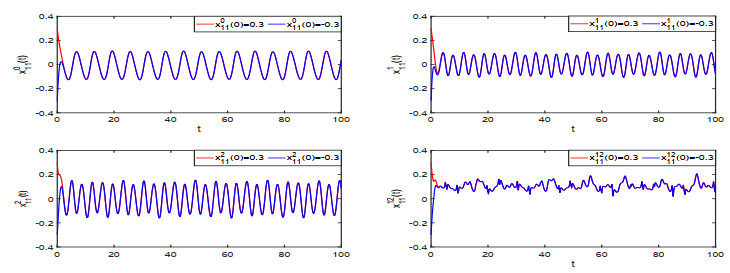
We investigate the synchronizability of multilayer star-ring networks. Two types of multilayer networks, including aggregated coupling and divergent coupling, are established based on the connections between the hub node and the leaf nodes in the subnetwork. The eigenvalue spectrum of the two types of multilayer networks is strictly derived, and the correlation between topological parameters and synchronizability is analyzed by the master stability function framework. Moreover, the variable coupling strength has been investigated, revealing that it is significantly related to the synchronizability of the aggregated coupling while having no influence on the divergent coupling. Furthermore, the validity of the synchronizability analysis is obtained by implementing adaptive control on the multilayer star-ring networks previously mentioned. Calculations and comparisons show that the differences caused by the sizes of multilayer networks and interlayer coupling strength are not negligible. Finally, numerical examples are also provided to validate the effectiveness of the theoretical analysis.
Citation: Shuang Liu, Bigang Xu, Qingyun Wang, Xia Tan. Synchronizability of multilayer star-ring networks with variable coupling strength[J]. Electronic Research Archive, 2023, 31(10): 6236-6259. doi: 10.3934/era.2023316
[1] | Nina Huo, Bing Li, Yongkun Li . Global exponential stability and existence of almost periodic solutions in distribution for Clifford-valued stochastic high-order Hopfield neural networks with time-varying delays. AIMS Mathematics, 2022, 7(3): 3653-3679. doi: 10.3934/math.2022202 |
[2] | Yongkun Li, Xiaoli Huang, Xiaohui Wang . Weyl almost periodic solutions for quaternion-valued shunting inhibitory cellular neural networks with time-varying delays. AIMS Mathematics, 2022, 7(4): 4861-4886. doi: 10.3934/math.2022271 |
[3] | Ardak Kashkynbayev, Moldir Koptileuova, Alfarabi Issakhanov, Jinde Cao . Almost periodic solutions of fuzzy shunting inhibitory CNNs with delays. AIMS Mathematics, 2022, 7(7): 11813-11828. doi: 10.3934/math.2022659 |
[4] | Yuwei Cao, Bing Li . Existence and global exponential stability of compact almost automorphic solutions for Clifford-valued high-order Hopfield neutral neural networks with D operator. AIMS Mathematics, 2022, 7(4): 6182-6203. doi: 10.3934/math.2022344 |
[5] | Jin Gao, Lihua Dai . Weighted pseudo almost periodic solutions of octonion-valued neural networks with mixed time-varying delays and leakage delays. AIMS Mathematics, 2023, 8(6): 14867-14893. doi: 10.3934/math.2023760 |
[6] | Hedi Yang . Weighted pseudo almost periodicity on neutral type CNNs involving multi-proportional delays and D operator. AIMS Mathematics, 2021, 6(2): 1865-1879. doi: 10.3934/math.2021113 |
[7] | Yanshou Dong, Junfang Zhao, Xu Miao, Ming Kang . Piecewise pseudo almost periodic solutions of interval general BAM neural networks with mixed time-varying delays and impulsive perturbations. AIMS Mathematics, 2023, 8(9): 21828-21855. doi: 10.3934/math.20231113 |
[8] | Zhangir Nuriyev, Alfarabi Issakhanov, Jürgen Kurths, Ardak Kashkynbayev . Finite-time synchronization for fuzzy shunting inhibitory cellular neural networks. AIMS Mathematics, 2024, 9(5): 12751-12777. doi: 10.3934/math.2024623 |
[9] | Abdulaziz M. Alanazi, R. Sriraman, R. Gurusamy, S. Athithan, P. Vignesh, Zaid Bassfar, Adel R. Alharbi, Amer Aljaedi . System decomposition method-based global stability criteria for T-S fuzzy Clifford-valued delayed neural networks with impulses and leakage term. AIMS Mathematics, 2023, 8(7): 15166-15188. doi: 10.3934/math.2023774 |
[10] | Xiaofang Meng, Yongkun Li . Pseudo almost periodic solutions for quaternion-valued high-order Hopfield neural networks with time-varying delays and leakage delays on time scales. AIMS Mathematics, 2021, 6(9): 10070-10091. doi: 10.3934/math.2021585 |
We investigate the synchronizability of multilayer star-ring networks. Two types of multilayer networks, including aggregated coupling and divergent coupling, are established based on the connections between the hub node and the leaf nodes in the subnetwork. The eigenvalue spectrum of the two types of multilayer networks is strictly derived, and the correlation between topological parameters and synchronizability is analyzed by the master stability function framework. Moreover, the variable coupling strength has been investigated, revealing that it is significantly related to the synchronizability of the aggregated coupling while having no influence on the divergent coupling. Furthermore, the validity of the synchronizability analysis is obtained by implementing adaptive control on the multilayer star-ring networks previously mentioned. Calculations and comparisons show that the differences caused by the sizes of multilayer networks and interlayer coupling strength are not negligible. Finally, numerical examples are also provided to validate the effectiveness of the theoretical analysis.
As stated in [1], a nervous system in the real world, synaptic transmission is a noisy process caused by random fluctuations in neurotransmitter release and other probabilistic factors. Therefore, it is necessary to consider stochastic neural networks (NNs) because random inputs may change the dynamics of the (NN) [2,3,4,5].
SICNNs, which were proposed in [6], have attracted the interest of many scholars since their introduction due to their special roles in psychophysics, robotics, adaptive pattern recognition, vision, and image processing. In the above applications, their dynamics play an important role. Thereupon, their various dynamics have been extensively studied (see [7,8,9,10,11,12,13] and references therein). However, there is limited research on the dynamics of stochastic SICNNs. Therefore, it is necessary to further study the dynamics of such NNs.
On the one hand, research on the dynamics of NNs that take values from a non commutative algebra, such as quaternion-valued NNs [14,15,16], octonion-valued NNs [17,18,19,20], and Clifford-valued NNs [21,22,23], has gained the interest of many researchers because such neural networks can include typical real-valued NNs as their special cases, and they have superior multi-dimensional signal processing and data storage capabilities compared to real-valued NNs. It is worth mentioning that in recent years, many authors have conducted extensive research on various dynamics of Clifford-valued NNs, such as the existence, multiplicity and stability of equilibrium points, and the existence, multiplicity and stability of almost periodic solutions as well as the synchronization problems [22,23,24,25,26,27,28,29,30]. However, most of the existing results for the dynamics of Clifford-valued NNs has been obtained through decomposition methods [24,25,26,27]. However, the results obtained by decomposition methods are generally not convenient for direct application, and there is little research on Clifford-valued NNs using non decomposition methods [28,29,30]. Therefore, further exploration of using non decomposition methods to study the dynamics of Clifford-valued NNs has important theoretical significance and application value.
On the other hand, Bohr's almost periodicity is a special case of Stepanov's almost periodicity, but there is little research on the Stepanov periodic oscillations of NNs [19,31,32,33], especially the results of Stepanov's almost periodic solutions of stochastic SICNNs with discrete and infinitely distributed delays have not been published yet.
Motivated by the discussion above, our purpose of this article is to establish the existence and global exponential stability of Stepanov almost periodic solutions in the distribution sense for a stochastic Clifford-valued SICNN with mixed delays via non decomposition methods.
The subsequent sections of this article are organized as follows. Section 2 introduces some concepts, notations, and basic lemmas and gives a model description. Section 3 discusses the existence and stability of Stepanov almost periodic solutions in the distribution sense of the NN under consideration. An example is provided in Section 4. Finally, Section 5 provides a brief conclusion.
Let A={∑ϑ∈Pxϑeϑ,xϑ∈R} be a real Clifford-algebra with N generators e∅=e0=1, and eh,h=1,2,⋯,N, where P={∅,0,1,2,⋯,ϑ,⋯,12⋯N}, e2i=1,i=1,2,⋯,r,e2i=−1,i=r+1,r+2,⋯,m,eiej+ejei=0,i≠j and i,j=1,2,⋯,N. For x=∑ϑ∈Pxϑeϑ∈A, we indicate ‖x‖♭=maxϑ∈P{|xϑ|},xc=∑ϑ≠∅xϑeϑ,x0=x−xc, and for x=(x11,x12,…,x1n,x21,x22,…,x2n,…,xmn)T∈Am×n, we denote ‖x‖0=max{‖xij‖♭,1≤i≤m,1≤j≤n}. The derivative of x(t)=∑ϑ∈Pxϑ(t)eϑ is defined by ˙x(t)=∑ϑ∈P˙xϑ(t)eϑ and the integral of x(t)=∑ϑ∈Pxϑ(t)eϑ over the interval [a,b] is defined by ∫bax(t)dt=∑ϑ∈P(∫baxϑ(t)dt)eϑ.
Let (Y,ρ) be a separable metric space and P(Y) the collection of all probability measures defined on Borel σ-algebra of Y. Denote by Cb(Y) the set of continuous functions f:Y→R with ‖g‖∞:=supx∈Y{|g(x)|}<∞.
For g∈Cb(Y), μ,ν∈P(Y), let us define
‖g‖L=supx≠y|g(x)−g(y)|ρ(x,y),‖g‖BL=max{‖g‖∞,‖g‖L}, |
ρBL(μ,ν):=sup‖g‖BL≤1|∫Ygd(μ−ν)|. |
According to [34], (Y,ρBL(⋅,⋅)) is a Polish space.
Definition 2.1. [35] A continuous function g:R→Y is called almost periodic if for every ε>0, there is an ℓ(ε)>0 such that each interval with length ℓ has a point τ meeting
ρ(g(t+τ),g(t))<ε,forallt∈R. |
We indicate by AP(R,Y) the set of all such functions.
Let (X,‖⋅‖) signify a separable Banach space. Denote by μ(X):=P∘X−1 and E(X) the distribution and the expectation of X:(Ω,F,P)→X, respectively.
Let Lp(Ω,X) indicate the family of all X-valued random variables satisfying E(‖X‖p)=∫Ω‖X‖pdP<∞.
Definition 2.2. [21] A process Z:R→Lp(Ω,X) is called Lp-continuous if for any t0∈R,
limt→t0E‖Z(t)−Z(t0)‖p=0. |
It is Lp-bounded if supt∈RE‖Z(t)‖p<∞.
For 1<p<∞, we denote by Lploc(R,X) the space of all functions from R to X which are locally p-integrable. For g∈Lploc(R,X), we consider the following Stepanov norm:
‖g‖Sp=supt∈R(∫t+1t‖g(s)‖pds)1p. |
Definition 2.3. [35] A function g∈Lploc(R,X) is called p-th Stepanov almost periodic if for any ε>0, it is possible to find a number ℓ>0 such that every interval with length ℓ has a number τ such that
‖g(t+τ)−g(t)‖Sp<ε. |
Definition 2.4. [9] A stochastic process Z∈Lploc(R,Lp(Ω,X)) is said to be Sp-bounded if
‖Z‖Sps:=supt∈R(∫t+1tE‖Z(s)‖pds)1p<∞. |
Definition 2.5. [9] A stochastic process Z∈Lloc(R,Lp(Ω,H)) is called Stepanov almost periodic in p-th mean if for any ε>0, it is possible to find a number ℓ>0 such that every interval with length ℓ has a number τ such that
‖Z(t+τ)−Z(t)‖Sps<ε. |
Definition 2.6. [9] A stochastic process Z:R→Lp(Ω,X)) is said to be p-th Stepanov almost periodic in the distribution sense if for each ε>0, it is possible to find a number ℓ>0 such that any interval with length ℓ has a number τ such that
supa∈R(∫a+1adpBL(P∘[Z(t+τ)]−1,P∘[Z(t)]−1)dt)1p<ε. |
Lemma 2.1. [36] (Burkholder-Davis-Gundy inequality) If f∈L2(J,R), p>2, B(t) is Brownian motion, then
E[supt∈J|∫tt0f(s)dB(s)|p]≤CpE[∫Tt0|f(s)|2ds]p2, |
where cp=(pp+12(p−1)p−1)p2.
The model that we consider in this paper is the following stochastic Clifford-valued SICNN with mixed delays:
dxij(t)=[−aij(t)xij(t)+∑Ckl∈Nh1(i,j)Cklij(t)f(xkl(t−τkl(t)))xij(t)+∑Ckl∈Nh2(i,j)Bklij(t)∫∞0Kij(u)g(xkl(t−u))duxij(t)+Lij(t)]dt+∑Ckl∈Nh3(i,j)Eklij(t)δij(xij(t−σij(t)))dωij(t), | (2.1) |
where i=1,2,⋯,m,j=1,2,⋯,n, Cij(t) represents the cell at the (i,j) position, the h1-neighborhood Nh1(i,j) of Cij is given as:
Nh1(i,j)={Ckl:max(|k−i|,|l−j|)≤h1,1≤k≤m,1≤l≤n}, |
Nh2(i,j),Nh3(i,j) are similarly defined, xij denotes the activity of the cell Cij, Lij(t):R→A corresponds to the external input to Cij, the function aij(t):R→A represents the decay rate of the cell activity, Cklij(t):R→A,Bklij(t):R→A and Eklij(t):R→A signify the connection or coupling strength of postsynaptic activity of the cell transmitted to the cell Cij, and the activity functions f(⋅):A→A, and g(⋅):A→A are continuous functions representing the output or firing rate of the cell Ckl, and τkl(t),σij(t):R→R+ are the transmission delay, the kernel Kij(t):R→R is an integrable function, ωij(t) represents the Brownian motion defined on a complete probability space, δij(⋅):A→A is a Borel measurable function.
Let (Ω, F, {Ft}t⩾0, P) be a complete probability space in which {Ft}t⩾0 is a natural filtration meeting the usual conditions. Denote by BF0([−θ,0],An) the family of bounded, F0-measurable and An-valued random variables from [−θ,0]→An. The initial values of system (2.1) are depicted as
xi(s)=ϕi(s),s∈[−θ,0], |
where ϕi∈BF0([−θ,0],A),θ=max1≤i,j≤n{supt∈Rτij(t),supt∈Rσij(t)}.
For convenience, we introduce the following notations:
a_0=minij∈Λa_0ij=minij∈Λinft∈Ra0ij(t),ˉa0=maxij∈Λˉa0ij=maxij∈Λsupt∈Ra0ij(t),Cklij+=supt∈R‖Cklij(t)‖♭,¯ac=maxij∈Λˉacij=maxij∈Λsupt∈R‖acij(t)‖♭,Bklij+=supt∈R‖Bklij(t)‖♭,Eklij+=supt∈R‖Eklij(t)‖♭,K+ij=supt∈RKij(t),τ+kl=supt∈Rτkl(t),˙τ+kl=supt∈R˙τkl(t),σ+ij=supt∈Rσij(t),˙σ+ij=supt∈R˙σij(t),ML=maxij∈ΛL+ij=maxij∈Λsupt∈R‖Lij(t)‖♭,θ=maxij∈Λ{τ+ij,σ+ij},Λ={11,12,⋯,1n,⋯,mn}. |
Throughout this paper, we make the following assumptions:
(A1) For ij∈Λ, f,g,δij∈C(A,A) satisfy the Lipschitz condition, and f,g are bounded, that is, there exist constants Lf>0,Lg>0,Lδij>0,Mf>0,Mg>0 such that for all x,y∈A,
||f(x)−f(y)||♭≤Lf||x−y||♭,||g(x)−g(y)||♭≤Lg||x−y||♭,||δij(x)−δij(y)||♭≤Lδij||x−y||♭,||f(x)||♭≤Mf,||g(x)||♭≤Mg; |
furthermore, f(0)=g(0)=δij(0)=0.
(A2) For ij∈Λ, a0ij∈AP(R,R+),acij∈AP(R,A),τij,σij∈AP(R,R+)∩C1(R,R) satisfying 1−˙τ+ij,1−˙σ+ij>0, Cklij,Bklij,Eklij∈AP(R,A), L=(L11,L12,⋯,Lmn)∈Lploc(R,Lp(Ω,Am×n)) is almost periodic in the sense of Stepanov.
(A3) For p>2,1p+1q=1,
0<r1:=8p4maxij∈Λ{(pqa_0ij)pqqpa_0ij[(ˉacij)p+(∑Ckl∈Nh1(i,j)(Cklij+)q)pq(2κLf+Mf)p+(∑Ckl∈Nh2(i,j)(Bklij+)q)pq((2κLg+Mg)∫∞0|Kij(u)|du)p]+Cp(p−22a_0ij)p−22qpa_0ij(∑Ckl∈Nh3(i,j)(Eklij+)q)pq(Lδij)p}<1, |
and for p=2,
0<r2:=16maxij∈Λ{1(a_0ij)2[(ˉacij)2+∑Ckl∈Nh1(i,j)(Cklij+)2(2κLf+Mf)2+∑Ckl∈Nh2(i,j)(Bklij+)2×((2κLg+Mg)∫∞0|Kij(u)|du)2]+12a_0ij∑Ckl∈Nh3(i,j)(Eklij+)2(Lδij)2}<1. |
(A4) For 1p+1q=1,
0<qpa_0ρ1:=16p−1qpa_0maxij∈Λ{(pqa_0ij)pq[(ˉacij)p+(∑Ckl∈Nh1(i,j)(Cklij+)q)pq[2p−1(Lf)p×∑Ckl∈Nh1(i,j)epqa_0ijτkl+(2κ)p1−˙τ+kl+(Mf)p]+(∑Ckl∈Nh2(i,j)(Bklij+)q)pq[(2κLg×∫∞0|Kij(u)|du)p+(Mg∫∞0|Kij(u)|du)p]]+2p−1Cp(p−22a_0ij)p−22×(∑Ckl∈Nh3(i,j)(Eklij+)q)pq(Lδij)pepqa_0ijσ+ij1−˙σ+ij}<1,(p>2), |
0<ρ2a_0:=32a_0maxij∈Λ{(1a_0ij)∑Ckl∈Nh1(i,j)(Cklij+)2[(Lf)2∑Ckl∈Nh1(i,j)ea_0ijτkl+(2κ)21−˙τ+kl+(Mf)22]+∑Ckl∈Nh3(i,j)(Eklij+)2(Lδij)2e2a_0ijσ+ij1−˙σ+ij+12a_0ij∑Ckl∈Nh2(i,j)(Bklij+)2(4κ2L2g+M2g)×(∫∞0|Kij(u)|du)2+(ˉacij)22a_0ij}<1,(p=2). |
(A5) The kernel Kij is almost periodic and there exist constants M>0 and u>0 such that |Kij(t)|≤Me−ut for all t∈R.
Let X indicate the space of all Lp-bounded and Lp-uniformly continuous stochastic processes from R to Lp(Ω,Am×n), then with the norm ‖ϕ‖X=supt∈R{E‖ϕ(t)‖p0}1p, where ϕ=(ϕ11,ϕ12,…,ϕmn)∈X, it is a Banach space.
Set ϕ0=(ϕ011,ϕ012,…,ϕ0mn)T, where ϕ0ij(t)=∫t−∞e−∫tsa0ij(u)duLij(s)ds,t∈R,ij∈Λ. Then, ϕ0 is well defined under assumption (A2). Consequently, we can take a constant κ such that κ≥‖ϕ0‖X.
Definition 3.1. [37] An Ft-progressively measurable stochastic process x(t)=(x11(t),x12(t),…,xmn(t))T is called a solution of system (2.1), if x(t) solves the following integral equation:
xij(t)=xij(t0)e−∫tt0a0ij(u)du+∫tt0e−∫tsa0ij(u)du[−acij(s)xij(s)+∑Ckl∈Nh1(i,j)Cklij(s)×f(xkl(s−τkl(s)))xij(s)+∑Ckl∈Nh2(i,j)Bklij(s)∫∞0Kij(u)g(x(s−u))duxij(s)+Lij(s)]ds+∫tt0e−∫tsa0ij(u)du∑Ckl∈Nh3(i,j)Eklij(s)δij(xij(s−σij(s)))dwij(s). | (3.1) |
In (3.1), let t0→−∞, then one gets
xij(t)=∫t−∞e−∫tsa0ij(u)du[−acij(s)xij(s)+∑Ckl∈Nh1(i,j)Cklij(s)f(xkl(s−τkl(s)))xij(s)+∑Ckl∈Nh2(i,j)Bklij(s)∫∞0Kij(u)g(x(s−u))duxij(s)+Lij(s)]ds+∫t−∞e−∫tsa0ij(u)du×∑Ckl∈Nh3(i,j)Eklij(s)δij(xij(s−σij(s)))dwij(s),t≥t0,ij∈Λ. | (3.2) |
It is easy to see that if x(t) solves (3.2), then it also solves (2.1).
Theorem 3.1. Assume that (A1)–(A4) hold. Then the system (2.1) has a unique Lp-bounded and Lp-uniformly continuous solution in X∗={ϕ∈X:‖ϕ−ϕ0‖X≤κ}, where κ is a constant satisfying κ≥‖ϕ0‖X.
Proof. Define an operator ϕ:X∗→X as follows:
(Ψϕ)(t)=((Ψ11ϕ)(t),(Ψ12ϕ)(t),…,(Ψmnϕ)(t))T, |
where (ϕ11,ϕ12,…,ϕmn)T∈X, t∈R and
(Ψijϕ)(t)=∫t−∞e−∫tsa0ij(u)du[−acij(s)ϕij(s)+∑Ckl∈Nh1(i,j)Cklij(s)f(ϕkl(s−τkl(s)))ϕij(s)+∑Ckl∈Nh2(i,j)Bklij(s)∫∞0Kij(u)g(ϕkl(s−u))duϕij(s)+Lij(s)]ds+∫t−∞e−∫tsa0ij(u)du∑Ckl∈Nh3(i,j)Eklij(s)δij(ϕij(s−σij(s)))dωij(s),ij∈Λ. | (3.3) |
First of all, let us show that E‖Ψϕ(t)−ϕ0(t)‖p0≤κ for all ϕ∈X∗.
Noticing that for any ϕ∈X∗, it holds
‖ϕ‖X≤‖ϕ0‖X+‖ϕ−ϕ0‖X≤2κ. |
Then, we deduce that
E‖Ψϕ(t)−ϕ0(t)‖p0≤4p−1maxij∈Λ{E‖∫t−∞−e−∫tsa0ij(u)duacij(s)ϕij(s)‖p♭}+4p−1maxij∈Λ{E‖∫t−∞e−∫tsa0ij(u)du×∑Ckl∈Nh1(i,j)Cklij(s)f(ϕkl(s−τkl(s)))ϕij(s)ds‖p♭}+4p−1maxij∈Λ{E‖∫t−∞e−∫tsa0ij(u)du×∑Ckl∈Nh2(i,j)Bklij(s)∫∞0Kij(u)g(ϕkl(s−u))duϕij(s)ds‖p♭}+4p−1maxij∈Λ{E‖∫t−∞e−∫tsa0ij(u)du∑Ckl∈Nh3(i,j)Eklij(s)δij(ϕij(s−σij(s)))dωij(s)‖p♭}:=F1+F2+F3+F4. | (3.4) |
By the Hölder inequality, we have
F2≤4p−1maxij∈Λ{E‖[∫t−∞e−qp∫tsa0ij(u)duds]pq[∫t−∞e−pq∫tsa0ij(u)du×(∑Ckl∈Nh1(i,j)Cklij(s)f(ϕkl(s−τkl(s)))ϕij(s))pds]‖♭}≤4p−1maxij∈Λ{(pqa_0ij)pqE[∫t−∞e−pq∫tsa0ij(u)du(∑Ckl∈Nh1(i,j)(‖Cklij(s)‖♭)q)pq×∑ij∈Λ(2κLf)p‖ϕij(s)‖p♭ds]}≤4p−1maxij∈Λ{(pqa_0ij)pqqpa_0ij(∑Ckl∈Nh1(i,j)(Cklij+)q)pq(2κLf)p}‖ϕ‖pX. | (3.5) |
Similarly, one has
F1≤4p−1maxij∈Λ{(pqa_0ij)pqqpa_0ij(ˉacij)p}‖ϕ‖pX, | (3.6) |
F3≤4p−1maxij∈Λ{(pqa_0ij)pqqpa_0ij(∑Ckl∈Nh2(i,j)(Bklij+)q)pq(2κLg∫∞0|Kij(u)|du)p}‖ϕ‖pX. | (3.7) |
By the Burkolder-Davis-Gundy inequality and the Hölder inequality, when p>2, we infer that
F4≤4p−1Cpmaxij∈Λ{E[∫t−∞‖e−∫tsa0ij(u)du∑Ckl∈Nh3(i,j)Eklij(s)δij(ϕij(s−σij(s)))‖2♭ds]p2}≤4p−1Cpmaxij∈Λ{E[e−2∫tsa0ij(u)du‖∑Ckl∈Nh3(i,j)Eklijδij(ϕij(s−σij(s)))‖2♭ds]p2}≤4p−1Cpmaxij∈Λ{E[∫t−∞(e−2∫tsa0ij(u)du)pp−2×1pds]p−2p×p2×E[∫t−∞(e−2∫tsa0ij(u)du)1q×p2(‖∑Ckl∈Nh3(i,j)Eklij(s)δijϕij(s−σij(s))‖2♭)p2ds]}≤4p−1Cpmaxij∈Λ{(p−22a_0ij)p−22qpa_0ijE‖∑Ckl∈Nh3(i,j)Eklij(s)δij(ϕij(s−σij(s)))‖p♭}≤4p−1Cpmaxij∈Λ{(p−22a_0ij)p−22qpa_0ij(∑Ckl∈Nh3(i,j)(Eklij+)q)pq(Lδij)p}‖ϕ‖pX. | (3.8) |
When p=2, by the Itˆo isometry, it follows that
F4≤4maxij∈Λ{E[∫t−∞e−2∫tsa0ij(u)du‖∑Ckl∈Nh3(i,j)Eklij(s)δij(ϕij(s−σij(s)))‖2Ads]}≤4maxij∈Λ{12a_0ij∑Ckl∈Nh3(i,j)(Eklij+)2(Lδij)2}‖ϕ‖2X. | (3.9) |
Putting (3.5)–(3.9) into (3.4), we obtain that
‖Ψϕ−ϕ0‖pX≤4p−1maxij∈Λ{(pqa_0ij)pqqpa_0ij[(ˉacij)p+(∑Ckl∈Nh1(i,j)(Cklij+)q)pq(2κLf)p+(∑Ckl∈Nh2(i,j)(Bklij+)q)pq(2κLg∫∞0|Kij(u)|du)p]+Cp(p−22a_0ij)p−22qpa_0ij(∑Ckl∈Nh3(i,j)(Eklij+)q)pq(Lδij)p}‖ϕ‖pX≤κp,(p>2), | (3.10) |
and
‖Ψϕ−ϕ0‖2X≤4maxij∈Λ{1(a−ij)2[(ˉacij)2+∑Ckl∈Nh1(i,j)(Cklij+)2(2κLf)2+∑Ckl∈Nh2(i,j)(Bklij+)2(2κLg×∫∞0|Kij(u)|du)2]+12a_0ij∑Ckl∈Nh3(i,j)(Eklij+)2(Lδij)2}‖ϕ‖2X≤κ2,(p=2). | (3.11) |
It follows from (3.10), (3.11) and (A3) that ‖Ψϕ−ϕ0‖X≤κ.
Then, using the same method as that in the proof of Theorem 3.2 in [21], we can show that Ψϕ is Lp-uniformly continuous. Therefore, we have Ψ(X∗)⊂X∗.
Last, we will show that Ψ is a contraction mapping. Indeed, for any ψ,φ∈X∗, when p>2, we have
E‖(Φφ)(t)−(Φψ)(t)‖p0≤4p−1maxij∈Λ{E‖∫t−∞e−∫tsa0ij(u)du(−acij(s)φij(s)+acij(s)ψij(s))ds‖p♭}+4p−1maxij∈Λ{E‖∫t−∞e−∫tsa0ij(u)du∑Ckl∈Nh1(i,j)Cklij(s)[f(φkl(s−τkl(s)))φij(s)−f(ψkl(s−τkl(s)))ψij(s)]ds‖p♭}+4p−1maxij∈Λ{E‖∫t−∞e−∫tsa0ij(u)du∑Ckl∈Nh2(i,j)Bklij(s)×[∫∞0Kij(u)g(φkl(s−u))duφij(s)−∫∞0Kij(u)g(ψkl(s−u))duψij(u)]ds‖p♭}+4p−1maxij∈Λ{E‖∫t−∞e−∫tsa0ij(u)du∑Ckl∈Nh3(i,j)Eklij(s)[δij(φij(s−σij(s)))−δij(ψij(s−σij(s)))]dωij(s)‖p♭}≤4p−1maxij∈Λ{(pqa_0ij)pqqpa_0ij[(ˉacij)p+(∑Ckl∈Nh1(i,j)(Cklij+)q)pq(2κLf+Mf)p+(∑Ckl∈Nh2(i,j)(Bklij+)q)pq((2κLg+Mg)∫∞0|Kij(u)|du)p]+Cp(p−22a_0ij)p−22qpa_0ij×(∑Ckl∈Nh3(i,j)(Eklij+)q)pq(Lδij)p}‖φ−ψ‖pX. | (3.12) |
Similarly, for , we can get
(3.13) |
From (3.12) and (3.13) it follows that
Hence, by virtue of , is a contraction mapping. So, has a unique fixed point in , i.e., (2.1) has a unique solution in .
Theorem 3.2. Assume that – hold. Then the system (2.1) has a unique -th Stepanov-like almost periodic solution in the distribution sense in , where is a constant satisfying .
Proof. From Theorem 3.1, we know that (2.1) has a unique solution in . Now, let us show that is Stepanov-like almost periodic in distribution. Since , it is -uniformly continuous and satisfies . So, for any , there exists , when , we have . Hence, we derive that
(3.14) |
For the above, according to , we have, for ,
As , by (3.14), there holds
Based on (3.2), we can infer that
in which is a Brownian motion having the same distribution as .
Let us consider the process
(3.15) |
From (3.2) and (3.15), we deduce that
(3.16) |
Employing the Hölder inequality, we can obtain
By a change of variables and Fubini's theorem, we infer that
(3.17) |
where
Similarly, when , one can obtain
(3.18) |
where
(3.19) |
and when , we have
(3.20) |
where
(3.21) |
In the same way, we can get
(3.22) |
(3.23) |
(3.24) |
(3.25) |
(3.26) |
(3.27) |
(3.28) |
(3.29) |
Noting that
(3.30) |
We can gain
(3.31) |
(3.32) |
(3.33) |
(3.34) |
when , we have
(3.35) |
for , we get
(3.36) |
Substituting (3.17)–(3.36) into (3.16), we have the following two cases:
Case 1. When , we have
where is the same as that in and
By , we know . Hence, we derive that
(3.37) |
Case 2. When , we can obtain
where is defined in and
Similar to the previous case, by , we know and hence, we can get that
(3.38) |
Noting that
Hence, we have
(3.39) |
Combining (3.37)–(3.39), we can conclude that is -th Stepanov almost periodic in the distribution sense. The proof is complete.
Similar to the proof of Theorem 3.7 in [21], one can easily show that.
Theorem 3.3. Suppose that – are fulfilled and let be the Stepanov almost periodic solution in the distribution sense of system (2.1) with initial value . Then there exist constants and such that for an arbitrary solution with initial value satisfies
where , i.e., the solution is globally exponentially stable.
The purpose of this section is to demonstrate the effectiveness of the results obtained in this paper through a numerical example.
In neural network (2.1), choose , , and
and let . Then we get
Take , , then we have
And when , we have
Thus, all assumptions in Theorems 3.2 and 3.3 are fulfilled. So we can conclude that the system (2.1) has a unique -almost periodic solution in the distribution sense which is globally exponentially stable.
The results are also verified by the numerical simulations in Figures 1–4.
From these figures, we can observe that when the four primitive components of each solution of this system take different initial values, they eventually tend to stabilize. It can be seen that these solutions that meet the above conditions do exist and are exponentially stable.
In this article, we establish the existence and global exponential stability of Stepanov almost periodic solutions in the distribution sense for a class of stochastic Clifford-valued SICNNs with mixed delays. Even when network (2.1) degenerates into a real-valued NN, the results of this paper are new. In fact, uncertainty, namely fuzziness, is also a problem that needs to be considered in real system modeling. However, we consider only the disturbance of random factors and do not consider the issue of fuzziness. In a NN, considering the effects of both random perturbations and fuzziness is our future direction of effort.
The authors declare that they have not used Artificial Intelligence (AI) tools in the creation of this article.
This work is supported by the National Natural Science Foundation of China under Grant No. 12261098.
The authors declare that they have no conflicts of interest.
[1] |
R. Albert, A. L. Barabási, Statistical mechanics of complex networks, Rev. Mod. Phys., 74 (2002), 47–97. https://doi.org/10.1103/RevModPhys.74.47 doi: 10.1103/RevModPhys.74.47
![]() |
[2] |
A. L. Barabasi, Z. N. Oltvai, Network biology: understanding the cell's functional organization, Nat. Rev. Genet., 5 (2004), 101–113. https://doi.org/10.1038/nrg1272 doi: 10.1038/nrg1272
![]() |
[3] |
M. E. J. Newman, Communities, modules and large-scale structure in networks, Nat. Phys., 8 (2012), 25–31. https://doi.org/10.1038/nphys2162 doi: 10.1038/nphys2162
![]() |
[4] |
J. Lin, Y. Ban, Complex network topology of transportation systems, Transport Rev., 33 (2013), 658–685. https://doi.org/10.1080/01441647.2013.848955 doi: 10.1080/01441647.2013.848955
![]() |
[5] |
R. E. Mirollo, S. H. Strogatz, Synchronization of pulse-coupled biological oscillators, SIAM J. Appl. Math., 50 (1990), 1645–1662. https://doi.org/10.1137/0150098 doi: 10.1137/0150098
![]() |
[6] |
X. Zhang, S. Boccaletti, S. Guan, Z. Liu, Explosive synchronization in adaptive and multilayer networks, Phys. Rev. Lett., 114 (2015), 038701. https://doi.org/10.1103/PhysRevLett.114.038701 doi: 10.1103/PhysRevLett.114.038701
![]() |
[7] |
P. Wang, G. Wen, X. Yu, W. Yu, T. Huang, Synchronization of multi-layer networks: from node-to-node synchronization to complete synchronization, IEEE Trans. Circuits Syst. I Regul. Pap., 66 (2018), 1141–1152. https://doi.org/10.1109/TCSI.2018.2877414 doi: 10.1109/TCSI.2018.2877414
![]() |
[8] |
H. Liu, Y. Li, Z. Li, J. Lü, J. Lu, Topology identification of multilink complex dynamical networks via adaptive observers incorporating chaotic exosignals, IEEE Trans. Cybern., 52 (2022), 6255–6268. https://doi.org/10.1109/TCYB.2020.3042223 doi: 10.1109/TCYB.2020.3042223
![]() |
[9] |
G. Mei, X. Wu, Y. Wang, M. Hu, J. Lu, G. Chen, Compressive-sensing-based structure identification for multilayer networks, IEEE Trans. Cybern., 48 (2017), 754–764. https://doi.org/10.1109/TCYB.2017.2655511 doi: 10.1109/TCYB.2017.2655511
![]() |
[10] |
X. Wang, A. Tejedor, Y. Wang, Y. Moreno, Unique superdiffusion induced by directionality in multiplex networks, New J. Phys., 23 (2021), 013016. https://doi.org/10.1088/1367-2630/abdb71 doi: 10.1088/1367-2630/abdb71
![]() |
[11] |
M. Turalska, K. Burghardt, M. Rohden, A. Swami, R. M. D'Souza, Cascading failures in scale-free interdependent networks, Phys. Rev. E: Stat. Nonlinear Soft Matter Phys., 99 (2019), 032308. https://doi.org/10.1103/PhysRevE.99.032308 doi: 10.1103/PhysRevE.99.032308
![]() |
[12] |
J. Chang, X. Yin, C. Ma, D. Zhao, Y. Sun, Estimation of the time cost with pinning control for stochastic complex networks, Electron. Res. Arch., 30 (2022), 3509–3526. https://doi.org/10.3934/era.2022179 doi: 10.3934/era.2022179
![]() |
[13] |
S. S. Sajjadi, D. Baleanu, A. Jajarmi, H. M. Pirouz, A new adaptive synchronization and hyperchaos control of a biological snap oscillator, Chaos, Solitons Fractals, 138 (2020), 109919. https://doi.org/10.1016/j.chaos.2020.109919 doi: 10.1016/j.chaos.2020.109919
![]() |
[14] |
S. Liu, R. Zhang, Q. Wang, X. He, Sliding mode synchronization between uncertain Watts-Strogatz small-world spatiotemporal networks, Appl. Math. Mech., 41 (2020), 1833–1846. https://doi.org/10.1007/s10483-020-2686-6 doi: 10.1007/s10483-020-2686-6
![]() |
[15] |
K. Hengster-Movric, K. You, F. L. Lewis, L. Xie, Synchronization of discrete-time multi-agent systems on graphs using Riccati design, Automatica, 49 (2013), 414–423. https://doi.org/10.1016/j.automatica.2012.11.038 doi: 10.1016/j.automatica.2012.11.038
![]() |
[16] |
L. M. Pecora, T. L. Carrollton, Synchronization in chaotic systems, Phys. Rev. Lett., 64 (1990), 821–827. https://doi.org/10.1103/PhysRevLett.64.821 doi: 10.1103/PhysRevLett.64.821
![]() |
[17] |
Z. Wu, X. Fu, Complex projective synchronization in drive-response networks coupled with complex-variable chaotic systems, Nonlinear Dyn., 72 (2013), 9–15. https://doi.org/10.1007/s11071-012-0685-7 doi: 10.1007/s11071-012-0685-7
![]() |
[18] |
H. Hong, M. Y. Choi, B. J. Kim, Synchronization on small-world networks, Phys. Rev. E: Stat. Nonlinear Soft Matter Phys., 65 (2002), 026139. https://doi.org/10.1103/PhysRevE.65.026139 doi: 10.1103/PhysRevE.65.026139
![]() |
[19] |
K. Li, S. Guan, X. Gong, C. H. Lai, Synchronization stability of general complex dynamical networks with time-varying delays, Phys. Lett. A, 372 (2008), 7133–7139. https://doi.org/10.1016/j.physleta.2008.10.054 doi: 10.1016/j.physleta.2008.10.054
![]() |
[20] |
X. Zhao, J. Zhou, J. Lu, Pinning synchronization of multiplex delayed networks with stochastic perturbations, IEEE Trans. Cybern., 49 (2018), 4262–4270. https://doi.org/10.1109/TCYB.2018.2861822 doi: 10.1109/TCYB.2018.2861822
![]() |
[21] |
A. Fan, J. Li, J. Li, Adaptive event-triggered prescribed performance learning synchronization for complex dynamical networks with unknown time-varying coupling strength, Nonlinear Dyn., 100 (2020), 2575–2593. https://doi.org/10.1007/s11071-020-05648-w doi: 10.1007/s11071-020-05648-w
![]() |
[22] |
S. Gomez, A. Diaz-Guilera, J. Gomez-Gardenes, C. J. Pérez-Vicente, Y. Moreno, A. Arenas, Diffusion dynamics on multiplex networks, Phys. Rev. Lett., 110 (2013), 028701. https://doi.org/10.1103/PhysRevLett.110.028701 doi: 10.1103/PhysRevLett.110.028701
![]() |
[23] |
J. A. Almendral, A. Díaz-Guilera, Dynamical and spectral properties of complex networks, New J. Phys., 9 (2007), 187. https://doi.org/10.1088/1367-2630/9/6/187 doi: 10.1088/1367-2630/9/6/187
![]() |
[24] |
C. Granell, S. Gómez, A. Arenas, Dynamical interplay between awareness and epidemic spreading in multiplex networks, Phys. Rev. Lett., 111 (2013), 128701. https://doi.org/10.1103/PhysRevLett.111.128701 doi: 10.1103/PhysRevLett.111.128701
![]() |
[25] |
J. Aguirre, R. Sevilla-Escoboza, R. Gutierrez, D. Papo, M. Buldú, Synchronization of interconnected networks: the role of connector nodes, Phys. Rev. Lett., 112 (2014), 248701. https://doi.org/10.1103/PhysRevLett.112.248701 doi: 10.1103/PhysRevLett.112.248701
![]() |
[26] |
M. Xu, J. Lu, J. Zhou, Synchronizability and eigenvalues of two-layer star networks (in Chinese), Acta Phys. Sin., 65 (2016), 028902. https://doi.org/10.7498/aps.65.028902 doi: 10.7498/aps.65.028902
![]() |
[27] |
J. Li, Y. Luan, X. Wu, J. Lu, Synchronizability of double-layer dumbbell networks, Chaos, 31 (2021), 073101. https://doi.org/10.1063/5.0049281 doi: 10.1063/5.0049281
![]() |
[28] |
Y. Deng, Z. Jia, F. Yang, Synchronizability of multilayer star and star-ring networks, Discrete Dyn. Nat. Soc., 2020 (2020), 9143917. https://doi.org/10.1155/2020/9143917 doi: 10.1155/2020/9143917
![]() |
[29] |
P. Peng, J. Wang, K. Huang, Reliable fiber sensor system with star-ring-bus architecture, Sensors, 10 (2010), 4194–4205. https://doi.org/10.3390/s100504194 doi: 10.3390/s100504194
![]() |
[30] |
C. Christodoulou, G. Ellinas, Resilient architecture for optical access networks, Photonic Network Commun., 41 (2021), 1–16. https://doi.org/10.1007/s11107-020-00910-y doi: 10.1007/s11107-020-00910-y
![]() |
[31] |
S. Liu, L. Chen, Second-order terminal sliding mode control for networks synchronization, Nonlinear Dyn., 79 (2015), 205–213. https://doi.org/10.1007/s11071-014-1657-x doi: 10.1007/s11071-014-1657-x
![]() |
[32] |
R. Li, H. Wu, J. Cao, Exponential synchronization for variable-order fractional discontinuous complex dynamical networks with short memory via impulsive control, Neural Networks, 148 (2022), 13–22. https://doi.org/10.1016/j.neunet.2021.12.021 doi: 10.1016/j.neunet.2021.12.021
![]() |
[33] |
Y. Tang, F. Qian, H. Gao, J. Kurths, Synchronization in complex networks and its application–a survey of recent advances and challenges, Annu. Rev. Control, 38 (2014), 184–198. https://doi.org/10.1016/j.arcontrol.2014.09.003 doi: 10.1016/j.arcontrol.2014.09.003
![]() |
[34] |
Y. Shi, Y. Ma, Finite/fixed-time synchronization for complex networks via quantized adaptive control, Electron. Res. Arch., 29 (2021), 2047–2061. https://doi.org/10.3934/era.2020104 doi: 10.3934/era.2020104
![]() |
[35] |
Z. Qin, J. Wang, Y. Huang, S. Ren, Analysis and adaptive control for robust synchronization and H∞ synchronization of complex dynamical networks with multiple time-delays, Neurocomputing, 289 (2018), 241–251. https://doi.org/10.1016/j.neucom.2018.02.031 doi: 10.1016/j.neucom.2018.02.031
![]() |
[36] |
A. Zentani, N. Zulkifli, A. Ramli, Network resiliency and fiber usage of Tree, Star, ring and wheel based wavelength division multiplexed passive optical network Topologies: a comparative review, Opt. Fiber Technol., 73 (2022), 103038. https://doi.org/10.1016/j.yofte.2022.103038 doi: 10.1016/j.yofte.2022.103038
![]() |
[37] |
M. Xu, K. An, L. H. Vu, Z. Ye, J. Feng, E. Chen, Optimizing multi-agent based urban traffic signal control system, J. Intell. Transp. Syst., 23 (2019), 357–369. https://doi.org/10.1080/15472450.2018.1501273 doi: 10.1080/15472450.2018.1501273
![]() |
[38] |
L. Xing, Cascading failures in internet of things: review and perspectives on reliability and resilience, IEEE Internet Things J., 8 (2020), 44–64. https://doi.org/10.1109/JIOT.2020.3018687 doi: 10.1109/JIOT.2020.3018687
![]() |
[39] |
J. Wei, X. Wu, J. Lu, X. Wei, Synchronizability of duplex regular networks, EuroPhys. Lett., 120 (2018), 20005. https://doi.org/10.1209/0295-5075/120/20005 doi: 10.1209/0295-5075/120/20005
![]() |
[40] |
L. Tang, X. Wu, J. Lü, J. Lu, R. M. D'Souza, Master stability functions for complete, intralayer, and interlayer synchronization in multiplex networks of coupled Rössler oscillators, Phys. Rev. E: Stat. Nonlinear Soft Matter Phys., 99 (2019), 012304. https://doi.org/10.1103/PhysRevE.99.012304 doi: 10.1103/PhysRevE.99.012304
![]() |
[41] |
L. M. Pecora, T. L. Carroll, Master stability functions for synchronized coupled systems, Phys. Rev. Lett., 80 (1998), 2109. https://doi.org/10.1103/PhysRevLett.80.2109 doi: 10.1103/PhysRevLett.80.2109
![]() |
[42] |
J. Sun, X. Li, J. Zhang, Y. Shen, Y. Li, Synchronizability and eigenvalues of multilayer star networks through unidirectionally coupling (in Chinese), Acta Phys. Sin., 66 (2017), 188901. https://doi.org/10.7498/aps.66.188901 doi: 10.7498/aps.66.188901
![]() |
[43] |
X. Jin, Z. Wang, H. Yang, Q. Song, M. Xiao, Synchronization of multiplex networks with stochastic perturbations via pinning adaptive control, J. Franklin Inst., 358 (2021), 3994–4012. https://doi.org/10.1016/j.jfranklin.2021.03.004 doi: 10.1016/j.jfranklin.2021.03.004
![]() |
[44] |
X. Mao, A note on the LaSalle-type theorems for stochastic differential delay equations, J. Math. Anal. Appl., 268 (2002), 125–142. https://doi.org/10.1006/jmaa.2001.7803 doi: 10.1006/jmaa.2001.7803
![]() |
[45] |
F. Yang, Z. Jia, Y. Deng, Eigenvalue spectrum and synchronizability of two types of double-layer star-ring networks with hybrid directional coupling, Discrete Dyn. Nat. Soc., 2021 (2021), 1–20. https://doi.org/10.1155/2021/6623648 doi: 10.1155/2021/6623648
![]() |
[46] |
J. L. Hindmarsh, R. M. Rose, A model of the nerve impulse using two first-order differential equations, Nature, 296 (1982), 162–164. https://doi.org/10.1038/296162a0 doi: 10.1038/296162a0
![]() |
[47] |
L. Xu, G. Qi, J. Ma, Modeling of memristor-based Hindmarsh-Rose neuron and its dynamical analyses using energy method, Appl. Math. Modell., 101 (2022), 503–516. https://doi.org/10.1016/j.apm.2021.09.003 doi: 10.1016/j.apm.2021.09.003
![]() |
[48] |
L. Shi, C. Zhang, S. Zhong, Synchronization of singular complex networks with time-varying delay via pinning control and linear feedback control, Chaos, Solitons Fractals, 145 (2021), 110805. https://doi.org/10.1016/j.chaos.2021.110805. doi: 10.1016/j.chaos.2021.110805
![]() |
[49] |
Y. Deng, Z. Jia, G. Deng, Q. Zhang, Eigenvalue spectrum and synchronizability of multiplex chain networks, Physica A, 537 (2020), 122631. https://doi.org/10.1016/j.physa.2019.122631 doi: 10.1016/j.physa.2019.122631
![]() |
[50] |
Y. Li, X. Wu, J. Lu, J. Lü, Synchronizability of duplex networks, IEEE Trans. Circuits Syst. Ⅱ Express Briefs, 63 (2015), 206–210. https://doi.org/10.1109/TCSⅡ.2015.2468924 doi: 10.1109/TCSⅡ.2015.2468924
![]() |