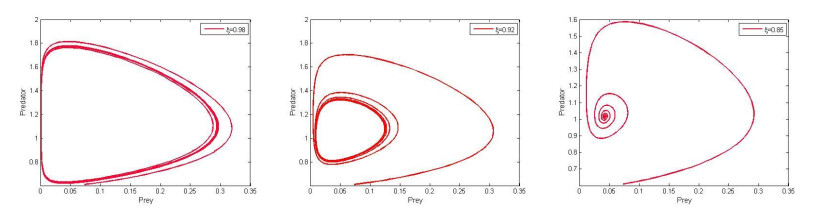
The main objective of our research was to explore and develop a fractional-order derivative within the predator-prey framework. The framework includes prey refuge and selective nonlinear harvesting, where the harvesting progressively approaches a threshold value as the density of the harvested population advances. For memory effect, a non-integer order derivative is better than an integer-order derivative. The solutions to the fractional framework were shown to be existence, uniqueness, non-negativity, and boundedness. Matignon's condition was used for analysing local stability, and a suitable Lyapunov function provided global stability. While discussing the Hopf bifurcation's existence condition, we explored derivative order and refuge as bifurcation parameters. We aimed at redefining the predator-prey framework to incorporate fractional order, refuge, and harvesting. This kind of nonlinear harvesting is more realistic and reasonable than the model with constant yield harvesting and constant effort harvesting. The Adams-Bashforth-Moulton PECE algorithm in MATLAB software was used to simulate the proposed outcomes, investigate the impact on various factors, and analyse harvesting's effect on non-integer order predator-prey interactions.
Citation: Kottakkaran Sooppy Nisar, G Ranjith Kumar, K Ramesh. The study on the complex nature of a predator-prey model with fractional-order derivatives incorporating refuge and nonlinear prey harvesting[J]. AIMS Mathematics, 2024, 9(5): 13492-13507. doi: 10.3934/math.2024657
[1] | Jie Liu, Qinglong Wang, Xuyang Cao, Ting Yu . Bifurcation and optimal harvesting analysis of a discrete-time predator–prey model with fear and prey refuge effects. AIMS Mathematics, 2024, 9(10): 26283-26306. doi: 10.3934/math.20241281 |
[2] | Heping Jiang . Complex dynamics induced by harvesting rate and delay in a diffusive Leslie-Gower predator-prey model. AIMS Mathematics, 2023, 8(9): 20718-20730. doi: 10.3934/math.20231056 |
[3] | Binfeng Xie, Na Zhang . Influence of fear effect on a Holling type III prey-predator system with the prey refuge. AIMS Mathematics, 2022, 7(2): 1811-1830. doi: 10.3934/math.2022104 |
[4] | San-Xing Wu, Xin-You Meng . Dynamics of a delayed predator-prey system with fear effect, herd behavior and disease in the susceptible prey. AIMS Mathematics, 2021, 6(4): 3654-3685. doi: 10.3934/math.2021218 |
[5] | A. Q. Khan, Ibraheem M. Alsulami . Complicate dynamical analysis of a discrete predator-prey model with a prey refuge. AIMS Mathematics, 2023, 8(7): 15035-15057. doi: 10.3934/math.2023768 |
[6] | Na Min, Hongyang Zhang, Xiaobin Gao, Pengyu Zeng . Impacts of hunting cooperation and prey harvesting in a Leslie-Gower prey-predator system with strong Allee effect. AIMS Mathematics, 2024, 9(12): 34618-34646. doi: 10.3934/math.20241649 |
[7] | Rongjie Yu, Hengguo Yu, Min Zhao . Steady states and spatiotemporal dynamics of a diffusive predator-prey system with predator harvesting. AIMS Mathematics, 2024, 9(9): 24058-24088. doi: 10.3934/math.20241170 |
[8] | Xin-You Meng, Fan-Li Meng . Bifurcation analysis of a special delayed predator-prey model with herd behavior and prey harvesting. AIMS Mathematics, 2021, 6(6): 5695-5719. doi: 10.3934/math.2021336 |
[9] | Xiao-Long Gao, Hao-Lu Zhang, Xiao-Yu Li . Research on pattern dynamics of a class of predator-prey model with interval biological coefficients for capture. AIMS Mathematics, 2024, 9(7): 18506-18527. doi: 10.3934/math.2024901 |
[10] | Chengchong Lu, Xinxin Liu, Zhicheng Li . The dynamics and harvesting strategies of a predator-prey system with Allee effect on prey. AIMS Mathematics, 2023, 8(12): 28897-28925. doi: 10.3934/math.20231481 |
The main objective of our research was to explore and develop a fractional-order derivative within the predator-prey framework. The framework includes prey refuge and selective nonlinear harvesting, where the harvesting progressively approaches a threshold value as the density of the harvested population advances. For memory effect, a non-integer order derivative is better than an integer-order derivative. The solutions to the fractional framework were shown to be existence, uniqueness, non-negativity, and boundedness. Matignon's condition was used for analysing local stability, and a suitable Lyapunov function provided global stability. While discussing the Hopf bifurcation's existence condition, we explored derivative order and refuge as bifurcation parameters. We aimed at redefining the predator-prey framework to incorporate fractional order, refuge, and harvesting. This kind of nonlinear harvesting is more realistic and reasonable than the model with constant yield harvesting and constant effort harvesting. The Adams-Bashforth-Moulton PECE algorithm in MATLAB software was used to simulate the proposed outcomes, investigate the impact on various factors, and analyse harvesting's effect on non-integer order predator-prey interactions.
The use of mathematics to model and comprehend biological processes has grown significantly in recent years. The prey-predator framework is an essential tool in mathematical biology that aids in interpreting the dynamics of population interactions in natural environments. In order to provide predictions, these mathematical models are built using data gathered from experiments and observations. The specific dynamics are crucial for comprehending the effects on the environment throughout the modelling phase. Mathematical systems, mostly representing biological events using differential equations, encapsulate their dynamics. Not only do they have extensive uses in mathematics but also fields as diverse as engineering, biology, physics, and meteorology. All of ecology's activities are essentially the scientific investigation and creative analysis of the complex interactions that exist between living things and their surroundings. It includes not only living things but also the communities they build and the inanimate objects in their surroundings, all of which are constantly interacting with one another. The relationship between predators and their prey has always had significance in both the disciplines of ecology and mathematical ecology due to its global relevance [1,2,3]. A key component of population dynamics is the relationship between these two entities. Numerous investigators have worked to enhance the fundamental predator-prey system by including various biological factors, such as the prey's fear effect [4], prey refuge [5], selective and mixed-form harvesting [6,7,8], and many more.
There has been a lot of mathematical model building around the effects of prey refuge in recent years [9,10,11,12]. Wang et al. [13] used both analytical and numerical techniques to investigate an impact on prey refuge as well as predator fear on anti-predator activity. Prey refuges generally manifest in one of two ways: either the number of refuges is directly related to the density of prey population, or a fixed maximal capacity is in place [14,15,16,17,18,19]. In our model, we focus on the first kind, where ε(0⩽ε<1) is the fixed maximum availability of refuge for prey. Another important aspect of predator-prey dynamics is the harvesting of either group. Fisheries, forests, and wildlife management are common places to find biological resources being harvested. In order to ensure that renewable resources are preserved for future generations, bio-economic models have been created to aid in the scientific management of these assets. The concept of predator-prey interactions is discussed in [20], where the predator incorporates a prey refuge and the prey engages in harvesting. In addition to discussing the impact of harvesting, Mukhopadhyay and Bhattacharyya [21] examined a scenario in which two predators were vying for only one prey. The predator-prey model with various harvesting scenarios is examined from an economic perspective in [22]. Nonlinear harvesting allows our predator-prey system to choose to harvest a single species while protecting the other one. In reality, we limit population growth by harvesting prey or predators. This formulates a predator-prey framework based on the Holling type Ⅱ dynamics, including the effects of nonlinear harvesting.
dx1dt=rx1(1−x1k)−bx1x2a+x1−hx1h+x1,dx2dt=b1x1x2a+x1−cx2. | (1) |
The above model is predicated on the following assumptions:
(ⅰ) Prey and predator population densities at a given instance of time t are denoted by the variables x1andx2, respectively.
(ⅱ) The inherent growth rate and environmental carrying capacity of prey are randk, respectively.
(ⅲ) The term bx1x2a+x1 denotes Holling type Ⅱ functional response that supports predator species progress, a is the half-saturation constant, b(>0) is the predator's maximal consumption rate, b1(>0) is the biomass transformation amount (0<b1<b), and c(>0) represents the predator's inherent level of mortality. Here, we can see that if b1<c, the right portion of the second equation of (1) never becomes positive. Therefore, we assume that b1−c>0 for model (1).
(ⅳ) The maximum prey harvesting rate is represented by the parameter h. With an increase in the harvesting population, the harvesting function hx1h+x1 reaches gradually the saturation limit value h. From a biological point of view, we think this harvesting role makes more sense.
The suggested model (1) was built using a couple of first-order derivative nonlinear differential equations, in which the instantaneous population density determines how the population density changes over time. However, in actuality, a phenomenon known as the memory effect occurs, wherein the history of all past situations also influences the present state [23]. A fractional differential system may depict phenomena or systems with memory and genetic properties [24]. Drawing on the work of L'Hôpital and Leibniz, who provided a rigorous evaluation of derivatives of order 1/2, Liouville [25] developed the notion of fractional-order derivative. Riemann reinterpreted Liouville's formulation as the Riemann-Liouville fractional derivative operator [26], which he achieved by directly generalising the Cauchy formula. Euler's investigation of non-integer integration particularly inspired him to develop the Gamma function to be a generalisation of the factorial notion for non-integer numbers, which is used to explain the non-integer order derivative idea proposed by Liouville and Riemann [27]. When solving differential equations, Michele Caputo changed the Riemann-Liouville operator in 1967 to eliminate the need for beginning conditions. The modified operator's definition has become known as the Caputo fractional-order derivative operator. This derivative takes into account the memory effects and long-range dependencies present in many real-world systems, allowing for a more accurate representation of their behaviour. The Caputo fractional derivative can handle both smooth and non-smooth functions, making it applicable to a wide range of systems and phenomena. In addition, it possesses desirable mathematical properties, including linearity, compatibility with initial conditions, and the ability to preserve the order of differentiability. Many studies have been conducted on predator-prey frameworks involving non-integer derivatives of the Caputo type [28,29,30,31,32,33,34,35]. So, using the Caputo non-integer order derivative operator, we apply several modifications and analyses within the predator-prey framework (1) that include selective nonlinear harvesting and refuge for prey.
The following is the outline of the article. Section 2 outlines the process of developing the model and its fundamental attributes. One of the fundamental characteristics is the ability to confirm that the model's solutions are non-negative, exist, unique, and bounded. Section 3 presents the outcomes of the dynamical analysis. The findings include the presence and consistency of equilibrium points. The Hopf bifurcation is the one that is studied, and both local and global stability are examined. Verification of analytical findings is accomplished in Section 4 using numerical simulations and interpretations. In Section 5, we summarise our findings.
The model is created using the Caputo non-integer order derivative operator to the left side of the framework (1), which incorporates selective nonlinear harvesting and refuge for the prey.
CDξ0x1(t)=rx1(1−x1k)−b(1−ε)x1x2a+(1−ε)x1−hx1h+x1,CDξ0x2(t)=b1(1−ε)x1x2a+(1−ε)x1−cx2, | (2) |
with ξ∈R, 0<ξ⩽1, and CDξ0 indicates the ξth order of Caputo non-integer derivative operator given by Dξtf(t)=1Γ(1−ξ)t∫0(t−ζ)−ξf(ζ)dζ. All of system (2)'s parameters are defined in terms of system (1)'s assumptions.
Theorem 1. [36] Let dξudtξ=Au;u(0)=u0 be the linear and non-integer order differential equation, where 0<ξ⩽1,x∈Rn and A is any arbitrary matrix of size n. In addition, there are:
(ⅰ) For u=0 to be asymptotically resilient, it is necessary and sufficient that every latent value λi of A fulfil |arg(λi)|>ξπ2,
(ⅱ) for u=0 to be a stable solution, each latent value λi of A must satisfy |arg(λi)|⩾ξπ2, and all latent values that fulfil |arg(λi)|=ξπ2 must have the equal geometric and algebraic multiplicity.
Theorem 2. [37] Let dξudtξ=f(u);u(0)=u0, be the fractional order differential equation with 0<ξ⩽1,x∈Rn. The aforementioned system's equilibrium points may be found by solving f(u)=0. If the equilibrium points meets the criteria |arg(λi)|>ξπ2, then the system is locally asymptotically stable for each latent value λi of the community matrix J=∂f∂u.
Lemma 1. [38] Assume that ξ is a member of (0,1]. Let us take a function f(t) that is a member of C[0,a] such that Dξtf(t)∈C[0,a]. If Dξtf(t)⩾0, for every t∈(0,a), then it is non-decreasing function for all t∈[0,a]. If Dξtf(t)⩽0, then it is non-increasing function for all t∈[0,a].
Theorem 3. The framework (2) has a unique solution to every non-negative starting condition.
Proof. Establishing this theorem requires finding a unique solution to the dynamical framework within the domain [0,∞)×χ, where χ={(x1,x2)∈R2:max{|x1|,|x2|}⩽T}.
Let us take V=(x1,x2),ˉV=(ˉx1,ˉx2) and now consider a mapping H(V)=(H1(V),H2(V)), where
H1(V)=rx1(1−x1k)−b(1−ε)x1x2a+(1−ε)x1−hx1h+x1,H2(V)=b1(1−ε)x1x2a+(1−ε)x1−cx2. | (3) |
Then, for any V,ˉV∈χ we have
‖H(V)−H(ˉV)‖=|H1(V)−H1(ˉV)|+|H2(V)−H2(ˉV)|,⩽(r+2rTk+h2(h+T)2+a(1−ε)T(a+(1−ε)T)2(b+b1))|x1−ˉx1|+(c+(1−ε)Ta+(1−ε)T(b+b1))|x2−ˉx2|,≤L‖V−¯V‖, | (4) |
where L=max{(r+2rTk+h2(h+T)2+a(1−ε)T(a+(1−ε)T)2(b+b1)),(c+(1−ε)Ta+(1−ε)T(b+b1))}.
Consequently, the Lipschitz criteria is satisfied by the mapping H(V). Therefore, it may be concluded that, given the starting condition V0=(x10,x20), where x10⩾0 and x20⩾0, the existence and uniqueness theorem of [39] states that there is a single solution to the system of differential equations (2).
Theorem 4. There exist non-negative and uniformly bounded solutions to a pair of differential equations (2) that begin in the area R2+.
Proof. We begin by determining if the solution, which originates in the domain R2+, is non-negativity. Hence, it is necessary to establish that, for any t⩾0, x1(t)⩾0, and x2(t)⩾0. Assume that not each t⩾0 achieve the criteria of x1(t)⩾0. Then, there exist t1>0 such that x1(t)>0 for t∈[0,t1),x1(t1)=0 and x1(t+1)<0. Subsequently, we may deduce from the initial equation in (2) that Dξtx1(t)|t=t1=0. We find that x1(t)⩾0 for every t⩾0 because Lemma 1 suggests that x1(t+1)=0, which goes against the assumption x1(t+1)<0. It is simple to establish that the remaining equation of (2) has a non-negative solution that begins within the domain of R2+ using a similar procedure. Thus, for any t⩾0, it provides x2(t)⩾0 in the same approach. Following that, we have to establish the uniform boundedness of the (2) solutions, which begin in the domain R2+.
Considering S(t)=x1(t)+bb1x2(t), we can use Eq (2) to get
DξtS(t)+cS(t)=Dξtx1(t)+bb1Dξtx2(t)+c(x1(t)+bb1x2(t)),⩽−rk(x1−k(r+c)2r)2+k(r+c)24r,DξtS(t)+cS(t)⩽k(r+c)24r. | (5) |
It is deduced from Lemma 3 of [40] that
S(t)⩽(S(0)−k(r+c)24r)Eξ(−(r+c)tξ)+k(r+c)24r, |
where Eξ represents the Mittag-Leffler function. By applying Lemma 5 and Corollary 6 from [41], we obtain S(t)⩽k(r+c)24r,t→∞. Consequently, any solution to (2) that begins in R2+ is confined to the region Ω={(x1,x2)∈R2+:S⩽k(r+c)24r+δ,δ>0}.
The steady state condition is solved at the equilibrium points of the framework (2), which are found by making Dξ0x1(t)=0 and Dξ0x2(t)=0. We can obtain the following equilibrium points:
(ⅰ) The extinction equilibrium E0=(0,0),
(ⅱ) the auxiliary equilibrium point E1=(x1,0), where rx21+r(h−k)x1+kh(1−r)=0, x1=r(k−h)+√r2(h+k)2−4rkh2r and x1 is positive for k>h, r(h+k)24kh>1,
(ⅲ) the interior equilibrium point E2=(x∗1,x∗2), where x∗1=ac(b1−c)(1−ε) which is positive for ε<1 and x∗2=ab1[kh(r−1)(b1−c)2(1−ε)2+rac(k−h)(b1−c)(1−ε)−ra2c2]kb(b1−c)2(1−ε)2[h(b1−c)(1−ε)+ac] which is positive for r>1 and (k−h)(b1−c)(1−ε)ac>1.
The local stability of the equilibrium points of the framework (2) is shown by the findings of the community matrix's latent values and the application of the stability criteria theorem in Petras [37]. Assuming that E∗ is an equilibrium point of the framework (2). According to the Matignon stability criteria theorem, E∗ is local asymptotically stable provided each of the latent values λi of a community matrix,
J(E∗)=(r−2rx1k−b(1−ε)(a+x1)x2(a+(1−ε)x1)2−h2(h+x1)2−b(1−ε)x1a+(1−ε)x1b1(1−ε)(a+x1)x2(a+(1−ε)x1)2b(1−ε)x1a+(1−ε)x1−c), | (6) |
that satisfies |arg(λi)|>ξπ2.
Theorem 5. The origin point E0(0,0) is locally asymptotically stable if r<1 and saddle point if r>1.
Proof. The Jacobian matrix for E0=(0,0) is
J(E0)=(r−100−c). | (7) |
The latent values of J(E0) are obtained as λ1=r−1 and λ2=−c<0. When r<1, then the eigenvalue λ1 is not positive. Consequently, we get |arg(λ1)|=|arg(λ2)|=π, indicating that the criterion |arg(λi)|>ξπ2, i=1,2 has been achieved. Thus, E0 is asymptotically stable. If r>1, then one latent value is positive and another is negative, with |arg(λ1)|=0 and |arg(λ2)|=π. Thus, we have the situation where one latent value meets the requirement |arg(λi)|>ξπ2, another meets the requirement |arg(λi)|<ξπ2. E0 is the saddle point as a result.
Remark 1. According to the aforementioned study, the extinction equilibrium E0 remains unstable h=0 (the system has no harvesting).
Theorem 6. The equilibrium point E1=(x∗1,0) is locally asymptotically stable if √hk/r−h<x∗1<ac(1−ε)(b1−c).
Proof. The community matrix for E1 is
J(E1)=(r−2rx1k−h2(h+x1)2−b(1−ε)x1a+(1−ε)x10b1(1−ε)x1a+(1−ε)x1−c). | (8) |
The latent values of J(E1) are λ1=−[rx1k−hx1(h+x1)2], which is negative if x1>(√hk/r−h) and λ2=b1(1−ε)x1a+(1−ε)x1−c, which is negative if x1<ac(1−ε)(b1−c). Consequently, we get |arg(λ1)|=|arg(λ2)|=π, indicating that the criterion |arg(λi)|>ξπ2, i=1,2 has been achieved. Thus, E1 is asymptotically stable if √hk/r−h<x∗1<ac(1−ε)(b1−c).
Theorem 7. The positive equilibrium point E∗=(x∗1,x∗2) is locally asymptotically stable if any of the conditions listed below are fulfilled:
ⅰ) trace(J(E∗))⩽0,
ⅱ) trace(J(E∗))>0,trace2(J(E∗))−4det(J(E∗))<0 and
sqrt(|trace2(J(E∗))−4det(J(E∗))|)>trace(J(E∗))tan(ξπ2).
Proof. The community matrix for E∗=(x∗1,x∗2) is given by
J(E∗)=(−rx1k+b(1−ε)2x1x2(a+(1−ε)x1)2+hx1(h+x1)2−b(1−ε)x1a+(1−ε)x1ab1(1−ε)x2(a+(1−ε)x1)20). | (9) |
Then, trace(J(E∗))=−rx1k+b(1−ε)2x1x2(a+(1−ε)x1)2+hx1(h+x1)2 and det(J(E∗))=abb1(1−ε)2x1x2(a+(1−ε)x1)3
(1) If trace(J(E∗))⩽0, then we have to consider three cases:
Case ⅰ) For trace(J(E∗))=0, we will get |arg(λi)|=π2,i=1,2 and E∗ is locally asymptotically stable.
Case ⅱ) For trace(J(E∗))<0 and trace2(J(E∗))−4det(J(E∗))⩾0, then both latent roots are negative and |arg(λi)|=π,i=1,2. Thus, E∗ is locally asymptotically stable.
Case ⅲ) For trace(J(E∗))<0 and trace2(J(E∗))−4det(J(E∗))<0, then both latent roots are complex conjugates with non-positive real part and |arg(λi)|>ξπ2,i=1,2. Therefore, E∗ is locally asymptotically stable for any ξ.
(2) If trace(J(E∗))>0, trace2(J(E∗))−4det(J(E∗))<0 and sqrt(|trace2(J(E∗))−4det(J(E∗))|)>trace(J(E∗))tan(ξπ2), then we obtain Img(λ1)=−Img(λ2)=4det(J(E∗))−trace2(J(E∗)) and Re(λi)=trace(J(E∗))>0,i=1,2. From these we can conclude that |Img(λi)|>tan(ξπ2)(Re(λi)),i=1,2. Consequently, E∗ is locally asymptotically stable if |arg(λi)|>ξπ2,i=1,2.
We next proceed to examine E∗'s global stability. To achieve this objective, Lemma 3.1 of [42] and the generalised LaSalle's invariance principle by Huo et al. [43] are employed.
Now, consider a function V(x1,x2)=(x1−x∗1−x∗1logx1x∗1)+a1(x2−x∗2−x∗2logx2x∗2), where a1>0 is a constant. Lemma 3.1 of above reference and the ξ-order non-integer derivative of V(x1,x2) along the solution of (2) give us
dξVdtξ⩽(x1−x∗1x1)dξx1dtξ+a1(x2−x∗2x2)dξx2dtξ, |
=(x1−x∗1x1)(rx1(1−x1k)−b(1−ε)x1x2a+(1−ε)x1−hx1h+x1)+a1(x2−x∗2x2)(b1(1−ε)x1x2a+(1−ε)x1−cx2), |
=−rk(x1−x∗1)2−b(1−ε)(x1−x∗1)(a(x2−x∗2)+(1−ε)(x∗1x2−x1x∗2)(a+(1−ε)x∗1)(a+(1−ε)x1))+a1b1(1−ε)(x2−x∗2)(a(x1−x∗1)(a+(1−ε)x∗1)(a+(1−ε)x1)), |
=−[rk−b(1−ε)2x∗2(a+(1−ε)x∗1)(a+(1−ε)x1)](x1−x∗1)2+(1−ε)[aa1b1−(ab+b(1−ε)x∗1)(a+(1−ε)x∗1)(a+(1−ε)x1)](x1−x∗1)(x2−x∗2), |
the following can be derived by assuming aa1b1=(ab+b(1−ε)x∗1),
dξVdtξ=−[rk−b(1−ε)2x∗2(a+(1−ε)x∗1)(a+(1−ε)x1)](x1−x∗1)2,dξVdtξ⩽0. |
Therefore, the positive equilibrium point E∗ is globally asymptotically stable.
The transition from stable spiral to unstable behaviour at the equilibrium point causes Hopf bifurcation to occur when the bifurcation parameter reaches the critical value. It is obvious from Lemma 1 that the order of the derivative ξ determines the stability of a dynamical system. Therefore, ξ may be considered a bifurcation parameter, even if the non-integer order system does not possess the identical Hopf bifurcation condition as the integer-order framework.
Let us examine a system with fractional order
Dξtx(t)=f(m,x), | (10) |
where ξ∈[0,1),x∈ℜ2 and let E∗ is an equilibrium point. Based on the analysis of a non-integer order Hopf bifurcation in [44,45], it can be concluded that, under certain circumstances, system (10) experiences a Hopf bifurcation given that the value m passes the threshold m∗ around the equilibria E∗.
ⅰ) The characteristic equation has two complex conjugate eigenvalues
λ1,2(m)=ν(m)±iπ(m), |
ⅱ) ν1,2(ξ,m∗)=0,whereν1,2=ξπ2−|arg(λi(m))|,
ⅲ) ∂ν1,2∂m|m=m∗≠0.
Theoretical results have been validated through numerical simulations employing the predictor-corrector technique on non-integer differential equations. In order to resolve fractional order nonlinear differential equations, numerical methods are employed. This research explores the impact of altering several factors on the non-integer order predator-prey dynamical framework (2).
Figure 1 illustrates the phase portraits of the framework (2) about E2 for parameter values as r=2.5,k=0.899,β=0.945,ε=0.058,α=0.559,h=0.291, d=0.059,β1=0.895 and three different values of ξ=0.98,0.92,and0.85 in order to study the influence of the derivative order (ξ) on the predator-prey dynamics. Based on these numbers, we know that system (2) goes from unstable to stable and that at ξ=0.92, it shows evidence of Hopf bifurcation. Hence, we deduce that the dynamics of the system under consideration are significantly affected by the non-integer order derivative.
The influence of prey refuge rate ε has been visually explored for ξ=1,0.92, with the values of ε=0.08,0.6, and 0.8, as well as the set of parameter values r=2.5,k=0.899, β=0.945,ε=0.058,h=0.291,d=0.059,β1=0.895 shown in Figures 2 and 3. The equilibrium point E2, as seen in Figure 2, is not stable at ξ=1 and ε=0.08 but, it should be emphasised, becomes stable at ξ=0.92 and ε=0.08. This leads us to the conclusion that the existence of prey refuge affects the prey-predator system, and fractional order ξ contributes to species persistence by stabilising the system.
Considering the identical selection of parameter values as illustrated in Figure 1, the influence of prey harvesting rate h has been visually explored for ξ=0.98 and 1. Figures 4 and 5 illustrate that, when ξ=0.98, the system (2) is stable at h=0.5 then not stable for h=0.1. In connection with the parameter h, bifurcation diagrams are provided in Figure 6.
In the present study, we explored a fractional-order Holling type Ⅱ predator-prey framework that includes selective nonlinear harvesting and refuge in prey. As soon as the density of the harvested population reaches a certain limit, the harvesting grows smoothly to that value. The basic objective of this research is to understand how fractional order, refuge, and nonlinear harvesting affect the model's dynamic behaviour. The existence, uniqueness, non-negativity, and boundedness of the system's solution were defined first (2). Next, the local resilience of each feasible equilibrium point in the predator-prey framework was investigated using Matignon's criterion. Through the subsequent development of a suitable Lyapunov function, a global stability analysis of system (2) has been explored. Next, using ξ and h as parameters, the presence of Hopf bifurcation was investigated. Furthermore, the theoretical conclusions were numerically confirmed by employing the Adams-Bashforth PECE method (time-domain).
The system is stabilised by the prey refuge rate, according to numerical simulations. The framework exhibits stability in both integer-order as well as fractional orders when the refuge rates of prey populations are reduced. When the rate of prey refuge increases at a certain level of predation and harvesting, the system becomes unstable. Furthermore, it has been shown that nonlinear harvesting plays a vital part in maintaining system stability. The system shows stable behaviour at larger values of prey harvesting, whereas fractional order ξ=0.98 shows stability at lower prey population harvesting. Therefore, given a constant value of the prey refuge amount, the system shows stable behaviour when there is a significant quantity of prey harvesting. Analysing the influence of spatial organisation on the overall behaviour of the system in the presence of a nonlinear harvesting term might provide valuable insights. Although this model can include spatial structure inpattern-forming Turing dynamics, it may be challenging to conduct comparable analyses and simulations for nonlinear harvesting techniques in this particular scenario. Therefore, more investigation into this matter is necessary. Also, the incorporation of prey-refuging strategy switching based on the prey-to-predator density ratio in the proposed model offers a more realistic ecological representation. These results underscore the importance of threshold-based refuge seeking for preserving species and maintaining ecosystem stability.
The authors declare they have not used Artificial Intelligence (AI) tools in the creation of this article.
The authors extend their appreciation to Prince Sattam bin Abdulaziz University for funding this research work through the project number (PSAU/2024/01/78916).
The authors state that none of the work presented in this study may have been influenced by any known conflicting financial interests or personal relationships.
[1] |
P. A. Abrams, Implications of dynamically variable traits for identifying, classifying, and measuring direct and indirect effects in ecological communities, Am. Nat., 146 (1995), 112–134. https://doi.org/10.1086/285789 doi: 10.1086/285789
![]() |
[2] | E. L. Preisser, D. I. Bolnick, M. F. Benard, Scared to death? The effects of intimidation and consumption in predator-prey interactions, Ecology, 86 (2005), 501–509. |
[3] |
S. L. Lima, Nonlethal effects in the ecology of predator-prey interactions, Bioscience, 48 (1998), 25–34. https://doi.org/10.2307/1313225 doi: 10.2307/1313225
![]() |
[4] |
X. Wang, L. Zanette, X. Zou, Modelling the fear effect in predator-prey interactions, J. Math. Biol., 73 (2016), 1179–1204. https://doi.org/10.1007/s00285-016-0989-1 doi: 10.1007/s00285-016-0989-1
![]() |
[5] |
H. Zhang, Y. Cai, S. Fu, W. Wang, Impact of the fear effect in a prey-predator model incorporating a prey refuge, Appl. Math. Comput., 356 (2019), 328–337. https://doi.org/10.1016/j.amc.2019.03.034 doi: 10.1016/j.amc.2019.03.034
![]() |
[6] |
S. Chakraborty, S. Pal, N. Bairagi, Predator-prey interaction with harvesting: Mathematical study with biological ramifications, Appl. Math. Model., 36 (2012), 4044–4059. https://doi.org/10.1016/j.apm.2011.11.029 doi: 10.1016/j.apm.2011.11.029
![]() |
[7] |
K. S. Chaudhuri, S. S. Ray, On the combined harvesting of a prey-predator system, J. Biol. Syst., 4 (1996), 373–389. https://doi.org/10.1142/S0218339096000 doi: 10.1142/S0218339096000
![]() |
[8] |
D. Xiao, W. Li, M. Han, Dynamics in a ratio-dependent predator-prey model with predator harvesting, J. Math. Anal. Appl., 324 (2006), 14–29. https://doi.org/10.1016/j.jmaa.2005.11.048 doi: 10.1016/j.jmaa.2005.11.048
![]() |
[9] |
Z. Bi, S. Liu, M. Ouyang, X. Wu, Pattern dynamics analysis of spatial fractional predator-prey system with fear factor and refuge, Nonlinear Dynam., 111 (2023), 10653–10676. https://doi.org/10.1007/s11071-023-08353-6 doi: 10.1007/s11071-023-08353-6
![]() |
[10] |
B. Mondal, S. Roy, U. Ghosh, P. K. Tiwari, A systematic study of autonomous and non autonomous predator-prey models for the combined effects of fear, refuge, cooperation and harvesting, Eur. Phys. J. Plus, 137 (2022), 724. https://doi.org/10.1140/epjp/s13360-022-02915-0 doi: 10.1140/epjp/s13360-022-02915-0
![]() |
[11] |
Z. Wei, F. Chen, Dynamics of a delayed predator-prey model with prey refuge, Allee effect and fear effect, Int. J. Bifurc. Chaos, 33 (2023), 2350036. https://doi.org/10.1142/S0218127423500360 doi: 10.1142/S0218127423500360
![]() |
[12] |
S. Khajanchi, S. Banerjee, Role of constant prey refuge on stage structure predator-prey model withratio dependent functional response, Appl. Math. Comput., 314 (2017), 193–198. https://doi.org/10.1016/j.amc.2017.07.017 doi: 10.1016/j.amc.2017.07.017
![]() |
[13] |
J. Wang, Y. Cai, S. Fu, W. Wang, The effect of the fear factor on the dynamics of a predator-prey model incorporating the prey refuge, Chaos Int. J. Nonlinear Sci., 29 (2019), 083109. https://doi.org/10.1063/1.5111121 doi: 10.1063/1.5111121
![]() |
[14] |
K. Sarkar, S. Khajanchi, An eco-epidemiological model with the impact of fear, Chaos Int. J. Nonlinear Sci., 32 (2022), 083126. https://doi.org/10.1063/5.0099584 doi: 10.1063/5.0099584
![]() |
[15] |
S. Biswas, B. Ahmad, S. Khajanchi, Exploring dynamical complexity of a cannibalistic eco-epidemiological model with multiple time delay, Math. Method. Appl. Sci., 46 (2023), 4184–4211. https://doi.org/10.1002/mma.8749 doi: 10.1002/mma.8749
![]() |
[16] |
K. Sarkar, S. Khajanchi, Spatiotemporal dynamics of a predator-prey system with fear effect, J. Frankl. I., 360 (2023), 7380–7414. https://doi.org/10.1016/j.jfranklin.2023.05.034 doi: 10.1016/j.jfranklin.2023.05.034
![]() |
[17] |
C. C. García, Bifurcations in a Leslie-Gower model with constant and proportional prey refuge at high and low density, Nonlinear Anal.-Real, 72 (2023), 103861. https://doi.org/10.1016/j.nonrwa.2023.103861 doi: 10.1016/j.nonrwa.2023.103861
![]() |
[18] |
C. C. García, Impact of prey refuge in a discontinuous Leslie-Gower model with harvesting and alternative food for predators and linear functional response, Math. Comput. Simulat., 206 (2023), 147–165. https://doi.org/10.1016/j.matcom.2022.11.013 doi: 10.1016/j.matcom.2022.11.013
![]() |
[19] |
C. Maji, Impact of fear effect in a fractional-order predator-prey system incorporating constant prey refuge, Nonlinear Dynam., 107 (2022), 1329–1342. https://doi.org/10.1007/s11071-021-07031-9 doi: 10.1007/s11071-021-07031-9
![]() |
[20] |
T. K. Kar, Modelling and analysis of a harvested prey-predator system incorporating a prey refuge, J. Comput. Appl. Math., 185 (2006), 19–33. https://doi.org/10.1016/j.cam.2005.01.035 doi: 10.1016/j.cam.2005.01.035
![]() |
[21] |
B. Mukhopadhyay, R. Bhattacharyya, Effects of harvesting and predator interference in a model of two-predators competing for a single prey, Appl. Math. Model., 40 (2016), 3264–3274. https://doi.org/10.1016/j.apm.2015.10.018 doi: 10.1016/j.apm.2015.10.018
![]() |
[22] |
J. Hoekstra, J. C. J. M. van den Bergh, Harvesting and conservation in a predator-prey system, J. Econ. Dyn. Control, 29 (2005), 1097–1120. https://doi.org/10.1016/j.jedc.2004.03.006 doi: 10.1016/j.jedc.2004.03.006
![]() |
[23] |
A. Suryanto, I. Darti, S. Anam, Stability analysis of a fractional order modified Leslie-Gower model with additive Allee effect, Int. J. Math. Math. Sci., 2017 (2017), 1–9. https://doi.org/10.1155/2017/8273430 doi: 10.1155/2017/8273430
![]() |
[24] |
Z. Li, L. Liu, S. Dehghan, Y. Chen, D. Xue, A review and evaluation of numerical tools for fractional calculus and fractional order controls, Int. J. Control, 90 (2017), 1165–1181. https://doi.org/10.1080/00207179.2015.1124290 doi: 10.1080/00207179.2015.1124290
![]() |
[25] | J. Liouville, Sur le calcul des differentielles a indices quelconques, 1832. Available from: https://books.google.com/books?id = 6jfBtwAACAAJ. |
[26] | S. G. Samko, A. A. Kilbas, O. I. Marichev, Fractional integrals and derivatives: Theory and applications, Switzerland/Philadelphia: Gordon and Breach Science Publishers, 1993. |
[27] |
G. A. Farid, Unified integral operator and further its consequences, Open. J. Math. Anal., 4 (2020), 1–7. https://doi:10.30538/psrp-oma2020.0047 doi: 10.30538/psrp-oma2020.0047
![]() |
[28] |
H. S. Panigoro, E. Rahmi, N. Achmad, S. L. Mahmud, The influence of additive Allee effect and periodic harvesting to the dynamics of Leslie-Gower predator-prey model, Jambura J. Math., 2 (2020), 87–96. https://doi.org/10.34312/jjom.v2i2.4566 doi: 10.34312/jjom.v2i2.4566
![]() |
[29] |
H. S. Panigoro, A. Suryanto, W. M. Kusumawinahyu, I. Darti, Dynamics of an eco-epidemic predator-prey model involving fractional derivatives with power-law and Mittag-Leffler kernel, Symmetry., 13 (2021), 785. https://doi.org/10.3390/sym13050785 doi: 10.3390/sym13050785
![]() |
[30] |
E. Rahmi, I. Darti, A. Suryanto, Trisilowati, A modified Leslie-Gower model incorporating Beddington-Deangelis functional response, double Allee effect and memory effect, Fractal Fract., 5 (2021), 84. https://doi.org/10.3390/fractalfract5030084 doi: 10.3390/fractalfract5030084
![]() |
[31] |
M. Alqhtani, K. M. Owolabi, K. M. Saad, Spatiotemporal (target) patterns in sub-diffusive predator-prey system with the Caputo operator, Chaos Soliton. Fract., 160 (2022), 112267. https://doi.org/10.1016/j.chaos.2022.112267 doi: 10.1016/j.chaos.2022.112267
![]() |
[32] |
A. Waleed, Y. A. Amer, E. S. M. Youssef, A. M. S. Mahdy, Mathematical analysis and simulations for a Caputo-Fabrizio fractional COVID-19 model, Partial Differ. Eq. Appl. Math., 8 (2023), 100558. https://doi.org/10.1016/j.padiff.2023.100558 doi: 10.1016/j.padiff.2023.100558
![]() |
[33] |
S. A. M Abdelmohsen, D. S. Mohamed, H. A. Alyousef, M. R. Gorji, A. M. S. Mahdy, Mathematical modeling for solving fractional model cancer bosom malignant growth, AIMS Biophys., 10 (2023), 263–280. https://doi:10.3934/biophy.2023018 doi: 10.3934/biophy.2023018
![]() |
[34] |
A. M. S. Mahdy, D. S. Mohamed, Approximate solution of Cauchy integral equations by using Lucas polynomials, Comput. Appl. Math., 41 (2022), 403. https://doi.org/10.1007/s40314-022-02116-6 doi: 10.1007/s40314-022-02116-6
![]() |
[35] |
A. G. Khaled, S. M. Mohamed, A. Hammad, A. M. S. Mahdy, Dynamical behaviors of nonlinear coronavirus (COVID-19) model with numerical studies, CMC-Comput. Mater. Con., 67 (2021), 675–686. https://doi.org/10.32604/cmc.2021.012200 doi: 10.32604/cmc.2021.012200
![]() |
[36] | D. Matignon, Stability results for fractional differential equations with applications to control processing, Comput. Eng. Syst. Appl., 2 (1996), 963–968. |
[37] | I. Petráš, Fractional-order nonlinear systems: Modeling, analysis and simulation, Berlin: Springer, 2011. https://doi.org/10.1007/978-3-642-18101-6 |
[38] |
Z. M. Odibat, N. T. Shawagfeh, Generalized Taylor's formula, Appl. Math. Comput., 186 (2007), 286–293. https://doi.org/10.1016/j.amc.2006.07.102 doi: 10.1016/j.amc.2006.07.102
![]() |
[39] |
Y. Li, Y. Chen, I. Podlubny, Stability of fractional-order nonlinear dynamic systems: Lyapunov direct method and generalized Mittag-Leffler stability, Comput. Math. Appl., 59 (2010), 1810–1821. https://doi.org/10.1016/j.camwa.2009.08.019 doi: 10.1016/j.camwa.2009.08.019
![]() |
[40] |
H. Li, L. Zhang, C. Hu, Y. Jiang, Z. Teng, Dynamical analysis of a fractional-order predator-prey model incorporating a prey refuge, J. Appl. Math. Comput., 54 (2017), 435–449. https://doi.org/10.1007/s12190-016-1017-8 doi: 10.1007/s12190-016-1017-8
![]() |
[41] |
S. K. Choi, B. Kang, N. Koo, Stability for Caputo fractional differential systems, Abstr. Appl. Anal., 2014 (2014), 1–6. https://doi.org/10.1155/2014/631419 doi: 10.1155/2014/631419
![]() |
[42] |
C. Vargas-De-Leon, Volterra-type Lyapunov functions for fractional-order epidemic systems, Commun. Nonlinear Sci., 24 (2015), 75–85. https://doi.org/10.1016/j.cnsns.2014.12.013 doi: 10.1016/j.cnsns.2014.12.013
![]() |
[43] |
J. Huo, H. Zhao, L. Zhu, The effect of vaccines on backward bifurcation in a fractional order HIV model, Nonlinear Anal.-Real, 26 (2015), 289–305. https://doi.org/10.1016/j.nonrwa.2015.05.014 doi: 10.1016/j.nonrwa.2015.05.014
![]() |
[44] |
M. S. Abdelouahab, N. Hamri, J. Wang, Hopf bifurcation and chaos in fractional-ordermodified hybrid optical system, Nonlinear Dynam., 69 (2012), 275–284. https://doi.org/10.1007/s11071-011-0263-4 doi: 10.1007/s11071-011-0263-4
![]() |
[45] |
X. Li, R. Wu, Hopf bifurcation analysis of a new commensurate fractional-order hyperchaotic system, Nonlinear Dynam., 78 (2014), 279–288. https://doi.org/10.1007/s11071-014-1439-5 doi: 10.1007/s11071-014-1439-5
![]() |
1. | Nursena Günhan Ay, Emrullah Yaşar, Analytical solutions and physical interpretation of a predator–prey system with Allee effect using fractional derivative operators, 2024, 11, 26668181, 100785, 10.1016/j.padiff.2024.100785 |