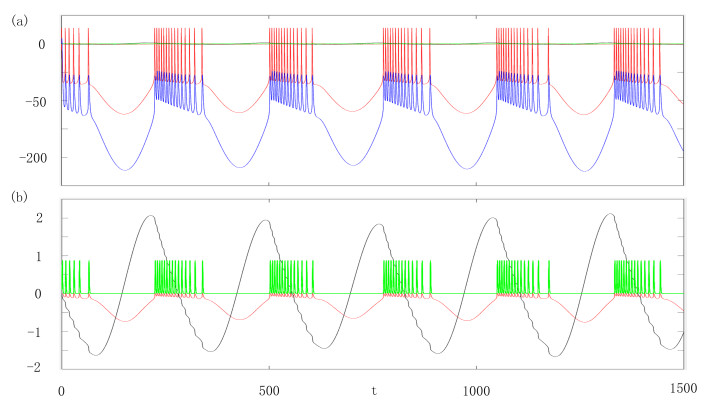
Half-center oscillators are typical small circuits that are crucial for understanding CPG. The complex rhythms of CPG are closely related to certain diseases, such as epilepsy. This paper considered the influence of electromagnetic induction on the discharge mode of the half-center oscillators. First, we analyzed the response of individual firing neuron rhythms to electromagnetic induction when the slow-variable parameters vary. We also discussed the changes in the dynamic bifurcation structure when the intensity of electromagnetic induction varies. Furthermore, we determined the effects of mutually inhibitory and self-inhibitory synaptic parameters on the firing rhythm of the half-center oscillators. The different responses induced by electromagnetic induction interventions, showed that mutually inhibitory synapses modulate the firing rhythm weakly and self-inhibition synapses have a significant impact on firing rhythm. Finally, with the change of synaptic parameter values, the combined effects of autapse and mutually inhibitory synapses on the discharge rhythm of half-center oscillators were analyzed in symmetric and asymmetric autapse modes. It was found that the synchronous state of the half-center oscillators had a more robust electromagnetic induction response than the asynchronous state.
Citation: Feibiao Zhan, Jian Song. Complex rhythm and synchronization of half-center oscillators under electromagnetic induction[J]. Electronic Research Archive, 2024, 32(7): 4454-4471. doi: 10.3934/era.2024201
[1] | Feibiao Zhan, Jian Song, Shenquan Liu . The influence of synaptic strength and noise on the robustness of central pattern generator. Electronic Research Archive, 2024, 32(1): 686-706. doi: 10.3934/era.2024033 |
[2] | Qixiang Wen, Shenquan Liu, Bo Lu . Firing patterns and bifurcation analysis of neurons under electromagnetic induction. Electronic Research Archive, 2021, 29(5): 3205-3226. doi: 10.3934/era.2021034 |
[3] | Xiaojing Zhu, Yufan Liu, Suyuan Huang, Ranran Li, Yuan Chai . Modulation of epileptiform discharges by heterogeneous interneurons and external stimulation strategies in the thalamocortical model. Electronic Research Archive, 2025, 33(4): 2391-2411. doi: 10.3934/era.2025106 |
[4] | Ariel Leslie, Jianzhong Su . Modeling and simulation of a network of neurons regarding Glucose Transporter Deficiency induced epileptic seizures. Electronic Research Archive, 2022, 30(5): 1813-1835. doi: 10.3934/era.2022092 |
[5] | Yuzhi Zhao, Honghui Zhang, Zilu Cao . The important role of astrocytes in activity pattern transition of the subthalamopallidal network related to Parkinson's disease. Electronic Research Archive, 2024, 32(6): 4108-4128. doi: 10.3934/era.2024185 |
[6] | Moutian Liu, Lixia Duan . In-phase and anti-phase spikes synchronization within mixed Bursters of the pre-Bözinger complex. Electronic Research Archive, 2022, 30(3): 961-977. doi: 10.3934/era.2022050 |
[7] | Yapeng Zhang, Yu Chen, Quanbao Ji . Dynamical analysis of spontaneous Ca2+ oscillations in astrocytes. Electronic Research Archive, 2024, 32(1): 405-417. doi: 10.3934/era.2024020 |
[8] | Janarthanan Ramadoss, Asma Alharbi, Karthikeyan Rajagopal, Salah Boulaaras . A fractional-order discrete memristor neuron model: Nodal and network dynamics. Electronic Research Archive, 2022, 30(11): 3977-3992. doi: 10.3934/era.2022202 |
[9] | Taher S. Hassan, Amır AbdelMenaem, Mouataz Billah Mesmouli, Wael W. Mohammed, Ismoil Odinaev, Bassant M. El-Matary . Oscillation criterion for half-linear sublinear functional noncanonical dynamic equations. Electronic Research Archive, 2025, 33(3): 1351-1366. doi: 10.3934/era.2025062 |
[10] | Hao Yang, Peihan Wang, Fang Han, Qingyun Wang . An interpretable mechanism for grating-induced cross-inhibition and gamma oscillation based on a visual cortical neuronal network model. Electronic Research Archive, 2024, 32(4): 2936-2954. doi: 10.3934/era.2024134 |
Half-center oscillators are typical small circuits that are crucial for understanding CPG. The complex rhythms of CPG are closely related to certain diseases, such as epilepsy. This paper considered the influence of electromagnetic induction on the discharge mode of the half-center oscillators. First, we analyzed the response of individual firing neuron rhythms to electromagnetic induction when the slow-variable parameters vary. We also discussed the changes in the dynamic bifurcation structure when the intensity of electromagnetic induction varies. Furthermore, we determined the effects of mutually inhibitory and self-inhibitory synaptic parameters on the firing rhythm of the half-center oscillators. The different responses induced by electromagnetic induction interventions, showed that mutually inhibitory synapses modulate the firing rhythm weakly and self-inhibition synapses have a significant impact on firing rhythm. Finally, with the change of synaptic parameter values, the combined effects of autapse and mutually inhibitory synapses on the discharge rhythm of half-center oscillators were analyzed in symmetric and asymmetric autapse modes. It was found that the synchronous state of the half-center oscillators had a more robust electromagnetic induction response than the asynchronous state.
Central pattern generator (CPG) rhythms have been discovered in invertebrates such as crustacean pyloric or gastric and leech heartbeats. CPGs are typical small circuits of neural networks, providing a new perspective on how circuit dynamics depend on neurons and synapses. CPG can spontaneously generate multiple rhythmic patterns in the absence of external stimuli [1,2], and it is associated with various motor behaviors, such as swimming and walking [3,4]. The characteristics of CPG are described as the stability and robustness of the firing rhythm of neuronal small circuits. Recently, a large amount of research has focused on the working mechanism of CPG from both theoretical and experimental perspectives, providing a biological theoretical basis for the study of CPG-inspired rhythmic motion control [5,6,7,8]. Still, only some have directly studied the discharge rhythm of CPG. However, how to efficiently control and use the flexibility and robustness of CPG to guide research inspired by it is still being explored. Some researchers believe that the connection of CPG forms attractors, and each attractor corresponds to a rhythmic motor behavior [9]. Theoretical researchers have explained the potential internal mechanisms of CPG from a dynamic perspective [10], revealing the mechanism of the emergence, disappearance, and stabilization of neuronal rhythms in a tri-neuron network with mutual inhibition under changes in synaptic parameters. Lu et al. investigated the synchronization and stochastic resonance characteristics of small-world networks based on CPG [11]. Researchers have studied the impact of transient input on neuronal firing using phase response curves[12,13].
Recent studies have shown how the morphology of neurons can significantly affect the rhythmic patterns of CPG circuits [14]. There are many studies on the mechanism of neuronal rhythm generation [15,16,17,18,19], but it is unclear whether the different firing mechanisms of neurons mean the diversity of CPG circuit functions. Can the same CPG circuit generate multiple motion behaviors, making its motion functions diverse [20,21]. Research has shown that the generation of motor behavior depends on the rhythmic modulation of CPG. One proposal suggests that the CPG rhythm pattern can simulate the human central system's rhythmic activity and control the arm movement rhythm [22]. In addition, CPG rhythm patterns can improve the diagnosis of multiple diseases. For instance, Mader et al. [1] explained that CPG can assist in developing therapeutic methods for the recovery of spinal solid cord injuries. Tassinari et al. reviewed the relationship of central pattern generators with parasomnias and sleep-related epileptic seizures. They explained some epileptic seizures and parasomnias using the rhythm patterns of the CPG [23,24]. Therefore, studying the functioning principle of CPG rhythm activities is essential for understanding related motor behaviors, improving motor control and disease diagnosis. It is necessary to examine the diversity of rhythm patterns to understand the function of CPG. The study of CPG rhythm patterns has recently been discussed [25,26].
A half-center oscillator (HCO) is a unit composed of two neurons that mutually inhibit each other, and studying the rhythmic pattern of HCO is crucial for understanding CPG [27,28]. Studying motion control is facilitated by the easy identification of HCO rhythm patterns. Researchers discussed how neuron parameters affect the rhythmic patterns of HCO to achieve motion control [29], and analyze how the system parameter space changes the robustness of HCO rhythmic patterns [30]. Recently, delayed half-center oscillator (DHCO) in-phase and antiphase dynamics behavior and the coexistence of multiple routes leads to chaos was studied[31,32]. Noise closely affects the robustness of HCO rhythm patterns [33], and the impact of electromagnetic induction on neuronal firing rhythms and diseases such as epilepsy cannot be ignored [34,35]. Few people have paid attention to the effects of electromagnetic induction and connection modes on the HCO discharge rhythm. Here, the synaptic conductance parameters and the effects of electromagnetic induction on the HCO rhythm pattern are analyzed. Mutual inhibitory neurons can improve the stability of HCO. Here, we explore the rhythmic pattern of HCO under electromagnetic induction, and a model containing self-inhibitory synapses and mutual inhibitory synapses is established. We examine the impact of electromagnetic induction and connection modes on the HCO rhythm pattern.
This study investigates complex rhythm and synchronization of HCOs under electromagnetic induction. First, the dynamic changes of individual neurons under electromagnetic induction are analyzed. Moreover, we discuss the effects of mutual inhibition and self-inhibition synaptic parameters on the rhythmic patterns of HCO and show their different responses to electromagnetic induction. The results indicate that mutual inhibition has a weaker effect on the discharge rhythm than self-inhibition. The study examines the impact of self-inhibition synapses, mutual inhibitory synapses, and electromagnetic induction on the HCO rhythm pattern, both symmetrically and asymmetrically. It has been found that the synchronous state of the HCO exhibits a more robust electromagnetic induction response than the asynchronous state. Rhythm patterns are closely related to motor control. The research of rhythmic patterns of small circuits contributes to the development of intelligent science. Intelligent science is inspired by the principle of biological CPG and the establishment of spiking neural networks [36,37].
The paper is organized as follows. Section 2 presents the materials and methods we used to build HCO, including the Hodgkin–Huxley (HH) neuron model. Then, Section 3.1 illustrates the rhythmic discharge of HCOs with different synaptic coupling. Section 3.2 investigates the synchronization of HCOs with different symmetric and asymmetric autapses. Finally, we have a discussion and conclusion in Section 4.
In our CPG, each interneuron is modeled by a simplified HH model. Variable ϕ denotes the magnetic flux across the membrane, and ρ(ϕ) represents the incremental memductance function of flux controlled memristor [38], which is used to describe the coupling between membrane potential and magnetic flux. The incremental memductance function is often described by ρ(ϕ)=α+3βϕ2, and α,β are fixed parameters [38,39]. The term kρ(ϕ)V could be viewed as induction current on the membrane as follows:
i′=dq(ϕ)dt=dq(ϕ)dϕdϕdt=ρ(ϕ)V0=kρ(ϕ)V. |
This microcircuit captures the dynamics by a single compartment, which is described by the following differential equations [17,34,40]:
CmdVdt=−INa−IK−ILeak+I+kρ(ϕ)V,dndt=n∞−nτn,dIdt=ε(−80−V).dϕdt=(k1V−k2ϕ). | (2.1) |
Here, Cm denotes the neural membrane capacitance density (μF/cm2); V represents the membrane potential (mV); the gating variable n of activated K+ channels denotes the activation probability of potassium ion channels; the h variable (the formulations of INa in the original model) represents a merged refractory variable (Na+ inactivation and K+ activation). It is replaced by 1−n in the simplified HH model. So, the n gate appears in the formulations of INa. τn is the time constant (ms); I is a voltage-dependent linear control current; electromagnetic induction parameters are α=0.1,β=0.02,k1=0.9,k2=0.5; and INa, IK, and ILeak are sodium ion current, potassium ion current, and leakage current. Their expressions are as follows.
INa=gNam∞(V)(1−n)(V−ENa),IK=gKn(V−EK),ILeak=gL(V−EL), |
where the parameters gNa and gK are the maximal conductances (mS/cm2); ENa, EK, and EL are the reversal potentials (mV); and m∞ and n∞ are the steady-state of the ionic gating channels. They are modeled using:
m∞(V)=11+exp(−V+355),n∞(V)=11+exp(−V+365). |
First, we present a time series diagram of the neuron model under electromagnetic induction. It is found that the membrane potential of neurons exhibits a regular burster discharge pattern (in the Figure 1 red trace), and the time series of the electromagnetic induction parameter ϕ (in the Figure 1 blue trace) and the peak time of the membrane potential sequence are synchronous. The electromagnetic induction parameter ϕ affects the membrane potential of neurons at all times, although their amplitudes differ. The neuronal membrane potential strongly responds to the fluctuations of electromagnetic induction parameter ϕ. We enlarge the green curve near the zero line in the Figure 1(a) to obtain Figure 1(b), where the green trajectory represents the time series of the gating variable; the black trajectory represents the feedback current I, which is a slow regulating variable. The red trajectory shows the sequence diagram of the electromagnetic induction term ρ(ϕ) changing over time. Figure 1(b) shows that compared to the slowly regulated variable I, the change in the electromagnetic induction term is weaker, but the regulation of the system variables does not weaken. Its impact on the system is equivalent to adding external stimuli that change over time with a red trajectory and the membrane potential time series displayed by the system under this external stimulus. Below, we will provide the trend of the dynamic bifurcation diagram of the system as the electromagnetic induction parameters change.
From the above analysis, we know that the neuronal system's membrane potential strongly responds to electromagnetic induction as an external stimulus. Here, we take the feedback current I of the system as a slow variable and provide a bifurcation diagram of the system with the participation of electromagnetic induction. First, we can see that the system membrane potential exhibits burster discharge with ten peaks per burster when the electromagnetic induction parameter k=0 (in the Figure 2(c)). With k increased to 0.000005, the positive response of the system membrane potential to electromagnetic induction leads to a significant increase in the number of peaks per burster (in the Figure 2(d)). Based on the review about classication of bursters[15], here may show a Circle/Circle bifurcation type. With k reduced to −0.000008, electromagnetic induction exhibits inhibition of action potential, resulting in burster discharge of membrane potential with only 4 peaks appearing in each burster (in the Figure 2(b)). When the electromagnetic induction k=−0.000012, the membrane potential exhibits 2 peaks per burster (in the Figure 2(a)). If the electromagnetic induction k further decreases, the system membrane potential sequence will exhibit a single spiking.
Here, we investigate the dynamic changes in membrane potential discharge rhythm by adding electromagnetic induction under different slow variable parameters ε. In the Figure 3, we can see that the discharge rhythm of the membrane potential exhibits a regular rectangular wave burster (in the Figure 3(b1)) when the parameter ε=0.001, and the discharge rhythm of the membrane potential exhibits a parabolic burster when electromagnetic induction is added to the system (in the Figure 3(a1)). The number of peaks per burster significantly increases. When the parameter ε=0.005, the system exhibits a regular burster discharge rhythm pattern, and the number of bursters within the same time interval significantly increases (in the Figure 3(b2)), and the burster interval decreases. The addition of electromagnetic induction transforms the discharge rhythm of the membrane potential from an initial irregular burster to a regular burster, and the number of peaks per burster increases (in the Figure 3(a2)). The membrane potential of the system exhibits a periodic burster when the parameter ε=0.01 (in the Figure 3(b3)), and two peaks appear in each burster, with a smaller burster interval than the first two groups. Adding electromagnetic induction results in an irregular discharge rhythm of the membrane potential. The system's sensitivity increases with the addition of electromagnetic induction under this parameter (in the Figure 3(a3)). In summary, electromagnetic induction significantly impacts the membrane potential rhythm of individual neurons.
Next, we will consider the improved firing rhythm of two neurons that mutually inhibit each other, also known as HCOs. We have added two self-synapses of neurons here. Considering how the synchronization of neurons changes with changes in two sets of synaptic parameters, we first examine whether inhibition from autapse and mutual inhibition is consistent. Furthermore, we research HCOs in both symmetric and asymmetric autapse modes. When only g12 is not 0 and only g11 or g22 is not 0, we investigate the difference in the firing rhythm of neurons. The inhibitory relationship between neurons is a crucial factor in rhythm generation. The most common structure describing firing rhythmic activities consists of two coupled neurons that inhibit each other (in the Figure 4). This structure is widely known as an HCO and is symmetrically coupled through both inhibitory connections with g12 and g21. We will start with the most straightforward network where two cells next section and extended self-inhibition from neurons. Neurons are color-coded (blue and green) based on firing activity. The synaptic transmission in the structure of the HCO in the Figure 4 is ionotropic synapses. First-order kinetic equations model these synapses, which are inhibitory and conductance-based, similar to previous studies [41,42,43,44]:
{Ipre→post=gpre→postH∞(Vpre)(Vpre−Epre→post),H∞(Vpre)=11+exp(−Vpre−θσ). | (2.2) |
Here, Vpre is the presynaptic voltage, σ=1mV is the steepness, θ=−60mV sets the value when the function is semi-activated, Epre→post=−110 is the reversal potential, and gpre→post is the maximal conductance (gij, i,j=1,2 in the Figure 4).
For numerical integration of network system, the fourth-order Runge–Kutta algorithm was used with a time step of 0.05 ms. The total integration time length of each simulation run was 5000 ms. Simulations were implemented in Python 3.9.7 on PC with 12th Gen Intel(R) Core(TM) i7-12700H 2.30 GHz CPU.
The section discusses the reciprocal inhibition between two neurons, which are HCOs (in the Figure 4). The synchronous firing of neurons contains essential neural information. Here, we investigate the synchrony of HCO rhythm activity. First, we investigate the firing rhythm when the synaptic conductance g12 is not zero; only neuron 1 inhibits neuron 2. When g12=0, neurons 1 and 2 have a wholly synchronized discharge pattern (in the Figure 5(b1)), which is reasonable. By changing g12 to 0.02 in Figure 5(b2), it is found that the firing rhythm of neuron 1 does not change, while the firing rhythm of neuron 2 gradually changed from the state of synchronous neuron 1 (the first two burster patterns) to an asynchronous state, until it reached an asynchronous state. When g12=0.2, the discharge mode of neuron 1 remains unchanged, and the discharge modes of the two neurons directly exhibit a strictly asynchronous state (in the Figure 5(b3)), with the burster mode states of neurons 1 and 2 alternating. We obtain the firing rhythm on the left side of Figure 5 when only neuron 1 in the system adds electromagnetic induction. We can observe that the rhythmic pattern of neuron 1 under electromagnetic induction is consistent under all three sets of coupled conductance parameters. Under weak coupling conductance (g12=0.02), the rhythmic pattern of neuron 2 transitions to irregular discharge, meaning that the discharge rhythm of neuron 2 is greatly affected by electromagnetic induction (in the Figure 5(a2)). At a relatively strong coupling conductance coefficient (g12=0.2) (in the Figure 5(a3)), the burster firing rhythm pattern of neuron 2 is strengthened. We can see a significant increase in the number of peaks per burster, and the stability of the burster pattern is also more robust.
Here, we consider the discharge rhythm when the self-inhibitory synaptic conductance changes, where g12=g21=0. It is evident from the righthand side of Figure 6 that the firing neuron 2 has a consistent firing rhythm, unaffected by neuron 1 within a fixed g22. Its firing rhythm is different from that when the coupling conductance g11=0 (blue trace in the Figure 6(b1)), and at this point, the firing rhythm of neuron 1 (in the Figure 6(b1)) is entirely consistent with that of neuron 1 in the Figure 5(b1). In the Figure 6(b2), when g11=0.2, neuron 1 shows a regular burster pattern with four peaks in each burster. This pattern is distinct from the firing rhythm pattern in the Figure 5(b3), where the coupling parameter g12 between neuron 1 and neuron 2 is 0.2. The different firing patterns might indicate that the inhibitory effects of autapse and mutually inhibitory synaptic conductance on neurons are different. This is an exciting observation worth noting. Here, self-inhibition reduces the number of peaks per burster in neuron 1 (in the Figure 6(b2)). When increasing g11 to 0.35, the discharge pattern of neuron 1 exhibits an irregular rhythm, appearing to be a burster pattern with three peaks in each burster (in the Figure 6(b3)). Continuing to increase the self-coupling synaptic conductance g11, the firing rhythm of neuron 1 may transform into a burster rhythm pattern with two peaks in each burster and a regular burster discharge pattern, which is not shown here. These all indicate that self-synaptic coupling is essential to regulating the diversity of discharge rhythms. The left side of Figure 6 shows the discharge rhythm pattern obtained by adding electromagnetic induction to the system. In all three cases, the discharge pattern of neuron 2 is undoubtedly wholly consistent. The firing pattern of neuron 1 (in the Figure 6(b1)) is consistent with the firing rhythm of neuron 1 in the Figure 5(b1) when g11=0.2 (in the Figure 6(a2)), and the addition of electromagnetic induction increases the number of peaks per burster in the neuron 1. When g11=0.35, the irregular discharge pattern of neuron 1 remains irregular under electromagnetic induction as if the number of peaks in each burster increases. Under these three types of autapse, perhaps electromagnetic induction enhances the discharge of membrane potential. We also found that the impact of electromagnetic induction on the discharge mode of HCOs varies depending on the different synaptic connections. The effects of self-synaptic inhibition and mutual inhibition on the firing patterns of neurons are also other, as shown by comparing Figures 5 and 6. Therefore, this suggests that the effects of electromagnetic induction on HCOs must be carefully considered in terms of their synaptic connections.
Here, we examine the synchronization state of two neurons with a half-center oscillator by phase difference Δ(n) and average phase difference S=1N∑Nn=1Δ(n)(N is the number of samples within the range of values of the vertical coordinate parameter)[45], as shown in the Figure 7. Figure 7(a1) shows the branch diagram of the phase difference when two mutually inhibitory coupling conductance and self-synaptic conductance are equal, respectively, under the introduction of electromagnetic induction in neuron 1. The calculation results show that, without introducing electromagnetic induction, the HCO exhibits a fully synchronized state. We will discuss the discharge mode of the HCO under symmetric self-synaptic conductance, i.e., fixed self-synaptic conductance g11=g22=0 (in the Figure 8) and g11=g22=0.2 (in the Figure 9). When we fixed g11=0.2 and g12=0.1, we analyzed the synchronous branches of the HCO in the presence or absence of electromagnetic induction (in the Figure 7(a2), (a3)), respectively. It can be observed that the addition of electromagnetic induction fundamentally changes the phase difference of the HCO. We show the change in phase difference before and after the addition of electromagnetic induction in the Figure 7(b), which is obtained by taking the difference in phase difference at the corresponding points in the Figure 7(a2), (a3) as the dependent variable. The independent variables are conductance g21 and g22. We will also discuss the discharge rhythm of the HCO under asymmetric self-coupling conductance (in the Figure 10).
We present the phase difference diagram of the HCO when electromagnetic induction stimulates neuron 1, and when the autapse conductance and mutual inhibition conductance are equal, respectively (in the Figure 7(a1)). We also calculated that when the autapse conductance and mutual inhibition conductance are equal (not shown in the Figure), the HCO, without introducing electromagnetic induction, is in an absolute and completely synchronized state. Here, Figure 8(b2) is a special case of complete synchronization (where g11=g22=0, g12=g21=0.2). At this point, whether reducing the conductance g12 to 0.02 or increasing g12 to 0.4 (in the Figure 8(b1), (b3)), not only will it change the synchronization state of the discharge patterns of the two neurons, but it will also affect the discharge rhythm patterns of the two neurons. We observed that the addition of electromagnetic induction to the synchronized state not only fundamentally altered the synchronization of the two neurons but also resulted in significant changes in the firing neuron rhythms (as shown in the Figure 8(a2)). This suggests that the discharge mode of the system in the synchronous state is more responsive to the addition of electromagnetic induction than the asynchronous rhythm modes. These findings are consistent with our previous analysis. The number of peaks in each neurons 1 and 2 burster in the HCO gradually increases as the conductance parameter g21 increases from 0.02 to 0.4. Electromagnetic induction seems to make the peak changes in the firing rhythm of neurons not continuous in each burst but rather a direct burst of multiple peaks. Therefore, electromagnetic induction has a more profound impact on the synchronization of HCOs.
According to the phase difference analysis above, the two neurons in the HCO exhibit a fully synchronized state when the autapse conductance and mutual inhibition conductance are equal, respectively, and no electromagnetic induction is introduced (not shown in the Figure). Figure 8(b2) shows the discharge rhythm when g11=g22=0. In the Figure 9(b2), neurons 1 and 2 demonstrate an HCO with synchronized discharge rhythm when autapse and mutual inhibition conductance parameters are 0.2. Here, we present the discharge rhythms of the HCO when the two autapse conductance parameters are equal to 0 and 0.2, respectively (in the Figure 8(b2) and Figure 9(b2)). It is evident that the conductance parameters of autapse significantly affect the neuron's discharge rhythm. With g12 reduced to 0.02 (in the Figure 9(b1)), synchronization disappears, peak count decreases, and amplitude increases. When increasing g12=0.4 (in the Figure 9(b3)), the synchronization state of the system will also disappear, the amplitude of the peaks will increase, and the number of peaks in each burster will decrease, but it is still more than when g12=0.02. In an HCO, the left side of Figure 9 is obtained when electromagnetic induction stimulates neuron 1, and we can see that the synchronization state of the system immediately disappears (in the Figure 9(a2)). Neuron 1 is more sensitive to electromagnetic induction when g12=0.02 (in the Figure 9(a1)), while at this time, neuron 2 has almost no change in the peak number of each burster except for the change in the initial discharge time of the burster. When the value of g12 is 0.4 (in the Figure 9(a3)), the changes in the firing rhythms of neurons 1 and 2 show an enhanced response to electromagnetic induction. From the variation of the discharge rhythm from top to bottom on the left side, we can see that the larger the g12, the better the robustness of the HCO, and the discharge rhythm in the synchronous state is more sensitive to the intervention of electromagnetic induction.
Previously, we discussed the discharge rhythms of HCOs in two cases where the conductance of two autapses is equal. Here, we will consider the discharge rhythm of the HCO at g11=0.2, g12=0.1, g21=0.2, and g22=0.1 (in the Figure 10(a2), (b2)), that is, how the discharge rhythm of the HCO changes when the autapse conductance is not equal (corresponding to a position point in the Figure 7(a2), (a3)). In addition, we also discussed the discharge rhythms of the system at g12=0.02 and 0.4, as shown in the Figure 10 (a1), (b1), (a3), (b3), respectively. Compared with Figure 9(b2) (g12=g22=0.2), here we change g12 and g22 from 0.2 to 0.1, respectively, to obtain Figure 10(b2) (g12=g22=0.1). The number of bursts and the intensity of the burst mode vary considerably. The discharge timing of the burster mode has undergone significant changes. When the value of g12 is 0.02, the firing rhythm of neuron 1 is significantly affected by electromagnetic induction. The peak number of each burster in neuron 2 remains unchanged, but the firing time of the burster mode is changing (in the Figure 10(a1)). When g12 increases by 0.1 or 0.4, the HCO becomes more sensitive to the addition of electromagnetic induction (in the Figure 10(a2), (a3)). The firing rhythm of neuron 1 directly receiving electromagnetic induction stimulation will undergo fundamental changes. The left panel of Figure 10 shows the firing rhythm of neuron 2. The larger the conductance g12, the better the robustness of the system, which is consistent with our previous analysis (in the Figure 9). Here, we discuss the changes in the HCO discharge patterns of symmetric autapse (in the Figures 8 and 9) and asymmetric autapse (in the Figure 10), as well as the influence of electromagnetic induction on the discharge patterns. It is important to consider the connection modes of autapse and non-autapse connections.
In this article, we analyze the discharge rhythm of an individual neuron model under electromagnetic induction and demonstrate it as a classical burster pattern. We show the time series of slow variables, gate variables, and the electromagnetic induction term ρ(ϕ). It is found that the electromagnetic induction term ρ(ϕ) has a minor regulatory effect on the firing rhythm of neurons compared to slow variables. Moreover, we demonstrate an individual neuron's fast-slow dynamics branch diagram, which varies with different electromagnetic induction parameters. We discover that electromagnetic induction has a profound impact on the dynamic characteristics of individual neurons. Next, we examined the firing rhythms of three groups of neurons with different slow variable parameters ε. We concluded that the addition of electromagnetic induction fundamentally changes the firing rhythm of an individual neuron. We are interested in the effect of electromagnetic induction on the HCO. Here, we examine the improved HCO discharge mode, which includes the addition of autapse. The impact of autapse and mutually inhibitory synapses under electromagnetic induction on the rhythmic patterns of the HCO are considered (in the Figures 5 and 6). When the synaptic parameter values are the same, there is a significant difference in the performance of self-inhibitory synaptic and mutually inhibitory synaptic discharge patterns. Electromagnetic induction affects the discharge rhythm of the HCO differently based on the type of synapses, whether self-inhibitory or mutually inhibitory. Therefore, it is essential to take note of the connection modes of the synapses during the experiment.
Furthermore, we investigate the synchronization phenomenon of the improved HCO. The change in phase difference of the HCO with and without the introduction of electromagnetic induction is analyzed when synaptic parameters change. We discuss a comparison plot of the phase difference before and after the introduction of electromagnetic induction, which more intuitively shows the change in phase difference caused by the introduction of electromagnetic induction. By further exploring the discharge modes of the HCO and summarizing the changes in the discharge modes of the two symmetric autapses (in the Figures 8 and 9), it can be found that the discharge mode in the synchronous state of the system has a more robust response to electromagnetic induction compared to the asynchronous rhythmic mode. It is apparent that the larger the conductance g12, the greater the robustness of the HCO. At the same time, the discharge rhythm of neuron 1 directly receiving electromagnetic induction stimulation changes significantly, while the discharge rhythm of neuron 2 passively receiving stimulation is affected by the value of g12. The larger the value of g12, the stronger the response of neuron 2. Finally, we analyze the changes in the discharge mode of the HCO with changes in synaptic conductance parameters under asymmetric autapse. The response patterns of the HCO of symmetric and asymmetric autapse to electromagnetic induction are different, manifested by the diverse discharge modes of the HCO.
Therefore, these rules of the HCO need to be paid attention to, including different synaptic connections, the response of different synaptic connections to electromagnetic induction, the different effects of electromagnetic induction on its synchronization, and the different effects of symmetric and asymmetric autapse on the discharge mode of the HCO. In our study, we examined chemical synapses between neurons and did not consider any electrical coupling that may have been present between them. In future studies, we will analyze the synergistic effects of electrical and chemical coupling in HCO or CPG, which can lead to very different rhythmic patterns. Our current model demonstrates that synaptic conductance can influence the rhythm pattern of the HCO. The diversity of rhythm activities in CPG is essential for its flexibility and robustness, which can resist interference from external information. Synaptic conductance and electromagnetic induction changes can produce many different rhythm activities, making the CPG less susceptible to external interference. Of course, the relationship between rhythmic activities of HCO (or CPG) and epilepsy and other diseases will also be paid attention to.
The authors declare they have not used Artificial Intelligence (AI) tools in the creation of this article.
This work was funded by the National Natural Science Foundation of China (Grant Nos. 12202208), the Basic Science (Natural Science) Research Project of Colleges and Universities of Jiangsu Province (Grant No. 22KJB130009), the Research and Cultivation Project for Young Teachers of Nanjing Audit University (Grant No. 2021QNPY015), 2022 Doctoral program of Entrepreneurship and Innovation in Jiangsu Province (Grant No. JSSCBS20220717), the China Scholarship Council (Grant No. 202206150096).
The authors declare there is no conflicts of interest.
[1] |
E. Marder, D. Bucher, Central pattern generators and the control of rhythmic movements, Curr. Biol., 11 (2001), R986–R996. https://doi.org/10.1016/S0960-9822(01)00581-4 doi: 10.1016/S0960-9822(01)00581-4
![]() |
[2] |
E. Marder, R. L. Calabrese, Principles of rhythmic motor pattern generation, Physiol. Rev., 76 (1996), 687–717. https://doi.org/10.1152/physrev.1996.76.3.687 doi: 10.1152/physrev.1996.76.3.687
![]() |
[3] |
D. N. Masaev, A. A. Suleimanova, N. V. Prudnikov, M. V. Serenko, A. V. Emelyanov, V. A. Demin, et al., Memristive circuit-based model of central pattern generator to reproduce spinal neuronal activity in walking pattern, Front. Neurosci., 17 (2023), 1124950. https://doi.org/10.3389/fnins.2023.1124950 doi: 10.3389/fnins.2023.1124950
![]() |
[4] |
D. Alaçam, A. Shilnikov, Making a swim central pattern generator out of latent parabolic bursters, Int. J. Bifurcation Chaos, 25 (2015), 1540003. https://doi.org/10.1142/S0218127415400039 doi: 10.1142/S0218127415400039
![]() |
[5] |
E. Marder, S. Kedia, E. O. Morozova, New insights from small rhythmic circuits, Curr. Opin. Neurobiol., 76 (2022), 102610. https://doi.org/10.1016/j.conb.2022.102610 doi: 10.1016/j.conb.2022.102610
![]() |
[6] |
E. Marder, Neuromodulation of neuronal circuits: back to the future, Neuron, 76 (2012), 1–11. https://doi.org/10.1016/j.neuron.2012.09.010 doi: 10.1016/j.neuron.2012.09.010
![]() |
[7] |
T. Nowotny, M. I. Rabinovich, Dynamical origin of independent spiking and bursting activity in neural microcircuits, Phys. Rev. Lett., 98 (2007), 128106. https://doi.org/10.1103/PhysRevLett.98.128106 doi: 10.1103/PhysRevLett.98.128106
![]() |
[8] |
M. Lodi, A. L. Shilnikov, M. Storace, Design principles for central pattern generators with preset rhythms, IEEE Trans. Neural Networks Learn. Syst., 31 (2019), 3658–3669. https://doi.org/10.1109/TNNLS.2019.2945637 doi: 10.1109/TNNLS.2019.2945637
![]() |
[9] |
J. T. C. Schwabedal, A. B. Neiman, A. L. Shilnikov, Robust design of polyrhythmic neural circuits, Phys. Rev. E, 90 (2014), 022715. https://doi.org/10.1103/PhysRevE.90.022715 doi: 10.1103/PhysRevE.90.022715
![]() |
[10] |
J. Collens, K. Pusuluri, A. Kelley, D. Knapper, T. Xing, S. Basodi, et al., Dynamics and bifurcations in multistable 3-cell neural networks, Chaos, 30 (2020), 072101. https://doi.org/10.1063/5.0011374 doi: 10.1063/5.0011374
![]() |
[11] |
Q. Lu, J. Tian, Synchronization and stochastic resonance of the small-world neural network based on the CPG, Cognit. Neurodyn., 8 (2014), 217–226. https://doi.org/10.1007/s11571-013-9275-8 doi: 10.1007/s11571-013-9275-8
![]() |
[12] |
Y. Zang, S. Hong, S. E. De, Firing rate-dependent phase responses of Purkinje cells support transient oscillations, eLife, 9 (2020), e60692. https://doi.org/10.7554/eLife.60692 doi: 10.7554/eLife.60692
![]() |
[13] |
B. S. Gutkin, G. B. Ermentrout, A. D. Reyes, Phase-response curves give the responses of neurons to transient inputs, J. Neurophysiol., 94 (2005), 1623–1635. https://doi.org/10.1152/jn.00359.2004 doi: 10.1152/jn.00359.2004
![]() |
[14] |
Y. Zang, E. Marder, Neuronal morphology enhances robustness to perturbations of channel densities, PNAS, 120 (2023), e2219049120. https://doi.org/10.1073/pnas.2219049120 doi: 10.1073/pnas.2219049120
![]() |
[15] | E. M. Izhikevich, Neural excitability, spiking, and bursting, Int. J. Bifurcation Chaos, 10 (2000), 1171–1266. https://doi.org/10.1142/S0218127400000840 |
[16] |
B. Lu, X. Jiang, Reduced and bifurcation analysis of intrinsically bursting neuron model, Electron. Res. Arch., 31 (2023), 5928–5945. https://doi.org/10.3934/era.2023301 doi: 10.3934/era.2023301
![]() |
[17] |
F. Zhan, S. Liu, X. Zhang, J. Wang, B. Lu, Mixed-mode oscillations and bifurcation analysis in a pituitary model, Nonlinear Dyn., 94 (2018), 807–826. https://doi.org/10.1007/s11071-018-4395-7 doi: 10.1007/s11071-018-4395-7
![]() |
[18] |
H. Zhou, B. Lu, H. Gu, X. Wang, Y. Liu, Complex nonlinear dynamics of bursting of thalamic neurons related to Parkinson's disease, Electron. Res. Arch., 32 (2024), 109–133. https://doi.org/10.3934/era.2024006 doi: 10.3934/era.2024006
![]() |
[19] |
Z. Song, J. Xu, Codimension-two bursting analysis in the delayed neural system with external stimulations, Nonlinear Dyn., 67 (2012), 309–328. https://doi.org/10.1007/s11071-011-9979-4 doi: 10.1007/s11071-011-9979-4
![]() |
[20] |
W. B. Kristan, Neuronal decision-making circuits, Curr. Biol., 18 (2008), R928–R932. https://doi.org/10.1016/j.cub.2008.07.081 doi: 10.1016/j.cub.2008.07.081
![]() |
[21] |
K. L. Briggman, W. B. Kristan, Multifunctional pattern-generating circuits, Annu. Rev. Neurosci., 31 (2008), 271–294. https://doi.org/10.1146/annurev.neuro.31.060407.125552 doi: 10.1146/annurev.neuro.31.060407.125552
![]() |
[22] |
J. Wojcik, J. Schwabedal, R. Clewley, A. L. Shilnikov, Key bifurcations of bursting polyrhythms in 3-cell central pattern generators, PLoS One, 9 (2014), e92918. https://doi.org/10.1371/journal.pone.0092918 doi: 10.1371/journal.pone.0092918
![]() |
[23] |
C. A. Tassinari, G. Cantalupo, B. Hoegl, P. Cortelli, L. Tassi, S. Francione, et al., Neuroethological approach to frontolimbic epileptic seizures and parasomnias: the same central pattern generators for the same behaviours, Rev. Neurol., 165 (2009), 762–768. https://doi.org/10.1016/j.neurol.2009.08.002 doi: 10.1016/j.neurol.2009.08.002
![]() |
[24] |
C. A. Tassinari, E. Gardella, G. Cantalupo, G. Rubboli, Relationship of central pattern generators with parasomnias and sleep-related epileptic seizures, Sleep Med. Clin., 7 (2012), 125–134. https://doi.org/10.1016/j.jsmc.2012.01.003 doi: 10.1016/j.jsmc.2012.01.003
![]() |
[25] |
F. Zhan, J. Song, S. Liu, The influence of synaptic strength and noise on the robustness of central pattern generator, Electron. Res. Arch., 32 (2024), 686–706. https://doi.org/10.3934/era.2024033 doi: 10.3934/era.2024033
![]() |
[26] |
V. Baruzzi, M. Lodi, M. Storace, A. Shilnikov, Towards more biologically plausible central-pattern-generator models, Phys. Rev. E, 104 (2021), 064405. https://doi.org/10.1103/PhysRevE.104.064405 doi: 10.1103/PhysRevE.104.064405
![]() |
[27] | R. L. Calabrese, Half-center oscillators underlying rhythmic movements, in The Handbook of Brain Theory and Neural Networks, (1998), 444–447. |
[28] |
A. Sakurai, P. S. Katz, The central pattern generator underlying swimming in Dendronotus iris: a simple half-center network oscillator with a twist, J. Neurophysiol., 116 (2016), 1728–1742. https://doi.org/10.1152/jn.00150.2016 doi: 10.1152/jn.00150.2016
![]() |
[29] |
A. Doloc-Mihu, R. L. Calabrese, A database of computational models of a half-center oscillator for analyzing how neuronal parameters influence network activity, J. Biol. Phys., 37 (2011), 263–283. https://doi.org/10.1007/s10867-011-9215-y doi: 10.1007/s10867-011-9215-y
![]() |
[30] | A. Doloc-Mihu, R. L. Calabrese, Analysis of family structures reveals robustness or sensitivity of bursting activity to parameter variations in a half-center oscillator (HCO) model, eNeuro, 3 (2016). https://doi.org/10.1523/ENEURO.0015-16.2016 |
[31] |
Z. Song, J. Xu, Multiple switching and bifurcations of in-phase and anti-phase periodic orbits to chaotic coexistence in a delayed half-center CPG oscillator, Nonlinear Dyn., 111 (2023), 16569–16584. https://doi.org/10.1007/s11071-023-08670-w doi: 10.1007/s11071-023-08670-w
![]() |
[32] |
Z. Song, J. Xu, Multi-coexistence of routes to chaos in a delayed half-center oscillator (DHCO) system, Nonlinear Dyn., 112 (2024), 1469–1486. https://doi.org/10.1007/s11071-023-09089-z doi: 10.1007/s11071-023-09089-z
![]() |
[33] |
A. J. White, Sensory feedback expands dynamic complexity and aids in robustness against noise, Biol. Cybern., 116 (2022), 267–269. https://doi.org/10.1007/s00422-021-00917-2 doi: 10.1007/s00422-021-00917-2
![]() |
[34] |
F. Zhan, S. Liu, Response of electrical activity in an improved neuron model under electromagnetic radiation and noise, Front. Comput. Neurosci., 11 (2017), 107. https://doi.org/10.3389/fncom.2017.00107 doi: 10.3389/fncom.2017.00107
![]() |
[35] | Z. Wang, Y. Yang, L. Duan, Control effects of electromagnetic induction on epileptic seizures, Nonlinear Dyn., 112 (2024). https://doi.org/10.1007/s11071-024-09373-6 |
[36] |
A. S. Lele, Y. Fang, J. Ting, A. Raychowdhury, Learning to walk: bio-mimetic hexapod locomotion via reinforcement-based spiking central pattern generation, IEEE J. Emerging Sel. Top. Circuits Syst., 10 (2020), 536–545. https://doi.org/10.1109/JETCAS.2020.3033135 doi: 10.1109/JETCAS.2020.3033135
![]() |
[37] |
T. Sun, Z. Dai, P. Manoonpong, Distributed-force-feedback-based reflex with online learning for adaptive quadruped motor control, Neural Networks, 142 (2021), 410–427. https://doi.org/10.1016/j.neunet.2021.06.001 doi: 10.1016/j.neunet.2021.06.001
![]() |
[38] |
B. Muthuswamy, Implementing memristor based chaotic circuits, Int. J. Bifurcation Chaos, 20 (2010), 1335–1350. https://doi.org/10.1142/S0218127410026514 doi: 10.1142/S0218127410026514
![]() |
[39] |
M. Lv, C. Wang, G. Ren, J. Ma, X. Song, Model of electrical activity in a neuron under magnetic flow effect, Nonlinear Dyn., 85 (2016), 1479–1490. https://doi.org/10.1007/s11071-016-2773-6 doi: 10.1007/s11071-016-2773-6
![]() |
[40] |
F. Zhan, S. Liu, J. Wang, B. Lu, Bursting patterns and mixed-mode oscillations in reduced Purkinje model, Int. J. Mod. Phys. B, 32 (2018), 1850043. https://doi.org/10.1142/S0217979218500431 doi: 10.1142/S0217979218500431
![]() |
[41] |
D. Terman, J. E. Rubin, A. C. Yew, C. J. Wilson, Activity patterns in a model for the subthalamopallidal network of the basal ganglia, J. Neurosci., 22 (2002), 2963–2976. https://doi.org/10.1523/JNEUROSCI.22-07-02963.2002 doi: 10.1523/JNEUROSCI.22-07-02963.2002
![]() |
[42] |
F. Su, J. Wang, S. Niu, H. Li, B. Deng, C. Liu, et al., Nonlinear predictive control for adaptive adjustments of deep brain stimulation parameters in basal ganglia–thalamic network, Neural Networks, 98 (2018), 283–295. https://doi.org/10.1016/j.neunet.2017.12.001 doi: 10.1016/j.neunet.2017.12.001
![]() |
[43] |
J. Song, S. Liu, H. Lin, Model-based quantitative optimization of deep brain stimulation and prediction of Parkinson's states, Neuroscience, 498 (2022), 105–124. https://doi.org/10.1016/j.neuroscience.2022.05.019 doi: 10.1016/j.neuroscience.2022.05.019
![]() |
[44] |
J. Song, H. Lin, S. Liu, Basal ganglia network dynamics and function: role of direct, indirect and hyper-direct pathways in action selection, Network: Comput. Neural Syst., 34 (2023), 84–121. https://doi.org/10.1080/0954898X.2023.2173816 doi: 10.1080/0954898X.2023.2173816
![]() |
[45] |
Z. Song, F. Ji, J. Xu, Is there a user-friendly building unit to replicate rhythmic patterns of CPG systems? Synchrony transition and application of the delayed bursting-HCO model, Chaos, Solitons Fractals, 182 (2024), 114820. https://doi.org/10.1016/j.chaos.2024.114820 doi: 10.1016/j.chaos.2024.114820
![]() |