopfield neural networks driven by infinite dimensional Wiener processes, IEEE Trans. Neural Networks Learn. Syst., 27 (2016), 1816–1826. https://doi.org/10.1109/TNNLS.2015.2460117 doi: 10.1109/TNNLS.2015.2460117
![]() |
[155] |
T. Wei, P. Lin, Q. Zhu, L. Wang, Y. Wang, Dynamical behavior of nonautonomous stochastic reaction-diffusion neural-network models, IEEE Trans. Neural Networks Learn. Syst., 30 (2019), 1575–1580. https://doi.org/10.1109/TNNLS.2018.2869028 doi: 10.1109/TNNLS.2018.2869028
![]() |
[156] |
Q. Yao, P. Lin, L. Wang, Y. Wang, Practical exponential stability of impulsive stochastic reaction-diffusion systems with delays, IEEE Trans. Cybern., 52 (2022), 2687–2697. https://doi.org/10.1109/TCYB.2020.3022024 doi: 10.1109/TCYB.2020.3022024
![]() |
[157] |
Q. Ma, S. Xu, Y. Zou, G. Shi, Synchronization of stochastic chaotic neural networks with reaction-diffusion terms, Nonlinear Dyn., 67 (2012), 2183–2196. https://doi.org/10.1007/s11071-011-0138-8 doi: 10.1007/s11071-011-0138-8
![]() |
[158] |
Y. Sheng, Z. Zeng, Impulsive synchronization of stochastic reaction-diffusion neural networks with mixed time delays, Neural Networks, 103 (2018), 83–93. https://doi.org/10.1016/j.neunet.2018.03.010 doi: 10.1016/j.neunet.2018.03.010
![]() |
[159] |
M. S. Ali, L. Palanisamy, J. Yogambigai, L. Wang, Passivity-based synchronization of Markovian jump complex dynamical networks with time-varying delays, parameter uncertainties, reaction–diffusion terms, and sampled-data control, J. Comput. Appl. Math., 352 (2019), 79–92. https://doi.org/10.1016/j.cam.2018.10.047 doi: 10.1016/j.cam.2018.10.047
![]() |
[160] |
X. Yang, Q. Song, J. Cao, J. Lu, Synchronization of coupled Markovian reaction-diffusion neural networks with proportional delays via quantized control, IEEE Trans. Neural Networks Learn. Syst., 30 (2019), 951–958. https://doi.org/10.1109/TNNLS.2018.2853650 doi: 10.1109/TNNLS.2018.2853650
![]() |
[161] |
X. Song, J. Man, S. Song, Z. Wang, Finite-time nonfragile time-varying proportional retarded synchronization for Markovian inertial memristive NNs with reaction–diffusion items, Neural Networks, 123 (2020), 317–330. https://doi.org/10.1016/j.neunet.2019.12.011 doi: 10.1016/j.neunet.2019.12.011
![]() |
[162] |
H. Shen, X. Wang, J. Wang, J. Cao, L. Rutkowski, Robust composite synchronization of Markov jump reaction-diffusion neural networks via a disturbance observer-based method, IEEE Trans. Cybern., 52 (2022), 12712–12721. https://doi.org/10.1109/TCYB.2021.3087477 doi: 10.1109/TCYB.2021.3087477
![]() |