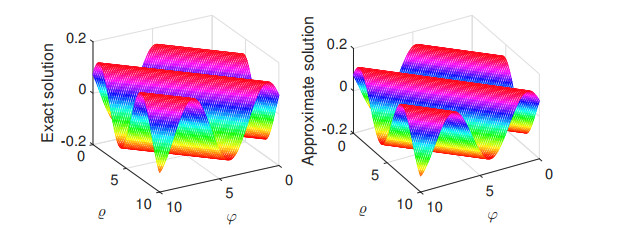
Finite-time synchronization is a critical problem in the study of neural networks. The primary objective of this study was to construct feedback controllers for various models based on fuzzy shunting inhibitory cellular neural networks (FSICNNs) and find out the sufficient conditions for the solutions of those systems to reach synchronization in finite time. In particular, by imposing global assumptions of Lipschitz continuous and bounded activation functions, we prove the existence of finite-time synchronization for three basic FSICNN models that have not been studied before. Moreover, we suggest both controllers and Lyapunov functions that would yield a feasible convergence time between solutions that takes into account the chosen initial conditions. In general, we consecutively explore models of regular delayed FSICNNs and then consider them in the presence of either inertial or diffusion terms. Using criteria derived by means of the maximum-value approach in its different forms, we give an upper bound of the time up to which synchronization is guaranteed to occur in all three FSICNN models. These results are supported by 2D and 3D computer simulations and two respective numerical examples for 2×2 and 2×3 cases, which show the behavior of the solutions and errors under different initial conditions of FSICNNs in the presence and absence of designed controllers.
Citation: Zhangir Nuriyev, Alfarabi Issakhanov, Jürgen Kurths, Ardak Kashkynbayev. Finite-time synchronization for fuzzy shunting inhibitory cellular neural networks[J]. AIMS Mathematics, 2024, 9(5): 12751-12777. doi: 10.3934/math.2024623
[1] | Aslı Alkan, Halil Anaç . A new study on the Newell-Whitehead-Segel equation with Caputo-Fabrizio fractional derivative. AIMS Mathematics, 2024, 9(10): 27979-27997. doi: 10.3934/math.20241358 |
[2] | Qasim Khan, Anthony Suen, Hassan Khan, Poom Kumam . Comparative analysis of fractional dynamical systems with various operators. AIMS Mathematics, 2023, 8(6): 13943-13983. doi: 10.3934/math.2023714 |
[3] | Manal Alqhtani, Khaled M. Saad, Rasool Shah, Thongchai Botmart, Waleed M. Hamanah . Evaluation of fractional-order equal width equations with the exponential-decay kernel. AIMS Mathematics, 2022, 7(9): 17236-17251. doi: 10.3934/math.2022949 |
[4] | Aslı Alkan, Halil Anaç . The novel numerical solutions for time-fractional Fornberg-Whitham equation by using fractional natural transform decomposition method. AIMS Mathematics, 2024, 9(9): 25333-25359. doi: 10.3934/math.20241237 |
[5] | Naveed Iqbal, Saleh Alshammari, Thongchai Botmart . Evaluation of regularized long-wave equation via Caputo and Caputo-Fabrizio fractional derivatives. AIMS Mathematics, 2022, 7(11): 20401-20419. doi: 10.3934/math.20221118 |
[6] | Azzh Saad Alshehry, Naila Amir, Naveed Iqbal, Rasool Shah, Kamsing Nonlaopon . On the solution of nonlinear fractional-order shock wave equation via analytical method. AIMS Mathematics, 2022, 7(10): 19325-19343. doi: 10.3934/math.20221061 |
[7] | Zeliha Korpinar, Mustafa Inc, Dumitru Baleanu . On the fractional model of Fokker-Planck equations with two different operator. AIMS Mathematics, 2020, 5(1): 236-248. doi: 10.3934/math.2020015 |
[8] | Emad Salah, Ahmad Qazza, Rania Saadeh, Ahmad El-Ajou . A hybrid analytical technique for solving multi-dimensional time-fractional Navier-Stokes system. AIMS Mathematics, 2023, 8(1): 1713-1736. doi: 10.3934/math.2023088 |
[9] | Yudhveer Singh, Devendra Kumar, Kanak Modi, Vinod Gill . A new approach to solve Cattaneo-Hristov diffusion model and fractional diffusion equations with Hilfer-Prabhakar derivative. AIMS Mathematics, 2020, 5(2): 843-855. doi: 10.3934/math.2020057 |
[10] | Yousef Jawarneh, Humaira Yasmin, M. Mossa Al-Sawalha, Rasool Shah, Asfandyar Khan . Fractional comparative analysis of Camassa-Holm and Degasperis-Procesi equations. AIMS Mathematics, 2023, 8(11): 25845-25862. doi: 10.3934/math.20231318 |
Finite-time synchronization is a critical problem in the study of neural networks. The primary objective of this study was to construct feedback controllers for various models based on fuzzy shunting inhibitory cellular neural networks (FSICNNs) and find out the sufficient conditions for the solutions of those systems to reach synchronization in finite time. In particular, by imposing global assumptions of Lipschitz continuous and bounded activation functions, we prove the existence of finite-time synchronization for three basic FSICNN models that have not been studied before. Moreover, we suggest both controllers and Lyapunov functions that would yield a feasible convergence time between solutions that takes into account the chosen initial conditions. In general, we consecutively explore models of regular delayed FSICNNs and then consider them in the presence of either inertial or diffusion terms. Using criteria derived by means of the maximum-value approach in its different forms, we give an upper bound of the time up to which synchronization is guaranteed to occur in all three FSICNN models. These results are supported by 2D and 3D computer simulations and two respective numerical examples for 2×2 and 2×3 cases, which show the behavior of the solutions and errors under different initial conditions of FSICNNs in the presence and absence of designed controllers.
Fractional calculus (FC) is a discipline of mathematics concerned with the study of derivatives and integrals of non-integer orders. It was invented in September 1695 by L'Hospital. In a letter to L'-Hospital [1], who discussed the differentiation of product functions of order 12, which laid the groundwork for FC [2,3,4]. It provides a great tool for characterizing memory and inherited qualities of different materials and procedures [4,5,6]. FC has grown in interest in recent decades as a result of the intensive development of fractional calculus theory and its applications in diverse sectors of science and engineering due to its high precision and applicability, for example, fractional control theory, image processing, signal processing, bio-engineering, groundwater problems, heat conduction, and behavior of viscoelastic and visco-plastic materials, see [7,8,9]. In addition, the electrical RLC circuit's performance has been determined using the fractional model [10].
In the last few decades, numerical and analytical solutions of fractional partial differential equations (FPDEs) have drawn a lot of attention among researchers [11,12,13,14,15]. The qualitative behavior of these mathematical models is significantly influenced by the fractional derivatives that are employed in FPDEs. This has numerous applications in the fields of solid-state physics, plasma physics, mathematical biology, electrochemistry, diffusion processes, turbulent flow, and materials science [16,17,18].
However, solving PDEs is not an easy task. A lot of mathematicians have put their effort into formulating analytical and numerical methods to solve fractional partial differential equations. The widely recognized methods for the solution of (FPDEs) are the Adomian decomposition method [19], homotopy analysis method [20,21], q-homotopy analysis transform method [22], homotopy perturbation method [23], variation iteration method [24], differential transform method [25], projected differential transform method [26], meshless method [27], backlund transformation method [28], Haar wavelet method [29], G'/G expansion method [30], residual power series method [31], Adam Bashforth's moulton technique [32], operational matrix method [33].
The nonlinear partial differential Navier-Stokes (N-S) equation, which expresses viscous fluid motion, was first developed by Claude Louis and Gabriel Stokes in 1822 [34]. This equation describes the conservation of mass and conservation of momentum for Newtonian and is referred to as the Newton's second law for fluids. The N-S equation has wide applications in engineering science, for example, examining liquid flow, studying wind current around wings, climate estimation, and blood flow [35,36]. Furthermore, along with Maxwell's equations the (N-S) equation can be applied to study and model magnetohydrodynamics, plasma physics, geophysics, etc. Also, fluid-solid interaction problems have been modeled and investigated by the N-S equation [37].
The multi-dimensional Navier-Stokes equation (MDNSE) stands as a fundamental cornerstone in fluid dynamics, providing a comprehensive mathematical framework to describe the motion of fluid substances in multiple dimensions. Derived from the Navier-Stokes equation, which govern the conservation of momentum for incompressible fluids, the MDNSE extends these principles to encompass the complexities of fluid flow in more than one spatial dimension. The equation accounts for the conservation of mass and the interplay of viscous and inertial forces, offering a powerful tool to model and analyze fluid behavior in diverse physical scenarios. The application of the multi-dimensional Navier-Stokes equation spans a wide range of scientific and engineering disciplines, playing a crucial role in understanding fluid dynamics across various contexts. In the field of aerospace engineering, MDNSE is employed to simulate the airflow around aircraft, aiding in the design and optimization of aerodynamic profiles. In marine engineering, it finds application in predicting the behavior of water currents around ships and offshore structures. Additionally, MDNSE is instrumental in weather modeling, allowing meteorologists to simulate and analyze atmospheric conditions in multiple dimensions for more accurate weather predictions. In the realm of biomedical engineering, it contributes to the study of blood flow in arteries and the behavior of biological fluids. Overall, the multi-dimensional Navier-Stokes equation serves as a versatile and indispensable tool for gaining insights into the intricate dynamics of fluid motion in diverse scientific and engineering.
In literature, many researchers have used numerous techniques to analyze the N-S equation. First of all, the authors of [38] solved the fractional-order N-S equation by using the Laplace transform, Fourier sine transform, and Hankel transform. The authors of [39,40,41] investigated the time-fractional N-S equation by using the homotopy perturbation method. Biraider [42] used the Adomian decomposition method to find a numerical solution. Recently, many researchers have focused on examining the multi-dimensional time-fractional N-S equation, by combining a variety of techniques with different transforms, see [43,44,45,46].
Motivated by the mentioned work, in the present article the new iterative transform method (NITM) and homotopy perturbation transform method (HPTM) combined with natural transform are implemented to analyze the solution of the time-fractional multi-dimensional Navier-Stokes equation in the sense of Caputo-Fabrizio operator. The article is structured in the following way: In Section 2, some basic definitions and properties are explained. In Section 3, the interpretation of the NITM is explained for the solution of fractional PDEs. In Section 4, the above-mentioned method's convergence analysis is also presented. In Section 5, the outcome of the suggested method is illustrated by examples, and validated graphically. In Section 6, the HPTM is explicated. In Section 7, similar examples are presented to elucidate the HPTM.
Definition 1 ([47]). The Caputo fractional derivative of f(ℓ) is defined as
C0Dθℓf(ℓ)={1Γ(m−θ)∫ℓ0(ℓ−ζ)m−θ−1fm(ζ)dζ,m−1<θ<m,fm(ℓ),θ=m. | (2.1) |
where, m∈Z+,θ∈R+.
Definition 2 ([48]). The Caputo-Fabrizio fractional derivative of f(ℓ) is defined as
CF0Dθℓf(ℓ)=(2−θ)B(θ)2(1−θ)∫ℓ0exp(−θ(ℓ−ζ)1−θ)D(f(ζ))dζℓ≥0. | (2.2) |
where θ∈[0,1], and B(θ) is a normalization function and satisfies the condition B(0)=B(1)=1.
Definition 3 ([49]). The fractional integral of function f(ℓ) of order θ, is defined as
CF0Iθℓf(ℓ)=2(1−θ)(2−θ)B(θ)f(ℓ)+2θ(2−θ)B(θ)∫ℓ0f(ζ)dζ,ℓ≥0. | (2.3) |
From Eq (2.3), the following results hold:
2(1−θ)(2−θ)B(θ)+2θ(2−θ)B(θ)=1, |
which gives,
B(θ)=22−θ,0≤θ≤1. |
Thus, Losada and Nieto [49] redefined the Caputo-Fabrizio fractional derivative as
CF0Dθℓf(ℓ)=11−θ∫ℓ0exp(−θ(ℓ−ζ)1−θ)D(f(ζ))dζℓ≥0. | (2.4) |
Definition 4 ([50]). The natural transform of ℧(ℓ) is given by
N(℧(ℓ))=U(s,v)=∫∞−∞e−sℓ℧(vℓ)dℓ,s,v∈(−∞,∞). | (2.5) |
For ℓ∈(0,∞), the natural transform of ℧(ℓ) is given by
N(℧(ℓ)H(ℓ))=N+=U+(s,v)=∫∞0e−sℓ℧(vℓ)dℓs,v∈(0,∞), | (2.6) |
where H is the Heaviside function.
The inverse of natural transform of U(s,v) is defined as
N−1[U(s,v)]=℧(ℓ),∀ℓ>0. |
Definition 5 ([51]). The natural transform of the fractional Caputo differential operator C0Dθℓ℧(ℓ) is defined as
N[C0Dθℓ℧(ℓ)]=(1s)θ(N[℧(ℓ)]−(1s)℧(0)). | (2.7) |
Definition 6 ([52]). The natural transform of the fractional Caputo-Fabrizio differential operator CF0Dθℓ℧(ℓ) is defined as
N[CF0Dθℓ℧(ℓ)]=11−θ+θ(vs)(N[℧(ℓ)]−(1s)℧(0)). | (2.8) |
This section considers, NITM with the CF fractional derivative operator in order to evaluate the multi-dimensional (N-S) problem. This iterative method is a combination of the new iterative method introduced in [53] and the natural transform [50].
Consider the fractional PDE of the form
CF0Dθℓ℧(φ,ϱ,ℓ)+R(℧(φ,ϱ,ℓ))+N(℧(φ,ϱ,ℓ))−P(φ,ϱ,ℓ)=0, | (3.1) |
with respect to the initial condition
℧(φ,ϱ,0)=h(φ,ϱ). | (3.2) |
CF0Dθℓ is the Caputo-Fabrizio fractional differential operator of order θ,R and N are linear and non-linear terms, and P is the source term.
By employing the natural transform on both sides of Eq (3.1), we get
N[CF0Dθℓ℧(φ,ϱ,ℓ)+R(℧(φ,ϱ,ℓ))+N(℧(φ,ϱ,ℓ))−P(φ,ϱ,ℓ)=0], | (3.3) |
N[℧(φ,ϱ,ℓ)]=s−1℧(φ,ϱ,0)+(1−θ+θ(vs))N{P(φ,ϱ,ℓ)−[R(℧(φ,ϱ,ℓ))+N(℧(φ,ϱ,ℓ))]}. | (3.4) |
By using the inverse natural transform, Eq (3.4) can reduced to the form
℧(φ,ϱ,ℓ)=N−1{s−1℧(φ,ϱ,0)+(1−θ+θ(vs))N{P(φ,ϱ,ℓ)−[R(℧(φ,ϱ,ℓ))+N(℧(φ,ϱ,ℓ))]}}. | (3.5) |
The nonlinear operator N as in [53], can be decomposed as
N(℧(φ,ϱ,ℓ))=N(∞∑r=0℧r(φ,ϱ,ℓ))=N(℧0(φ,ϱ,ℓ))+∞∑r=1{N(r∑i=0℧i(φ,ϱ,ℓ))−N(r−1∑i=0℧i(φ,ϱ,ℓ))}. | (3.6) |
Now, define an mth-order approximate series
D(m)(φ,ϱ,ℓ)=m∑r=0℧r(φ,ϱ,ℓ)=℧0(φ,ϱ,ℓ)+℧1(φ,ϱ,ℓ)+℧2(φ,ϱ,ℓ)+–−+℧m(φ,ϱ,ℓ),m∈N. | (3.7) |
Consider the solution of Eq (3.1) in a series form as
℧(φ,ϱ,ℓ)=limm⟶∞D(m)(φ,ϱ,ℓ)=∞∑r=0℧r(φ,ϱ,ℓ). | (3.8) |
By substituting Eqs (3.6) and (3.7) into Eq (3.5), we get
∞∑r=0℧r(φ,ϱ,ℓ)=N−1{s−1℧(φ,ϱ,0)+(1−θ+θ(vs))N[P(φ,ϱ,ℓ)−[R(℧0(φ,ϱ,ℓ))+N(℧0(φ,ϱ,ℓ))]]}−N−1{(1−θ+θ(vs))N[∞∑r=1{R(℧r(φ,ϱ,ℓ))+[N(r∑i=0℧i(φ,ϱ,ℓ))−N(r−1∑i=0℧i(φ,ϱ,ℓ))]}]}. | (3.9) |
From Eq (3.9), the following iterations are obtained.
℧0(φ,ϱ,ℓ)=N−1[s−1℧(φ,ϱ,0)+(1−θ+θ(vs))N[P(φ,ϱ,ℓ)]], | (3.10) |
℧1(φ,ϱ,ℓ)=−N−1[(1−θ+θ(vs))N[R(℧0(φ,ϱ,ℓ))+N(℧0(φ,ϱ,ℓ))]],⋮ | (3.11) |
ur+1(φ,ϱ,ℓ)=−N−1{(1−θ+θ(vs))N[∞∑r=1{R(℧r(φ,ϱ,ℓ))+[N(r∑i=0℧i(φ,ϱ,ℓ))−N(r−1∑i=0℧i(φ,ϱ,ℓ))]}]}. | (3.12) |
In this section, we demonstrate the uniqueness and convergence of the NITMCF.
Theorem 1. The solution derived with the aid of the NITMCF of Eq (3.1) is unique whenever 0<(℘1,℘2)[1−θ+θℓ]<1.
Proof. Let X=(C[J],∥.∥) be the Banach space for all continuous functions over the interval J=[0,T], with the norm ∥ϕ(ℓ)=maxℓ∈J|ϕ(ℓ)|.
Define the mapping F:X→X, where
℧Cr+1=℧C0−N−1[(1−θ+θvs)N{R(℧(φ,ϱ,ℓ))+N(℧(φ,ϱ,ℓ))−P(φ,ϱ,ℓ)}],r≥0. |
Now, assume that R(℧) and N(℧) satisfy the Lipschitz conditions with Lipschitz constants ℘1,℘2 and |R(℧)−R(ˉ℧)|<℘1|℧−ˉ℧|, |N(℧)−N(ˉ℧)|<℘2|℧−ˉ℧|, where ℧=℧(φ,ϱ,ℓ) and ˉ℧=℧(φ,ϱ,ℓ) are the values of two distinct functions.
∥F(℧)−F(ˉ℧)∥≤maxℓ∈J|N−1[(1−θ+θvs)N{R(℧(φ,ϱ,ℓ))−R(ˉ℧(φ,ϱ,ℓ))}+(1−θ+θvs)N{N(℧(φ,ϱ,ℓ))−N(ˉ℧(φ,ϱ,ℓ))}]|≤maxℓ∈J[℘1N−1{(1−θ+θvs)N|℧(φ,ϱ,ℓ)−ˉ℧(φ,ϱ,ℓ)|}+℘2N−1{(1−θ+θvs)N|℧(φ,ϱ,ℓ)−ˉ℧(φ,ϱ,ℓ)|}]≤maxℓ∈J(℘1+℘2)[N−1{(1−θ+θvs)N|℧(φ,ϱ,ℓ)−ˉ℧(φ,ϱ,ℓ)|}]≤(℘1+℘2)[N−1{(1−θ+θvs)N|℧(φ,ϱ,ℓ)−ˉ℧(φ,ϱ,ℓ)|}]≤(℘1+℘2)[1−θ+θℓ]∥℧−ˉ℧∥. |
F is contraction as 0<(℘1+℘2)[1−θ+θℓ]<1. Thus, the result of (3.1) is unique with the aid of the Banach fixed-point theorem.
Theorem 2. The solution derived from Eq (3.1) using the NITMCF converges if 0<℧<1 and ∥℧i∥<∞, where ℧=(℘1+℘2)[1−θ+θℓ].
Proof. Let ℧n=∑nr=0℧r(φ,ϱ,ℓ) be a partial sum of series. To prove that {℧n} is a Cauchy sequence in the Banach space X, we consider
∥(℧m−℧n∥=maxℓ∈J|m∑r=n+1℧r(φ,ϱ,ℓ)|,n=1,2,3,...≤maxℓ∈J|N−1[(1−θ+θvs)N{m∑r=n+1[R(℧r−1(φ,ϱ,ℓ))+N(℧r−1(φ,ϱ,ℓ))]}]|≤maxℓ∈J|N−1[(1−θ+θvs)N{R(℧m−1)−R(℧n−1)+N(℧m−1)−N(℧n−1)}]| |
≤℘1maxℓ∈J|N−1[(1−θ+θvs)N{R(℧m−1)−R(℧n−1)}]|+℘2maxℓ∈J|N−1[(1−θ+θvs)N{N(℧m−1)−N℧n−1)}]|=(℘1+℘2)[1−θ+θℓ]∥℧m−1−℧n−1∥. |
If m=n+1, then
∥℧n+1−℧n∥≤℘∥℧n−℧n−1∥≤℘2∥℧n−1−℧n−2∥≤...≤℘n∥℧1−℧0∥, |
where ℘=(℘1+℘2)[1−θ+θℓ]. In a similar way
∥℧m−℧n∥≤∥℧n+1−℧n∥≤∥℧n+2−℧n+1∥≤...∥℧m−℧m−1∥,≤(℘n+℘n+1+...+℘m−1)∥℧1−℧0∥,≤℘n(1−℘m−n1−℘)∥℧1∥. |
We see that, 1−℘m−n<1, as 0<℘<1. Thus,
∥℧m−℧n∥≤(℘n1−℘)maxℓ∈J∥℧1∥. |
Since ∥℧1∥<∞, ∥℧m−℧n∥→0 as n→∞. Hence, ℧m is a Cauchy sequence in X. So, the series ℧m is convergent.
In this section, we demonstrate the effectiveness of the NITM with the natural transformation for the Caputo-Fabrizio fractional derivative to solve the two-dimensional fractional N-S equation.
Consider the two-dimensional fractional N-S equation
CF0Dθℓ(μ)+μ∂μ∂φ+ν∂μ∂ϱ=ρ[∂2μ∂φ2+∂2μ∂ϱ2]+q,CF0Dθℓ(ν)+μ∂ν∂φ+ν∂ν∂ϱ=ρ[∂2ν∂φ2+∂2ν∂ϱ2]−q, | (5.1) |
with initial conditions
{μ(φ,ϱ,0)=−sin(φ+ϱ),ν(φ,ϱ,0)=sin(φ+ϱ). | (5.2) |
From Eqs (5.1) and (5.2), we set the following
{P1(φ,ϱ,ℓ)=q,R(μ(φ,ϱ,ℓ))=−ρ[∂2μ∂φ2+∂2μ∂ϱ2],N(μ(φ,ϱ,ℓ))=μ∂μ∂φ+ν∂μ∂ϱ,P2(φ,ϱ,ℓ)=−q,R(ν(φ,ϱ,ℓ))=−ρ[∂2ν∂φ2+∂2ν∂ϱ2],N(ν(φ,ϱ,ℓ))=μ∂ν∂φ+ν∂ν∂ϱ,μ0(φ,ϱ,0)=−sin(φ+ϱ),ν0(φ,ϱ,0)=sin(φ+ϱ). |
Using the iteration process outlined in Section 3, we have
μ0(φ,ϱ,ℓ)=N−1[s−1μ(φ,ϱ,0)+(1−θ+θ(vs))N[P1(φ,ϱ,ℓ)]],0<θ≤1=−sin(φ+ϱ)+q.[(1−θ)+θℓ],ν0(φ,ϱ,ℓ)=N−1[s−1ν(φ,ϱ,0)+(1−θ+θ(vs))N[P2(φ,ϱ,ℓ)]]=sin(φ+ϱ)−q.[(1−θ)+θℓ], | (5.3) |
μ1(φ,ϱ,ℓ)=−N−1[(1−θ+θ(vs))N{R(μ0(φ,ϱ,ℓ))+N(μ0(φ,ϱ,ℓ))}]=−N−1[(1−θ+θ(vs))N(−ρ[∂2μ0∂φ2+∂2μ0∂ϱ2]+μ0∂μ0∂φ+ν0∂μ0∂ϱ)]=2ρsin(φ+ϱ)[(1−θ)+θℓ],ν1(φ,ϱ,ℓ)=−N−1[(1−θ+θ(vs))N(R(ν0(φ,ϱ,ℓ))+N(ν0(φ,ϱ,ℓ)))]=−N−1[(1−θ+θ(vs))N(−ρ[∂2ν0∂φ2+∂2ν0∂ϱ2]+μ0∂ν0∂φ+ν0∂ν0∂ϱ)]=−2ρsin(φ+ϱ)[(1−θ)+θℓ], | (5.4) |
μ2(φ,ϱ,ℓ)=−N−1[(1−θ+θ(vs))N[(R(μ1(φ,ϱ,ℓ))+{N(μ0(φ,ϱ,ℓ)+μ1(φ,ϱ,ℓ))−N(μ0(φ,ϱ,ℓ))}]]=−N−1[(1−θ+θ(vs))N(−ρ[∂2μ1∂φ2+∂2μ1∂ϱ2]+(μ0+μ1)∂(μ0+μ1)∂φ+(ν0+ν1)∂(μ0+μ1)∂ϱ−μ0∂μ0∂φ−ν0∂μ0∂ϱ)]=−(2ρ)2sin(φ+ϱ)[(1−θ)2+2θ(1−θ)ℓ+θ2ℓ22!],ν2(φ,ϱ,ℓ)=−N−1[(1−θ+θ(vs))N[(R(ν1(φ,ϱ,ℓ))+{N(ν0(φ,ϱ,ℓ)+ν1(φ,ϱ,ℓ))−N(ν0(φ,ϱ,ℓ))}]]=−N−1[(1−θ+θ(vs))N(−ρ[∂2ν1∂φ2+∂2ν1∂ϱ2]+(μ0+μ1)∂(ν0+ν1)∂φ+(ν0+ν1)∂(ν0+ν1)∂ϱ−μ0∂ν0∂φ−ν0∂ν0∂ϱ)]=(2ρ)2sin(φ+ϱ)[(1−θ)2+2θ(1−θ)ℓ+θ2ℓ22!], | (5.5) |
μ3(φ,ϱ,ℓ)=−N−1[(1−θ+θ(vs))N[(R(μ2(φ,ϱ,ℓ))+{N(μ0(φ,ϱ,ℓ)+μ1(φ,ϱ,ℓ)+μ2(φ,ϱ,ℓ))−N(μ0(φ,ϱ,ℓ)+μ1(φ,ϱ,ℓ))}]]=−N−1[(1−θ+θ(vs))N(−ρ[∂2μ2∂φ2+∂2μ2∂ϱ2]+(μ0+μ1+μ2)∂(μ0+μ1+μ2)∂φ+(ν0+ν1+ν2)∂(μ0+μ1+μ2)∂ϱ−(μ0+μ1)∂(μ0+μ1)∂φ−(ν0+ν1)∂(μ0+μ1)∂ϱ)]=(2ρ)3sin(φ+ϱ)[(1−θ)3+3θ(1−θ)2ℓ+3θ2(1−θ)ℓ22!+θ3ℓ33!], | (5.6) |
ν3(φ,ϱ,ℓ)=−N−1[(1−θ+θ(vs))N[(R(ν2(φ,ϱ,ℓ))+{N(ν0(φ,ϱ,ℓ)+ν1(φ,ϱ,ℓ)+ν2(φ,ϱ,ℓ))−N(ν0(φ,ϱ,ℓ)+ν1(φ,ϱ,ℓ))}]]=−N−1[(1−θ+θ(vs))N(−ρ[∂2ν2∂φ2+∂2ν2∂ϱ2]+(μ0+μ1+μ2)∂(ν0+ν1+ν2)∂φ+(ν0+ν1+ν2)∂(ν0+ν1+ν2)∂ϱ−(μ0+μ1)∂(ν0+ν1)∂φ−(ν0+ν1)∂(ν0+ν1)∂ϱ)]=−(2ρ)3sin(φ+ϱ)[(1−θ)3+3θ(1−θ)2ℓ+3θ2(1−θ)ℓ22!+θ3ℓ33!],⋮ | (5.7) |
In a general way,
μ(φ,ϱ,ℓ)=∞∑r=0μr(φ,ϱ,ℓ)=μ0(φ,ϱ,ℓ)+μ1(φ,ϱ,ℓ)+μ2(φ,ϱ,ℓ)+⋯.ν(φ,ϱ,ℓ)=∞∑r=0νr(φ,ϱ,ℓ)=ν0(φ,ϱ,ℓ)+ν1(φ,ϱ,ℓ)+ν2(φ,ϱ,ℓ)+⋯. |
With the addition of all μ and ν,
μ(φ,ϱ,ℓ)=−sin(φ+ϱ)+q.[(1−θ)+θℓ]+2ρsin(φ+ϱ)[(1−θ)+θℓ]−(2ρ)2sin(φ+ϱ)[(1−θ)2+2θ(1−θ)ℓ+θ2ℓ22!]+(2ρ)3sin(φ+ϱ)×[(1−θ)3+3θ(1−θ)2ℓ+3θ2(1−θ)ℓ22!+θ3ℓ33!]−⋯, |
ν(φ,ϱ,ℓ)=sin(φ+ϱ)−q.[(1−θ)+θℓ]−2ρsin(φ+ϱ)[(1−θ)+θℓ]+(2ρ)2sin(φ+ϱ)[(1−θ)2+2θ(1−θ)ℓ+θ2ℓ22!]−(2ρ)3sin(φ+ϱ)×[(1−θ)3+3θ(1−θ)2ℓ+3θ2(1−θ)ℓ22!+θ3ℓ33!]+⋯. |
The exact solution of Eq (5.1) at θ=1 and q=0 is given by
μ(φ,ϱ,ℓ)=−e−2ρℓsin(φ+ϱ),ν(φ,ϱ,ℓ)=e−2ρℓsin(φ+ϱ). | (5.8) |
Consider the two-dimensional fractional N-S equation
CF0Dθℓ(μ)+μ∂μ∂φ+ν∂μ∂ϱ=ρ[∂2μ∂φ2+∂2μ∂ϱ2]+q,CF0Dθℓ(ν)+μ∂ν∂φ+ν∂ν∂ϱ=ρ[∂2ν∂φ2+∂2ν∂ϱ2]−q, | (5.9) |
with the initial conditions
{μ(φ,ϱ,0)=−e(φ+ϱ),ν(φ,ϱ,0)=e(φ+ϱ). | (5.10) |
From Eqs (5.9) and (5.10), we set the following:
{P1(φ,ϱ,ℓ)=q,R(μ(φ,ϱ,ℓ))=−ρ[∂2μ∂φ2+∂2μ∂ϱ2],N(μ(φ,ϱ,ℓ))=μ∂μ∂φ+ν∂μ∂ϱ,P2(φ,ϱ,ℓ)=−q,R(ν(φ,ϱ,ℓ))=−ρ[∂2ν∂φ2+∂2ν∂ϱ2],N(ν(φ,ϱ,ℓ))=μ∂ν∂φ+ν∂ν∂ϱ,μ0(φ,ϱ,0)=−e(φ+ϱ),ν0(φ,ϱ,0)=e(φ+ϱ). |
Using the iteration process outlined in Section 3, we have
μ0(φ,ϱ,ℓ)=N−1[s−1μ(φ,ϱ,0)+(1−θ+θ(vs))N[P1(φ,ϱ,ℓ)]],0<θ≤1=−e(φ+ϱ)+q.[(1−θ)+θℓ],ν0(φ,ϱ,ℓ)=N−1[s−1ν(φ,ϱ,0)+(1−θ+θ(vs))N[P2(φ,ϱ,ℓ)]]=e(φ+ϱ)−q.[(1−θ)+θℓ], | (5.11) |
μ1(φ,ϱ,ℓ)=−N−1[(1−θ+θ(vs))N{R(μ0(φ,ϱ,ℓ))+N(μ0(φ,ϱ,ℓ))}]=−N−1[(1−θ+θ(vs))N(−ρ[∂2μ0∂φ2+∂2μ0∂ϱ2]+μ0∂μ0∂φ+ν0∂μ0∂ϱ)]=−2ρe(φ+ϱ)[(1−θ)+θℓ],ν1(φ,ϱ,ℓ)=−N−1[(1−θ+θ(vs))N(R(ν0(φ,ϱ,ℓ))+N(ν0(φ,ϱ,ℓ)))]=−N−1[(1−θ+θ(vs))N(−ρ[∂2ν0∂φ2+∂2ν0∂ϱ2]+μ0∂ν0∂φ+ν0∂ν0∂ϱ)]=2ρe(φ+ϱ)[(1−θ)+θℓ],μ2(φ,ϱ,ℓ)=−N−1[(1−θ+θ(vs))N[(R(μ1(φ,ϱ,ℓ))+{N(μ0(φ,ϱ,ℓ)+μ1(φ,ϱ,ℓ))−N(μ0(φ,ϱ,ℓ))}]]=−N−1[(1−θ+θ(vs))N(−ρ[∂2μ1∂φ2+∂2μ1∂ϱ2]+(μ0+μ1)∂(μ0+μ1)∂φ+(ν0+ν1)∂(μ0+μ1)∂ϱ−μ0∂μ0∂φ−ν0∂μ0∂ϱ)]=−(2ρ)2e(φ+ϱ)[(1−θ)2+2θ(1−θ)ℓ+θ2ℓ22!], | (5.12) |
ν2(φ,ϱ,ℓ)=−N−1[(1−θ+θ(vs))N[(R(ν1(φ,ϱ,ℓ))+{N(ν0(φ,ϱ,ℓ)+ν1(φ,ϱ,ℓ))−N(ν0(φ,ϱ,ℓ))}]]=−N−1[(1−θ+θ(vs))N(−ρ[∂2ν1∂φ2+∂2ν1∂ϱ2]+(μ0+μ1)∂(ν0+ν1)∂φ+(ν0+ν1)∂(ν0+ν1)∂ϱ−μ0∂ν0∂φ−ν0∂ν0∂ϱ)]=(2ρ)2e(φ+ϱ)[(1−θ)2+2θ(1−θ)ℓ+θ2ℓ22!], | (5.13) |
μ3(φ,ϱ,ℓ)=−N−1[(1−θ+θ(vs))N[(R(μ2(φ,ϱ,ℓ))+{N(μ0(φ,ϱ,ℓ)+μ1(φ,ϱ,ℓ)+μ2(φ,ϱ,ℓ))−N(μ0(φ,ϱ,ℓ)+μ1(φ,ϱ,ℓ))}]]=−N−1[(1−θ+θ(vs))N(−ρ[∂2μ2∂φ2+∂2μ2∂ϱ2]+(μ0+μ1+μ2)∂(μ0+μ1+μ2)∂φ+(ν0+ν1+ν2)∂(μ0+μ1+μ2)∂ϱ−(μ0+μ1)∂(μ0+μ1)∂φ−(ν0+ν1)∂(μ0+μ1)∂ϱ)]=−(2ρ)3e(φ+ϱ)[(1−θ)3+3θ(1−θ)2ℓ+3θ2(1−θ)ℓ22!+θ3ℓ33!],ν3(φ,ϱ,ℓ)=−N−1[(1−θ+θ(vs))N[(R(ν2(φ,ϱ,ℓ))+{N(ν0(φ,ϱ,ℓ)+ν1(φ,ϱ,ℓ)+ν2(φ,ϱ,ℓ))−N(ν0(φ,ϱ,ℓ)+ν1(φ,ϱ,ℓ))}]]=−N−1[(1−θ+θ(vs))N(−ρ[∂2ν2∂φ2+∂2ν2∂ϱ2]+(μ0+μ1+μ2)∂(ν0+ν1+ν2)∂φ+(ν0+ν1+ν2)∂(ν0+ν1+ν2)∂ϱ−(μ0+μ1)∂(ν0+ν1)∂φ−(ν0+ν1)∂(ν0+ν1)∂ϱ)]=(2ρ)3e(φ+ϱ)[(1−θ)3+3θ(1−θ)2ℓ+3θ2(1−θ)ℓ22!+θ3ℓ33!],⋮ | (5.14) |
In a general way,
μ(φ,ϱ,ℓ)=∞∑r=0μr(φ,ϱ,ℓ)=μ0(φ,ϱ,ℓ)+μ1(φ,ϱ,ℓ)+μ2(φ,ϱ,ℓ)+⋯,ν(φ,ϱ,ℓ)=∞∑r=0νr(φ,ϱ,ℓ)=ν0(φ,ϱ,ℓ)+ν1(φ,ϱ,ℓ)+ν2(φ,ϱ,ℓ)+⋯. |
With the addition of all μ and ν,
μ(φ,ϱ,ℓ)=−e(φ+ϱ)+q.[(1−θ)+θℓ]−2ρe(φ+ϱ)[(1−θ)+θℓ]−(2ρ)2e(φ+ϱ)[(1−θ)2+2θ(1−θ)ℓ+θ2ℓ22!]−(2ρ)3e(φ+ϱ)[(1−θ)3+3θ(1−θ)2ℓ+3θ2(1−θ)ℓ22!+θ3ℓ33!]−⋯, |
ν(φ,ϱ,ℓ)=e(φ+ϱ)−q.[(1−θ)+θℓ]+2ρe(φ+ϱ)[(1−θ)+θℓ]+(2ρ)2e(φ+ϱ)[(1−θ)2+2θ(1−θ)ℓ+θ2ℓ22!]+(2ρ)3e(φ+ϱ)[(1−θ)3+3θ(1−θ)2ℓ+3θ2(1−θ)ℓ22!+θ3ℓ33!]+⋯. |
The exact solution of Eq (5.9) at θ=1 and q=0 is given by
μ(φ,ϱ,ℓ)=−eφ+ϱ+2ρℓ,ν(φ,ϱ,ℓ)=eφ+ϱ+2ρℓ. | (5.15) |
Consider the following non-linear fractional PDEs
CF0Dθℓ℧(φ,ϱ,ℓ)+R(℧(φ,ϱ,ℓ))+N(℧(φ,ϱ,ℓ))−P(φ,ϱ,ℓ)=0,0<θ≤1, | (6.1) |
subject to the initial condition
℧(φ,ϱ,0)=℧0(φ,ϱ). | (6.2) |
CF0Dθℓ is the Caputo-Fabrizio fractional differential operator of order θ,R and N are linear and non-linear terms, and P is the source term.
By using the natural transform on both sides of Eq (6.1), we get
N[CF0Dθℓ℧(φ,ϱ,ℓ)+R(℧(φ,ϱ,ℓ))+N(℧(φ,ϱ,))−P(φ,ϱ,ℓ)=0], | (6.3) |
N[℧(φ,ϱ,ℓ)]=ϖ(℧(φ,ϱ,s))−(1−θ+θ(vs))N{[R(℧(φ,ϱ,ℓ))+N(℧(φ,ϱ,ℓ))]}, | (6.4) |
where
ϖ(℧(φ,ϱ,s))=s−1℧(φ,ϱ,0)+(1−θ+θ(vs))˜P(φ,ϱ,s). |
By applying the inverse natural transform, Eq (6.4) is reduced to the form
℧(φ,ϱ,ℓ)=ϖ(℧(φ,ϱ,ℓ))−N−1[(1−θ+θ(vs))N{[R(℧(φ,ϱ,ℓ))+N(℧(φ,ϱ,ℓ))]}], | (6.5) |
where ϖ(℧(φ,ϱ,ℓ)) represents the term arising from the source term. Now, applying the HPTM to find the solution of Eq (6.5), we get
℧(φ,ϱ,ℓ)=∞∑r=0zr℧r(φ,ϱ,ℓ), | (6.6) |
and the non-linear tern can be decomposed as
N(℧(φ,ϱ,ℓ))=∞∑r=0zrHr(φ,ϱ,ℓ). | (6.7) |
Cnsider some He's polynomials [54], given as
Hr(℧0,℧1,...,℧r)=1r!∂r∂zr[N(∞∑j=0zj℧j)],r=0,1,2,⋯. | (6.8) |
By substituting Eqs (6.6) and (6.7) into Eq (6.5), we get
∞∑r=0℧r(φ,ϱ,ℓ)zr=ϖ(℧(φ,ϱ,ℓ))−z.N−1[(1−θ+θ(vs))N{R∞∑r=0zr℧r(φ,ϱ,ℓ)+N∞∑r=0zrHr(φ,ϱ,ℓ)}]. | (6.9) |
Comparing the coefficients of like powers of z, the following approximations are obtained:
z0:℧0(φ,ϱ,ℓ)=ϖ(℧(φ,ϱ,ℓ)) | (6.10) |
z1:℧1(φ,ϱ,ℓ)=−N−1[(1−θ+θ(vs))N{R[℧0(φ,ϱ,ℓ)]+H0(℧)}]⋮ | (6.11) |
zr+1:℧r+1(φ,ϱ,ℓ)=−N−1[(1−θ+θ(vs))N{R[℧r(φ,ϱ,ℓ)]+Hr(℧)}]. | (6.12) |
Consider the two-dimensional fractional N-S equation
CF0Dθℓ(μ)+μ∂μ∂φ+ν∂μ∂ϱ=ρ[∂2μ∂φ2+∂2μ∂ϱ2]+q,CF0Dθℓ(ν)+μ∂ν∂φ+ν∂ν∂ϱ=ρ[∂2ν∂φ2+∂2ν∂ϱ2]−q, | (6.13) |
with initial conditions
{μ(φ,ϱ,0)=−sin(φ+ϱ),ν(φ,ϱ,0)=sin(φ+ϱ). | (6.14) |
Applying the natural transform and inversion in Eq (6.13), we obtain
μ(φ,ϱ,ℓ)=μ(φ,ϱ,0)+N−1[(1−θ+θ(vs))N[q]]+N−1[(1−θ+θ(vs))×N{ρ(∂2μ∂φ2+∂2μ∂ϱ2)−(μ∂μ∂φ+ν∂μ∂ϱ)}],ν(φ,ϱ,ℓ)=ν(φ,ϱ,0)−N−1[(1−θ+θ(vs))N[q]]+N−1[(1−θ+θ(vs))×N{ρ(∂2ν∂φ2+∂2ν∂ϱ2)−(μ∂ν∂φ+ν∂ν∂ϱ)}]. | (6.15) |
By implementing HPTM in Eq (6.15), we get
∞∑r=0zrμ(φ,ϱ,ℓ)=−sin(φ+ϱ)+N−1[(1−θ+θ(vs))N[q]]+z.N−1[(1−θ+θ(vs))×N{ρ∞∑r=0zr(∂2μ∂φ2+∂2μ∂ϱ2)−∞∑r=0zrHr(φ,ϱ)}],∞∑r=0zrν(φ,ϱ,ℓ)=sin(φ+ϱ)−N−1[(1−θ+θ(vs))N[q]]+z.N−1[(1−θ+θ(vs))×N{ρ∞∑r=0zr(∂2ν∂φ2+∂2ν∂ϱ2)−∞∑r=0zrIr(φ,ϱ)}]. | (6.16) |
where Hr(φ,ϱ)=μ∂μ∂φ+ν∂μ∂ϱ and Ir(φ,ϱ)=μ∂ν∂φ+ν∂ν∂ϱ, represent the nonlinear term.
From Eq (6.16), comparing the powers of z, we get
z0:μ0(φ,ϱ,ℓ)=−sin(φ+ϱ)+q.[(1−θ)+θℓ],z0:ν0(φ,ϱ,ℓ)=sin(φ+ϱ)−q.[(1−θ)+θℓ], | (6.17) |
z1:μ1(φ,ϱ,ℓ)=N−1[(1−θ+θ(vs))N{ρ(∂2μ0∂φ2+∂2μ0∂ϱ2)−H0(φ,ϱ)}]=2ρsin(φ+ϱ)[(1−θ)+θℓ],z1:ν1(φ,ϱ,ℓ)=N−1[(1−θ+θ(vs))N{ρ(∂2ν0∂φ2+∂2ν0∂ϱ2)−I0(φ,ϱ)}]=−2ρsin(φ+ϱ)[(1−θ)+θℓ], | (6.18) |
where H0(φ,ϱ)=μ0∂μ0∂φ+ν0∂μ0∂ϱ and I0(φ,ϱ)=μ0∂ν0∂φ+ν0∂ν0∂ϱ.
z2:μ2(φ,ϱ,ℓ)=N−1[(1−θ+θ(vs))N{ρ(∂2μ1∂φ2+∂2μ1∂ϱ2)−H1(φ,ϱ)}]=−(2ρ)2sin(φ+ϱ)[(1−θ)2+2θ(1−θ)ℓ+θ2ℓ22!],z2:ν2(φ,ϱ,ℓ)=N−1[(1−θ+θ(vs))N{ρ(∂2ν1∂φ2+∂2ν1∂ϱ2)−I1(φ,ϱ)}]=(2ρ)2sin(φ+ϱ)[(1−θ)2+2θ(1−θ)ℓ+θ2ℓ22!], | (6.19) |
where H1(φ,ϱ)=(μ0∂μ1∂φ+μ1∂μ0∂φ)+(ν0∂μ1∂ϱ+ν1∂μ0∂ϱ),
and I1(φ,ϱ)=(μ0∂ν1∂φ+μ1∂ν0∂φ)+(ν0∂ν1∂ϱ+ν1∂ν0∂ϱ).
z3:μ3(φ,ϱ,ℓ)=N−1[(1−θ+θ(vs))N{ρ(∂2μ2∂φ2+∂2μ2∂ϱ2)−H2(φ,ϱ)}]=(2ρ)3sin(φ+ϱ)[(1−θ)3+3θ(1−θ)2ℓ+3θ2(1−θ)ℓ22!+θ3ℓ33!],z3:ν3(φ,ϱ,ℓ)=N−1[(1−θ+θ(vs))N{ρ(∂2ν2∂φ2+∂2ν2∂ϱ2)−I2(φ,ϱ)}]=−(2ρ)3sin(φ+ϱ)[(1−θ)3+3θ(1−θ)2ℓ+3θ2(1−θ)ℓ22!+θ3ℓ33!], | (6.20) |
where H2(φ,ϱ)=(μ0∂μ2∂φ+μ1∂μ1∂φ+μ2∂μ0∂φ)+(ν0∂μ2∂ϱ+ν1∂μ1∂ϱ+ν2∂μ0∂ϱ),
and I2(φ,ϱ)=(μ0∂ν2∂φ+μ1∂ν1∂φ+μ2∂ν0∂φ)+(ν0∂ν2∂ϱ+ν1∂ν1∂ϱ+ν2∂ν0∂ϱ).
⋮
In a general way,
μ(φ,ϱ,ℓ)=∞∑r=0μr(φ,ϱ,ℓ)=μ0(φ,ϱ,ℓ)+μ1(φ,ϱ,ℓ)+μ2(φ,ϱ,ℓ)+⋯,ν(φ,ϱ,ℓ)=∞∑r=0νr(φ,ϱ,ℓ)=ν0(φ,ϱ,ℓ)+ν1(φ,ϱ,ℓ)+ν2(φ,ϱ,ℓ)+⋯. |
With the addition of all μ and ν,
μ(φ,ϱ,ℓ)=−sin(φ+ϱ)+q.[(1−θ)+θℓ]+2ρsin(φ+ϱ)[(1−θ)+θℓ]−(2ρ)2sin(φ+ϱ)[(1−θ)2+2θ(1−θ)ℓ+θ2ℓ22!]+(2ρ)3sin(φ+ϱ)×[(1−θ)3+3θ(1−θ)2ℓ+3θ2(1−θ)ℓ22!+θ3ℓ33!]−⋯. |
ν(φ,ϱ,ℓ)=sin(φ+ϱ)−q.[(1−θ)+θℓ]−2ρsin(φ+ϱ)[(1−θ)+θℓ]+(2ρ)2sin(φ+ϱ)[(1−θ)2+2θ(1−θ)ℓ+θ2ℓ22!]−(2ρ)3sin(φ+ϱ)×[(1−θ)3+3θ(1−θ)2ℓ+3θ2(1−θ)ℓ22!+θ3ℓ33!]+⋯. |
The exact solution of Eq (6.13) at θ=1 and q=0 is given by
μ(φ,ϱ,ℓ)=−e−2ρℓsin(φ+ϱ),ν(φ,ϱ,ℓ)=e−2ρℓsin(φ+ϱ). | (6.21) |
Consider the two-dimensional fractional order N-S equation
CF0Dθℓ(μ)+μ∂μ∂φ+ν∂μ∂ϱ=ρ[∂2μ∂φ2+∂2μ∂ϱ2]+q,CF0Dθℓ(ν)+μ∂ν∂φ+ν∂ν∂ϱ=ρ[∂2ν∂φ2+∂2ν∂ϱ2]−q, | (6.22) |
with initial conditions
{μ(φ,ϱ,0)=−e(φ+ϱ),ν(φ,ϱ,0)=e(φ+ϱ). | (6.23) |
Applying the natural transform and inversion in Eq (6.22), we obtain
μ(φ,ϱ,ℓ)=μ(φ,ϱ,0)+N−1[(1−θ+θ(vs))N[q]]+N−1[(1−θ+θ(vs))×N{ρ(∂2μ∂φ2+∂2μ∂ϱ2)−(μ∂μ∂φ+ν∂μ∂ϱ)}],ν(φ,ϱ,ℓ)=ν(φ,ϱ,0)−N−1[(1−θ+θ(vs))N[q]]+N−1[(1−θ+θ(vs))×N{ρ(∂2ν∂φ2+∂2ν∂ϱ2)−(μ∂ν∂φ+ν∂ν∂ϱ)}]. | (6.24) |
By implementing HPTM in Eq (6.24), we get
∞∑r=0zrμ(φ,ϱ,ℓ)=−e(φ+ϱ)+N−1[(1−θ+θ(vs))N[q]]+z.N−1[(1−θ+θ(vs))×N{ρ∞∑r=0zr(∂2μ∂φ2+∂2μ∂ϱ2)−∞∑r=0zrHr(φ,ϱ)}],∞∑r=0zrν(φ,ϱ,ℓ)=e(φ+ϱ)−N−1[(1−θ+θ(vs))N[q]]+z.N−1[(1−θ+θ(vs))×N{ρ∞∑r=0zr(∂2ν∂φ2+∂2ν∂ϱ2)−∞∑r=0zrIr(φ,ϱ)}]. | (6.25) |
where Hr(φ,ϱ)=μ∂μ∂φ+ν∂μ∂ϱ and Ir(φ,ϱ)=μ∂ν∂φ+ν∂ν∂ϱ represent the nonlinear terms.
From Eq (6.25), comparing the powers of z, we get
z0:μ0(φ,ϱ,ℓ)=−e(φ+ϱ)+q.[(1−θ)+θℓ],z0:ν0(φ,ϱ,ℓ)=e(φ+ϱ)−q.[(1−θ)+θℓ], | (6.26) |
z1:μ1(φ,ϱ,ℓ)=N−1[(1−θ+θ(vs))N{ρ(∂2μ0∂φ2+∂2μ0∂ϱ2)−H0(φ,ϱ)}]=−2ρe(φ+ϱ)[(1−θ)+θℓ],z1:ν1(φ,ϱ,ℓ)=N−1[(1−θ+θ(vs))N{ρ(∂2ν0∂φ2+∂2ν0∂ϱ2)−I0(φ,ϱ)}]=2ρe(φ+ϱ)[(1−θ)+θℓ]. | (6.27) |
where H0(φ,ϱ)=μ0∂μ0∂φ+ν0∂μ0∂ϱ and I0(φ,ϱ)=μ0∂ν0∂φ+ν0∂ν0∂ϱ.
z2:μ2(φ,ϱ,ℓ)=N−1[(1−θ+θ(vs))N{ρ(∂2μ1∂φ2+∂2μ1∂ϱ2)−H1(φ,ϱ)}]=−(2ρ)2e(φ+ϱ)[(1−θ)2+2θ(1−θ)ℓ+θ2ℓ22!],z2:ν2(φ,ϱ,ℓ)=N−1[(1−θ+θ(vs))N{ρ(∂2ν1∂φ2+∂2ν1∂ϱ2)−I1(φ,ϱ)}]=(2ρ)2e(φ+ϱ)[(1−θ)2+2θ(1−θ)ℓ+θ2ℓ22!], | (6.28) |
where H1(φ,ϱ)=(μ0∂μ1∂φ+μ1∂μ0∂φ)+(ν0∂μ1∂ϱ+ν1∂μ0∂ϱ),
and I1(φ,ϱ)=(μ0∂ν1∂φ+μ1∂ν0∂φ)+(ν0∂ν1∂ϱ+ν1∂ν0∂ϱ).
z3:μ3(φ,ϱ,ℓ)=N−1[(1−θ+θ(vs))N{ρ(∂2μ2∂φ2+∂2μ2∂ϱ2)−H2(φ,ϱ)}]=−(2ρ)3e(φ+ϱ)[(1−θ)3+3θ(1−θ)2ℓ+3θ2(1−θ)ℓ22!+θ3ℓ33!],z3:ν3(φ,ϱ,ℓ)=N−1[(1−θ+θ(vs))N{ρ(∂2ν2∂φ2+∂2ν2∂ϱ2)−I2(φ,ϱ)}]=(2ρ)3e(φ+ϱ)[(1−θ)3+3θ(1−θ)2ℓ+3θ2(1−θ)ℓ22!+θ3ℓ33!], | (6.29) |
where H2(φ,ϱ)=(μ0∂μ2∂φ+μ1∂μ1∂φ+μ2∂μ0∂φ)+(ν0∂μ2∂ϱ+ν1∂μ1∂ϱ+ν2∂μ0∂ϱ),
and I2(φ,ϱ)=(μ0∂ν2∂φ+μ1∂ν1∂φ+μ2∂ν0∂φ)+(ν0∂ν2∂ϱ+ν1∂ν1∂ϱ+ν2∂ν0∂ϱ).
⋮
In a general way,
μ(φ,ϱ,ℓ)=∞∑r=0μr(φ,ϱ,ℓ)=μ0(φ,ϱ,ℓ)+μ1(φ,ϱ,ℓ)+μ2(φ,ϱ,ℓ)+⋯,ν(φ,ϱ,ℓ)=∞∑r=0νr(φ,ϱ,ℓ)=ν0(φ,ϱ,ℓ)+ν1(φ,ϱ,ℓ)+ν2(φ,ϱ,ℓ)+⋯. |
With the addition of all μ and ν,
μ(φ,ϱ,ℓ)=−e(φ+ϱ)+q.[(1−θ)+θℓ]−2ρe(φ+ϱ)[(1−θ)+θℓ]−(2ρ)2e(φ+ϱ)[(1−θ)2+2θ(1−θ)ℓ+θ2ℓ22!]−(2ρ)3e(φ+ϱ)×[(1−θ)3+3θ(1−θ)2ℓ+3θ2(1−θ)ℓ22!+θ3ℓ33!]−... |
ν(φ,ϱ,ℓ)=e(φ+ϱ)−q.[(1−θ)+θℓ]+2ρe(φ+ϱ)[(1−θ)+θℓ]+(2ρ)2e(φ+ϱ)[(1−θ)2+2θ(1−θ)ℓ+θ2ℓ22!]+(2ρ)3e(φ+ϱ)×[(1−θ)3+3θ(1−θ)2ℓ+3θ2(1−θ)ℓ22!+θ3ℓ33!]+... |
The exact solution of Eq (6.22) at θ=1 and q=0 is given by
μ(φ,ϱ,ℓ)=−eφ+ϱ+2ρℓ,ν(φ,ϱ,ℓ)=eφ+ϱ+2ρℓ. | (6.30) |
Effective analytical techniques were used to analyze the solution of the time-fractional multi-dimensional N-S equation. The fractional derivatives are defined in the form of Caputo-Fabrizio, and are examined by the NITM and HPTM, along with NT. To verify that the suggested approaches are accurate and applicable, the graphical interpretation is illustrated for both fractional and integer orders for some examples.
Figures 1 and 2 demonstrate the behavior of the exact and analytical solutions of Example 1 for μ(φ,ϱ,ℓ) and ν(φ,ϱ,ℓ) at θ=1, and demonstrate that the NITM solution figures are identical and in close contact with the exact solution of the example.
The physical attributes of μ(φ,ϱ,ℓ) corresponding to the various fractional-orders θ=0.2,0.4,0.6,0.8 of Example 1 are plotted in Figures 3 and 4.
Similarly, the graphical solutions of ν(φ,ϱ,ℓ) for various fractional-orders θ=0.2,0.4,0.6,0.8 of Example 1 are examined in Figures 5 and 6. It is shown that the NITM solutions are in strong agreement with the exact solutions and show a high rate of convergence.
Figures 7 and 8 represent the analytical and exact solutions of Examples 2 and 4 for μ(φ,ϱ,ℓ) and ν(φ,ϱ,ℓ) at θ=1.
It can be seen that the NITM solution figures are identical and in close contact with the exact solution of the example. Furthermore, in Figures 9 and 10, Examples 2 and 4 are calculated by the NITM method, and the value of μ(φ,ϱ,ℓ) is examined corresponding to the various fractional orders θ=0.2,0.4,0.6,0.8 by graphical interpretation.
Similarly, the graphical solution of ν(φ,ϱ,ℓ) for various fractional orders θ=0.2,0.4,0.6,0.8 of Example 2 is analyzed in Figures 11 and 12.
It is observed that the outcome of the NITM method and its graphical interpretation demonstrate the accuracy and applicability of the suggested techniques, and it is noted that the fractional-order solution exhibits the same convergence trends as that of integer-order solutions.
This article presents the successful implementation of NITM and HPTM to evaluate the solution of the time-fractional multi-dimensional N-S equation analytically. The efficacy and accuracy of the proposed methods are examined with the support of four examples, and the outcomes show how effective, precise, and easy the methods are to use. The graphical interpretation of different values of the fractional-order θ on the solution profile is displayed in Figures 2–6 and in Figures 9–12, which demonstrate some interesting dynamics of the model. The results obtained by these methods are in a series form, and close agreement with those solutions is given by [44,45]. It is noted that there is a high rate of convergence between the series solutions obtained towards the solutions of integer order. Furthermore, the suggested methods are simple to use, and they may be used to solve additional fractional PDEs that arise in applied research.
The authors declare they have not used Artificial Intelligence (AI) tools in the creation of this article.
The authors extend their appreciation to the Deputyship for Research and Innovation, Ministry of Education in Saudi Arabia for funding their research work through project number ISP-2024.
There is no competing interest among the authors regarding the publication of the article.
[1] |
G. Velmurugan, R. Rakkiyappan, J. D. Cao, Finite-time synchronization of fractional-order memristor-based neural networks with time delays, Neural Networks, 73 (2016), 36–46. https://doi.org/10.1016/j.neunet.2015.09.012 doi: 10.1016/j.neunet.2015.09.012
![]() |
[2] | A. Bouzerdoum, Classification and function approximation using feed-forward shunting inhibitory artificial neural networks, In: Proceedings Of The IEEE-INNS-ENNS International Joint Conference On Neural Networks. IJCNN 2000. Neural Computing: New Challenges And Perspectives For The New Millennium, 2000, 613–618. https://doi.org/10.1109/IJCNN.2000.859463 |
[3] | F. H. C. Tivive, A. Bouzerdoum, A face detection system using shunting inhibitory convolutional neural networks, In: 2004 IEEE International Joint Conference On Neural Networks, 2004, 2571–2575. https://doi.org/10.1109/IJCNN.2004.1381049 |
[4] |
S. Yan, Z. Gu, Ju. H. Park, X. P. Xie, Synchronization of delayed fuzzy neural networks with probabilistic communication delay and its application to image encryption, IEEE Trans. Fuzzy Syst., 31 (2023), 930–940. https://doi.org/10.1109/TFUZZ.2022.3193757 doi: 10.1109/TFUZZ.2022.3193757
![]() |
[5] |
H. M. Oliveira, L. V. Melo, Huygens synchronization of two clocks, Sci. Rep., 5 (2015), 11548. https://doi.org/10.1038/srep11548 doi: 10.1038/srep11548
![]() |
[6] |
S. Y. Dong, X. Z. Liu, S. M. Zhong, K. B. Shi, H. Zhu, Practical synchronization of neural networks with delayed impulses and external disturbance via hybrid control, Neural Networks, 157 (2023), 54–64. https://doi.org/10.1016/j.neunet.2022.09.025 doi: 10.1016/j.neunet.2022.09.025
![]() |
[7] |
C. Xu, X. S. Yang, J. Q. Lu, J. W. Feng, F. E. Alsaadi, T. Hayat, Finite-time synchronization of networks via quantized intermittent pinning control, IEEE Trans. Cybernetics, 48 (2018), 3021–3027. https://doi.org/10.1109/TCYB.2017.2749248 doi: 10.1109/TCYB.2017.2749248
![]() |
[8] |
X. Y. Liu, H. S. Su, M. Z. Q. Chen, A switching approach to designing finite-time synchronization controllers of coupled neural networks, IEEE Trans. Neur. Net. Lear., 27 (2015), 471–482. https://doi.org/10.1109/TNNLS.2015.2448549 doi: 10.1109/TNNLS.2015.2448549
![]() |
[9] | P. Pucci, J. Serrin, The maximum principle, Basel: Birkhäuser, 2007. https://doi.org/10.1007/978-3-7643-8145-5 |
[10] |
V. Zeidan, C. Nour, H. Saoud, A nonsmooth maximum principle for a controlled nonconvex sweeping process, J. Differ. Equations, 269 (2020), 9531–9582. https://doi.org/10.1016/j.jde.2020.06.053 doi: 10.1016/j.jde.2020.06.053
![]() |
[11] |
Q. Du, L. L. Ju, X. Li, Z. H. Qiao, Maximum principle preserving exponential time differencing schemes for the nonlocal Allen–Cahn equation, SIAM J. Numer. Anal., 57 (2019), 875–898. https://doi.org/10.1137/18M118236X doi: 10.1137/18M118236X
![]() |
[12] |
A. Kashkynbayev, M. Koptileuova, A. Issakhanov, J. D. Cao, Almost periodic solutions of fuzzy shunting inhibitory CNNs with delays, AIMS Mathematics, 7 (2022), 11813–11828. https://doi.org/10.3934/math.2022659 doi: 10.3934/math.2022659
![]() |
[13] |
X. G. Tan, C. C. Xiang, J. D. Cao, W. Y. Xu, G. H. Wen, L. Rutkowski, Synchronization of neural networks via periodic self-triggered impulsive control and its application in image encryption, IEEE Trans. Cybernetics, 52 (2022), 8246–8257. https://doi.org/10.1109/TCYB.2021.3049858 doi: 10.1109/TCYB.2021.3049858
![]() |
[14] |
Y. Wang, S. B. Ding, R. X. Li, Master–slave synchronization of neural networks via event-triggered dynamic controller, Neurocomputing, 419 (2021), 215–223. https://doi.org/10.1016/j.neucom.2020.08.062 doi: 10.1016/j.neucom.2020.08.062
![]() |
[15] |
F. Liu, C. Liu, H. X. Rao, Y. Xu, T. W. Huang, Reliable impulsive synchronization for fuzzy neural networks with mixed controllers, Neural Networks, 143 (2021), 759–766. https://doi.org/10.1016/j.neunet.2021.08.013 doi: 10.1016/j.neunet.2021.08.013
![]() |
[16] |
L. Y. Duan, J. M. Li, Fixed-time synchronization of fuzzy neutral-type BAM memristive inertial neural networks with proportional delays, Inform. Sciences, 576 (2021), 522–541. https://doi.org/10.1016/j.ins.2021.06.093 doi: 10.1016/j.ins.2021.06.093
![]() |
[17] |
M. Abudusaimaiti, A. Abdurahman, H. J. Jiang, C. Hu, Fixed/predefined-time synchronization of fuzzy neural networks with stochastic perturbations, Chaos Soliton. Fract., 154 (2022), 111596. https://doi.org/10.1016/j.chaos.2021.111596 doi: 10.1016/j.chaos.2021.111596
![]() |
[18] |
X. N. Li, H. Q. Wu, J. D. Cao, A new prescribed-time stability theorem for impulsive piecewise-smooth systems and its application to synchronization in networks, Appl. Math. Model., 115 (2023), 385–397. https://doi.org/10.1016/j.apm.2022.10.051 doi: 10.1016/j.apm.2022.10.051
![]() |
[19] |
X. N. Li, H. Q. Wu, J. D. Cao, Prescribed-time synchronization in networks of piecewise smooth systems via a nonlinear dynamic event-triggered control strategy, Math. Comput. Simulat., 203 (2023), 647–668. https://doi.org/10.1016/j.matcom.2022.07.010 doi: 10.1016/j.matcom.2022.07.010
![]() |
[20] |
X. Z. Jin, G. H. Yang, Adaptive pinning synchronization of a class of nonlinearly coupled complex networks, Commun. Nonlinear Sci., 18 (2013), 316–326. https://doi.org/10.1016/j.cnsns.2012.07.011 doi: 10.1016/j.cnsns.2012.07.011
![]() |
[21] |
Q. Chen, B. Li, W. Yin, X. W. Jiang, X. Y. Chen, Bifurcation, chaos and fixed-time synchronization of memristor cellular neural networks, Chaos Soliton. Fract., 171 (2023), 113440. https://doi.org/10.1016/j.chaos.2023.113440 doi: 10.1016/j.chaos.2023.113440
![]() |
[22] |
F. F. Du, J.-G. Lu, Adaptive finite-time synchronization of fractional-order delayed fuzzy cellular neural networks, Fuzzy Set. Syst., 466 (2023), 108480. https://doi.org/10.1016/j.fss.2023.02.001 doi: 10.1016/j.fss.2023.02.001
![]() |
[23] |
X. Z. Jin, J. H. Jiang, J. Chi, X. M. Wu, Adaptive finite-time pinned and regulation synchronization of disturbed complex networks, Commun. Nonlinear Sci., 124 (2023), 107319. https://doi.org/10.1016/j.cnsns.2023.107319 doi: 10.1016/j.cnsns.2023.107319
![]() |
[24] |
J. H. Jiang, X. Z. Jin, J. Chi, X. M. Wu, Distributed adaptive fixed-time synchronization for disturbed complex networks, Chaos Soliton. Fract., 173 (2023), 113612. https://doi.org/10.1016/j.chaos.2023.113612 doi: 10.1016/j.chaos.2023.113612
![]() |
[25] |
C. J. Cheng, T. L. Liao, C. C. Hwang, Exponential synchronization of a class of chaotic neural networks, Chaos Soliton. Fract., 24 (2005), 197–206. https://doi.org/10.1016/j.chaos.2004.09.022 doi: 10.1016/j.chaos.2004.09.022
![]() |
[26] | M. H. Protter, H. F. Weinberger, Maximum principles in differential equations, New York: Springer, 1984. https://doi.org/10.1007/978-1-4612-5282-5 |
[27] | T. Yang, L.-B. Yang, C. W. Wu, L. O. Chua, Fuzzy cellular neural networks: applications, In: 1996 Fourth IEEE International Workshop On Cellular Neural Networks And Their Applications Proceedings (CNNA-96), 1996,225–230. https://doi.org/10.1109/CNNA.1996.566560 |
[28] |
T. Yang, L. B. Yang, Fuzzy cellular neural network: a new paradigm for image processing, Int. J. Circ. Theor. Appl., 25 (1997), 469–481. https://doi.org/10.1002/(SICI)1097-007X(199711/12)25:6<469::AID-CTA967>3.0.CO;2-1 doi: 10.1002/(SICI)1097-007X(199711/12)25:6<469::AID-CTA967>3.0.CO;2-1
![]() |
[29] |
P. V. De Campos Souza, Fuzzy neural networks and neuro-fuzzy networks: a review the main techniques and applications used in the literature, Appl. Soft Comput., 92 (2020), 106275. https://doi.org/10.1016/j.asoc.2020.106275 doi: 10.1016/j.asoc.2020.106275
![]() |
[30] |
A. Kashkynbayev, J. D. Cao, Z. Damiyev, Stability analysis for periodic solutions of fuzzy shunting inhibitory CNNs with delays, Adv. Differ. Equ., 2019 (2019), 384. https://doi.org/10.1186/s13662-019-2321-z doi: 10.1186/s13662-019-2321-z
![]() |
[31] |
S. C. Lee, E. T. Lee, Fuzzy neural networks, Math. Biosci., 23 (1975), 151–177. https://doi.org/10.1016/0025-5564(75)90125-X doi: 10.1016/0025-5564(75)90125-X
![]() |
[32] |
M. M. Gupta, D. H. Rao, On the principles of fuzzy neural networks, Fuzzy Set. Syst., 61 (1994), 1–18. https://doi.org/10.1016/0165-0114(94)90279-8 doi: 10.1016/0165-0114(94)90279-8
![]() |
[33] |
T. Yang, L.-B. Yang, The global stability of fuzzy cellular neural network, IEEE Trans. Circuits Syst. I, 43 (1996), 880–883. https://doi.org/10.1109/81.538999 doi: 10.1109/81.538999
![]() |
[34] |
A. Kashkynbayev, A. Issakhanov, M. Otkel, J. Kurths, Finite-time and fixed-time synchronization analysis of shunting inhibitory memristive neural networks with time-varying delays, Chaos Soliton. Fract., 156 (2022), 111866. https://doi.org/10.1016/j.chaos.2022.111866 doi: 10.1016/j.chaos.2022.111866
![]() |
[35] |
C. Foias, G. R. Sell, R. Temam, Inertial manifolds for nonlinear evolutionary equations, J. Differ. Equations, 73 (1988), 309–353. https://doi.org/10.1016/0022-0396(88)90110-6 doi: 10.1016/0022-0396(88)90110-6
![]() |
[36] |
E. S. Titi, On approximate inertial manifolds to the Navier-Stokes equations, J. Math. Anal. Appl., 149 (1990), 540–557. https://doi.org/10.1016/0022-247X(90)90061-J doi: 10.1016/0022-247X(90)90061-J
![]() |
[37] |
M. S. Jolly, I. G. Kevrekidis, E. S. Titi, Approximate inertial manifolds for the Kuramoto-Sivashinsky equation: analysis and computations, Physica D, 44 (1990), 38–60. https://doi.org/10.1016/0167-2789(90)90046-R doi: 10.1016/0167-2789(90)90046-R
![]() |
[38] |
J. D. Cao, Y. Wan, Matrix measure strategies for stability and synchronization of inertial BAM neural network with time delays, Neural Networks, 53 (2014), 165–172. https://doi.org/10.1016/j.neunet.2014.02.003 doi: 10.1016/j.neunet.2014.02.003
![]() |
[39] |
S. Lakshmanan, M. Prakash, C. P. Lim, R. Rakkiyappan, P. Balasubramaniam, S. Nahavandi, Synchronization of an inertial neural network with time-varying delays and its application to secure communication, IEEE Trans. Neur. Net. Lear., 29 (2018), 195–207. https://doi.org/10.1109/TNNLS.2016.2619345 doi: 10.1109/TNNLS.2016.2619345
![]() |
[40] |
X. Y. Li, X. T. Li, C. Hu, Some new results on stability and synchronization for delayed inertial neural networks based on non-reduced order method, Neural Networks, 96 (2017), 91–100. https://doi.org/10.1016/j.neunet.2017.09.009 doi: 10.1016/j.neunet.2017.09.009
![]() |
[41] |
W. H. Li, X. B. Gao, R. X. Li, Stability and synchronization control of inertial neural networks with mixed delays, Appl. Math. Comput., 367 (2020), 124779. https://doi.org/10.1016/j.amc.2019.124779 doi: 10.1016/j.amc.2019.124779
![]() |
[42] |
Z. Q. Zhang, J. D. Cao, Finite-time synchronization for fuzzy inertial neural networks by maximum value approach, IEEE Trans. Fuzzy Syst., 30 (2022), 1436–1446. https://doi.org/10.1109/TFUZZ.2021.3059953 doi: 10.1109/TFUZZ.2021.3059953
![]() |
[43] |
J.-L. Wang, H.-N. Wu, T. W. Huang, S.-Y. Ren, Pinning control strategies for synchronization of linearly coupled neural networks with reaction–diffusion terms, IEEE Trans. Neur. Net. Lear., 27 (2016), 749–761. https://doi.org/10.1109/TNNLS.2015.2423853 doi: 10.1109/TNNLS.2015.2423853
![]() |
[44] |
Y. Y. Cao, Y. T. Cao, Z. Y. Guo, T. W. Huang, S. P. Wen, Global exponential synchronization of delayed memristive neural networks with reaction–diffusion terms, Neural Networks, 123 (2020), 70–81. https://doi.org/10.1016/j.neunet.2019.11.008 doi: 10.1016/j.neunet.2019.11.008
![]() |
[45] |
Q. Ma, S. Y. Xu, Y. Zou, G. D. Shi, Synchronization of stochastic chaotic neural networks with reaction-diffusion terms, Nonlinear Dyn., 67 (2012), 2183–2196. https://doi.org/10.1007/s11071-011-0138-8 doi: 10.1007/s11071-011-0138-8
![]() |
[46] |
L. Shanmugam, P. Mani, R. Rajan, Y. H. Joo, Adaptive synchronization of reaction–diffusion neural networks and its application to secure communication, IEEE Trans. Cybernetics, 50 (2020), 911–922. https://doi.org/10.1109/TCYB.2018.2877410 doi: 10.1109/TCYB.2018.2877410
![]() |
[47] |
C. Hu, H. J. Jiang, Z. D. Teng, Impulsive control and synchronization for delayed neural networks with reaction–diffusion terms, IEEE Trans. Neural Network., 21 (2010), 67–81. https://doi.org/10.1109/TNN.2009.2034318 doi: 10.1109/TNN.2009.2034318
![]() |
[48] |
Z. Y. Wang, J. D. Cao, Z. W. Cai, X. G. Tan, R. S. Chen, Finite-time synchronization of reaction-diffusion neural networks with time-varying parameters and discontinuous activations, Neurocomputing, 447 (2021), 272–281. https://doi.org/10.1016/j.neucom.2021.02.065 doi: 10.1016/j.neucom.2021.02.065
![]() |
[49] |
Z. Y. Wang, J. D. Cao, Z. W. Cai, L. Rutkowski, Anti-synchronization in fixed time for discontinuous reaction–diffusion neural networks with time-varying coefficients and time delay, IEEE Trans. Cybernetics, 50 (2020), 2758–2769. https://doi.org/10.1109/TCYB.2019.2913200 doi: 10.1109/TCYB.2019.2913200
![]() |
[50] | G. H. Hardy, J. E. Littlewood, G. Pólya, Inequalities, Cambridge university press, 1952. |
1. | Hassan Eltayeb Gadain, Said Mesloub, Application of Triple- and Quadruple-Generalized Laplace Transform to (2+1)- and (3+1)-Dimensional Time-Fractional Navier–Stokes Equation, 2024, 13, 2075-1680, 780, 10.3390/axioms13110780 | |
2. | Manoj Singh, Fractional view analysis of coupled Whitham- Broer-Kaup and Jaulent-Miodek equations, 2024, 15, 20904479, 102830, 10.1016/j.asej.2024.102830 | |
3. | Manoj Singh, Mohammad Tamsir, Yasser Salah El Saman, Sarita Pundhir, Approximation of Two-Dimensional Time-Fractional Navier-Stokes Equations involving Atangana-Baleanu Derivative, 2024, 9, 2455-7749, 646, 10.33889/IJMEMS.2024.9.3.033 | |
4. | Elkhateeb Sobhy Aly, Manoj Singh, Mohammed Ali Aiyashi, Mohammed Daher Albalwi, Modeling monkeypox virus transmission: Stability analysis and comparison of analytical techniques, 2024, 22, 2391-5471, 10.1515/phys-2024-0056 | |
5. | Manoj Singh, Mukesh Pal Singh, Mohammad Tamsir, Mohammad Asif, Analysis of Fractional-Order Nonlinear Dynamical Systems by Using Different Techniques, 2025, 11, 2349-5103, 10.1007/s40819-025-01865-2 |