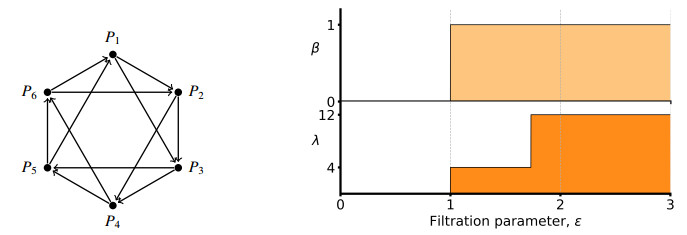
Topological data analysis (TDA) has experienced significant advancements with the integration of various advanced mathematical tools. While traditional TDA has primarily focused on point cloud data, there is a growing emphasis on the analysis of graph data. In this work, we proposed a spectral analysis method for digraph data, grounded in the theory of Hochschild cohomology. To enable efficient computation and practical application of Hochschild spectral analysis, we introduced the concept of truncated path algebras, along with key mathematical results that support the computation of the Hochschild Laplacian. Our study established key mathematical results, including a relationship between Hochschild Betti numbers and the Euler characteristic of digraphs, as well as efficient representations of Hochschild Laplacian matrices. These innovations enabled us to extract multiscale topological and geometric features from graph data. We demonstrated the effectiveness of our method by analyzing the molecular structures of common drugs, such as ibuprofen and aspirin, producing visualized Hochschild feature curves that capture intricate topological properties. This work provides a novel perspective on digraph analysis and offers practical tools for topological data analysis in molecular and broader scientific applications.
Citation: Yunan He, Jian Liu. Multi-scale Hochschild spectral analysis on graph data[J]. AIMS Mathematics, 2025, 10(1): 1384-1406. doi: 10.3934/math.2025064
[1] | Zhe Su, Yiying Tong, Guo-Wei Wei . Persistent de Rham-Hodge Laplacians in Eulerian representation for manifold topological learning. AIMS Mathematics, 2024, 9(10): 27438-27470. doi: 10.3934/math.20241333 |
[2] | Ruzhi Song, Fengling Li, Jie Wu, Fengchun Lei, Guo-Wei Wei . Multi-scale Jones polynomial and persistent Jones polynomial for knot data analysis. AIMS Mathematics, 2025, 10(1): 1463-1487. doi: 10.3934/math.2025068 |
[3] | Huan Xu, Tao Yu, Fawaz E. Alsaadi, Madini Obad Alassafi, Guidong Yu, Jinde Cao . Some spectral sufficient conditions for a graph being pancyclic. AIMS Mathematics, 2020, 5(6): 5389-5401. doi: 10.3934/math.2020346 |
[4] | Li Shen, Jian Liu, Guo-Wei Wei . Evolutionary Khovanov homology. AIMS Mathematics, 2024, 9(9): 26139-26165. doi: 10.3934/math.20241277 |
[5] | Wei Xue, Pengcheng Wan, Qiao Li, Ping Zhong, Gaohang Yu, Tao Tao . An online conjugate gradient algorithm for large-scale data analysis in machine learning. AIMS Mathematics, 2021, 6(2): 1515-1537. doi: 10.3934/math.2021092 |
[6] | Shama Liaqat, Zeeshan Saleem Mufti, Yilun Shang . Newly defined fuzzy Misbalance Prodeg Index with application in multi-criteria decision-making. AIMS Mathematics, 2024, 9(8): 20193-20220. doi: 10.3934/math.2024984 |
[7] | Jinfang Li, Chunjiang Wang, Li Zhang, Jian Zhang . Multi-solitons in the model of an inhomogeneous optical fiber. AIMS Mathematics, 2024, 9(12): 35645-35654. doi: 10.3934/math.20241691 |
[8] | Qingbing Liu, Aimin Xu, Shuhua Yin, Zhe Tu . A note on the preconditioned tensor splitting iterative method for solving strong $ \mathcal{M} $-tensor systems. AIMS Mathematics, 2022, 7(4): 7177-7186. doi: 10.3934/math.2022400 |
[9] | Muhammad Riaz, Khadija Akmal, Yahya Almalki, S. A. Alblowi . Cubic m-polar fuzzy topology with multi-criteria group decision-making. AIMS Mathematics, 2022, 7(7): 13019-13052. doi: 10.3934/math.2022721 |
[10] | Changlong Yu, Jing Li, Jufang Wang . Existence and uniqueness criteria for nonlinear quantum difference equations with $ p $-Laplacian. AIMS Mathematics, 2022, 7(6): 10439-10453. doi: 10.3934/math.2022582 |
Topological data analysis (TDA) has experienced significant advancements with the integration of various advanced mathematical tools. While traditional TDA has primarily focused on point cloud data, there is a growing emphasis on the analysis of graph data. In this work, we proposed a spectral analysis method for digraph data, grounded in the theory of Hochschild cohomology. To enable efficient computation and practical application of Hochschild spectral analysis, we introduced the concept of truncated path algebras, along with key mathematical results that support the computation of the Hochschild Laplacian. Our study established key mathematical results, including a relationship between Hochschild Betti numbers and the Euler characteristic of digraphs, as well as efficient representations of Hochschild Laplacian matrices. These innovations enabled us to extract multiscale topological and geometric features from graph data. We demonstrated the effectiveness of our method by analyzing the molecular structures of common drugs, such as ibuprofen and aspirin, producing visualized Hochschild feature curves that capture intricate topological properties. This work provides a novel perspective on digraph analysis and offers practical tools for topological data analysis in molecular and broader scientific applications.
Topological data analysis (TDA), as a relatively new method, has gained increasing attention across various fields. The key tool in TDA is persistent homology, introduced by G. Carlsson, H. Edelsbrunner, and others [6,18]. The main idea of persistent homology is to extract multi-scale topological features that capture the geometric shape and topological structures of data. Persistent homology has increasingly found success in fields such as molecular biology [4,30], materials science [22,23], image science [8,20], and artificial intelligence [15,28].
In recent years, with the rapid development of TDA, many advanced topological theories have been applied to this field. These methods include cohomology rings [16], de Rham-Hodge theory [13], Steenrod algebra [27], and others, which have enriched and expanded the scope of topological data analysis. Overall, the development of TDA has progressed across three main areas: topological objects, topological persistence, and topological features. In terms of topological objects, simplicial complex models such as Vietoris-Rips complexes and Čech complexes are no longer sufficient for broader applications, as they typically handle point cloud data but struggle with graph data. As a result, TDA based on the topological theories like embedded homology of hypergraphs [26], path homology of digraphs [14], and hyperdigraph homology [12] has emerged. In terms of topological persistence, new theories have been developed, including multiparameter persistence [9,10], zigzag persistence [7], and Cayley-persistence algebra [3]. Regarding topological features, in addition to the aforementioned theories, other forms of persistent homology have been introduced, such as persistent intersection homology [2] and persistent interaction homology [24]. The persistent Laplacian, first introduced by Guo-Wei Wei and his coauthors [13,29], marks an important advancement in topological data analysis. It has since been widely applied in molecular biology, proving effective in capturing the complex structural and functional properties of biomolecules.
Hochschild homology was initially introduced by Gerhard Hochschild [21] for studying the cohomology of associative algebras. Over time, it has been developed as a tool for investigating topological invariants of algebraic structures such as rings, Hopf algebras, Lie algebras, and more. Today, Hochschild homology serves as a fundamental concept in algebraic topology and algebraic geometry, providing insights into the internal structure and symmetries of these algebraic objects. Given a quiver, the path algebra can be constructed in a standard way, allowing us to define Hochschild homology on this path algebra, referred to as the path algebra of the quiver [17]. In particular, Hochschild homology can be defined on digraphs. In [5], the authors introduced persistent Hochschild homology using connectivity digraphs. Inspired by the above work, in this paper, we develop a multiscale Hochschild spectral analysis on graph data.
Traditional simplicial complexes struggle to capture directed edges and multiscale topological features, creating a gap in the application of TDA to directed graph data, which are increasingly common in fields like molecular biology and network science. Motivated by these challenges, we develop a spectral analysis framework for digraphs using Hochschild cohomology. This approach not only addresses the computational inefficiencies of infinite-dimensional path algebras but also integrates harmonic and non-harmonic information to provide a richer characterization of graph data.
In this paper, we introduce the Hochschild Laplacian, and propose a multiscale Hochschild spectral analysis on digraphs. Hochschild cohomology offers a new perspective for analyzing digraph data; however, homological information alone is often insufficient for fully characterizing the structure of graph data. To address this, we introduce the spectral information of the Hochschild Laplacian to study the multiscale topological features and geometric properties of digraphs. The Hochschild Laplacian is of particular interest because its harmonic component contains Hochschild cohomology information, while its non-harmonic component encodes the geometric information of digraphs in a metric space. One challenge in computing and applying the Hochschild Laplacian is that the path algebra is often infinite-dimensional. Instead of adopting the connectivity digraph construction from [5] to circumvent this issue, we consider the truncated path algebra, which is more computationally feasible and whose Hochschild Laplacian construction also encompasses information from the traditional graph Laplacian. Additionally, some mathematical results in this paper simplify the computation of certain Hochschild Laplacian matrices. For specific molecules, we utilize the distances between atoms to construct multiscale information, enabling the computation of their topological features and geometric properties across scales. We demonstrate the application of this method on common drug molecules, such as ibuprofen and aspirin, yielding rich topological and geometric insights. Compared to existing methods, our approach combines computational efficiency with the ability to capture both harmonic and non-harmonic features of digraphs, including higher-order topological and geometric information. These advantages make it particularly suitable for analyzing complex graph structures, such as molecular data.
The paper is organized as follows. In the next section, we review some fundamental concepts and results related to Hochschild cohomology and path algebras. Section 3 outlines our main methods and relevant mathematical results, including truncated path algebras, the Hochschild Laplacian, and multiscale Hochschild spectra. In Section 4, we apply the Hochschild spectral analysis to drug molecules. The final section provides a summary of the paper.
In this section, we will review the basic concepts of Hochschild cohomology and path algebras. From now on, K is assumed to be a field of characteristic zero.
Let A be an associative algebra over K. From now on, unless otherwise specified, the tensor product ⊗ denotes ⊗K, and Hom(−,−) denotes HomK(−,−) for simplicity. Let A⊗n=A⊗⋯⊗A⏟n. For an A-bimodule M, let Cn(A,M)=Hom(A⊗n,M). Then, we have homomorphisms di:Cn(A,M)→Cn+1(A,M) given by
(d0m)(a)=am−ma,(dnf)(a1⊗⋯⊗an+1)=a1f(a2⊗⋯⊗an+1)+n∑i=1(−1)if(a1⊗⋯⊗aiai+1⊗⋯⊗an+1)−(−1)nf(a1⊗⋯⊗an)an+1. |
It can be proved that dn+1dn=0 for n≥0. The Hochschild complex C∗(A,M) is the cochain complex
0⟶Md0→C1(A,M)d1→⋯dn−2→Cn−1(A,M)dn−1→Cn(A,M)dn→⋯. |
The differential dn is called the Hochschild differential. Let us denote Zn(A,M)=kerdn and Bn(A,M)=imdn−1. Then, we have Bn(A,M)⊆Zn(A,M).
Definition 2.1. The Hochschild cohomology of A with coefficients in M is defined by
HHn(A,M)=Zn(A,M)/Bn(A,M),n≥0. |
In particular, we denote HHn(A)=HHn(A,A). The rank of the Hochschild cohomology, denoted by βnH(A,M)=rankKHHn(A,M) for n≥0, is called the Hochschild Betti number. The following lemma is obtained by a straightforward calculation.
Lemma 2.1. (i) HH0(A,M)=ZA(M)={x∈M|xa=ax for any a∈A}.
(ii) Z1(A,M)=DerA(M)={δ∈Hom(A,M)|δ(ab)=δ(a)b+aδ(b) for any a,b∈A}.
Example 2.1. (i) Let A=K[x] be a one-variable polynomial algebra. Then, the Hochschild cohomology of A is HHn(A)≅{A,n=0,1;0,otherwise.
(ii) Consider the truncated polynomial algebra A=K[x]/(xk) for k>1. Then, we have the Hochschild cohomology HHn(A)={A, n=0; (x)A,n>0iseven;A/(xk−1),n>0isodd.
The Hochschild cohomology generators offer critical insights into the algebraic and geometric structures of a given algebra. In dimension 0, the Hochschild cohomology (HH0) corresponds to the center of the algebra A, consisting of elements that commute with every other element. This reflects the algebra's symmetries. Geometrically, HH0 represents zero-dimensional topological features of the associated manifold, such as fixed points or isolated structures that remain invariant under transformations. In dimension 1, the Hochschild cohomology (HH1) captures the infinitesimal deformations of the algebra A, describing how the algebra changes under small perturbations or parameter adjustments. Geometrically, HH1 corresponds to vector fields on the manifold, which represent directional flows or infinitesimal symmetries, illustrating how the structure can be locally deformed. For higher dimensions (HHn,n≥2), the generators encode more complex interactions within the algebra, such as multi-variable deformations and nested relationships among elements of A. Geometrically, these cohomology classes generalize the concepts of vector fields and symmetries to higher dimensions, representing multi-directional flows, higher-order deformations, or constraints within the manifold's structure.
A directed graph (digraph) G=(V,E) is a finite nonempty set V, called the vertex set, equipped with an edge set E⊆V×V. For an edge e=(v,v′)∈E, we denote s(e)=v as the source of e, and t(e)=v′ as the target of e. A path on G is a sequence of v0v1⋯vk such that (vi−1,vi)∈E for i=1,2,…,k. The length of a path γ=v0v1⋯vk is defined as ℓ(γ)=k. The vertices can be regarded as trivial paths of length zero.
Let K be a field, and let G be a digraph. The path algebra Ω(G) of G is the K-linear space generated by all the paths on G, with the product defined as follows:
(v0v1⋯vk)∗(w0w1⋯wl)={v0v1⋯vkw1⋯wl,if vk=w0;0,otherwise. |
Note that ℓ((v0v1⋯vk)∗(w0w1⋯wl))={k+l, vk=w0; 0,otherwise. Hence, Ω(G) is a graded algebra, and we can express it as Ω(G)=⨁i≥0Ωi(G). A cycle in G is a path v0v1⋯vk of k≥1 such that v0=vk.
Proposition 2.2. Let G=(V,E) be a digraph. Then, Ω(G) is a finite-dimensional associative algebra if and only if G has no cycles.
Proof. If G has no cycles, then each path is of the form γ=v0v1⋯vk for distinct vertices v0,v1,…,vk in V. Since V is finite, the length of γ is at most N−1, where N=|V| denotes the number of elements in V. The number of directed paths of length no greater than N−1 is less than (N+1)N. Therefore, we have dimΩ(G)<(N+1)N.
On the other hand, if G contains a cycle γ=v0v1⋯vk for some k≥1, then there exists a family of paths {γr}r≥1. Since γr≠0 for any r≥1 and ℓ(γr)=kr, it follows that there are infinitely many paths in Ω(G). Thus, Ω(G) is not a finite-dimensional algebra.
Example 2.2. Let G=(V,E) be a digraph given by V={v} and E={(v,v)}. Then, Ω(G)=K[x] is a polynomial algebra. The corresponding Hochschild cohomology is given by HHk(Ω(G))={K[x],k=0,1;0,otherwise. Thus, the Hochschild cohomology HHk(Ω(G)) is an infinite dimensional algebra.
Example 2.3. Consider the digraph G=(V,E) given by
V={v0,v1,v2},E={(v0,v1),(v0,v2),(v1,v2)}. |
The corresponding path algebra Ω(G) is a K-linear space generated by v0,v1,v2, and v0v1,v0v2,v1v2. The identity element is v0+v1+v2. The nontrivial product is given by
v0∗v0v1=v0v1∗v1=v0v1,v0∗v0v2=v0v2∗v2=v0v2,v1∗v1v2=v1v2∗v2=v1v2,v0v1∗v1v2=v0v1v2. |
By a direct computation, we have
HH0(Ω(G))=K{v0+v1+v2}. |
Let Cn(Ω(G))=Cn(Ω(G),Ω(G)). We have the following cochain complex:
0⟶Ω(G)d0→C1(Ω(G))d1→C2(Ω(G))d2→⋯. |
Let us compute
kerd1={δ∈Hom(Ω(G),Ω(G))|δ(ab)=δ(a)b+aδ(b) for any a,b∈Ω(G)}. |
By considering the equation δ(vs∗vt)=δ(vs)∗vt+vs∗δ(vt), we have
δ(v0)=a1v0v1+a2v0v2+b1v0v1v2,δ(v1)=−a1v0v1+a3v1v2,δ(v2)=−a2v0v2−a3v1v2−b1v0v1v2, |
where a1,a2,a3,b1∈K. Similarly, by using the Leibniz rule on δ(vs∗v0v1) and δ(v0v1∗vt), we have
δ(v0v1)=c1v0v1+a3v0v1v2,δ(v0v2)=c2v0v2+b2v0v1v2,δ(v1v2)=c3v1v2−a1v0v1v2, |
where c1,c2,c3,b2∈K. Besides, we have δ(v0v1v2)=(c1+c3)v0v1v2. For path γ1,γ1 on G, let us denote γ1⋅γ♯2:Ω(G)→Ω(G) as follows:
(γ1⋅γ♯2)(γ)={γ1,γ=γ2;0,otherwise. |
Then, Z1(Ω(G))=kerd1 is a K-linear space generated by the elements
v0v1⋅(v♯0−v♯1)+v0v1v2⋅(v1v2)♯,v0v2⋅(v♯0−v♯2),v1v2⋅(v♯1−v♯2)+v0v1v2⋅(v0v1)♯,v0v1v2⋅(v♯0−v♯2),v0v1⋅(v0v1)♯+v0v1v2⋅(v0v1v2)♯,v0v2⋅(v0v2)♯,v1v2⋅(v1v2)♯+v0v1v2⋅(v0v1v2)♯,v0v1v2⋅(v0v2)♯. |
On the other hand, a step-by-step calculation shows that imd0 is generated by
v0v1⋅(v♯0−v♯1)+v0v1v2⋅(v1v2)♯,v0v2⋅(v♯0−v♯2),v1v2⋅(v♯1−v♯2)+v0v1v2⋅(v0v1)♯,v0v1v2⋅(v♯0−v♯2),v0v1⋅(v0v1)♯+v0v1v2⋅(v0v1v2)♯,v1v2⋅(v1v2)♯+v0v1v2⋅(v0v1v2)♯. |
It follows that
HH1(Ω(G))≅K{v0v2⋅(v0v2)♯,v0v1v2⋅(v0v2)♯}. |
Let K be a simplicial complex. The subdivision sd(K) of K is the simplicial complex whose p-simplices are of the form σ0σ1⋯σp, where σ0⊂σ1⊂⋯⊂σp and σ0,σ1,…,σp∈K. We can regard sd(K) as a digraph, whose vertex set is the set of simplices of K, and whose edge set consists of pairs (σ,τ) where τ⊆σ. Let Λ(K) denote the path algebra associated with the digraph obtained from sd(K). It is a classical result that the Hochschild cohomology of Λ(K) coincides with the simplicial cohomology of K.
Theorem 2.3. [19] HH∗(Λ(K))≅H∗(K).
Digraphs are common mathematical objects and data structures. A natural idea presented in this paper is to use Hochschild homology information as a topological feature of digraphs for data analysis. As mentioned in Proposition 2.2 in the previous section, the path algebra of a digraph with directed cycles is infinite-dimensional, which is unsuitable for computation and practical applications. Therefore, we consider working with truncated path algebras to obtain Hochschild homology and spectral information for data analysis. In this section, we will establish the theoretical foundation for Hochschild homotopy and Hochschild Laplacians based on truncated path algebras.
Let G be a digraph. The N-truncated path algebra Ω≤N(G) of G is the K-linear space generated by all paths of length not larger than N. The product of two paths γ⋅γ′ in Ω≤N(G) coincides with the product in Ω(G) if ℓ(γ)+ℓ(γ′)≤N, and γ⋅γ′=0 if ℓ(γ)+ℓ(γ′)>N. A digraph is simple if it has no loops or parallel edges. From now on, for the sake of simplicity, all the digraphs considered are assumed to be simple.
Lemma 3.1. Let A=K{x1,…,xr} be a K-algebra with the multiplication given by
xixj={xi,xi=xj;0,otherwise. |
Then, we have
HHn(A)={A,n=0;0,n≥1. |
Proof. Let Aop be the opposite algebra of A, and let Ae=A⊗Aop be the enveloping algebra of A. Then, we have a free resolution of A as follows:
⋯η→Aeλ→Aeη→Aeλ→Aeμ→A⟶0. |
Here, μ(x⊗y)=xy, λ(xi⊗xj)={xi⊗xj,i≠j;0,i=j and η(xi⊗xj)={xi⊗xj,i=j;0,i≠j. By applying the left exact functor HomAe(−,A), we have the sequence
0⟶HomAe(Ae,A)λ∗→HomAe(Ae,A)η∗→HomAe(Ae,A)λ∗→⋯ |
0⟶Aλ∗→Aη∗→Aλ∗→Aη∗→⋯, |
where λ∗=0 and η∗=id. By the well-known isomorphism HHn(A,M)=ExtnAe(A,M), we have the desired result.
Proposition 3.2. Let G=(V,E) be a digraph. Then, we have
HHn(Ω≤0(G))={Ω≤0(G),n=0;0,n≥1. |
Proof. It is a direct result of Lemma 3.1.
Theorem 3.3. Let G be a digraph. Then, we have
β0H(Ω≤1(G))=1,β1H(Ω≤1(G))=1−χ(G). |
Here, χ(G) denotes the Euler characteristic of G as a 1-dimensional simplicial complex.
Proof. By definition, we have
HH0(Ω≤1(G))=Z(Ω≤1(G))=K{∑v∈Vv}. |
Let V={v1,v2,…,vk} and E={e1,e1,…,vl}. Consider the following cochain complex:
0⟶Ω≤1(G)d0→C1(Ω≤1(G))d1→C2(Ω≤1(G))d2→⋯. |
Note that kerd1=Der(Ω≤1(G)). For any δ∈kerd1, we have δ(ab)=δ(a)b+aδ(b) for a,b∈Ω≤1(G). By applying δ(vs∗vt)=δ(vs)∗vt+vs∗δ(vt) for the case s=t, we obtain that
δ(vs)=∑ejas,jej,as,j∈K, |
where the sum ∑ runs over all edges of the form (vs,−) or (−,vs). Here, we use the edge e to denote a path of length 1 for simplicity. For s≠t, if ej is of the form (vs,vt) or (vt,vs), we obtain
as,j+at,j=0. |
Thus, the basis of δ on v1,v2,…,vk is given by {vsvt⋅(vs−vt)♯}s,t, where vsvt or vtvs is an edge in E. Note that
δ(vrvs∗vt)=δ(vrvs)∗vt+vrvs∗δ(vt)=δ(vrvs)∗vt. |
Assume that δ(vrvs)=k∑i=1bivi+l∑j=1cjej for some bi,cj∈K. We have
k∑i=1bivi+l∑j=1cjej=bsvs+l∑j=1cjejvs. |
It follows that bi=0 for i≠s. Similarly, by applying the Leibniz rule on vr∗vsvt, we obtain bi=0 for i≠r. Since G is simple, we have r≠s. It follows that bi=0 for any i. Note that l∑j=1cjej=l∑j=1cjejvs and l∑j=1cjej=vrl∑j=1cjej, and we obtain that
δ(vrvs)=crsvrvs,crs∈K. |
Let Sv denote the set of edges of the form (v,−) or (−,v). Hence, the linear space kerd1 is generated by {vsvt⋅(vs−vt)♯}s,t for edges vsvt or vtvs and
e1⋅e♯1,e2⋅e♯2,…,el⋅e♯l. |
Thus, we have
dimkerd1=(k∑i=1di)−l+l=2l. |
Here, di denotes the degree of the vertex vi, meaning the number of edges connected to vi. On the other hand,
dimimd0=dimΩ≤1(G)−dimkerd0=k+l−1. |
It follows that
β1H(Ω≤1(G))=dimkerd1−dimimd0=l−k+1. |
By the Euler formula, we have χ(G)=β0(G)−β1(G)=k−l. It follows that β1H=1−χ(G).
Example 3.1. Let G=Cm be a digraph with the vertex set and edge set
V={v1,v2,…,vm},E={(v1,v2),…,(vm−1,vm),(vm,v1)}. |
By Lemma 2.1, one has that
HH0(Ω≤1(G))=K{v1+v2+⋯+vm}. |
On the other hand, the space kerd1=Der(Ω≤1(G)) is generated by the elements
v1v2⋅(v♯1−v♯2),…,vm−1vm⋅(v♯m−1−v♯m),vmv1⋅(v♯m−v♯1),v1v2⋅(v1v2)♯,…,vm−1vm⋅(vm−1vm)♯,vmv1⋅(vmv1)♯. |
Recall that d0:Ω≤1(G)→C1(Ω≤1(G)) is given by d0(a)x=xa−ax. It follows that
d0(vr)(x)={−x,x=vrvr+1;x,x=vr−1vr;0,otherwise. |
Here, we make the convention that v−1=vm and vm+1=v1 for convenience. Moreover, we have
d0(vrvr+1)(x)={vrvr+1,x=vr;−vrvr+1,x=vr+1;0,otherwise. |
It follows that imd0 is generated by
vr−1vr⋅(vr−1vr)♯−vrvr+1⋅(vrvr+1)♯,vrvr+1⋅(v♯r−v♯r+1) |
for r=1,2,…,m. Thus, the Hochschild homology is
HH1(Ω≤1(G))=K{[v1v2⋅(v♯1−v♯2)]}. |
Here, [v1v2⋅(v♯1−v♯2)] is an equivalent class of the homology generator. The Euler characteristic of a circle is 0. By Theorem 3.3, one has β1H(Ω≤1(G))=1−χ(G)=1. This aligns with the result from the above calculations.
In this section, the field K is taken to be the real number field R.
Let V and W be inner product spaces. Then the tensor product V⊗W is also an inner product space, with the inner product defined as:
⟨v1⊗w1,v2⊗w2⟩V⊗W=⟨v1,v2⟩V⟨w1,w2⟩W, |
where v1,v2∈V and w1,w2∈W. For any map f∈Hom(V,W), the adjoint map f∗ of f is defined as
⟨f(v),w⟩=⟨v,f∗(w)⟩,for any v∈V,w∈W. |
Then, the space Hom(V,W) is an inner product space, with the inner product, known as the Frobenius inner product, given by
⟨f,g⟩Hom(V,W)=tr(f∗g)=tr(gf∗), |
where f,g∈Hom(V,W) and tr denotes the trace of linear transformations.
From now on, for simplicity, all inner products will be denoted by ⟨−,−⟩. Let A be a finite dimensional algebra over R. Suppose A is an inner product space with the inner product structure ⟨−,−⟩. Then, A⊗n and Cn(A) are also inner product spaces.
We have a Hochschild complex C∗(A) as follows:
0⟶Ad0→C1(A)d1→⋯dn−2→Cn−1(A)dn−1→Cn(A)dn→⋯. |
The n-th Hochschild Laplacian of A is
Δn=(dn)∗∘dn+dn−1∘(dn−1)∗,n≥0. |
Specifically, for n=0, we have Δ0=(d0)∗∘d0. It is clear that Δn is a self-adjoint and non-negative operator.
Let G be a digraph. The truncated algebra Ω≤N(G) is a finite dimensional algebra. We endow Ω≤N(G) with the inner product structure defined as follows:
⟨γ,γ′⟩={1,γ=γ′;0,otherwise. |
Thus, we can obtain the Hochschild Laplacian ΔnN:Cn(Ω≤N(G))→Cn(Ω≤N(G)) of truncated algebra Ω≤N(G).
Theorem 3.4. Let G be a digraph. Then, we have
HHn(Ω≤N(G))≅kerΔnN,n≥0. |
Proof. Consider the cochain complex
0⟶Ω≤N(G)d0→C1(Ω≤N(G))d1→⋯dn−1→Cn(Ω≤N(G))dn→⋯. |
It follows that kerΔnN=kerdn∩ker(dn−1)∗. Note that the inclusion ι:(kerΔ∗N,0)↪C∗(Ω≤N(G) is a morphism of cochain complexes, which induces a K-linear map ι∗:kerΔ∗N→HH∗(Ω≤N(G)). Here, kerΔ∗N=⨁n≥0ΔnN. If ι(x) is a coboundary in C∗(Ω≤N(G) for x∈kerΔnN, we can write x=dn−1z for some z∈Cn−1(Ω≤N(G). But x∈kerdn, so it follows that x=0. Thus the map ι∗ is an injection. On the other hand, for any cocycle y in Cn(Ω≤N(G)), by [25, Proposition 3.1], we can write y=y0+dn−1y1 for y0∈kerΔnN and y1∈Cn−1(Ω≤N(G)). It follows that ι∗([y0])=[y]. Thus the map ι∗ is a surjection. The desired result follows.
The Laplacian matrix of a graph is a fundamental tool for analyzing graph structure, connectivity, and spectral clustering, with its eigenvalues and eigenvectors revealing essential properties of the graph. It also has broad applications in signal processing, random walks, and optimization problems, making it a crucial instrument for studying networks and graph data, as illustrated in recent studies [1]. Note that a digraph G can be regarded as a graph if the direction of its edges is ignored. The corresponding Laplacian matrix for this graph is referred to as the symmetric Laplacian of G.
Let G be a digraph. Let V={v1,v2,…,vk} be the set of vertices in G, and let E={e1,e2,…,el}. We denote v=(v1,v2,…,vk)T and e=(e1,e2,…,el)T. Besides, let v♯=(v♯1,v♯2,…,v♯k)T and e♯=(e♯1,e♯2,…,e♯l)T. Then the representation matrix D0 of the differential d0:C0(Ω≤1(G))→C1(Ω≤1(G)) is given by
d0(ve)=D0(v⊗v♯v⊗e♯e⊗v♯e⊗e♯). |
Here, v⊗v♯=(v1⋅v♯⋮vk⋅v♯), v⊗e♯=(v1⋅e♯⋮vk⋅e♯), e⊗v♯=(e1⋅v♯⋮el⋅v♯), and e⊗e♯=(e1⋅e♯⋮el⋅e♯).
Theorem 3.5. Let G=(V,E) be a digraph. Suppose the symmetrization of G is a simple graph. Then the representation matrix of Δ01 is given by
L01=(LG002I|E|). |
Here, LG is the symmetric Laplacian matrix of G, and I|E| is the identity matrix of ord |E|.
Proof. Similar to Example 3.1, the differential d0:Ω≤1(G)→C1(Ω≤1(G)) is given by
d0(vr)(x)={−x,x=vrvs;x,x=vsvr;0,otherwise, |
and
d0(vrvs)(x)={vrvs,x=vr;−vrvs,x=vs;0,otherwise. |
Hence, the representation matrix D0 is of the form (000A00B0). Here, the matrices A and B are given by
d0v=Ae⊗e♯,d0e=Be⊗v♯. |
Note that the coefficients of er⋅e♯s in Ae⊗e♯ are zero. Let us denote AAT=(ai,j)1≤i,j≤k. It follows that
aij={degvi,i=j;−1,vivj or vjvi is an edge;0,otherwise. |
Thus, AAT is the Laplacian matrix. Similarly, let BBT=(bi,j)1≤i,j≤l. One can obtain:
bij={2,i=j;0,otherwise. |
Hence, the matrix BBT is given by 2I|E|.
Remark 3.1. Let G=(V,E) be a digraph. Recall that the Laplacian matrix of a graph is given by L=D−M, where D is the degree matrix, and M is the adjacency matrix of G. Alternatively, the Laplacian can be expressed using the incidence matrix. The incidence matrix N=(Nij) is defined as follows:
Nij={1,if ej originates from vi,−1,if ej points to vi,0,otherwise. |
With this definition, the Laplacian matrix can be written as L=NNT.
Let G=(V,E) be a digraph. Define Ωi as the linear subspace generated by paths of length i. Then, the space Ω≤N(G) admits a direct sum decomposition:
Ω≤N(G)=N⨁i=0Ωi(G). |
Consequently, we have
C1(Ω≤N(G))=⨁i,jHom(Ωi(G),Ωj(G)). |
This decomposition provides a clearer understanding of the differential d0.
Lemma 3.6. Let G be a digraph. The differential d0:Ω≤N(G)→C1(Ω≤N(G)) has a decomposition d0=N⨁i=1d0i, where d0i:Ωi(G)→Hom(Ωi(G),Ωi(G)).
Proof. It can be obtained directly by definition.
HH0(Ω≤N(G))=N⨁i=1HH0,i(Ω≤N(G)). |
Here, HH0,i(Ω≤N(G)) denotes the subspace of HH0(Ω≤N(G)) spanned by the generators derived from the elements of Ωi(G). We denote the rank of HH0,i(Ω≤N(G)) by β0,iH,N, which is equal to the number of zero eigenvalues of Δ0N,i.
Proposition 3.7. Let G=(V,E) be a digraph. The Hochschild Laplacian Δ0N:Ω≤N(G)→Ω≤N(G) has a decomposition Δ0N=N⨁i=1Δ0N,i, where Δ0N,i=(d0i)∗d0i:Ωi(G)→Ωi(G).
Proof. By Lemma 3.6, we have that (d0i)∗d0j=0 for any i≠j. It follows that
Δ0N=(d0)∗d0=(N⨁i=1d0i)∗N⨁i=1d0i=N⨁i=1N⨁j=1(d0i)∗d0j=N⨁i=1(d0i)∗d0i. |
The desired result follows.
Theorem 3.8. Let G=(V,E) be a digraph. The representation matrix of Δ0N,0 is given by N∑i=1L0N,i, where L0N,i=(xrs)1≤r,s≤|V| is defined by
xrs={degi(vr),if r=s,−nrs,otherwise. |
Here, degi(v) denotes the number of paths of length i that either begin from or end at v, and nrs represents the number of paths of length i between vr and vs.
Proof. Let γ1,…,γki be the basis of Ωi(G). Note that the image of
d0i:Ωi(G)→Hom(Ωi(G),Ωi(G)) |
contracts to the space generated by γ1⋅γ♯1,…,γki⋅γ♯ki. Thus the representation matrix of d0i is given by
d0i(v1⋮vk)=Di(γ1⋅γ♯1⋮γki⋅γ♯ki), |
where v1,…,vk is the basis of Ωi(G), and Di=(ars)1≤r≤k,1≤s≤ki is given by
ars={1,γs ends at vr;−1,γs begins from vr;0,otherwise. |
It is worth noting that for a path γ that both starts and ends at v, we have d0i(v)(γ)=0. Thus, the representation of Δ0N,i is given by DiDTi=(xrs)1≤r,s≤k, where
xrs={degi(vr),if r=s,−nrs,otherwise, |
where degi(v) is the number of paths of length i that either begin or end at v, and nrs is the number of paths of length i between vr and vs. The desired result follows from Proposition 3.7.
The computation of the combinatorial Laplacian is generally more complex compared to the graph Laplacian. The graph Laplacian is computationally the least expensive, as it primarily involves operations on adjacency or degree matrices, which are typically of size n×n, where n is the number of vertices. In contrast, the combinatorial Laplacian requires more computational resources due to the need for higher-dimensional boundary operators and larger matrices associated with simplicial complexes. The size of these matrices depends on the number of k-simplices, which can grow significantly in higher dimensions. The Hochschild Laplacian involves even higher-order algebraic operations, leading to larger and more complex matrices. However, we have developed results such as Theorem 3.5, Proposition 3.7, and Theorem 3.8, which offer efficient methods for computing the Hochschild Laplacian in certain special cases. These results enable our computational approach to achieve efficiency comparable to that of the graph Laplacian and combinatorial Laplacian.
For the Hochschild Laplacian in dimensions larger than 0, it is also possible to compute explicit examples. Below, we provide a simple example to demonstrate the computation process.
Example 3.2. Let us consider the digraph G=(V,E) from Example 2.2. Note that Ω(G)=K[x] is a polynomial algebra, and the Hochschild cohomology in this case is infinite-dimensional. To simplify, we consider the truncated algebra Ω≤1(G)=K{1,x} with the relation x2=0. This results in the following cochain complex:
0⟶K{1,x}d0→Hom(K{1,x},K{1,x})d1→Hom(K{1,x}⊗K{1,x},K{1,x})d2→⋯. |
Now, consider the linear maps δ1,δx:K{1,x}→K{1,x} defined as follows:
δ1(a)={1,if a=1;0,if a=x;δx(a)={0,if a=1;1,if a=x. |
It is worth noting that the space Hom(K{1,x},K{1,x}) is a K-linear space spanned by the four elements δ1,δx,xδ1,xδx, which are mutually orthogonal. Here, the actions of xδ1 and xδx are given by (xδ1)(a)=xδ1(a) and (xδx)(a)=xδx(a), respectively. On the other hand, consider the linear maps ω11,ω1x,ωx1,ωxx:K{1,x}⊗K{1,x}→K{1,x} defined as follows:
ω11(a⊗b)=δ1(a)δ1(b),ω1x(a⊗b)=δ1(a)δx(b),ωx1(a⊗b)=δx(a)δ1(b),ωxx(a⊗b)=δx(a)δx(b). |
The space Hom(K{1,x}⊗K{1,x},K{1,x}) is spanned by the following mutually orthogonal elements:
ω11,ω1x,ωx1,ωxx,xω11,xω1x,xωx1,xωxx. |
Here, the action of xωuv for u,v∈{1,x} is defined by
(xωuv)(a⊗b)=xωuv(a⊗b). |
Now, by definition, we have
d1(δ1)(a⊗b)=aδ1(b)+δ1(a)b−δ1(ab). |
It follows that
d1(δ1)(a⊗b)={1,if a=b=1;x,if a=1,b=x;x,if a=x,b=1;0,if a=b=x. |
Thus, we obtain:
d1(δ1)=ω11+xω1x+xωx1. |
Similar calculations yield
d1(δx)=2xωxx,d1(xδ1)=xω11,d1(xδx)=0. |
Thus, we can express the action of d1 in matrix form as
d1(δ1δxxδ1xδx)=(10000110000000020000100000000000)(ω11ω1xωx1ωxxxω11xω1xxωx1xωxx). |
Note that d0=0 on K{1,x}. It follows that the representation matrix of the Laplacian Δ11:Hom(K{1,x},K{1,x})→Hom(K{1,x},K{1,x}) is
(3000040000100000). |
The corresponding spectrum is Spectra(Δ11)={0,1,3,4}.
A filtration of digraphs is a family of digraphs {Gε}ε∈R parameterized by a variable ε∈R, such that for any ε≤ε′, the digraph Gε is a subgraph of Gε′. More precisely, for any real numbers ε0≤ε1≤⋯≤εm, there exists a sequence of digraphs
Gε0↪Gε1↪⋯↪Gεm. |
This filtration of digraphs captures the multi-scale structure of the digraph.
Let G be a digraph embedded in Euclidean space, meaning that the vertices of the digraph are points in Euclidean space and the edges are line segments. We define a function ℓ:E→R by ℓ(e)=‖e‖2, where ‖⋅‖2 denotes the L2-norm. For any real number ε, we define the set Eε={e∈E∣ℓ(e)≤ε}. This gives rise to the subgraph Gε=(V,Eε) of G. It is clear that the sequence of digraphs {Gε}ε∈R forms a filtration.
Given a digraph G, recall that the number of zero eigenvalues of the Hochschild Laplacian ΔnN:Cn(ΩN(G))→Cn(ΩN(G)) equals the Betti number of the corresponding Hochschild homology HHn(ΩN(G)) (Theorem 3.4). The number of zero eigenvalues of the Hochschild Laplacian corresponds to the harmonic feature. Furthermore, the non-zero eigenvalues of the Hochschild Laplacian Δn reflect its non-harmonic information. Specifically, the harmonic part refers to the component that corresponds to the most stable or long-lasting features of the graph structure, often capturing the core topological structure. The non-harmonic part, on the other hand, represents more transient features, highlighting the finer details and variations that are not captured by the harmonic part. In particular, the smallest non-zero eigenvalue is a crucial non-harmonic feature and serves as a key geometric characteristic in our applications.
Let {Gε}ε∈R be a filtration of digraphs. Fix an integer N≥0. We can define the function βnH,N:R→R given by βnH,N(ε)=βnH,N(Ω≤N(Gε)) for each n≥0. This function provides a Betti number for each scale, characterizing the multi-scale topological information of the filtration of digraphs. Additionally, we can consider the smallest non-zero eigenvalue of the Hochschild Laplacian to define the function λnH,N:R→R, given by the smallest non-zero eigenvalue of the Hochschild Laplacian ΔnN on Gε for each n≥0. The two functions βnH,N and λnH,N are the main topological features we develop in this work for data analysis. For computational convenience, the functions β0H,N and λ0H,N, or more specifically, the functions β0,iH,N and λ0,iH,N, are used to compute Hochschild features. Here, λ0,iH,N denotes the smallest nonzero eigenvalue of the Laplacian component Δ0N,i.
Example 3.3. Consider the finite set of points X in the Euclidean plane given by
P1(0,1),P2(√32,12),P3(√32,−12),P4(0,−1),P5(−√32,−12),P6(−√32,12). |
The digraph G is given in Example 3.3. Note that the algebra Ω(G) is infinite-dimensional. We consider the truncated algebra Ω≤2(G). The filtration parameter changes at the values ε=1 and ε=√3. By a direct calculation, the representation matrix of Δ0N,0 at ε=1 is given by
(4−1−10−1−1−14−1−10−1−1−14−1−100−1−14−1−1−10−1−14−1−1−10−1−14). |
The spectra of Δ0N,0 is given by
Spectra(Δ0N,0)={0,4,4,4,6,6}. |
Similarly, we can obtain the spectra of Δ0N,1 as follows:
Spectra(Δ0N,1)={0.693,12,12,12,17.307,18}. |
Thus, we obtain the functions β0,0H,2 and λ0,0H,2, as shown in Figure 1. The simplicity of the curves is due to the high symmetry of the digraph in this example. For more complex structural applications, we can observe greater variability in the functions.
To further highlight the strengths of the multi-scale Hochschild spectra, we provide a comparative analysis with traditional persistent homology and multi-scale graph spectra approaches across three key aspects: directed edge support, topological and geometric information, and high-dimensional information, as summarized in Table 1.
Aspect | Persistent Homology | Multi-scale Graph Spectra | Multi-scale Hochschild Spectra |
Directed Edge Support | Not supported | Undirected graphs | Supports directed edges |
Topological and Geometric Information | Harmonic only | Harmonic and non-harmonic | Harmonic and non-harmonic (via multi-scale Hochschild spectras) |
High-Dimensional Information | Supports high dimensions | Limited to 0th and 1st dimensions | Supports high dimensions |
First, in terms of directed edge support, traditional persistent homology relies on simplicial complexes such as Vietoris-Rips and Čech complexes, which are primarily designed for point clouds and undirected graphs. As a result, they cannot represent directed edges, making them unsuitable for digraph analysis. Similarly, the multi-scale graph spectra is defined for undirected graphs and fails to account for edge directionality. In contrast, the multi-scale Hochschild spectra leverages Hochschild cohomology, enabling the direct incorporation of directed edges into the analysis. This makes it particularly effective for studying digraph data.
Second, regarding topological and geometric information, traditional persistent homology exclusively captures harmonic information, focusing on stable topological features while neglecting non-harmonic components. On the other hand, the multi-scale graph spectra combines both harmonic and non-harmonic information, offering insights into the spectral and geometric properties of undirected graphs. The multi-scale Hochschild spectra advances this further by integrating both harmonic and non-harmonic information within the framework of Hochschild cohomology. This integration allows for a richer characterization of the topological and geometric features of digraphs, making it highly suitable for analyzing complex directed networks.
Finally, when considering high-dimensional information, the multi-scale graph spectra is restricted to the 0th and 1st dimensions, limiting its applicability to higher-dimensional analysis. In contrast, the persistent homology and multi-scale Hochschild spectra support high-dimensional analysis by utilizing truncated path algebras, balancing computational efficiency with the ability to extract complex topological features.
These comparisons demonstrate the unique advantages of the multi-scale Hochschild spectra over traditional approaches, particularly in its capacity to handle directed edges, integrate harmonic and non-harmonic information, and efficiently analyze high-dimensional structures.
In the previous sections, we explore Hochschild cohomology of path algebra and multi-scale Hochschild spectra. The harmonic information provided by the multi-scale Hochschild spectra is reflected in the Betti numbers, while its non-harmonic information is captured by the positive eigenvalues. In this section, our main work is to demonstrate how to extract Hochschild characteristics from the three-dimensional structure of molecules. We will not perform a deeper analysis of these Hochschild characteristics in this work, as there are many powerful methods for analyzing topological features, including deep learning and natural language models [11].
In this work, we will consider two of the most common drugs: ibuprofen (C13H18O2) and aspirin (C9H8O4). Ibuprofen is a nonsteroidal anti-inflammatory drug (NSAID) commonly used to reduce pain, fever, and inflammation in conditions such as headaches and arthritis. Aspirin, also an NSAID, is used to relieve pain and inflammation and, in low doses, helps reduce the risk of heart attacks and strokes due to its blood-thinning effects.
As shown in Figure 3(a), green balls represent hydrogen atoms, brown balls represent carbon atoms, and blue balls represent oxygen atoms. Based on the electronegativities of these atoms, carbon: 2.55, hydrogen: 2.20, and oxygen: 3.44, we can determine the directed edges in the graph, with the direction always pointing from the atom with lower electronegativity to the atom with higher electronegativity. For any filtration parameter ε, we connect atoms within a distance of ε with directed edges, resulting in a digraph. As the filtration parameter increases, we obtain a filtration of digraphs. Subsequently, we compute the Hochschild cohomology and Hochschild Laplacian to obtain the Hochschild Betti number β0,0H,N and the smallest positive eigenvalue λ0,0H,N. Here, we typically use N=2, indicating the use of the 2-truncated path algebra.
As shown in Figure 2(a), the benzene molecule, composed of 6 carbon atoms and 6 hydrogen atoms, develops directed edges between atoms as the filtration parameter increases. At a parameter value of approximately 1.086 Å, directed edges from hydrogen atoms to carbon atoms appear. At around 1.395 Å, two directed edges emerge between each pair of adjacent carbon atoms, pointing to various carbon atoms. At approximately 2.154 Å, six new directed edges from hydrogen atoms to carbon atoms are formed. This process continues, resulting in a filtration of digraphs. Figure 2(b) shows the variation of the Hochschild Betti number and the smallest positive eigenvalue for benzene.
For ibuprofen and aspirin, we can similarly obtain their corresponding filtrations of digraphs, analogous to the treatment of the benzene structure, and compute their Hochschild topological features. Figures 3(a) and (b) provide graphical representations of ibuprofen and aspirin, respectively. Figures 3(c) and (d) show the Hochschild topological features for ibuprofen and aspirin, namely the Hochschild Betti number β0,0H,2 and the smallest positive eigenvalue λ0,0H,2. For more general values of N, our calculations follow a similar approach; however, computations for higher-dimensional Laplacians become more complex. Therefore, the algorithm still has potential for improvement.
In our computations, we calculated the filtration parameter from 0 to its maximum value, which ensures that any two points are connected. The filtration range shown in Figures 2 and 3 is chosen from 0 to 5 Å to highlight the variations in Betti numbers, which are primarily observed within the 1–1.5 Å range. The computational cost for calculating the Hochschild spectra for ibuprofen and aspirin is approximately 2.05 seconds and 0.38 seconds, respectively. These computations were performed on a personal laptop equipped with an AMD Ryzen 5 5600H processor, Radeon Graphics, and 16 GB of RAM. We believe these computation times are reasonable for larger datasets and can be further optimized when executed on a dedicated server with higher computational resources.
The development of topological data analysis has become increasingly advanced, with a growing array of topological and geometric methods being explored. It is challenging to determine the absolute effectiveness of these methods, as their performance can vary depending on the dataset and application context. Generally, a method is considered effective if it meets at least two criteria: first, it should have a suitable framework for modeling the dataset; second, it should ensure computational efficiency. The multiscale Hochschild spectral analysis developed in this work meets both of these criteria. This method is applicable to digraph data and can even model certain weighted point cloud data. Additionally, the corresponding Hochschild spectral information is relatively straightforward to compute.
In this work, we introduce the Hochschild Laplacians for digraphs and propose a Hochschild spectral analysis for digraph data. Compared to the classical spectral analysis of graphs, Hochschild spectral analysis offers a new perspective based on a different mathematical theory. We begin by providing an overview of Hochschild cohomology and path algebras for digraphs. For digraphs with directed cycles, the path algebra is infinite-dimensional, as shown in Proposition 2.2. To address this, we consider truncated path algebras, which are finite-dimensional. We present some interesting results on truncated path algebras, such as the relationship between the 1st Hochschild Betti number and the Euler characteristic of the digraph, as discussed in Theorem 3.3. Additionally, we introduce the Hochschild Laplacian, whose harmonic spectral information aligns with Hochschild cohomology, as stated in Theorem 3.4, while its non-harmonic components provide further geometric insights. We also compute the matrix representation of the Hochschild Laplacian and find connections between this matrix and the classical graph Laplacian, with details provided in Section 3.3. Furthermore, we define and compute the multiscale Hochschild spectra. Finally, we apply this method to calculate the Hochschild Betti numbers and the smallest positive eigenvalue for common drug molecules such as ibuprofen and aspirin, which serve as visualizable topological features. The proposed framework not only extends Hochschild cohomology to a practical tool for digraph analysis but also achieves a balance between computational feasibility and the richness of extracted features, demonstrating its potential in both theoretical and applied domains.
This work introduces a novel method for processing graph data; however, it has certain limitations that require further refinement. From a theoretical perspective, extending Hochschild cohomology into a persistent cohomology framework remains challenging, largely because Hochschild cohomology is not functorial with respect to path algebras. In terms of applications, while we have demonstrated the potential of Hochschild spectral analysis in extracting features of molecular structures, its application to concrete datasets through topological feature analysis remains underdeveloped. Future research will address these challenges. Additionally, we focus on further optimization of computational methods for handling large-scale digraphs. Moreover, integrating the multi-scale Hochschild spectra with machine learning models for feature extraction and prediction opens new possibilities for interdisciplinary applications, bridging topology and data-driven approaches. Consequently, there is significant scope for further exploration of Hochschild spectral analysis, and we anticipate that it will provide valuable insights in both theoretical and applied topology.
Yunan He: Formal analysis, Created visualizations, Validated results, Writing–original draft. Jian Liu: Designed project, Writing–original draft, Writing–review and editing. All authors have read and approved the final version of the manuscript for publication.
The authors declare that they have not used Artificial Intelligence (AI) tools in the creation of this article.
This work was supported in part by the Natural Science Foundation of China (NSFC) grant (12401080), and the Natural Science Foundation of Chongqing, China (CSTB2024NSCQ-LZX0136).
The authors declare that there is no conflict of interest.
[1] |
Y. Ahamad, U. Ali, I. Siddique, A. Iampan, W. A. Afifi, H. A. Khalifa, Computing the normalized Laplacian spectrum and spanning tree of the strong prism of octagonal network, J. Math., 2022 (2022), 9269830. https://doi.org/10.1155/2022/9269830 doi: 10.1155/2022/9269830
![]() |
[2] |
P. Bendich, J. Harer, Persistent intersection homology, Found. Comput. Math., 11 (2011), 305–336. https://doi.org/10.1007/s10208-010-9081-1 doi: 10.1007/s10208-010-9081-1
![]() |
[3] | W. Y. Bi, J. Y. Li, J. Liu, J. Wu, On the Cayley-persistence algebra, 2022, arXiv: 2205.10796. |
[4] |
Z. X. Cang, L. Mu, K. D. Wu, K. Opron, K. L. Xia, G.-W. Wei, A topological approach for protein classification, Mol. Based Math. Biol., 3 (2015), 140–162. https://doi.org/10.1515/mlbmb-2015-0009 doi: 10.1515/mlbmb-2015-0009
![]() |
[5] |
L. Caputi, H. Riihimäki, Hochschild homology, and a persistent approach via connectivity digraphs, J. Appl. and Comput. Topology, 8 (2024), 1121–1170. https://doi.org/10.1007/s41468-023-00118-9 doi: 10.1007/s41468-023-00118-9
![]() |
[6] |
G. Carlsson, Topology and data, Bull. Amer. Math. Soc., 46 (2009), 255–308. https://doi.org/10.1090/S0273-0979-09-01249-x doi: 10.1090/S0273-0979-09-01249-x
![]() |
[7] |
G. Carlsson, V. De Silva, Zigzag persistence, Found. Comput. Math., 10 (2010), 367–405. https://doi.org/10.1007/s10208-010-9066-0 doi: 10.1007/s10208-010-9066-0
![]() |
[8] |
G. Carlsson, T. Ishkhanov, V. De Silva, A. Zomorodian, On the local behavior of spaces of natural images, Int. J. Comput. Vis., 76 (2008), 1–12. https://doi.org/10.1007/s11263-007-0056-x doi: 10.1007/s11263-007-0056-x
![]() |
[9] | G. Carlsson, G. Singh, A. Zomorodian, Computing multidimensional persistence, In: Algorithms and computation, Heidelberg: Springer, 2009,730–739. https://doi.org/10.1007/978-3-642-10631-6_74 |
[10] |
G. Carlsson, A. Zomorodian, The theory of multidimensional persistence, Discrete Comput. Geom., 42 (2009), 71–93. https://doi.org/10.1007/s00454-009-9176-0 doi: 10.1007/s00454-009-9176-0
![]() |
[11] |
D. Chen, J. Liu, G.-W. Wei, Multiscale topology-enabled structure-to-sequence transformer for protein–ligand interaction predictions, Nat. Mach. Intell., 6 (2024), 799–810. https://doi.org/10.1038/s42256-024-00855-1 doi: 10.1038/s42256-024-00855-1
![]() |
[12] |
D. Chen, J. Liu, J. Wu, G.-W. Wei, Persistent hyperdigraph homology and persistent hyperdigraph Laplacians, Found. Data Sci., 5 (2023), 558–588. https://doi.org/10.3934/fods.2023010 doi: 10.3934/fods.2023010
![]() |
[13] |
J. H. Chen, R. D. Zhao, Y. Y. Tong, G.-W. Wei, Evolutionary de Rham-Hodge method, Discrete Cont. Dyn.-B, 26 (2021), 3785–3821. https://doi.org/10.3934/dcdsb.2020257 doi: 10.3934/dcdsb.2020257
![]() |
[14] | S. Chowdhury, F. Mémoli, Persistent path homology of directed networks, In: Proceedings of the twenty-ninth annual ACM-SIAM symposium on discrete algorithms, 2018, 1152–1169. https://doi.org/10.1137/1.9781611975031.75 |
[15] |
J. R. Clough, N. Byrne, I. Oksuz, V. A. Zimmer, J. A. Schnabel, A. P. King, A topological loss function for deep-learning based image segmentation using persistent homology, IEEE T. Pattern Anal., 44 (2020), 8766–8778. https://doi.org/10.1109/TPAMI.2020.3013679 doi: 10.1109/TPAMI.2020.3013679
![]() |
[16] |
V. De Silva, D. Morozov, M. Vejdemo-Johansson, Persistent cohomology and circular coordinates, Discrete Comput. Geom., 45 (2011), 737–759. https://doi.org/10.1007/s00454-011-9344-x doi: 10.1007/s00454-011-9344-x
![]() |
[17] | H. Derksen, J. Weyman, Quiver representations, Notices of the AMS, 52 (2005), 200–206. |
[18] |
H. Edelsbrunner, D. Letscher, A. Zomorodian, Topological persistence and simplification, Discrete Comput. Geom., 28 (2002), 511–533. https://doi.org/10.1007/s00454-002-2885-2 doi: 10.1007/s00454-002-2885-2
![]() |
[19] |
M. Gerstenhaber, S. D. Schack, Simplicial cohomology is Hochschild cohomology, J. Pure Appl. Algebra, 30 (1983), 143–156. https://doi.org/10.1016/0022-4049(83)90051-8 doi: 10.1016/0022-4049(83)90051-8
![]() |
[20] | N. Giansiracusa, R. Giansiracusa, C. Moon, Persistent homology machine learning for fingerprint classification, 2019 18th IEEE International Conference on Machine Learning and Applications (ICMLA), Boca Raton, FL, USA, 2019, 1219–1226. https://doi.org/10.1109/ICMLA.2019.00201 |
[21] |
G. Hochschild, On the cohomology groups of an associative algebra, Ann. Math., 46 (1945), 58–67. https://doi.org/10.2307/1969145 doi: 10.2307/1969145
![]() |
[22] |
Y. Jiang, D. Chen, X. Chen, T. Y. Li, G.-W. Wei, F. Pan, Topological representations of crystalline compounds for the machine-learning prediction of materials properties, npj Comput. Mater., 7 (2021), 28. https://doi.org/10.1038/s41524-021-00493-w doi: 10.1038/s41524-021-00493-w
![]() |
[23] |
J. Liu, D. Chen, F. Pan, J. Wu, Neighborhood path complex for the quantitative analysis of the structure and stability of carboranes, J. Comput. Biophys. Che., 22 (2023), 503–511. https://doi.org/10.1142/S2737416523500229 doi: 10.1142/S2737416523500229
![]() |
[24] | J. Liu, D. Chen, G.-W. Wei, Persistent interaction topology in data analysis, 2024, arXiv: 2404.11799. |
[25] |
J. Liu, J. Y. Li, J. Wu, The algebraic stability for persistent Laplacians, Homol. Homotopy Appl., 26 (2024), 297–323. https://dx.doi.org/10.4310/HHA.2024.v26.n2.a15 doi: 10.4310/HHA.2024.v26.n2.a15
![]() |
[26] |
X. Liu, X. J. Wang, J. Wu, K. L. Xia, Hypergraph-based persistent cohomology (HPC) for molecular representations in drug design, Brief. Bioinform., 22 (2021), bbaa411. https://doi.org/10.1093/bib/bbaa411 doi: 10.1093/bib/bbaa411
![]() |
[27] |
U. Lupo, A. M. Medina-Mardones, G. Tauzin, Persistence Steenrod modules, J. Appl. and Comput. Topology, 6 (2022), 475–502. https://doi.org/10.1007/s41468-022-00093-7 doi: 10.1007/s41468-022-00093-7
![]() |
[28] |
C. S. Pun, S. X. Lee, K. L. Xia, Persistent-homology-based machine learning: a survey and a comparative study, Artif. Intell. Rev., 55 (2022), 5169–5213. https://doi.org/10.1007/s10462-022-10146-z doi: 10.1007/s10462-022-10146-z
![]() |
[29] |
R. Wang, D. D. Nguyen, G.-W. Wei, Persistent spectral graph, Int. J. Numer. Meth. Bio., 36 (2020), e3376. https://doi.org/10.1002/cnm.3376 doi: 10.1002/cnm.3376
![]() |
[30] |
K. L. Xia, G.-W. Wei, Persistent homology analysis of protein structure, flexibility, and folding, Int. J. Numer. Meth. Bio., 30 (2014), 814–844. https://doi.org/10.1002/cnm.2655 doi: 10.1002/cnm.2655
![]() |
Aspect | Persistent Homology | Multi-scale Graph Spectra | Multi-scale Hochschild Spectra |
Directed Edge Support | Not supported | Undirected graphs | Supports directed edges |
Topological and Geometric Information | Harmonic only | Harmonic and non-harmonic | Harmonic and non-harmonic (via multi-scale Hochschild spectras) |
High-Dimensional Information | Supports high dimensions | Limited to 0th and 1st dimensions | Supports high dimensions |
Aspect | Persistent Homology | Multi-scale Graph Spectra | Multi-scale Hochschild Spectra |
Directed Edge Support | Not supported | Undirected graphs | Supports directed edges |
Topological and Geometric Information | Harmonic only | Harmonic and non-harmonic | Harmonic and non-harmonic (via multi-scale Hochschild spectras) |
High-Dimensional Information | Supports high dimensions | Limited to 0th and 1st dimensions | Supports high dimensions |