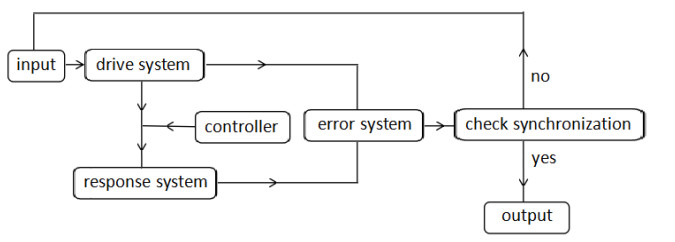
In this paper, we investigate the fixed-time synchronization of fuzzy stochastic cellular neural networks (FSCNNs) with mixed delay and Lˊevy noise. We designed the feedback controller and adaptive controller for cellular neural networks with Lˊevy noise to achieve fixed-time synchronization. Using the Lyapunov theory and the Itˆo formula, we established the criterion for fixed-time synchronization of FSCNNs with Lˊevy noise. Additionally, we obtained the resulting settling time, which is independent of the initial values of the system. The practicality and validity of the theoretical conclusions are demonstrated through two examples. The research results show that when the intensity of random interference is not large, FSCNNs can achieve synchronization through appropriate control means.
Citation: Tianyi Li, Xiaofeng Xu, Ming Liu. Fixed-time synchronization of mixed-delay fuzzy cellular neural networks with Lˊevy noise[J]. Electronic Research Archive, 2025, 33(4): 2032-2060. doi: 10.3934/era.2025090
[1] | Jiaqi Chang, Xiangxin Yin, Caoyuan Ma, Donghua Zhao, Yongzheng Sun . Estimation of the time cost with pinning control for stochastic complex networks. Electronic Research Archive, 2022, 30(9): 3509-3526. doi: 10.3934/era.2022179 |
[2] | Minglei Fang, Jinzhi Liu, Wei Wang . Finite-/fixed-time synchronization of leakage and discrete delayed Hopfield neural networks with diffusion effects. Electronic Research Archive, 2023, 31(7): 4088-4101. doi: 10.3934/era.2023208 |
[3] | Xiangwen Yin . A review of dynamics analysis of neural networks and applications in creation psychology. Electronic Research Archive, 2023, 31(5): 2595-2625. doi: 10.3934/era.2023132 |
[4] | Shuang Liu, Tianwei Xu, Qingyun Wang . Effect analysis of pinning and impulsive selection for finite-time synchronization of delayed complex-valued neural networks. Electronic Research Archive, 2025, 33(3): 1792-1811. doi: 10.3934/era.2025081 |
[5] | Yu-Jing Shi, Yan Ma . Finite/fixed-time synchronization for complex networks via quantized adaptive control. Electronic Research Archive, 2021, 29(2): 2047-2061. doi: 10.3934/era.2020104 |
[6] | Jun Guo, Yanchao Shi, Shengye Wang . Synchronization analysis of delayed quaternion-valued memristor-based neural networks by a direct analytical approach. Electronic Research Archive, 2024, 32(5): 3377-3395. doi: 10.3934/era.2024156 |
[7] | Xinling Li, Xueli Qin, Zhiwei Wan, Weipeng Tai . Chaos synchronization of stochastic time-delay Lur'e systems: An asynchronous and adaptive event-triggered control approach. Electronic Research Archive, 2023, 31(9): 5589-5608. doi: 10.3934/era.2023284 |
[8] | Xingting Geng, Jianwen Feng, Yi Zhao, Na Li, Jingyi Wang . Fixed-time synchronization of nonlinear coupled memristive neural networks with time delays via sliding-mode control. Electronic Research Archive, 2023, 31(6): 3291-3308. doi: 10.3934/era.2023166 |
[9] | Yilin Li, Jianwen Feng, Jingyi Wang . Mean square synchronization for stochastic delayed neural networks via pinning impulsive control. Electronic Research Archive, 2022, 30(9): 3172-3192. doi: 10.3934/era.2022161 |
[10] | Jun Guo, Yanchao Shi, Weihua Luo, Yanzhao Cheng, Shengye Wang . Exponential projective synchronization analysis for quaternion-valued memristor-based neural networks with time delays. Electronic Research Archive, 2023, 31(9): 5609-5631. doi: 10.3934/era.2023285 |
In this paper, we investigate the fixed-time synchronization of fuzzy stochastic cellular neural networks (FSCNNs) with mixed delay and Lˊevy noise. We designed the feedback controller and adaptive controller for cellular neural networks with Lˊevy noise to achieve fixed-time synchronization. Using the Lyapunov theory and the Itˆo formula, we established the criterion for fixed-time synchronization of FSCNNs with Lˊevy noise. Additionally, we obtained the resulting settling time, which is independent of the initial values of the system. The practicality and validity of the theoretical conclusions are demonstrated through two examples. The research results show that when the intensity of random interference is not large, FSCNNs can achieve synchronization through appropriate control means.
Neural networks are widely used in many fields such as biomedical, image processing, automatic control, pattern recognition, signal processing, and secure communication; the synchronization problem of neural networks has been widely studied in recent years[1,2,3,4,5,6,7]. In 1988, Chua and Yang first proposed cellular neural networks through parallel processing [8]; subsequently, CNNs attracted the attention of many scholars. In order to deal with some uncertain and incomplete data, Yang et al. added fuzzy logic to the structure of traditional CNNs and first proposed the fuzzy cellular neural network model (FCNNs) [9]. Compared with traditional CNNs, FCNNs have many advantages such as better adaptability, fault tolerance and robustness, and the ability to process information faster [10]. In addition, neural networks in the real environment are inevitably affected by signal transmission delay and external stochastic interference, which make the analysis of the networks more complicated.
Synchronization is a very important dynamic behavior of neural networks and has become a hot topic. In most of the research on neural networks synchronization, scholars have focused on the asymptotic and exponential synchronization of neural networks[11,12,13,14,15]. Asymptotic or exponential synchronization means that the synchronization can only be achieved when time approaches infinity. The applications of these theories are limited, because many systems in practical applications such as secure communications, manufacturing engineering, and power networks require achieving synchronization in finite time. Therefore, the finite-time synchronization of complex networks has gradually been studied by many researchers. In [16], Yang and Cao investigated the finite-time synchronization of complex networks with white noise perturbations by using the finite-time stability theorem and inequality techniques. In [17], Wang investigated the finite-time synchronization of fuzzy cellular neural networks with proportional delays. In [18], Duan et al. researched the finite-time synchronization of delayed fuzzy cellular neural networks with discontinuous activations. In [19], Wu et al. obtained the finite-time synchronization criteria for a chaotic dynamic neural networks with mixed time-varying delays and stochastic disturbance by using the state feedback control and adaptive control. In [20], Abdurahman et al. studied the finite-time synchronization of fuzzy cellular neural networks with time-varying delays. Other literature on finite-time synchronization of neural networks can be found in references [21,22,23,24,25].
Compared with the asymptotic synchronization, the finite-time synchronization can be achieved in a finite time and has faster convergence speed and stronger anti-interference. However, the convergence time of the finite-time synchronization is usually very dependent on the initial state of the systems. That is, different initial states can lead to different convergence times. The initial state of the systems in many practical problems are difficult to know. Therefore, obtaining a fixed convergence time that does not depend on the initial states of the systems has been the focus of scholars in recent years. In [26], Yang et al. investigated the fixed-time synchronization of complex networks with impulsive effects by designing a new Lyapunov function and constructing comparison systems. In [27], Khanzadeh and Pourgholi investigated the fixed-time synchronization of complex dynamical networks with nonidentical nodes in the presence of bounded uncertainties and disturbances by using the sliding mode control technique. In [28], Zhang et al. studied the fixed-time synchronization of stochastic complex networks with white noise and designed a continuous controller to avoid the chattering phenomenon. In [29], Ren et al. investigated the fixed-time synchronization of stochastic memristor-based neural networks with white noise via the state feedback control and the adaptive control. In [30], Kong et al. studied the fixed-time synchronization of a class of discontinuous fuzzy inertial neural networks with time-varying delays by relaxing the conditions of the C-regular Lyapunov function. Other literature on fixed-time synchronization of stochastic neural networks can be found in references [31,32,33,34,35].
It is worth noting that in the above studies on fixed-time synchronization, stochastic neural networks are all driven by white noise. White noise is a continuous effect that can be used to characterize continuous stochastic interference. However, discontinuous stochastic perturbations should also be considered, which can be used to describe sudden external influences. Lˊevy noise combines time, Brownian motion, and random jumps and can better simulate extremely unusual and sudden events such as earthquakes, flash floods, and epidemics, which can not be depicted just by white noise. The introduction of Lˊevy noise brings new difficulties and challenges to the research of neural networks. In [36], Zhu proved several Razumikhin-type theorems for the pth moment exponential stability of stochastic functional differential equations with Lˊevy noise. In [37], Zhou et al. investigated the stabilization of stochastic coupled systems with time-varying delays and Lˊevy noise via the periodically intermittent control. In [38], Zhou et al. studied the cluster synchronization of coupled neural networks with Lˊevy noise by proposing a trigger mechanism and designing a data sampling strategy. In [39], Shi et al. discussed the pth moment exponential synchronization of one-leader multi-follower systems by using the Lyapunov stability theory and the exponential stability criterion. In [40], Zhang et al. researched the semi-globally exponential synchronization of stochastic systems with mixed time delay and Lˊevy noise under the aperiodic intermittent delayed sampled-data control. However, few studies on fixed-time synchronization of stochastic neural networks with Lˊevy noise can be found. In [41], a fixed-time synchronization criterion for stochastic cellular neural networks with Lˊevy noise was established by utilizing the Lyapunov function method and the inequality technique. The research in this area is far from enough and deserves further study.
Based on the above analysis, this article is devoted to the fixed-time synchronization of FSCNNs with white noise, Lˊevy noise, and mixed time delay under feedback control and adaptive control. The following three points reflect the core contributions of this paper.
● A first attempt is made to study the fixed-time synchronization of FSCNNs with Lˊevy noise. Based on the Lyapunov theory, a fixed-time synchronization criterion for FSCNNs under the influence of Lˊevy noise is derived. The obtained synchronization time is independent of the initial value of the systems.
● This paper designs two control methods, feedback control and adaptive control, to achieve the fixed-time synchronization of FSCNNs with Lˊevy noise.
● In the discussion of the fixed-time synchronization of FSCNNs with Lˊevy noise, we use high-order terms to limit the impact of Lˊevy noise.
In fact, the main purpose of this paper is to generalize the fixed-time synchronization method of stochastic neural networks with white noise in reference [29] to stochastic neural networks with Lˊevy noise. Moreover, compared with the Lˊevy noises in existing literature such as [36,37,38,39,40,41], the assumption about the intensity function of Lˊevy noise in this paper is different.
The remainder of the article is divided into the sections below. Section 2 presents some preliminary descriptions and model characterizations. Section 3 presents the derivation of a fixed-time synchronization criterion with Lˊevy noise based on the Lyapunov theory and the Itˆo formula. In Section 4, some numerical examples are given to validate the proposed criterion.
This paper considers a class of FSCNNs with discrete and distributed delays under white noise and Lˊevy noise
dαi(t)=[−aiαi(t)+l∑j=1(ςij˜f(αj(t))+νij˜f(αj(t−h))+θij∫tt−h˜f(αj(s))ds)+l⋀j=1mij˜g(αj(t−h))+l⋁j=1ιij˜g(αj(t−h))+l⋀j=1oij∫tt−h˜g(αj(s))ds+l⋁j=1ξij∫tt−h˜g(αj(s))ds+l⋀j=1Gijpj+l⋁j=1Hijpj+Di]dt+wi(αi(t),t)dB(t)+∫Sγi(αi(t),t,u)˜N(dt,du), | (2.1) |
where αi(t) represents the state of the ith node at time t with i∈{1,2,⋯,l}, and l is the total number of neuron nodes. ai represents the passive decay rate to the ith node; h represents the delay that occurs at the dynamic node; ςij, νij, and θij represent the elements of the feedback template; pi represents the input of the ith neuron; Di is the deviation of the ith neuron. mij, oij, ιij, ξij are the elements of the fuzzy feedback template; Gij, Hij are the elements of the fuzzy feed-forward template. ˜f(⋅):R→R and ˜g(⋅):R→R are the activation functions. (Ω,F,F,P) is the complete probability of the sample space with the filtration F={Ft}t≥0 satisfying the usual conditions (i.e., it is right continuous and F0 contains all P-null sets). The intensity of white noise is denoted by wi:R×R+→R, and B(t) is the one-dimensional Brownian motion defined on (Ω,F,F,P). γ(⋅):R×R+×S→R serves as the Lˊevy noise intensity function; the compensated Poisson random measure is represented as ˜N(dt,du)=N(dt,du)−λ(du), where N(dt,du) is the Poisson counting measure on [0,+∞]×S. λ is the intensity measure and S is the measurable subset of R+ such that λ(S)<∞ and λ(S)=ˉλ. Write L2F0([−h,0];Rl) for the family of all F0-measurable C([−h,0];Rl)-value random variables x such that E(||x||2)<∞, where E(⋅) denotes the mathematical expectation with respect to the given probability measure P.
The system (2.1) is used as a drive system and the following is the response system
dβi(t)=[−aiβi(t)+l∑j=1(ςij˜f(βj(t))+νij˜f(βj(t−h))+θij∫tt−h˜f(βj(s))ds)+l⋀j=1mij˜g(βj(t−h))+l⋁j=1ιij˜g(βj(t−h))+l⋀j=1oij∫tt−h˜g(βj(s))ds+l⋁j=1ξij∫tt−h˜g(βj(s))ds+l⋀j=1Gijpj+l⋁j=1Hijpj+Di+ui(t)]dt+wi(βi(t),t)dB(t)+∫Sγi(βi(t),t,u)˜N(dt,du), | (2.2) |
where ui(t) is a suitable controller and will be designed to ensure that systems (2.1) and (2.2) are synchronized at a fixed time. The initial conditions of systems (2.1) and (2.2) are
ϕ(s)=(ϕ1(s),ϕ2(s),⋯,ϕl(s))∈L2F0([−h,0];Rl),ψ(s)=(ψ1(s),ψ2(s),⋯,ψl(s))∈L2F0([−h,0];Rl), |
respectively. Define the error as ζi(t)=βi(t)−αi(t). From (2.1) and (2.2), the error system is as follows
dζi(t)=[−aiζi(t)+l∑j=1(ςij˜F(ζj(t))+νij˜F(ζj(t−h))+θij∫tt−h˜F(ζj(s))ds)+l⋀j=1mij˜G(ζj(t−h))+l⋁j=1ιij˜G(ζj(t−h))+l⋀j=1oij∫tt−h˜G(ζj(s))ds+l⋁j=1ξij∫tt−h˜G(ζj(s))ds+ui(t)]dt+WidB(t)+∫SΓi˜N(dt,du), |
where
˜F(ζj(⋅))=˜f(βj(⋅))−˜f(αj(⋅)),l⋀j=1mij˜G(ζj(⋅))=l⋀j=1mij˜g(βj(⋅))−l⋀j=1mij˜g(αj(⋅)),Wi=wi(βi(t),t)−wi(αi(t),t),Γi=γi(βi(t),t,u)−γi(αi(t),t,u). |
Consider a stochastic system as follows
dρ(t)=˜f(t,ρ(t))d(t)+w(t,ρ(t))dB(t)+∫Sγ(ρ(t),t,u)˜N(dt,du), | (2.3) |
where ρ(0)=ρ0∈Rl. The system (2.3) is a Lˊevy process-driven stochastic differential equation, and the Itˆo formulation for the generalization of such equations is given in the monograph [42]. The first hitting time is a function about the settling time expressed as T(ρ0,ϵ)=inf{t|ρ(t)=0,t≥0}, where ϵ∈Ω is a fundamental event.
Definition 2.1. [29] For any initial state ρ0∈Rl, if the following are satisfied,
1) The origin is globally stochastic finite-time stable in probability.
2) The mathematical expectation of T(ρ0,ϵ) has a upper bound M>0, which is not dependent on the selection of initial values,
Tϵ=E(T(ρ0,ϵ))≤M,∀ρ0∈Rl, |
then the trivial solution of system (2.3) is considered to be stochastic fixed-time stable in probability.
Assumption 2.2. There exists a positive number ϰi, such that
Tr[WTiWi]≤ϰiζTi(t)ζi(t). |
Assumption 2.3. For any s1,s2∈R, there exist positive constants L1 and L2 satisfying
|˜f(s1)−˜f(s2)|≤L1|s1−s2|,|˜g(s1)−˜g(s2)|≤L2|s1−s2|. |
Assumption 2.4. For any ζ(t)∈Rl, t∈R+, u∈S and m1,m2,…,mn>1, there exists a positive number φi, such that
|Γi|≤φi|ζi(t)|mi. |
Lemma 2.5. [9] Assuming ρ1 and ρ2 represent two states of system (2.1), then
|l⋀j=1mij˜g(ρ1)−l⋀j=1mij˜g(ρ2)|≤l∑j=1|mij||˜g(ρ1)−˜g(ρ2)|,|l⋁j=1ιij˜g(ρ1)−l⋁j=1ιij˜g(ρ2)|≤l∑j=1|ιij||˜g(ρ1)−˜g(ρ2)|. |
Lemma 2.6. [43] If S1,S2,…,Sl≥0 and 0<m<1, M>1, then
∑li=1Smi≥(∑li=1Si)m,∑li=1SMi≥l1−M(∑li=1Si)M. |
This section establishes an adequate criterion for fixed-time synchronization of FSCNNs based on the Lyapunov method.
State feedback control aims to generate a control input that drives a system to a desired state by utilizing the state variables of the system. By monitoring the current state of the system, the controller will adjust the control input so that the error between the current state and the expected state of the system is constantly reduced and the two states are finally in agreement. Because the control rules are fixed and the feedback gain does not change over time, the design process of the feedback controller is relatively simple and easy to implement. In addition, the synchronization criterion of a system under feedback control is also easier to derive. The advantages of feedback controller are its simple design, small amount of computation, easy derivation of synchronization criteria, and convenient realization. These advantages make the state feedback control a most commonly used technique in modern control theory. First, we design a state feedback controller ui(t) to synchronize FSCNNs at a fixed time as follows:
ui(t)={−ℵiζi(t)−l∑j=1(ωij|ζj(t−h)|sign(ζi(t))−12ϖij∫tt−h|ζi(s)|2dsζi(t)|ζi(t)|2)−μ1|ζi(t)|qsign(ζi(t))−η1[|ζi(t)|2M−1+|ζi(t)|2m−1]sign(ζi(t)),ifζi(t)≠0,0,ifζi(t)=0, | (2.4) |
where ℵi, ωij, μ1, η1, ϖij are all nonnegative numbers, 0<q<1, and M>m>1. The block diagram of feedback control model is shown in Figure 1.
Theorem 2.7. Under the Assumptions 2.2–2.4, the systems (2.1) and (2.2) are stochastic fixed-time synchronization under the feedback control (2.4), if the following four conditions hold
−2ai−2ℵi+ϰi+l∑j=1(L1ςij+L1ςji+θij+oij+ξij)≤0,2l∑j=1(L1νij+|mij|L2+|ιij|L2−ωij)≤0,l∑j=1(hθij(L1)2+hsji(L2)2+hcji(L2)2−ϖij)≤0,2η1l1−M−ˉλlˉφ>0. |
And the settling time is
Tϵ=1ˆμ111−Q+1ˆη11M−1, |
where ˉφ=max[φ2i], M=max[mi], m=min[mi], ˆμ1=2μ1, Q=1+q2, ˆη1=2η1l1−M−ˉλlˉφ.
Proof. We structure the Lyapunov function V(ζ(t))=∑li=1ζTi(t)ζi(t). According to the Itˆo formula, we have
LV=2l∑i=1ζTi(t)[−aiζi(t)+l∑j=1(ςij˜F(ζj(t))+νij˜F(ζj(t−h))+θij∫tt−h˜F(ζj(s))ds)+l⋀j=1mij˜G(ζj(t−h))+l⋁j=1ιij˜G(ζj(t−h))+l⋀j=1oij∫tt−h˜G(ζj(s))ds+l⋁j=1ξij∫tt−h˜G(ζj(s))ds+ui(t)]+l∑i=1Tr(WTiWi)+l∑i=1∫S[(ζi(t)+Γi)T(ζi(t)+Γi)−ζTi(t)ζi(t)−2ζTi(t)Γi]λ(du)=2l∑i=1−aiζTi(t)ζi(t)+2l∑i=1ζTi(t)l∑j=1ςij˜F(ζj(t))+2l∑i=1ζTi(t)l∑j=1νij˜F(ζj(t−h))+2l∑i=1ζTi(t)l∑j=1θij∫tt−h˜F(ζj(s))ds+2l∑i=1ζTi(t)l⋀j=1mij˜G(ζj(t−h))+2l∑i=1ζTi(t)l⋁j=1ιij˜G(ζj(t−h))+2l∑i=1ζTi(t)l⋀j=1oij∫tt−h˜G(ζj(s))ds+2l∑i=1ζTi(t)l⋁j=1ξij∫tt−h˜G(ζj(s))ds+2l∑i=1ζTi(t)ui(t)+l∑i=1Tr(WTiWi)+l∑i=1∫S[(ζi(t)+Γi)T(ζi(t)+Γi)−ζTi(t)ζi(t)−2ζTi(t)Γi]λ(du). | (2.5) |
By calculation, we can write the expression for LV (2.5) as LV=∑10i=1LVi, where
LV1=2l∑i=1ζTi(t)l∑j=1ςij˜F(ζj(t)),LV2=2l∑i=1ζTi(t)l∑j=1νij˜F(ζj(t−h)),LV3=2l∑i=1ζTi(t)l⋀j=1mij˜G(ζj(t−h)),LV4=2l∑i=1ζTi(t)l⋁j=1ιij˜G(ζj(t−h)),LV5=2l∑i=1ζTi(t)l∑j=1θij∫tt−h˜F(ζj(s))ds,LV6=2l∑i=1ζTi(t)(l⋀j=1oij∫tt−h˜G(ζj(s))ds+l⋁j=1ξij∫tt−h˜G(ζj(s))ds),LV7=2l∑i=1ζi(t)ui(t),LV8=l∑i=1∫S[(ζi(t)+Γi)T(ζi(t)+Γi)−ζTi(t)ζi(t)−2ζTi(t)Γi]λ(du)LV9=2l∑i=1−aiζTi(t)ζi(t),LV10=l∑i=1Tr(WTiWi). |
Now, we use the inequality scaling for each part of LV. From Assumption 2.3, we can obtain that
LV1≤2l∑i=1|ζi(t)|l∑j=1ςijL1|ζj(t)|≤2l∑i,j=1ςijL1|ζi(t)||ζj(t)|≤l∑i,j=1(ςijL1|ζi(t)|2+ςijL1|ζj(t)|2)≤l∑i,j=1ςijL1|ζi(t)|2+l∑i,j=1ςjiL1|ζi(t)|2≤l∑i,j=1(ςijL1+ςjiL1)|ζi(t)|2, | (2.6) |
and
LV2≤2l∑i=1|ζi(t)|l∑j=1νijL1|ζj(t−h)|≤2l∑i,j=1νijL1|ζi(t)||ζj(t−h)|. | (2.7) |
By using Assumption 2.3 and Lemma 2.5, one has
LV3≤2l∑i=1|ζi(t)|l∑j=1|mij||˜G(ζj(t−h))|≤2l∑i,j=1|mij|L2|ζi(t)||(ζj(t−h))|, | (2.8) |
and
LV4≤2l∑i=1|ζi(t)|l∑j=1|ιij||˜G(ζj(t−h))|≤2l∑i,j=1|ιij|L2|ζi(t)||(ζj(t−h))|. | (2.9) |
In the same way, we have
LV5≤2l∑i,j=1θij|ζi(t)|∫tt−h˜F(ζj(s))ds≤l∑i,j=1θij(|ζi(t)|2+(∫tt−h˜F(ζj(s))ds)2)≤l∑i,j=1(θij|ζi(t)|2+θijh∫tt−h(˜F(ζj(s)))2ds)≤l∑i,j=1(θij|ζi(t)|2+θijh(L1)2∫tt−h|ζj(s)|2ds), | (2.10) |
and
LV6≤2l∑i=1ζTi(t)(l∑j=1oij∫tt−h˜G(ζj(s))ds+l∑j=1ξij∫tt−h˜G(ζj(s))ds)≤l∑i,j=1((oij+ξij)|ζi(t)|2+oijh(L2)2∫tt−h|ζj(s)|2ds+ξijh(L2)2∫tt−h|ζj(s)|2ds). | (2.11) |
In addition, it follows from Lemma 2.6, Assumptions 2.2 and 2.4 that
LV7≤−2l∑i=1ℵi|ζi(t)|2−2l∑i,j=1ωij|ζi(t)||ζj(t−h)|−2μ1(l∑i=1ζTi(t)ζi(t))1+q2−2η1l1−M(l∑i=1ζTi(t)ζi(t))M−2η1l1−m(l∑i=1ζTi(t)ζi(t))m−l∑i,j=1ϖij∫tt−h|ζi(s)|2ds, | (2.12) |
LV8≤l∑i=1∫S[|ζi(t)+Γi|2−|ζi(t)|2−2ζTi(t)Γi]λ(du)≤l∑i=1∫SΓ2iλ(du)≤l∑i=1∫Sφ2i|ζmii(t)|2λ(du)=ˉλl∑i=1φ2i|ζmii(t)|2, | (2.13) |
and
LV10≤l∑i=1ϰi|ζi(t)|2. | (2.14) |
Substituting (2.6)–(2.14) into LV=∑10i=1LVi, we have
LV≤l∑i=1(−2ai−2Ki+l∑j=1(ςijL1+ςjiL1+θij+oij+ξij)+ϰi)|ζi(t)|2+2l∑i,j=1(νijL1+|mij|L2+|ιij|L2−ωij)|ζi(t)||ζj(t−h)|+l∑i,j=1(hbji(L1)2+hsji(L2)2+hcji(L2)2−ϖij)∫tt−h|ζi(s)|2ds−2μ1(l∑i=1ζTi(t)ζi(t))1+q2−2η1l1−M(l∑i=1ζTi(t)ζi(t))M−2η1l1−m(l∑i=1ζTi(t)ζi(t))m+ˉλl∑i=1φ2i|ζmii(t)|2. |
Further, if the conditions in Theorem 2.7 hold, we can obtain that
LV≤−2μ1(l∑i=1ζTi(t)ζi(t))1+q2−2η1l1−M(l∑i=1ζTi(t)ζi(t))M−2η1l1−m(l∑i=1ζTi(t)ζi(t))m+ˉλl∑i=1φ2i|ζmii(t)|2. | (2.15) |
Let ˉφ=max[φ2i], M=max[mi], m=min[mi] and recall that V(ζ(t))=∑li=1ζTi(t)ζi(t). Then
ˉλl∑i=1φ2i|ζmii(t)|2≤ˉλl∑i=1ˉφ|ζmii(t)|2≤ˉλl∑i=1ˉφ(ζTi(t)ζi(t))mi≤ˉλˉφl∑i=1[V(ζ(t))]mi≤ˉλˉφl∑i=1[(V(ζ(t)))M+(V(ζ(t)))m]≤ˉλlˉφ[(V(ζ(t)))M+(V(ζ(t)))m]. | (2.16) |
Substituting (2.16) into the expression for (2.15), we have
LV≤−2μ1(V(ζ(t)))1+q2−2η1l1−M(V(ζ(t)))M−2η1l1−m(V(ζ(t)))m+ˉλl∑i=1φ2i(V(ζ(t)))mi≤−2μ1(V(ζ(t)))1+q2−2η1l1−M(V(ζ(t)))M−2η1l1−m(V(ζ(t)))m+ˉλlˉφ[(V(ζ(t)))M+(V(ζ(t)))m]≤−2μ1(V(ζ(t)))1+q2−(2η1l1−M−ˉλlˉφ)(V(ζ(t)))M−(2η1l1−m−ˉλlˉφ)(V(ζ(t)))m≤−2μ1(V(ζ(t)))1+q2−(2η1l1−M−ˉλlˉφ)(V(ζ(t)))M≤−ˆμ1(V(ζ(t)))Q−ˆη1(V(ζ(t)))M, | (2.17) |
where ˆμ1=2μ1, Q=1+q2, ˆη1=2η1l1−M−ˉλlˉφ.
The next step is to estimate the settling time. According to [44], since the V(ζ) is a positive and radially unbounded function, there are two K∞ class functions ~μ1 and ~μ2 such that ~μ1(|ζ|)≤V(ζ)≤~μ2(|ζ|) for any ζ∈Rl.
Let r>0 and 0<ε<1 be arbitrary, and define σr=inf{t;|ζ(t;ζ0)|>r}, where ζ0=β(0)−α(0). By supermartingale inequality,
P(σr≤t)~μ1(r)≤E[Iσr≤tV(ζ(σr))]≤E[V(ζ(t∧σr))]≤V(ζ0)≤~μ2(|ζ0|). |
Taking δ=~μ2−1(~μ1(r)ε), we can obtain that P(σr≤t)≤ε when |ζ0|≤δ. Let t→∞, then we have P(σr≤∞)≤ε, which implies that P(supt≥0|ζ(t;ζ0)|≤r)≥1−ε.
Next, we prove the fixed-time attraction in probability. Structure a function
R(V(ζ))=∫V(ζ)01ˆμ1sQ+ˆη1sMds. |
The function R(V(ζ)) is positive definite and twice continuously differentiable in Rl∖{0}. For any initial condition ζ0∈Rl∖{0}, the stopping time is characterized as hk=inf{t≥0;|ζ(t;ζ0)|∉(1k,k)}. For t≤hk,
R(V(ζ(t∧hk)))=R(V(ζ0))+∫t∧hk0LR(V(ζ(s)))ds+∫t∧hk01ˆμ1VQ+ˆη1VM∂V∂ζ˜g(ζ(s))dB(s)+∫t∧hk0L(1)R(V(ζ(s)))˜Nd(dt,du). | (2.18) |
Since 1ˆμ1VQ+ˆη1VM∂V∂ζ˜g(ζ(s)) is bounded in interval 1k<|ζ|<k, we have
E[1ˆμ1VQ+ˆη1VM∂V∂ζ˜g(ζ(s))]=0. |
Taking expectations on both sides of (2.18), it follows from E[∫t∧hk0L(1)R(V(ζ(s)))˜Nd(dt,du)]=0 that
E[R(V(ζ(t∧hk)))]=R(V(ζ0))+∫t∧hk0LR(V(ζ(s)))ds, | (2.19) |
where
LR(V(ζ(t)))=1ˆμ1VQ+ˆη1VM⋅LV(ζ(t))−12⋅ˆμ1QVQ−1+ˆη1MVM−1(ˆμ1VQ+ˆη1VM)2⋅Tr[(∂V∂ζ˜g(ζ))T(∂V∂ζ˜g(ζ))]+∫S[∫V(ζ+γ)V(ζ)1ˆμ1sQ+ˆη1sMds−1ˆμ1VQ+ˆη1VM(V(ζ+γ)−V(ζ))]λ(du). |
Because the function V(ζ) is positive definite and ˆμ1,ˆη1,Q,M are positive constants, we have
12⋅ˆμ1QVQ−1+ˆη1MVM−1(ˆμ1VQ+ˆη1VM)2⋅Tr[(∂V∂ζ˜g(ζ))T(∂V∂ζ˜g(ζ))]>0. |
Note that ˆμ1VQ+ˆη1VM is positive and increasing. Thus
∫S[∫V(ζ+γ)V(ζ)1ˆμ1sQ+ˆη1sMds−1ˆμ1VQ+ˆη1VM(V(ζ+γ)−V(ζ))]λ(du)=∫S[(1ˆμ1V(ζ∗)Q+ˆη1V(ζ∗)M−1ˆμ1VQ+ˆη1VM)(V(ζ+γ)−V(ζ))]λ(du)<0, |
where ζ∗∈(ζ,ζ+γ) when γ>0 and ζ∗∈(ζ+γ,ζ) when γ<0. According to (2.17), we can obtain that
LR(V(ζ(t)))≤1ˆμ1VQ+ˆη1VM⋅LV(ζ(t))≤−1. |
It follows from (2.19) that
E[R(V(ζ(t∧hk)))]≤R(V(ζ0))−(t∧hk), |
which implies
E[R(V(ζ(t∧hk)))]≤R(V(ζ0)). |
Let k→∞, then we have t∧hk→Tϵ a.s. Thus the settling time could be obtained as
Tϵ=E[T(ζ,ϵ)]≤R(V(ζ0))=∫V(ζ0)01ˆμ1sQ+ˆη1sMds≤1ˆμ111−Q+1ˆη11M−1. |
That is Tϵ<+∞ and independent of the initial state.
Although feedback control has many advantages, it does not mean that other control methods are meaningless. The fixed structure of feedback controller will not only make the design and implementation simple but also lead to the waste of resources. For example, the feedback gain coefficient is usually so large that it would result in an unreasonable use of resources. In order to avoid such waste of resources, the adaptive controller has been proposed. The adaptive controller constantly measures the states of the system and makes decisions to change the structure and parameters of the controller. Or, it changes the control rules according to the adaptive laws. The main purpose of this controller is to make the system achieve the desired states with less consumption by changing the control rules. Compared with the feedback control, the design of the adaptive control is complicated, its calculation is larger, and its synchronization criterion is more challenging to deduce. However, if the adaptive control scheme is feasible, it can achieve the same goal with a smaller control input. Moreover, the adaptive control generally has better stability and robustness than the feedback control. The comparison of the feedback controller and the adaptive controller can be seen in Table 1 below.
Controller | Gains | Calculation | Design | Synchronization time | Consume resources | Stability |
feedback controller | fixed | simple | simple | more | more | weak |
adaptive controller | dynamic | complex | complex | less | fewer | strong |
Next, we design an adaptive controller to synchronize FSCNNs within a fixed time as follows:
ui(t)={−ℵi(t)ζi(t)−l∑j=1[ωij|ζj(t−h)|sign(ζi(t))−12ϖij∫tt−h|ζi(s)|2dsζi(t)|ζi(t)|2]−μ2|ζi(t)|qsign(ζi(t))−η2[|ζi(t)|2M−1+|ζi(t)|2m−1]sign(ζi(t)),ifζi(t)≠0,0,ifζi(t)=0, | (2.20) |
where ℵi(t) is the adaptive adjustment feedback gain, and the designed adaptive rate is
˙ℵi(t)=ζTi(t)ζi(t)−μ2sign(ℵi(t)−ℵ1)(ℵi(t)−ℵ1)q−η2sign(ℵi(t)−ℵ1)[(ℵi(t)−ℵ1)2M−1+(ℵi(t)−ℵ1)2m−1], | (2.21) |
where ℵ1 is a constant to be determined.
Theorem 2.8. Assume that the Assumptions 2.2–2.4 hold. The systems (2.1) and (2.2) are stochastic fixed-time synchronization under the adaptive control (2.20) with adaptive rate (2.21), if the following four conditions hold:
−2ai−2ℵ1+ϰi+l∑j=1(L1ςij+L1ςji+θij+oij+ξij)≤0,2l∑j=1(|L1|νij+|mij|L2+|ιij|L2−ωij)≤0,l∑j=1(hbji(L1)2+hsji(L2)2+hcji(L2)2−ϖij)≤0,(2η2l1−M−ˉλlˉφ)21−M>0. |
And the settling time is
Tϵ=1ˆμ211−Q+1ˆη21M−1, |
where ˆμ2=2μ2 and ˆη2=(2η2l1−M−ˉλlˉφ)21−M.
Proof. We structure the Lyapunov function V(t,ζ(t))=∑li=1[ζTi(t)ζi(t)+(ℵi(t)−ℵ1)2]. According to the Itˆo formula, we have
LV=2l∑i=1ζTi(t)[−aiζi(t)+l∑j=1(ςij˜F(ζj(t))+νij˜F(ζj(t−h))+θij∫tt−h˜F(ζj(s))ds)+ui(t)+l⋀j=1mij˜G(ζj(t−h))+l⋁j=1ιij˜G(ζj(t−h))+l⋀j=1oij∫tt−h˜G(ζj(s))ds+l⋁j=1ξij∫tt−h˜G(ζj(s))ds]+l∑i=1Tr(WTiWi)+2l∑i=1(ℵi(t)−ℵ1)⋅˙ℵi(t)+l∑i=1∫S[(ζi(t)+γi)T(ζi(t)+γi)−ζTi(t)ζi(t)−2ζTi(t)γi]λ(du)=2l∑i=1−aiζTi(t)ζi(t)+2l∑i=1ζTi(t)l∑j=1ςij˜F(ζj(t))+2l∑i=1ζTi(t)l∑j=1νij˜F(ζj(t−h))+2l∑i=1ζTi(t)l∑j=1θij∫tt−h˜F(ζj(s))ds+2l∑i=1ζTi(t)l⋀j=1mij˜G(ζj(t−h))+2l∑i=1ζTi(t)l⋁j=1ιij˜G(ζj(t−h))+2l∑i=1ζTi(t)l⋀j=1oij∫tt−h˜G(ζj(s))ds+2l∑i=1ζTi(t)l⋁j=1ξij∫tt−h˜G(ζj(s))ds+2l∑i=1ζTi(t)ui(t)+l∑i=1Tr(WTiWi)+2l∑i=1(ℵi(t)−ℵ1)⋅˙ℵi(t)+l∑i=1∫S[(ζi(t)+Γi)T(ζi(t)+Γi)−ζTi(t)ζi(t)−2ζTi(t)Γi]λ(du). | (2.22) |
Comparing (2.5) and (2.22), we can find that the terms in (2.22) are all scaled in the proof of Theorem 2.7, except for the term
2l∑i=1ζTi(t)ui(t)+2l∑i=1(ℵi(t)−ℵ1)⋅˙ℵi(t). |
By using (2.21), we obtain that
2l∑i=1ζTi(t)ui(t)+2l∑i=1(ℵi(t)−ℵ1)⋅˙ℵi(t)≤2l∑i=1ζTi(t)(−ℵi(t)ζi(t)−l∑j=1(ωij|ζj(t−h)|−12ϖij∫tt−h|ζi(s)|2dsζi(t)|ζi(t)|2)−μ2|ζi(t)|q(ζi(t))−η2[|ζi(t)|2M−1+|ζi(t)|2m−1](ζi(t)))+2l∑i=1(ℵi(t)−ℵ1)⋅(ζTi(t)ζi(t)−μ2(ℵi(t)−ℵ1)(ℵi(t)−ℵ1)q−η2(ℵi(t)−ℵ1)[(ℵi(t)−ℵ1)2M−1+(ℵi(t)−ℵ1)2m−1])≤2l∑i=1ζTi(t)(−(ℵi(t)−ℵ1)ζi(t)−l∑j=1(ωij|ζj(t−h)|−12ϖij∫tt−h|ζi(s)|2dsζi(t)|ζi(t)|2)−μ2|ζi(t)|q(ζi(t))−η2[|ζi(t)|2M−1+|ζi(t)|2m−1](ζi(t)))+2l∑i=1(ℵi(t)−ℵ1)⋅(ζTi(t)ζi(t)−μ2(ℵi(t)−ℵ1)(ℵi(t)−ℵ1)q−η2(ℵi(t)−ℵ1)[(ℵi(t)−ℵ1)2M−1+(ℵi(t)−ℵ1)2m−1])−2l∑i=1ℵ1ζTi(t)ζi(t). |
Therefore, it follows from Lemma 2.6 that
2l∑i=1ζTi(t)ui(t)+2l∑i=1(ℵi(t)−ℵ1)⋅˙ℵi(t)≤−2l∑i,j=1ωij|ζi(t)||ζj(t−h)|−l∑i,j=1ϖij∫tt−h|ζi(s)|2ds−2μ2(l∑i=1ζTi(t)ζi(t))1+q2−2η2l1−M(l∑i=1ζTi(t)ζi(t))M−2η2l1−m(l∑i=1ζTi(t)ζi(t))m−2μ2l∑i=1(ℵi(t)−ℵ1)1+q−2η2l1−M(l∑i=1(ℵi(t)−ℵ1)2)M−2η2l1−m(l∑i=1(ℵi(t)−ℵ1)2)m−2l∑i=1ℵ1ζTi(t)ζi(t). | (2.23) |
Combined with (2.6)–(2.14), (2.16), and (2.23), we have
LV≤l∑i=1(−2ai−2ℵ1+l∑j=1(ςijL1+ςjiL1+θij+oij+ξij)+ϰij)|ζi(t)|2+2l∑i,j=1(νijL1+|mij|L2+|ιij|L2−ωij)|ζi(t)||ζj(t−h)|+l∑i,j=1(hbji(L1)2+hsji(L2)2+hcji(L2)2−ϖij)∫tt−h|ζi(s)|2ds−2μ2(l∑i=1ζTi(t)ζi(t))1+q2−2η2l1−M(l∑i=1ζTi(t)ζi(t))M−2η2l1−m(l∑i=1ζTi(t)ζi(t))m−2μ2(l∑i=1(ℵi(t)−ℵ1)2)1+q2−2η2l1−M(l∑i=1(ℵi(t)−ℵ1)2)M−2η2l1−m(l∑i=1(ℵi(t)−ℵ1)2)m+ˉλlˉφ(l∑i=1ζTi(t)ζi(t))M+ˉλlˉφ(l∑i=1ζTi(t)ζi(t))m. |
Assume that the conditions in Theorem 2.8 hold, then
LV≤−2μ2(l∑i=1ζTi(t)ζi(t))1+q2−2η2l1−M(l∑i=1ζTi(t)ζi(t))M−2η2l1−m(l∑i=1ζTi(t)ζi(t))m−2μ2(l∑i=1(ℵi(t)−ℵ1)2)1+q2−2η2l1−M(l∑i=1(ℵi(t)−ℵ1)2)M−2η2l1−m(l∑i=1(ℵi(t)−ℵ1)2)m+ˉλlˉφ(l∑i=1ζTi(t)ζi(t))M+ˉλlˉφ(l∑i=1ζTi(t)ζi(t))m. |
Thus, it follows from Lemma 2.6 that
LV≤−2μ2(l∑i=1ζTi(t)ζi(t))1+q2−2η2l1−M(l∑i=1ζTi(t)ζi(t))M−2η2l1−m(l∑i=1ζTi(t)ζi(t))m−2μ2(l∑i=1(ℵi(t)−ℵ1)2)1+q2−2η2l1−M(l∑i=1(ℵi(t)−ℵ1)2)M−2η2l1−m(l∑i=1(ℵi(t)−ℵ1)2)m+ˉλlˉφ(l∑i=1ζTi(t)ζi(t))M+ˉλlˉφ(l∑i=1ζTi(t)ζi(t))m+ˉλlˉφ(l∑i=1(ℵi(t)−ℵ1)2)M+ˉλlˉφ(l∑i=1(ℵi(t)−ℵ1)2)m≤−2μ2(l∑i=1ζTi(t)ζi(t))1+q2−2η2l1−M(l∑i=1ζTi(t)ζi(t))M−2μ2(l∑i=1(ℵi(t)−ℵ1)2)1+q2−2η2l1−M(l∑i=1(ℵi(t)−ℵ1)2)M+ˉλlˉφ(l∑i=1ζTi(t)ζi(t))M+ˉλlˉφ(l∑i=1(ℵi(t)−ℵ1)2)M≤−2μ2(l∑i=1(ζTi(t)ζi(t)+(ℵi(t)−ℵ1)2))1+q2−(2η2l1−M−ˉλlˉφ)21−M(l∑i=1(ζTi(t)ζi(t)+(ℵi(t)−ℵ1)2))M≤−2μ2(V(ζ(t)))1+q2−(2η2l1−M−ˉλlˉφ)21−M(V(ζ(t)))M≤−ˆμ2(V(ζ(t)))Q−ˆη2(V(ζ(t)))M, |
where ˉφ=max[φ2i], M=max[mi], m=min[mi], ˆμ2=2μ2, Q=1+q2, ˆη2=(2η2l1−M−ˉλlˉφ)21−M. By using a similar approach in the proof of Theorem 2.7, we can easily obtain taht the settling time is as follows:
Tϵ=1ˆμ211−Q+1ˆη21M−1. |
In this section, two detailed numerical simulations are provided to demonstrate the validity of our results. First, we give the simulation process:
Step 1. Take the values of the system parameters including the connection weights of coefficient matrix.
Step 2. Define the initial values of the drive and response systems.
Step 3. Generate discrete time nodes. Randomly generate white noise perturbations and the time nodes of Lˊevy jumps. Merge them with the original time series to obtain a new time series.
Step 4. Treatment of fuzzy terms.
Step 5. Establish the iterative format of the drive system.
Step 6. Compute control item and establish the iterative format of the response system.
Step 7. Obtain the error data by iteration.
Let l=8, then the drive system is as follows:
dαi(t)=[−aiαi(t)+8∑j=1(ςij˜f(αj(t))+νij˜f(αj(t−h))+θij∫tt−h˜f(αj(s))ds)+8⋀j=1mij˜g(αj(t−h))+8⋁j=1ιij˜g(αj(t−h))+8⋀j=1oij∫tt−h˜g(αj(s))ds+8⋁j=1ξij∫tt−h˜g(αj(s))ds+8⋀j=1Gijpj+8⋁j=1Hijpj+Di]dt+wi(αi(t),t)dB(t)+∫Sγi(αi(t),t,u)˜N(dt,du). | (3.1) |
ai=1.6, h=0.01, Di=0, L1=L2=1, ˜f(⋅)=˜g(⋅)=tan(⋅), pj=0.1, Gij=Hij=1, w1=3α1(t), w2=3.5α2(t), w3=3α3(t), w4=4.5α4(t), w5=−3α5(t), w6=−3.5α6(t), w7,t)=−4α7(t), w8=−4.5α8(t), γ1=log(1+α1(t)), γ2=α2(t)log(1+α2(t)), γ3=α3(t)12log(1+α3(t)), γ4=log(1+α4(t)), γ5=α5(t)log(1+α5(t)), γ6=α6(t)12log(1+α6(t)), γ7=log(1+α7(t)), γ8=α8(t)log(1+α8(t)), and
(ςij)8×8=0.3∗[000.6000000.600000.50000.7000.500000.50000000000000.600000.6000.50000.500000.6000000.600], |
(νij)8×8=0.2∗[000.6000000.600000.50000.7000.500000.50000000000000.600000.6000.50000.500000.6000000.600], |
(θij)8×8=0.4∗[000.6000000.600000.50000.7000.500000.50000000000000.600000.6000.50000.500000.6000000.600], |
(mij)8×8=0.4∗[000.6000000.600000.50000.7000.500000.50000000000000.600000.6000.50000.500000.6000000.600], |
(ιij)8×8=(oij)8×8=0.2∗[00.7000000000.50.600000.5000000.70000.7000.600000.80000000.5000000.700000.70.6000000000.80], |
(ξij)8×8=1.3∗[00.3000000000.30.300000.3000000.20000.2000.100000.30000000.1000000.300000.30.2000000000.30]. |
The response system is
dβi(t)=[−aiβi(t)+8∑j=1(ςij˜f(βj(t))+νij˜f(βj(t−h))+θij∫tt−h˜f(βj(s))ds)+8⋀j=1mij˜g(βj(t−h))+8⋁j=1ιij˜g(βj(t−h))+8⋀j=1oij∫tt−h˜g(βj(s))ds+8⋁j=1ξij∫tt−h˜g(βj(s))ds+8⋀j=1Gijpj+8⋁j=1Hijpj+Di+ui(t)]dt+wi(βi(t),t)dB(t)+∫Sγi(βi(t),t,u)˜N(dt,du), | (3.2) |
w1=3β1(t), w2=3.5β2(t), w3=3β3(t), w4=4.5β4(t), w5=−3β5(t), w6=−3.5β6(t), w7=−4β7(t), w8=−4.5β8(t), γ1=log(1+β1(t)), γ2=β2(t)log(1+β2(t)), γ3=β3(t)12log(1+β3(t)), γ4=log(1+β4(t)), γ5=β5(t)log(1+β5(t)), γ6=β6(t)12log(1+β6(t)), γ7=log(1+β7(t)), γ8=β8(t)log(1+β8(t)). Thus, we can obtain that the error system is
dζi(t)=[−aiζi(t)+8∑j=1(ςij˜F(ζj(t))+νij˜F(ζj(t−h))+θij∫tt−h˜F(ζj(s))ds)+8⋀j=1mij˜G(ζj(t−h))+8⋁j=1nij˜G(ζj(t−h))+8⋀j=1oij∫tt−h˜G(ζj(s))ds+8⋁j=1ξij∫tt−h˜G(ζj(s))ds+ui(t)]dt+WidB(t)+∫SΓi(ζi(t),t,u)˜N(dt,du). | (3.3) |
Example 3.1. Consider the effectiveness of fixed-time synchronization of CNNs with Lˊevy noise by under the influence of feedback control.
The feedback control is given by
ui(t)={−ℵiζi(t)−8∑j=1ωij|ζj(t−h)|sign(ζi(t))−128∑j=1ϖij∫tt−h|ζi(s)|2dsζi(t)|ζi(t)|2−μ1|ζi(t)|qsign(ζi(t))−η1[|ζi(t)|2M−1+|ζi(t)|2m−1]sign(ζi(t)),ifζi(t)≠0,0,ifζi(t)=0. | (3.4) |
where ℵi=50, μ1=0.5, η1=0.6, ϖii=4, q=0.1, M=3, m=1.1, ωij=ξij, and all other values not mentioned are zero.
It is not difficult to verify that all the conditions in Theorem 2.7 can be satisfied. The trajectories of the drive system (3.1) and the response system (3.2) are shown in Figures 2 and 3, respectively.
The trajectories of the error system (3.3) with and without feedback control (3.4) are shown in Figures 4 and 5, respectively.
In Figure 4, it is obvious that the trajectory of the error system (3.3) tends to zero at a fixed time, which implies that the drive system (3.1) and the response system (3.2) are synchronized at a fixed time. This result shows that Theorem 2.7 is valid. Moreover, Figure 5 shows that the error system (3.3) in the absence of control does not tend to zero, which implies that the drive system (3.1) and the response system (3.2) cannot achieve synchronization.
Example 3.2. Consider the effectiveness of fixed-time synchronization of CNNs with Lˊevy noise under adaptive control.
The adaptive control is
ui(t)={−ℵi(t)ζi(t)−8∑j=1(ωij|ζj(t−h)|sign(ζi(t))−12ϖij∫tt−h|ζi(s)|2dsζi(t)|ζi(t)|2)−μ2|ζi(t)|qsign(ζi(t))−η2[|ζi(t)|2M−1+|ζi(t)|2m−1]sign(ζi(t)),ifζi(t)≠0,0,ifζi(t)=0, | (3.5) |
and the adaptive rate is
˙ℵi(t)=ζTi(t)ζi(t)−μ2sign(ℵi(t)−ℵ1)(ℵi(t)−ℵ1)q−η2sign(ℵi(t)−ℵ1)[(ℵi(t)−ℵ1)2M−1+(ℵi(t)−ℵ1)2m−1], | (3.6) |
where ℵ1=90, μ2=0.5, η2=0.6, ϖii=4, q=0.1, M=1.4, m=1.1, ωij=ξij, and all other values not mentioned are zero.
It is easy to verify that all the conditions in Theorem 2.8 can be satisfied. The trajectories of the drive system (3.1), the response system (3.2), and the error system (3.3) under the adaptive control (3.6) are shown in Figures 6–8, respectively.
In Figure 8, it is obvious that the trajectory of the error system (3.3) tends to zero at a fixed time, which implies that the drive system (3.1) and the response system (3.2) are synchronized in a fixed time. This result shows that Theorem 2.8 is valid.
In addition, we can also summarize some rules from the above simulation results. It takes about two units of time for the adaptive controller to synchronize the drive system (3.1) and the response system (3.2), while it takes about three units of time for the feedback controller. This shows that the adaptive controller can synchronize a system faster than the feedback controller.
Based on the Lyapunov theory and the Itˆo formula, we research the fixed-time synchronization of FSCNNs with mixed delays driven by white noise and Lˊevy noise. Theorem 2.7 shows a sufficient criterion for fixed-time synchronization of FSCNNs under a feedback control and a method to compute the stabilization time. In addition, in order to reduce the consumption of resources, we design an adaptive controller. By Theorem 2.8, we obtain a sufficient criterion for fixed-time synchronization of FSCNNs under the adaptive control and the upper bound of the stabilization time. The results show that when the intensities of white noise and Lˊevy noise are small, FSCNNs can achieve synchronization through appropriate control means.
At present, there are many works on the synchronization problem of stochastic neural networks with continuous random perturbations (white noise). However, there are few studies on the synchronization problem of stochastic neural networks with Levy noise. In fact, discontinuous random perturbations (Lˊevy noise) should also be considered, which can be used to describe sudden external influences. Moreover, many works on neural network synchronization focus on asymptotic synchronization. But the infinite synchronization time cannot be used for practical problems in engineering. People are more likely to expect neural networks to be synchronized in a finite time. Since the initial state of a neural network is generally difficult to master, the fixed-time synchronization that does not depend on the initial state of the system is more practical. Our results can be applied to neural networks with Lˊevy noise and obtain a synchronization time independent of the initial state of the system, which complements the synchronization theory of stochastic neural networks.
There are many interesting questions that deserve to be investigated for the fixed-time synchronization of FSCNNs with Lˊevy noise. In this paper, we use two kinds of control methods: the feedback control and the adaptive control. There are many other control methods that are useful and efficient. The effectiveness of other control methods such as pinning control, intermittent control, and state observation control is also worth studying. Moreover, the parameters of the neural networks in this paper are assumed to be constants. In fact, the time-varying parameters and parameters with switching characteristics such as Markov switch and semi-Markov switch should also be considered. These time-varying and switching parameters can make the dynamic behavior of the system more complex. The construction of the Lyapunov function will be more difficult, and the derivation of the synchronization theorem is not known to be feasible. Of course, we have only theoretically demonstrated the synchronization criteria for FSCNNs with Lˊevy noise. How to apply theoretical results to practical problems is also a very challenging problem for us. The difficulties for the practical application of the synchronization theorem mainly focus on the technical realization, environmental factors, and system complexity. In order to overcome these difficulties, it is necessary to use a variety of technical means, and optimize the design according to the specific application scenario.
The authors declare they have not used Artificial Intelligence (AI) tools in the creation of this article.
This work is supported by the Natural Science Foundation of Heilongjiang Province (No. LH2022A002) and the National Natural Science Foundation of China (No. 12371160).
The authors declare there is no conflict of interest.
[1] |
J. L. Wang, L. H. Zhao, PD and PI control for passivity and synchronization of coupled neural networks with multi-weights, IEEE Trans. Network Sci. Eng., 8 (2021), 790–802. https://doi.org/10.1109/TNSE.2021.3052889 doi: 10.1109/TNSE.2021.3052889
![]() |
[2] |
X. W. Liu, T. P. Chen, Synchronization of nonlinear coupled networks via aperiodically intermittent pinning control, IEEE Trans. Neural Networks Learn. Syst., 26 (2015), 113–126. https://doi.org/10.1109/TNNLS.2014.2311838 doi: 10.1109/TNNLS.2014.2311838
![]() |
[3] |
K. B. Shi, Y. Y. Tang, S. M. Zhong, C. Yin, X. G. Huang, W. Q. Wang, Nonfragile asynchronous control for uncertain chaotic Lurie network systems with Bernoulli stochastic process, Int. J. Robust Nonlinear Control, 28 (2018), 1693–1714. https://doi.org/10.1002/rnc.3980 doi: 10.1002/rnc.3980
![]() |
[4] |
J. X. Lv, X. Z. Ju, C. H. Wang, Neural network prescribed-time observer-based output-feedback control for uncertain pure-feedback nonlinear systems, Expert Syst. Appl., 264 (2024). https://doi.org/10.1016/j.eswa.2024.125813 doi: 10.1016/j.eswa.2024.125813
![]() |
[5] |
D. Y. Li, S. Tong, H. Y. Yang, Q. L. Hu, Time-synchronized control for spacecraft reorientation with time-varying constraints, IEEE-ASME Trans. Mechatron., 2024 (2024), 1–11. https://doi.org/10.1109/TMECH.2024.3430953 doi: 10.1109/TMECH.2024.3430953
![]() |
[6] |
X. Y. Zhang, Y. H. Liu, C. Y. Su, Adaptive pseudoinverse control for constrained hysteretic nonlinear systems and its application on dielectric elastomer actuator, IEEE-ASME Trans. Mechatron., 28 (2023), 2142–2154. https://doi.org/10.1109/TMECH.2022.3231263 doi: 10.1109/TMECH.2022.3231263
![]() |
[7] |
C. Q. Guo, J. P. Hu, S. Celikovsky, Non-singular fixed-time tracking control of uncertain nonlinear pure-feedback systems with practical state constraints, IEEE Trans. Circuits Syst. I Regul. Pap., 70 (2023), 3746–3758. https://doi.org/10.1109/TCSI.2023.3291700 doi: 10.1109/TCSI.2023.3291700
![]() |
[8] |
L. O. Chua, L. Yang, Cellular neural networks theory, IEEE Trans. Circuits Syst., 35 (1988), 1257–1272. https://doi.org/10.1109/31.7600 doi: 10.1109/31.7600
![]() |
[9] | T. Yang, L. B. Yang, C. W. Wu, L. O. Chua, Fuzzy cellular neural networks: Applications, in 1996 Fourth IEEE International Workshop on Cellular Neural Networks and Their Applications Proceedings (CNNA-96), (1996), 225–230. https://doi.org/10.1109/cnna.1996.566560 |
[10] |
K. Ratnavelu, M. Kalpana, P. Balasubramaniam, K. Wong, P. Raveendran, Image encryption method based on chaotic fuzzy cellular neural networks, Signal Process., 140 (2017), 87–96. https://doi.org/10.1016/j.sigpro.2017.05.002 doi: 10.1016/j.sigpro.2017.05.002
![]() |
[11] |
J. Karbowski, N. Kopell, Multispikes and synchronization in a large neural network with temporal delays, Neural Comput., 12 (2000), 1573–1606. https://doi.org/10.1162/089976600300015277 doi: 10.1162/089976600300015277
![]() |
[12] |
J. D. Cao, J. Q. Lu, Adaptive synchronization of neural networks with or without time-varying delay, Chaos, 16 (2006). https://doi.org/10.1063/1.2178448 doi: 10.1063/1.2178448
![]() |
[13] |
Y. R. Liu, Z. D. Wang, J. L. Liang, X. H. Liu, Synchronization and state estimation for discrete-time complex networks with distributed delays, IEEE Tran. Syst. Man Cybern. Part B Cybern., 38 (2008), 1314–1325. https://doi.org/10.1109/TSMCB.2008.925745 doi: 10.1109/TSMCB.2008.925745
![]() |
[14] |
L. L. Hou, P. F. Ma, X. Ma, H. B. Sun, H∞ Exponential synchronization of switched cellular neural networks based on disturbance observer-based control, Int. J. Control Autom. Syst., 22 (2024), 1430–1441. https://doi.org/10.1007/s12555-022-0917-7 doi: 10.1007/s12555-022-0917-7
![]() |
[15] |
T. Y. Jing, T. Y. He, Synchronization of fractional-order neural networks via intermittent quantized control: Optimal algorithm, Int. J. Theor. Phys., 63 (2024). https://doi.org/10.1007/s10773-024-05701-z doi: 10.1007/s10773-024-05701-z
![]() |
[16] |
X. S. Yang, J. D. Cao, Finite-time stochastic synchronization of complex networks, Appl. Math. Modell., 34 (2010), 3631–3641. https://doi.org/10.1016/j.apm.2010.03.012 doi: 10.1016/j.apm.2010.03.012
![]() |
[17] |
W. T. Wang, Finite-time synchronization for a class of fuzzy cellular neural networks with time-varying coefficients and proportional delays, Fuzzy Sets Syst., 338 (2018), 40–49. https://doi.org/10.1016/j.fss.2017.04.005 doi: 10.1016/j.fss.2017.04.005
![]() |
[18] |
L. Duan, H. Wei, L. H. Huang, Finite-time synchronization of delayed fuzzy cellular neural networks with discontinuous activations, Fuzzy Sets Syst., 361 (2019), 56–70. https://doi.org/10.1016/j.fss.2018.04.017 doi: 10.1016/j.fss.2018.04.017
![]() |
[19] |
H. Q. Wu, X. W. Zhang, R. X. Li, R. Yao, Finite-time synchronization of chaotic neural networks with mixed time-varying delays and stochastic disturbance, Memet. Comput., 7 (2015), 231–240. https://doi.org/10.1007/s12293-014-0150-x doi: 10.1007/s12293-014-0150-x
![]() |
[20] |
A. Abdurahman, H. J. Jiang, Z. D. Teng, Finite-time synchronization for fuzzy cellular neural networks with time-varying delays, Fuzzy Sets Syst., 297 (2016), 96–111. https://doi.org/10.1016/j.fss.2015.07.009 doi: 10.1016/j.fss.2015.07.009
![]() |
[21] |
D. S. Xu, Y. Liu, M. Liu, Finite-time synchronization of multi-coupling stochastic fuzzy neural networks with mixed delays via feedback control, Fuzzy Sets Syst., 411 (2021), 85–104. https://doi.org/10.1016/j.fss.2020.07.015 doi: 10.1016/j.fss.2020.07.015
![]() |
[22] |
J. Kumar, V. D. Murugan, M. Hymavathi, M. S. Ali, G. K. Thakur, Finite-time synchronization of neutral type fractional-order bidirectional associative memory neural networks with delays via adaptive feedback control strategy, Discrete Contin. Dyn. Syst. Ser. S, 2024 (2024). https://doi.org/10.3934/dcdss.2024157 doi: 10.3934/dcdss.2024157
![]() |
[23] |
H. N. Nie, Y. Zhang, Finite-time cluster synchronization of multi-weighted fractional-order coupled neural networks with and without impulsive effects, Neural Networks, 180 (2024). https://doi.org/10.1016/j.neunet.2024.106646 doi: 10.1016/j.neunet.2024.106646
![]() |
[24] |
J. Zhang, S. Zhu, K. N. Wu, M. Q. Shen, S. P. Wen, Finite-time stabilization of semi-markov reaction-diffusion memristive NNs With unbounded time-varying delays, IEEE Trans. Circuits Syst. I Regul. Pap., 72 (2025), 1832–1842. https://doi.org/10.1109/TCSI.2024.3459913 doi: 10.1109/TCSI.2024.3459913
![]() |
[25] |
Y. G. Liu, Z. Y. Zhang, X. H. Wang, Z. Wang, C. Lin, Finite-time stabilization for fuzzy complex-valued neural networks with mixed delays via comparison approach, IEEE Tran. Fuzzy Syst., 32 (2024), 621–633. https://doi.org/10.1109/TFUZZ.2023.3304648 doi: 10.1109/TFUZZ.2023.3304648
![]() |
[26] |
X. S. Yang, J. Lam, D. W. Ho, Z. G. Feng, Fixed-time synchronization of complex networks with impulsive effects via nonchattering control, IEEE Trans. Autom. Control, 62 (2017), 5511–5521. https://doi.org/10.1109/TAC.2017.2691303 doi: 10.1109/TAC.2017.2691303
![]() |
[27] |
A. Khanzadeh, M. Pourgholi, Fixed-time sliding mode controller design for synchronization of complex dynamical networks, Nonlinear Dyn., 88 (2017), 2637–2649. https://doi.org/10.1007/s11071-017-3400-x doi: 10.1007/s11071-017-3400-x
![]() |
[28] |
W. L. Zhang, X. S. Yang, C. D. Li, Fixed-time stochastic synchronization of complex networks via continuous control, IEEE Trans. Cybern., 49 (2019), 3099–3104. https://doi.org/10.1109/TCYB.2018.2839109 doi: 10.1109/TCYB.2018.2839109
![]() |
[29] |
H. W. Ren, Z. P. Peng, Y. Gu, Fixed-time synchronization of stochastic memristor-based neural networks with adaptive control, Neural Networks, 130 (2020), 165–175. https://doi.org/10.1016/j.neunet.2020.07.002 doi: 10.1016/j.neunet.2020.07.002
![]() |
[30] |
F. C. Kong, Q. X. Zhu, T. W. Huang, New fixed-time stability lemmas and applications to the discontinuous fuzzy inertial neural networks, IEEE Tran. Fuzzy Syst., 29 (2021), 3711–3722. https://doi.org/10.1109/TFUZZ.2020.3026030 doi: 10.1109/TFUZZ.2020.3026030
![]() |
[31] |
C. Hu, J. Yu, Z. H. Chen, H. J. Jiang, T. W. Huang, Fixed-time stability of dynamical systems and fixed-time synchronization of coupled discontinuous neural networks, Neural Networks, 89 (2017), 74–83. https://doi.org/10.1016/j.neunet.2017.02.001 doi: 10.1016/j.neunet.2017.02.001
![]() |
[32] |
J. D. Cao, R. X. Li, Fixed-time synchronization of delayed memristor-based recurrent neural networks, Sci. China Inf. Sci., 60 (2017). https://doi.org/10.1007/s11432-016-0555-2 doi: 10.1007/s11432-016-0555-2
![]() |
[33] | Y. Liu, M. Liu, X. F. Xu, Adaptive control design for fixed-time synchronization of fuzzy stochastic cellular neural networks with discrete and distributed delay, Iran. J. Fuzzy Syst., 18 (2021), 13–28. |
[34] |
A. Abdurahman, R. Tohti, C. C. Li, New results on fixed-time synchronization of impulsive neural networks via optimized fixed-time stability, J. Appl. Math. Comput., 70 (2024), 2809–2826. https://doi.org/10.1007/s12190-024-02072-w doi: 10.1007/s12190-024-02072-w
![]() |
[35] |
J. A. Wang, R. R. Cai, J. R. Zhang, J. Zhang, M. J. Li, Z. C. Zhao, Fixed-time pinning synchronization of intermittently coupled complex network via economical controller, Neural Process. Lett., 56 (2024). https://doi.org/10.1007/s11063-024-11441-2 doi: 10.1007/s11063-024-11441-2
![]() |
[36] |
Q. X. Zhu, Razumikhin-type theorem for stochastic functional differential equations with Lˊevy noise and Markov switching, Int. J. Control, 90 (2017), 1703–1712. https://doi.org/10.1080/00207179.2016.1219069 doi: 10.1080/00207179.2016.1219069
![]() |
[37] |
H. Zhou, Y. Zhang, W. X. Li, Synchronization of stochastic Lˊevy noise systems on a multi-weights network and its applications of Chua's circuits, IEEE Trans. Circuits Syst. I Regul. Pap., 66 (2019), 2709–2722. https://doi.org/10.1109/TCSI.2019.2899375 doi: 10.1109/TCSI.2019.2899375
![]() |
[38] |
W. E. Zhou, Y. Q. Sun, P. Shi, Cluster synchronization of coupled neural networks with Lˊevy noise via event-triggered pinning control, IEEE Trans. Neural Networks Learn. Syst., 33 (2022), 6144–6157. https://doi.org/10.1109/TNNLS.2021.3072475 doi: 10.1109/TNNLS.2021.3072475
![]() |
[39] |
M. Shi, D. B. Tong, Q. Y. Chen, W. N. Zhou, pth moment exponential synchronization for delayed multi-agent systems with Lˊevy noise and Markov switching, IEEE Trans. Circuits Syst. II Express Briefs, 71 (2024), 697–701. https://doi.org/10.1109/TCSII.2023.3304635 doi: 10.1109/TCSII.2023.3304635
![]() |
[40] |
N. Zhang, Z. Wang, J. H. Park, W. X. Li, Semi-global synchronization of stochastic mixed time-delay systems with Lˊevy noise under aperiodic intermittent delayed sampled-data control, Automatica, 171 (2024). https://doi.org/10.1016/j.automatica.2024.111963 doi: 10.1016/j.automatica.2024.111963
![]() |
[41] |
C. Li, X. F. Xu, M. Liu, The stochastic fixed-time synchronization of delays neural networks driven by Lˊevy noise, Syst. Control Lett., 190 (2024). https://doi.org/10.1016/j.sysconle.2024.105839 doi: 10.1016/j.sysconle.2024.105839
![]() |
[42] | R. M. Balan, C. B. Ndongo, Itˆo formula for integral processes related to space-time Lˊevy white noise, preprint, arXiv: 1505.04685. |
[43] |
Z. Y. Zuo, L. Tie, Distributed robust finite-time nonlinear consensus protocols for multi-agent systems, Int. J. Syst. Sci., 47 (2016), 1366–1375. https://doi.org/10.1080/00207721.2014.925608 doi: 10.1080/00207721.2014.925608
![]() |
[44] |
J. L. Yin, S. Khoo, Z. H. Man, X. H. Yu, Finite-time stability and instability of stochastic nonlinear systems, Automatica, 47 (2011), 2671–2677. https://doi.org/10.1016/j.automatica.2011.08.050 doi: 10.1016/j.automatica.2011.08.050
![]() |
Controller | Gains | Calculation | Design | Synchronization time | Consume resources | Stability |
feedback controller | fixed | simple | simple | more | more | weak |
adaptive controller | dynamic | complex | complex | less | fewer | strong |